The Power of AI in Climate Predictions and Solutions
As we stand at the crossroads of environmental change and technological advancement, the role of artificial intelligence (AI) in addressing climate change challenges is becoming increasingly vital. Imagine a world where we can predict climate patterns with pinpoint accuracy, allowing us to prepare for natural disasters before they strike. This is not just a fantasy; it’s a reality that AI is helping to create. By harnessing the power of data and advanced algorithms, AI is revolutionizing climate science and offering innovative solutions that can significantly impact our planet's future.
Climate change is one of the most pressing issues of our time, affecting ecosystems, weather patterns, and human livelihoods. Traditional methods of climate modeling have their limitations, often struggling to process the vast amounts of data generated daily. However, AI steps in as a game-changer. By analyzing complex datasets, AI can identify patterns that humans might overlook, leading to more accurate climate predictions. This capability is crucial as it enables scientists and policymakers to make informed decisions that can mitigate the impacts of climate change.
Moreover, AI doesn’t just stop at predictions; it also provides actionable solutions. From optimizing agricultural practices to enhancing energy efficiency, AI applications are diverse and impactful. For instance, consider how AI can analyze weather patterns to suggest the best times for planting crops, ensuring farmers can maximize yields while using fewer resources. This intersection of technology and sustainability is where the true power of AI lies, and it’s an exciting frontier for environmental protection.
As we delve deeper into the transformative impact of AI on climate science, we will explore various AI-driven climate prediction models, the role of machine learning, and how integrating AI with existing climate models can lead to more robust predictions. Additionally, we will examine practical AI solutions that are not just theoretical but are already being implemented in fields like agriculture and energy. The future is not just about surviving climate change; it's about thriving in a world where technology and nature coexist harmoniously.
- How does AI improve climate predictions?
AI enhances climate predictions by analyzing large datasets and identifying patterns that improve the accuracy of forecasts. - What are some examples of AI applications in climate science?
Examples include smart agriculture practices, energy efficiency innovations, and advanced climate modeling techniques. - Can AI help in mitigating climate change?
Yes, AI provides solutions that promote sustainability, such as optimizing resource use in agriculture and enhancing energy efficiency.
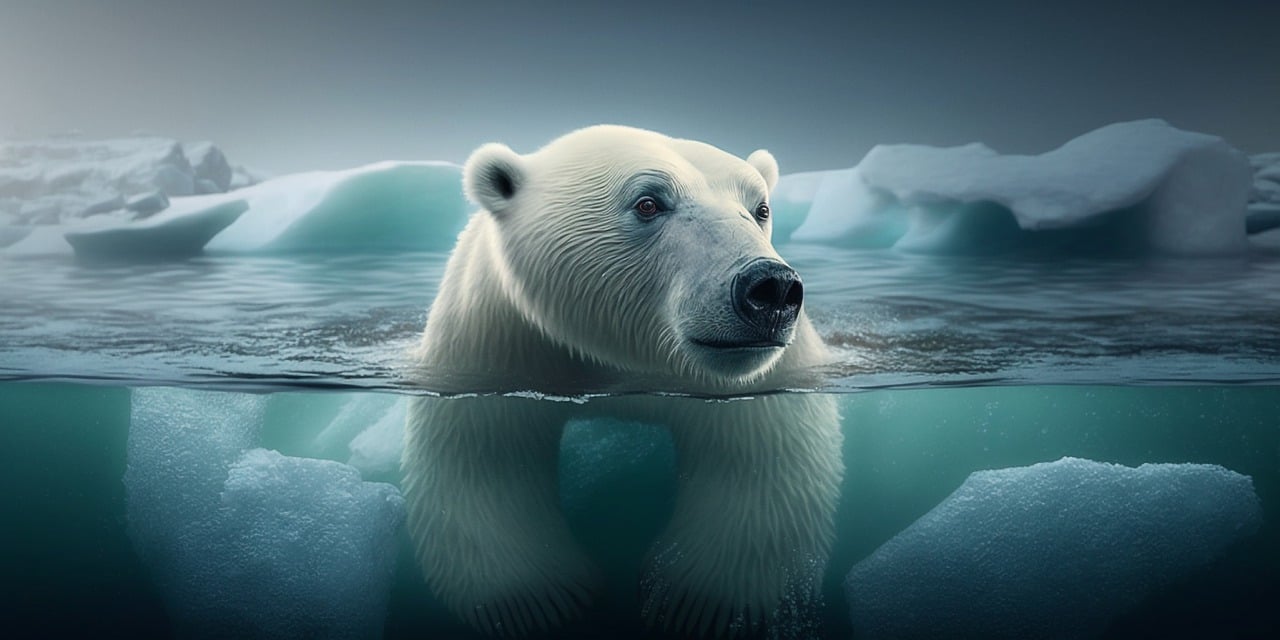
Understanding AI's Role in Climate Science
Artificial intelligence (AI) is not just a buzzword; it’s becoming a game changer in the realm of climate science. Imagine trying to solve a complex puzzle with thousands of pieces scattered everywhere. That’s what climate scientists face every day. With the help of AI, they can sift through vast datasets, identify patterns, and improve the accuracy of their models. It's like having a super-smart assistant who never tires and can analyze information at lightning speed. This ability to process large amounts of data allows scientists to gain insights that were previously unimaginable.
One of the fundamental ways AI contributes to understanding climate dynamics is through data analysis. Traditional methods of climate modeling often rely on limited datasets and simplified assumptions. In contrast, AI can handle multiple variables and complex interactions simultaneously. For example, AI algorithms can analyze temperature, humidity, wind patterns, and even human activities to create a holistic view of our climate system. This comprehensive approach leads to better forecasts and a deeper understanding of how different factors influence climate change.
Moreover, AI enhances climate modeling by enabling real-time data processing. Think about it: as climate-related events unfold, such as wildfires or hurricanes, AI can quickly analyze incoming data, update models, and provide timely predictions. This agility is crucial for disaster preparedness and response. When communities are armed with accurate forecasts, they can take proactive measures to protect lives and property. In essence, AI acts as a powerful ally for climate scientists, enhancing their ability to predict and respond to environmental changes.
To illustrate the impact of AI in climate science, let’s take a look at some specific applications:
Application | Description |
---|---|
Climate Modeling | AI improves the accuracy of climate models by processing complex datasets and identifying patterns. |
Extreme Weather Prediction | AI analyzes real-time data to provide timely forecasts for hurricanes, floods, and other extreme weather events. |
Impact Assessment | AI helps assess the potential impacts of climate change on various ecosystems and human activities. |
In summary, AI is revolutionizing climate science by enhancing our understanding of climate dynamics and improving predictive capabilities. As we continue to grapple with the challenges posed by climate change, the role of AI will only grow more critical. It’s like having a powerful magnifying glass that helps us see the intricate details of our planet’s climate system, allowing us to make informed decisions for a sustainable future.
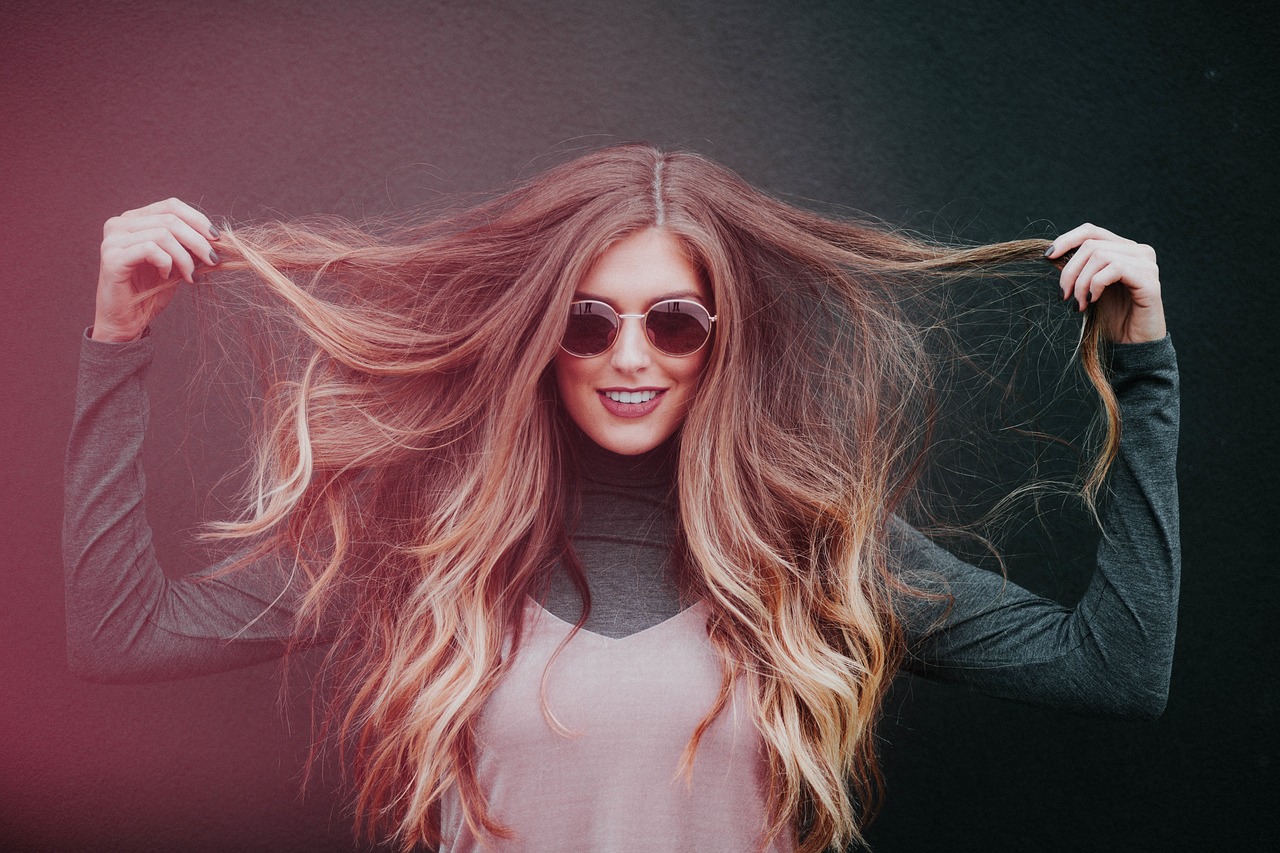
AI-Driven Climate Prediction Models
In recent years, the landscape of climate prediction has undergone a profound transformation, largely fueled by the advancements in artificial intelligence (AI). Traditional climate models, while useful, often struggled with the complexity and sheer volume of data that climate science entails. Enter AI-driven climate prediction models, which are not just an evolution but a revolution in how we approach forecasting our planet's future. These innovative models leverage the power of machine learning, neural networks, and data mining techniques to enhance the reliability and precision of climate forecasts.
Imagine trying to piece together a jigsaw puzzle with thousands of pieces scattered across a table. This is akin to the challenge faced by climate scientists who must sift through immense datasets to find patterns and make predictions. AI acts as a skilled puzzle solver, swiftly identifying connections and relationships that might otherwise remain hidden. By analyzing historical climate data, AI models can discern trends and anomalies, ultimately leading to more accurate predictions of future climate scenarios.
One of the standout features of AI-driven climate prediction models is their ability to process massive datasets at an unprecedented speed. For instance, while traditional models might take weeks or even months to analyze data, AI can accomplish this in a matter of hours or days. This rapid analysis is crucial, especially when we consider the urgency of climate-related decision-making. The quicker we can access reliable forecasts, the better equipped we are to respond to extreme weather events and other climate-related challenges.
Moreover, AI-driven models are not static; they continuously learn and evolve. As new data becomes available, these models refine their predictions, adapting to changing climate patterns. This adaptability is vital in a world where climate change is not a distant threat but a current reality. For example, AI can help predict shifts in weather patterns, such as the increased frequency of hurricanes or droughts, allowing communities to prepare and respond effectively.
To illustrate the impact of AI on climate prediction, consider the following table showcasing the differences between traditional climate models and AI-driven models:
Feature | Traditional Climate Models | AI-Driven Climate Models |
---|---|---|
Data Processing Speed | Weeks/Months | Hours/Days |
Adaptability | Static | Dynamic Learning |
Accuracy | Moderate | High |
Complex Pattern Recognition | Limited | Advanced |
This table highlights just how transformative AI can be in the realm of climate science. By embracing these AI-driven models, scientists and policymakers can gain a clearer, more nuanced understanding of our planet's climate dynamics. This, in turn, enables them to devise effective strategies for mitigation and adaptation, ultimately contributing to a more sustainable future.
As we continue to face the pressing challenges posed by climate change, the integration of AI into climate prediction models stands out as a beacon of hope. It empowers us to not only predict the future but also to take proactive steps to safeguard our planet. In the next section, we will delve deeper into the specific AI techniques that are reshaping climate predictions and how they enhance our preparedness for extreme weather events.
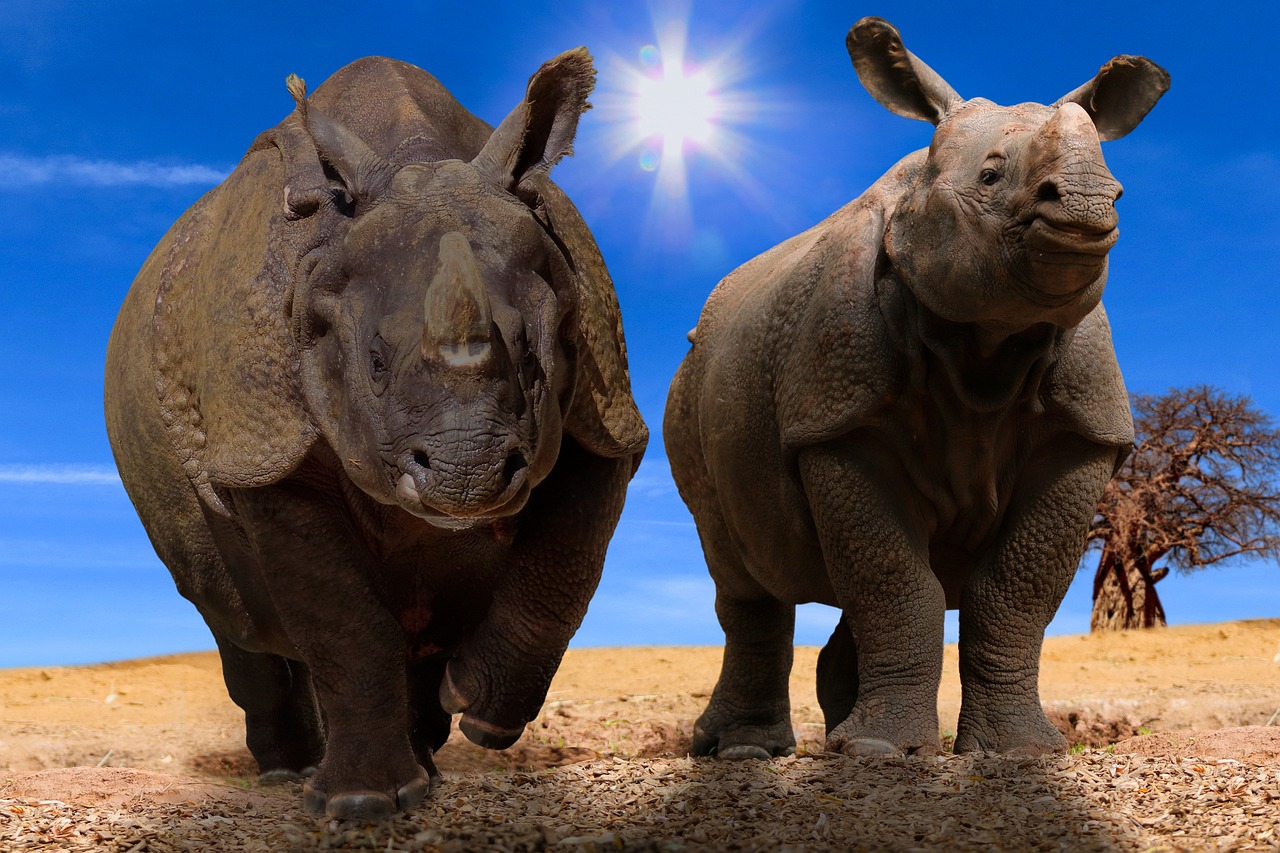
Machine Learning Algorithms
When it comes to understanding the complexities of our planet's climate, are like the superheroes of data analysis. These algorithms have the incredible ability to sift through mountains of historical climate data, learning patterns and making predictions that would be impossible for humans to achieve alone. Imagine having a personal assistant who not only remembers everything you’ve ever told them but also predicts what you might need next based on trends. That's exactly what machine learning does for climate science!
At the core of machine learning is the concept of training. Just as a student learns from textbooks and experiences, machine learning algorithms are trained on vast datasets. They analyze variables such as temperature, humidity, and atmospheric pressure, identifying correlations and trends over time. Once trained, these algorithms can forecast future climate conditions with remarkable accuracy. For instance, they can predict how a rise in global temperatures might lead to increased hurricane activity or drought in specific regions.
One of the most exciting aspects of machine learning is its ability to improve over time. As more data becomes available, these algorithms refine their predictions, becoming increasingly reliable. This is akin to a chef perfecting a recipe after numerous attempts; each iteration brings them closer to the perfect dish. In the realm of climate science, this means that our understanding of potential climate scenarios is constantly evolving, allowing policymakers and researchers to make informed decisions.
Furthermore, machine learning algorithms can be categorized into different types, each with unique strengths:
- Supervised Learning: This method involves training algorithms on labeled datasets, where the outcomes are known. For example, it can be used to predict rainfall patterns based on historical weather data.
- Unsupervised Learning: Here, algorithms identify patterns in data without prior labels. This is useful for discovering hidden trends in climate data, such as unusual temperature spikes.
- Reinforcement Learning: This approach allows algorithms to learn through trial and error, making it valuable for dynamic climate modeling where conditions constantly change.
The integration of machine learning in climate science is not just a trend; it’s a game changer. For instance, researchers have utilized these algorithms to develop models predicting the likelihood of extreme weather events, enabling communities to prepare and respond more effectively. By analyzing past weather patterns, machine learning can help forecast future occurrences, providing vital information for disaster preparedness.
In conclusion, machine learning algorithms are revolutionizing our approach to climate science. Their ability to analyze vast datasets, learn from them, and make predictions is invaluable in our fight against climate change. As we continue to refine these technologies, the potential for more accurate climate predictions and effective solutions grows exponentially, making our planet a safer place for future generations.
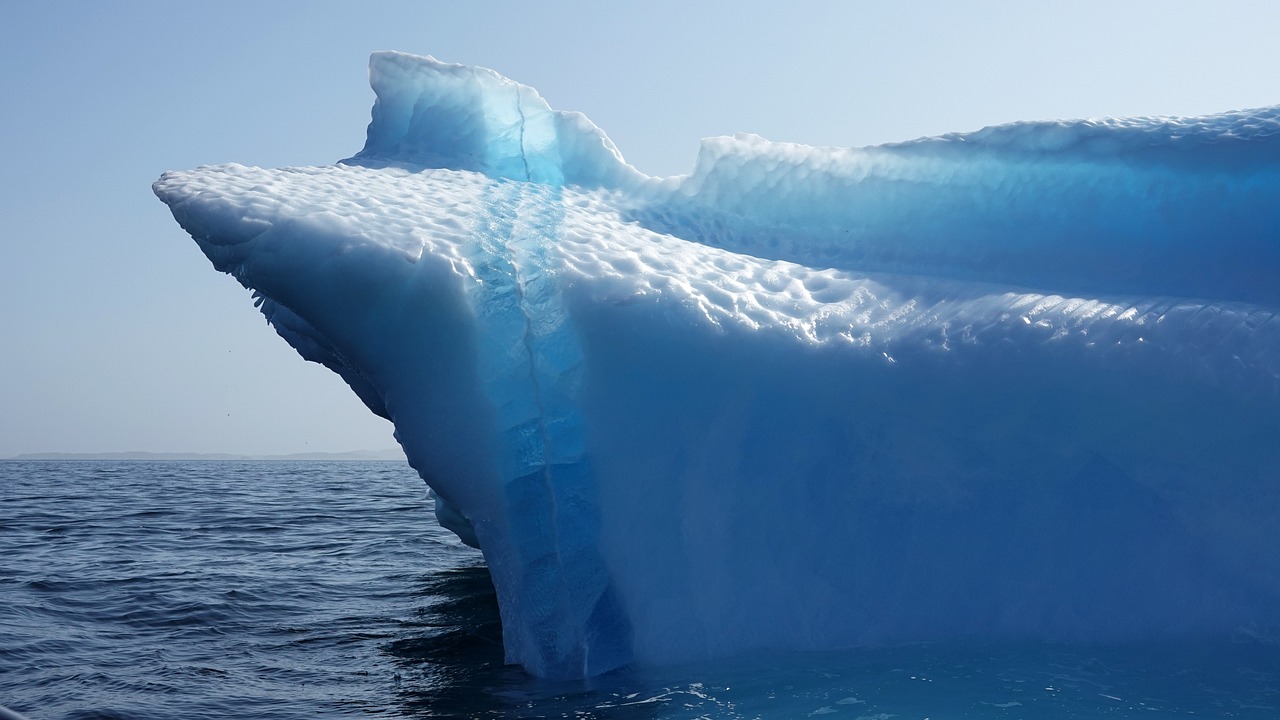
Neural Networks in Climate Studies
Neural networks have emerged as a powerful tool in the realm of climate studies, revolutionizing how scientists analyze and interpret complex climate data. These advanced algorithms mimic the way the human brain operates, allowing them to learn from vast amounts of information and identify intricate patterns that traditional models might miss. By processing data from various sources—such as satellite imagery, historical weather records, and oceanographic measurements—neural networks can uncover relationships between different climate variables, providing deeper insights into the dynamics of our planet's climate.
One of the most striking applications of neural networks is their ability to predict climate phenomena with remarkable accuracy. For instance, they can analyze historical data on temperature, precipitation, and atmospheric pressure to forecast future climate conditions. This predictive power is crucial for understanding and preparing for extreme weather events, such as hurricanes, droughts, and floods. In fact, studies have shown that neural networks can outperform traditional climate models in terms of accuracy and reliability. This is particularly important as we face an increasing frequency of climate-related disasters that demand immediate and effective responses.
Moreover, neural networks excel in recognizing patterns over time, which is essential for long-term climate projections. They can assess how factors like greenhouse gas emissions, deforestation, and urbanization influence climate change, helping researchers to develop more effective mitigation strategies. By integrating neural networks into climate models, scientists can create a more comprehensive view of the future climate landscape, leading to better-informed policy decisions and climate action plans.
To illustrate the effectiveness of neural networks in climate studies, consider the following table that highlights key advantages:
Advantages of Neural Networks | Description |
---|---|
Pattern Recognition | Neural networks can identify complex relationships in large datasets, which traditional models may overlook. |
Improved Accuracy | They often provide more reliable predictions compared to conventional climate models. |
Adaptability | Neural networks can be retrained with new data, allowing them to adapt to changing climate conditions. |
Real-Time Analysis | They can process data quickly, enabling real-time monitoring and forecasting of climate events. |
In summary, the integration of neural networks into climate studies represents a significant leap forward in our ability to understand and respond to climate change. By leveraging the power of these sophisticated algorithms, researchers can gain valuable insights that drive effective climate action. As we continue to face unprecedented environmental challenges, the role of neural networks in climate science will undoubtedly grow, offering hope and innovative solutions in our fight against climate change.
- What are neural networks? Neural networks are computational models inspired by the human brain that can learn from data and identify patterns.
- How do neural networks improve climate predictions? They analyze complex datasets, recognize patterns, and provide more accurate forecasts compared to traditional methods.
- Can neural networks adapt to new data? Yes, neural networks can be retrained with new information, allowing them to stay relevant as climate conditions change.
- What is the future of neural networks in climate studies? As technology advances, the use of neural networks in climate research is expected to expand, offering more innovative solutions to combat climate change.
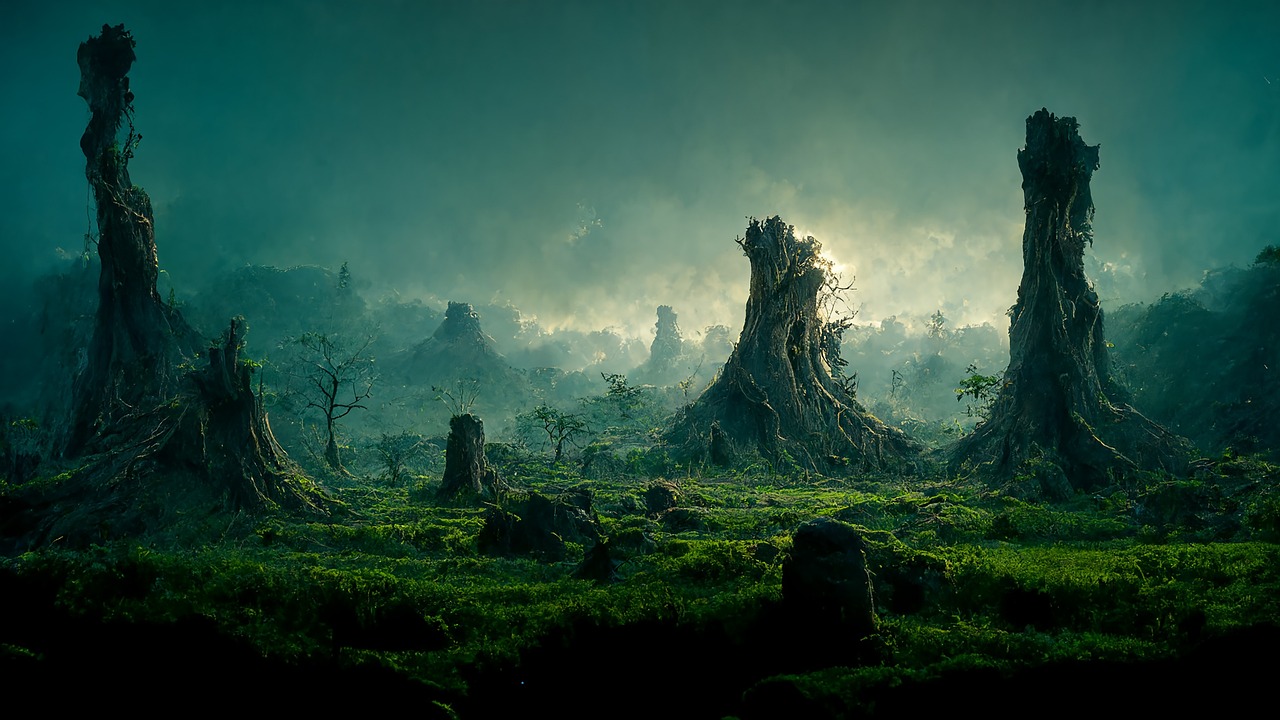
Data Mining for Climate Insights
Data mining is like digging for gold in a vast field of information. In the context of climate science, it involves sifting through enormous datasets to unearth valuable insights that can help us understand the complexities of climate change. By applying sophisticated algorithms, researchers can identify hidden patterns and trends that might otherwise go unnoticed. This process is crucial because the climate system is influenced by a multitude of factors, from greenhouse gas emissions to ocean currents. By leveraging data mining techniques, scientists can paint a clearer picture of how these factors interact and evolve over time.
One of the key advantages of data mining in climate studies is its ability to process data from various sources. Think about it: climate data comes from satellites, weather stations, ocean buoys, and even social media. Each of these sources generates massive amounts of information that, when analyzed collectively, can provide insights into climate trends and anomalies. For instance, researchers can analyze historical weather patterns alongside current satellite imagery to predict future climate scenarios more accurately.
To illustrate the impact of data mining on climate insights, consider the following table that summarizes how different data mining techniques contribute to understanding climate change:
Data Mining Technique | Application in Climate Science | Benefits |
---|---|---|
Clustering | Grouping similar climate events | Identifies trends and outliers |
Classification | Predicting climate events based on historical data | Enhances forecasting accuracy |
Regression Analysis | Understanding relationships between variables | Informs policy decisions and mitigation strategies |
Furthermore, data mining techniques can help in assessing the impacts of climate change on various ecosystems. For example, by analyzing data on temperature changes and species distribution, scientists can predict which species are at risk of extinction. This information is invaluable for conservation efforts, allowing policymakers to make informed decisions about resource allocation and habitat protection.
In summary, data mining serves as a powerful tool in the arsenal of climate scientists. By unlocking the secrets hidden within vast datasets, it enables a deeper understanding of climate dynamics and informs strategies for mitigation and adaptation. As we continue to face the challenges posed by climate change, the role of data mining will only become more critical in shaping our responses and ensuring a sustainable future.
- What is data mining in climate science? Data mining in climate science involves analyzing large datasets to uncover patterns and trends related to climate change.
- How does data mining improve climate predictions? By identifying hidden relationships and trends, data mining enhances the accuracy of climate models and forecasts.
- What are some common data mining techniques used in climate studies? Techniques include clustering, classification, and regression analysis, each serving different purposes in analyzing climate data.
- Why is data mining important for environmental policy? It provides critical insights that inform policy decisions, helping to allocate resources effectively and implement strategies for climate mitigation.
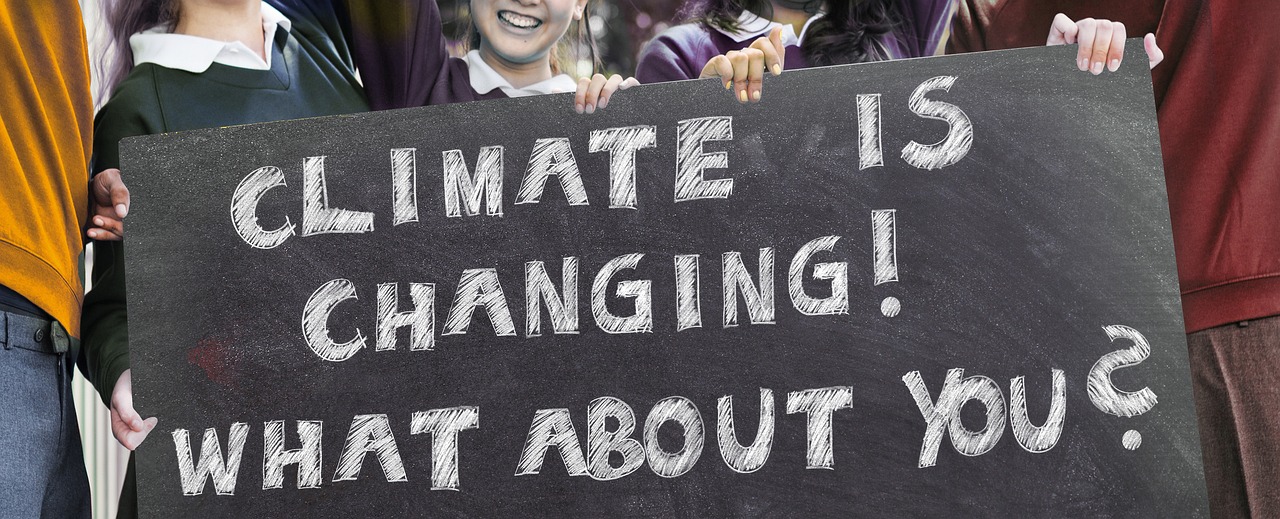
Integrating AI with Climate Models
As we venture deeper into the complexities of climate science, the integration of artificial intelligence (AI) with traditional climate models emerges as a game changer. Imagine trying to solve a massive jigsaw puzzle with thousands of pieces, where each piece represents a different variable affecting our climate. Alone, traditional models can struggle to fit these pieces together accurately, often leading to gaps in our understanding. However, when we introduce AI into the mix, it acts like a skilled puzzle master, helping to identify patterns and connections that might otherwise go unnoticed.
The beauty of integrating AI with climate models lies in its ability to enhance predictive capabilities. For instance, AI can process vast amounts of data—from satellite imagery to historical weather records—far more efficiently than a human ever could. This capability allows scientists to refine their models and improve their accuracy significantly. By utilizing machine learning algorithms, AI can learn from past climate data, adjusting its predictions based on new information. This dynamic learning process means that our climate models become more robust over time, adapting to the ever-changing nature of our environment.
One of the most exciting aspects of this integration is the potential for real-time data analysis. With AI, climate models can continuously update as new data comes in, providing more immediate insights into weather patterns and climate trends. This real-time capability is crucial for governments and organizations aiming to prepare for and respond to extreme weather events. When a hurricane is approaching, for example, having access to the most current predictive models can mean the difference between life and death.
Moreover, the synergy between AI and traditional models also fosters collaboration across various disciplines. Climate scientists, data analysts, and AI specialists can work together to create comprehensive models that incorporate social, economic, and environmental factors. This holistic approach not only enhances the accuracy of predictions but also informs better policy decisions that address the root causes of climate change.
In summary, the integration of AI with climate models is not just a technological advancement; it represents a paradigm shift in how we understand and respond to climate change. As we harness the power of AI, we can look forward to more reliable predictions and effective solutions that will help us navigate the challenges of a warming planet.
- How does AI improve climate predictions? AI enhances climate predictions by processing large datasets, identifying patterns, and continuously learning from new data.
- What are the benefits of integrating AI with traditional climate models? The integration leads to more accurate predictions, real-time updates, and a collaborative approach that considers various factors influencing climate change.
- Can AI help with immediate responses to climate-related disasters? Yes, AI can provide real-time data analysis, enabling quicker responses to extreme weather events and better preparedness.
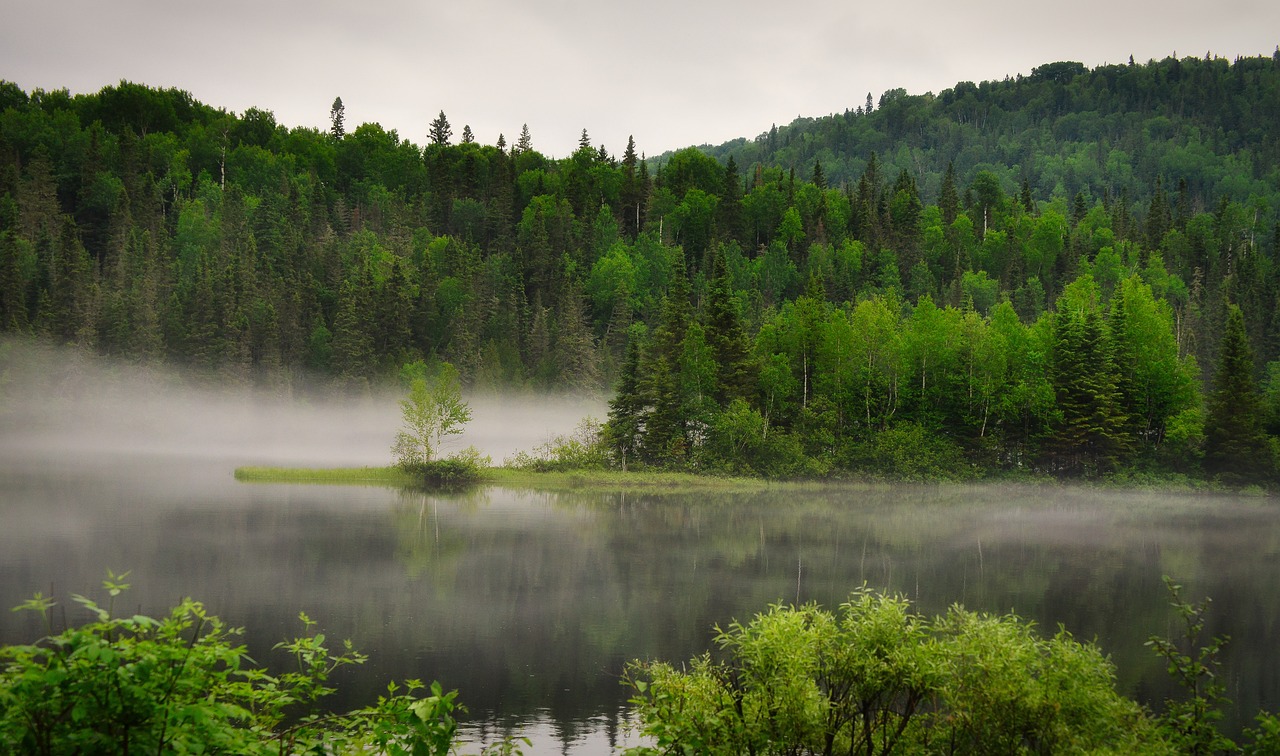
AI Solutions for Mitigating Climate Change
Artificial Intelligence (AI) is not just a tool for analyzing data; it is a beacon of hope in our fight against climate change. As we grapple with the pressing challenges posed by global warming, AI emerges as a key player, offering innovative solutions that go beyond mere predictions. Imagine a world where technology not only forecasts the weather but also actively contributes to a sustainable future. This is the reality we are moving towards, thanks to the integration of AI in various sectors.
One of the most significant areas where AI is making waves is in smart agriculture. Traditional farming practices often lead to resource wastage and environmental degradation. However, AI technologies are revolutionizing the agricultural landscape by optimizing resource use. For instance, AI can analyze soil health, weather patterns, and crop conditions to provide farmers with actionable insights. This means farmers can use water more efficiently, apply fertilizers in a targeted manner, and ultimately increase crop resilience against climate-related stresses. This not only boosts productivity but also significantly reduces the carbon footprint associated with agricultural practices.
Consider the following benefits of AI in agriculture:
- Precision Farming: AI tools enable farmers to monitor their fields in real-time, ensuring that inputs are applied only where needed.
- Predictive Analytics: By analyzing historical data, AI can forecast crop yields and identify potential issues before they escalate.
- Resource Optimization: AI systems can suggest the best times for planting and harvesting, minimizing waste and maximizing output.
Moreover, AI is crucial in driving energy efficiency innovations across various sectors. The energy sector is one of the largest contributors to greenhouse gas emissions, and AI is stepping up to tackle this challenge head-on. By employing machine learning algorithms, AI can analyze energy consumption patterns in real-time, identifying inefficiencies and suggesting improvements. For example, smart grids, powered by AI, can dynamically adjust energy distribution based on demand, reducing waste and optimizing resource use.
Additionally, AI can facilitate the transition to renewable energy sources. It can predict energy production from solar panels or wind turbines, allowing for better integration of these resources into the grid. This not only enhances reliability but also encourages the adoption of cleaner energy alternatives. The table below illustrates some of the key applications of AI in enhancing energy efficiency:
Application | Description |
---|---|
Smart Grids | AI optimizes energy distribution based on real-time demand. |
Predictive Maintenance | AI predicts equipment failures, reducing downtime and maintenance costs. |
Energy Management Systems | AI analyzes consumption patterns to suggest energy-saving measures. |
As we explore these AI-driven solutions, it becomes clear that technology is not just a spectator in the climate crisis; it is an active participant. By harnessing the power of AI, we can address some of the most pressing environmental challenges of our time. The synergy between AI and sustainability creates a pathway towards a greener future, where innovation and environmental stewardship go hand in hand.
In conclusion, the integration of AI into climate action strategies is not merely advantageous; it is essential. As we continue to develop and implement these technologies, we must remain committed to ensuring that they are used ethically and responsibly, prioritizing the health of our planet and future generations. The journey towards sustainability is complex, but with AI by our side, we are more equipped than ever to navigate the challenges ahead.
Q: How does AI help in predicting climate change?
A: AI enhances climate predictions by analyzing vast datasets, identifying patterns, and improving the accuracy of climate models, allowing for better forecasting of future scenarios.
Q: What role does AI play in agriculture?
A: AI optimizes resource use in agriculture by providing insights into soil health, weather patterns, and crop conditions, promoting sustainable farming practices.
Q: Can AI contribute to renewable energy sources?
A: Yes, AI helps predict energy production from renewable sources like solar and wind, facilitating their integration into the energy grid and enhancing overall efficiency.
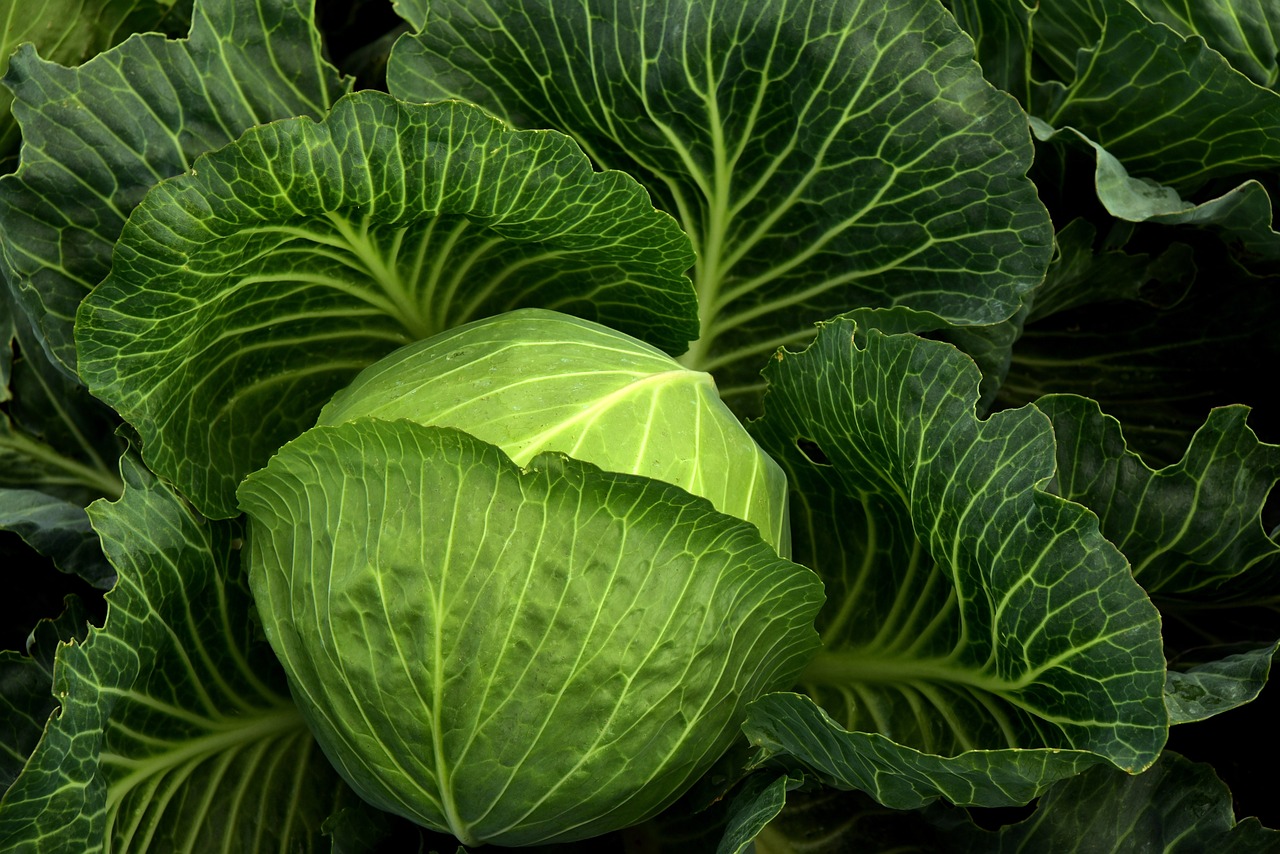
Smart Agriculture Practices
In the era of climate change, the way we approach agriculture is undergoing a significant transformation, thanks to the infusion of artificial intelligence. Imagine a world where farmers can predict weather patterns with pinpoint accuracy, optimize water usage, and enhance crop yields—all while minimizing environmental impact. This is not just a dream; it's the reality being shaped by AI technologies in smart agriculture practices.
At the heart of this revolution is the ability of AI to analyze vast amounts of data. Farmers are no longer solely reliant on traditional methods; instead, they harness data from various sources, including satellite imagery, soil sensors, and weather forecasts. By integrating this information, AI systems can provide actionable insights that lead to better decision-making. For instance, AI can help identify the optimal time for planting and harvesting, ensuring that crops are grown under the best possible conditions.
One of the most exciting applications of AI in agriculture is its role in precision farming. This approach utilizes technology to monitor and manage field variability in crops. By employing AI algorithms, farmers can achieve a level of precision that was previously unimaginable. For example, AI can analyze soil health and moisture levels, allowing for targeted irrigation that conserves water and reduces costs. This not only leads to healthier crops but also promotes sustainability by minimizing the overuse of resources.
Moreover, AI-driven solutions can enhance crop resilience against climate-related challenges. By predicting pest infestations or disease outbreaks, farmers can take proactive measures to protect their crops. This is particularly crucial in a world where extreme weather events are becoming more frequent. With AI's predictive capabilities, farmers can adapt their strategies to mitigate the impacts of climate change, ensuring food security for future generations.
To further illustrate the impact of AI in smart agriculture, consider the following table that highlights key AI applications and their benefits:
AI Application | Benefits |
---|---|
Crop Monitoring | Real-time data on crop health, enabling timely interventions. |
Soil Analysis | Improved understanding of soil conditions for better crop management. |
Weather Forecasting | Accurate predictions to optimize planting and harvesting schedules. |
Pest and Disease Management | Proactive measures to prevent crop loss, enhancing yield. |
In conclusion, the integration of AI into agriculture is not just a trend; it's a necessity for sustainable farming in a world facing climate change. By adopting smart agriculture practices, farmers can not only enhance their productivity but also contribute to a healthier planet. As we continue to innovate and leverage these technologies, the future of agriculture looks promising, paving the way for a more sustainable and resilient food system.
- How does AI improve crop yields? AI improves crop yields by providing data-driven insights that help farmers optimize their farming practices, such as irrigation and pest management.
- What is precision farming? Precision farming is an agricultural approach that utilizes AI and technology to monitor and manage field variability, leading to more efficient use of resources.
- Can AI help in reducing water usage in agriculture? Yes, AI can analyze soil moisture levels and weather forecasts to optimize irrigation schedules, thereby reducing water usage.
- How does AI contribute to sustainability in farming? AI contributes to sustainability by minimizing resource waste, enhancing crop resilience, and promoting efficient farming practices.
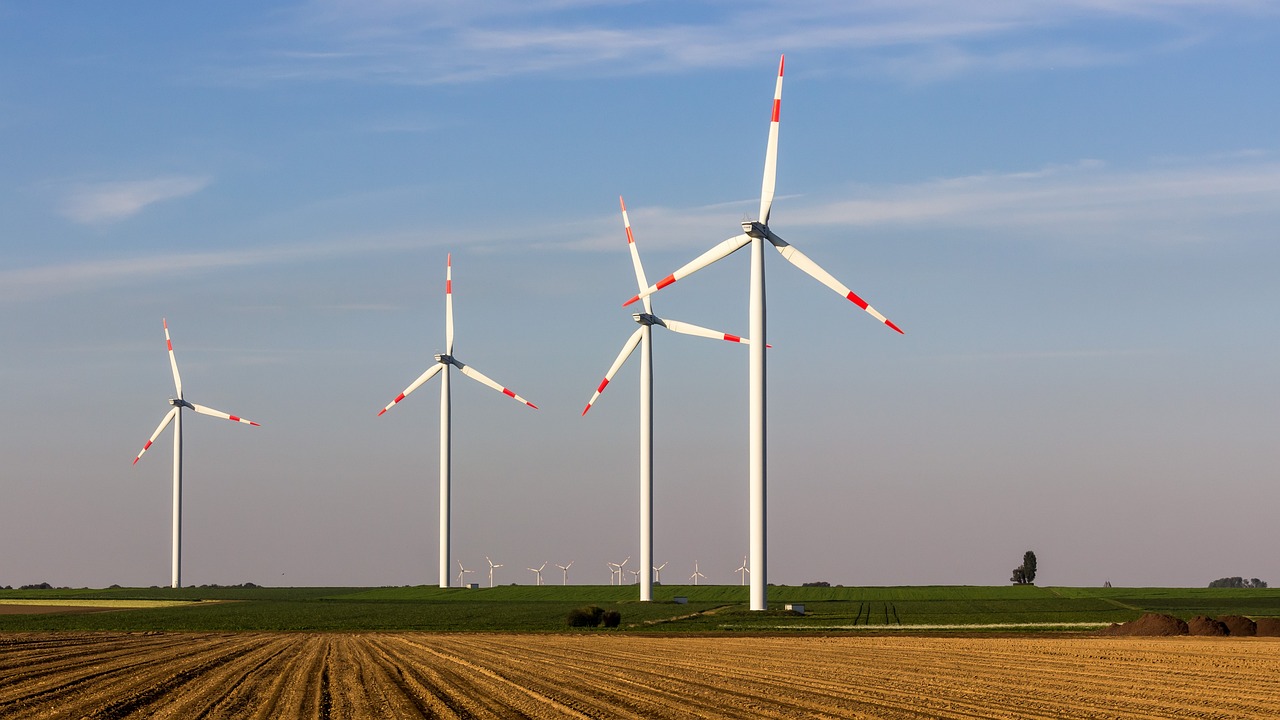
Energy Efficiency Innovations
In our quest to combat climate change, are emerging as a beacon of hope. These innovations not only help in reducing energy consumption but also play a critical role in minimizing greenhouse gas emissions. Imagine a world where our homes and industries consume less energy while maintaining the same level of comfort and productivity. This is not just a dream; it's becoming a reality through the power of artificial intelligence (AI).
AI technologies are revolutionizing the way we approach energy efficiency. From smart thermostats that learn your heating preferences to sophisticated energy management systems that optimize power usage in real-time, AI is at the forefront of this transformation. For instance, AI can analyze data from various sensors in a building to determine when to adjust heating, cooling, and lighting systems, ensuring that energy is used only when necessary. This not only saves money but also reduces the overall carbon footprint of buildings.
One of the most exciting developments in energy efficiency is the use of AI in smart grids. Traditional electrical grids are often inefficient, leading to energy loss and wastage. However, AI-driven smart grids can predict energy demand patterns and adjust the supply accordingly. This dynamic response capability ensures that energy is distributed efficiently, reducing the need for backup power plants and lowering emissions. In fact, studies have shown that integrating AI into grid management can improve efficiency by up to 30%.
Moreover, AI is also making waves in the industrial sector. Factories equipped with AI systems can monitor machinery in real-time, predicting when maintenance is needed and preventing energy waste. By optimizing processes and reducing downtime, these innovations can significantly lower energy consumption and increase productivity. For example, a manufacturing plant that implements AI-driven predictive maintenance can save thousands of dollars annually while also contributing to sustainability goals.
To illustrate the impact of AI on energy efficiency, consider the following table showcasing various AI applications and their benefits:
AI Application | Description | Benefits |
---|---|---|
Smart Thermostats | Devices that learn user preferences to optimize heating and cooling. | Reduces energy bills and enhances comfort. |
Energy Management Systems | Systems that analyze energy consumption patterns in real-time. | Improves energy use efficiency and lowers operational costs. |
Smart Grids | Automated electrical grids that manage energy distribution. | Enhances reliability and reduces energy waste. |
Predictive Maintenance | AI systems that predict equipment failures before they occur. | Minimizes downtime and conserves energy. |
As we continue to innovate, the integration of AI in energy efficiency is set to expand even further. With advancements in machine learning and data analytics, we can expect more sophisticated solutions that will not only save energy but also promote a sustainable future. It's an exciting time for energy efficiency, and the role of AI is pivotal in shaping how we consume energy.
- What is energy efficiency? Energy efficiency refers to using less energy to provide the same service or achieve the same outcome.
- How does AI improve energy efficiency? AI optimizes energy consumption by analyzing data, predicting demand, and automating energy management systems.
- What are smart grids? Smart grids are electrical grids that use digital technology to monitor and manage the transport of electricity from all generation sources to meet the varying electricity demands of end users.
- Can AI help reduce carbon emissions? Yes, by improving energy efficiency and optimizing energy use, AI can significantly reduce carbon emissions associated with energy production and consumption.
Frequently Asked Questions
- What is the role of AI in climate science?
AI plays a transformative role in climate science by enhancing climate modeling, processing vast datasets, and identifying complex patterns. This allows scientists to make more accurate predictions about climate dynamics and future scenarios.
- How do machine learning algorithms improve climate predictions?
Machine learning algorithms analyze historical climate data to identify trends and patterns. By learning from past data, these algorithms can make reliable forecasts about future climate behaviors, helping us prepare for extreme weather events.
- What are neural networks, and how are they used in climate studies?
Neural networks are advanced computational models that mimic the human brain's learning process. In climate studies, they are used to recognize complex climate patterns, enhancing the accuracy of climate forecasts and improving our understanding of climate change.
- How does data mining contribute to climate insights?
Data mining techniques help uncover hidden trends and relationships within climate data. By analyzing large datasets, these methods provide valuable insights that can inform policy decisions and enhance our understanding of climate change impacts.
- What does integrating AI with traditional climate models achieve?
Integrating AI with traditional climate models leads to more robust and accurate predictions. This synergy enhances the overall predictive capabilities, allowing for better preparedness and response strategies to climate-related challenges.
- How is AI transforming agriculture for climate change mitigation?
AI technologies are revolutionizing agriculture by optimizing resource usage, improving crop resilience, and promoting sustainable practices. These innovations help farmers adapt to climate change while minimizing environmental impact.
- What innovations in energy efficiency are driven by AI?
AI plays a crucial role in enhancing energy efficiency across various sectors by optimizing energy consumption and reducing waste. These innovations support the transition to renewable energy sources, contributing to a more sustainable future.