How AI can Revolutionize Future Performance Metrics
In today's fast-paced world, the demand for efficient performance metrics has never been more critical. Organizations across various sectors are constantly seeking ways to enhance their performance evaluations, and that's where artificial intelligence (AI) comes into play. Imagine a scenario where businesses can not only gather data at lightning speed but also analyze it to uncover hidden insights that traditional methods might miss. Sounds like a dream, right? Well, it's becoming a reality! This article dives deep into the transformative potential of AI in reshaping how we measure and interpret performance metrics. We will explore the methodologies, benefits, and future implications of integrating AI into performance evaluation processes, making it a game-changer for organizations aiming for excellence.
AI is revolutionizing the way organizations approach data collection. Gone are the days of tedious manual processes and the risk of human error. With AI, companies can harness powerful tools and techniques that not only streamline data collection but also enhance its accuracy and relevance. For instance, AI algorithms can sift through vast amounts of data in real-time, identifying patterns and anomalies that would take humans much longer to detect. This means businesses can make informed decisions based on reliable data, ultimately leading to improved performance metrics.
Once data is collected, the next step is analysis, and this is where machine learning algorithms shine. These sophisticated algorithms are designed to analyze performance data in ways that traditional methods simply cannot. They identify trends, correlations, and patterns that help organizations understand their performance at a deeper level. For example, a retail company might use machine learning to analyze sales data, uncovering insights about customer preferences and purchasing behaviors. This level of analysis enables businesses to tailor their strategies effectively, ensuring they remain competitive in their respective markets.
One of the most exciting applications of AI in performance metrics is predictive analytics. By utilizing historical data, predictive models can forecast future performance, allowing organizations to make proactive decisions. Imagine a manufacturing company that can predict equipment failures before they happen, saving time and resources. This capability is invaluable for strategic planning and decision-making. Companies that leverage predictive analytics can stay ahead of the curve, adapting their strategies based on anticipated trends and challenges.
In a world where time is money, real-time data processing is a game changer. Organizations can now respond to performance changes almost instantaneously, thanks to advancements in technology. Real-time analytics tools enable businesses to monitor their performance metrics continuously, allowing for quick adjustments and improvements. This agility can be the difference between success and failure in today’s competitive landscape. Imagine being able to tweak a marketing campaign on the fly based on real-time customer feedback—this is the power of AI!
While the benefits of predictive analytics are clear, implementing these systems is not without its challenges. Organizations often face obstacles such as data quality issues, integration difficulties, and a lack of skilled personnel. However, by acknowledging these challenges and developing strategies to overcome them, businesses can successfully implement predictive analytics. For instance, investing in training for staff and ensuring data integrity can significantly improve the effectiveness of predictive models.
AI is also making waves in the realm of employee performance tracking. By providing personalized insights, AI tools empower managers to assess and enhance both individual and team performance effectively. Imagine a scenario where an employee receives tailored feedback based on their specific strengths and weaknesses. This personalized approach not only boosts morale but also drives productivity. With AI, organizations can create a more engaged and motivated workforce, ultimately leading to better performance metrics.
Another significant advantage of AI is its ability to facilitate continuous performance evaluations. AI-driven feedback systems foster a culture of growth and development within organizations. Instead of waiting for annual reviews, employees receive ongoing feedback that helps them improve continuously. This shift not only enhances individual performance but also contributes to a more dynamic and responsive organizational culture.
The key to effective performance improvement lies in customized feedback. AI excels in tailoring feedback to meet the unique needs of each employee. By analyzing individual performance data, AI can provide specific recommendations that resonate with employees on a personal level. This personalized feedback encourages a growth mindset, motivating employees to strive for excellence.
Finally, integrating AI with current performance metrics can significantly enhance their effectiveness. Organizations can take steps to combine traditional and AI-driven approaches seamlessly. By doing so, they can create a comprehensive performance evaluation system that leverages the strengths of both methodologies. This integration not only improves accuracy but also provides a more holistic view of performance metrics, enabling better decision-making.
- What is the role of AI in performance metrics? AI enhances data collection, analysis, and feedback processes, leading to more accurate and actionable performance evaluations.
- How does predictive analytics work? Predictive analytics uses historical data to forecast future performance, helping organizations make proactive decisions.
- What are the challenges of implementing AI in performance metrics? Common challenges include data quality issues, integration difficulties, and a lack of skilled personnel.
- Can AI improve employee performance tracking? Yes, AI provides personalized insights that help managers assess and enhance employee performance effectively.
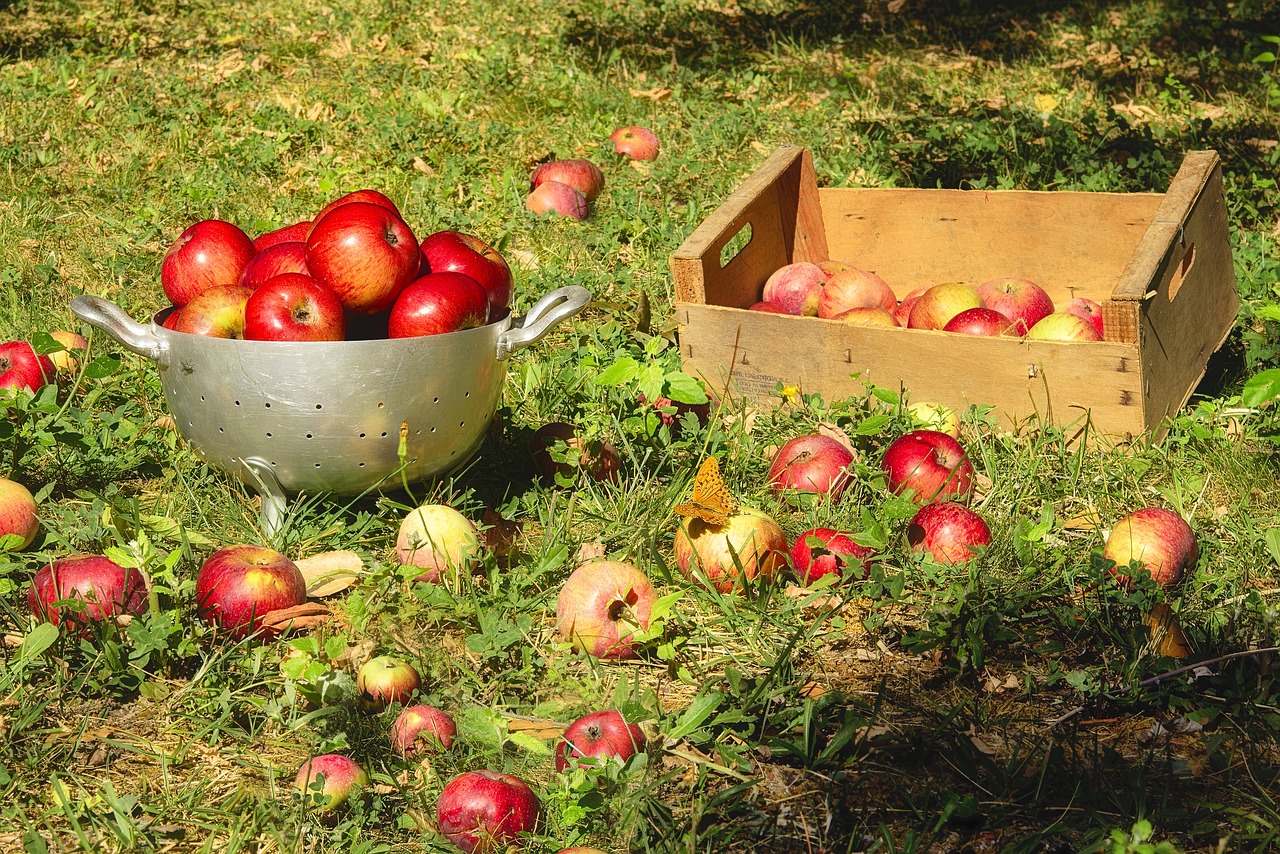
The Role of AI in Data Collection
In today's fast-paced world, the way we gather and analyze data has undergone a radical transformation, thanks to artificial intelligence (AI). Imagine trying to sift through mountains of data manually—it's like searching for a needle in a haystack! AI streamlines this process, enabling organizations to collect vast amounts of information with remarkable efficiency and accuracy. By employing advanced algorithms and machine learning techniques, AI can automate data collection, ensuring that the information gathered is not only extensive but also relevant and precise.
One of the key benefits of AI in data collection is its ability to process information from multiple sources simultaneously. For example, organizations can gather data from:
- Social media platforms
- Customer feedback and surveys
- Website analytics
- Sales and transaction records
This multifaceted approach allows businesses to paint a comprehensive picture of their operations and customer behavior. Moreover, AI tools can filter out noise and focus on the data that truly matters, enhancing the overall quality of insights derived from the collected data.
Another exciting aspect of AI in data collection is its capability to learn and adapt over time. As AI systems are exposed to more data, they improve their accuracy and relevance, allowing organizations to make informed decisions based on the most current and pertinent information available. This not only saves time but also reduces the likelihood of human error, which can often skew results when data is collected manually.
Furthermore, AI can assist in identifying trends and patterns that might not be immediately visible through traditional data collection methods. For instance, through natural language processing (NLP), AI can analyze text data from customer reviews or social media posts to uncover sentiments and opinions that can influence business strategies. This capability creates a feedback loop where organizations can continuously refine their data collection processes based on the insights gained.
In summary, the role of AI in data collection is transformative. By automating processes, enhancing accuracy, and revealing hidden insights, AI empowers organizations to make better, data-driven decisions. As we move forward, the integration of AI into data collection methodologies will likely become a standard practice across various industries, paving the way for more informed strategies and improved performance metrics.
Q1: How does AI improve data accuracy?
A1: AI enhances data accuracy by automating the collection process, reducing human error, and using advanced algorithms to filter and analyze relevant information.
Q2: What types of data can AI collect?
A2: AI can collect a wide range of data, including customer feedback, social media interactions, website analytics, and sales records, among others.
Q3: Can AI adapt to new data sources?
A3: Yes, AI systems can learn and adapt over time, improving their ability to process and analyze new data sources as they become available.

Machine Learning Algorithms for Analysis
In today's fast-paced digital world, machine learning algorithms have become the backbone of effective data analysis. These algorithms are designed to learn from data, identify patterns, and make decisions with minimal human intervention. Imagine having a super-smart assistant who not only sorts through mountains of data but also picks out the most relevant insights for you. That's precisely what machine learning does for performance metrics!
One of the most exciting aspects of machine learning is its ability to uncover hidden trends that traditional analysis methods often miss. For instance, while a human analyst might spend hours poring over spreadsheets, a machine learning model can process vast datasets in seconds, pinpointing anomalies and correlations that would take ages to discover manually. This capability is particularly valuable in sectors like finance, healthcare, and marketing, where understanding performance metrics can directly influence strategic decisions.
But how does it work? At its core, machine learning relies on algorithms that can be categorized into several types, including supervised learning, unsupervised learning, and reinforcement learning. Each of these approaches has its unique applications:
- Supervised Learning: This involves training a model on a labeled dataset, where the outcome is known. For example, in employee performance analysis, a model can learn from past performance reviews to predict future outcomes.
- Unsupervised Learning: Here, the model works with unlabeled data to find patterns. This is useful for clustering employees into performance groups based on various metrics without predefined categories.
- Reinforcement Learning: This type of learning is about making decisions based on feedback. It’s akin to teaching a dog new tricks—rewarding the right behavior leads to better performance over time.
Moreover, machine learning algorithms continuously improve as they process more data. This characteristic is akin to a fine wine maturing over time; the more data the algorithms consume, the better they become at making accurate predictions. For example, an algorithm analyzing sales performance can adjust its predictions based on seasonal trends, customer behavior, and market shifts, providing organizations with a dynamic tool for performance management.
However, it's essential to note that the effectiveness of these algorithms largely depends on the quality of the data fed into them. Garbage in, garbage out, as the saying goes. Organizations must ensure that their data is clean, relevant, and comprehensive to reap the full benefits of machine learning. This might involve investing in data cleansing tools and processes to eliminate inconsistencies and inaccuracies.
In summary, the application of machine learning algorithms in analyzing performance metrics is not just a trend—it's a revolution. By leveraging these powerful tools, organizations can gain deeper insights, make informed decisions, and ultimately drive better performance outcomes. The future of performance analysis is here, and it’s powered by machine learning!
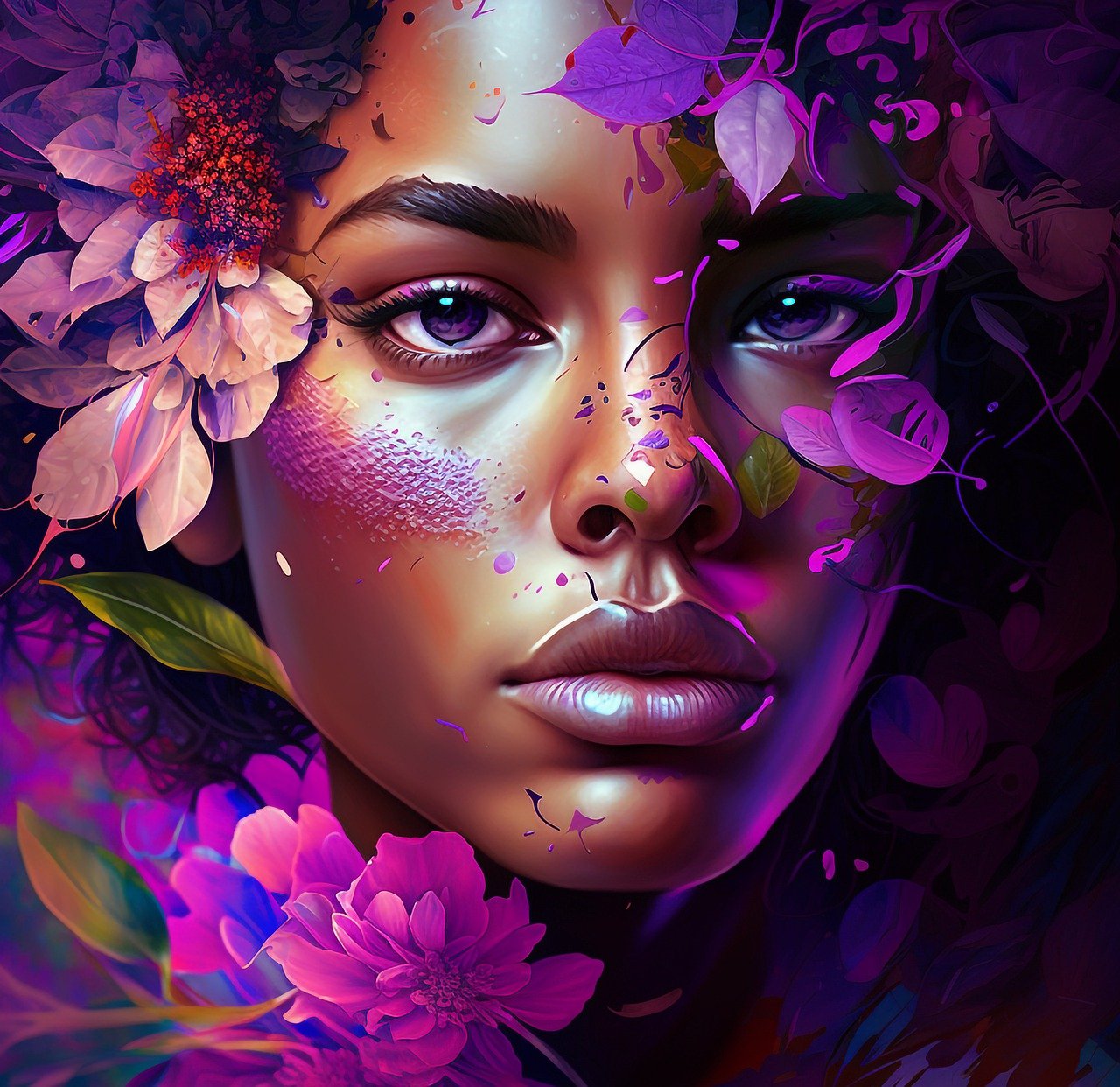
Predictive Analytics in Performance Metrics
Predictive analytics is like having a crystal ball for performance metrics. By leveraging historical data, organizations can anticipate future outcomes with remarkable accuracy. Imagine being able to foresee potential challenges before they arise or identifying opportunities that could propel your business forward. This is the power of predictive analytics, and it’s transforming how companies strategize and make decisions.
At its core, predictive analytics involves the use of statistical algorithms and machine learning techniques. These tools sift through vast amounts of data, uncovering hidden patterns that might otherwise go unnoticed. For instance, a retail company could analyze past sales data to predict future trends, enabling them to stock up on popular items before demand surges. This proactive approach not only boosts sales but also enhances customer satisfaction by ensuring that products are available when customers want them.
One of the significant benefits of predictive analytics in performance metrics is its ability to inform strategic planning. Organizations can use predictive models to evaluate various scenarios and their potential impact on performance. This means decision-makers can weigh their options more effectively, choosing strategies that are likely to yield the best results. For example, a marketing team might use predictive analytics to determine which campaigns are likely to resonate with their audience, allowing them to allocate resources more efficiently.
However, predictive analytics is not without its challenges. Organizations must ensure that they have high-quality data to feed into their models. Poor data quality can lead to inaccurate predictions, which can be detrimental to decision-making processes. Additionally, there is often a steep learning curve associated with implementing these advanced analytics tools. Companies may need to invest in training their staff or hiring data scientists to harness the full potential of predictive analytics.
To illustrate the impact of predictive analytics, consider the following table that outlines its applications across different sectors:
Sector | Application | Benefits |
---|---|---|
Retail | Demand Forecasting | Improved inventory management and customer satisfaction |
Healthcare | Patient Outcome Prediction | Enhanced treatment plans and resource allocation |
Finance | Risk Assessment | Minimized financial losses and better investment strategies |
Manufacturing | Predictive Maintenance | Reduced downtime and maintenance costs |
In conclusion, predictive analytics is a game-changer for performance metrics. By harnessing the power of historical data and advanced algorithms, organizations can not only anticipate future trends but also make informed decisions that drive success. As we move forward, embracing predictive analytics will be crucial for companies aiming to stay ahead of the competition and foster a culture of continuous improvement.
- What is predictive analytics? Predictive analytics uses historical data and statistical algorithms to forecast future outcomes.
- How can predictive analytics benefit my organization? It can enhance decision-making, improve resource allocation, and increase efficiency by anticipating future trends.
- What challenges are associated with implementing predictive analytics? Common challenges include data quality issues and the need for specialized skills to interpret the data.
- Can predictive analytics be applied in any industry? Yes, predictive analytics can be utilized across various sectors, including retail, healthcare, finance, and manufacturing.
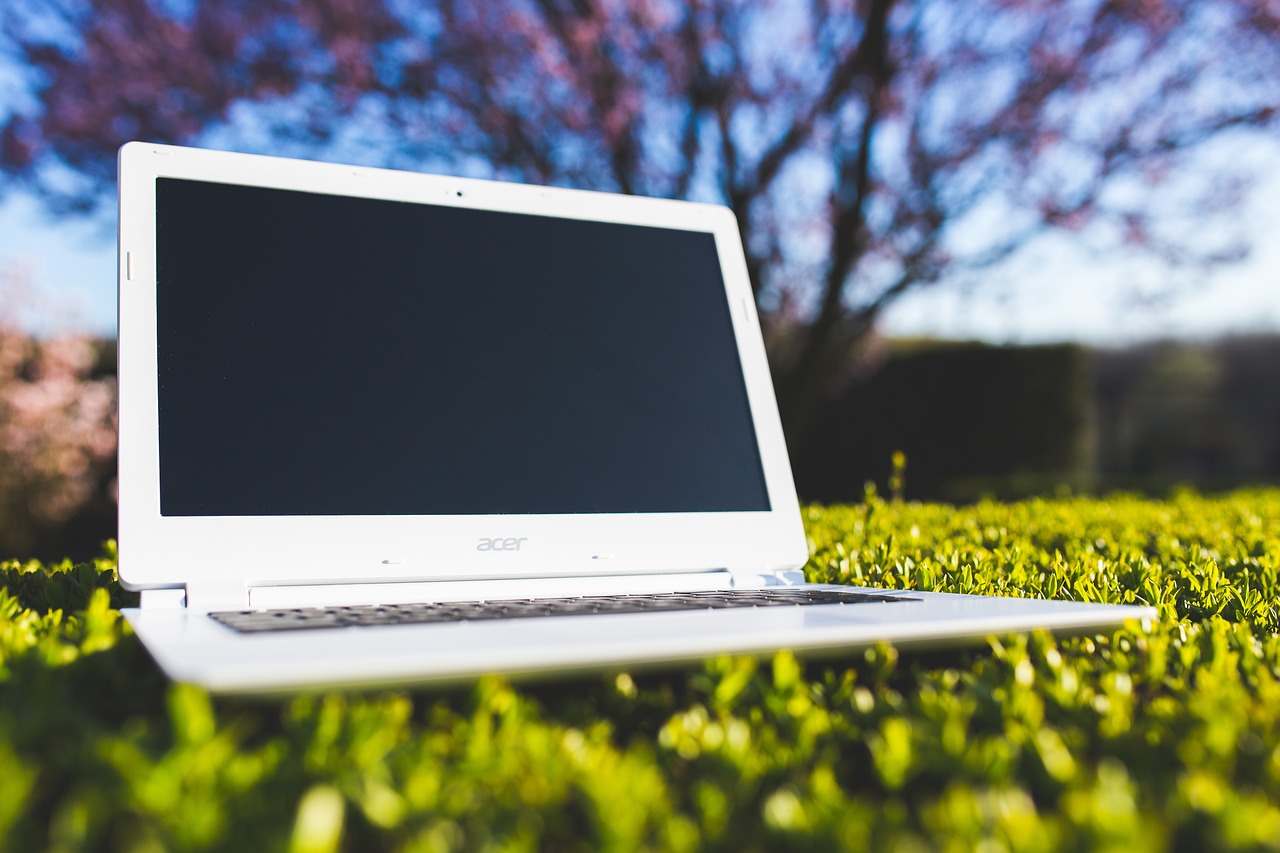
Real-Time Data Processing
In today's fast-paced world, the ability to process data in real-time is not just a luxury; it's a necessity. Imagine being able to make decisions based on the most current information available, rather than relying on outdated reports. empowers organizations to respond to performance changes instantly, which can be a game-changer in competitive industries. This capability is made possible through advanced technologies that leverage artificial intelligence, machine learning, and big data analytics.
One of the most significant benefits of real-time data processing is its impact on decision-making. Organizations can monitor key performance indicators (KPIs) as they happen, allowing for immediate adjustments to strategies and operations. For instance, in a retail setting, real-time data can help managers identify which products are selling well and which are not, enabling them to make quick inventory decisions. This immediate insight can lead to increased sales and improved customer satisfaction.
Technologies such as stream processing and event-driven architecture are at the forefront of enabling real-time data processing. These technologies allow data to be processed as it arrives, rather than waiting for batch processing at scheduled intervals. This not only speeds up the analysis but also enhances the accuracy of the insights derived from the data. For example, a company can use real-time analytics to track customer behavior on their website, adjusting marketing strategies on-the-fly based on user interactions.
Furthermore, real-time data processing facilitates a more proactive approach to performance management. Organizations can set up alerts that trigger when certain thresholds are met, allowing teams to address issues before they escalate. This proactive stance can significantly reduce downtime and improve overall efficiency. Consider a manufacturing plant that monitors equipment performance in real-time; if sensors detect an anomaly, the system can alert maintenance personnel immediately, preventing costly breakdowns.
However, implementing real-time data processing is not without its challenges. Organizations must invest in the right infrastructure and tools to handle the influx of data effectively. This often includes robust data storage solutions, powerful analytics tools, and skilled personnel who can interpret the data correctly. Additionally, ensuring data security and compliance with regulations is paramount, as real-time processing often involves sensitive information.
In summary, real-time data processing is a transformative capability that allows organizations to stay agile and responsive in an ever-changing environment. By harnessing the power of AI and advanced technologies, businesses can not only enhance their performance metrics but also create a culture of continuous improvement. As we move forward, the organizations that embrace real-time data processing will likely lead the pack, setting new standards for efficiency and effectiveness.
- What is real-time data processing? Real-time data processing refers to the immediate processing and analysis of data as it is generated, allowing organizations to make timely decisions based on current information.
- What are the benefits of real-time data processing? Key benefits include improved decision-making, enhanced operational efficiency, proactive performance management, and increased customer satisfaction.
- What technologies enable real-time data processing? Technologies such as stream processing, event-driven architecture, and advanced analytics tools are essential for effective real-time data processing.
- What challenges are associated with implementing real-time data processing? Challenges include the need for robust infrastructure, data security, compliance with regulations, and the requirement for skilled personnel to interpret the data.

Challenges in Implementing Predictive Analytics
Implementing predictive analytics can be a game-changer for organizations looking to enhance their performance metrics, but it doesn't come without its fair share of challenges. One of the primary hurdles is the quality of data. If the data collected is inaccurate or incomplete, the insights generated will be flawed, leading to poor decision-making. Organizations must invest in robust data collection and management systems to ensure the integrity of their data.
Another significant challenge is staff resistance to adopting new technologies. Employees may feel threatened by advanced analytics, fearing that their jobs could be at risk or that they lack the skills to work with these tools. To combat this, companies should focus on training and education, helping employees understand the benefits of predictive analytics and how it can complement their roles rather than replace them.
Furthermore, integrating predictive analytics into existing systems can be a daunting task. Organizations often face technical barriers when trying to merge new analytics tools with legacy systems. This can lead to data silos, where information is trapped in various departments and not easily accessible for analysis. To overcome this, it's essential to have a clear integration strategy that outlines how new tools will work with existing infrastructures.
Additionally, there are resource constraints to consider. Not all organizations have the budget to invest in advanced analytics tools or hire data scientists. This can limit their ability to fully leverage predictive analytics. To address this, organizations can explore cloud-based solutions that offer scalable analytics capabilities without the need for significant upfront investments.
Lastly, the interpretation of results can pose a challenge. Even when organizations successfully implement predictive analytics, understanding what the data is telling them can be complex. It's crucial to have skilled analysts who can translate data insights into actionable strategies. This means investing in training for existing staff or hiring new talent with the necessary expertise.
In summary, while the potential of predictive analytics is immense, organizations must navigate several challenges to implement it successfully. Focusing on data quality, employee training, system integration, resource allocation, and effective interpretation of results can pave the way for a smoother transition into the world of predictive analytics.
- What is predictive analytics? Predictive analytics is a branch of advanced analytics that uses historical data, statistical algorithms, and machine learning techniques to identify the likelihood of future outcomes based on historical data.
- How can organizations benefit from predictive analytics? Organizations can make data-driven decisions, improve operational efficiency, enhance customer satisfaction, and gain a competitive advantage by anticipating trends and behaviors.
- What are the key components of a successful predictive analytics strategy? Key components include high-quality data, skilled personnel, proper integration with existing systems, and a commitment to continuous learning and adaptation.
- Is predictive analytics suitable for all industries? Yes, predictive analytics can be applied across various industries, including healthcare, finance, retail, and manufacturing, to improve decision-making and performance metrics.
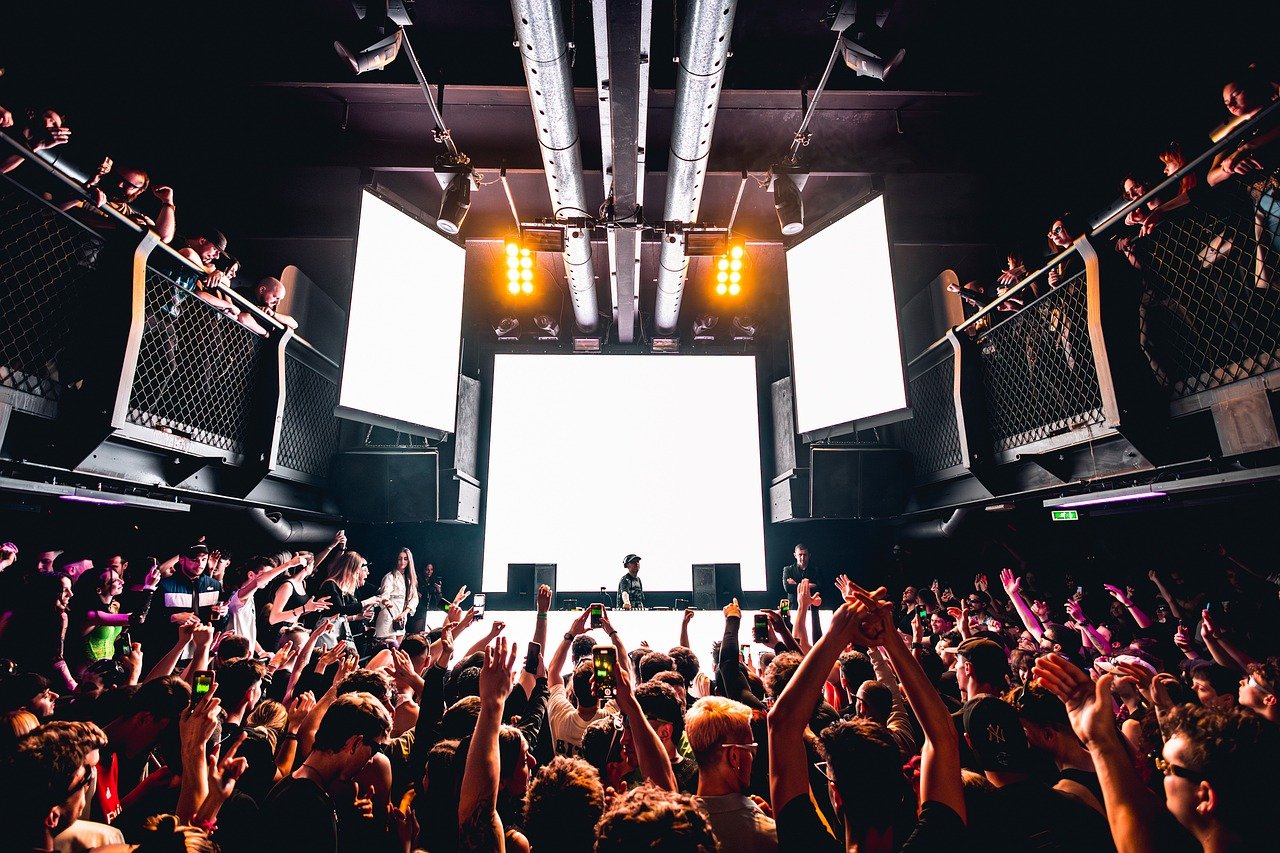
Improving Employee Performance Tracking
In today's fast-paced work environment, tracking employee performance has become more than just a checkbox exercise; it’s a critical component for driving organizational success. Traditional methods often relied on annual reviews and subjective assessments, which, let’s be honest, can feel outdated and ineffective. Enter artificial intelligence (AI)—the game-changer that’s transforming how we monitor and enhance employee performance. Imagine having a tool that not only tracks performance metrics but also provides personalized insights tailored to each employee's unique strengths and weaknesses. Sounds like a dream, right?
AI-powered tools can analyze a myriad of data points, from project completion rates to team collaboration metrics. This means managers can gain a comprehensive view of an employee's performance in real-time. For instance, platforms like Performance Pro and 15Five utilize AI to provide continuous feedback, enabling managers to identify areas for improvement as they arise rather than waiting for a quarterly review. This proactive approach not only boosts productivity but also enhances employee satisfaction by fostering a culture of open communication and growth.
One of the most exciting aspects of AI in performance tracking is its ability to deliver customized feedback. Instead of generic evaluations, AI systems can analyze individual performance data and suggest specific areas for improvement. For example, if an employee excels in project management but struggles with time management, the AI can recommend targeted training programs or resources. This level of personalization is invaluable; it transforms feedback from a one-size-fits-all approach into a tailored roadmap for success.
Moreover, AI-driven performance tracking can also facilitate team assessments. By analyzing group dynamics and collaboration efforts, AI can provide insights into team performance that help managers understand how to better support their teams. This holistic view can be instrumental in identifying high-performing teams and those that may need additional resources or training. As a result, organizations can allocate their resources more effectively, ensuring that every team has what it needs to thrive.
However, it’s essential to acknowledge that while AI enhances performance tracking, it doesn’t replace the human element. Managers still play a crucial role in interpreting data and fostering a supportive environment. AI simply acts as a tool that augments their capabilities, enabling them to make more informed decisions. Therefore, the best results come from a harmonious blend of AI insights and human intuition.
In summary, AI is revolutionizing employee performance tracking by offering real-time insights, personalized feedback, and a comprehensive view of both individual and team performance. As organizations continue to embrace these technologies, they will not only enhance productivity but also create a more engaged and motivated workforce.
- How does AI improve employee performance tracking?
AI analyzes vast amounts of data to provide real-time insights and personalized feedback, enabling managers to monitor performance effectively. - Can AI replace traditional performance reviews?
No, AI complements traditional reviews by providing continuous feedback and insights, but human judgment remains essential. - What are some examples of AI tools for performance tracking?
Tools like Performance Pro and 15Five utilize AI to enhance performance tracking and feedback processes. - Is employee privacy a concern with AI performance tracking?
Yes, organizations must ensure that they handle employee data responsibly and transparently to maintain trust.
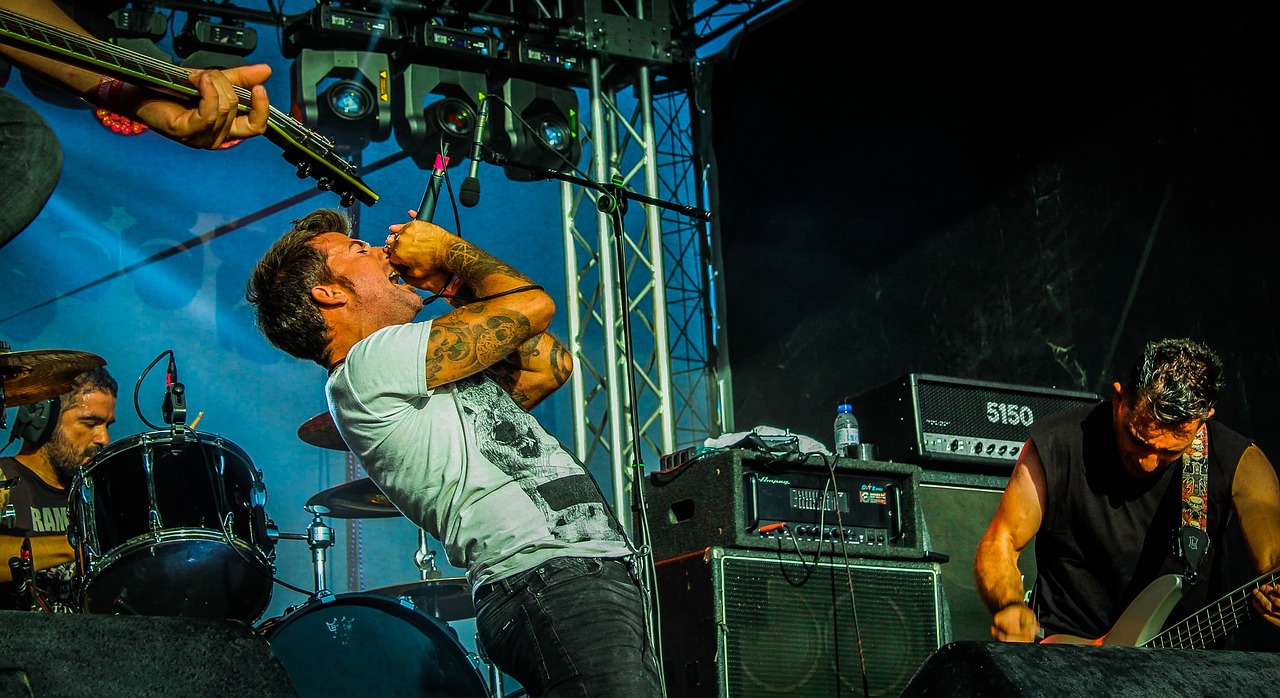
AI-Driven Performance Feedback Systems
In today's fast-paced business environment, are becoming essential tools for organizations aiming to foster a culture of continuous improvement. These systems leverage advanced algorithms to provide real-time, actionable insights into employee performance, allowing managers to make informed decisions that propel growth and development. Imagine a world where feedback is not just a periodic event but a constant flow of valuable information tailored to each individual's strengths and weaknesses. This is the promise of AI in performance management.
One of the most significant advantages of AI-driven feedback systems is their ability to analyze vast amounts of data quickly and accurately. By utilizing machine learning algorithms, these systems can identify patterns in performance metrics that human analysts might miss. For instance, an AI system can detect that an employee consistently excels in project management but struggles with time management. With this insight, managers can provide targeted support, such as time management training or tools, to help the employee improve.
Moreover, AI-driven feedback systems can enhance employee engagement by making feedback more relevant and personalized. Instead of generic evaluations, employees receive specific insights that resonate with their unique roles and responsibilities. This personalization can significantly boost morale and motivation, creating a workforce that is not only more productive but also more satisfied with their jobs. Feedback becomes a two-way street, where employees feel heard and valued, leading to a more cohesive workplace environment.
Another exciting aspect of these systems is their ability to integrate with existing performance metrics, providing a more holistic view of employee performance. Organizations can combine traditional metrics—like sales numbers or project completion rates—with AI-driven insights to create a comprehensive performance profile for each employee. This integration not only enhances the effectiveness of performance evaluations but also helps in setting realistic and achievable goals.
To illustrate the impact of AI-driven feedback systems, consider the following table that outlines key benefits:
Benefit | Description |
---|---|
Real-Time Feedback | Employees receive immediate insights into their performance, allowing for quick adjustments and improvements. |
Personalization | Feedback is tailored to individual needs, enhancing relevance and engagement. |
Data-Driven Insights | AI analyzes performance data to uncover trends and patterns that inform strategic decisions. |
Continuous Improvement | Fosters a culture of ongoing development, where feedback is part of everyday work life. |
However, it's essential to recognize that implementing AI-driven performance feedback systems does come with its challenges. Organizations must ensure that they have the right infrastructure in place to support these technologies. This includes investing in training for both managers and employees to understand how to interpret and act on AI-generated feedback effectively. Additionally, there may be concerns around data privacy and the ethical use of employee information, which organizations must address proactively.
In conclusion, AI-driven performance feedback systems are revolutionizing the way organizations approach employee evaluations. By providing real-time, personalized insights, these systems not only enhance performance management but also contribute to a more engaged and motivated workforce. As technology continues to evolve, the integration of AI in performance feedback will undoubtedly become a standard practice, paving the way for a future where feedback is not just a formality but a vital component of organizational success.
- What is an AI-driven performance feedback system?
It is a technology that uses artificial intelligence to analyze employee performance data and provide real-time, personalized feedback. - How can AI improve employee engagement?
By offering tailored feedback that resonates with individual roles, AI can make employees feel valued and motivated. - Are there any challenges in implementing these systems?
Yes, challenges include ensuring proper infrastructure, training, and addressing data privacy concerns. - Can AI feedback systems integrate with existing performance metrics?
Absolutely! They can enhance traditional metrics by providing a more comprehensive view of performance.
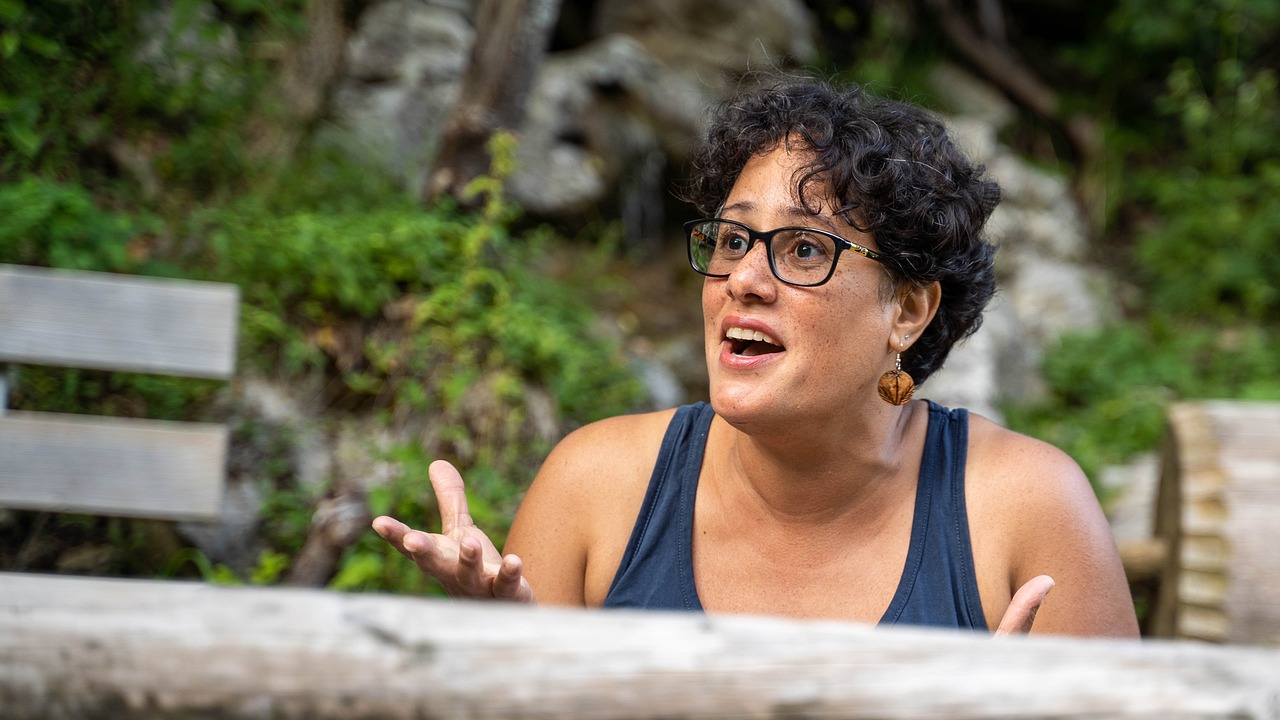
Customizing Feedback for Individual Needs
In today's fast-paced work environment, the one-size-fits-all approach to performance feedback just doesn't cut it anymore. Employees are unique individuals with different strengths, weaknesses, and learning styles. This is where customized feedback comes into play, revolutionizing the way organizations support their teams. By leveraging the power of AI, companies can tailor feedback mechanisms to meet the specific needs of each employee, enhancing engagement and productivity.
Imagine a scenario where a manager provides feedback based on generic performance metrics. While the intention is good, it often leads to confusion and frustration. Instead, AI-driven systems analyze individual performance data and generate insights that are not only relevant but also actionable. For instance, if an employee excels in creative problem-solving but struggles with time management, the feedback can focus on enhancing their organizational skills while recognizing their creativity. This targeted approach fosters a sense of personal investment in the employee's development.
Furthermore, AI can help identify patterns in feedback reception. Some employees may thrive on direct criticism, while others may prefer a more nurturing approach. By analyzing responses to previous feedback sessions, AI can suggest the most effective communication style for each individual. This not only improves the quality of feedback but also strengthens the manager-employee relationship, creating a more positive work environment.
To illustrate the impact of customized feedback, consider the following table that outlines how AI can adapt feedback based on different employee profiles:
Employee Profile | Preferred Feedback Style | Focus Areas |
---|---|---|
Creative Thinker | Encouraging and supportive | Innovation and brainstorming techniques |
Analytical Performer | Direct and data-driven | Efficiency and process optimization |
Team Player | Collaborative and constructive | Team dynamics and communication skills |
This table highlights how feedback can be customized to resonate with different employee types. By doing so, organizations can not only enhance individual performance but also cultivate a culture of continuous improvement. The ultimate goal is to create a feedback loop that empowers employees to take charge of their development, leading to greater job satisfaction and retention rates.
In conclusion, customizing feedback for individual needs through AI not only aligns with modern workplace expectations but also drives performance to new heights. By understanding and addressing the unique challenges and strengths of each employee, organizations can unlock the full potential of their workforce, paving the way for a more productive and engaged team.
- What is customized feedback? Customized feedback refers to personalized performance evaluations tailored to the unique needs and preferences of individual employees.
- How does AI help in providing customized feedback? AI analyzes performance data and employee responses to generate insights that cater to individual strengths and weaknesses, allowing for more relevant feedback.
- Why is customized feedback important? It enhances employee engagement, improves performance, and fosters a positive work environment by addressing each employee's specific learning style and needs.
- Can all organizations implement AI-driven feedback systems? While many organizations can benefit from AI, the implementation may vary based on resources, technology, and existing performance management systems.

Integrating AI with Existing Performance Metrics
Integrating artificial intelligence with existing performance metrics is not just a trend; it’s a game changer for organizations looking to enhance their operational efficiency. Imagine trying to fit a square peg into a round hole. That’s how it feels when companies attempt to implement AI without considering their current metrics. The key is to create a harmonious relationship between traditional performance indicators and advanced AI tools.
First, organizations must conduct a thorough assessment of their existing metrics. This involves identifying which metrics are effective and relevant, and which ones may need to be updated or discarded. For instance, if a company has been using sales figures as a primary metric, they should consider integrating data-driven insights from AI that can predict future sales trends based on historical data. This blend of traditional and modern methods can provide a more holistic view of performance.
Once the assessment is complete, the next step is to select the right AI tools. There are numerous AI solutions available, each with its own strengths. For example, some tools excel in data visualization, while others are better suited for predictive analytics. It’s crucial to choose tools that complement the existing metrics. By doing so, organizations can leverage AI to enhance their current frameworks rather than replace them entirely.
Moreover, training staff is an essential element in this integration process. Employees need to understand how to utilize AI tools effectively in conjunction with existing metrics. This can be achieved through workshops and training sessions that focus on the benefits of AI and how it can streamline their daily tasks. When employees are well-versed in both traditional metrics and AI capabilities, they can make more informed decisions, leading to improved performance across the board.
Another important aspect is ensuring that there is a feedback loop in place. This means continuously monitoring the effectiveness of the integrated metrics. If certain AI tools are not providing the expected insights, organizations should be agile enough to make necessary adjustments. This iterative process not only helps in refining the metrics but also fosters a culture of innovation within the organization.
In summary, integrating AI with existing performance metrics requires a strategic approach that includes assessment, tool selection, employee training, and establishing feedback mechanisms. By taking these steps, organizations can create a robust performance measurement system that not only enhances productivity but also drives growth and innovation.
- What are the benefits of integrating AI with existing performance metrics?
Integrating AI can enhance data accuracy, provide predictive insights, and improve decision-making processes, leading to better overall performance. - How can organizations choose the right AI tools?
Organizations should assess their specific needs, evaluate the capabilities of various AI tools, and consider how these tools can complement their existing metrics. - Is employee training necessary for AI integration?
Yes, training is crucial to ensure that employees can effectively use AI tools alongside traditional metrics to maximize their benefits. - What should organizations do if AI tools are not delivering expected results?
Organizations should establish a feedback loop to monitor performance and be ready to adjust their strategies and tools as necessary.
Frequently Asked Questions
- How does AI improve data collection for performance metrics?
AI enhances data collection by automating the process, allowing organizations to gather vast amounts of information quickly and accurately. With tools like natural language processing and machine learning, AI can filter out irrelevant data, ensuring that only the most pertinent information is collected. This efficiency leads to better decision-making and more reliable performance metrics.
- What role do machine learning algorithms play in analyzing performance data?
Machine learning algorithms are pivotal in analyzing performance data as they can identify patterns and trends that traditional analytical methods might miss. By processing historical data, these algorithms can provide insights into employee performance, operational efficiency, and even customer behavior, helping organizations make informed strategic decisions.
- Can predictive analytics really forecast future performance?
Absolutely! Predictive analytics uses historical data to build models that forecast future performance. By analyzing trends and behaviors, organizations can anticipate outcomes and make proactive decisions. This capability is especially valuable in strategic planning, enabling businesses to allocate resources effectively and enhance overall performance.
- What are the benefits of real-time data processing?
Real-time data processing allows organizations to react swiftly to changes in performance metrics. With immediate access to data, teams can make quick adjustments, identify issues before they escalate, and capitalize on opportunities as they arise. This agility can significantly improve operational efficiency and responsiveness.
- What challenges might organizations face when implementing predictive analytics?
While predictive analytics offers many advantages, organizations may encounter challenges such as data quality issues, lack of skilled personnel, and resistance to change. To overcome these obstacles, companies should invest in training, ensure data integrity, and foster a culture that embraces data-driven decision-making.
- How does AI help in tracking employee performance?
AI enhances employee performance tracking by providing personalized insights tailored to individual and team needs. With AI-driven tools, managers can assess performance more accurately, identify areas for improvement, and implement targeted development plans that resonate with employees, fostering a more engaged workforce.
- What is the significance of AI-driven performance feedback systems?
AI-driven performance feedback systems offer continuous evaluations, enabling organizations to create a culture of growth and development. By providing real-time feedback, these systems help employees understand their strengths and areas for improvement, ultimately leading to enhanced performance and job satisfaction.
- How can feedback be customized using AI?
AI customizes feedback by analyzing individual performance data and tailoring insights to meet each employee's unique needs. This personalized approach ensures that feedback is relevant and actionable, making it more effective in driving performance improvements and employee engagement.
- What steps should organizations take to integrate AI with existing performance metrics?
To integrate AI with current performance metrics, organizations should start by assessing their existing data infrastructure and identifying areas where AI can add value. Next, they should invest in training for staff and ensure that AI tools are compatible with existing systems. Finally, a phased approach to implementation can help smooth the transition and maximize the benefits of AI integration.