Artificial Intelligence in Genomics and Healthcare
Artificial Intelligence (AI) is not just a buzzword; it's a game-changer in the fields of genomics and healthcare. Imagine a world where complex genetic data can be analyzed in seconds, leading to faster diagnoses and more effective treatments. Sounds incredible, right? Well, that’s exactly what AI is making possible. By harnessing the power of machine learning and deep learning, AI is transforming how we understand genetics and deliver healthcare services. The implications are profound, as AI tools are not only streamlining research but also paving the way for personalized medicine that caters to individual needs.
One of the most exciting aspects of AI in genomics is its ability to process vast amounts of data at unprecedented speeds. Traditional methods of genetic analysis can take weeks or even months, but with AI, researchers can uncover insights almost instantaneously. This rapid analysis is crucial in a world where time is often of the essence, especially when it comes to diagnosing genetic disorders or developing targeted therapies.
Moreover, AI is enhancing our understanding of genetic variations and their implications for health. By analyzing patterns within genomic data, AI can help identify potential health risks before they manifest as serious conditions. This proactive approach not only improves patient outcomes but also reduces healthcare costs by emphasizing prevention over treatment.
However, it’s essential to recognize that the integration of AI into genomics and healthcare is not without its challenges. Issues related to data privacy, ethical considerations, and the need for informed consent are paramount. As we delve deeper into the capabilities of AI, we must also address these concerns to ensure that technological advancements do not come at the expense of patient trust and safety.
In the subsequent sections, we will explore the various applications of AI in genomics, the impact on disease diagnosis, and the ethical considerations that accompany these advancements. The future of AI in healthcare is bright, and understanding its potential will help us navigate the complexities of this evolving landscape.
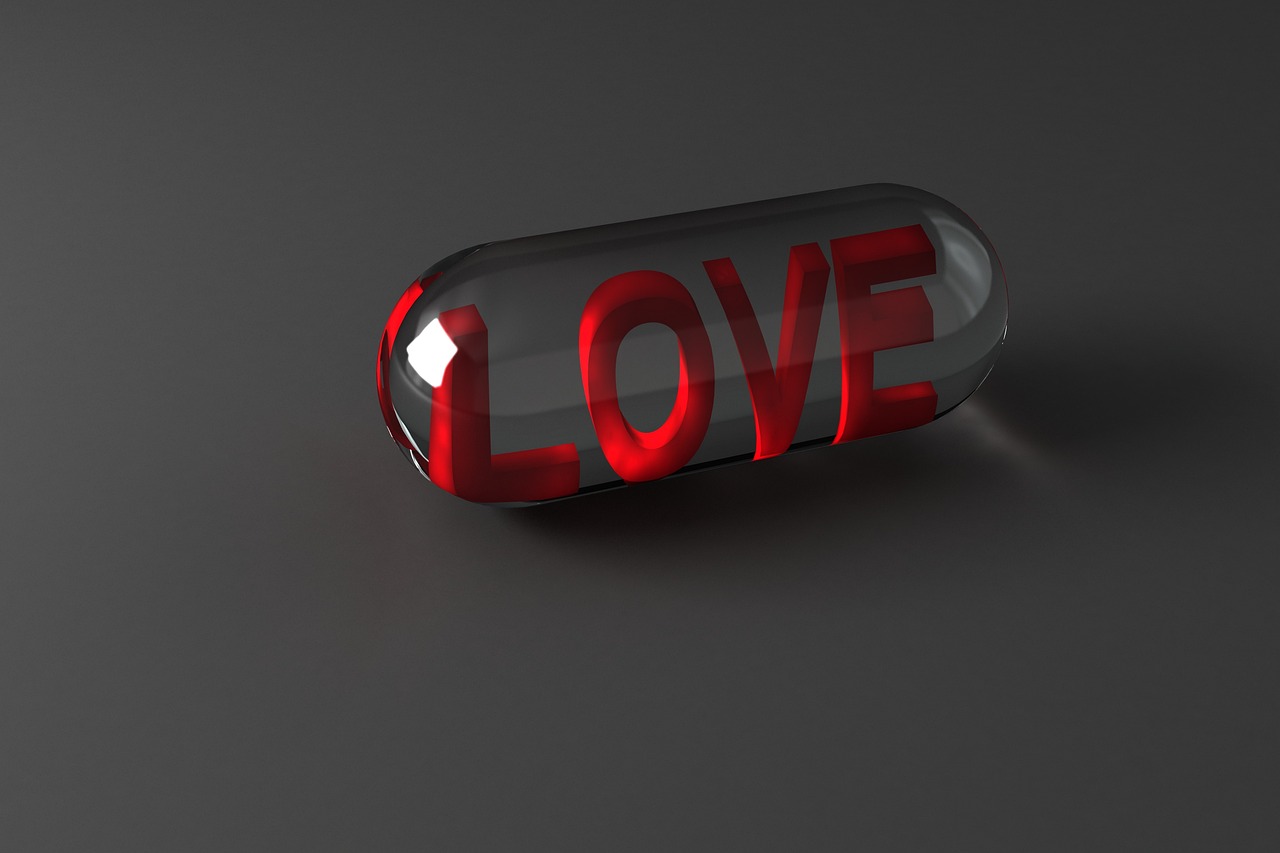
AI Applications in Genomics
Artificial Intelligence (AI) is not just a buzzword anymore; it’s a game-changer in the field of genomics. Imagine being able to analyze massive amounts of genetic data in a fraction of the time it would take a human. That's exactly what AI is doing! By leveraging advanced algorithms, researchers can now uncover insights that were previously hidden in the vast sea of genetic information. This is crucial as it leads to faster discoveries in areas such as gene editing and personalized medicine.
One of the most exciting applications of AI in genomics is its ability to enhance gene editing technologies. Techniques like CRISPR have revolutionized how we approach genetic modifications, but AI takes it a step further. By predicting the outcomes of gene edits, AI can help scientists make more informed decisions, reducing the risk of unintended consequences. For instance, AI can analyze the potential effects of a specific gene edit on an organism's overall health, leading to safer and more effective treatments.
Moreover, AI plays a significant role in personalized medicine. Imagine a world where your treatment plan is tailored specifically to your genetic makeup. With AI, this is becoming a reality. By analyzing genomic data, AI can identify which treatments are likely to be the most effective for individual patients based on their unique genetic profiles. This not only improves patient outcomes but also minimizes the trial-and-error approach that is often seen in traditional medicine.
In addition, AI tools such as machine learning and deep learning are being employed to sift through vast datasets, identifying patterns and anomalies that human researchers might overlook. For example, researchers can use machine learning algorithms to analyze genetic sequences, identifying mutations that may be linked to specific diseases. This capability is crucial in the early detection of genetic disorders, allowing for timely interventions that can save lives. In fact, studies have shown that AI can increase the accuracy of genomic analyses significantly, leading to better diagnostic outcomes.
AI Techniques | Applications in Genomics |
---|---|
Machine Learning | Identifying genetic mutations and patterns in data. |
Deep Learning | Analyzing complex genomic datasets for insights. |
Natural Language Processing | Extracting information from unstructured genomic data. |
Furthermore, the integration of Natural Language Processing (NLP) in genomics is transforming how researchers access and interpret literature. With the explosion of genomic studies published daily, it can be overwhelming for scientists to keep up. NLP tools can automatically mine research papers, extracting relevant information and summarizing findings that can aid in ongoing research. This not only saves time but also enhances collaboration across different fields of study.
In conclusion, the applications of AI in genomics are vast and varied, from accelerating gene editing to enabling personalized medicine. As technology advances, we can expect even more innovative uses of AI that will continue to push the boundaries of what is possible in genomic research and healthcare. The future is bright, and the potential for AI to enhance our understanding of genetics is truly exciting!
- What is the role of AI in genomics? AI helps in analyzing large datasets, enhancing gene editing, and personalizing medical treatments based on genetic profiles.
- How does AI improve disease diagnosis? AI algorithms analyze genomic data to identify genetic disorders and predict disease susceptibility, leading to earlier interventions.
- What are the ethical concerns surrounding AI in genomics? Key concerns include data privacy, informed consent, and the implications of genetic information on individuals and society.
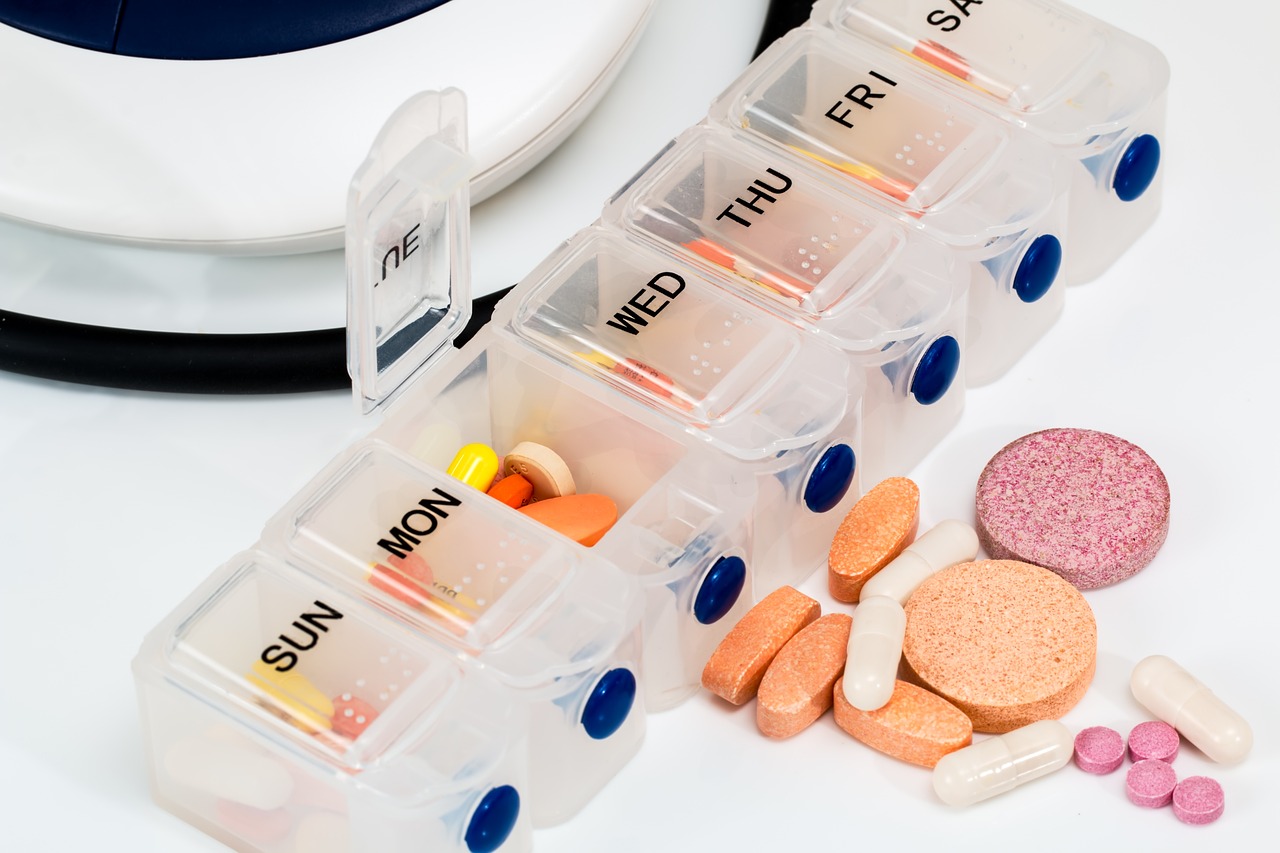
Impact on Disease Diagnosis
The integration of artificial intelligence (AI) in healthcare is a game-changer, particularly when it comes to disease diagnosis. Imagine a world where identifying genetic disorders and predicting disease susceptibility is as quick as a snap of your fingers. That's the power of AI! By analyzing vast amounts of genomic data, AI algorithms can pinpoint abnormalities that human eyes might miss, leading to earlier interventions and more effective treatments. This not only improves patient outcomes but also significantly reduces the burden on healthcare systems.
AI's ability to sift through complex datasets at lightning speed allows for a level of precision that was previously unimaginable. For instance, machine learning models can learn from historical data and continuously improve their accuracy over time. This means that the more data these systems process, the better they become at identifying patterns linked to various diseases. It's like having a super-smart assistant who never forgets and always learns from past experiences!
In the realm of genetic disorders, early diagnosis can make all the difference. For example, conditions like Cystic Fibrosis or Huntington's Disease can be detected much earlier through AI-driven genomic analysis. Here’s how it typically works:
Step | Description |
---|---|
1 | Data Collection: Genomic data is gathered from various sources, including blood samples and family histories. |
2 | Data Processing: AI algorithms process this data to identify genetic markers associated with specific diseases. |
3 | Diagnosis: The system provides insights that help healthcare professionals make informed decisions regarding patient care. |
This process not only enhances diagnostic accuracy but also allows for tailored treatment plans. For instance, a patient diagnosed with a genetic predisposition to a certain disease can be monitored more closely, and preventative measures can be taken before symptoms even appear. It’s like having a crystal ball that offers glimpses into potential health issues before they manifest!
Moreover, AI is revolutionizing how we think about disease susceptibility. By analyzing genomic data alongside environmental factors, AI can help predict not just who might get sick, but when and why. This comprehensive approach enables healthcare providers to implement proactive strategies, making healthcare more preventative rather than reactive.
However, it's important to acknowledge that while AI is making significant strides in disease diagnosis, it also comes with its own set of challenges. Data quality is paramount; if the data fed into these algorithms is flawed or biased, the results could lead to misdiagnosis. Thus, ongoing validation and refinement of these AI systems are crucial to ensure they provide accurate and reliable outcomes.
In summary, the impact of AI on disease diagnosis is profound and far-reaching. By enhancing the accuracy of genetic disorder identification and predicting disease susceptibility, AI is not just changing the way we diagnose diseases; it is fundamentally transforming patient care and paving the way for a healthier future.
- How does AI improve disease diagnosis? AI improves disease diagnosis by analyzing large sets of genomic data quickly and accurately, identifying patterns that may indicate genetic disorders.
- What role does machine learning play in this process? Machine learning algorithms learn from historical data to enhance their predictive capabilities, allowing for more precise diagnosis over time.
- Can AI predict diseases before symptoms appear? Yes, AI can analyze genetic and environmental factors to predict disease susceptibility, enabling early intervention.
- What are the challenges associated with AI in disease diagnosis? Challenges include data quality, potential biases, and the need for ongoing validation of AI systems to ensure accuracy.
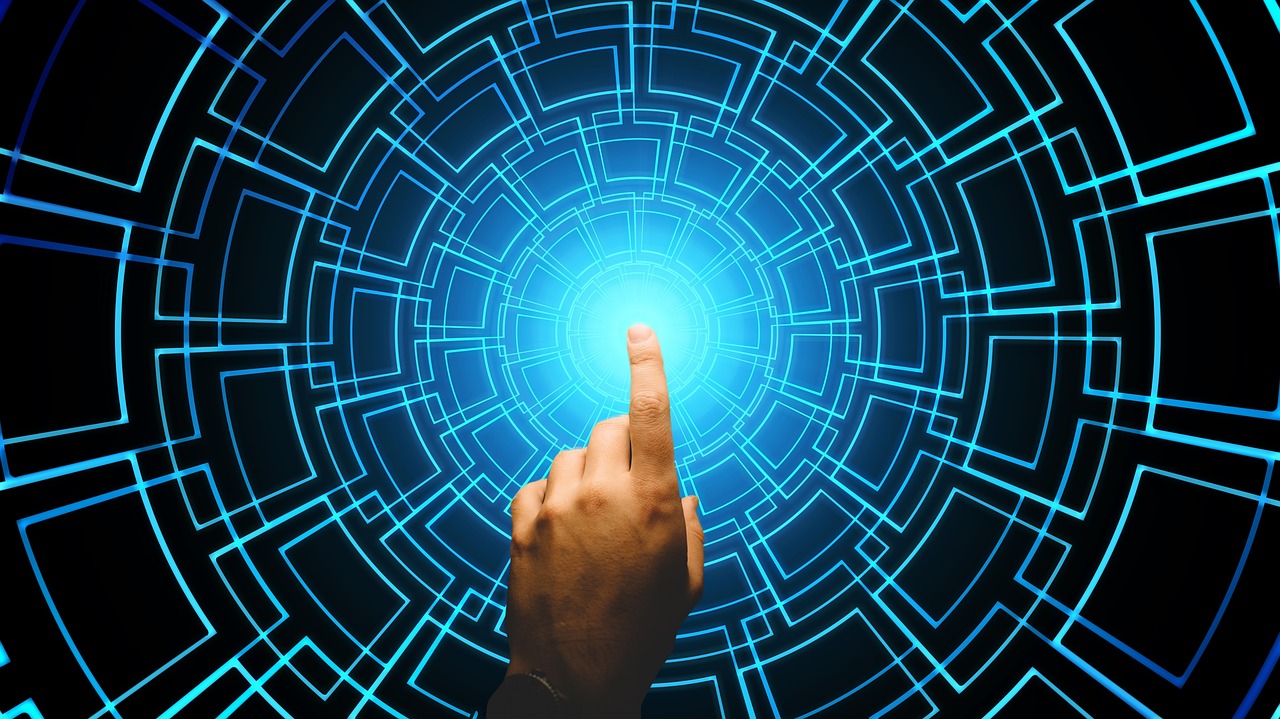
Machine Learning Techniques
When we talk about the transformative power of artificial intelligence in genomics, we can't overlook the pivotal role of . These techniques are like the secret sauce that allows us to sift through mountains of complex genomic data and uncover patterns that were previously hidden from view. Imagine trying to find a needle in a haystack; that's what genomic data analysis can feel like without the right tools. But with machine learning, we can not only find that needle but also understand its significance in the broader context of health and disease.
Machine learning algorithms can be divided into several categories, each with its strengths and applications in genomics. For instance, supervised learning techniques, where the model is trained on labeled data, are particularly effective in identifying genetic disorders. On the other hand, unsupervised learning helps in discovering new patterns and groupings in the data without prior labels, which can lead to exciting new insights into genetic variations.
Some of the most commonly used machine learning algorithms in genomics include:
- Support Vector Machines (SVM): These are powerful for classification tasks, helping to categorize genetic data into distinct groups.
- Random Forests: This ensemble method enhances prediction accuracy by combining multiple decision trees, making it excellent for handling complex datasets.
- Neural Networks: Particularly useful for modeling intricate relationships in data, neural networks are the backbone of many deep learning applications in genomics.
One of the most exciting aspects of machine learning in genomics is its ability to improve disease diagnosis and treatment planning. By analyzing vast amounts of genomic data, these algorithms can identify subtle patterns associated with specific diseases, enabling healthcare professionals to make more accurate diagnoses. Imagine a doctor being able to predict a patient's risk for certain genetic disorders based on their unique genetic makeup—this is not just a dream; it's becoming a reality thanks to machine learning!
Furthermore, as we continue to gather more genomic data, the potential for machine learning to uncover new insights will only grow. The integration of machine learning in genomics is like opening a treasure chest filled with invaluable information that can lead to breakthroughs in personalized medicine and targeted therapies. The future is bright, and the possibilities are endless!
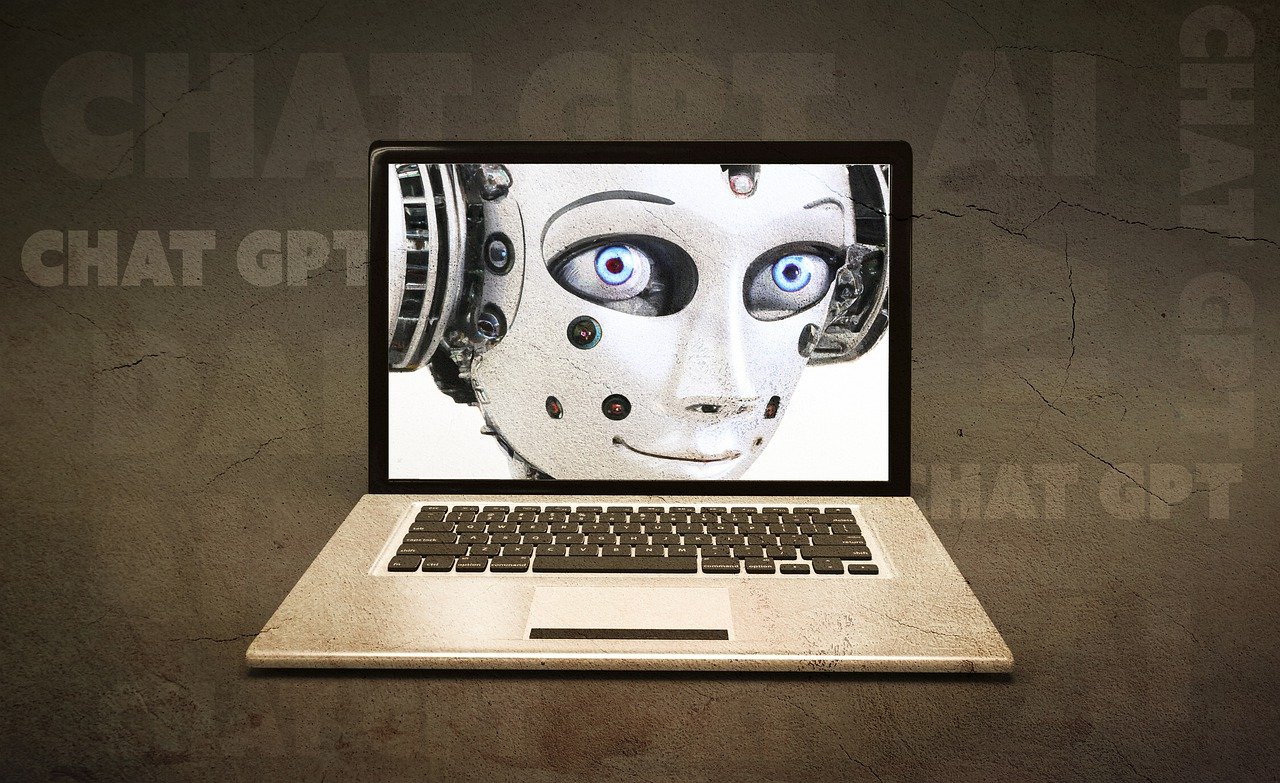
Deep Learning in Genomics
Deep learning has emerged as a groundbreaking technology in the field of genomics, enabling researchers to analyze vast datasets with unprecedented accuracy and efficiency. Imagine trying to find a needle in a haystack; now, picture having a super-powered magnet that not only finds the needle but also identifies its type, origin, and even its potential impact on health. That’s the power of deep learning in genomics!
At its core, deep learning is a subset of machine learning that uses neural networks with many layers (hence the term "deep") to process data. This technology is particularly well-suited for genomics because of the complexity and volume of genomic data generated by sequencing technologies. Traditional methods of data analysis often fall short when it comes to uncovering the intricate patterns hidden within genomic sequences. Deep learning, however, excels in this domain, allowing for the identification of genetic variations and their clinical significance.
For instance, deep learning models can analyze genomic sequences to predict how specific mutations may influence the development of diseases. These models are trained on large datasets that include both genomic information and corresponding clinical outcomes, enabling them to learn patterns that are often imperceptible to human researchers. As a result, deep learning can lead to more accurate predictions of disease susceptibility, aiding in early diagnosis and personalized treatment plans.
One of the most exciting applications of deep learning in genomics is in the identification of rare genetic disorders. By leveraging convolutional neural networks (CNNs), researchers can classify genomic sequences and pinpoint anomalies that may be linked to specific conditions. This capability not only enhances our understanding of genetic diseases but also opens the door to targeted therapies that can significantly improve patient outcomes.
Moreover, the integration of deep learning with other technologies, such as CRISPR for gene editing, represents a new frontier in genomics. Imagine being able to not only identify a genetic mutation but also edit it with precision. Deep learning algorithms can help design optimal guide RNAs for CRISPR, increasing the efficiency and accuracy of gene editing processes. This synergy between deep learning and gene editing technologies is poised to revolutionize the treatment of genetic disorders.
However, it's important to note that the application of deep learning in genomics is not without challenges. The complexity of genomic data and the need for high-quality datasets can pose hurdles. Additionally, the interpretability of deep learning models is often questioned—how do we understand the decisions made by these “black box” systems? Addressing these issues is crucial for the widespread adoption of deep learning in clinical settings.
In conclusion, deep learning is transforming the landscape of genomics by providing powerful tools for data analysis and interpretation. As we continue to refine these technologies and address the associated challenges, the potential for deep learning to enhance our understanding of genetics and improve patient care is nothing short of revolutionary.
- What is deep learning?
Deep learning is a subset of machine learning that uses neural networks with multiple layers to analyze data and identify patterns. - How does deep learning apply to genomics?
Deep learning enables the analysis of complex genomic data, leading to improved disease diagnosis, personalized medicine, and targeted therapies. - What are the challenges of using deep learning in genomics?
Challenges include data quality, the need for large datasets, and the interpretability of models. - Can deep learning help with gene editing?
Yes, deep learning can optimize the design of guide RNAs for CRISPR, improving the efficiency of gene editing.
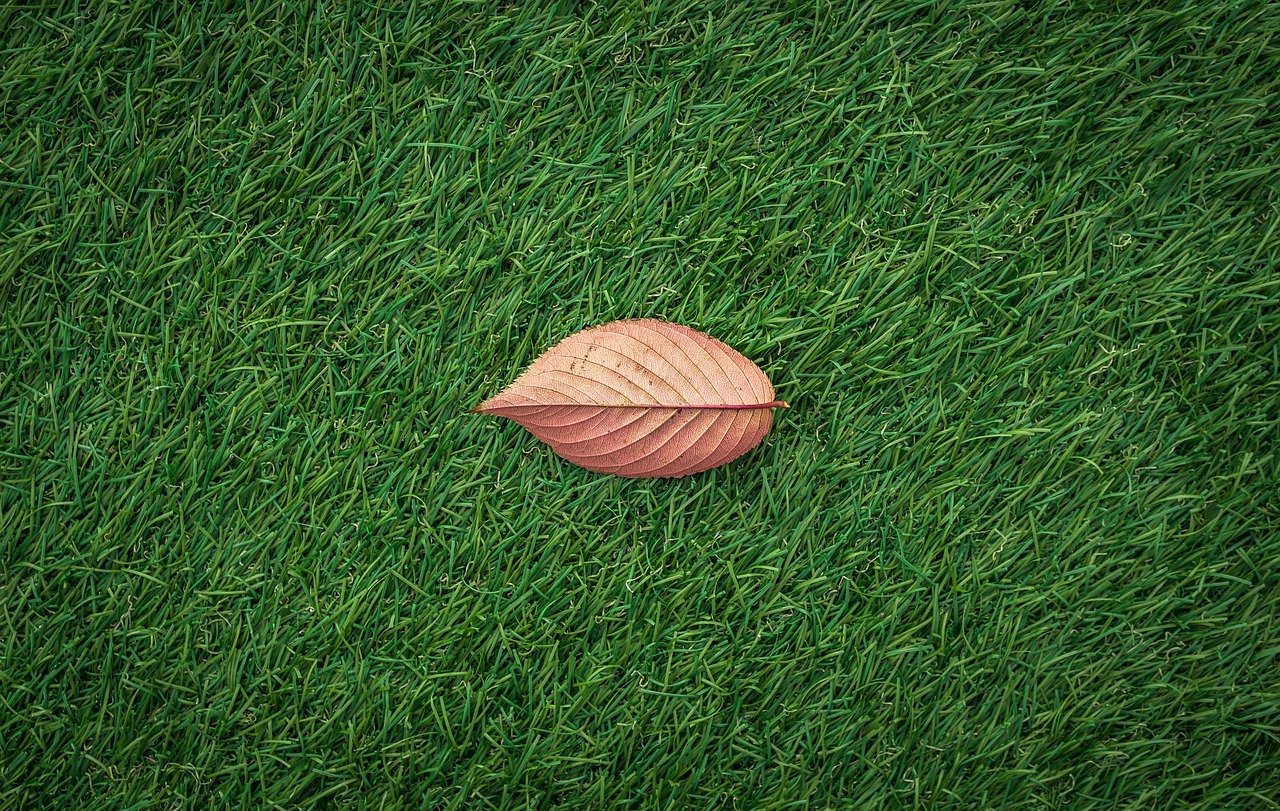
Natural Language Processing
Natural Language Processing (NLP) is an astonishingly powerful tool that is making waves in the field of genomics. Imagine being able to sift through mountains of unstructured data—like research papers, clinical notes, and laboratory reports—to extract meaningful insights in mere seconds. That’s the magic of NLP! It allows researchers and healthcare professionals to decode complex information and make informed decisions faster than ever before. This capability is particularly crucial in genomics, where the sheer volume of data can be overwhelming.
One of the most significant applications of NLP in genomics is in literature mining. With the explosion of genomic research, thousands of papers are published every month, and keeping up with the latest findings can feel like trying to drink from a fire hose. NLP algorithms can automate the process of scanning these publications, identifying relevant studies, and summarizing findings. This not only saves time but also ensures that researchers and clinicians are aware of the latest advancements that could impact patient care.
Moreover, NLP can facilitate knowledge discovery by identifying relationships between genes, diseases, and treatments that may not be immediately apparent. For instance, by analyzing clinical narratives, NLP can uncover patterns and correlations that lead to new hypotheses about disease mechanisms or potential therapeutic targets. This is akin to finding hidden treasures in a vast ocean of data—NLP acts as a sophisticated treasure map guiding researchers to valuable insights.
To illustrate the power of NLP in genomics, consider the following table that highlights its key applications:
Application | Description |
---|---|
Literature Mining | Automated extraction of relevant information from scientific papers. |
Clinical Narrative Analysis | Understanding patient histories and clinical notes to identify genetic predispositions. |
Data Integration | Combining information from various sources to create a comprehensive view of genomic data. |
Hypothesis Generation | Identifying new research avenues based on existing literature and data. |
In addition to these applications, NLP also plays a crucial role in enhancing patient communication. By translating complex genomic information into understandable language, healthcare providers can better explain genetic risks and treatment options to patients. This not only empowers patients to take an active role in their healthcare but also fosters trust and transparency in the provider-patient relationship.
As we look to the future, the integration of NLP with other AI technologies, such as machine learning and deep learning, promises even greater advancements in genomics. The ability to analyze both structured and unstructured data in real-time could revolutionize how we approach disease diagnosis, treatment, and prevention. It’s an exciting time to be involved in this field, as we stand on the brink of new discoveries that could change the landscape of healthcare forever.
In conclusion, Natural Language Processing is not just a technical innovation; it’s a transformative force in genomics and healthcare. By harnessing the power of NLP, we can unlock the potential of genomic data, leading to improved patient outcomes and a deeper understanding of the complexities of human health.
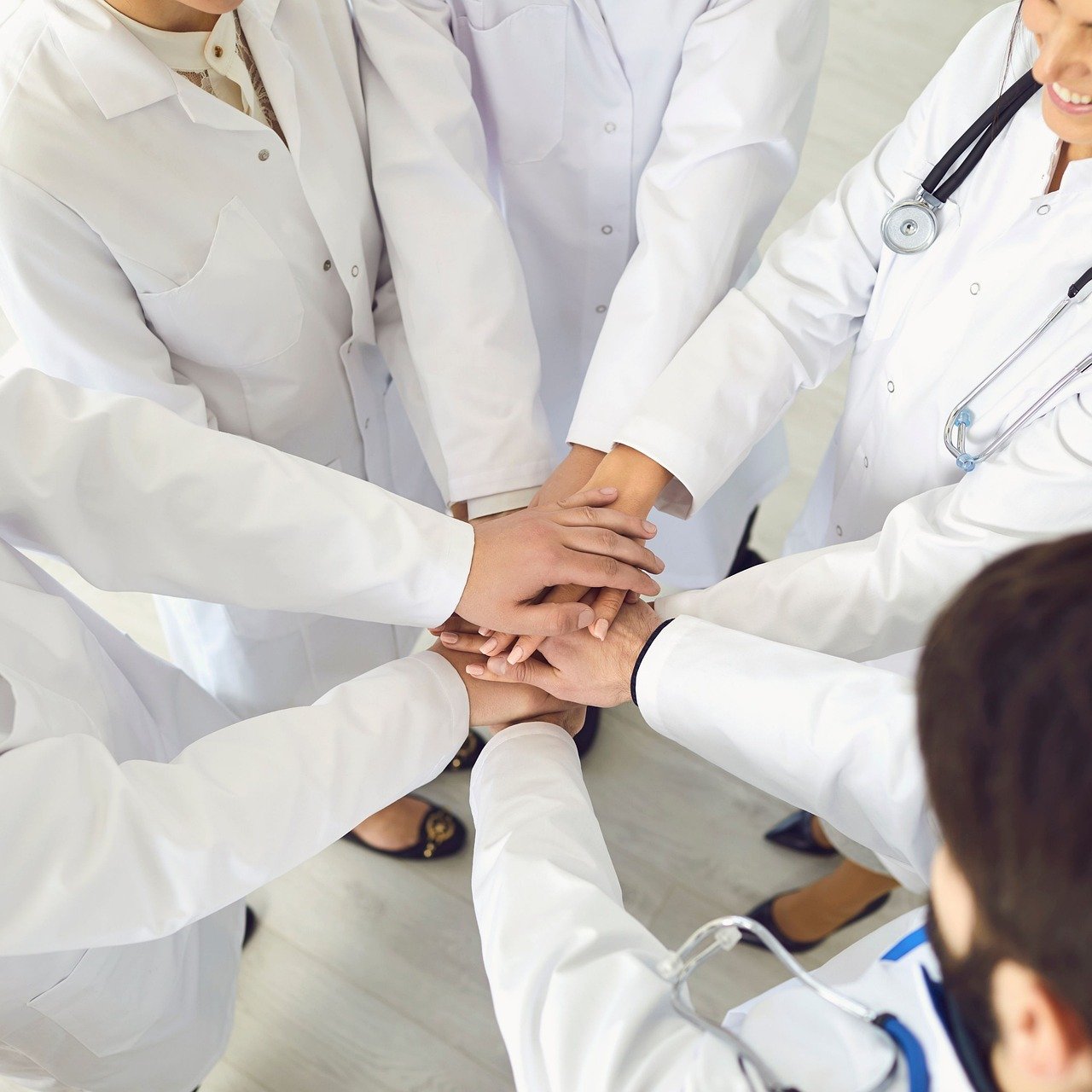
AI in Personalized Medicine
Artificial Intelligence (AI) is not just a buzzword; it’s a game-changer in the realm of personalized medicine. Imagine a world where treatments are tailored specifically to your genetic makeup, lifestyle, and environment. Sounds futuristic, right? Well, it’s happening now! AI algorithms analyze vast amounts of genomic data to identify unique genetic markers that can guide treatment decisions. This means that instead of a one-size-fits-all approach, healthcare providers can offer therapies that are specifically designed for you, increasing the chances of successful outcomes.
One of the most exciting aspects of AI in personalized medicine is its ability to predict how patients will respond to specific treatments. By leveraging machine learning techniques, AI can sift through historical patient data to uncover patterns and correlations that would be nearly impossible for humans to detect. For example, an AI model might analyze thousands of patient records to determine which cancer therapies work best for individuals with a specific genetic mutation. This level of precision not only enhances treatment efficacy but also minimizes the risk of adverse effects.
Moreover, AI helps in the development of targeted therapies. These are treatments designed to target specific characteristics of cancer cells or other diseases. With AI, researchers can identify potential drug candidates much faster than traditional methods. They can also simulate how these drugs will interact with different genetic profiles, leading to quicker clinical trials and, ultimately, faster access to life-saving therapies. The integration of AI in this process is like having a supercharged research assistant that never tires, always learns, and constantly improves.
To illustrate the impact of AI in personalized medicine, consider the following table, which outlines some key applications:
Application | Description | Benefits |
---|---|---|
Genetic Profiling | Analyzing individual genetic data to tailor treatments. | Increases treatment effectiveness and reduces side effects. |
Drug Discovery | Using AI to identify potential new drugs and their effects. | Speeds up the development process and lowers costs. |
Predictive Analytics | Forecasting patient responses to therapies. | Enhances treatment planning and patient outcomes. |
As we look to the future, the role of AI in personalized medicine is only expected to grow. Researchers and healthcare providers are continuously exploring new ways to integrate AI technologies into clinical practice. The potential is immense, and we are just scratching the surface of what is possible. Imagine a future where diseases can be predicted before they manifest, and treatments are not only personalized but also preventative.
In conclusion, AI is revolutionizing personalized medicine by providing insights that lead to more effective and tailored treatments. As technology advances, the hope is that we can enhance patient care and improve health outcomes on an unprecedented scale. So, the next time you hear about AI in healthcare, remember—it’s not just about robots and algorithms; it’s about creating a healthier future for all of us.
- What is personalized medicine? Personalized medicine is a medical model that tailors healthcare, with decisions and treatments customized to the individual patient based on their predicted response or risk of disease.
- How does AI contribute to personalized medicine? AI analyzes large datasets to identify patterns and correlations that help in predicting treatment responses and developing targeted therapies.
- Are there ethical concerns with AI in personalized medicine? Yes, ethical concerns include data privacy, informed consent, and potential biases in AI algorithms that could affect treatment equity.
- What is the future of AI in healthcare? The future looks promising, with ongoing advancements expected to enhance patient care, improve treatment accuracy, and facilitate quicker drug discovery processes.
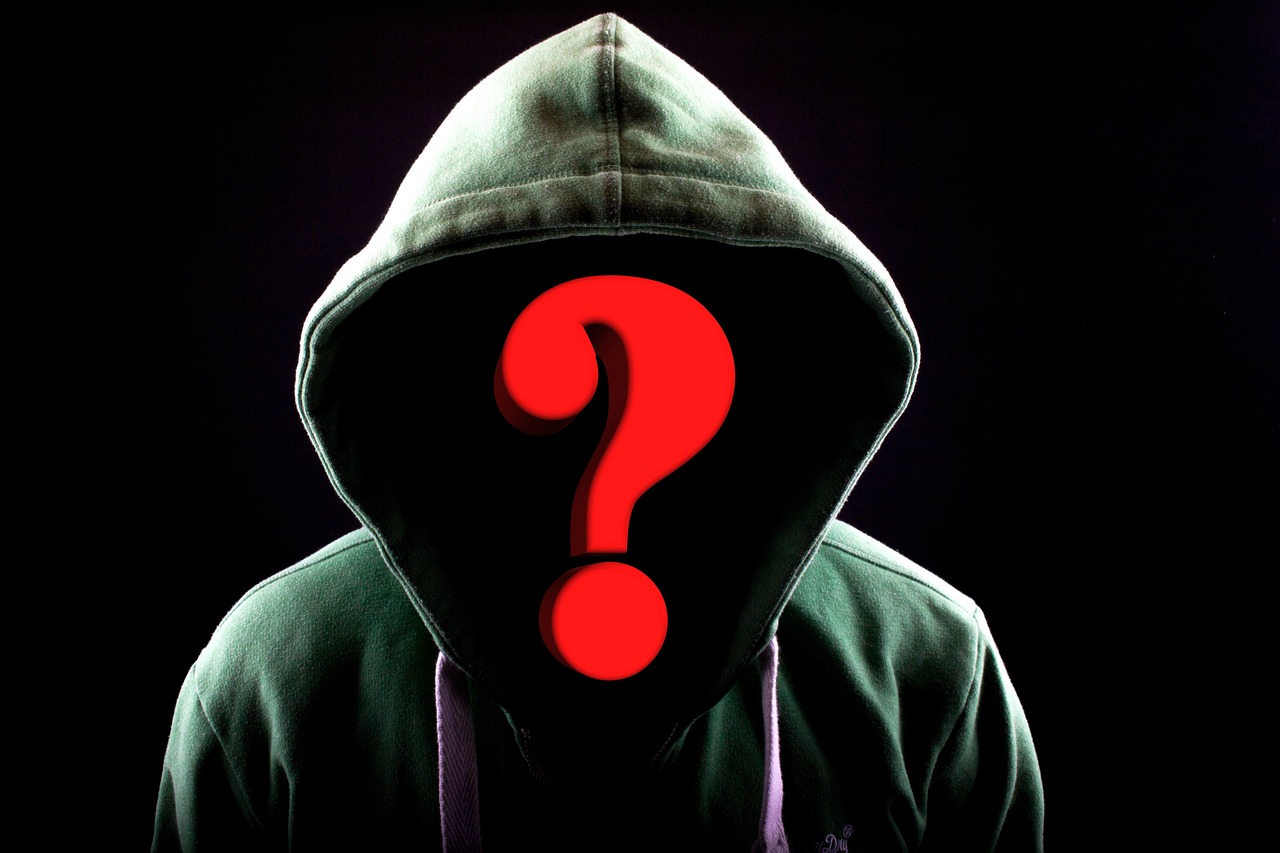
Ethical Considerations
As we delve deeper into the world of artificial intelligence (AI) in genomics, it becomes increasingly clear that the ethical implications of this technology cannot be overlooked. With the power to analyze vast amounts of genetic data, AI presents both incredible opportunities and significant challenges. One of the foremost concerns is data privacy. Genomic data is inherently sensitive, as it contains information that is unique to each individual and can reveal predispositions to various health conditions. Thus, safeguarding this information is paramount.
Moreover, the question of informed consent arises. Patients must be fully aware of how their genomic data will be used, especially when it comes to AI applications. This is not merely about ticking a box on a consent form; it's about ensuring that individuals understand the potential implications of sharing their genetic information. Transparency in communication is essential, as it fosters trust and respect between healthcare providers and patients.
Another ethical consideration is the potential for discrimination. There is a risk that individuals with certain genetic markers could face biases in healthcare, insurance, or employment. For instance, if AI algorithms identify a genetic predisposition to a particular disease, could this lead to increased insurance premiums or denial of coverage? These concerns highlight the need for robust ethical frameworks to guide the use of AI in genomics.
To address these concerns, several key measures can be implemented:
- Data Anonymization: Ensuring that genomic data is anonymized can help protect individual identities while still allowing for valuable research.
- Robust Security Protocols: Implementing advanced security measures is crucial to prevent unauthorized access to sensitive genomic data.
- Clear Guidelines for Use: Establishing clear ethical guidelines for the use of AI in genomics can help mitigate risks associated with discrimination and misuse of data.
In summary, while the integration of AI in genomics holds immense potential for advancing healthcare, it is imperative that we navigate the ethical landscape with caution. By prioritizing data privacy, informed consent, and the prevention of discrimination, we can harness the benefits of this technology while minimizing its risks. As we look to the future, ongoing dialogue among stakeholders—including researchers, healthcare providers, ethicists, and patients—will be essential in shaping a responsible approach to AI in genomics.
- What is the main ethical concern regarding AI in genomics?
The primary concern revolves around data privacy and the potential misuse of sensitive genetic information. - How can informed consent be effectively obtained?
Informed consent should involve clear communication about how genomic data will be used, ensuring patients fully understand the implications. - What measures can be taken to protect genomic data?
Measures such as data anonymization and robust security protocols are vital to safeguarding sensitive information.
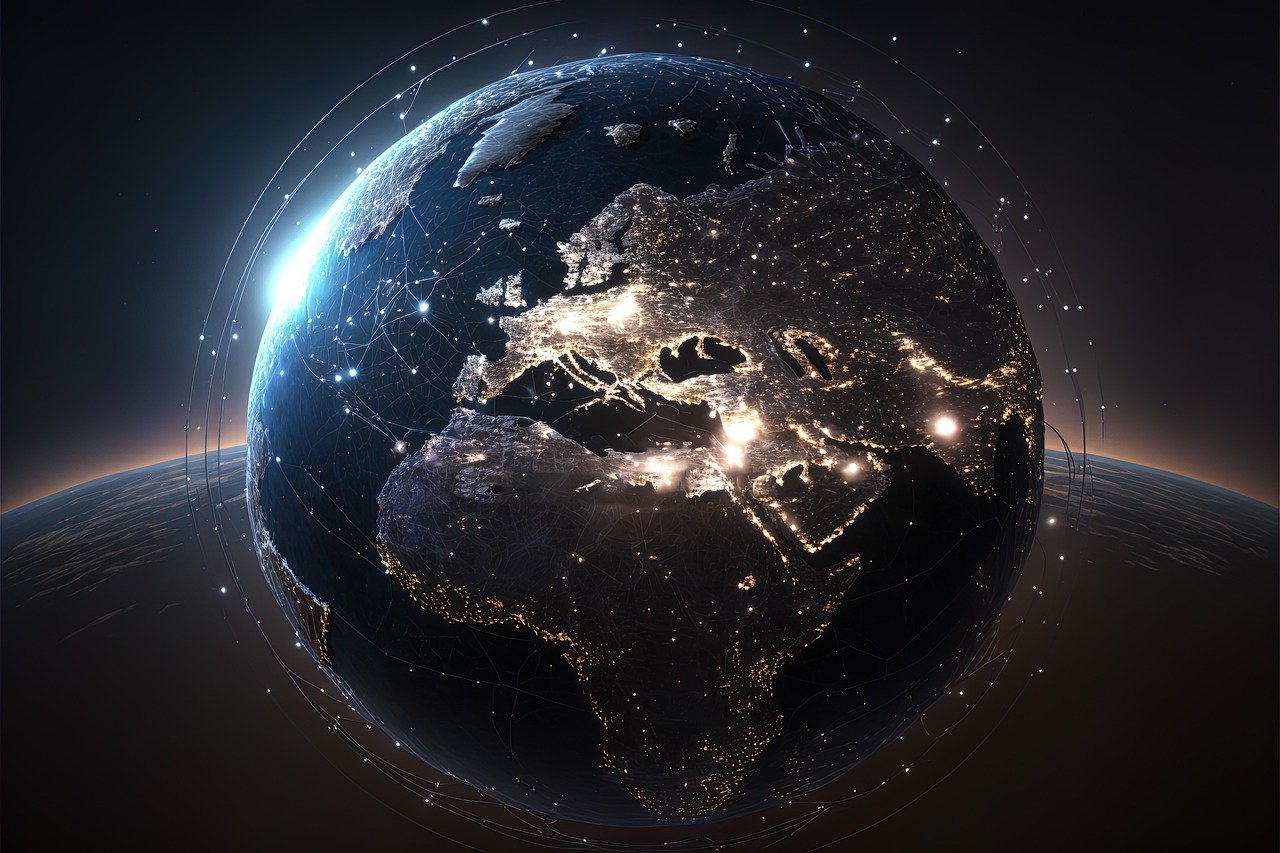
Data Privacy and Security
In the ever-evolving landscape of genomics and healthcare, the integration of artificial intelligence (AI) brings forth remarkable advancements, but it also raises significant concerns regarding . As genomic data is inherently sensitive, containing intricate details about an individual's genetic makeup, the potential for misuse or unauthorized access cannot be overlooked. Imagine your genetic information being treated like a highly classified document—only accessible to those who genuinely need it. This analogy highlights the critical importance of safeguarding such data.
One of the primary challenges in ensuring data privacy in AI-driven genomic research is the sheer volume of information collected. Healthcare providers and researchers often accumulate vast datasets to train AI algorithms, which can inadvertently expose sensitive information if not properly managed. To mitigate these risks, organizations must implement robust security measures that include:
- Encryption: Encrypting genomic data both at rest and in transit ensures that even if data is intercepted, it remains unreadable without the proper decryption keys.
- Access Controls: Limiting access to genomic data to only those individuals who require it for legitimate purposes helps to minimize the risk of unauthorized access.
- Regular Audits: Conducting regular security audits and vulnerability assessments can help organizations identify and address potential weaknesses in their data protection strategies.
Moreover, the ethical implications of genomic data sharing cannot be ignored. Patients often provide their genomic information with the expectation that it will be used responsibly and ethically. This leads to the necessity for clear and transparent communication regarding how their data will be utilized, stored, and shared. Informed consent is not just a checkbox; it is a fundamental aspect of building trust between patients and healthcare providers. Without it, patients may feel hesitant to share their genetic data, ultimately hindering progress in genomic research.
As we look towards the future, the importance of in genomics will only grow. With advancements in AI technology and an increasing number of organizations utilizing genomic data, the demand for stringent privacy measures will become paramount. The challenge lies in balancing the need for data accessibility to drive research and innovation while simultaneously protecting individual rights and privacy. Striking this balance is essential for fostering trust and ensuring that the benefits of AI in genomics are realized without compromising the integrity of personal data.
Ultimately, the conversation surrounding data privacy and security in the realm of AI and genomics is ongoing. Stakeholders, including researchers, healthcare providers, and patients, must collaborate to develop comprehensive policies and practices that prioritize the protection of sensitive genomic information. By doing so, we can harness the full potential of AI in genomics while safeguarding the privacy and security of individuals.
Q1: Why is data privacy important in genomics?
A1: Data privacy is crucial in genomics because it involves sensitive information about an individual's genetic makeup. Protecting this data is essential to prevent misuse and maintain patient trust.
Q2: What measures can organizations take to secure genomic data?
A2: Organizations can implement encryption, access controls, and regular audits to safeguard genomic data from unauthorized access and breaches.
Q3: How does informed consent relate to genomic data usage?
A3: Informed consent ensures that patients are fully aware of how their genomic data will be used and shared, fostering trust and ethical practices in research.
Q4: What are the potential consequences of poor data security in genomics?
A4: Poor data security can lead to unauthorized access, data breaches, and loss of patient trust, ultimately hindering research and advancements in healthcare.
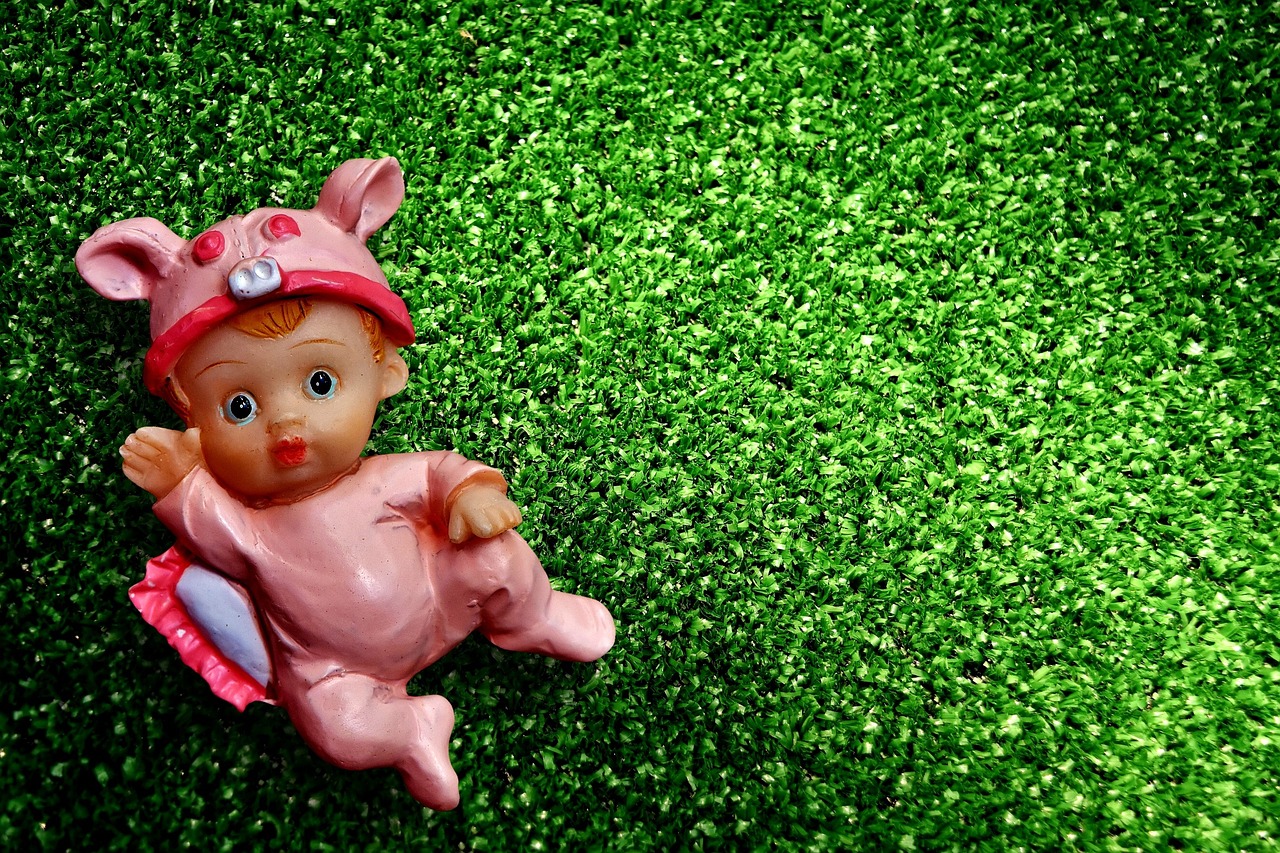
Informed Consent in Genomics
Informed consent is a fundamental principle in the realm of genomics, ensuring that individuals understand what they are agreeing to when their genetic information is collected, analyzed, and utilized. As we dive deeper into the world of artificial intelligence (AI) in genomics, the complexities surrounding informed consent become even more pronounced. It's not just about signing a piece of paper; it's about empowering individuals with knowledge and understanding the implications of their genetic data.
One of the primary challenges in obtaining informed consent lies in the intricate nature of genomic data itself. Unlike traditional medical data, genomic information can reveal not just an individual’s health risks but also insights about their family members. This raises questions about privacy and the extent to which individuals can control their genetic information. For instance, if one person consents to share their genomic data, what happens if it inadvertently exposes their relatives to potential risks?
Moreover, the rapid advancements in AI technology mean that the applications of genomic data are continuously evolving. This creates a scenario where consent given today may become outdated tomorrow. Individuals must be made aware that their genomic data could be used for purposes not initially envisioned at the time of consent. Therefore, it is crucial for researchers and healthcare providers to communicate transparently about:
- The specific uses of genomic data
- Potential risks and benefits
- How long the data will be stored and used
- Who will have access to the data
Effective communication is key. Researchers must strive to present information in a way that is not only comprehensive but also digestible for individuals without a scientific background. This means avoiding jargon and using analogies that resonate with everyday experiences. For example, one might compare genomic data sharing to sharing a personal diary; it's essential to understand who might read it and how it could affect relationships.
Furthermore, the ethical implications of genomic data use extend beyond individual consent. Societal considerations come into play, especially concerning issues of equity and access to genomic technologies. If certain populations are underrepresented in genomic research, it can lead to biased outcomes and perpetuate health disparities. Thus, informed consent must also encompass discussions about inclusivity and fairness in genomic studies.
In conclusion, informed consent in genomics is a dynamic and multifaceted issue that requires ongoing dialogue between researchers, healthcare providers, and the public. As AI continues to shape the landscape of genomic research, fostering a culture of transparency and ethical responsibility will be paramount. Only then can we ensure that individuals feel confident and secure in their decisions regarding their genomic data.
- What is informed consent in genomics?
Informed consent in genomics refers to the process by which individuals are educated about the implications of sharing their genetic data and agree to its use in research or clinical applications. - Why is informed consent important?
It ensures that individuals understand the risks and benefits of sharing their genomic information, thus empowering them to make informed decisions about their participation in genomic research. - What challenges are associated with obtaining informed consent?
Challenges include the complexity of genomic data, the evolving nature of AI applications, and the need for clear communication to non-experts. - How can researchers improve the informed consent process?
Researchers can improve the process by using clear language, providing comprehensive information, and ensuring transparency about data usage and potential risks.
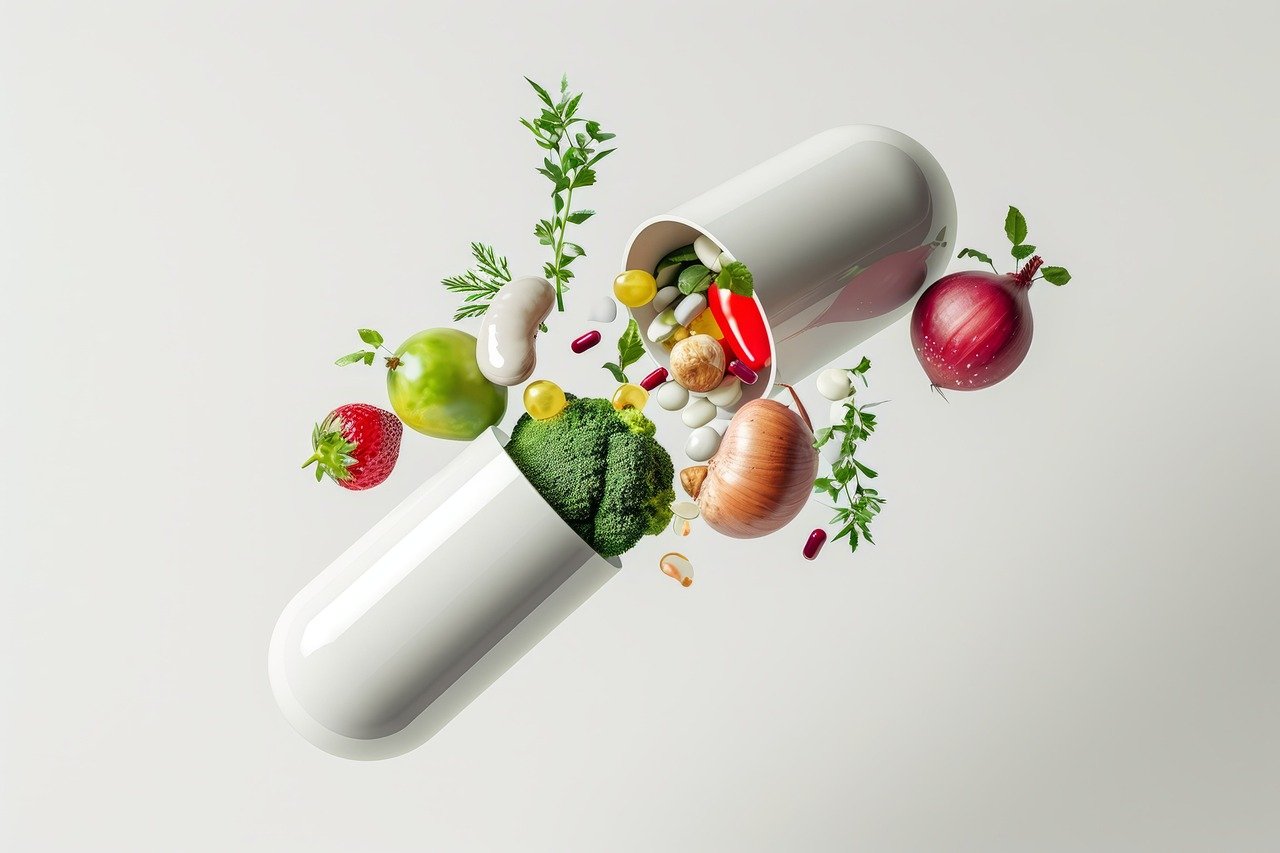
The Future of AI in Healthcare
The future of artificial intelligence in healthcare is not just bright; it's bursting with potential! As technology continues to advance at a breakneck pace, we stand on the cusp of a healthcare revolution that promises to enhance patient care, streamline processes, and improve outcomes like never before. Imagine a world where diseases are diagnosed with pinpoint accuracy, treatments are tailored to individual genetic profiles, and healthcare professionals are equipped with advanced tools to make informed decisions. Sounds incredible, right?
One of the most exciting aspects of AI's future in healthcare is its ability to facilitate predictive analytics. By harnessing vast amounts of data from various sources, including electronic health records, genomic data, and even social determinants of health, AI algorithms can identify trends and predict potential health issues before they arise. This proactive approach could lead to a paradigm shift from reactive to preventive care, ultimately saving lives and reducing healthcare costs.
Moreover, as AI technology evolves, we can expect to see more sophisticated machine learning models that can analyze complex data sets with greater accuracy. For instance, consider the role of AI in drug discovery. Traditional methods can take years and cost billions, but AI can significantly accelerate the process by predicting how different compounds will interact with biological systems. Imagine being able to bring life-saving drugs to market faster, ultimately benefiting patients who need them the most!
Another area ripe for innovation is the integration of AI-powered telemedicine. With the rise of remote healthcare services, AI can enhance virtual consultations by providing real-time data analysis and decision support to healthcare providers. This means that even from a distance, doctors can access vital patient information, analyze symptoms, and make informed decisions, all while improving the patient experience. It’s like having a personal health assistant right in your pocket!
However, with all this potential comes challenges. As we look to the future, the healthcare industry must address critical issues such as data privacy and security. The sensitive nature of health data requires robust protection measures to ensure patient confidentiality. Striking the right balance between innovation and privacy will be essential for building trust and ensuring that AI technologies are embraced rather than feared.
In addition, the ethical implications of AI in healthcare cannot be overlooked. Questions surrounding informed consent, algorithmic bias, and equitable access to AI-driven solutions must be addressed to create a fair and just healthcare system. Engaging stakeholders—from patients to policymakers—in discussions about these issues will be crucial for navigating the future landscape of AI in healthcare.
In conclusion, the future of AI in healthcare is not just about technological advancements; it’s about enhancing the human experience in medicine. As we continue to explore the possibilities, one thing is clear: AI has the potential to transform healthcare into a more efficient, personalized, and equitable system. The journey ahead may be challenging, but the rewards for patients and healthcare providers alike are immeasurable.
- What are some potential benefits of AI in healthcare?
AI can enhance diagnostic accuracy, streamline administrative tasks, and enable personalized treatment plans based on genetic data. - How does AI contribute to preventive care?
AI analyzes data to identify health trends and predict potential issues, allowing for early interventions and better health outcomes. - What are the ethical concerns surrounding AI in healthcare?
Key concerns include data privacy, informed consent, and the risk of algorithmic bias affecting patient care. - Will AI replace healthcare professionals?
No, AI is designed to assist healthcare professionals, enhancing their capabilities rather than replacing them.
Frequently Asked Questions
- What is the role of AI in genomics?
AI plays a transformative role in genomics by enabling faster data analysis, improving gene editing techniques, and facilitating personalized medicine. It helps researchers and healthcare professionals analyze complex genomic data, leading to better understanding and treatment of genetic disorders.
- How does AI enhance disease diagnosis?
AI enhances disease diagnosis by analyzing genomic data to identify genetic disorders and predict disease susceptibility. This allows for early intervention and treatment, significantly improving patient outcomes.
- What machine learning techniques are used in genomics?
Popular machine learning techniques in genomics include decision trees, support vector machines, and neural networks. These algorithms help uncover patterns in complex data, aiding in diagnosis and treatment planning.
- What is deep learning, and why is it important in genomics?
Deep learning is a subset of machine learning that uses neural networks with many layers to analyze vast datasets. In genomics, it is crucial for understanding genetic variations and their clinical significance, leading to more accurate diagnoses and personalized treatments.
- How does natural language processing contribute to genomics?
Natural language processing (NLP) aids in extracting insights from unstructured genomic data by enabling literature mining and knowledge discovery. This helps researchers stay updated with the latest findings and integrate them into their work.
- What are the ethical considerations surrounding AI in genomics?
Ethical considerations include data privacy, informed consent, and the implications of genetic information on individuals and society. It is essential to address these concerns to maintain trust and ensure responsible use of AI in healthcare.
- How is patient data privacy protected in AI-driven genomic research?
To protect patient data privacy, strict measures such as data anonymization, encryption, and secure storage are implemented. Researchers must adhere to regulations and ethical standards to ensure that sensitive information remains confidential.
- Why is informed consent important in genomic research?
Informed consent is crucial because it ensures that patients understand how their genomic data will be used, the potential risks, and benefits. Transparent communication fosters trust and allows individuals to make educated decisions about their participation in research.
- What does the future hold for AI in healthcare?
The future of AI in healthcare looks promising, with ongoing advancements in technology expected to enhance patient care and research capabilities. Innovations in AI could lead to more accurate diagnostics, improved treatment options, and better overall health outcomes.