Bridging the AI Skills Gap for the Future Workforce
In today’s rapidly changing job market, the importance of artificial intelligence (AI) cannot be overstated. As industries evolve, the demand for skilled professionals who can navigate the complexities of AI technologies is skyrocketing. Yet, despite this growing need, a significant skills gap exists, leaving many organizations struggling to find qualified talent. Bridging this gap is not just a necessity; it's a crucial step toward ensuring that our workforce is prepared for the future. So, what does it mean to bridge the AI skills gap? It means equipping individuals with the tools, knowledge, and hands-on experience they need to thrive in an AI-driven economy. This article explores the strategies, challenges, and the pivotal role of education in this transformative journey.
Understanding the significance of AI skills is essential for both job seekers and employers. Think of AI as the new electricity; just as electricity transformed industries over a century ago, AI is reshaping how we work today. From healthcare to finance, AI competencies are increasingly becoming a prerequisite in various sectors. Employers are not just looking for candidates who can perform tasks; they want individuals who can leverage AI to drive innovation and efficiency. For job seekers, possessing AI skills can be a game changer, opening doors to numerous opportunities and providing a competitive edge in the job market. As a result, those who invest in learning AI technologies are likely to see substantial returns on that investment.
Analyzing the current landscape reveals a significant skills gap in AI. Many educational institutions and training programs have not yet adapted to the fast-paced advancements in AI technologies. This gap impacts workforce readiness, leaving employers frustrated with the lack of qualified candidates. Key areas where training and education are lacking include practical experience with machine learning, understanding data ethics, and proficiency in programming languages like Python and R. To truly bridge this gap, a comprehensive understanding of these areas is essential.
Diving deeper, let’s explore the specific competencies needed in AI. These include:
- Machine Learning: Understanding algorithms that allow computers to learn from and make predictions based on data.
- Data Analysis: The ability to interpret complex data sets and derive actionable insights.
- Programming Languages: Proficiency in languages such as Python, R, and Java, which are crucial for developing AI applications.
Let’s take a moment to unpack machine learning. At its core, machine learning is about teaching computers to learn from data. Imagine teaching a child to recognize animals by showing them pictures; similarly, machine learning algorithms analyze data and improve their accuracy over time. This foundational skill is critical for professionals in technology and data-driven fields, as it enables them to create systems that can make decisions with minimal human intervention.
Data analysis techniques are equally important in the realm of AI. Businesses today are inundated with data, and the ability to sift through this information to find meaningful patterns is invaluable. Techniques such as regression analysis, clustering, and data visualization play a crucial role in informed decision-making. By mastering these techniques, professionals can help organizations harness the power of their data, driving better outcomes and fostering innovation.
The world of AI is constantly evolving, with new technologies and trends emerging at a rapid pace. Staying updated with these changes is vital for workforce development. For instance, advancements in natural language processing and computer vision are revolutionizing how we interact with machines. Professionals who keep their skills current and embrace these innovations will find themselves at the forefront of their industries, ready to tackle new challenges head-on.
So, how can we effectively bridge the AI skills gap? The answer lies in actionable strategies that individuals and organizations can implement.
Focusing on the importance of upskilling and reskilling, various programs and initiatives are aimed at enhancing AI capabilities among existing employees. Organizations can offer training sessions, workshops, and online courses that focus on the latest AI trends and technologies. This not only benefits the employees but also enhances the overall productivity of the organization.
Highlighting the need for partnerships between educational institutions and industry leaders is crucial. Collaborative efforts can better prepare students for AI careers by aligning curricula with real-world needs. Internship programs, guest lectures from industry experts, and hands-on projects can provide students with invaluable experience, making them job-ready upon graduation.
In an ever-evolving AI landscape, continuous learning is crucial. The importance of lifelong learning and professional development cannot be overstated. As technologies change, professionals must adapt and grow their skill sets to remain relevant. Whether through online courses, workshops, or industry conferences, committing to continuous education is the key to success in the AI field.
- What is the AI skills gap? The AI skills gap refers to the disparity between the demand for AI skills in the job market and the available talent equipped with those skills.
- How can I improve my AI skills? You can improve your AI skills through online courses, workshops, and practical experience with AI technologies and tools.
- Why is continuous learning important in AI? Continuous learning is essential in AI as the field is constantly evolving, and staying updated with the latest technologies and trends is crucial for career success.
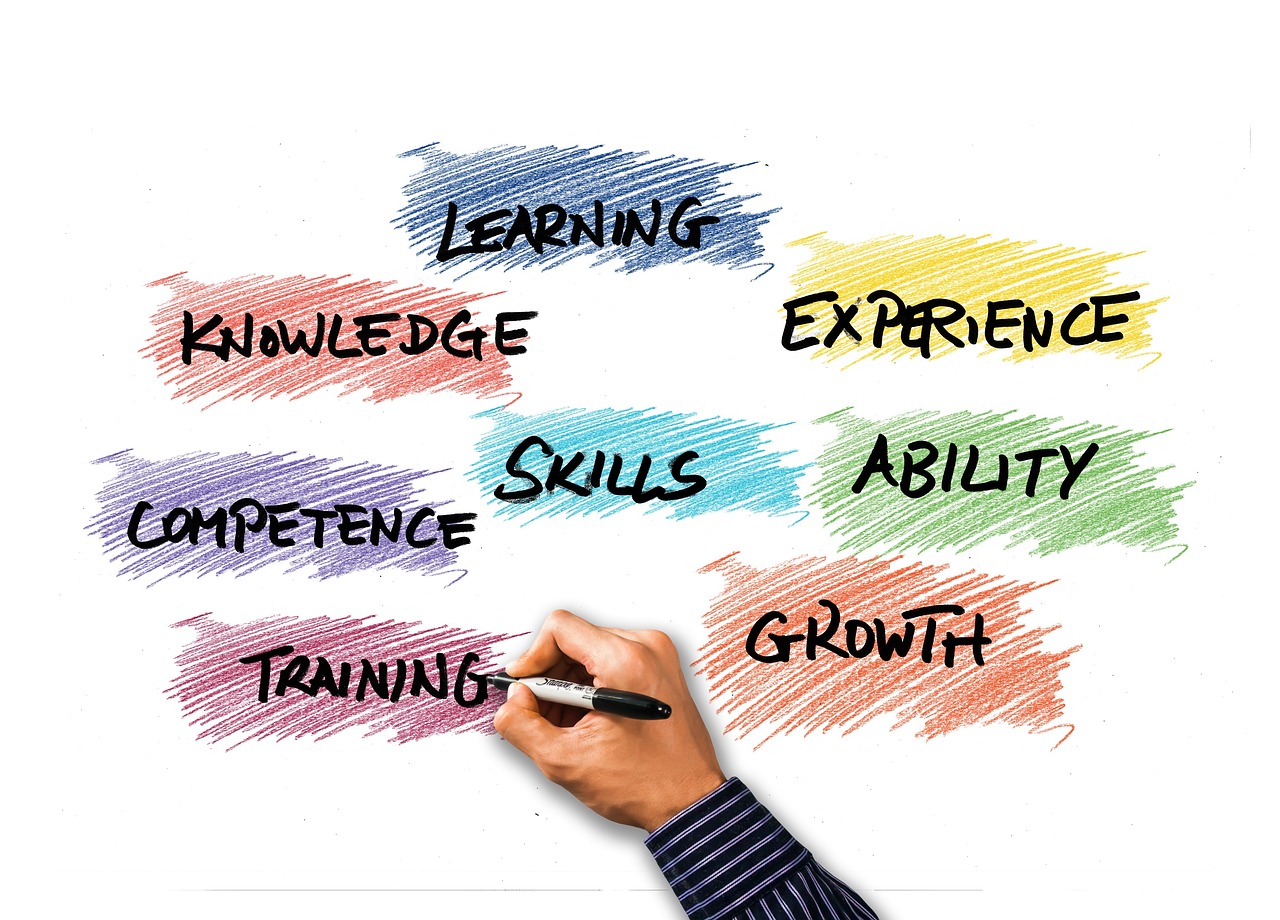
The Importance of AI Skills in Today's Job Market
In today's rapidly evolving job market, the significance of AI skills cannot be overstated. As industries across the globe increasingly integrate artificial intelligence into their operations, the demand for professionals equipped with AI competencies is skyrocketing. Imagine walking into a job interview where the employer asks you about your experience with AI technologies—how would you respond if you didn’t have the skills to back it up? That's the reality many job seekers face today.
Employers are not just looking for traditional qualifications; they are actively seeking candidates who can leverage AI to drive innovation and efficiency. In fact, a recent study revealed that over 70% of companies consider AI skills as a critical factor when hiring. This paradigm shift means that if you want to stand out in the crowded job market, acquiring AI skills is not just an option—it’s a necessity.
Let’s break it down a bit. AI skills are becoming prerequisites in various sectors, including but not limited to:
- Healthcare: AI is revolutionizing patient care, from predictive analytics to personalized medicine.
- Finance: Algorithms are used for risk assessment and fraud detection, making AI expertise invaluable.
- Manufacturing: Automation and robotics are heavily reliant on AI technologies for efficiency.
- Marketing: Understanding consumer behavior through AI-driven data analysis is essential for targeted campaigns.
As you can see, the integration of AI is not limited to tech companies; it spans across various industries, making AI literacy a vital asset. Moreover, the World Economic Forum predicts that by 2025, 85 million jobs may be displaced due to the shift in labor between humans and machines, while 97 million new roles could emerge that are more adapted to the new division of labor. This means that those who possess AI skills will be at a significant advantage in the job market.
Furthermore, organizations that prioritize AI skills in their workforce can expect to see enhanced productivity and innovation. Companies that invest in AI training for their employees often experience a substantial return on investment, as skilled workers can implement AI solutions that streamline processes and improve decision-making. It’s a win-win situation—employees gain valuable skills, and employers benefit from a more competent and efficient workforce.
In conclusion, as the job market continues to evolve, the importance of AI skills will only increase. Whether you’re a recent graduate, a seasoned professional, or someone looking to pivot careers, embracing AI education and training is crucial for staying relevant and competitive. The future belongs to those who adapt, and in this case, adapting means mastering the skills that will drive the next wave of innovation.

Identifying the Skills Gap in AI
In the rapidly evolving world of technology, the skills gap in artificial intelligence (AI) has become a pressing concern for both employers and employees. As organizations increasingly rely on AI to drive innovation and efficiency, the demand for skilled professionals who can navigate this complex landscape is skyrocketing. However, many industries are facing a significant shortage of qualified candidates, leading to a mismatch between job requirements and available talent. This gap not only hinders organizational growth but also limits career opportunities for individuals.
To truly understand the skills gap in AI, we must first analyze the current landscape. A recent survey indicated that over 60% of companies reported difficulty in finding candidates with the necessary AI skills. This statistic paints a clear picture of the urgency required to address this issue. The skills that are often lacking include a deep understanding of machine learning algorithms, data analysis techniques, and proficiency in programming languages such as Python and R. Without these competencies, professionals struggle to meet the demands of their roles, resulting in a workforce that is not fully equipped for the AI-driven future.
Moreover, the skills gap is not just a matter of technical knowledge; it also encompasses soft skills that are crucial for success in AI-related fields. Skills such as critical thinking, problem-solving, and effective communication are essential for collaborating on AI projects. Unfortunately, many educational programs have yet to incorporate these skills into their curricula, leaving graduates ill-prepared for the workplace. This disconnect between education and industry needs exacerbates the skills gap and highlights the necessity for a more integrated approach to workforce training.
Furthermore, let's take a closer look at some of the key areas where training and education are lacking:
- Machine Learning: Many professionals have a basic understanding of AI concepts but lack hands-on experience with machine learning frameworks.
- Data Management: The ability to collect, manage, and analyze large data sets is critical, yet many lack proficiency in tools like SQL and data visualization software.
- Programming Skills: While some candidates may have theoretical knowledge, practical programming skills are often underdeveloped.
Addressing the skills gap in AI requires a multifaceted approach. Educational institutions must collaborate with industry leaders to ensure that their programs align with current market demands. Additionally, organizations should invest in training and development initiatives to upskill their existing workforce. By identifying these gaps and implementing targeted strategies, we can bridge the divide and prepare a future workforce that is ready to tackle the challenges of an AI-driven world.
Q1: What is the AI skills gap?
The AI skills gap refers to the disparity between the demand for skilled AI professionals and the supply of qualified candidates in the job market.
Q2: Why is it important to address the AI skills gap?
Addressing the AI skills gap is crucial for organizational success, innovation, and economic growth. A skilled workforce can leverage AI technologies to improve efficiency and drive business results.
Q3: What are some key competencies needed in AI?
Key competencies include machine learning, data analysis, programming languages (like Python and R), and essential soft skills such as problem-solving and communication.
Q4: How can individuals improve their AI skills?
Individuals can improve their AI skills through online courses, certifications, workshops, and hands-on projects that provide practical experience and knowledge.
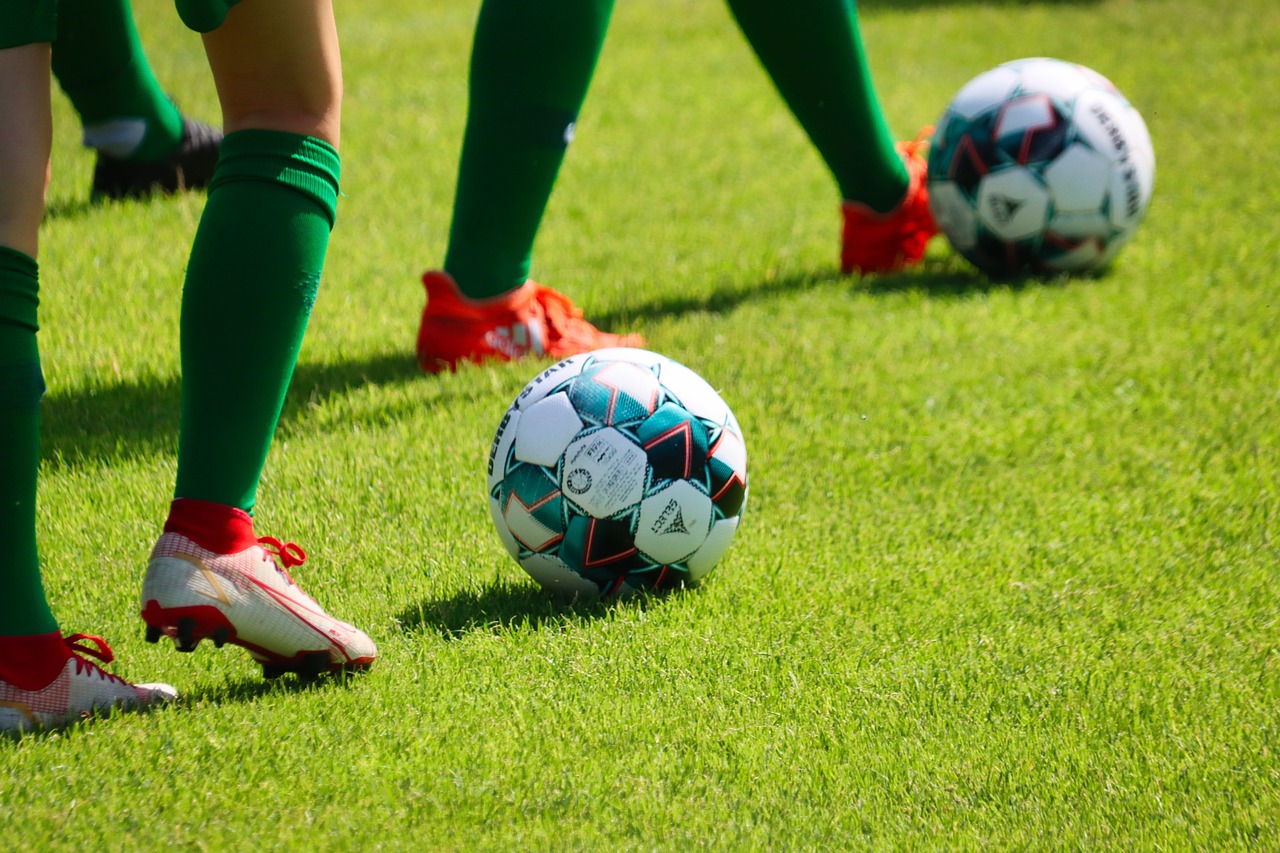
Key AI Competencies Required
In today's rapidly evolving job market, possessing strong AI competencies is not just advantageous; it's becoming essential. As industries integrate artificial intelligence into their operations, the demand for professionals equipped with specific skills is skyrocketing. So, what are these key competencies that can set you apart in the crowded job landscape? Let's dive into the core areas that are critical for success in the AI domain.
First and foremost, machine learning stands out as a foundational skill. It’s the engine that drives many AI applications, enabling systems to learn from data and improve their performance over time. Understanding the principles of machine learning, such as supervised and unsupervised learning, is crucial. For instance, professionals should be familiar with algorithms like decision trees, neural networks, and support vector machines. But it's not just about knowing the theory; practical experience in implementing these algorithms using programming languages like Python or R is equally important.
Next, let’s talk about data analysis. In the world of AI, data is the new oil. The ability to analyze and interpret vast amounts of data is vital for making informed decisions that can propel a business forward. Professionals should be adept at using statistical tools and techniques to extract meaningful insights from data sets. This includes understanding concepts such as regression analysis, data visualization, and hypothesis testing. The more proficient one is in data analysis, the better equipped they will be to contribute to AI-driven projects.
Additionally, programming skills are indispensable in the AI toolkit. Knowledge of programming languages such as Python, Java, and C++ allows professionals to build and refine AI models. Python, in particular, has gained immense popularity due to its simplicity and the vast array of libraries available for machine learning, such as TensorFlow and scikit-learn. Being comfortable with coding not only enhances problem-solving capabilities but also fosters innovation in developing new AI applications.
Moreover, familiarity with cloud computing is becoming increasingly important. As businesses shift towards cloud-based solutions, understanding platforms like AWS, Google Cloud, or Azure can provide a significant edge. These platforms often host AI tools and resources that facilitate large-scale data processing and model deployment. Knowing how to leverage these services can streamline workflows and enhance productivity.
Lastly, having a grasp of ethics in AI is emerging as a critical competency. As AI systems are deployed in sensitive areas such as healthcare, finance, and law enforcement, understanding the ethical implications of AI decisions is vital. Professionals should be aware of issues like bias in algorithms, data privacy concerns, and the societal impacts of AI technologies. This knowledge not only fosters responsible AI development but also builds trust with stakeholders and the public.
In summary, bridging the AI skills gap requires a multifaceted approach to skill development. By focusing on machine learning, data analysis, programming, cloud computing, and ethics, individuals can position themselves as valuable assets in the AI-driven workforce. As the landscape continues to evolve, staying ahead of these competencies will not just be an advantage; it will be a necessity.
- What is machine learning? Machine learning is a subset of AI that enables systems to learn from data and improve their performance without being explicitly programmed.
- Why is data analysis important in AI? Data analysis allows professionals to extract insights from data, which is crucial for making informed decisions in AI applications.
- Which programming languages are best for AI? Python, Java, and C++ are widely recognized as the most effective programming languages for AI development.
- How does cloud computing relate to AI? Cloud computing provides the infrastructure and tools necessary for deploying and scaling AI applications, making it easier to process large datasets.
- What are the ethical considerations in AI? Ethical considerations include bias in algorithms, data privacy, and the societal impacts of AI technologies.

Machine Learning Basics
Machine learning is not just a buzzword; it’s a transformative force that’s reshaping industries and redefining the way we interact with technology. At its core, machine learning is a subset of artificial intelligence (AI) that empowers systems to learn from data, identify patterns, and make decisions with minimal human intervention. Imagine teaching a child to recognize different animals by showing them pictures; similarly, machine learning algorithms learn from vast amounts of data to make predictions or decisions based on new inputs.
Understanding the fundamentals of machine learning is crucial for anyone looking to thrive in today’s tech-driven job market. It’s like learning the grammar of a new language; once you grasp the basics, you can communicate more effectively. The process typically involves three key stages: data collection, model training, and evaluation. In the data collection phase, vast datasets are gathered, which serve as the foundation for training the model. The model training phase is where the magic happens; algorithms analyze the data, learning to make predictions or classifications. Finally, in the evaluation phase, the model is tested against unseen data to assess its accuracy and effectiveness.
There are various types of machine learning techniques, each suited for different tasks. The primary categories include:
- Supervised Learning: This approach involves training a model on labeled data, allowing it to learn the relationship between input features and the corresponding output. Think of it as a teacher guiding a student.
- Unsupervised Learning: Here, the model works with unlabeled data, identifying hidden patterns or intrinsic structures. It’s akin to exploring a new city without a map, discovering interesting places along the way.
- Reinforcement Learning: This method teaches models to make decisions by rewarding desired behaviors and penalizing undesired ones. It’s similar to training a pet; consistent rewards encourage good behavior.
Machine learning has a plethora of applications across various sectors. In healthcare, it’s revolutionizing diagnostics and personalized treatment plans. In finance, it’s enhancing fraud detection and risk assessment. Retailers leverage machine learning for personalized recommendations, while manufacturers use it for predictive maintenance. As you can see, mastering machine learning basics is not merely beneficial; it’s becoming essential in navigating the future workforce.
In conclusion, grasping the basics of machine learning opens doors to a world of opportunities. It’s not just about understanding algorithms; it’s about harnessing the power of data to drive innovation and efficiency. As we continue to advance into an AI-driven future, those equipped with machine learning skills will undoubtedly stand out in the job market.
Q1: What is machine learning?
A1: Machine learning is a branch of artificial intelligence that enables systems to learn from data and improve their performance over time without being explicitly programmed.
Q2: How is machine learning used in everyday life?
A2: Machine learning is used in various applications, including recommendation systems (like Netflix and Amazon), voice recognition (like Siri and Alexa), and even in self-driving cars.
Q3: Do I need a background in programming to learn machine learning?
A3: While having programming knowledge can be beneficial, many resources and courses are designed for beginners that do not require extensive programming skills. It's more about understanding the concepts.
Q4: What are the best resources to learn machine learning?
A4: There are numerous online platforms like Coursera, edX, and Udacity that offer courses in machine learning. Additionally, books and tutorials can provide a solid foundation.
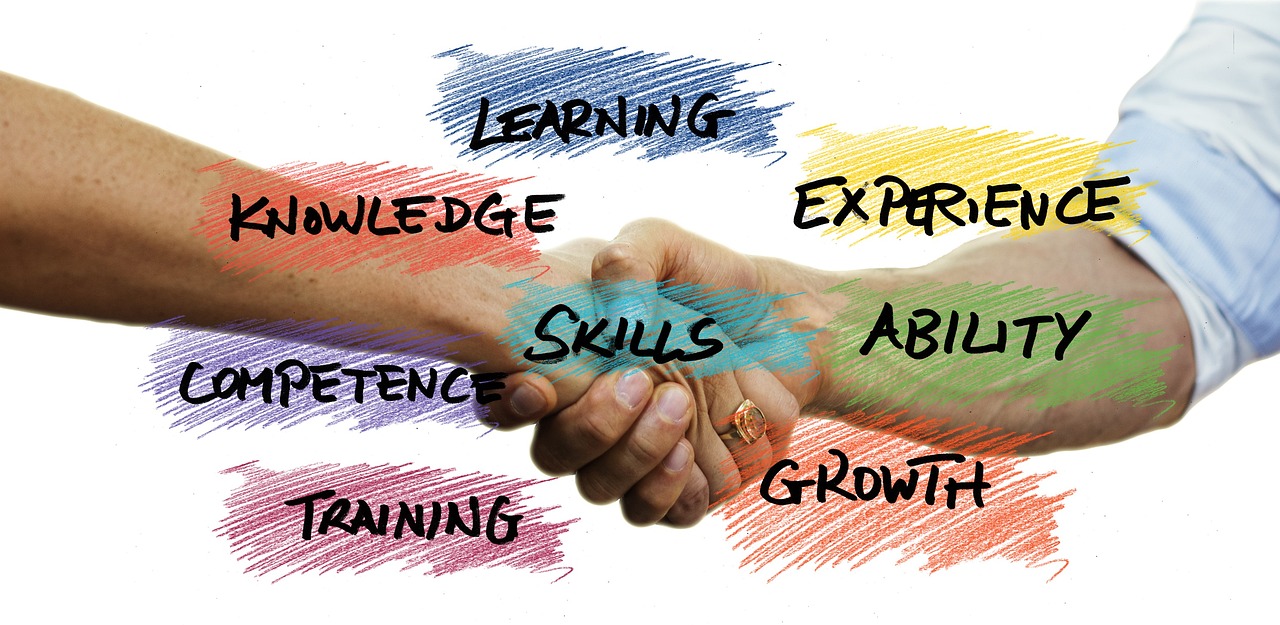
Data Analysis Techniques
In the rapidly evolving landscape of artificial intelligence, have emerged as vital tools for professionals aiming to harness the power of data. Understanding these techniques is not just beneficial; it's essential for making informed decisions in today’s data-driven world. At its core, data analysis involves systematically applying statistical and logical techniques to describe, summarize, and compare data. It’s akin to piecing together a puzzle, where each piece of data contributes to a clearer picture of trends, patterns, and insights that can drive business strategy.
One of the foundational techniques in data analysis is descriptive statistics. This technique allows analysts to summarize data sets and describe their main features, often through visualizations such as charts and graphs. Think of it as the first layer of understanding; it provides a snapshot of what the data looks like without diving too deep into the 'why' behind the numbers. For instance, a company might use descriptive statistics to analyze sales data over a quarter, identifying trends in customer purchases that can inform inventory decisions.
Moving deeper, we encounter inferential statistics, which takes us beyond mere description. This technique enables analysts to make predictions and generalizations about a population based on a sample of data. It’s like using a small taste of a dish to predict how the entire meal will taste. By employing methods such as hypothesis testing and confidence intervals, businesses can draw conclusions about their customer base or market trends with a certain degree of certainty.
Another critical technique is predictive analytics, which utilizes historical data to forecast future outcomes. This is where the magic of machine learning often comes into play. By leveraging algorithms, organizations can identify patterns in data that may not be immediately obvious. For example, a retail company might analyze customer purchasing behavior to predict future sales, allowing them to optimize marketing strategies and inventory levels. Predictive analytics acts like a crystal ball, offering insights that can shape future business decisions.
Furthermore, the advent of big data has transformed the way we approach data analysis. With the capability to process vast amounts of information, organizations can utilize advanced techniques such as data mining and text analytics. Data mining involves exploring large data sets to uncover hidden patterns and correlations, while text analytics focuses on extracting meaningful information from unstructured data sources, such as social media or customer reviews. These techniques empower businesses to tap into a wealth of information, enabling them to understand customer sentiment and market dynamics better.
To illustrate the significance of these techniques, consider the following table, which outlines key data analysis methods and their applications:
Technique | Description | Application |
---|---|---|
Descriptive Statistics | Summarizes data sets to describe main features | Sales analysis, customer demographics |
Inferential Statistics | Makes predictions about a population based on a sample | Market research, survey analysis |
Predictive Analytics | Forecasts future outcomes using historical data | Sales forecasting, risk assessment |
Data Mining | Uncovers hidden patterns in large data sets | Customer segmentation, fraud detection |
Text Analytics | Extracts insights from unstructured data | Sentiment analysis, brand monitoring |
In conclusion, mastering data analysis techniques is not just a nice-to-have skill; it’s a necessity in the AI-driven job market. As organizations increasingly rely on data to inform their strategies, professionals who can navigate these techniques will find themselves at a significant advantage. By combining descriptive, inferential, and predictive analytics with advanced methods like data mining and text analytics, individuals can transform raw data into actionable insights, paving the way for innovation and success in their careers.
- What is the most important data analysis technique? While all techniques have their importance, predictive analytics is often viewed as crucial due to its ability to forecast future trends.
- How can I learn data analysis techniques? There are numerous online courses, workshops, and resources available that can help you learn data analysis, including platforms like Coursera, edX, and Khan Academy.
- Is programming knowledge necessary for data analysis? While not mandatory, having a basic understanding of programming languages such as Python or R can significantly enhance your data analysis capabilities.

Emerging Technologies and Trends
In the fast-paced world of technology, emerging technologies and trends in artificial intelligence (AI) are not just buzzwords; they are the driving forces reshaping industries and redefining job roles. As we stand on the brink of a new era, it’s crucial for professionals to grasp the significance of these advancements. Think of AI as a river that is constantly flowing, with new tributaries of innovation branching out at every turn. If you're not paying attention, you might just miss the boat!
One of the most exciting trends is the rise of natural language processing (NLP). This technology enables machines to understand and respond to human language in a way that feels intuitive and natural. Imagine chatting with a computer that can not only understand your words but also grasp the context and sentiment behind them. This capability is transforming customer service, content creation, and even personal assistants, making them more efficient and user-friendly.
Another significant trend is the integration of AI with the Internet of Things (IoT). Picture a smart home where your refrigerator can analyze your dietary habits and suggest recipes based on what you have inside. This convergence is creating a seamless interaction between devices, allowing for smarter decision-making and enhanced user experiences. As IoT devices proliferate, the demand for professionals who can develop and manage these interconnected systems will skyrocket.
Moreover, the concept of explainable AI (XAI) is gaining traction. As AI systems become more complex, the need for transparency in how they make decisions is paramount. XAI aims to make AI's decision-making processes understandable to humans, which is crucial in sectors like healthcare, finance, and law, where trust and accountability are vital. Without this transparency, the risk of bias and ethical dilemmas increases, potentially undermining the very foundations of AI technology.
To put it simply, the landscape of AI is evolving, and staying updated on these trends is not just beneficial—it's essential. Professionals who embrace continuous learning and adapt to these changes will find themselves at the forefront of the industry. Whether it's through online courses, workshops, or industry conferences, investing time in understanding these technologies can make a significant difference in your career trajectory.
As we look ahead, it’s clear that the convergence of AI with other technological advancements will create new opportunities and challenges. The workforce must be prepared to navigate this shifting terrain. Companies that foster a culture of innovation and encourage their employees to engage with these emerging technologies will undoubtedly lead the pack. After all, in a world where change is the only constant, adaptability is the name of the game!
- What are the key emerging technologies in AI? Some of the key technologies include natural language processing, machine learning, and the integration of AI with IoT.
- Why is continuous learning important in the AI field? Continuous learning helps professionals stay updated with the latest trends and technologies, ensuring they remain relevant and competitive.
- How can organizations support their employees in bridging the AI skills gap? Organizations can offer training programs, workshops, and encourage participation in industry events to enhance their employees' skills.
- What is explainable AI (XAI)? Explainable AI refers to methods and techniques that make the decisions of AI systems understandable to humans, ensuring transparency and trust.

Strategies for Bridging the Skills Gap
As we stand on the brink of an AI revolution, it’s crucial to recognize that the skills gap in artificial intelligence is not just a hurdle; it's a call to action. Organizations and individuals alike must embrace innovative strategies to equip the workforce with the necessary AI competencies. After all, the future is not just about technology; it's about the people who can wield it effectively. So, what can be done to bridge this gap?
One of the most effective strategies is upskilling and reskilling. Companies can invest in their current employees by providing access to training programs that focus on AI technologies. This not only enhances the skills of the workforce but also boosts employee morale and retention. For instance, organizations might implement workshops that cover essential AI concepts, machine learning, and data analytics. By fostering a culture of continuous learning, companies can ensure their teams remain competitive in a rapidly changing job market.
Moreover, creating partnerships between educational institutions and industry leaders is vital. These collaborations can lead to the development of tailored curricula that meet the specific needs of the job market. For example, universities might work with tech companies to design courses that provide hands-on experience with real-world AI applications. This synergy not only prepares students for careers in AI but also gives businesses access to a pool of skilled candidates who are ready to hit the ground running.
Additionally, organizations should consider implementing mentorship programs. These programs can connect experienced professionals with newcomers in the AI field, fostering knowledge transfer and skill development. Mentorship not only accelerates learning but also builds a network of support within the industry. Imagine having a seasoned AI expert guiding you through your career journey—this kind of support can be invaluable!
To further enhance the effectiveness of these strategies, it’s essential to leverage technology. Online learning platforms have become a game-changer in education, offering flexible and accessible options for individuals looking to improve their AI skills. With platforms like Coursera, Udacity, and edX, learners can access courses from top universities and industry leaders from the comfort of their homes. This democratization of education allows anyone, regardless of their background, to gain the skills needed to thrive in an AI-driven world.
In summary, bridging the AI skills gap requires a multifaceted approach that includes upskilling existing employees, fostering collaborations between educational institutions and industry, implementing mentorship programs, and utilizing online learning platforms. By embracing these strategies, we can ensure that the workforce is not only prepared for the challenges of the future but is also empowered to innovate and lead in the age of artificial intelligence.
- What is the AI skills gap?
The AI skills gap refers to the disparity between the demand for skilled professionals in artificial intelligence and the available talent in the job market. - How can individuals improve their AI skills?
Individuals can improve their AI skills through online courses, workshops, and mentorship programs, as well as by gaining hands-on experience through projects. - Why is continuous learning important in AI?
Continuous learning is crucial in AI due to the rapid advancements in technology and methodologies, ensuring professionals remain relevant and competitive.
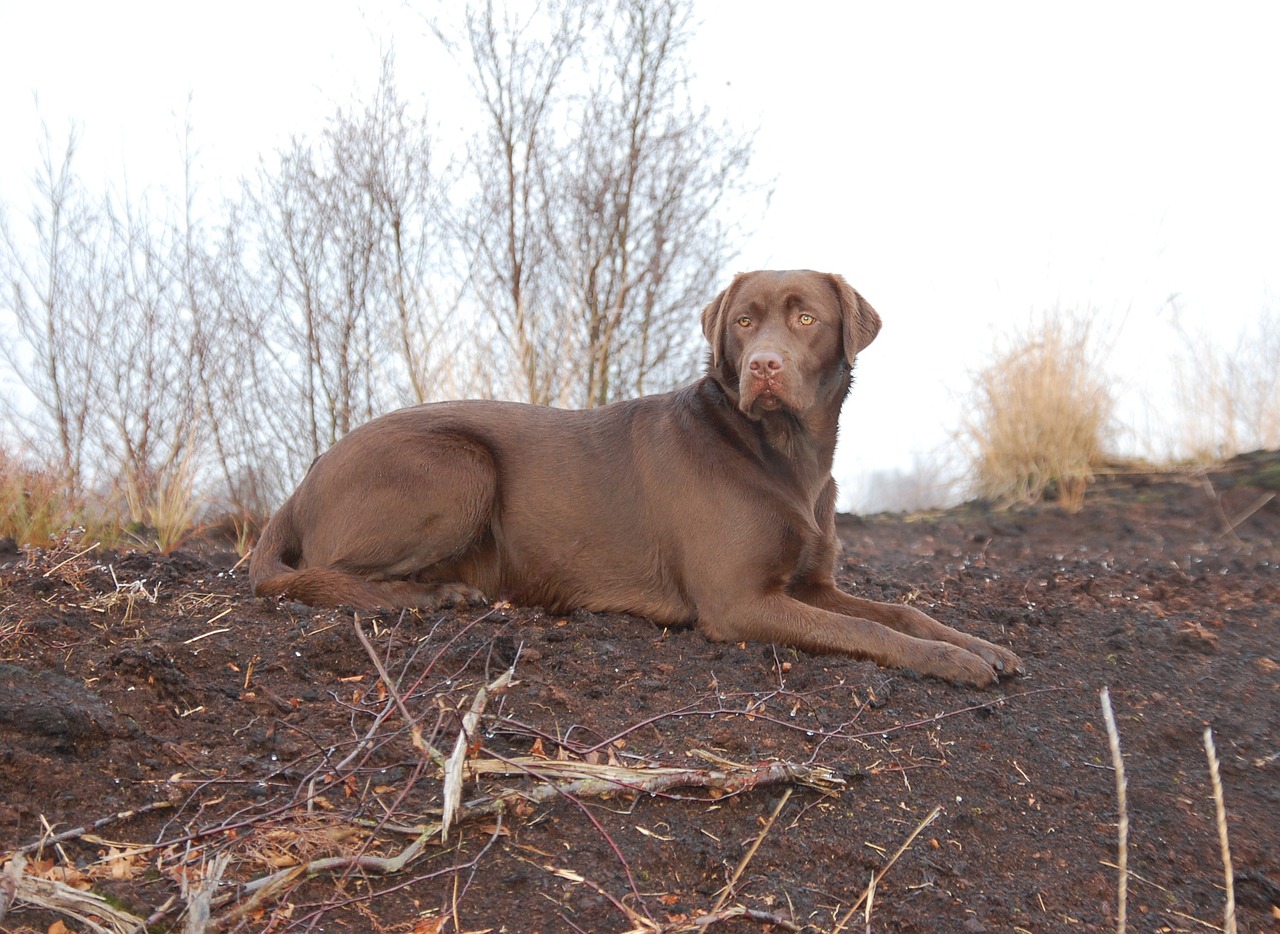
Upskilling and Reskilling Initiatives
In today's fast-paced world, where technology evolves at lightning speed, the need for upskilling and reskilling has never been more critical. As organizations strive to stay competitive, they are realizing that investing in their workforce is not just beneficial—it's essential. Upskilling refers to enhancing the existing skills of employees, while reskilling involves teaching them new skills to adapt to changing job requirements. This dual approach is vital for navigating the AI landscape, where traditional roles are rapidly transforming.
One of the most effective strategies for implementing upskilling and reskilling initiatives is through tailored training programs. These programs can be designed to meet the specific needs of both the organization and its employees. For instance, companies might offer workshops, online courses, or mentorship opportunities that focus on AI-related competencies. By doing so, they not only empower their employees but also foster a culture of continuous learning. After all, who wouldn’t want to be equipped with the latest tools and knowledge in their field?
Moreover, organizations can leverage partnerships with educational institutions to create a robust learning ecosystem. By collaborating with universities and technical schools, companies can develop curricula that align with industry demands. This not only ensures that the training is relevant but also helps students transition smoothly into the workforce. Imagine a scenario where students graduate with hands-on experience in AI, making them highly sought after by employers. It’s a win-win situation!
To further illustrate the importance of these initiatives, consider the following table that outlines the benefits of upskilling and reskilling:
Benefits | Details |
---|---|
Increased Employee Engagement | Employees feel valued and motivated when they see that their employer is investing in their growth. |
Enhanced Productivity | With updated skills, employees can perform tasks more efficiently, leading to improved overall productivity. |
Better Retention Rates | When employees are given opportunities to grow, they are less likely to seek employment elsewhere. |
Innovation and Adaptability | A skilled workforce is more capable of adapting to new technologies and driving innovation within the company. |
In addition to structured programs, companies can also encourage a culture of self-directed learning. Employees should be motivated to pursue online courses, attend seminars, and engage with professional communities. Platforms like Coursera, Udacity, and LinkedIn Learning offer a plethora of resources that can help individuals sharpen their AI skills at their own pace. The beauty of this approach is that it allows for flexibility—perfect for those juggling work and personal commitments.
Ultimately, the success of upskilling and reskilling initiatives hinges on a supportive environment. Leadership must champion these efforts, showcasing their commitment to employee development. When leaders actively participate in learning opportunities, it sends a powerful message: learning is a priority. This not only inspires employees but also cultivates a dynamic workplace where everyone is encouraged to grow and adapt.
In conclusion, as we stand on the brink of an AI-driven future, the need for upskilling and reskilling initiatives cannot be overstated. By investing in their workforce, organizations can bridge the skills gap, ensuring that their employees are not only equipped to handle current challenges but are also prepared for future opportunities. After all, in a world where change is the only constant, the ability to learn and adapt is the ultimate competitive advantage.
- What is the difference between upskilling and reskilling?
Upskilling enhances existing skills, while reskilling teaches new skills to adapt to changing job roles. - Why are upskilling and reskilling important?
They help employees stay relevant in their fields, increase productivity, and foster innovation within organizations. - How can organizations implement effective training programs?
By tailoring programs to meet specific needs, partnering with educational institutions, and encouraging self-directed learning.

Collaboration Between Educational Institutions and Industry
In today's fast-paced world, the collaboration between educational institutions and industry is not just beneficial; it's essential. As we navigate through the complexities of an AI-driven economy, the gap between what students learn and what employers need is becoming increasingly pronounced. This disconnect can lead to a workforce that is ill-prepared for the challenges of modern job markets. By joining forces, educational institutions and industry leaders can create a robust framework that equips students with the necessary skills to thrive in their careers.
One of the most effective ways to bridge this gap is through internship programs and apprenticeships. These initiatives allow students to gain hands-on experience while still in school, which not only enhances their learning but also gives them a competitive edge when entering the job market. Companies benefit from these programs as well, as they can identify and nurture talent that aligns with their organizational goals. Imagine a scenario where a student, while pursuing a degree, is simultaneously working on real-world projects that contribute to their employer's success. This symbiotic relationship fosters a culture of innovation and prepares students for the realities of their future roles.
Moreover, joint research projects between universities and companies can lead to groundbreaking advancements in AI technology. These collaborations often result in innovative solutions that address industry-specific problems. For instance, when students and professionals work together, they bring diverse perspectives that can lead to creative problem-solving. Educational institutions can also benefit from industry insights, allowing them to adapt their curricula to meet current and future demands. This dynamic exchange of knowledge helps ensure that the education provided is not only relevant but also forward-thinking.
However, for these collaborations to be successful, several factors must be considered:
- Clear Communication: Both parties need to establish open lines of communication to align their goals and expectations.
- Mutual Benefits: Collaborations should be designed to provide value to both students and employers, ensuring a win-win situation.
- Flexibility: The rapidly changing landscape of AI requires that both educational institutions and industries remain adaptable to new trends and technologies.
In conclusion, the collaboration between educational institutions and industry is a powerful strategy for bridging the AI skills gap. By working together, they can create a more competent and agile workforce ready to tackle the challenges of the future. As we continue to embrace AI technology, these partnerships will play a crucial role in shaping the careers of upcoming generations, ensuring they are not just participants in the workforce but leaders in innovation.
Q1: Why is collaboration between educational institutions and industry important?
A1: Collaboration is vital because it aligns educational outcomes with industry needs, ensuring that graduates are equipped with relevant skills and experience.
Q2: What are some examples of successful collaborations?
A2: Successful collaborations include internship programs, joint research initiatives, and curriculum development partnerships that involve industry professionals.
Q3: How can students benefit from these collaborations?
A3: Students gain practical experience, networking opportunities, and insights into industry trends, which significantly enhance their employability.
Q4: What role do companies play in shaping educational curricula?
A4: Companies can provide feedback on the skills they require and participate in curriculum development to ensure that educational programs are relevant and up-to-date.

The Role of Continuous Learning
In today's rapidly changing world, the concept of continuous learning has become more than just a buzzword; it’s a vital necessity for anyone looking to thrive in the AI landscape. As technology evolves at breakneck speed, the skills that were once deemed essential can quickly become obsolete. This is especially true in the field of artificial intelligence, where new tools, techniques, and methodologies emerge almost daily. So, how do we keep up? The answer lies in embracing a mindset of lifelong learning.
Imagine trying to navigate a vast ocean without a map. That’s what it feels like for professionals who neglect to update their skill sets in AI. The waves of innovation can easily sweep them away, leaving them stranded on the shores of outdated knowledge. Continuous learning acts as that map, guiding individuals through the tumultuous waters of change. It empowers them to adapt and seize new opportunities, ensuring they remain relevant in a competitive job market.
Moreover, continuous learning fosters a culture of curiosity and innovation within organizations. Companies that encourage their employees to pursue ongoing education not only enhance individual capabilities but also boost overall productivity and creativity. When employees are equipped with the latest knowledge and skills, they are more likely to contribute innovative ideas that can drive the company forward. This creates a win-win situation where both the individual and the organization can flourish.
But what does continuous learning look like in practice? It can take many forms, including:
- Online Courses: Platforms like Coursera, Udacity, and edX offer a plethora of courses tailored to AI skills, from machine learning to data science.
- Workshops and Seminars: Attending industry events allows professionals to learn from experts and network with peers.
- Self-Directed Learning: Reading books, articles, and research papers can help individuals stay informed about the latest trends and technologies.
- Mentorship Programs: Partnering with experienced professionals can provide invaluable insights and guidance.
Organizations can also play a pivotal role in promoting continuous learning. By offering training programs, providing access to educational resources, and creating an environment that values knowledge sharing, companies can ensure their workforce is well-prepared for the future. This investment in employee development not only enhances skills but also boosts morale and retention rates.
In conclusion, the role of continuous learning in bridging the AI skills gap cannot be overstated. It is the lifeline that keeps professionals afloat in an ocean of change. By fostering a culture of learning and encouraging individuals to embrace lifelong education, we can pave the way for a more competent and innovative workforce, ready to tackle the challenges of an AI-driven future.
Q1: Why is continuous learning important in AI?
A1: Continuous learning is crucial in AI because the field is constantly evolving. New technologies and methodologies emerge frequently, and staying updated ensures that professionals remain relevant and effective in their roles.
Q2: What are some effective ways to engage in continuous learning?
A2: Effective ways to engage in continuous learning include enrolling in online courses, attending workshops, reading industry-related materials, and seeking mentorship from experienced professionals.
Q3: How can organizations support continuous learning?
A3: Organizations can support continuous learning by providing access to training programs, encouraging knowledge sharing, and creating a culture that values ongoing education and professional development.
Frequently Asked Questions
- What is the AI skills gap?
The AI skills gap refers to the disparity between the skills needed for AI-related jobs and the skills that job seekers currently possess. As industries increasingly adopt AI technologies, the demand for skilled professionals in areas like machine learning and data analysis is growing faster than the supply.
- Why are AI skills important in today's job market?
AI skills are crucial because they enhance a candidate's employability across various sectors. Companies are looking for individuals who can leverage AI to drive innovation, improve efficiency, and make data-driven decisions. Without these skills, job seekers may find themselves at a disadvantage.
- What key competencies are necessary for a career in AI?
To thrive in AI, professionals should focus on competencies such as machine learning, data analysis, programming languages (like Python or R), and an understanding of algorithms. These skills are foundational for anyone looking to work in tech-driven roles.
- How can individuals bridge the AI skills gap?
Individuals can bridge the skills gap through upskilling and reskilling initiatives, online courses, and certifications in AI and data science. Engaging in continuous learning and staying updated with emerging technologies is also essential for maintaining relevance in the field.
- What role do educational institutions play in bridging the AI skills gap?
Educational institutions play a pivotal role by developing curricula that align with industry needs. Collaborations with businesses can provide students with practical experiences and insights into the skills required in the workforce, ultimately preparing them for AI careers.
- What are some effective strategies for organizations to address the skills gap?
Organizations can implement training programs, mentorship opportunities, and partnerships with educational institutions to enhance their workforce's AI competencies. Additionally, fostering a culture of continuous learning can help employees stay competitive in an ever-evolving landscape.
- Why is continuous learning important in the AI field?
Continuous learning is vital in the AI field because technology and methodologies are constantly evolving. Professionals must keep their skills updated to adapt to new tools and trends, ensuring they remain valuable assets to their organizations.