Artificial Intelligence: Providing Security in Digital Banking
In today's fast-paced digital world, the way we handle our finances has transformed dramatically. With the rise of online banking, the need for robust security measures has never been more critical. Enter artificial intelligence (AI), a game-changer in the realm of digital banking security. Imagine having a vigilant guardian that never sleeps, constantly monitoring your financial transactions and ensuring your sensitive information remains safe from prying eyes. This article delves into how AI enhances security in digital banking, examining its applications, benefits, challenges, and future prospects in protecting sensitive financial information.
Fraud detection is one of the most significant challenges in digital banking. Traditional methods often fall short, leaving banks vulnerable to sophisticated fraudsters. However, AI algorithms have revolutionized this aspect by analyzing transaction patterns to identify unusual activities. Think of AI as a detective with a magnifying glass, scrutinizing every detail of your transactions. By leveraging machine learning, these algorithms can significantly improve the detection of fraudulent transactions in real-time. This means that the moment a suspicious transaction occurs, banks can act swiftly, minimizing potential losses and enhancing the security of digital banking systems.
Machine learning is at the forefront of risk assessment in digital banking. By assessing customer behaviors and transaction histories, machine learning models can predict potential risks before they escalate. Picture this: a bank that knows you so well that it can anticipate your next move. With this knowledge, banks can implement proactive measures to mitigate fraud and enhance security protocols. This not only protects the bank's assets but also fosters trust between the bank and its customers, creating a safer banking environment.
In a world where passwords can be stolen or hacked, behavioral biometrics offers a cutting-edge solution. This technology uses AI to analyze unique user behavior patterns, such as typing speed and mouse movements, to create distinct user profiles. Imagine a fingerprint that’s not just physical but behavioral! By adding this additional layer of security, banks can effectively guard against unauthorized access, ensuring that only the rightful owner of an account can access it.
Adaptive authentication takes security a step further by adjusting security measures based on user behavior and context. It’s like having a security guard who knows you well enough to let you in without a hassle but gets suspicious if someone else tries to enter. This system ensures a seamless yet secure experience for customers while minimizing the risk of identity theft. With adaptive authentication, banks can balance security and convenience, leading to higher customer satisfaction.
One of the most remarkable features of AI systems is their ability to learn continuously from new data. This means that as fraud tactics evolve, so do the defenses against them. It’s akin to a chess player who learns from each game, improving their strategies over time. By improving their ability to detect anomalies, continuous learning systems are essential for maintaining the security of digital banking environments, ensuring that banks stay one step ahead of fraudsters.
AI-powered chatbots are another innovative solution in digital banking. These intelligent assistants provide immediate help to customers while simultaneously monitoring interactions for any suspicious activities. Imagine having a helpful assistant who not only answers your questions but also keeps an eye out for potential fraud. This dual function ensures both customer satisfaction and enhanced security measures, creating a safer digital banking experience.
Despite the numerous advantages, implementing AI in digital banking security is not without its challenges. One major concern is data privacy. Banks must comply with stringent data privacy regulations while leveraging AI technologies for security. Navigating these complex legal frameworks is crucial to ensuring customer data is protected. Furthermore, ensuring algorithmic transparency is essential for building trust among users. Banks must demonstrate how AI systems make decisions regarding security without compromising sensitive information. This transparency is vital for fostering customer confidence in these advanced technologies.
Compliance with data privacy regulations is crucial for banks utilizing AI. They must navigate complex legal frameworks to ensure customer data is protected. This not only safeguards the bank’s reputation but also builds trust with customers, who are increasingly concerned about how their data is used.
Ensuring transparency in AI algorithms is essential for building trust among users. Banks must demonstrate how AI systems make decisions regarding security without compromising sensitive information. This transparency helps customers feel secure in knowing that their data is handled responsibly.
The future of AI in digital banking security looks promising. With advancements in technology expected to lead to more sophisticated security measures, we can anticipate a landscape where customer trust is further enhanced, and financial transactions are better protected. As AI continues to evolve, it will play an increasingly vital role in safeguarding our digital finances, making banking not only more secure but also more convenient.
- What is the role of AI in digital banking security? AI enhances security by detecting fraud in real-time, assessing risks, and creating unique user profiles through behavioral biometrics.
- How does machine learning contribute to fraud prevention? Machine learning analyzes customer behavior to predict potential risks, allowing banks to take proactive measures against fraud.
- What are the challenges of implementing AI in banking? Data privacy concerns and the need for algorithmic transparency are significant challenges that banks face when implementing AI technologies.
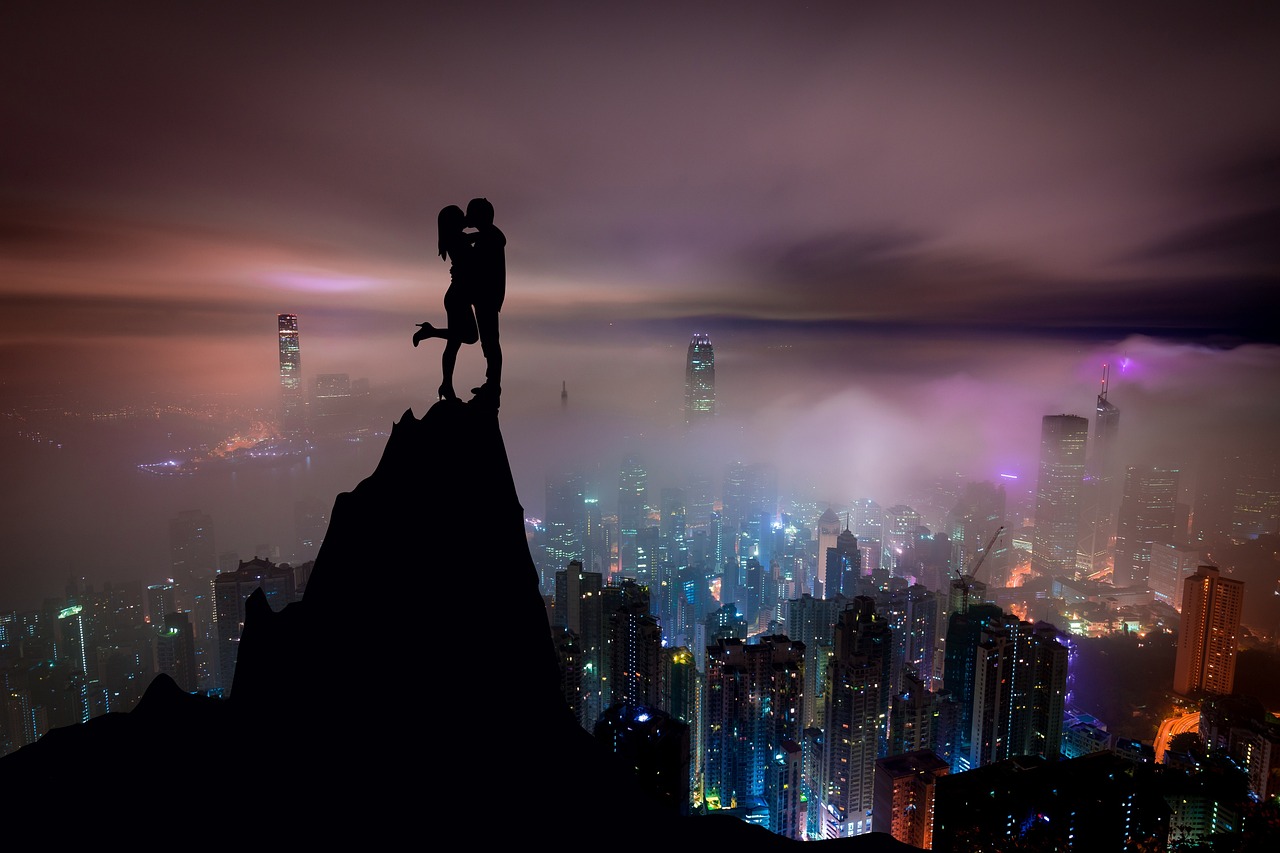
The Role of AI in Fraud Detection
In today's fast-paced digital banking landscape, where transactions occur at lightning speed, the need for robust fraud detection mechanisms has never been more critical. Artificial Intelligence (AI) plays a pivotal role in addressing this challenge, utilizing advanced algorithms to analyze transaction patterns and identify anomalies that may indicate fraudulent activity. Imagine a highly vigilant security guard who never sleeps; that's essentially what AI does for digital banking. By continuously monitoring and learning from vast amounts of data, AI systems can detect unusual behaviors in real-time, significantly enhancing the security of banking systems.
AI algorithms excel at examining transaction data, looking for patterns that deviate from the norm. For instance, if a customer who typically makes small purchases suddenly attempts to transfer a large sum of money, AI can flag this inconsistency as suspicious. This proactive approach allows banks to intervene before fraud occurs, potentially saving millions of dollars and protecting customers' sensitive information. The ability to analyze data quickly and accurately sets AI apart from traditional fraud detection methods, which often rely on outdated rules and manual reviews.
Moreover, AI's machine learning capabilities enable it to adapt and improve over time. As it processes more transactions, the system becomes better at distinguishing between legitimate and fraudulent activities. This not only increases the accuracy of fraud detection but also minimizes false positives, which can frustrate customers and lead to a poor banking experience. In fact, studies have shown that AI can reduce false positives by up to 80%, allowing banks to focus their resources on genuine threats.
To illustrate the effectiveness of AI in fraud detection, consider the following table that compares traditional methods with AI-driven approaches:
Aspect | Traditional Methods | AI-Driven Approaches |
---|---|---|
Speed of Detection | Slower, often after the fact | Real-time analysis |
Accuracy | High false positive rates | Lower false positive rates |
Adaptability | Static rules | Dynamic learning from data |
Resource Allocation | Manual reviews required | Automated monitoring |
In addition to transaction monitoring, AI also aids in customer authentication. By analyzing user behavior, such as typing speed and mouse movements, AI can create unique profiles for each user. This means that if someone else tries to log in using the same credentials, the system can detect the difference in behavior and flag it as suspicious. Such multi-layered security measures are essential in a world where cyber threats are constantly evolving.
In summary, the role of AI in fraud detection cannot be overstated. Its ability to analyze transaction patterns, adapt to new threats, and enhance customer authentication makes it an invaluable tool for digital banking security. As technology continues to advance, we can expect AI to play an even more significant role in safeguarding our financial transactions and personal data.
- How does AI detect fraudulent transactions? AI analyzes transaction patterns and identifies anomalies in real-time, allowing for immediate intervention.
- What are the benefits of using AI in fraud detection? AI improves accuracy, reduces false positives, and allows for real-time monitoring, enhancing overall security.
- Can AI adapt to new fraud tactics? Yes, AI systems continuously learn from new data, making them capable of adapting to evolving fraud tactics.
- What role does customer behavior play in AI fraud detection? AI analyzes user behavior patterns to create unique profiles, adding an extra layer of security against unauthorized access.
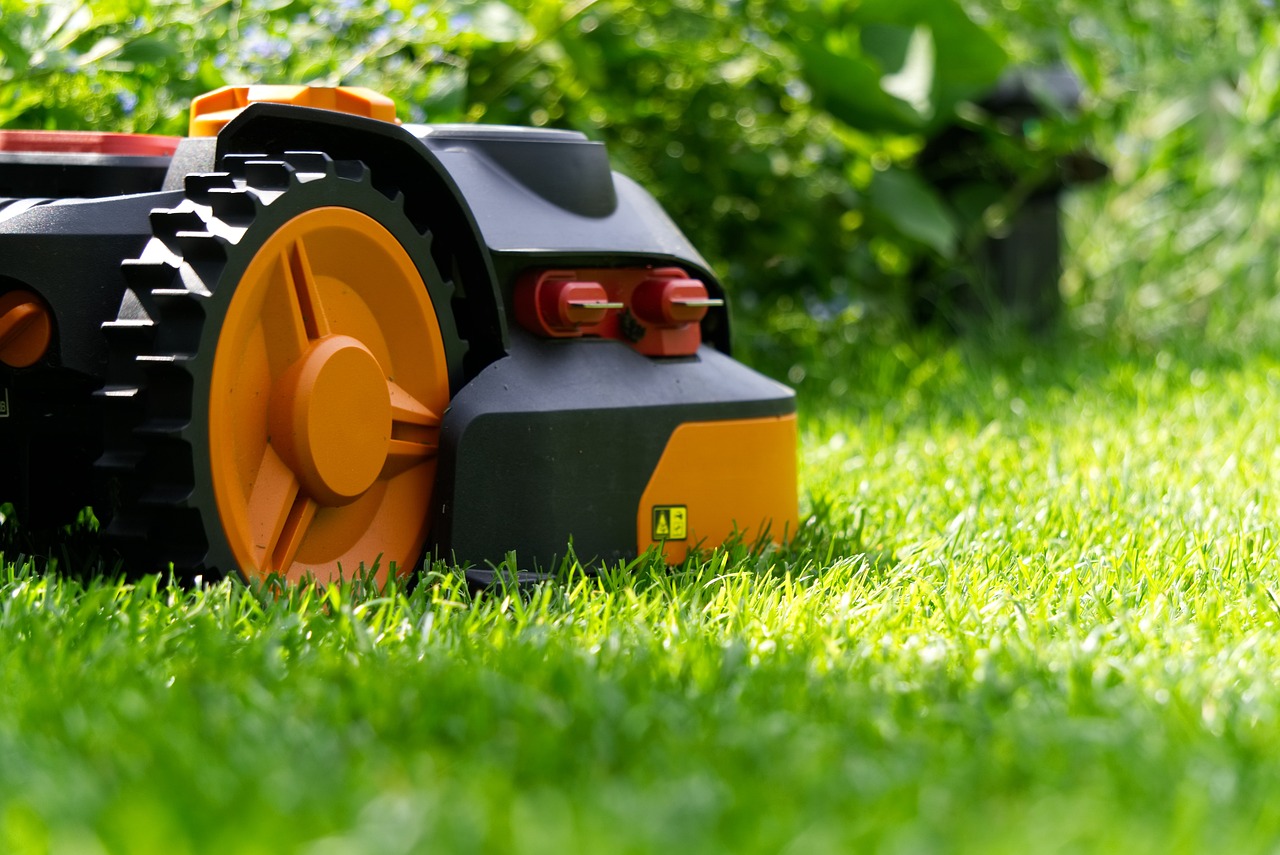
Machine Learning and Risk Assessment
In today's fast-paced digital banking landscape, the integration of machine learning has become a game-changer, particularly in the realm of risk assessment. Imagine having a vigilant guardian that never sleeps, constantly analyzing mountains of data to predict potential threats. That’s precisely what machine learning does. By examining customer behaviors and transaction histories, these sophisticated algorithms can identify patterns that may indicate fraudulent activities. This proactive approach not only helps in pinpointing risks but also empowers banks to take action before any damage occurs.
One of the most fascinating aspects of machine learning is its ability to evolve. As it processes more data, it becomes increasingly adept at recognizing what constitutes normal behavior for each customer. For instance, if a customer typically makes purchases in their local area but suddenly initiates a transaction from a different country, the machine learning model can flag this as a potential risk. This level of insight is invaluable, as it allows banks to implement tailored security measures that are specific to individual customer profiles.
Furthermore, the application of machine learning in risk assessment is not just about identifying fraud; it also plays a crucial role in enhancing overall security protocols. By continuously refining its algorithms, machine learning can adapt to new fraud tactics that emerge over time. This adaptability is akin to a chess player who learns from each game, improving their strategy with every move. As a result, banks can stay one step ahead of fraudsters, safeguarding sensitive financial information.
However, the effectiveness of machine learning in risk assessment hinges on the quality of the data it processes. To illustrate this point, consider the following table that highlights the key components necessary for effective machine learning in digital banking:
Component | Description |
---|---|
Data Quality | High-quality, accurate data is essential for training machine learning models. |
Algorithm Selection | Choosing the right machine learning algorithms is crucial for effective risk assessment. |
Continuous Monitoring | Regularly updating the algorithms with new data ensures they remain effective against evolving threats. |
Interdisciplinary Collaboration | Collaboration between data scientists, cybersecurity experts, and banking professionals enhances the overall strategy. |
In conclusion, machine learning is revolutionizing risk assessment in digital banking by providing insights that are not only timely but also actionable. With its ability to learn and adapt, it serves as a robust defense against fraud, ensuring that banks can protect their customers effectively. As we continue to embrace this technology, the future of secure digital banking looks brighter than ever.
- What is machine learning in digital banking? Machine learning in digital banking refers to the use of algorithms that analyze data to identify patterns and predict potential risks, enhancing security measures against fraud.
- How does machine learning improve risk assessment? By continuously learning from transaction data, machine learning models can detect anomalies and adapt to new fraud tactics, allowing banks to act swiftly to mitigate risks.
- What are the challenges of implementing machine learning in banking? Challenges include ensuring data quality, selecting appropriate algorithms, and maintaining compliance with data privacy regulations.
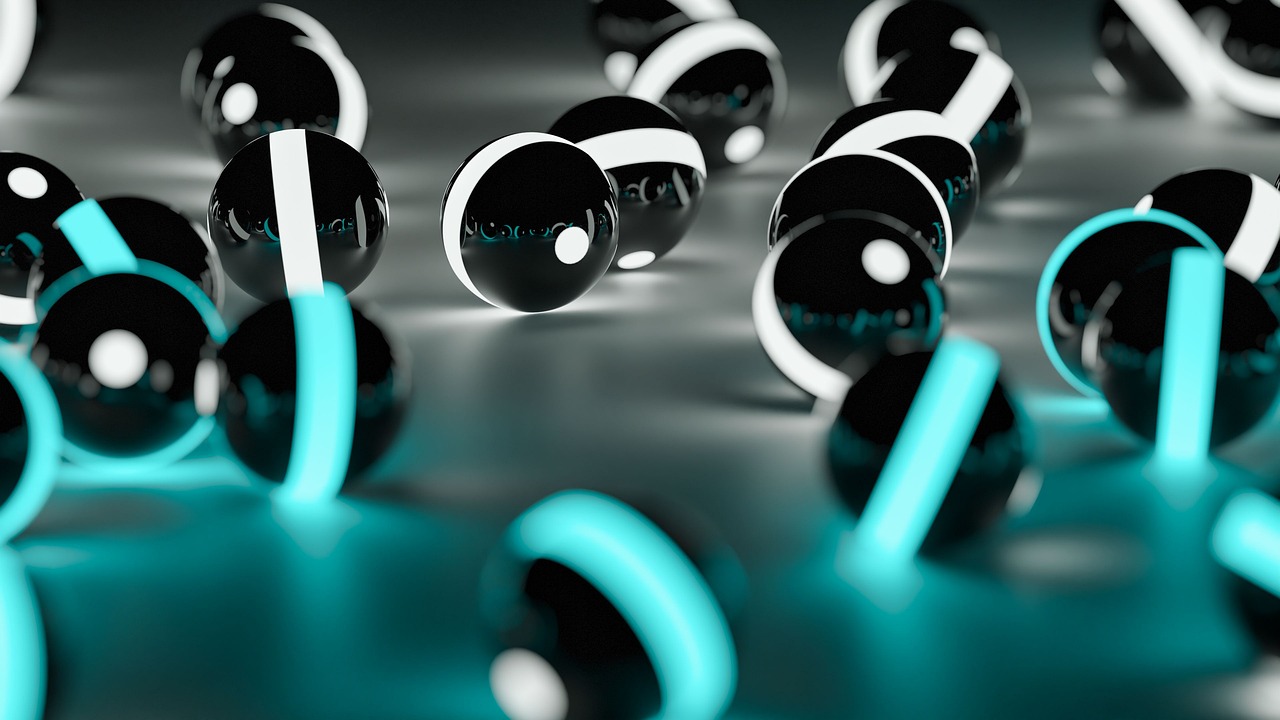
Behavioral Biometrics
In today's digital landscape, where cyber threats lurk around every corner, has emerged as a groundbreaking solution to enhance security in digital banking. Unlike traditional biometrics, which rely on physical attributes like fingerprints or facial recognition, behavioral biometrics analyzes unique patterns in user behavior. This includes a variety of factors such as typing speed, mouse movements, and even the way a user holds their device. Imagine a bank that knows you so well that it can tell if someone else is trying to access your account just by the way they type! Pretty cool, right?
One of the most fascinating aspects of behavioral biometrics is its ability to create a unique user profile. By continuously monitoring how you interact with your banking app or website, these systems learn what is normal for you. For instance, if you typically log in from your home computer at 8 AM, but suddenly there’s an attempt from a different location at midnight, the system can flag this as suspicious. This proactive approach not only enhances security but also minimizes the hassle of false alarms that can occur with traditional security measures.
Behavioral biometrics can be broken down into several key components that contribute to its effectiveness:
- Dynamic Analysis: This involves real-time monitoring of user interactions, allowing the system to adapt and respond instantly to any anomalies.
- Risk Scoring: Each user interaction is scored based on its risk level, helping banks prioritize which activities require further scrutiny.
- Fraud Prevention: By identifying unusual behavior patterns, banks can take immediate action to prevent unauthorized access before any damage is done.
However, like any technology, behavioral biometrics is not without its challenges. Privacy concerns are paramount, as users may feel uneasy about their behavior being constantly monitored. Banks must strike a balance between security and user trust. Transparency in how this data is used and ensuring that it is protected is crucial. Furthermore, as these systems evolve, they must continuously adapt to new fraudulent tactics, making it essential for banks to invest in ongoing research and development.
In conclusion, behavioral biometrics represents a significant leap forward in the quest for enhanced security in digital banking. By understanding and analyzing user behavior, banks can create a robust defense against unauthorized access while providing a seamless experience for legitimate users. As this technology continues to mature, it will undoubtedly play a critical role in shaping the future of secure banking.
- What is behavioral biometrics? Behavioral biometrics is a security measure that analyzes users' unique behavior patterns to verify their identity, such as typing speed and mouse movements.
- How does it enhance security? By creating a unique user profile and monitoring interactions in real-time, it can detect suspicious activities and prevent unauthorized access.
- Are there privacy concerns? Yes, users may have concerns about their behavior being monitored, so banks must ensure transparency and data protection.
- Can it adapt to new threats? Yes, behavioral biometrics systems continuously learn from new data, allowing them to adapt to evolving fraud tactics.
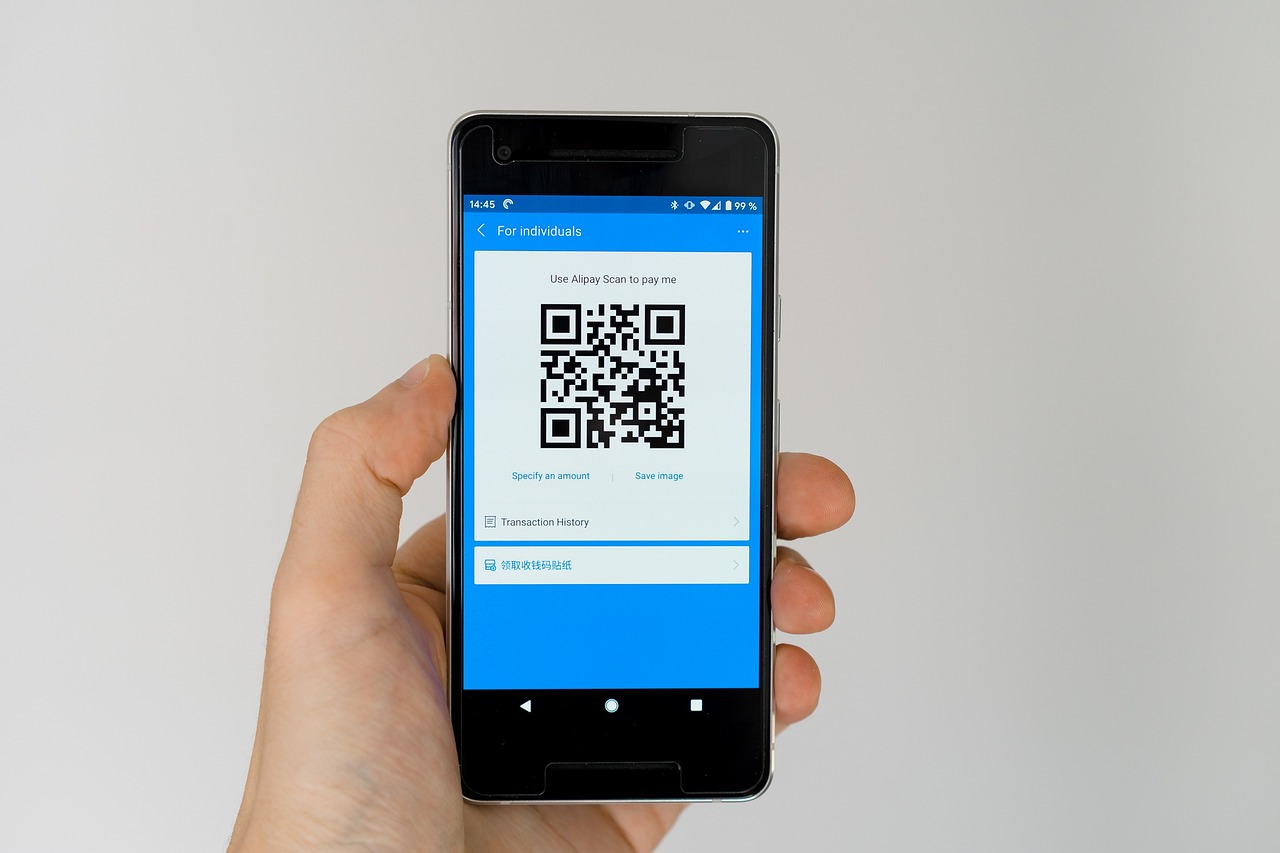
Adaptive Authentication
In the ever-evolving landscape of digital banking, emerges as a game-changer, brilliantly balancing security and user experience. Imagine walking into a bank where the security guards know you by name and can instantly recognize if something seems off. That's the essence of adaptive authentication! It’s not just about asking for a password; it’s about understanding the context of the user’s actions and adjusting security measures accordingly.
Adaptive authentication systems analyze various factors to assess the risk of a transaction or login attempt. For instance, they might consider:
- User Location: Is the user logging in from a familiar location or a new country?
- Device Recognition: Is the login attempt being made from a recognized device or a new one?
- Time of Access: Is the user trying to access their account at an unusual hour?
- Behavioral Patterns: Does the user’s current behavior align with their usual patterns?
By evaluating these factors, adaptive authentication can determine the level of security required. For example, if a user logs in from a new device or location, they might be prompted to answer security questions or verify their identity through a one-time password (OTP) sent to their registered mobile number. This dynamic approach not only enhances security but also minimizes friction for users who are accessing their accounts from familiar devices and locations.
Moreover, adaptive authentication systems are designed to learn over time. As they gather data on user behaviors, they become increasingly adept at distinguishing between legitimate and suspicious activities. This continuous learning process means that the system can adapt to new threats as they emerge, ensuring that security measures are always one step ahead of potential fraudsters.
In a world where identity theft and fraud are rampant, adaptive authentication offers a seamless yet fortified barrier against unauthorized access. It’s like having a personal security detail that knows when to kick into high gear and when to let you breeze through the door. As digital banking continues to grow, the importance of adaptive authentication will only increase, providing customers with peace of mind while navigating their financial transactions.
- What is adaptive authentication?
Adaptive authentication is a security measure that adjusts the level of authentication required based on the context of a user's behavior and transaction. - How does adaptive authentication enhance security?
By analyzing factors like user location, device, and behavior patterns, it can identify potential risks and prompt additional security measures when necessary. - Is adaptive authentication user-friendly?
Yes, it aims to provide a seamless experience by requiring additional verification only when suspicious activity is detected.
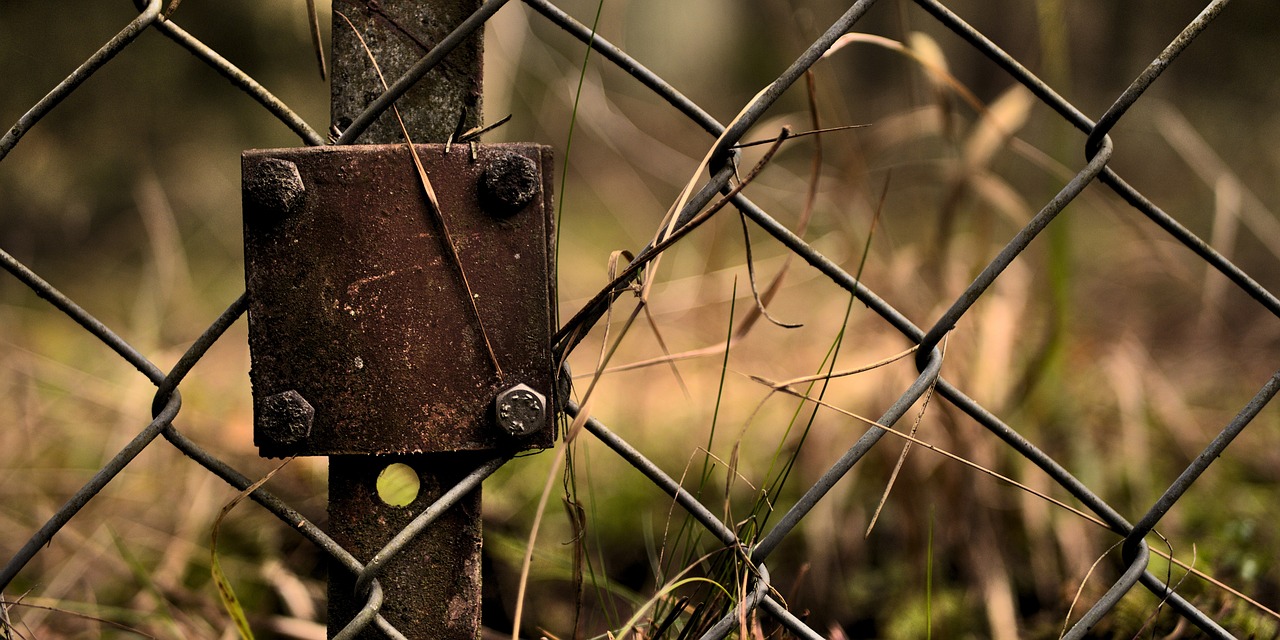
Continuous Learning Systems
In the ever-evolving landscape of digital banking, powered by artificial intelligence (AI) play a pivotal role in enhancing security protocols. Imagine a security system that not only learns from past experiences but also adapts to new threats as they emerge. This is precisely what continuous learning systems do; they are like a vigilant guard that never sleeps, constantly updating its knowledge base to stay ahead of potential fraudsters. By analyzing vast amounts of data, these systems can identify patterns and anomalies that may indicate fraudulent activity, making them indispensable in the fight against financial crime.
One of the most remarkable aspects of continuous learning systems is their ability to process new information in real-time. As they gather data from various transactions and user interactions, they refine their algorithms, thus improving their accuracy and efficiency. This dynamic approach allows banks to respond swiftly to emerging threats, providing a robust layer of security that traditional methods simply cannot match. For instance, if a new type of fraud is detected, these systems can quickly adjust their parameters to flag similar activities in the future, effectively closing the door on opportunistic criminals.
Furthermore, continuous learning systems can integrate with other security measures, creating a comprehensive defense strategy. They work synergistically with machine learning models and behavioral biometrics to provide a multi-faceted approach to security. By combining these technologies, banks can not only detect fraud more effectively but also enhance the overall customer experience. For example, when a transaction is flagged as suspicious, the system can automatically initiate additional verification steps, all while keeping the customer informed and engaged.
However, implementing continuous learning systems is not without challenges. Banks must ensure that these systems are trained on high-quality data to avoid biases that could lead to false positives or negatives. This is crucial, as a single misstep could alienate customers or allow fraudulent activities to slip through the cracks. Therefore, ongoing monitoring and adjustment of these systems are essential to maintain their efficacy and reliability.
In conclusion, continuous learning systems represent a significant advancement in the realm of digital banking security. By harnessing the power of AI to learn and adapt in real-time, these systems not only enhance fraud detection capabilities but also foster a more secure and trustworthy banking environment. As technology continues to evolve, the integration of continuous learning systems will undoubtedly play a crucial role in safeguarding sensitive financial information and ensuring customer confidence in digital banking.
- What are continuous learning systems? Continuous learning systems are AI-driven technologies that adapt and improve their algorithms based on new data and experiences, enhancing their ability to detect and respond to threats in real-time.
- How do continuous learning systems enhance digital banking security? They analyze transaction patterns and user behaviors continuously, allowing banks to identify potential fraud more effectively and implement proactive security measures.
- What challenges do banks face when implementing these systems? Banks must ensure data quality, avoid algorithmic biases, and maintain transparency to build trust among users while leveraging continuous learning systems for security.
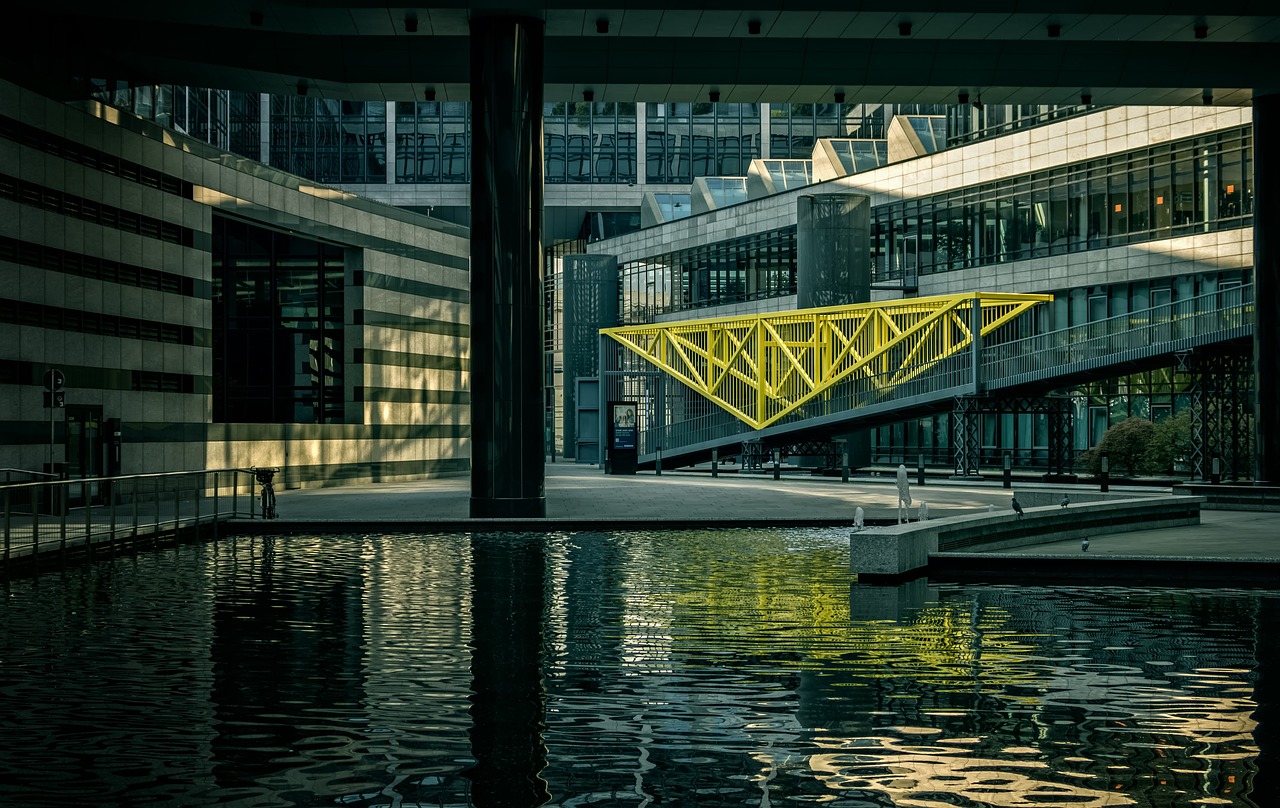
Automated Customer Support and Security
In the fast-paced world of digital banking, where every second counts, automated customer support powered by artificial intelligence (AI) is revolutionizing how financial institutions interact with their clients. Imagine having a virtual assistant available 24/7, ready to answer your questions and provide assistance at any moment. This is not just a dream; it's a reality that enhances both customer experience and security. AI-driven chatbots are designed to handle a multitude of inquiries, from simple account balance checks to more complex transaction queries, all while ensuring that sensitive information remains protected.
One of the standout features of AI in customer support is its ability to monitor interactions for suspicious activities. As these chatbots engage with customers, they are continuously analyzing conversations for red flags that may indicate fraudulent behavior. For instance, if a user suddenly requests to change their account details or make a large withdrawal, the AI can flag this as unusual and prompt further verification. This not only helps in identifying potential threats but also adds an extra layer of security that traditional support systems may lack.
Moreover, the integration of AI in customer support systems allows banks to provide a more personalized experience. By utilizing machine learning algorithms, these systems learn from past interactions, tailoring responses to individual customer preferences and behaviors. For example, if a customer frequently inquires about credit card rewards, the AI can proactively provide updates on new offers or changes to existing programs. This level of personalization not only enhances customer satisfaction but also fosters a sense of trust and loyalty towards the bank.
However, while AI-powered support systems offer many advantages, they are not without challenges. Banks must ensure that their chatbots are equipped to handle complex queries and can seamlessly escalate issues to human representatives when necessary. This hybrid approach helps maintain a balance between efficiency and the human touch, which is crucial in the banking sector. It’s like having a well-trained assistant who knows when to step back and let the expert take over.
As we look to the future, the role of automated customer support in digital banking security will only grow. With advancements in natural language processing and sentiment analysis, AI systems will become even more adept at understanding and responding to customer needs. This evolution will not only enhance the overall banking experience but will also play a critical role in safeguarding sensitive financial information. In a world where cyber threats are ever-evolving, having a robust AI system in place is not just an option; it’s a necessity.
- How do AI chatbots enhance security in digital banking?
AI chatbots monitor customer interactions for suspicious activities and can flag unusual behavior, helping to prevent fraud. - Can AI replace human customer service representatives?
While AI can handle many inquiries, it is essential to have human representatives available for complex issues that require personal attention. - What technologies are used in AI customer support?
Technologies such as machine learning, natural language processing, and sentiment analysis are commonly used to improve AI customer support systems. - Is my data safe when interacting with AI chatbots?
Yes, reputable banks implement strict data protection measures to ensure that customer information remains confidential and secure during AI interactions.
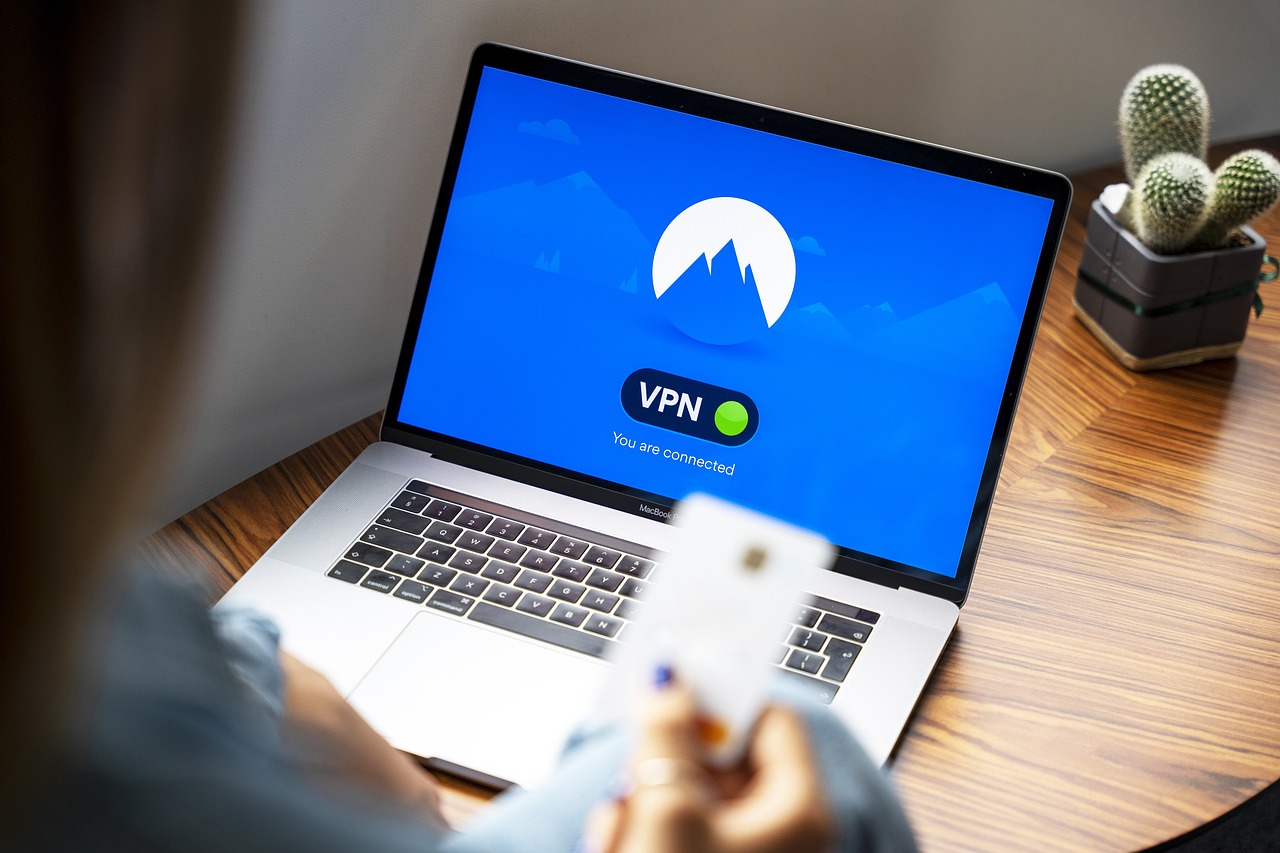
Challenges in Implementing AI Security
While the integration of artificial intelligence (AI) in digital banking security brings a plethora of benefits, it is not without its challenges. Banks and financial institutions must navigate a complex landscape filled with hurdles that can hinder the effective deployment of AI technologies. One major concern is data privacy. With the increasing reliance on AI systems, banks must ensure they comply with stringent data privacy regulations. These regulations often vary by region, making it essential for institutions to stay informed and agile. A single misstep can lead to hefty fines and a loss of customer trust, which is vital in the banking sector.
Furthermore, the need for robust algorithms cannot be overstated. AI systems rely heavily on data to function effectively. If the algorithms are not designed properly, they may produce inaccurate results, leading to either false positives or negatives in fraud detection. This not only undermines the security measures in place but can also frustrate customers who may find themselves wrongfully flagged. Additionally, the potential for bias in AI decision-making processes is a pressing issue. If the data used to train these algorithms is biased, it can result in unfair treatment of certain customer groups, raising ethical concerns and potentially violating anti-discrimination laws.
To illustrate these challenges, consider the following table that outlines key issues faced by banks when implementing AI security:
Challenge | Description |
---|---|
Data Privacy | Compliance with varying regulations to protect sensitive customer information. |
Algorithm Robustness | The necessity for well-designed algorithms to avoid inaccuracies in fraud detection. |
Bias in AI | The risk of biased data leading to unfair treatment of users. |
Moreover, achieving algorithmic transparency is crucial for building trust among users. Banks must demonstrate how AI systems make decisions regarding security while ensuring that sensitive information remains protected. Customers are increasingly concerned about how their data is used, and without transparency, skepticism can arise. This is where effective communication comes into play. Banks need to educate their customers about how AI enhances their security and the measures taken to safeguard their data.
Lastly, continuous monitoring and updating of AI systems are essential to keep pace with evolving threats. Cybercriminals are constantly refining their tactics, and if AI systems are not regularly updated, they may become less effective over time. This creates a cycle of needing to invest in new technologies and training staff to handle these systems, which can be resource-intensive.
In summary, while AI presents exciting opportunities for enhancing security in digital banking, the challenges it poses cannot be overlooked. From data privacy to algorithmic transparency, banks must navigate these hurdles carefully to ensure that they not only protect their customers but also build lasting trust in their digital services.
- What are the main challenges of implementing AI in banking security? The main challenges include data privacy concerns, the need for robust algorithms, potential bias in AI decision-making, and the necessity for algorithmic transparency.
- How can banks ensure compliance with data privacy regulations? Banks can ensure compliance by staying informed about regulations, conducting regular audits, and implementing strict data handling protocols.
- Why is algorithmic transparency important? Algorithmic transparency is important for building trust among customers, as it allows them to understand how their data is being used and how security decisions are made.
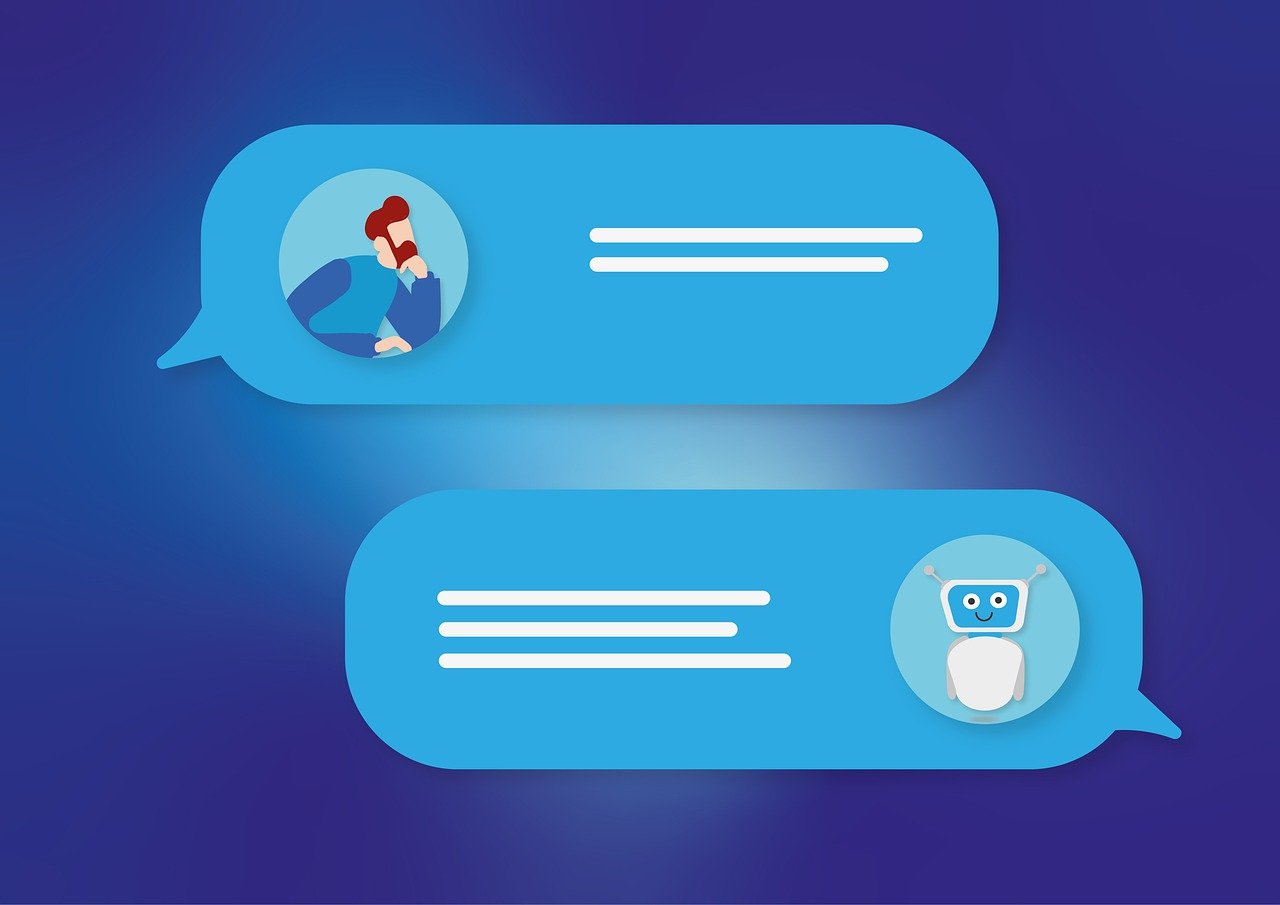
Data Privacy Regulations
In today’s digital landscape, are more critical than ever, especially for banks leveraging artificial intelligence (AI) to enhance security. As financial institutions increasingly rely on AI technologies, they must navigate a complex web of legal frameworks designed to protect customer data. These regulations, such as the General Data Protection Regulation (GDPR) in Europe and the California Consumer Privacy Act (CCPA) in the United States, set stringent guidelines on how personal data can be collected, processed, and stored.
Compliance with these regulations is not just a legal obligation; it’s a trust-building exercise between banks and their customers. When customers know that their data is being handled with care, they are more likely to engage with digital banking services. However, the challenge lies in balancing the use of AI for security purposes while adhering to these regulations. For example, banks must ensure that their AI systems do not inadvertently expose sensitive customer information during fraud detection processes.
Moreover, the implementation of AI can sometimes lead to conflicts with data privacy regulations. For instance, the need for transparency in AI decision-making processes can clash with the requirement to protect sensitive information. Banks must clearly communicate how their AI systems function, which can be a daunting task, especially when algorithms are complex and proprietary. This is where algorithmic transparency becomes essential. Financial institutions need to explain how AI makes decisions regarding security without compromising the privacy of their customers.
To effectively manage these challenges, banks are adopting various strategies. They are investing in robust data governance frameworks that outline how data is collected, processed, and shared. This includes:
- Conducting regular audits of AI systems to ensure compliance with data privacy regulations.
- Implementing data anonymization techniques to protect customer identities while still benefiting from AI insights.
- Training staff on the importance of data privacy and the implications of non-compliance.
As the regulatory landscape continues to evolve, banks must stay ahead of the curve by continuously updating their policies and practices. This proactive approach not only minimizes the risk of legal repercussions but also enhances customer confidence in digital banking services.
- What are data privacy regulations?
Data privacy regulations are laws that govern how personal information is collected, used, and protected by organizations, including banks.
- Why are data privacy regulations important for banks using AI?
These regulations are crucial to protect customer data from misuse and to build trust between banks and their customers.
- How can banks ensure compliance with data privacy regulations?
Banks can ensure compliance by implementing robust data governance frameworks, conducting audits, and training staff on data privacy issues.
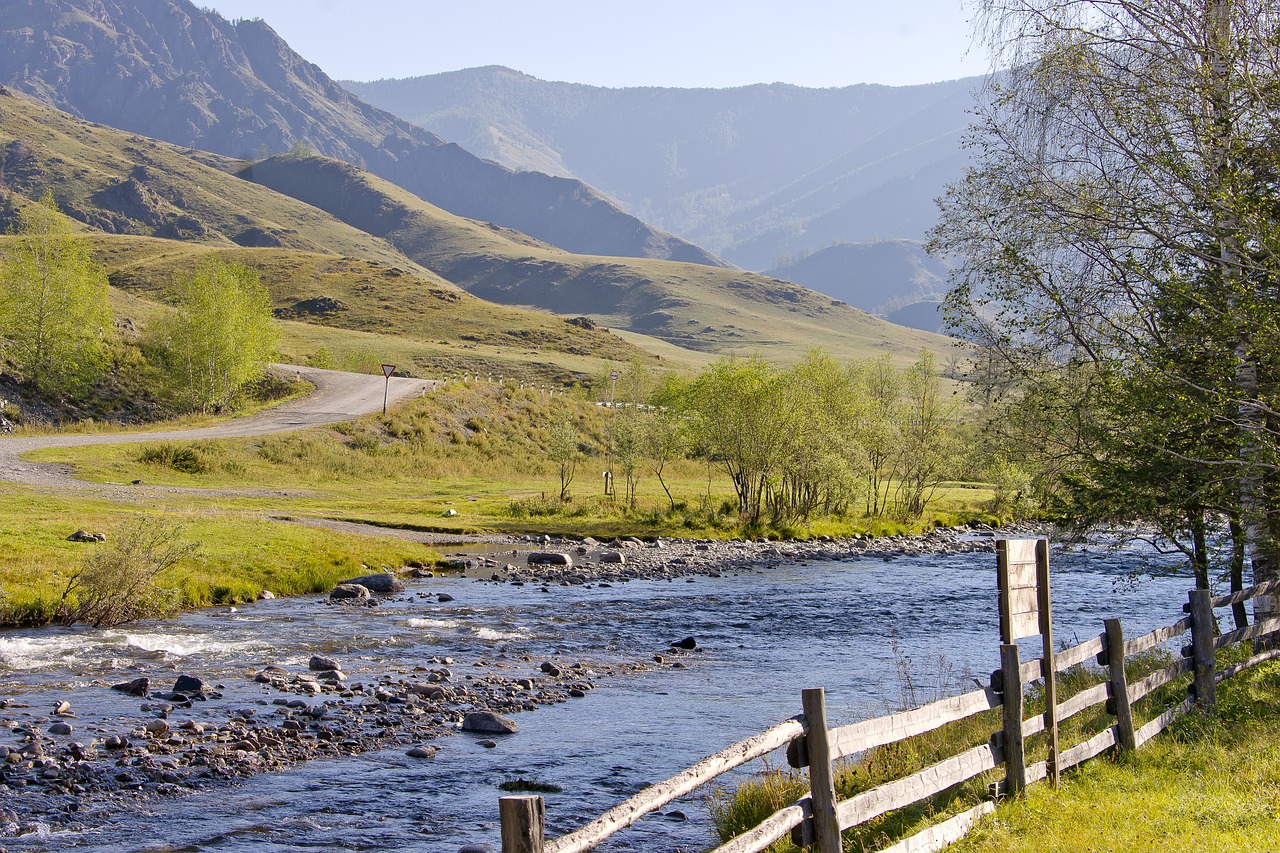
Algorithmic Transparency
is a critical aspect of integrating artificial intelligence into digital banking security. It refers to the clarity and openness about how AI algorithms operate, make decisions, and process data. In a world where financial transactions are becoming increasingly complex and digital, customers deserve to know how their data is being used and how decisions that affect their security are made. Think of it like a recipe: if you don’t know what goes into your meal, how can you trust that it’s safe and nutritious?
One of the main challenges banks face is ensuring that their AI systems are not just effective but also understandable. When users have a clear understanding of how their data is being analyzed, it fosters a sense of trust and confidence in the banking system. For example, if a bank uses an AI model to flag suspicious transactions, customers should be informed about the general criteria that trigger these alerts. This transparency can help alleviate fears of wrongful accusations or misunderstandings regarding their financial activities.
Moreover, algorithmic bias is another concern that must be addressed. If an AI system is trained on biased data, it could lead to unfair treatment of certain customer groups. Banks need to ensure that their algorithms are regularly audited and updated to reflect a diverse and representative dataset. This is not just about ethical responsibility; it’s about maintaining the integrity of the banking system. A bank that can demonstrate fairness in its AI processes will likely earn greater loyalty from its customers.
To achieve algorithmic transparency, banks can adopt several strategies:
- Regular Audits: Conduct periodic reviews of AI systems to ensure they operate fairly and effectively.
- Clear Communication: Provide customers with easy-to-understand information about how AI impacts their banking experience.
- Feedback Mechanisms: Implement systems that allow customers to report concerns or misunderstandings related to AI decisions.
In conclusion, achieving algorithmic transparency in digital banking security is not just a regulatory requirement; it’s a pathway to building stronger relationships with customers. As banks navigate the complexities of AI, they must prioritize transparency to foster trust and ensure that their security measures are both effective and accountable.
Q1: What is algorithmic transparency?
A1: Algorithmic transparency refers to the openness and clarity about how AI algorithms function, make decisions, and process data, particularly in the context of digital banking security.
Q2: Why is algorithmic transparency important in banking?
A2: It is crucial because it helps build trust with customers, ensures fairness in AI decision-making, and protects against potential biases that could affect certain groups of customers.
Q3: How can banks ensure algorithmic transparency?
A3: Banks can ensure transparency by conducting regular audits, communicating clearly with customers about AI processes, and implementing feedback mechanisms for customers to voice concerns.
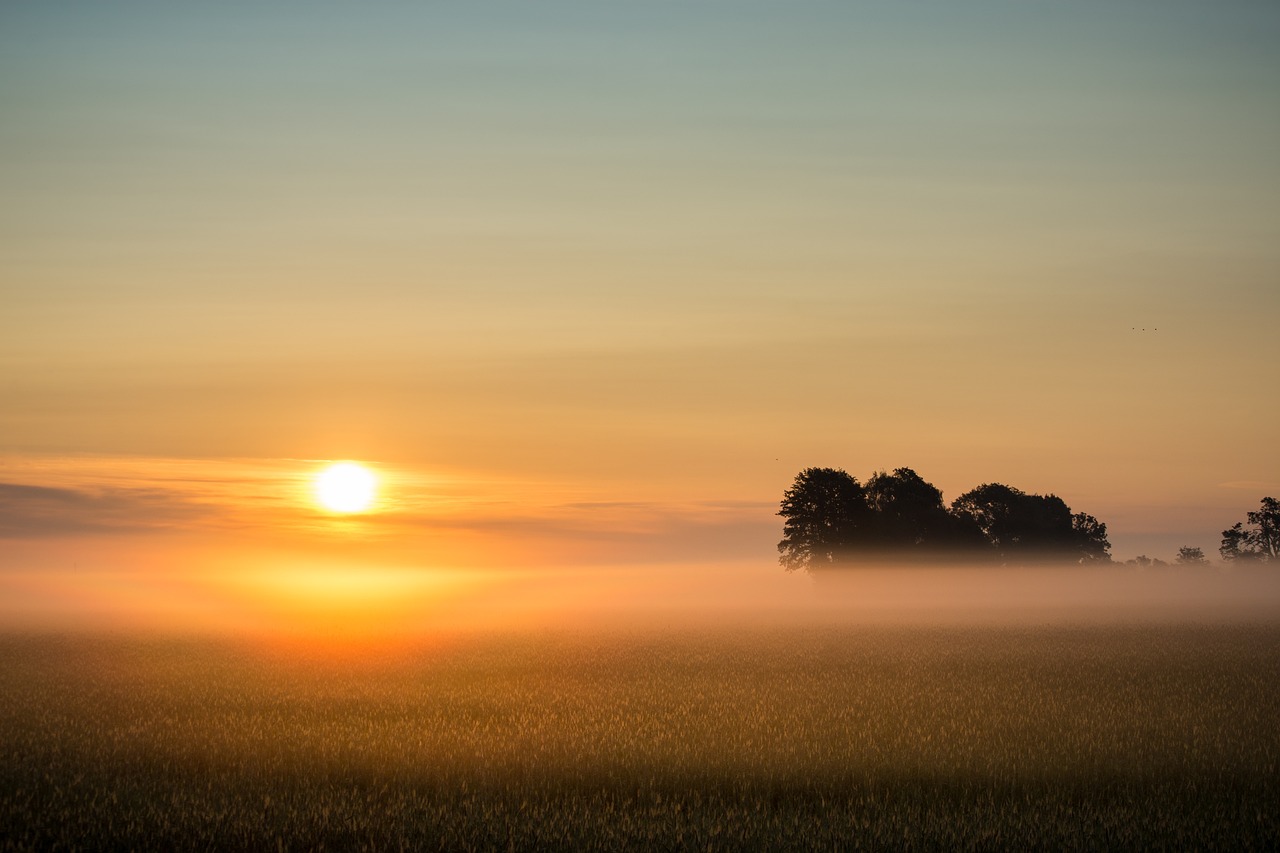
The Future of AI in Digital Banking Security
The future of Artificial Intelligence (AI) in digital banking security is not just bright; it's practically glowing with potential! As technology continues to evolve at lightning speed, the ways in which AI can enhance security measures are becoming more sophisticated and effective. Imagine a world where your bank not only protects your money but also anticipates threats before they even materialize. That’s the future we’re heading toward!
One of the most exciting prospects is the integration of advanced machine learning algorithms that can analyze vast amounts of transaction data in real-time. These algorithms will become increasingly adept at identifying patterns and anomalies, making it even harder for fraudsters to slip through the cracks. In fact, as these systems learn from each transaction, they’ll continuously improve, adapting to new tactics employed by cybercriminals. It’s like having a security guard who not only monitors the premises but also learns from every incident to become more vigilant over time!
Moreover, the implementation of predictive analytics will allow banks to foresee potential security threats based on historical data. By analyzing past fraud cases, AI systems can identify risk factors and predict where future threats might arise. This proactive approach can significantly reduce the chances of fraud, making digital banking a much safer experience for everyone. It’s akin to having a weather forecast that not only tells you when it might rain but also advises you on how to prepare for it!
Another thrilling development is the use of biometric security measures. As AI technology advances, we can expect to see more banks adopting multi-factor authentication processes that go beyond just passwords. Imagine logging into your banking app with your face or fingerprint, combined with behavioral biometrics that analyze how you interact with your device. This multi-layered approach will create an almost impenetrable fortress around your sensitive financial information.
However, with great power comes great responsibility. As AI becomes more integral to banking security, there will be a pressing need for ethical considerations and regulatory compliance. Banks must ensure that their AI systems are transparent and fair, avoiding biases that could lead to wrongful accusations or denials of service. It’s crucial that the algorithms are designed to protect user privacy while delivering top-notch security.
In summary, the future of AI in digital banking security is not just about keeping up with threats but staying ahead of them. As we embrace these technological advancements, we can look forward to a banking experience that is not only secure but also intuitive and user-friendly. The combination of predictive analytics, advanced machine learning, and biometric security could redefine how we view our finances, making us feel safer and more empowered than ever before.
- What role does AI play in digital banking security? AI enhances security by analyzing transaction patterns, detecting fraud, and implementing advanced authentication methods.
- How does machine learning improve banking security? Machine learning algorithms continuously learn from data, allowing banks to predict and mitigate potential risks effectively.
- What are behavioral biometrics? Behavioral biometrics analyze user behavior, such as typing speed and mouse movements, to create unique profiles for enhanced security.
- Will AI replace human security measures in banking? While AI will significantly enhance security measures, human oversight will still be crucial for ethical considerations and complex decision-making.
- What challenges does AI face in digital banking? Challenges include data privacy concerns, the need for transparency in algorithms, and avoiding biases in AI decision-making.
Frequently Asked Questions
- How does AI improve security in digital banking?
AI enhances security in digital banking by analyzing transaction patterns in real-time to detect fraudulent activities. Its algorithms can identify unusual behaviors and alert banks to potential threats before they escalate.
- What is the role of machine learning in risk assessment?
Machine learning models assess customer behaviors and transaction histories to predict potential risks. This proactive approach allows banks to implement security measures that can mitigate fraud before it occurs.
- What are behavioral biometrics?
Behavioral biometrics is a security measure that uses AI to analyze unique user behavior patterns, such as typing speed and mouse movements. This creates a distinct user profile, enhancing security against unauthorized access.
- What is adaptive authentication?
Adaptive authentication adjusts security measures based on user behavior and context. This ensures a smooth experience for customers while maintaining a high level of security, reducing the risk of identity theft.
- How do continuous learning systems work in AI security?
Continuous learning systems in AI security constantly learn from new data, improving their ability to detect anomalies and adapt to new fraud tactics. This makes them crucial for the ongoing security of digital banking environments.
- What challenges do banks face when implementing AI security measures?
Implementing AI in digital banking security presents challenges like data privacy concerns, the need for robust algorithms, and potential biases in decision-making processes. Navigating these issues is essential for effective security.
- How do data privacy regulations impact AI in banking?
Data privacy regulations require banks to ensure customer data is protected while leveraging AI technologies. Compliance with these regulations is crucial to maintain trust and safeguard sensitive information.
- Why is algorithmic transparency important?
Algorithmic transparency is vital for building trust among users. Banks must show how AI systems make security decisions without compromising sensitive information, ensuring customers feel secure in their transactions.
- What does the future hold for AI in digital banking security?
The future of AI in digital banking security is bright, with advancements expected to lead to more sophisticated security measures. These developments will further enhance customer trust and protect financial transactions.