Decoding the Intersection of AI and Ethics
In today's fast-paced digital world, the intersection of artificial intelligence (AI) and ethics has become a hot topic of discussion. As AI technology continues to evolve and permeate various aspects of our lives—from healthcare to finance and beyond—it's crucial to examine the ethical implications that accompany these advancements. But what exactly does it mean to navigate the ethical landscape of AI? It's not just about making decisions; it's about ensuring that those decisions are fair, transparent, and accountable.
Imagine a world where AI systems make decisions that impact our daily lives. Whether it's a hiring algorithm selecting candidates or a self-driving car determining the safest route, the stakes are incredibly high. With great power comes great responsibility, and this is where ethical considerations come into play. As we delve deeper into the complexities of AI, we must ask ourselves: Are we prepared to address the ethical dilemmas that arise? How can we ensure that AI serves humanity rather than undermines it?
The relationship between AI and ethics is not merely theoretical; it's a pressing reality that demands our attention. As we explore this intricate web, we will uncover key challenges such as bias, accountability, and transparency. Each of these elements plays a critical role in shaping the future of AI and its impact on society. With the rapid pace of technological advancement, the need for a robust ethical framework is more crucial than ever. This framework will guide the development and implementation of AI systems, ensuring they are designed with a strong moral compass.
In this article, we will navigate through the ethical dilemmas posed by AI, examining how biases in algorithms can lead to unfair outcomes. We will discuss the importance of accountability in AI development and explore the need for transparency and explainability in AI systems. Furthermore, we will highlight the role of policy in shaping ethical AI practices and the implications of these practices for the future. By the end of our journey, we hope to provide insights that not only inform but also empower stakeholders to engage in ethical AI development.
As we embark on this exploration, let's keep in mind that the conversation surrounding AI and ethics is ongoing. The landscape is constantly evolving, and so too must our understanding and approach to these critical issues. So, are you ready to dive in and decode the intersection of AI and ethics? Let's get started!
This section outlines the primary ethical dilemmas posed by AI, including bias, accountability, and transparency, emphasizing the need for a robust ethical framework to guide AI development and implementation.
- What is AI ethics? AI ethics refers to the moral implications and responsibilities associated with the use of artificial intelligence technologies.
- Why is bias in AI a concern? Bias in AI can lead to unfair treatment of individuals or groups, perpetuating existing inequalities and injustices.
- How can we ensure accountability in AI? Establishing clear guidelines and regulations, along with corporate responsibility, can help ensure accountability in AI development.
- What role does transparency play in AI? Transparency fosters trust and understanding, allowing users to comprehend how AI systems make decisions.

The Ethical Dilemmas of AI
The rapid evolution of artificial intelligence (AI) has ushered in a new era of technological advancements, but it has also brought forth a myriad of ethical dilemmas that we must confront. As we integrate AI into various aspects of our lives—from healthcare to finance and even our daily routines—we face questions that challenge our moral compass. What happens when an AI system makes a decision that adversely affects an individual? Who is responsible for those decisions? These questions aren't just academic; they have real-world implications that can shape society.
One of the primary ethical dilemmas in AI is bias. AI systems learn from data, and if that data reflects societal prejudices, the AI can perpetuate and even amplify those biases. This raises the question: how can we ensure that AI serves all segments of society fairly? The need for a robust ethical framework is more pressing than ever. Without it, we risk creating technology that not only fails to benefit everyone but actively harms marginalized communities.
Another critical issue is accountability. When an AI system causes harm, pinpointing responsibility can be incredibly challenging. Is it the developer, the company that deployed the AI, or the user who misinterpreted its outputs? To navigate these murky waters, we need clear guidelines that define accountability in AI development. This is not merely a legal issue; it’s a question of ethical responsibility and trust.
Furthermore, we cannot overlook the importance of transparency. Many AI systems operate as "black boxes," where even their creators struggle to understand how decisions are made. This lack of transparency can erode public trust and hinder ethical decision-making. If users cannot comprehend how an AI system arrived at a conclusion, how can they trust its outputs? The call for explainable AI is growing louder, emphasizing the need for systems that are not only effective but also understandable.
In conclusion, as we stand at the intersection of AI and ethics, we must grapple with these dilemmas head-on. The way we address issues such as bias, accountability, and transparency will define the future of AI and its role in society. It is imperative that we establish a comprehensive ethical framework that guides the development and implementation of AI technologies. Only then can we harness the full potential of AI while ensuring that it serves the greater good.
- What is the main ethical dilemma associated with AI? The primary ethical dilemmas include bias, accountability, and transparency.
- How does bias affect AI systems? Bias in AI arises from skewed data, leading to unfair outcomes that can disproportionately affect marginalized groups.
- Why is transparency important in AI? Transparency fosters trust, allowing users to understand how AI systems make decisions and ensuring ethical accountability.
- Who is responsible when an AI system causes harm? Responsibility can be complex, involving developers, companies, and users, highlighting the need for clear accountability guidelines.

Bias in AI Algorithms
When we think about artificial intelligence, we often envision a world where machines make decisions with unparalleled accuracy and efficiency. However, lurking beneath the surface is a troubling reality: bias in AI algorithms. This bias can lead to unfair and discriminatory outcomes, affecting various aspects of our lives, from hiring practices to law enforcement. It's like a double-edged sword—while AI has the potential to enhance our lives, it can also inadvertently perpetuate existing inequalities if not carefully managed.
At the heart of this issue lies the data that fuels AI systems. If the data used to train these algorithms is biased, the results will be too. For instance, imagine teaching a child with only one perspective of the world; their understanding would be severely limited. Similarly, AI systems trained on biased datasets may reflect historical prejudices or societal stereotypes, leading to skewed decision-making. This isn't just a technical problem; it’s a social one, demanding our immediate attention and action.
Understanding the sources of bias in AI is crucial for addressing the problem. Bias can stem from various places, including:
- Historical Data: AI systems often learn from historical data, which may contain past prejudices and inequalities.
- Societal Prejudices: If the data reflects societal biases, the AI will likely replicate these biases in its decision-making.
- Data Collection Practices: How data is collected can also introduce bias, especially if certain groups are underrepresented.
Each of these sources contributes to a cycle of bias that can severely impact the fairness and reliability of AI systems. For instance, a hiring algorithm trained on data from a company that historically favored one demographic may unfairly disadvantage qualified candidates from other backgrounds. This raises the question: how can we ensure that AI serves all members of society equitably?
Data collection practices play a pivotal role in determining the fairness of AI algorithms. If data is collected without considering diversity and representation, the resulting AI models may fail to account for the nuances of different groups. It’s like trying to bake a cake without all the necessary ingredients—something essential is missing, and the final product will be less than satisfying. Therefore, implementing diverse and representative datasets is not just a recommendation; it’s a necessity for creating equitable AI.
Another layer to this issue is the influence of human decisions on AI outcomes. The individuals who design and deploy AI systems bring their own biases, whether consciously or unconsciously. If an AI developer has a narrow worldview, it’s likely that their creation will reflect that limited perspective. This is where ethical considerations must come into play—ensuring that diverse teams are involved in the development process can help mitigate bias and lead to more well-rounded AI systems.
So, what can we do to combat bias in AI algorithms? The answer lies in a combination of strategies aimed at promoting fairness. For starters, implementing algorithmic fairness techniques can help identify and correct biases within AI models. Additionally, continuous monitoring of AI systems is essential to ensure that they remain equitable over time. Just like a garden needs regular tending to flourish, AI algorithms require ongoing evaluation to ensure they serve their intended purpose without perpetuating harm.
In conclusion, the challenge of bias in AI algorithms is multifaceted and requires a concerted effort from developers, policymakers, and society at large. By recognizing the sources of bias, improving data collection practices, and fostering diverse perspectives in AI development, we can pave the way for a more equitable future. As we continue to innovate, let’s ensure that our technological advancements uplift everyone, not just a select few.
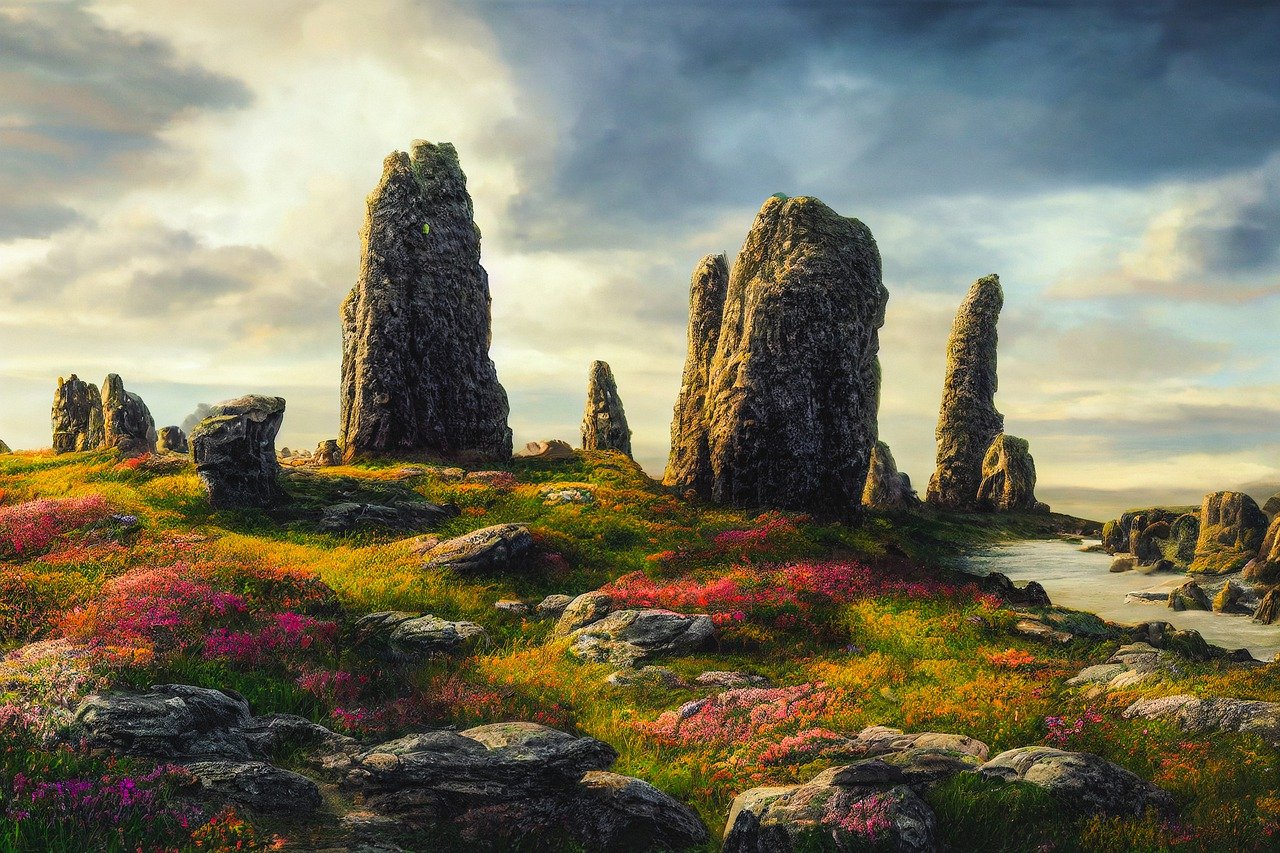
Sources of Bias
When we dive into the world of artificial intelligence, one of the most pressing issues we come across is bias. It's like a hidden trap that can skew the outcomes of AI systems, leading to unfair advantages or disadvantages for certain groups. But where does this bias come from? Understanding the sources of bias is crucial for developing fair and equitable AI technologies.
Bias in AI systems often stems from two primary sources: historical data and societal prejudices. Historical data refers to the datasets used to train AI algorithms, which can reflect past inequalities or injustices. For instance, if an AI system is trained on data that predominantly features one demographic, it may struggle to perform accurately for individuals outside that group. This is akin to teaching a child only about one culture; their understanding of the world will be limited and skewed.
On the other hand, societal prejudices play a significant role in shaping the data we collect. These biases can seep into AI systems through various channels, including:
- Data Collection Practices: If data is collected in a way that overlooks certain communities or perspectives, it creates a skewed representation.
- Human Influence: The decisions made by developers and data scientists can introduce their own biases, whether intentional or not.
- Feedback Loops: Once an AI system is deployed, it often learns from its interactions with users, which can reinforce existing biases if not monitored correctly.
To illustrate this further, consider a hiring algorithm that is trained on historical hiring data. If that data reflects a bias against certain groups, the AI is likely to continue that trend, perpetuating inequality in job recruitment. It's a vicious cycle that can have far-reaching consequences in sectors like finance, healthcare, and law enforcement.
Ultimately, recognizing these sources of bias is the first step toward addressing them. By understanding how biases enter AI systems, we can begin to develop strategies to mitigate their impact. This involves not just refining data collection practices but also fostering a culture of inclusivity and awareness among AI developers. The journey towards equitable AI is ongoing, but it starts with acknowledging the roots of bias.
- What is bias in AI? Bias in AI refers to systematic errors in the outcomes of AI systems that lead to unfair treatment of certain groups.
- How does bias enter AI systems? Bias can enter AI systems through historical data that reflect past prejudices, as well as through human influence during the design and implementation stages.
- Why is it important to address bias in AI? Addressing bias is crucial for ensuring that AI technologies are fair, equitable, and do not perpetuate existing inequalities in society.

Data Collection Practices
Data collection practices are at the heart of how artificial intelligence systems learn and evolve. The data used to train AI models can significantly influence their behavior and outcomes, making it crucial to understand the nuances of these practices. When we talk about data collection, we are essentially discussing the methods and processes that gather information from various sources to create datasets. These datasets are the lifeblood of AI, fueling everything from machine learning algorithms to predictive analytics. However, if not done carefully, data collection can lead to unintended consequences that may perpetuate bias or create ethical dilemmas.
One of the primary concerns with data collection is the representativeness of the data. If the data collected is skewed or lacks diversity, the AI models trained on this data will reflect those limitations. For instance, if an AI system is trained predominantly on data from one demographic group, it may struggle to perform effectively for users outside that group. This is particularly alarming in sensitive areas like healthcare or criminal justice, where biased AI outcomes can have serious repercussions.
Additionally, the methods employed in data collection can vary widely, influencing the quality and reliability of the data. Some common practices include:
- Surveys and Questionnaires: Often used to gather opinions or experiences from a specific group.
- Web Scraping: Automated tools extract data from websites, which can introduce biases based on the sources selected.
- Sensor Data: Devices collect real-time data, but issues can arise if the sensors are not calibrated correctly or if they are only deployed in specific locations.
Moreover, ethical considerations should guide data collection practices. For example, obtaining informed consent from individuals whose data is being collected is critical. Without transparency, users may feel uncomfortable or exploited, leading to a lack of trust in AI systems. Organizations must prioritize ethical data collection by ensuring that users understand how their data will be used and protected.
In conclusion, data collection practices are not just technical procedures; they are a reflection of our ethical values and societal norms. As we move forward in an increasingly AI-driven world, it is essential to adopt comprehensive strategies that promote diversity, equity, and transparency in data collection. By doing so, we can mitigate the risks associated with bias and ensure that AI technology serves all members of society fairly and justly.
- What is data collection in AI? Data collection in AI refers to the process of gathering and organizing information that will be used to train AI systems.
- Why is data diversity important? Data diversity ensures that AI models can perform effectively across different demographic groups, reducing the risk of biased outcomes.
- How can organizations ensure ethical data collection? Organizations can ensure ethical data collection by obtaining informed consent, being transparent about data usage, and prioritizing diversity in their datasets.

Human Influence on AI
When we think about artificial intelligence, it’s easy to imagine autonomous machines making decisions without human intervention. However, the reality is quite different. Human influence is deeply embedded in every stage of AI development, from the initial design to the final deployment. This influence can manifest in various ways, shaping the algorithms that drive AI systems and ultimately affecting their outcomes. Have you ever wondered how the choices made by developers can lead to unintended consequences in AI behavior? Let’s dive deeper into this fascinating topic.
At the core of AI is data, and the data we use is a reflection of our human biases, choices, and societal norms. For instance, if a dataset is predominantly composed of information from one demographic, the AI trained on that data may not perform well for others. This is where human influence becomes a double-edged sword; while we can create powerful tools, we also risk perpetuating existing inequalities. As a result, it’s crucial for developers to be aware of the implications of their data choices. The question then arises: how can we ensure that AI systems are fair and representative?
To address this challenge, we need to implement a few key strategies:
- Diverse Data Collection: Actively seek out diverse datasets that represent various demographics and perspectives. This helps create a more balanced AI model.
- Inclusive Design Teams: Assemble teams from different backgrounds to bring a variety of viewpoints into the design process. This can lead to more thoughtful and equitable AI solutions.
- Continuous Feedback Loops: Establish mechanisms for ongoing feedback from users and stakeholders to identify biases and improve AI systems over time.
Moreover, the human element extends beyond data. Developers and engineers make decisions about algorithms, which can also introduce bias. For example, the choice of which features to prioritize in a model can significantly impact its performance. If a developer unconsciously favors certain attributes over others, the AI may inadvertently learn to favor those attributes, leading to skewed results. This highlights the need for ethical considerations throughout the design process. Developers must ask themselves: what values are being encoded in these algorithms, and are they reflective of a fair society?
As we move forward in this rapidly evolving technological landscape, it’s essential to cultivate a culture of responsibility among AI practitioners. This means not only recognizing the power of their work but also understanding the potential consequences of their decisions. The influence of humans on AI is profound, and with that influence comes the responsibility to ensure that the technology we create serves everyone equitably.
In conclusion, the interplay between human influence and AI is complex, and it necessitates a thoughtful approach to development. By acknowledging our biases, prioritizing inclusivity, and fostering a culture of accountability, we can harness the full potential of AI while mitigating the risks associated with human influence. So, are we ready to take on this challenge and create a future where AI benefits all of humanity?
- What is the role of humans in AI development? Humans play a critical role in shaping AI systems through data selection, algorithm design, and ethical considerations.
- How can bias be introduced into AI systems? Bias can be introduced through unrepresentative datasets, flawed algorithm choices, and the unconscious biases of developers.
- What strategies can help mitigate bias in AI? Strategies include using diverse datasets, forming inclusive design teams, and establishing continuous feedback mechanisms.
- Why is transparency important in AI? Transparency helps build trust and allows users to understand how AI systems make decisions, which is essential for ethical practices.

Mitigating Bias in AI
As we delve deeper into the realm of artificial intelligence, the challenge of mitigating bias becomes more pressing than ever. Bias in AI systems can lead to significant consequences, affecting individuals and communities in profound ways. To tackle this issue, it's essential to adopt a multi-faceted approach that combines technological solutions with ethical considerations. One of the most effective strategies is to implement algorithmic fairness techniques, which aim to identify and rectify biases present in AI models. These techniques can help ensure that AI systems operate in a manner that is fair and equitable, promoting inclusivity rather than discrimination.
Moreover, continuous monitoring of AI systems is crucial. By regularly assessing the performance of AI algorithms, stakeholders can identify any emerging biases that may not have been apparent during the initial development phase. This proactive approach allows for timely interventions and adjustments, ensuring that AI systems remain aligned with ethical standards. For instance, organizations can establish feedback loops where users can report biased outcomes, thus fostering a culture of accountability and transparency.
Another key aspect of mitigating bias lies in the diversity of training data. AI systems learn from the data they are trained on, and if that data is unrepresentative or skewed, the resulting algorithms will likely perpetuate those biases. Therefore, it is crucial to curate datasets that reflect a wide range of perspectives and experiences. This might involve collecting data from underrepresented groups or utilizing synthetic data to fill gaps. By ensuring that AI systems are trained on diverse datasets, we can significantly reduce the risk of biased outcomes.
In addition to data diversity, the influence of human decisions on AI cannot be overlooked. Developers and data scientists must be cognizant of their own biases when designing AI systems. This awareness can be cultivated through training programs that emphasize ethical AI practices and the importance of bias mitigation. By fostering a mindset that prioritizes fairness and equity, we can create a new generation of AI practitioners who are equipped to tackle these complex challenges head-on.
Ultimately, mitigating bias in AI is not just a technical challenge; it is a societal imperative. As we continue to integrate AI into various facets of our lives, we must remain vigilant in our efforts to ensure that these technologies serve all individuals equitably. By embracing a comprehensive strategy that includes algorithmic fairness, continuous monitoring, diverse data practices, and ethical training, we can work towards a future where AI enhances our society without perpetuating existing inequalities.
- What is bias in AI? Bias in AI refers to systematic errors that lead to unfair outcomes, often resulting from biased training data or flawed algorithms.
- How can bias in AI be mitigated? Bias can be mitigated through algorithmic fairness techniques, continuous monitoring, diverse training datasets, and ethical training for developers.
- Why is diversity in training data important? Diversity ensures that AI systems learn from a wide range of perspectives, reducing the likelihood of biased outcomes.
- What role do developers play in mitigating bias? Developers must be aware of their own biases and prioritize ethical practices in AI design to create fair systems.

Accountability in AI Development
As artificial intelligence (AI) continues to weave itself into the fabric of our daily lives, the question of accountability in AI development becomes increasingly pressing. Who is responsible when an AI system makes a mistake? Is it the developers, the companies that deploy the AI, or perhaps the users who interact with it? These questions are not just academic; they have real-world implications that can affect people's lives, livelihoods, and even their safety.
One of the primary challenges in establishing accountability in AI is the complexity of these systems. Unlike traditional software, AI systems can learn and evolve over time, often in ways that are not fully understood even by their creators. This leads to a murky landscape where responsibility can be difficult to pinpoint. For instance, if an autonomous vehicle gets into an accident, should the blame fall on the manufacturer, the software developers, or the vehicle owner? This ambiguity creates a significant barrier to accountability, making it essential for stakeholders to establish clear guidelines.
To navigate these challenges, there needs to be a robust framework that defines roles and responsibilities in AI development. This framework should consider various factors, including:
- Design Decisions: Developers must be held accountable for the choices they make during the AI design process.
- Data Usage: Companies should ensure that the data used to train AI systems is ethically sourced and free of bias.
- Outcome Monitoring: Continuous monitoring of AI systems is crucial to identify and rectify any harmful outcomes.
Additionally, legal frameworks must evolve to address the unique challenges posed by AI. Current laws often lag behind technological advancements, creating gaps in accountability. For example, existing liability laws may not adequately cover situations where AI systems operate autonomously. This is where new regulations could play a pivotal role, ensuring that there are clear legal precedents for holding parties accountable in the event of AI-related harm.
Corporate responsibility also plays a vital role in AI accountability. Companies must adopt a culture of ethical AI development, which includes being transparent about how their systems work and the decisions made during their creation. This means not just adhering to legal requirements, but also going above and beyond to ensure that their AI technologies are safe and equitable. Corporate social responsibility initiatives can help foster this mindset, encouraging organizations to prioritize ethical considerations in their AI strategies.
Ultimately, the path to accountability in AI development is not straightforward. It requires collaboration among various stakeholders, including governments, industry leaders, and the public. By working together, we can create a comprehensive framework that not only holds parties accountable but also fosters trust in AI technologies. As we move forward, it will be crucial to engage in ongoing dialogue about these issues, ensuring that our ethical standards evolve alongside technological advancements.
- What is accountability in AI development? Accountability in AI development refers to the responsibility of developers, companies, and users for the outcomes produced by AI systems.
- Why is accountability important in AI? It is crucial for ensuring that AI technologies are safe, fair, and ethical, protecting individuals from potential harm.
- How can companies ensure accountability in AI? Companies can ensure accountability by adopting ethical practices in AI development, monitoring outcomes, and being transparent about their processes.
- What role do regulations play in AI accountability? Regulations can establish legal standards that define responsibilities and liabilities in AI-related incidents.
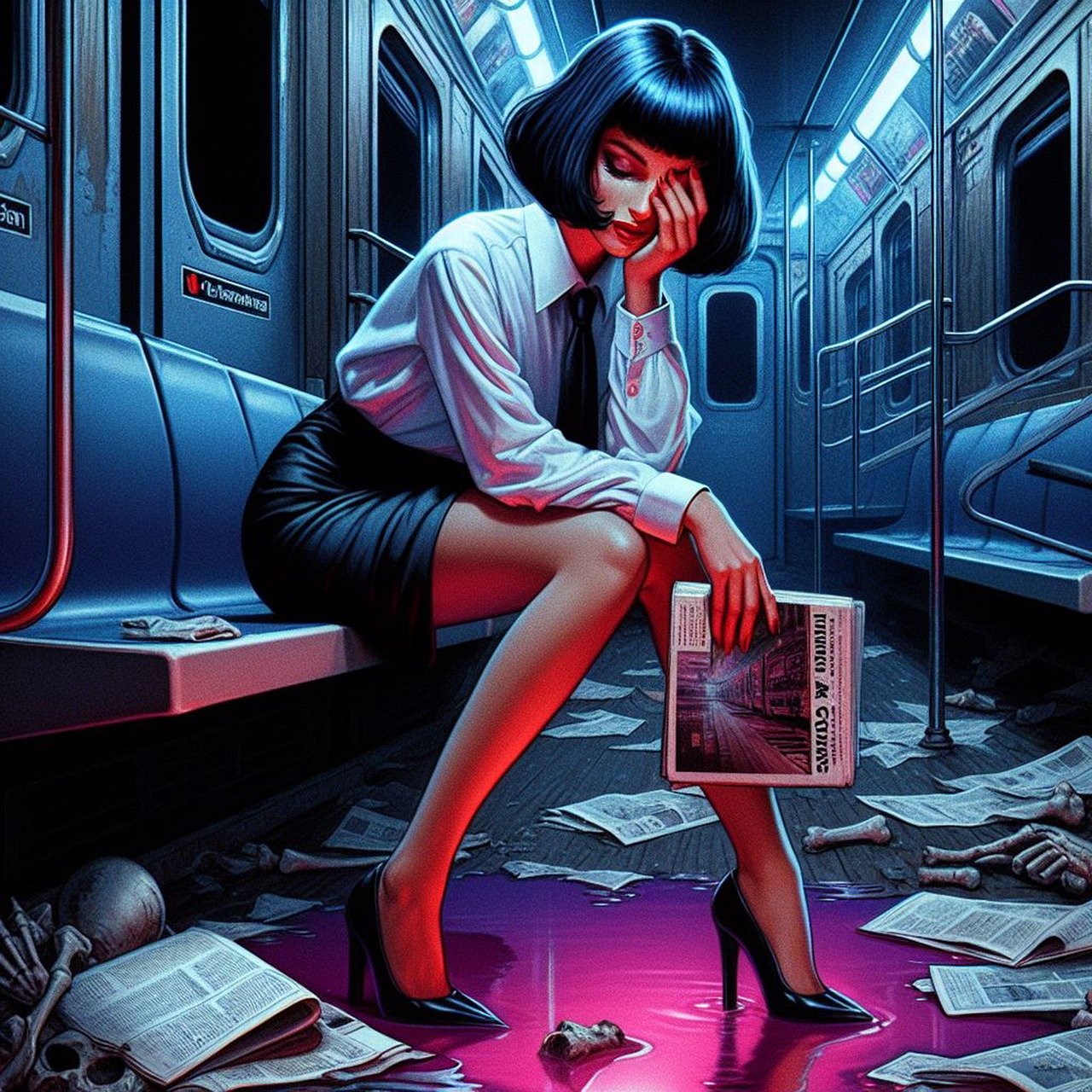
Legal Frameworks
As we delve into the complex world of artificial intelligence, one of the most pressing questions is: Who is responsible when AI systems go awry? The legal frameworks currently in place often struggle to keep pace with the rapid advancements in AI technology. Traditional laws were designed for a different era, and now we find ourselves in a situation where these frameworks are being stretched to their limits. This raises a critical need for new regulations that not only address accountability but also ensure that AI development is conducted ethically and responsibly.
Existing legal frameworks vary significantly across different jurisdictions, leading to a patchwork of regulations that can create confusion and inconsistency. For instance, in the European Union, the General Data Protection Regulation (GDPR) has made strides in protecting personal data, but it does not specifically address the unique challenges posed by AI systems. In contrast, the United States has a more fragmented approach, with regulations often left to individual states. This inconsistency can hinder innovation and complicate compliance for global companies.
One of the primary challenges in establishing effective legal frameworks is the dynamic nature of AI technology. Laws that are too rigid may stifle innovation, while those that are too lenient could lead to significant ethical breaches. Thus, a balance must be struck. Legal experts and policymakers are increasingly advocating for a flexible approach that allows for the evolution of laws as technology advances. This could involve:
- Regular reviews of existing laws to ensure they remain relevant.
- Involving technologists in the legislative process to provide insights into the implications of new technologies.
- Establishing a framework for international cooperation to develop cohesive global standards.
Furthermore, the question of liability becomes complex when AI systems make decisions autonomously. For example, if an autonomous vehicle causes an accident, is the manufacturer, the software developer, or the vehicle owner responsible? This ambiguity can lead to legal battles that drain resources and slow down the adoption of beneficial technologies.
To address these challenges, some countries are beginning to draft specific legislation aimed at AI accountability. For instance, proposals have been made for “AI liability laws” that would clearly define the responsibilities of AI developers and users. These laws could help clarify who is accountable in various scenarios, thereby reducing the uncertainty that currently hampers the industry.
Ultimately, the development of robust legal frameworks for AI is essential not just for accountability, but also for fostering public trust. If individuals and organizations believe that there are solid laws in place to protect their rights and interests, they are more likely to embrace AI technologies. As we navigate this uncharted territory, the collaboration between legal experts, technologists, and policymakers will be crucial in shaping a future where AI can thrive ethically and responsibly.
- What are the main legal challenges associated with AI?
The main challenges include accountability, liability, and the adequacy of existing laws to address the unique aspects of AI technology. - How can legal frameworks evolve to keep pace with AI advancements?
By incorporating flexibility into laws, regularly reviewing regulations, and involving technologists in the legislative process. - Who is responsible if an AI system causes harm?
This is a complex issue that may involve multiple parties, including manufacturers, software developers, and users, depending on the circumstances. - Are there specific laws for AI accountability?
Some countries are beginning to draft AI liability laws aimed at clarifying responsibilities, but this is still an emerging area of law.
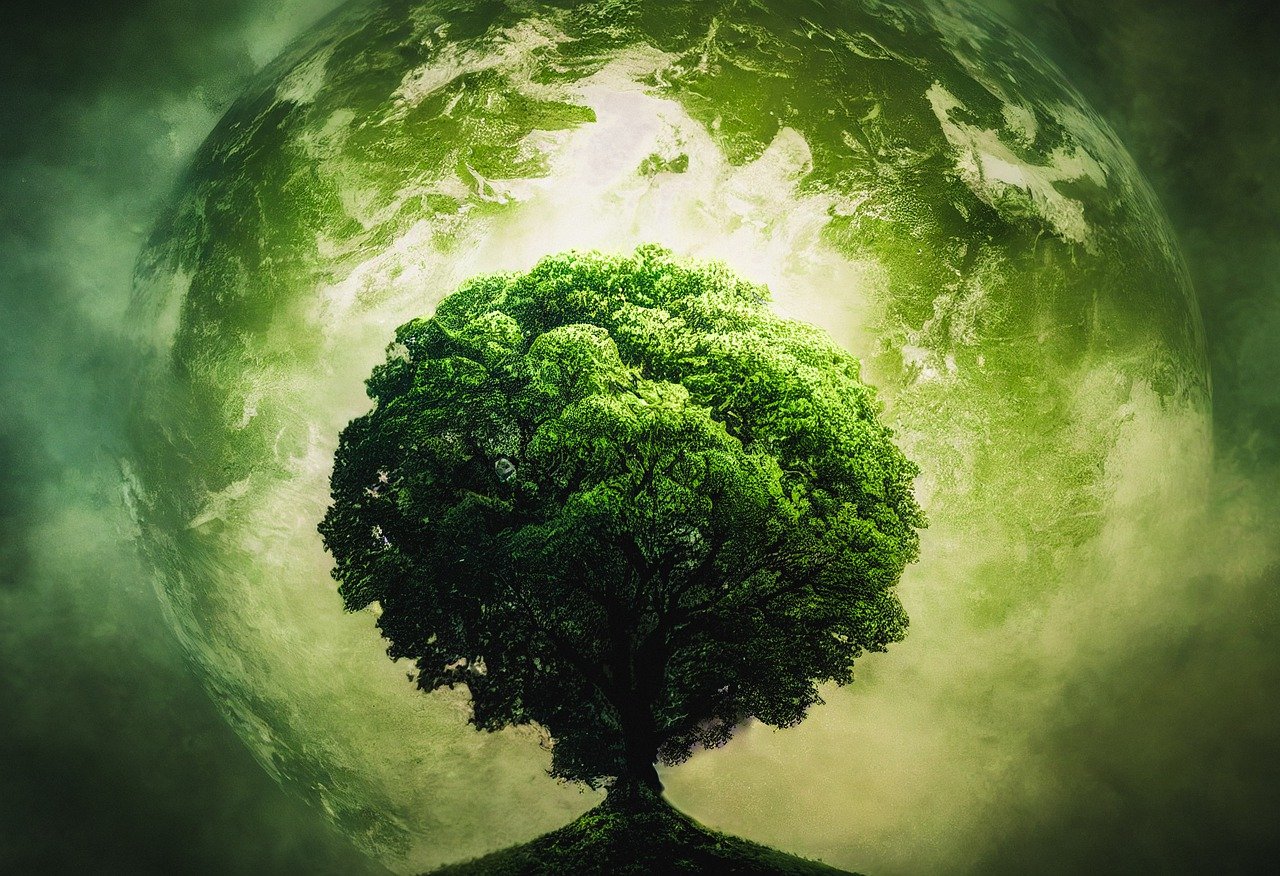
Corporate Responsibility
In today's fast-paced technological landscape, the role of corporations in ensuring ethical AI practices has never been more critical. As companies harness the power of artificial intelligence to enhance their products and services, they must also grapple with the ethical implications of their innovations. Corporate responsibility in AI is not just a buzzword; it is a fundamental aspect of sustainable business practices that can significantly impact society as a whole.
When we think about corporate responsibility, it often conjures images of companies donating to charity or engaging in environmentally friendly practices. However, in the context of AI, it goes much deeper. Corporations must take a proactive stance in addressing the ethical dilemmas that arise from AI development. This includes ensuring that their AI systems are free from bias, transparent in their decision-making processes, and accountable for their outcomes.
One of the key aspects of corporate responsibility in AI is the commitment to diversity and inclusion within their teams. A diverse workforce can bring a variety of perspectives that help identify potential biases in AI systems before they become problematic. For instance, when a team comprises individuals from different backgrounds, cultures, and experiences, they are more likely to recognize the nuances of how AI could affect various demographics. This recognition is crucial in mitigating risks associated with biased algorithms.
Moreover, corporations have a duty to engage in transparent communication with their stakeholders, including customers, employees, and the broader community. This transparency fosters trust and allows for a more informed dialogue about the implications of AI technologies. Companies can achieve this by publishing reports detailing their AI practices, the data sources they utilize, and how they ensure compliance with ethical standards. By doing so, they not only demonstrate accountability but also set a benchmark for others in the industry.
Additionally, it is essential for corporations to establish robust internal policies that prioritize ethical considerations throughout the AI development lifecycle. This can include implementing ethics training for employees, creating oversight committees, and conducting regular audits of AI systems to ensure they align with the company's ethical standards. By institutionalizing these practices, companies can create a culture of accountability that permeates their operations.
To illustrate the importance of corporate responsibility in AI, consider the following table that summarizes the key responsibilities that companies should uphold:
Responsibility | Description |
---|---|
Diversity and Inclusion | Fostering a diverse workforce to minimize biases in AI systems. |
Transparency | Communicating openly about AI practices and decision-making processes. |
Accountability | Establishing clear guidelines and policies to ensure ethical AI development. |
Continuous Improvement | Regularly reviewing and updating AI systems to align with evolving ethical standards. |
In conclusion, corporate responsibility in AI is not merely an ethical obligation; it is a strategic imperative that can drive long-term success and foster public trust. Companies that prioritize ethical AI practices will not only mitigate risks associated with bias and accountability but also enhance their brand reputation and customer loyalty. As we continue to navigate the complexities of AI, it is essential for corporations to lead by example, demonstrating that ethical considerations are at the forefront of technological advancement.
- What is corporate responsibility in AI? Corporate responsibility in AI refers to the ethical obligations that companies have to ensure their AI technologies are developed and implemented in a fair, transparent, and accountable manner.
- Why is diversity important in AI development? Diversity is important because it helps identify biases and ensures that AI systems are equitable and representative of all demographics.
- How can companies ensure transparency in their AI practices? Companies can ensure transparency by openly communicating their AI practices, publishing reports, and engaging with stakeholders about the implications of their technologies.
- What are some strategies for mitigating bias in AI? Strategies include using diverse datasets, implementing fairness algorithms, and conducting regular audits of AI systems to assess and address biases.

Transparency and Explainability
In the rapidly evolving world of artificial intelligence, transparency and explainability are not just buzzwords; they are essential pillars that support the ethical deployment of AI systems. Imagine driving a car with a complex navigation system that gives you directions but never explains why it chooses one route over another. Frustrating, right? This analogy highlights the importance of understanding AI decisions. If users cannot comprehend how AI systems arrive at their conclusions, it breeds mistrust, skepticism, and even fear, which can hinder the technology's acceptance and growth.
Transparency in AI refers to the clarity with which AI systems operate and make decisions. It involves making the inner workings of these systems visible and understandable to users. On the other hand, explainability goes a step further by ensuring that not only are the processes transparent, but users can also grasp the rationale behind specific outcomes. This is crucial for fostering a sense of trust and accountability in AI technologies.
Consider the following key aspects that highlight the significance of transparency and explainability in AI:
- Building Trust: When users can see and understand how AI systems function, they are more likely to trust the technology. This trust is vital for widespread adoption.
- Facilitating Ethical Decision-Making: Transparent AI systems allow stakeholders to make informed decisions, ensuring that ethical considerations are integrated into the development and deployment processes.
- Improving Accountability: When AI systems are transparent, it becomes easier to hold developers and organizations accountable for their actions and decisions.
To enhance transparency and explainability, developers can implement various techniques. For instance, creating detailed model documentation that outlines how an AI system works can provide valuable insights to users. Moreover, designing user-friendly interfaces helps clarify the decision-making processes of AI, making it easier for non-experts to understand. This is akin to providing a user manual for a complicated gadget; it demystifies the technology and empowers users.
In addition, employing visual aids such as flowcharts or decision trees can simplify complex AI processes, making them more accessible to the average person. By translating intricate algorithms into understandable formats, we can bridge the gap between technology and user comprehension. This is especially relevant in sectors like healthcare, finance, and law, where the stakes are high, and understanding AI decisions can significantly impact lives.
As we move forward, the demand for transparency and explainability in AI will only increase. Stakeholders—ranging from developers to end-users—must advocate for these principles to ensure that AI technologies serve humanity in an ethical and responsible manner. After all, in a world where machines increasingly influence our lives, understanding their workings is not just a luxury; it's a necessity.
- What is the difference between transparency and explainability in AI?
Transparency refers to how clearly AI systems operate, while explainability focuses on how well users understand the reasons behind AI decisions. - Why is transparency important in AI?
Transparency builds trust, facilitates ethical decision-making, and improves accountability among developers and organizations. - How can we enhance explainability in AI systems?
By implementing techniques such as model documentation, user-friendly interfaces, and visual aids like flowcharts and decision trees.
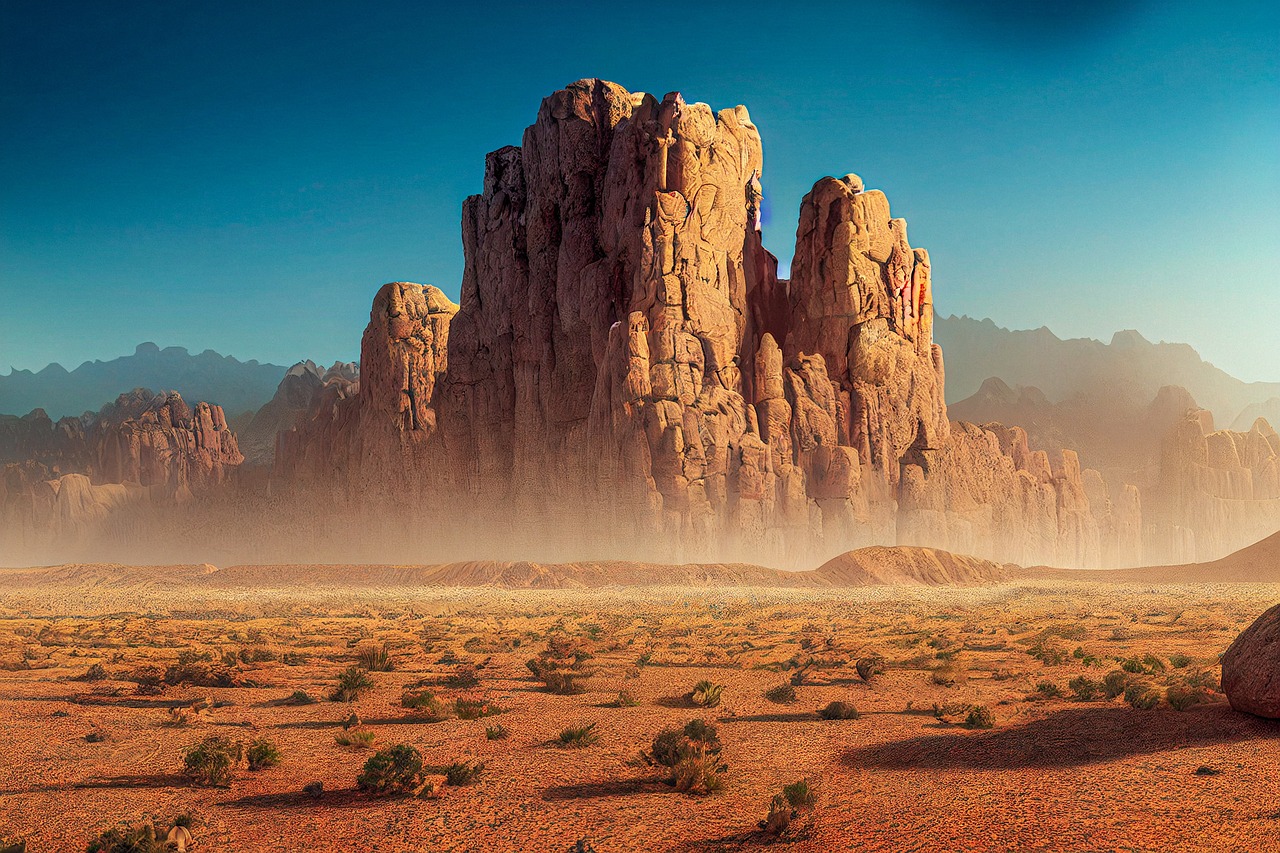
Importance of Explainable AI
In the rapidly evolving world of artificial intelligence, the concept of Explainable AI (XAI) has emerged as a critical focal point. As AI systems become more complex, understanding their decision-making processes becomes increasingly vital. Imagine driving a car that makes decisions on its own, like when to stop or accelerate, without any explanation. Wouldn’t that make you feel uneasy? This is precisely why explainability in AI is not just a technical requirement but a fundamental necessity for building trust between humans and machines.
One of the primary reasons for the importance of Explainable AI is the need for accountability. When AI systems make decisions that affect our lives—be it in healthcare, finance, or criminal justice—stakeholders demand to know how those decisions are reached. Without transparency, users are left in the dark, leading to skepticism and fear. For instance, if an AI algorithm denies a loan application, the applicant deserves to understand the reasoning behind such a decision. This clarity not only helps in building trust but also allows for better scrutiny of the AI’s functionality.
Moreover, explainable AI enhances user trust. When users comprehend how AI systems operate, they are more likely to engage with them. Think of it as a conversation between friends; when you understand each other, the relationship flourishes. Similarly, when AI systems are interpretable, users feel more comfortable relying on them. A study showed that when users were provided with explanations for AI decisions, their trust levels increased significantly. This trust is crucial as it leads to wider adoption of AI technologies across various sectors.
Furthermore, Explainable AI plays a significant role in identifying bias within algorithms. By understanding how decisions are made, developers can pinpoint areas where biases may exist and take corrective action. For example, if an AI system is found to favor one demographic over another, the ability to trace back through its decision-making process allows for a clearer path to rectifying these inequities. This is essential for ensuring that AI systems are fair and just, ultimately leading to more equitable outcomes for all users.
In addition to these benefits, the rise of Explainable AI also aligns with regulatory requirements. As governments and organizations begin to implement policies that demand transparency in AI, companies that prioritize explainability will not only comply with these regulations but also position themselves as leaders in ethical AI development. This proactive approach can lead to a competitive advantage in the marketplace.
In summary, the importance of Explainable AI cannot be overstated. It fosters accountability, builds user trust, helps in identifying biases, and ensures compliance with emerging regulations. As we continue to integrate AI into our daily lives, prioritizing explainability will be key to harnessing its full potential while safeguarding ethical standards.
- What is Explainable AI? Explainable AI refers to AI systems designed to provide clear insights into their decision-making processes, making it easier for users to understand how and why decisions are made.
- Why is Explainable AI important? It is crucial for accountability, user trust, bias identification, and regulatory compliance, ensuring AI systems operate fairly and transparently.
- How can Explainable AI benefit businesses? By fostering trust and transparency, businesses can enhance user engagement, improve decision-making processes, and comply with regulations, leading to a competitive advantage.
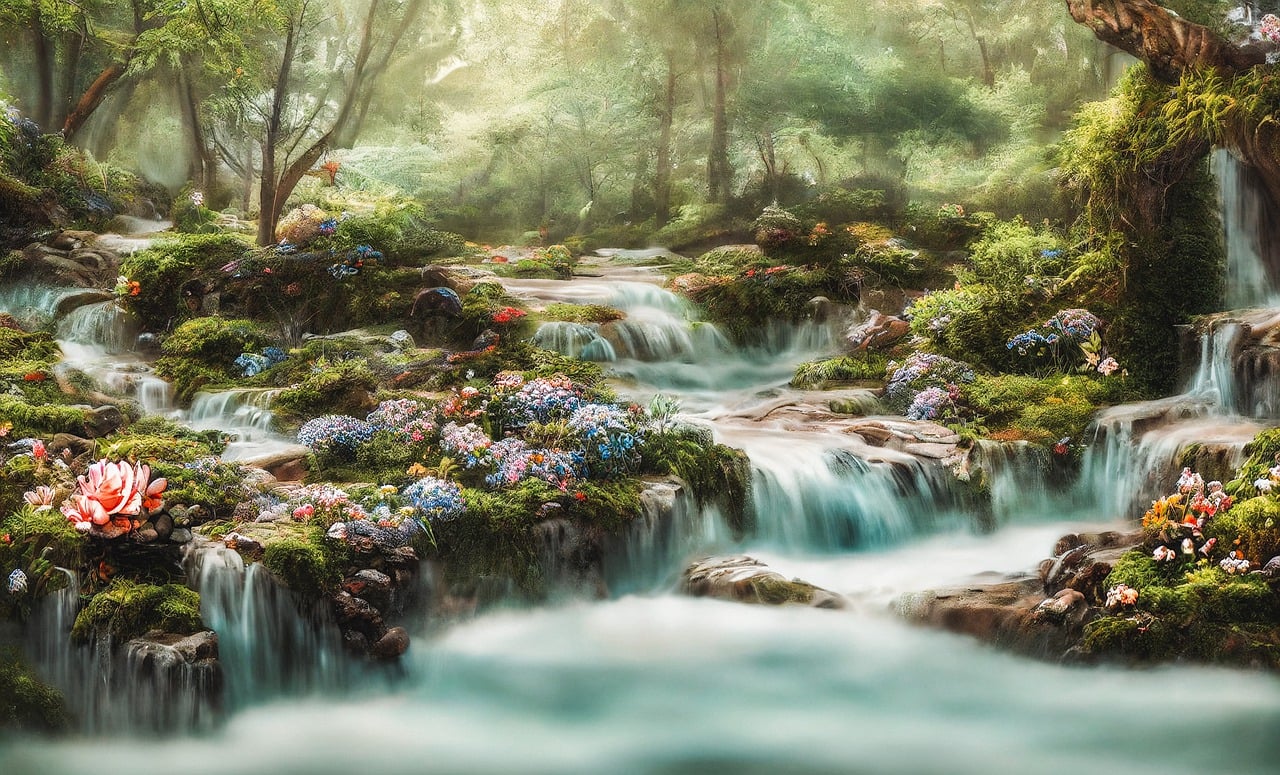
Techniques for Enhancing Transparency
In the rapidly evolving landscape of artificial intelligence, ensuring transparency is not just a best practice; it's a necessity. When we talk about transparency in AI, we're referring to the ability to understand how AI systems make decisions and the factors that influence those decisions. This understanding is crucial for building trust among users and stakeholders alike. One of the primary techniques for enhancing transparency is through the implementation of explainable AI (XAI). XAI aims to make AI systems more interpretable, allowing users to grasp the reasoning behind specific outcomes. Imagine trying to solve a puzzle without knowing what the picture looks like; it’s frustrating and often leads to mistrust. Similarly, when AI systems operate as black boxes, users are left in the dark.
Another effective technique is the use of model documentation. This involves creating comprehensive records that detail the design, development, and deployment processes of AI systems. By documenting the algorithms, data sources, and decision-making criteria, organizations can provide a clearer picture of how their AI operates. This is akin to having a roadmap for a road trip; it helps everyone understand the route taken and the stops made along the way. Moreover, such documentation can be invaluable during audits or assessments, ensuring that AI systems are held accountable for their actions.
Furthermore, user-friendly interfaces play a significant role in enhancing transparency. When AI systems are equipped with interfaces that communicate their processes in an easily digestible manner, users can better understand how decisions are made. For instance, consider a credit scoring AI that not only provides a score but also explains the factors that contributed to that score. This kind of transparency not only helps users feel more in control but also empowers them to make informed decisions based on AI outputs.
Lastly, continuous feedback mechanisms can also bolster transparency. By implementing systems that allow users to provide feedback on AI decisions, organizations can create a loop of accountability and improvement. This is similar to the way social media platforms evolve based on user input; when users feel heard, trust is built. In conclusion, enhancing transparency in AI is multifaceted, involving explainable AI, thorough documentation, user-friendly interfaces, and continuous feedback. As we advance in this field, adopting these techniques will be critical in fostering a culture of trust and accountability.
- What is explainable AI (XAI)?
XAI refers to AI systems designed to be interpretable, allowing users to understand how decisions are made.
- Why is transparency important in AI?
Transparency helps build trust among users and stakeholders, ensuring that AI systems are accountable for their decisions.
- How can organizations enhance transparency in AI?
Organizations can enhance transparency through explainable AI, thorough documentation, user-friendly interfaces, and continuous feedback mechanisms.
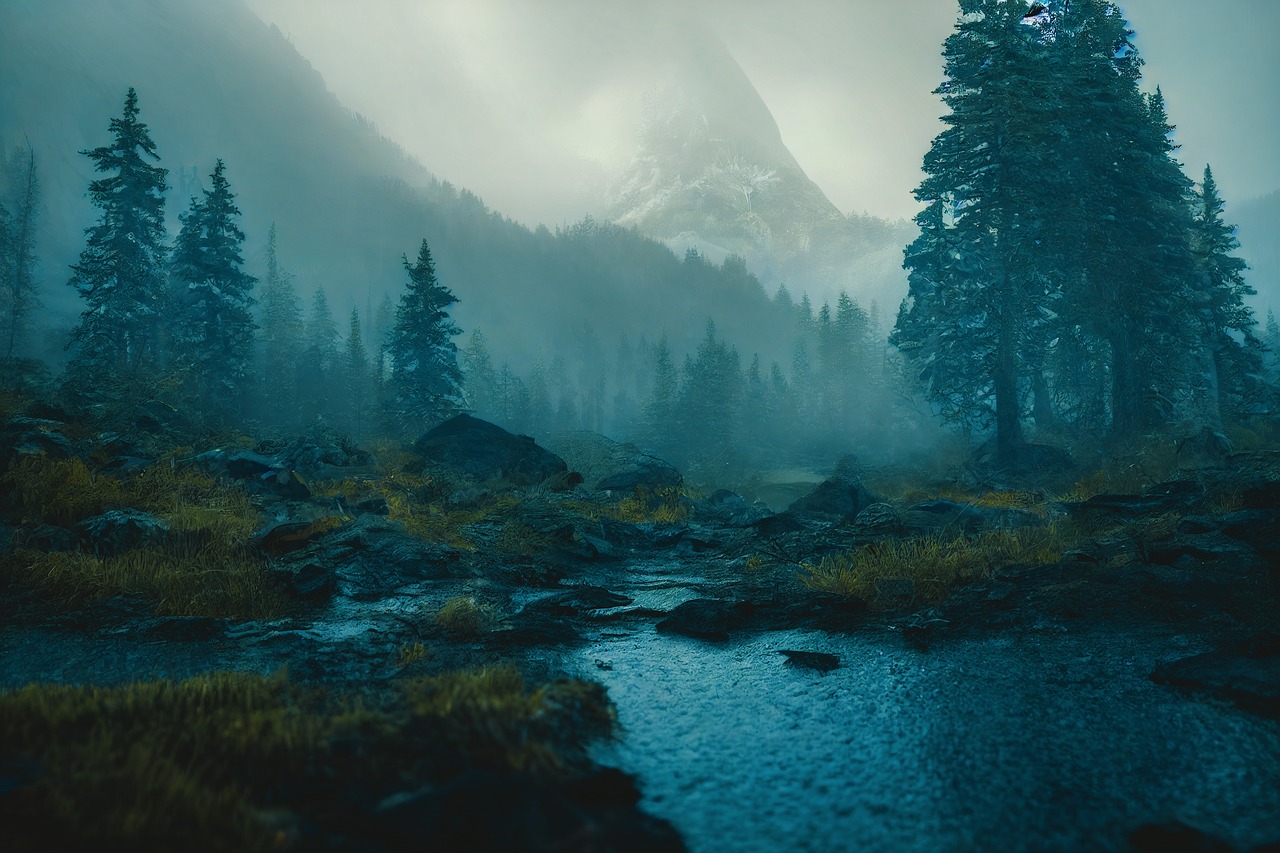
The Role of Policy in AI Ethics
The intersection of policy and AI ethics is a crucial aspect of ensuring that artificial intelligence technologies are developed and implemented responsibly. As AI continues to evolve at a breakneck pace, the policies that govern its use must also adapt to address the complex ethical challenges that arise. This is not just about creating rules; it’s about fostering a culture of ethical awareness among stakeholders, including governments, corporations, and the public. The need for comprehensive guidelines is more pressing than ever, as the implications of AI technology reach far beyond mere functionality.
One of the primary challenges in establishing effective AI policies is the rapidly changing landscape of technology. As new advancements emerge, they often outpace existing regulations, leaving gaps that can be exploited or lead to ethical dilemmas. For example, consider the rise of autonomous vehicles. Current traffic laws and liability frameworks may not adequately address the unique challenges posed by self-driving cars. This highlights the necessity for proactive policy-making that not only reacts to current developments but anticipates future needs.
Moreover, the role of international cooperation cannot be overstated. AI is a global phenomenon, and its ethical implications transcend borders. To create a cohesive framework for AI ethics, nations must collaborate to establish global standards. This can be achieved through international treaties and agreements that emphasize human rights and ethical considerations. By working together, countries can share best practices and create a unified approach to AI governance that respects diverse cultural values while promoting innovation.
In addition to international cooperation, local governments also play a vital role in shaping AI policies. They can tailor regulations to meet the specific needs of their communities, ensuring that the deployment of AI technologies aligns with local values and priorities. For instance, a city may implement regulations that require AI systems used in public services to undergo rigorous ethical reviews before deployment. This local approach can serve as a model for other regions, demonstrating how policy can effectively address the unique challenges posed by AI.
Furthermore, engaging various stakeholders in the policy-making process is essential for creating effective AI regulations. This includes not only policymakers but also technologists, ethicists, and the communities affected by AI technologies. By incorporating diverse perspectives, policies can be crafted that are more inclusive and reflective of societal values. Public consultations and forums can serve as platforms for dialogue, allowing citizens to voice their concerns and expectations regarding AI ethics.
To illustrate the potential impact of well-crafted AI policies, consider the following table that outlines key areas where policy can influence ethical AI development:
Policy Area | Impact on AI Ethics |
---|---|
Data Privacy | Ensures that user data is handled responsibly and ethically, protecting individual rights. |
Accountability | Establishes clear guidelines for who is responsible when AI systems cause harm. |
Transparency | Promotes open communication about AI decision-making processes, fostering trust. |
Bias Mitigation | Encourages the development of fair AI systems that do not discriminate against marginalized groups. |
In conclusion, the role of policy in AI ethics is multifaceted and indispensable. As we navigate the complexities of AI technology, it is crucial to establish robust policies that promote ethical standards while facilitating innovation. The future of AI will depend on our ability to create a balanced framework that considers the ethical implications of these powerful tools. By fostering collaboration among stakeholders and embracing a proactive approach to policy-making, we can ensure that AI serves the greater good of society.
- What is the importance of policy in AI ethics?
Policy provides a framework for the responsible development and use of AI technologies, ensuring that ethical considerations are prioritized. - How can international cooperation enhance AI ethics?
By collaborating on global standards, countries can share best practices and create unified guidelines that respect human rights. - What role do local governments play in AI policy?
Local governments can tailor regulations to meet the specific needs of their communities, ensuring AI aligns with local values. - Why is stakeholder engagement important in AI policy-making?
Engaging diverse stakeholders ensures that policies reflect a wide range of perspectives and address the concerns of all affected parties.
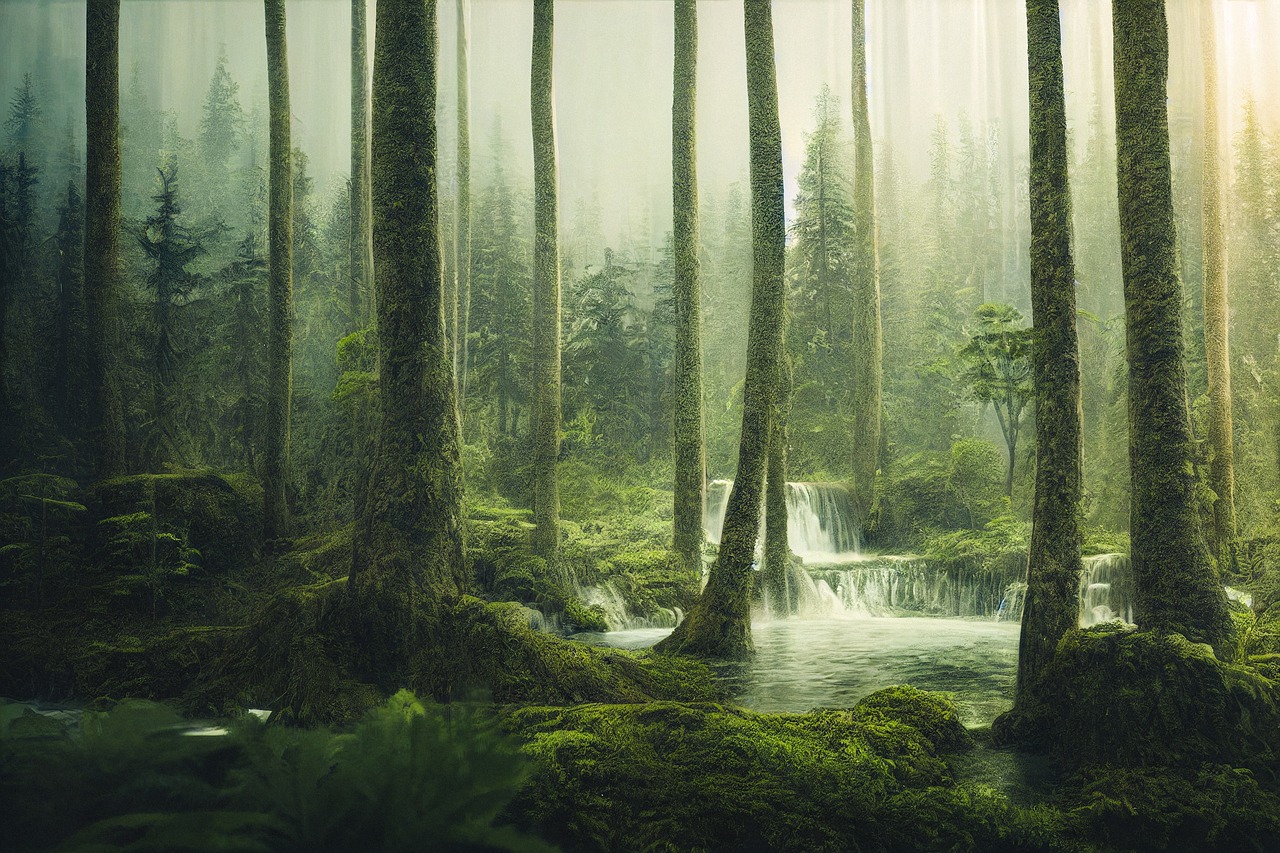
Global Standards for AI Ethics
The rapid evolution of artificial intelligence has ushered in a myriad of possibilities, but it has also raised significant ethical concerns that transcend national borders. As AI technologies integrate deeper into our lives, the pressing need for becomes increasingly evident. These standards serve as a guiding framework to ensure that AI development and deployment respect fundamental human rights and promote fairness, accountability, and transparency.
Establishing global standards is essential for several reasons. First, it helps to mitigate the risk of ethical fragmentation—where different countries or regions adopt conflicting ethical guidelines, leading to inconsistencies in AI practices. Such fragmentation can create a race to the bottom, where companies might prioritize profit over ethical considerations, potentially harming individuals and society as a whole.
Moreover, international cooperation in developing these standards can foster a sense of shared responsibility among nations, encouraging collaboration on ethical challenges posed by AI. This is particularly important in areas like data privacy, surveillance, and algorithmic bias, where the implications of AI technology can have far-reaching effects. By working together, countries can learn from one another’s experiences, successes, and failures, creating a more robust ethical framework.
To achieve effective global standards, several key principles should guide the development process:
- Inclusivity: Engaging diverse stakeholders—including governments, industry leaders, academia, and civil society—to ensure that various perspectives are considered.
- Adaptability: Recognizing that technology evolves rapidly, the standards must be flexible enough to accommodate new developments and ethical challenges.
- Transparency: Ensuring that the processes for developing these standards are open and accessible, fostering trust among stakeholders.
- Accountability: Establishing mechanisms to hold organizations and individuals accountable for unethical AI practices.
Many organizations and initiatives are already working towards creating these global standards. For instance, the IEEE Global Initiative on Ethics of Autonomous and Intelligent Systems aims to address ethical considerations in AI and autonomous systems by developing a comprehensive set of guidelines. Similarly, the European Union has proposed regulations that emphasize ethical AI, focusing on transparency, accountability, and risk management.
In conclusion, the establishment of global standards for AI ethics is not just a desirable goal; it is a necessity in our interconnected world. As we move forward, it is crucial that we prioritize these standards to ensure that AI technologies are developed and utilized in a manner that is ethical, equitable, and beneficial for everyone. The journey toward ethical AI is a collective effort, and by uniting our voices, we can shape a future where technology serves humanity responsibly.
- What are global standards for AI ethics?
Global standards for AI ethics are guidelines and principles designed to ensure that AI technologies are developed and used in ways that respect human rights and promote fairness, accountability, and transparency. - Why are global standards necessary?
They are necessary to prevent ethical fragmentation, foster international cooperation, and ensure consistent ethical practices across different countries and industries. - Who is involved in creating these standards?
Diverse stakeholders, including governments, industry leaders, academia, and civil society, should be involved to ensure a comprehensive approach to AI ethics. - How can I stay informed about AI ethics?
Staying informed can be achieved by following reputable organizations and initiatives focused on AI ethics, attending conferences, and engaging in discussions within your community.
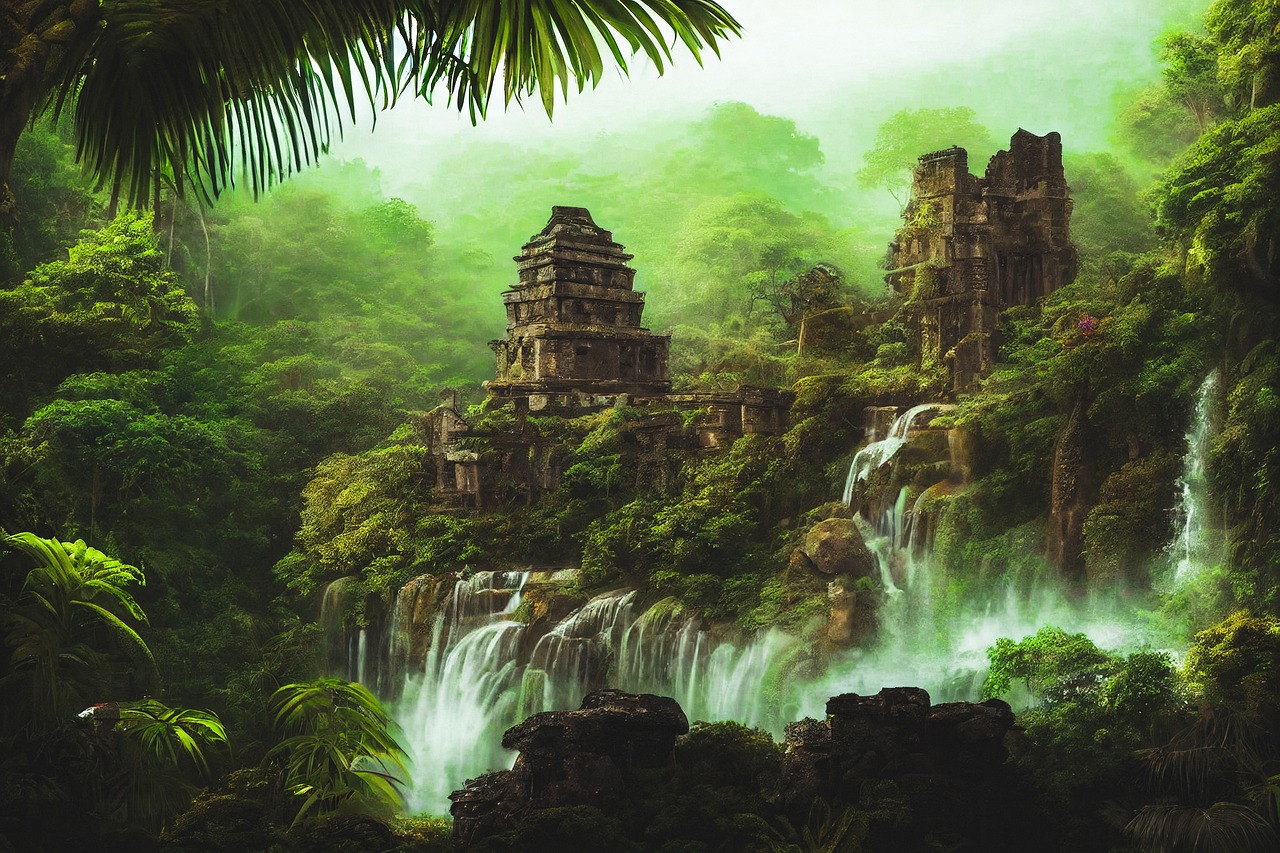
Policy Recommendations
As we stand at the crossroads of technological advancement and ethical responsibility, it becomes increasingly vital to establish clear and actionable policy recommendations that can guide the development and deployment of artificial intelligence systems. These recommendations should not only address current challenges but also anticipate future dilemmas that may arise as AI technologies continue to evolve. Here are several key recommendations that stakeholders should consider:
- Establish Comprehensive Regulatory Frameworks: Governments should work towards creating robust legal frameworks that specifically address the unique challenges posed by AI. This includes defining accountability measures and ensuring that there are consequences for unethical practices.
- Promote Transparency in AI Development: Organizations should be encouraged to adopt practices that enhance transparency. This can involve requiring companies to disclose their algorithms, data sources, and decision-making processes to foster trust among users.
- Encourage Diverse Data Collection: To combat bias, it is essential that AI developers prioritize the collection of diverse and representative datasets. Policies should incentivize organizations to invest in comprehensive data gathering that reflects the varied demographics of society.
- Implement Continuous Monitoring and Evaluation: AI systems should not be set and forgotten. Regular audits and evaluations should be mandated to assess the ethical implications of AI applications, ensuring they remain aligned with societal values over time.
- Foster Collaboration Across Sectors: Governments, academia, and industry leaders should collaborate to create interdisciplinary teams focused on ethical AI development. This collaboration can help bridge the gap between technological innovation and ethical considerations.
Moreover, it's imperative to engage the public in discussions about AI ethics. By fostering an environment where community voices are heard, policymakers can better understand societal concerns and aspirations regarding AI technologies. Public forums, workshops, and educational campaigns can serve as platforms for dialogue, ensuring that ethical considerations are not solely dictated by experts but are informed by the collective input of society.
Lastly, as new technologies emerge, the landscape of AI will undoubtedly shift. Therefore, policies should be adaptable and capable of evolving alongside technological advancements. This means establishing a framework for continuous ethical evaluation that can respond dynamically to the challenges posed by innovations like quantum computing and advanced machine learning.
In summary, the future of AI ethics hinges on the implementation of thoughtful, comprehensive policies that prioritize accountability, transparency, and public engagement. By taking these steps, we can navigate the complex terrain of AI development while ensuring that ethical considerations remain at the forefront of technological progress.
Q1: Why is it important to have policies for AI ethics?
A1: Policies for AI ethics are crucial because they provide a framework for accountability, transparency, and fairness in AI development. They help to mitigate risks associated with bias and ensure that AI technologies serve the public good.
Q2: How can organizations ensure their AI systems are ethical?
A2: Organizations can ensure ethical AI systems by adopting diverse data collection practices, conducting regular audits, and fostering a culture of transparency and accountability within their teams.
Q3: What role does public engagement play in AI policy development?
A3: Public engagement is vital as it allows policymakers to understand the concerns and values of society regarding AI. This input helps shape policies that reflect the needs and aspirations of the community.
Q4: How often should AI systems be evaluated for ethical compliance?
A4: AI systems should be evaluated regularly, ideally at set intervals or whenever significant updates are made to the system. Continuous monitoring is essential to adapt to new ethical challenges as technology evolves.
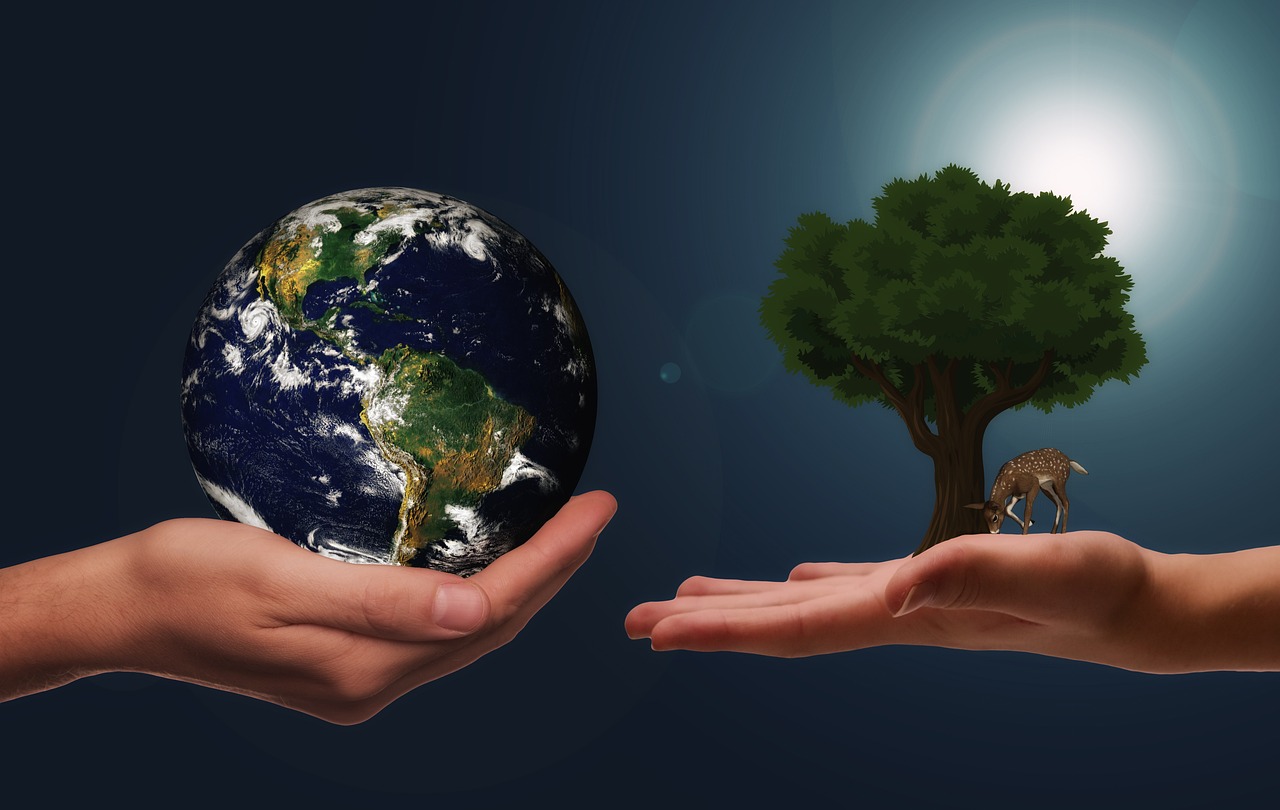
Future Implications of AI Ethics
The future of artificial intelligence (AI) is both thrilling and daunting, presenting a landscape filled with **unprecedented opportunities** and **complex ethical challenges**. As AI technologies continue to evolve at a breakneck pace, we find ourselves standing at a crossroads where ethical considerations are not just important—they are imperative. Imagine driving a car with no brakes; that’s how it feels navigating the future of AI without a robust ethical framework. What happens when these systems start making decisions that impact our daily lives? Who will be held accountable when things go wrong? These questions are not just hypothetical; they are the reality we must face as we forge ahead.
One of the most pressing issues is the emergence of **new technologies** such as quantum computing and advanced machine learning algorithms. These innovations could amplify existing ethical dilemmas or introduce new ones altogether. For instance, quantum computing has the potential to process vast amounts of data at lightning speed, which might lead to enhanced decision-making capabilities. However, this also raises concerns about privacy and surveillance, as more data becomes accessible and easier to analyze. Will these systems respect our privacy, or will they be used to monitor our every move? The ethical implications are profound and require careful consideration.
Moreover, as AI systems become more autonomous, the question of **continuous ethical evaluation** arises. Just as we wouldn’t set a ship to sail without a captain, we shouldn’t allow AI systems to operate independently without ongoing scrutiny. This means developing **adaptive ethical frameworks** that can evolve alongside technology. It’s not enough to create rules today; we must be prepared to revisit and revise them as AI continues to change the game. How do we ensure that these frameworks remain relevant and effective? This calls for a collaborative effort among technologists, ethicists, and policymakers.
In this rapidly changing environment, **international cooperation** becomes crucial. Establishing **global standards for AI ethics** is not just a lofty goal; it’s a necessity. Different countries have different regulations and ethical norms, which can lead to a patchwork of practices that may not adequately protect individuals' rights. Imagine a world where an AI system developed in one country operates in another with vastly different ethical standards—chaos could ensue. Therefore, a collective approach to creating comprehensive guidelines that respect human rights is essential.
As we look to the future, it’s essential to recognize that the conversation around AI ethics will not be static. It will require **ongoing dialogue** among various stakeholders. This includes government agencies, industry leaders, and civil society organizations. The more perspectives we include, the better we can understand the multifaceted implications of AI technologies. Together, we can explore the ethical landscape and ensure that AI serves humanity rather than undermines it.
In conclusion, the future implications of AI ethics are vast and complex. As we navigate this uncharted territory, we must remain vigilant and proactive. By fostering an environment of continuous ethical evaluation, promoting international cooperation, and engaging in open dialogue, we can harness the power of AI while safeguarding our values and rights. After all, the goal is not just to advance technology but to do so in a way that is fair, transparent, and beneficial for all.
- What are the main ethical concerns surrounding AI?
The primary concerns include bias, accountability, and transparency, which can significantly affect how AI systems operate and impact society. - How can we mitigate bias in AI?
Strategies include using diverse datasets, employing algorithmic fairness techniques, and continuously monitoring AI systems for equitable outcomes. - Why is international cooperation important in AI ethics?
Different countries have varying ethical standards, and cooperation helps establish global guidelines that protect human rights and promote fairness. - What role do policymakers play in AI ethics?
Policymakers are crucial in shaping regulations and guidelines that ensure ethical AI development and accountability. - How can organizations ensure ethical AI practices?
Organizations should adopt corporate social responsibility principles, engage in transparency, and commit to continuous ethical evaluation of their AI systems.
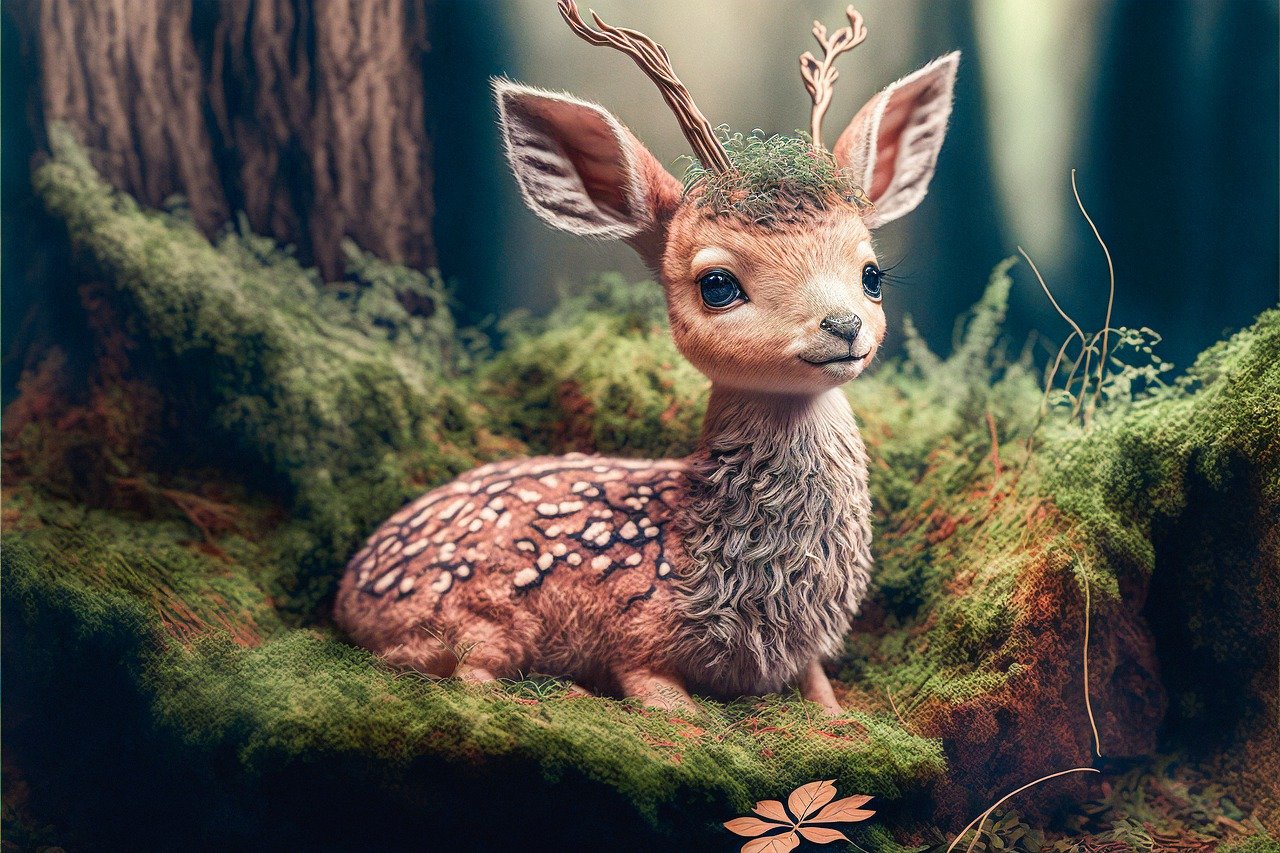
Emerging Technologies
As we stand on the brink of a technological revolution, the landscape of artificial intelligence (AI) is rapidly evolving, with paving the way for unprecedented advancements. These technologies, such as quantum computing, advanced machine learning, and natural language processing, are not just reshaping our understanding of AI but also raising critical ethical questions that society must address.
Quantum computing, for instance, promises to revolutionize the way we process information. Unlike traditional computers that use bits as the smallest unit of data, quantum computers utilize qubits, which can exist in multiple states simultaneously. This capability allows them to solve complex problems at speeds unimaginable with current technology. However, with such power comes the responsibility to ensure that these systems are developed ethically and used for the greater good, avoiding potential misuse that could deepen existing inequalities.
Similarly, advancements in machine learning are enabling AI systems to learn and adapt at an astounding rate. These systems can analyze vast amounts of data, uncovering patterns and insights that were previously inaccessible. While this can lead to remarkable innovations, such as personalized medicine and smarter cities, it also raises concerns about data privacy and the potential for misuse of sensitive information. The challenge lies in balancing innovation with the protection of individual rights.
Natural language processing (NLP) is another emerging technology that has transformed how we interact with machines. From virtual assistants to automated customer service, NLP allows machines to understand and respond to human language more effectively. However, this technology also presents ethical dilemmas, particularly regarding bias in language models. If not carefully managed, these biases can perpetuate stereotypes and discrimination, highlighting the need for ethical guidelines in the development and deployment of NLP systems.
To navigate the complexities of these emerging technologies, it is crucial for stakeholders—including governments, industries, and academia—to engage in ongoing dialogue and collaboration. Establishing global standards for ethical AI practices will be essential in addressing the challenges posed by these advancements. By prioritizing ethics in the development of emerging technologies, we can harness their potential while safeguarding against their risks.
In conclusion, as we embrace the future shaped by emerging technologies, we must remain vigilant about the ethical implications they carry. Continuous evaluation and adaptation of our ethical frameworks will be necessary to ensure that these powerful tools are used responsibly and equitably, ultimately benefiting all of society.
- What are the main ethical concerns surrounding emerging technologies in AI?
The primary concerns include data privacy, bias in algorithms, accountability for AI decisions, and the potential for misuse of powerful technologies. - How can we ensure that emerging technologies are developed ethically?
Establishing global standards, fostering collaboration among stakeholders, and continuously evaluating ethical frameworks are crucial steps in ensuring ethical development. - What role does government play in regulating emerging technologies?
Governments can create regulations and guidelines that promote ethical practices, protect individual rights, and ensure accountability in AI systems.
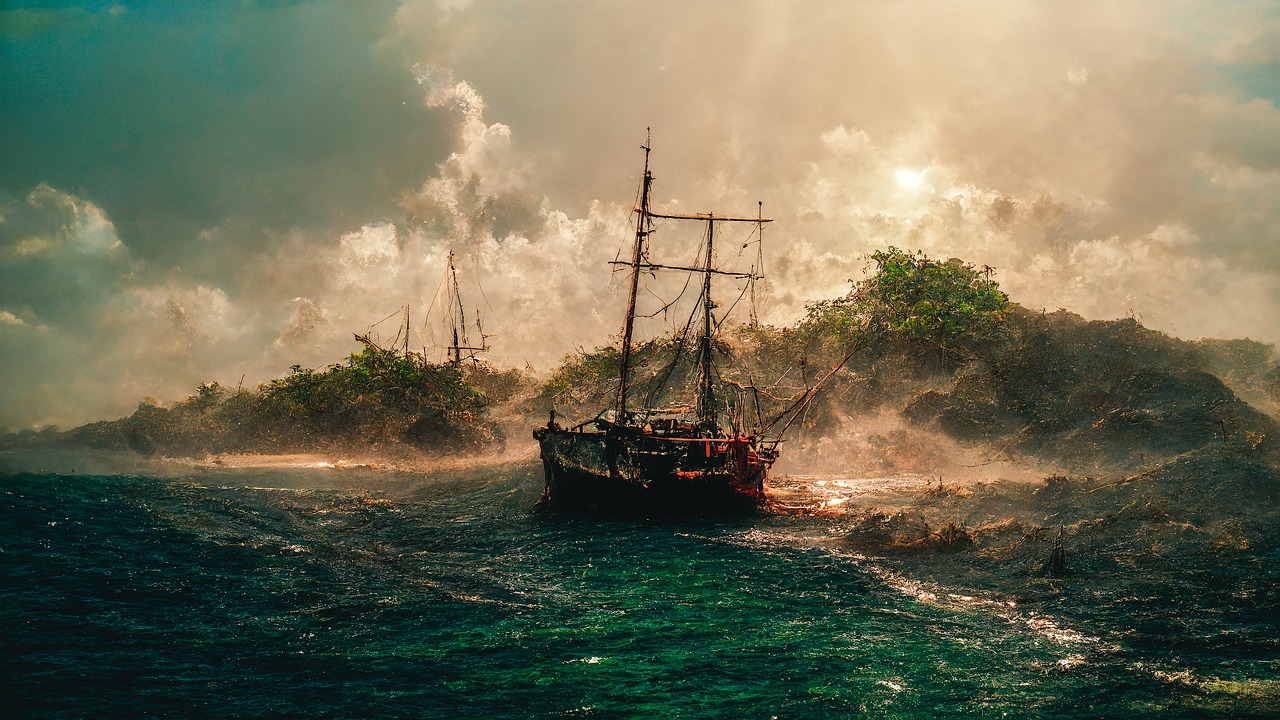
Continuous Ethical Evaluation
In the fast-paced world of artificial intelligence, where algorithms learn and evolve at lightning speed, the concept of becomes not just important, but essential. Imagine trying to navigate a ship through ever-changing waters; without constant adjustments and checks, you're bound to hit an iceberg at some point. Similarly, AI systems can drift into murky ethical waters if not regularly assessed against evolving societal norms and values.
As we integrate AI deeper into our daily lives—from autonomous vehicles to healthcare diagnostics—it’s crucial to implement ongoing assessments to ensure these technologies align with our ethical standards. This means that ethical evaluations should not be a one-time event but an ongoing process that adapts as technologies advance. Here are some key reasons why continuous ethical evaluation is vital:
- Adaptability: As AI technologies evolve, so too do their implications. Continuous evaluation allows us to adapt our ethical frameworks to new challenges.
- Accountability: Regular assessments help ensure that developers and organizations remain accountable for the impacts of their AI systems.
- Public Trust: Keeping the public informed about the ethical status of AI can foster trust and acceptance among users.
To effectively implement continuous ethical evaluation, organizations can adopt several strategies. First, they can establish multidisciplinary teams that include ethicists, technologists, and community representatives. This diverse group can provide a well-rounded perspective on the implications of AI systems. Additionally, organizations should create feedback loops where users can report concerns or experiences related to AI behavior, ensuring that real-world impacts are considered in ongoing evaluations.
Moreover, the use of auditing frameworks can help in assessing AI systems regularly. These frameworks can include metrics for fairness, accountability, and transparency, allowing organizations to benchmark their AI technologies against established ethical standards. Just like a car needs regular maintenance checks to ensure it runs smoothly, AI systems require similar scrutiny to prevent ethical mishaps.
In conclusion, as we continue to embrace the benefits of AI, we must also commit to a proactive approach in evaluating its ethical implications. Continuous ethical evaluation is not merely a safeguard; it is a pathway to ensuring that AI technologies serve humanity positively and equitably. By fostering an environment of ongoing scrutiny and adaptation, we can navigate the complexities of AI ethics and build a future where technology and ethics go hand in hand.
- What is continuous ethical evaluation in AI?
Continuous ethical evaluation refers to the ongoing assessment of AI systems to ensure they align with evolving ethical standards and societal values. - Why is it important?
It is crucial to adapt to new challenges, ensure accountability, and build public trust in AI technologies. - How can organizations implement it?
Organizations can form multidisciplinary teams, establish feedback loops, and utilize auditing frameworks to regularly assess their AI systems. - What are the consequences of neglecting ethical evaluation?
Neglecting ethical evaluation can lead to biases, unfair outcomes, and a loss of public trust in AI technologies.
Frequently Asked Questions
- What are the main ethical dilemmas associated with AI?
The primary ethical dilemmas of AI include issues like bias, accountability, and transparency. These challenges highlight the urgent need for a robust ethical framework to guide the development and implementation of AI technologies, ensuring they serve humanity responsibly.
- How does bias affect AI algorithms?
Bias in AI algorithms can lead to unfair and discriminatory outcomes. This often stems from biased historical data or societal prejudices that influence AI decision-making processes. Addressing these biases is crucial for creating equitable technology that benefits all users.
- What are the sources of bias in AI?
Sources of bias in AI can include historical data that reflects societal inequalities, as well as the data collection practices that may not capture diverse perspectives. Additionally, human influence during the design and training phases can inadvertently introduce bias into AI systems.
- What strategies can mitigate bias in AI?
To mitigate bias in AI, it is essential to implement algorithmic fairness techniques, utilize diverse and representative datasets, and continuously monitor AI systems for equitable outcomes. These strategies help ensure that AI technologies are fair and just for all users.
- Who is accountable when AI systems cause harm?
Accountability in AI development is a complex issue. It raises questions about who is responsible—be it the developers, the corporations, or the users—when AI systems cause harm. Clear guidelines and regulations are necessary to address these accountability challenges effectively.
- Why is transparency important in AI?
Transparency is vital in AI because it fosters trust and understanding among users. When AI systems are explainable and their decision-making processes are clear, users can make informed choices and feel more confident in the technology they are using.
- What role does policy play in AI ethics?
Policy plays a crucial role in shaping ethical AI practices. Collaborative efforts between governments, industry, and academia are essential to establish comprehensive guidelines that promote responsible AI development and ensure accountability.
- What are some future implications of AI ethics?
The future implications of AI ethics include the need for ongoing dialogue and adaptation as technologies evolve. Emerging technologies like quantum computing may introduce new ethical challenges, necessitating continuous ethical evaluation of AI systems to keep pace with advancements.