How AI Innovations are Shaping Data Analysis
In today's fast-paced digital world, the sheer volume of data generated is nothing short of astounding. With billions of bytes created every second, making sense of this data can feel like trying to find a needle in a haystack. Enter artificial intelligence (AI), a game-changer that is not just simplifying data analysis but revolutionizing it. AI innovations are reshaping how businesses approach data, enabling them to extract meaningful insights and make informed decisions faster than ever before. Imagine having a super-intelligent assistant that can sift through mountains of data, highlight trends, and even predict future outcomes—this is the power of AI!
AI technologies, particularly machine learning and natural language processing, are at the forefront of this transformation. They allow organizations to analyze data with unprecedented speed and accuracy. For instance, machine learning algorithms can identify patterns in data that would take humans much longer to uncover. This capability is particularly valuable in industries like finance, healthcare, and marketing, where timely and accurate insights can lead to significant competitive advantages.
Moreover, the integration of AI in data analysis is not just about speed; it's also about enhancing the quality of insights. Traditional data analysis methods often rely on structured data, which can limit the scope of analysis. However, AI can work with both structured and unstructured data, such as social media posts, customer reviews, and sensor data, providing a more comprehensive view of the situation at hand. This means businesses can tap into a wealth of information that was previously overlooked, leading to more robust decision-making.
As we delve deeper into this topic, we will explore the various facets of AI innovations in data analysis, from machine learning and natural language processing to predictive analytics and data visualization. Each of these elements plays a crucial role in shaping the future of data analysis, making it more efficient, insightful, and actionable.
Machine learning algorithms enhance data analysis by identifying patterns and making predictions, significantly improving decision-making processes across diverse sectors.
Natural language processing (NLP) enables machines to understand human language, facilitating the extraction of valuable insights from unstructured data sources like social media and customer feedback.
Sentiment analysis utilizes NLP to gauge public opinion, helping businesses tailor their strategies based on customer sentiment derived from large volumes of text data.
By analyzing customer feedback, companies can refine their marketing strategies, ensuring they resonate better with target audiences and enhance overall engagement.
NLP tools allow for real-time monitoring of brand sentiment, enabling businesses to respond swiftly to public perception and emerging trends.
Text mining involves extracting useful information from textual data, providing organizations with deeper insights into customer preferences and behavior.
Predictive analytics leverages historical data to forecast future trends, empowering organizations to make proactive decisions and optimize their operations.
By predicting potential outcomes, businesses can streamline their processes and allocate resources more effectively, leading to increased operational efficiency.
Predictive models assist organizations in identifying potential risks, allowing them to implement strategies that mitigate adverse impacts on their operations.
Data visualization techniques enhance the interpretability of complex data, making it easier for stakeholders to grasp insights and make informed decisions based on AI analysis.
Interactive dashboards provide real-time data visualizations, enabling users to explore data dynamically and uncover insights that drive strategic initiatives.
Effective data storytelling combines visual elements with narrative techniques, helping convey complex data insights in a compelling and understandable manner.
As AI technology continues to evolve, its role in data analysis will expand, leading to more sophisticated tools and methodologies that revolutionize how organizations interpret and utilize data.
- What is AI? AI, or artificial intelligence, refers to the simulation of human intelligence in machines, enabling them to perform tasks that typically require human intelligence.
- How does AI improve data analysis? AI enhances data analysis by automating processes, identifying patterns, and providing predictive insights that can lead to better decision-making.
- What industries benefit from AI in data analysis? Industries such as finance, healthcare, marketing, and e-commerce are significantly benefiting from AI innovations in data analysis.
- What is predictive analytics? Predictive analytics uses historical data and algorithms to forecast future outcomes, helping organizations make proactive decisions.
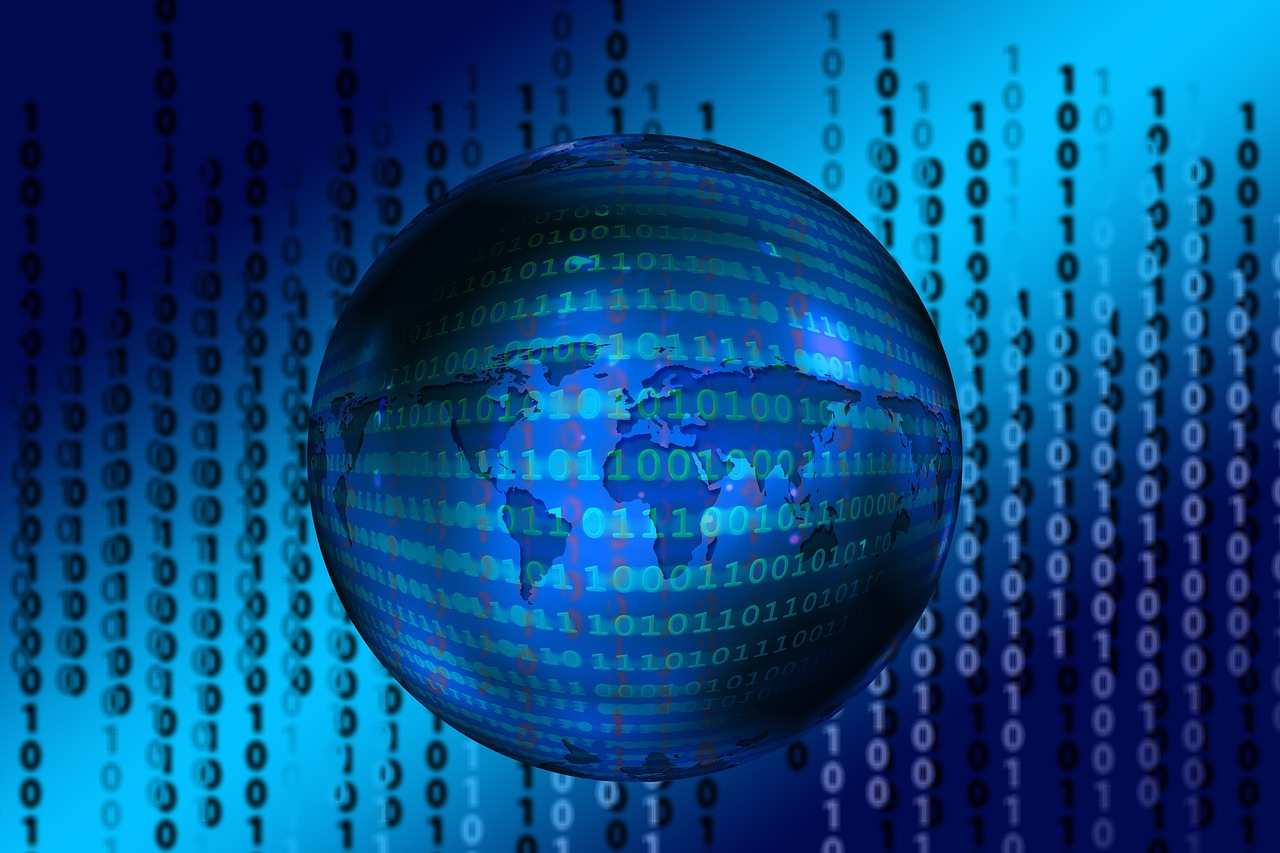
The Role of Machine Learning in Data Analysis
In today's fast-paced world, the **role of machine learning** in data analysis is nothing short of revolutionary. Imagine having a tool that can sift through mountains of data, identify patterns, and provide insights faster than a human ever could. That's precisely what machine learning does! By employing sophisticated algorithms, it enhances data analysis, making it a game-changer across various sectors, from healthcare to finance and beyond.
So, how does machine learning work its magic? At its core, machine learning algorithms learn from data. They analyze past data sets to find correlations and trends, enabling them to make predictions about future outcomes. This capability significantly improves decision-making processes. For example, in the retail sector, machine learning can predict which products are likely to be in demand based on historical sales data, seasonal trends, and even social media activity. With such insights, businesses can optimize their inventory and marketing strategies effectively.
Furthermore, machine learning isn't just about making predictions; it also enhances the **accuracy** of those predictions. Traditional data analysis methods often rely on static models, which can become outdated quickly. In contrast, machine learning algorithms continuously learn and adapt, refining their predictions as new data becomes available. This adaptability is crucial in industries like finance, where market conditions can change rapidly.
To illustrate the impact of machine learning on data analysis, consider the following table that highlights its key benefits across different industries:
Industry | Benefits of Machine Learning |
---|---|
Healthcare | Improved patient diagnosis and personalized treatment plans |
Finance | Fraud detection and risk assessment |
Retail | Enhanced customer experience through personalized recommendations |
Manufacturing | Predictive maintenance and supply chain optimization |
As we delve deeper into the world of machine learning, it's essential to note that it also empowers businesses to **uncover hidden insights** within their data. By utilizing techniques such as clustering and classification, organizations can segment their customer base more effectively, leading to targeted marketing efforts that resonate with specific demographics. This tailored approach not only enhances customer satisfaction but also drives sales and loyalty.
In conclusion, the role of machine learning in data analysis is pivotal. It transforms raw data into actionable insights, allowing organizations to make informed decisions swiftly. As technology continues to advance, we can expect machine learning to play an even more significant role in shaping the future of data analysis, making it an essential component for businesses aiming to stay ahead of the curve.
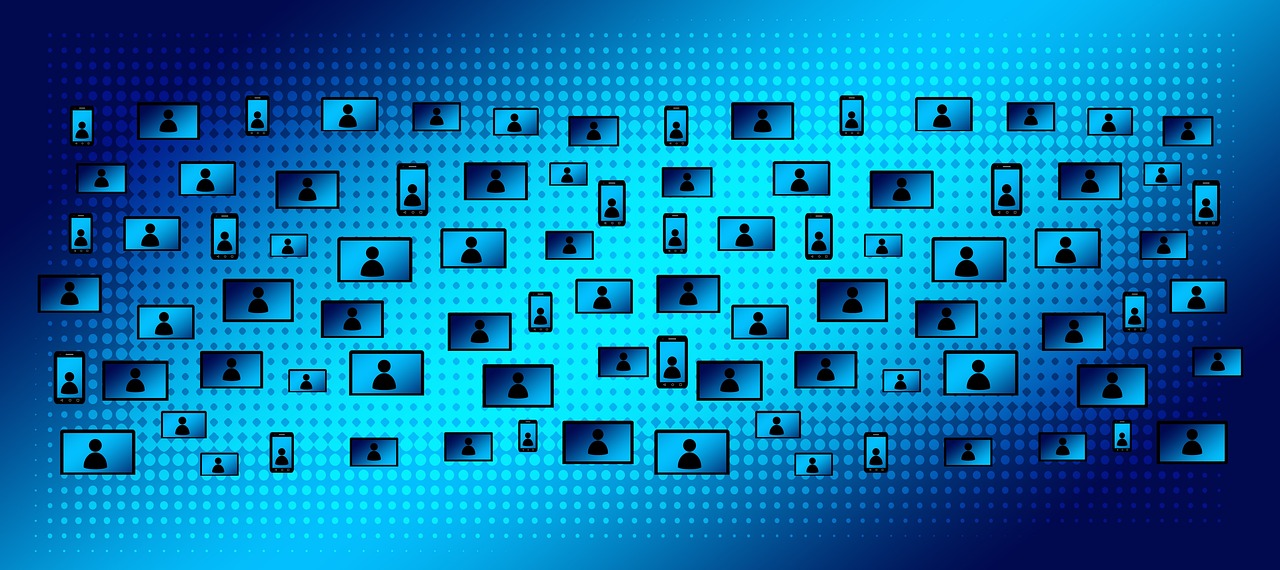
Natural Language Processing and Data Insights
Natural Language Processing, or NLP, is a fascinating branch of artificial intelligence that focuses on the interaction between computers and humans through natural language. Imagine trying to teach a machine to understand not just words, but the emotions and intentions behind those words. This is where NLP shines, as it enables machines to decipher human language and extract valuable insights from vast amounts of unstructured data. With the explosion of data generated from sources like social media, customer reviews, and online forums, the ability to analyze this information effectively is more crucial than ever.
One of the most significant advantages of NLP is its capability to transform unstructured data into structured insights. Businesses today are inundated with feedback from customers, and sifting through this data manually can be overwhelming. However, NLP algorithms can analyze text data at lightning speed, identifying trends, sentiments, and key themes that might otherwise go unnoticed. This not only saves time but also provides organizations with a deeper understanding of their customers' needs and preferences.
For instance, consider the way companies utilize sentiment analysis, a key application of NLP. By evaluating the tone and sentiment of customer feedback, businesses can gauge public opinion about their products or services. This process involves categorizing textual data into positive, negative, or neutral sentiments, allowing organizations to tailor their strategies accordingly. Here’s a quick overview of how sentiment analysis works:
Sentiment Type | Description |
---|---|
Positive | Indicates satisfaction or approval from customers. |
Negative | Reflects dissatisfaction or criticism. |
Neutral | Represents a balanced view without strong emotions. |
By implementing sentiment analysis, companies can refine their marketing strategies, ensuring they resonate better with target audiences. For example, if a business discovers a surge in negative sentiment regarding a specific product, it can take proactive measures to address the issue—whether that means improving the product, enhancing customer service, or launching a targeted marketing campaign to improve public perception. This agility in responding to customer sentiment can significantly enhance overall engagement and loyalty.
Moreover, NLP tools enable real-time monitoring of brand sentiment. Imagine being able to track how customers feel about your brand at any given moment. This capability allows businesses to respond swiftly to shifts in public perception and emerging trends. For instance, if a viral social media post negatively impacts a brand's reputation, organizations equipped with NLP can quickly identify the issue and strategize an appropriate response.
Additionally, text mining techniques, which fall under the umbrella of NLP, allow organizations to extract useful information from textual data. This process provides deeper insights into customer preferences and behaviors, enabling companies to tailor their offerings more effectively. By analyzing customer reviews, social media interactions, and other forms of textual feedback, businesses can uncover hidden patterns and trends that inform product development and marketing strategies.
In conclusion, the integration of Natural Language Processing into data analysis represents a significant leap forward in how organizations interpret and act on customer feedback. By harnessing the power of NLP, businesses can not only enhance their understanding of customer sentiment but also drive strategic initiatives that align closely with their audience’s needs. As we continue to explore the capabilities of AI, the potential for NLP to revolutionize data insights is truly exciting.
- What is Natural Language Processing?
NLP is a field of artificial intelligence that focuses on the interaction between computers and humans through natural language.
- How does sentiment analysis work?
Sentiment analysis categorizes text data into positive, negative, or neutral sentiments to gauge public opinion.
- What are the benefits of using NLP in business?
NLP helps businesses extract valuable insights from unstructured data, understand customer sentiment, and enhance their marketing strategies.
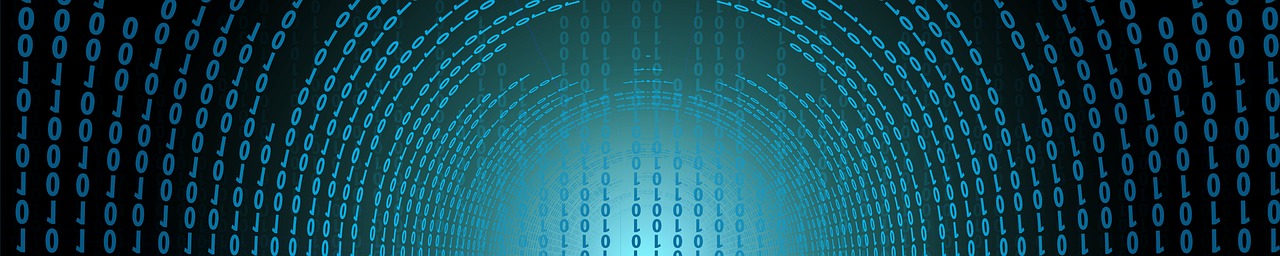
Sentiment Analysis Applications
Sentiment analysis, a fascinating application of natural language processing (NLP), is revolutionizing how businesses understand and interact with their customers. Imagine being able to tap into the collective feelings and opinions of your audience, all derived from the vast ocean of text data available online. This capability is not just a luxury; it’s becoming a necessity in today’s fast-paced market environment.
At its core, sentiment analysis involves evaluating text—be it from social media posts, customer reviews, or survey responses—to determine the emotional tone behind it. Businesses can categorize sentiments as positive, negative, or neutral, allowing them to gauge public opinion on various topics. The insights gained can be incredibly valuable, influencing everything from product development to marketing strategies.
Here are some key applications of sentiment analysis:
- Brand Monitoring: Companies can track how their brand is perceived in real-time. This allows them to address negative feedback promptly and leverage positive sentiments to enhance their reputation.
- Product Feedback: By analyzing customer reviews, businesses can identify strengths and weaknesses in their products, facilitating improvements and innovation.
- Market Research: Understanding customer sentiment helps organizations identify trends and shifts in consumer preferences, enabling them to stay ahead of the competition.
One of the most compelling aspects of sentiment analysis is its ability to provide actionable insights. For instance, if a company notices a surge in negative sentiment regarding a specific product, they can quickly investigate and address the underlying issues. This proactive approach not only helps mitigate potential damage but also fosters customer loyalty by showing that the brand values feedback.
Moreover, sentiment analysis tools can process vast amounts of data at lightning speed, something that would be impossible for a human team to accomplish. This efficiency means that organizations can make informed decisions based on real-time data rather than relying on outdated reports. As a result, businesses can pivot their strategies to align with current consumer sentiments, ensuring they remain relevant and competitive.
In summary, the applications of sentiment analysis are vast and varied, offering businesses a powerful means to connect with their customers on a deeper level. By harnessing the power of NLP, companies can not only react to customer feedback but also anticipate their needs, ultimately driving growth and enhancing customer satisfaction.
- What is sentiment analysis? Sentiment analysis is a technique used to determine the emotional tone behind a body of text, categorizing it as positive, negative, or neutral.
- How can businesses use sentiment analysis? Businesses can utilize sentiment analysis to monitor brand perception, gather product feedback, and conduct market research.
- What are some tools for sentiment analysis? There are numerous tools available, including Natural Language Toolkit (NLTK), TextBlob, and commercial platforms like Brandwatch and Hootsuite.
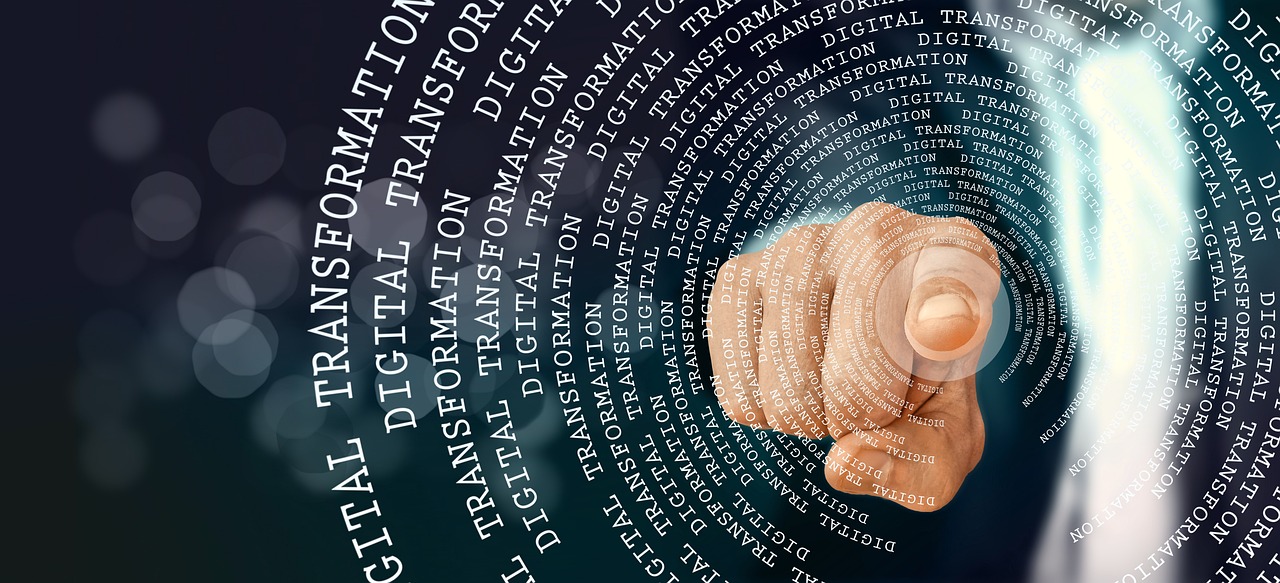
Impact on Marketing Strategies
The impact of artificial intelligence on marketing strategies is nothing short of revolutionary. Imagine being able to understand your customers on a level so profound that you can anticipate their needs before they even express them. With AI-driven tools, businesses can analyze vast amounts of data from various sources, including social media, surveys, and customer interactions, to gain valuable insights into consumer behavior.
By leveraging these insights, companies can refine their marketing strategies to ensure they resonate more effectively with their target audiences. For instance, AI can identify trending topics or sentiments among consumers, allowing businesses to tailor their messaging and campaigns accordingly. This not only enhances engagement but also builds stronger relationships with customers as they feel understood and valued.
Moreover, AI technologies enable businesses to segment their audiences more accurately. Instead of a one-size-fits-all approach, marketers can create personalized campaigns that cater to specific groups. This might include:
- Dynamic Content: Adjusting email campaigns based on user behavior.
- Targeted Advertising: Serving ads that reflect the interests and past purchases of potential customers.
- Customized Recommendations: Suggesting products or services based on previous interactions.
In addition to personalization, AI tools facilitate the automation of marketing processes. This means that routine tasks like email marketing, social media posting, and even customer service inquiries can be handled more efficiently, freeing up valuable time for marketing teams to focus on strategy and creativity.
Furthermore, AI-driven analytics provide marketers with real-time data, allowing them to adjust their strategies on the fly. For instance, if a particular campaign is underperforming, marketers can quickly identify the issue and pivot their approach, whether that means changing the messaging, targeting a different audience, or reallocating budget resources.
Ultimately, the integration of AI into marketing strategies not only enhances operational efficiency but also empowers businesses to create more meaningful connections with their customers. In a world where consumer preferences can change in the blink of an eye, having the ability to adapt and respond swiftly is invaluable. As we move forward, the role of AI in shaping marketing strategies will only become more pronounced, paving the way for innovative approaches that can redefine customer engagement.
- What is the role of AI in marketing? AI helps businesses analyze data, understand customer behavior, and automate marketing tasks, leading to more effective strategies.
- How does AI improve customer engagement? By providing personalized experiences and real-time insights, AI allows businesses to connect with customers on a deeper level.
- Can AI help with content creation? Yes, AI tools can assist in generating content ideas, optimizing SEO, and even drafting initial versions of marketing materials.
- What are some challenges of implementing AI in marketing? Challenges include data privacy concerns, the need for skilled personnel, and the potential for over-reliance on technology.
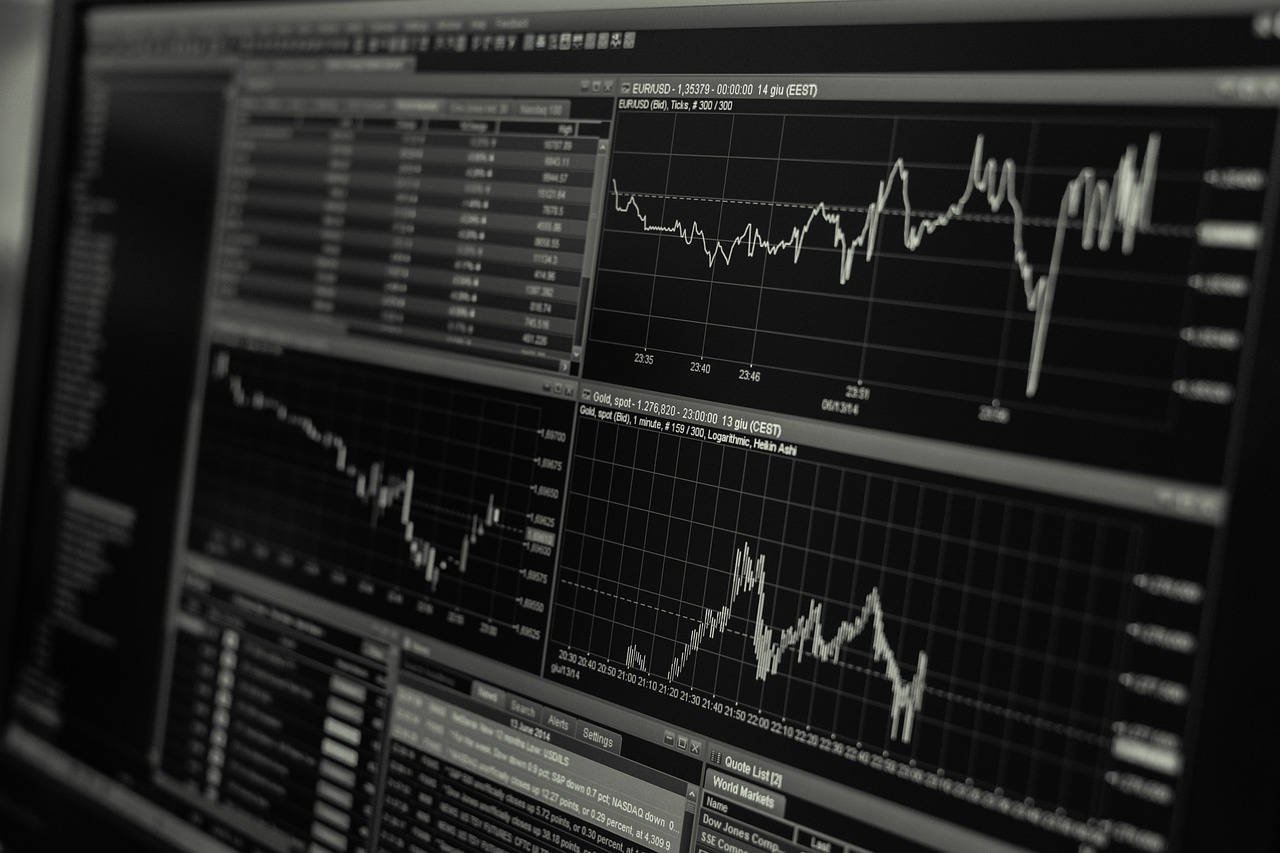
Real-time Monitoring Capabilities
In today's fast-paced digital landscape, the ability to monitor brand sentiment in real-time has become a game changer for businesses. Imagine being able to tap into the pulse of your audience as it beats—this is precisely what real-time monitoring capabilities offer. By leveraging advanced Natural Language Processing (NLP) tools, companies can instantly analyze social media chatter, customer reviews, and other unstructured data sources to gauge public sentiment. This immediate insight allows businesses to pivot their strategies quickly, ensuring they remain relevant and responsive to customer needs.
But how does this work in practice? Picture a scenario where a company launches a new product. Instead of waiting weeks for traditional market research to reveal customer reactions, they can utilize real-time sentiment analysis to see how the product is being received almost instantaneously. This capability not only enhances decision-making but also empowers brands to engage with their audience proactively. For example, if a surge in negative feedback is detected, companies can address issues head-on, demonstrating to customers that their opinions matter.
Furthermore, the integration of real-time monitoring tools can be illustrated in the following ways:
- Immediate Response: Companies can react to customer feedback as it happens, whether it’s addressing complaints or amplifying positive reviews.
- Trend Identification: By observing shifts in sentiment, businesses can identify emerging trends and adjust their marketing strategies accordingly.
- Competitive Advantage: Staying ahead of competitors by quickly adapting to consumer sentiment can lead to a stronger market position.
The beauty of real-time monitoring lies in its ability to provide actionable insights that can transform a company’s operational strategies. For instance, if a particular campaign is generating buzz, businesses can double down on those efforts, channeling resources where they are most effective. Conversely, if there’s a negative reaction, they can quickly investigate and rectify the situation, potentially mitigating damage to their brand reputation.
In summary, real-time monitoring capabilities powered by NLP not only enhance a company's understanding of its audience but also foster a culture of responsiveness and adaptability. In an era where consumer expectations are higher than ever, the ability to listen and respond in real-time can set a brand apart from its competitors, making it an essential tool for any forward-thinking organization.
- What is real-time monitoring in data analysis? Real-time monitoring refers to the ability to analyze data as it is generated, allowing businesses to gain immediate insights into customer sentiment and market trends.
- How does NLP contribute to real-time monitoring? Natural Language Processing (NLP) enables machines to understand and interpret human language, facilitating the extraction of insights from large volumes of unstructured data in real-time.
- Why is real-time monitoring important for businesses? It allows businesses to respond quickly to customer feedback, identify trends, and make informed decisions that can enhance their market position.
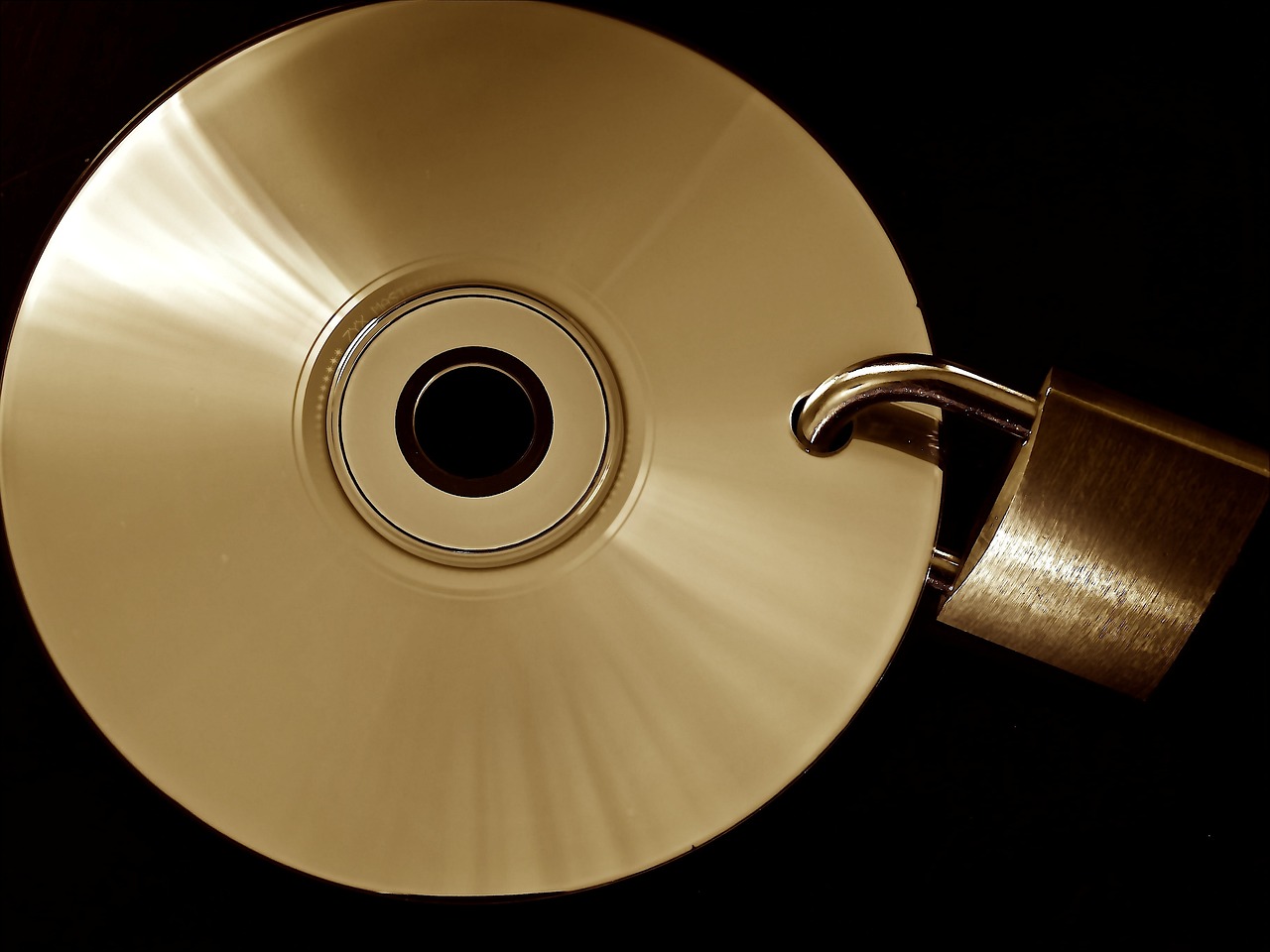
Text Mining Techniques
Text mining, often referred to as text data mining, is a powerful tool that allows organizations to extract meaningful information from unstructured text. Imagine sifting through a mountain of documents, emails, and social media posts, searching for gems of insight hidden within the chaos. That's precisely what text mining does—it transforms raw text into structured data, making it easier to analyze and understand. This process involves several key techniques that can significantly enhance decision-making and strategy formulation.
One of the primary techniques in text mining is tokenization, which breaks down text into smaller pieces, or tokens. These tokens can be words, phrases, or even sentences, and they serve as the building blocks for further analysis. By segmenting text into manageable parts, organizations can better identify patterns and trends. For example, in customer service, tokenization can help companies analyze feedback to pinpoint common issues or areas for improvement.
Another crucial technique is stemming and lemmatization. These processes reduce words to their base or root form, which helps in normalizing the data. For instance, the words "running," "ran," and "runner" can all be reduced to "run." This reduction is vital because it allows for a more accurate analysis of word frequency and sentiment. When companies understand the core sentiments expressed in customer feedback, they can tailor their responses and strategies accordingly.
Moreover, sentiment analysis plays a pivotal role in text mining. By analyzing the emotional tone behind words, businesses can gauge public opinion and customer satisfaction. This technique can be particularly beneficial for marketing teams looking to refine their campaigns based on real-time feedback. For instance, if a new product launch receives overwhelmingly negative sentiment on social media, companies can pivot their marketing strategies to address consumer concerns more effectively.
Text Mining Techniques | Description |
---|---|
Tokenization | Breaking down text into smaller, manageable pieces for easier analysis. |
Stemming | Reducing words to their root form to normalize data. |
Lemmatization | Similar to stemming, but considers the context of the word for more accurate results. |
Sentiment Analysis | Assessing the emotional tone behind a series of words to understand public opinion. |
Finally, topic modeling is another innovative text mining technique that helps organizations uncover hidden themes within large volumes of text. By clustering similar documents, businesses can identify emerging trends and topics that are relevant to their audience. This technique is particularly useful in content marketing, where understanding the interests and preferences of target demographics can lead to more effective content strategies.
In conclusion, text mining techniques are essential for organizations looking to harness the power of unstructured data. By employing these methodologies, businesses can gain deeper insights into customer behavior, enhance their marketing strategies, and ultimately drive better decision-making. As the digital landscape continues to evolve, the importance of text mining will only grow, making it a critical component of any data analysis strategy.
- What is text mining? Text mining is the process of extracting meaningful information from unstructured text data.
- How does sentiment analysis work? Sentiment analysis evaluates the emotional tone of text to determine public opinion or customer sentiment.
- What are the benefits of using text mining techniques? Text mining techniques help organizations identify patterns, improve customer understanding, and enhance decision-making.
- Can text mining be applied to social media data? Yes, text mining is particularly effective for analyzing social media data to gauge public sentiment and trends.
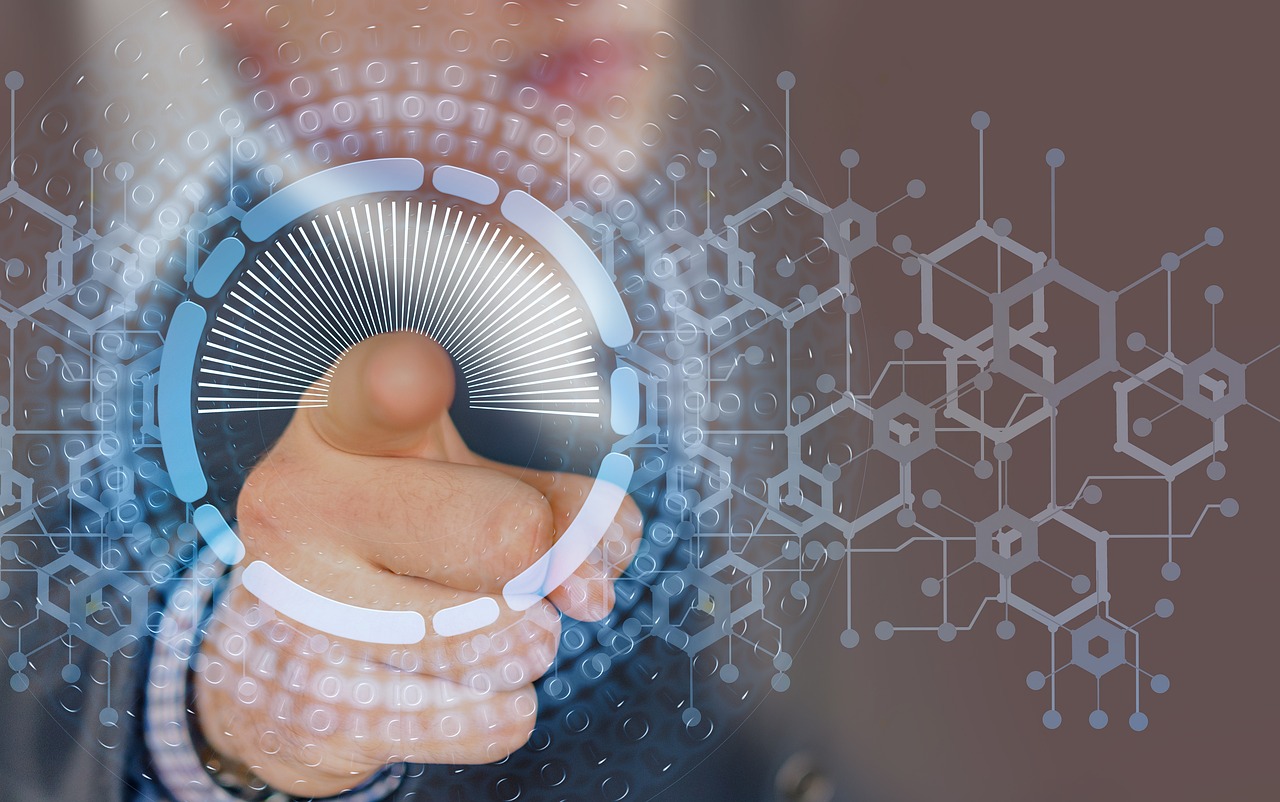
Predictive Analytics and Business Intelligence
Predictive analytics has emerged as a game-changer in the realm of business intelligence, providing organizations with the tools they need to forecast future trends and behaviors based on historical data. Imagine having a crystal ball that not only reveals what might happen next but also guides your decisions in real time. This is precisely what predictive analytics offers. By harnessing the power of advanced algorithms and machine learning techniques, businesses can analyze vast amounts of data to uncover patterns that would otherwise remain hidden.
At its core, predictive analytics involves the use of statistical techniques, machine learning, and data mining to analyze current and historical facts to make predictions about future events. This capability is crucial for organizations aiming to stay ahead of the competition. For instance, retail businesses can utilize predictive analytics to anticipate customer purchasing behavior, enabling them to optimize inventory levels and tailor marketing efforts. The result? Increased sales and improved customer satisfaction.
One of the most significant advantages of predictive analytics is its ability to enhance operational efficiency. By forecasting potential outcomes, companies can streamline their processes and allocate resources more effectively. For example, a manufacturing firm might use predictive models to determine when machinery is likely to fail, allowing them to perform maintenance proactively rather than reactively. This not only saves costs but also minimizes downtime, which can be detrimental to productivity.
Moreover, predictive analytics plays a crucial role in risk management strategies. Organizations can identify potential risks before they escalate into significant issues. By analyzing historical data, businesses can pinpoint trends that indicate possible challenges, such as market fluctuations or supply chain disruptions. This foresight enables them to implement strategies that mitigate adverse impacts on their operations, ensuring they remain resilient in the face of uncertainty.
To illustrate the impact of predictive analytics on business intelligence, consider the following table that outlines key applications and benefits:
Application | Benefit |
---|---|
Customer Segmentation | Targeted marketing campaigns leading to higher conversion rates. |
Churn Prediction | Identifying at-risk customers and implementing retention strategies. |
Sales Forecasting | Optimizing inventory and improving cash flow management. |
Fraud Detection | Minimizing financial losses through early identification of fraudulent activities. |
In summary, predictive analytics is not just a buzzword; it is a vital component of modern business intelligence that empowers organizations to make informed decisions. By leveraging historical data and advanced analytical techniques, companies can enhance their operational efficiency, manage risks more effectively, and ultimately drive growth. As we move forward, the integration of predictive analytics into business strategies will only become more pronounced, shaping the future of how organizations operate.
- What is predictive analytics?
Predictive analytics refers to the use of statistical techniques and machine learning to analyze historical data and make predictions about future events.
- How can businesses benefit from predictive analytics?
Businesses can benefit from predictive analytics by enhancing operational efficiency, improving risk management, and tailoring marketing strategies based on customer behavior.
- Is predictive analytics only for large companies?
No, predictive analytics can be beneficial for businesses of all sizes. Small and medium enterprises can also leverage these tools to gain insights and improve decision-making.
- What types of data are used in predictive analytics?
Predictive analytics can utilize a variety of data types, including transactional data, customer feedback, social media interactions, and operational metrics.
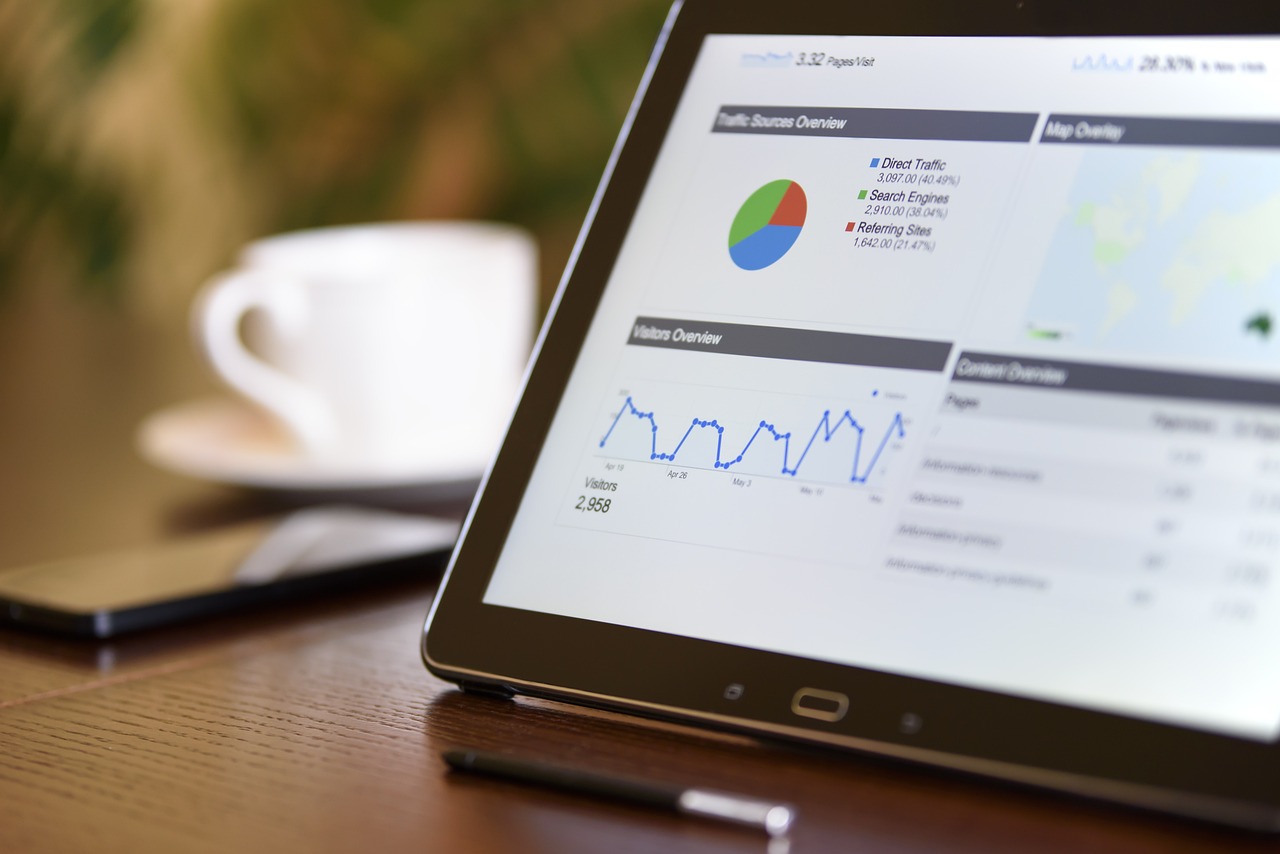
Enhancing Operational Efficiency
In today's fast-paced business environment, operational efficiency is more than just a buzzword; it's a necessity. With the advent of AI and its powerful predictive analytics capabilities, organizations are now equipped to transform their operational processes dramatically. Imagine having the ability to foresee potential challenges before they arise—this is where AI shines! By analyzing historical data, AI can identify patterns that might elude human analysts, allowing businesses to make proactive decisions rather than reactive ones.
Consider a manufacturing company that uses AI-driven predictive models. By analyzing previous production data, the system can forecast equipment failures or supply chain disruptions. This foresight enables the company to schedule maintenance before a breakdown occurs, thereby minimizing downtime and maximizing productivity. The result? A significant boost in operational efficiency, as resources are allocated more effectively and processes run smoothly.
Moreover, AI doesn't just stop at predicting outcomes; it also provides recommendations for optimization. For instance, if a retail company notices a trend in consumer purchasing behavior, AI can suggest adjustments in inventory levels or even marketing strategies to align with these insights. This level of adaptability is crucial in maintaining a competitive edge in any industry.
To illustrate the impact of AI on operational efficiency, let's take a look at a simple comparison of traditional methods versus AI-enhanced strategies:
Aspect | Traditional Methods | AI-Enhanced Strategies |
---|---|---|
Data Analysis | Manual analysis, time-consuming | Automated, real-time insights |
Decision-Making | Reactive, based on past experiences | Proactive, based on predictive models |
Resource Allocation | Static, often misaligned with needs | Dynamic, optimized for current demands |
As organizations continue to embrace these AI technologies, the potential for enhanced operational efficiency becomes even more apparent. The ability to not only forecast but also adapt to changing circumstances in real-time is invaluable. Businesses can streamline their workflows, reduce waste, and ultimately improve their bottom line.
In conclusion, the integration of AI in operational processes is not just a trend; it’s a revolution. Companies that harness these technologies will find themselves at the forefront of their industries, equipped to tackle challenges head-on and seize opportunities as they arise. The future of operational efficiency is bright, thanks to the remarkable capabilities of AI.
- What is operational efficiency? Operational efficiency refers to the ability of an organization to deliver products or services in the most cost-effective manner without compromising quality.
- How does AI improve operational efficiency? AI improves operational efficiency by providing predictive insights, automating routine tasks, and optimizing resource allocation based on data analysis.
- Can AI completely replace human decision-making? While AI can enhance decision-making, it is not a complete replacement for human judgment. The best results often come from a combination of AI insights and human expertise.
- What industries can benefit from AI-driven operational efficiency? Almost every industry, including manufacturing, retail, healthcare, and logistics, can benefit from AI-driven operational efficiency.
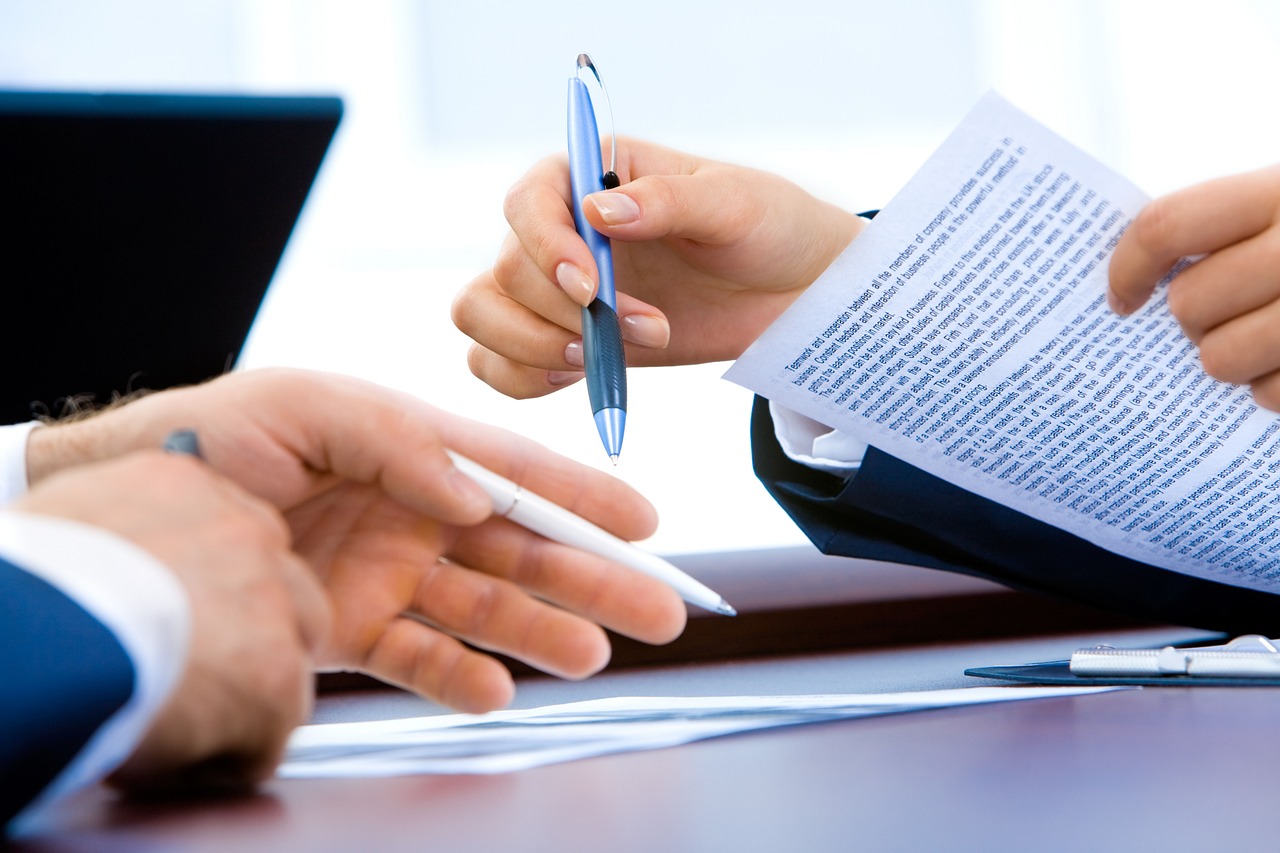
Risk Management Strategies
In today’s fast-paced business environment, risk management is more crucial than ever. Organizations face a myriad of challenges—from economic fluctuations to cybersecurity threats. Leveraging predictive analytics can significantly enhance risk management strategies, allowing businesses to anticipate potential issues before they escalate. By analyzing historical data and identifying patterns, companies can create a proactive approach to risk rather than a reactive one.
One of the primary benefits of using AI in risk management is the ability to develop predictive models. These models analyze various data points, such as market trends, consumer behavior, and operational metrics, to identify potential risks. For instance, a retail company might use predictive analytics to foresee inventory shortages during peak seasons, enabling them to adjust their supply chain strategies accordingly. This not only minimizes financial loss but also enhances customer satisfaction by ensuring product availability.
Furthermore, implementing a structured risk management framework can help organizations categorize and prioritize risks effectively. Here’s a simple breakdown of how companies can approach risk management:
- Identification: Recognizing potential risks that could impact the organization.
- Assessment: Evaluating the likelihood and potential impact of these risks.
- Mitigation: Developing strategies to minimize the impact of identified risks.
- Monitoring: Continuously tracking the risk environment and adjusting strategies as necessary.
Additionally, organizations can benefit from creating a risk management team dedicated to monitoring and reporting on risk factors. This team can utilize AI tools to conduct regular analyses, ensuring that the organization remains ahead of potential threats. By fostering a culture of risk awareness, companies can empower employees at all levels to contribute to risk management efforts.
In summary, integrating AI-driven predictive analytics into risk management strategies allows organizations to not only identify and mitigate risks effectively but also to create a resilient operational framework. As businesses continue to navigate uncertainties, adopting these strategies will be key to sustaining growth and maintaining a competitive edge.
- What is predictive analytics? Predictive analytics involves using historical data and statistical algorithms to forecast future outcomes, helping organizations make informed decisions.
- How can AI improve risk management? AI can analyze vast amounts of data quickly, identify patterns, and predict potential risks, allowing organizations to take proactive measures.
- What are some common risks businesses face? Common risks include financial instability, cybersecurity threats, supply chain disruptions, and compliance issues.
- Why is a risk management team important? A dedicated team ensures continuous monitoring and assessment of risks, fostering a culture of awareness and preparedness within the organization.
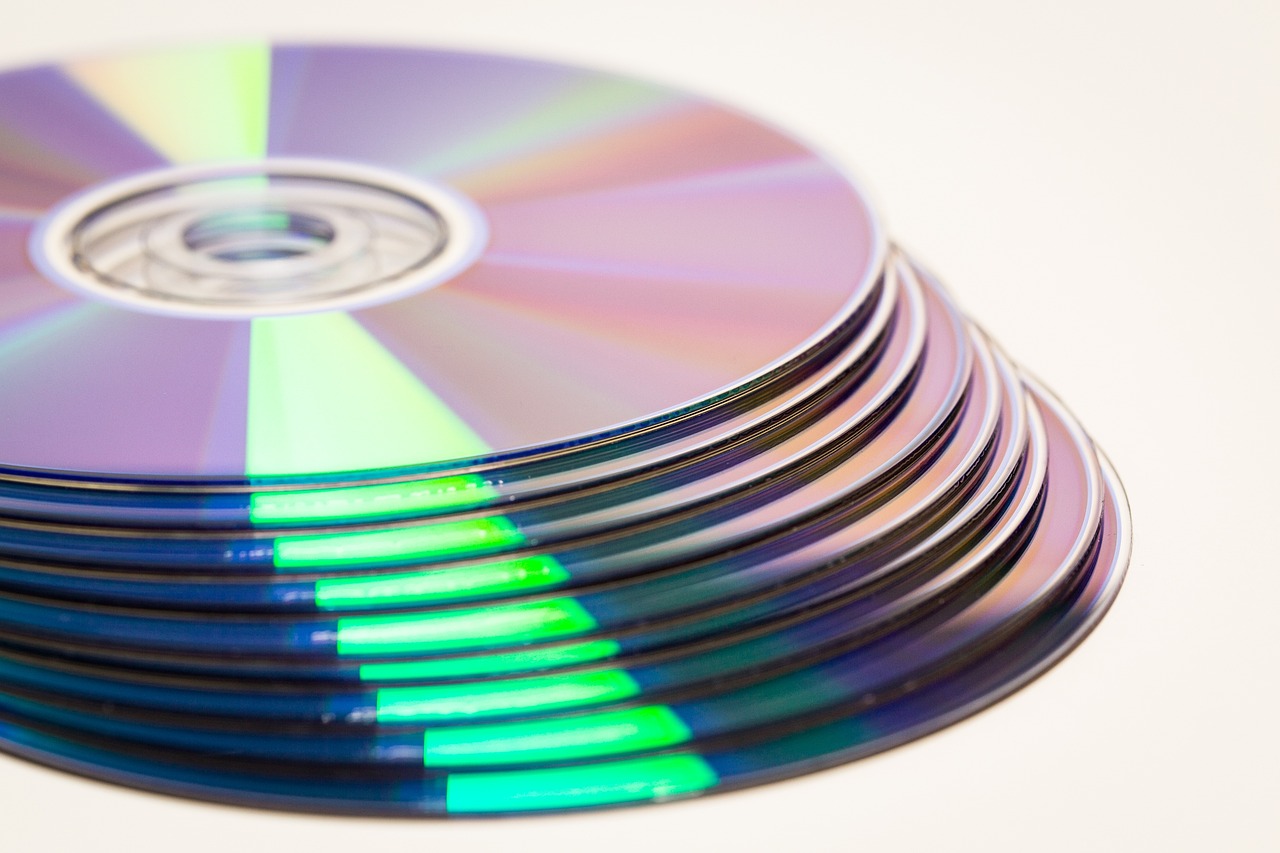
Data Visualization in AI-Driven Analysis
Data visualization is akin to giving life to numbers and statistics, transforming them into a narrative that speaks to the audience. In the realm of AI-driven analysis, it plays a pivotal role by helping stakeholders interpret complex datasets with ease. Imagine trying to decipher a dense forest of numbers without a map; that’s where visualization steps in, acting as a compass that guides users through intricate data landscapes. By leveraging various visualization techniques, organizations can uncover insights that might otherwise remain hidden in the depths of raw data.
One of the most significant advantages of data visualization in AI analysis is its ability to present vast amounts of information in a digestible format. This is especially crucial in today's fast-paced business environment, where decisions need to be made swiftly and accurately. For instance, AI algorithms can analyze customer behavior across multiple channels, but without effective visualization, the findings can be overwhelming. Here, visualization tools come into play, providing clear and concise representations of trends, patterns, and anomalies.
Interactive dashboards are a prime example of how data visualization can enhance decision-making. These dashboards allow users to engage with the data dynamically, filtering and drilling down into specifics that matter most to them. Instead of static reports, stakeholders can explore data in real-time, gaining deeper insights into performance metrics, customer engagement levels, and operational efficiencies. This interactivity not only fosters better understanding but also encourages collaborative discussions among team members, leading to more informed strategies.
Furthermore, effective data storytelling is a critical component of AI-driven analysis. It combines visual elements with narrative techniques to convey complex insights compellingly. Think of it as crafting a story where data points are characters, and their interactions reveal the plot. By using charts, graphs, and infographics, organizations can illustrate trends over time, highlight key performance indicators, and showcase the impact of strategic initiatives. This approach not only captivates the audience but also makes the information memorable.
To illustrate the impact of data visualization, consider the following table that compares traditional reporting methods with AI-driven visualization techniques:
Aspect | Traditional Reporting | AI-Driven Visualization |
---|---|---|
Data Interpretation | Static and often overwhelming | Dynamic and user-friendly |
Decision-Making Speed | Slower due to complexity | Faster with real-time insights |
Collaboration | Limited interaction | Facilitates discussions |
Insight Discovery | Requires manual exploration | Automated insights with AI |
As we look to the future, the role of data visualization in AI-driven analysis will only grow more prominent. With advancements in AI technology, visualization tools will become even more sophisticated, allowing for deeper insights and more comprehensive analyses. This evolution promises to revolutionize how organizations interpret and utilize data, turning it into a powerful asset that drives strategic decision-making.
- What is data visualization? Data visualization is the graphical representation of information and data, using visual elements like charts, graphs, and maps to make complex data more accessible and understandable.
- How does AI enhance data visualization? AI enhances data visualization by automating the analysis process, identifying patterns, and generating insights that can be visualized in real-time, making data interpretation faster and more accurate.
- Why is storytelling with data important? Storytelling with data is important because it helps convey complex insights in a relatable manner, making the information more engaging and memorable for the audience.
- What tools can I use for data visualization? There are several tools available for data visualization, including Tableau, Power BI, and Google Data Studio, each offering unique features for effective data representation.
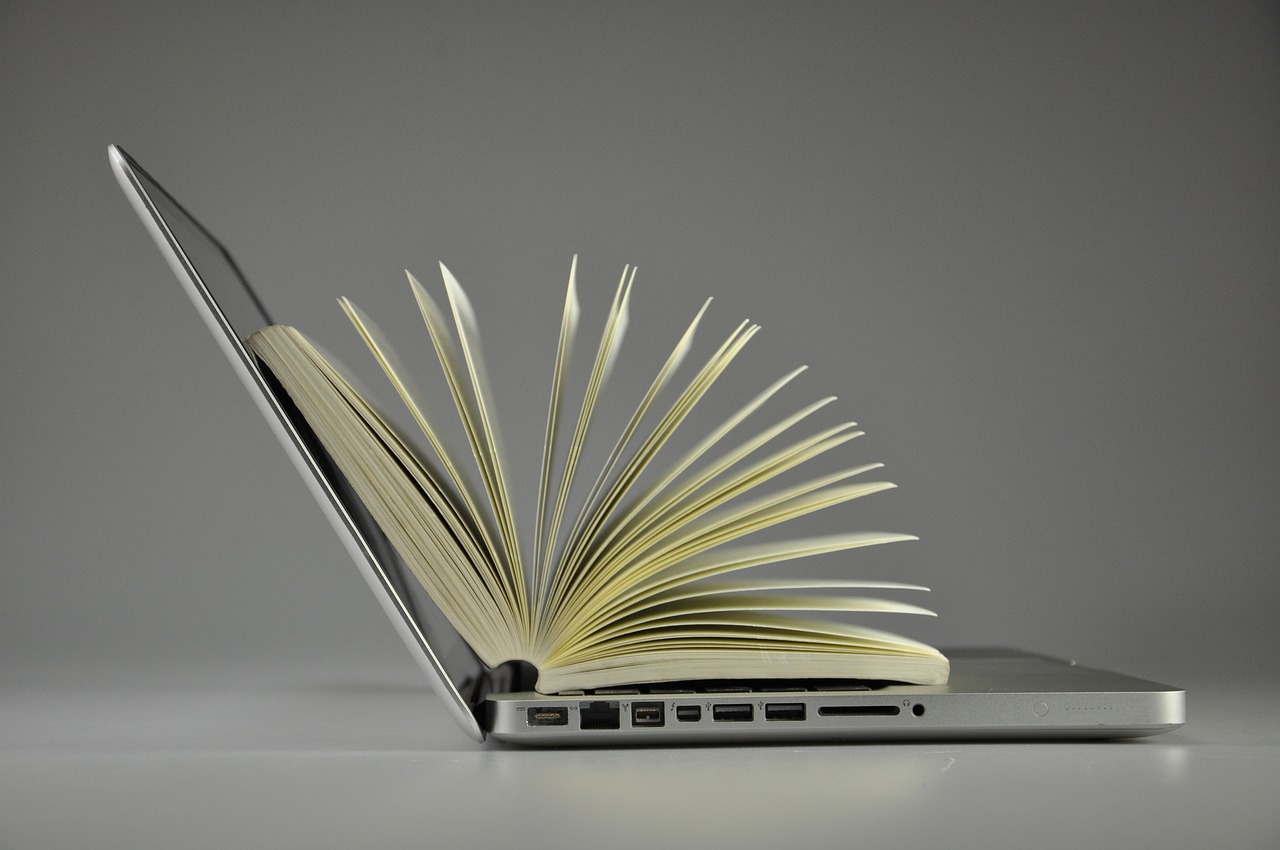
Interactive Dashboards
In the realm of data analysis, have emerged as a game-changer. Imagine having the power to visualize your data in real-time, where every click and hover reveals deeper insights. This level of interactivity transforms static data into a dynamic experience, allowing users to explore information in ways that were previously unimaginable. With the right tools, stakeholders can manipulate data on the fly, filtering through vast datasets to uncover trends and patterns that directly inform their decision-making processes.
One of the standout features of interactive dashboards is their ability to present complex data in a visually appealing manner. Instead of sifting through endless spreadsheets filled with rows and columns, users can engage with graphics that tell a story. Whether it’s through bar charts, pie charts, or heat maps, the visual representation of data makes it easier to digest and understand. This is particularly beneficial for organizations that deal with large volumes of data, as it enables teams to quickly identify key metrics and performance indicators.
Moreover, interactive dashboards foster collaboration among team members. When everyone has access to the same real-time data, it encourages discussions and collective problem-solving. For instance, a marketing team can track campaign performance live and adjust strategies on the spot. This agility not only enhances productivity but also leads to more informed decisions that can pivot based on current data trends.
To illustrate how interactive dashboards can be utilized effectively, consider the following table that outlines essential features:
Feature | Description |
---|---|
Real-Time Data Updates | Dashboards refresh automatically to display the latest data, ensuring users always have the most current information at their fingertips. |
User-Friendly Interface | Intuitive designs allow even non-technical users to navigate and manipulate data without extensive training. |
Customizable Views | Users can tailor the dashboard to display the metrics that matter most to them, enhancing personal relevance and engagement. |
Interactive Filters | Filters enable users to drill down into specific data segments, making it easy to analyze subsets of data for deeper insights. |
As we look to the future, the significance of interactive dashboards will only grow. With advancements in AI and machine learning, these tools will become even more sophisticated, incorporating predictive analytics and automated insights. Imagine a dashboard that not only shows you what has happened but also suggests what might happen next based on historical data trends. This is the direction we’re headed, and it’s an exciting prospect for businesses aiming to stay ahead of the curve.
- What is an interactive dashboard? An interactive dashboard is a data visualization tool that allows users to interact with and explore data in real time, providing insights through dynamic visual elements.
- How can I create an interactive dashboard? You can create an interactive dashboard using various data visualization tools such as Tableau, Power BI, or Google Data Studio, which offer user-friendly interfaces for building custom dashboards.
- What are the benefits of using interactive dashboards? Benefits include real-time data access, improved decision-making, enhanced collaboration among teams, and the ability to visualize complex data in an easily digestible format.
- Are interactive dashboards suitable for all businesses? Yes, interactive dashboards can be tailored to meet the needs of various industries, from marketing to finance, making them versatile tools for any organization.
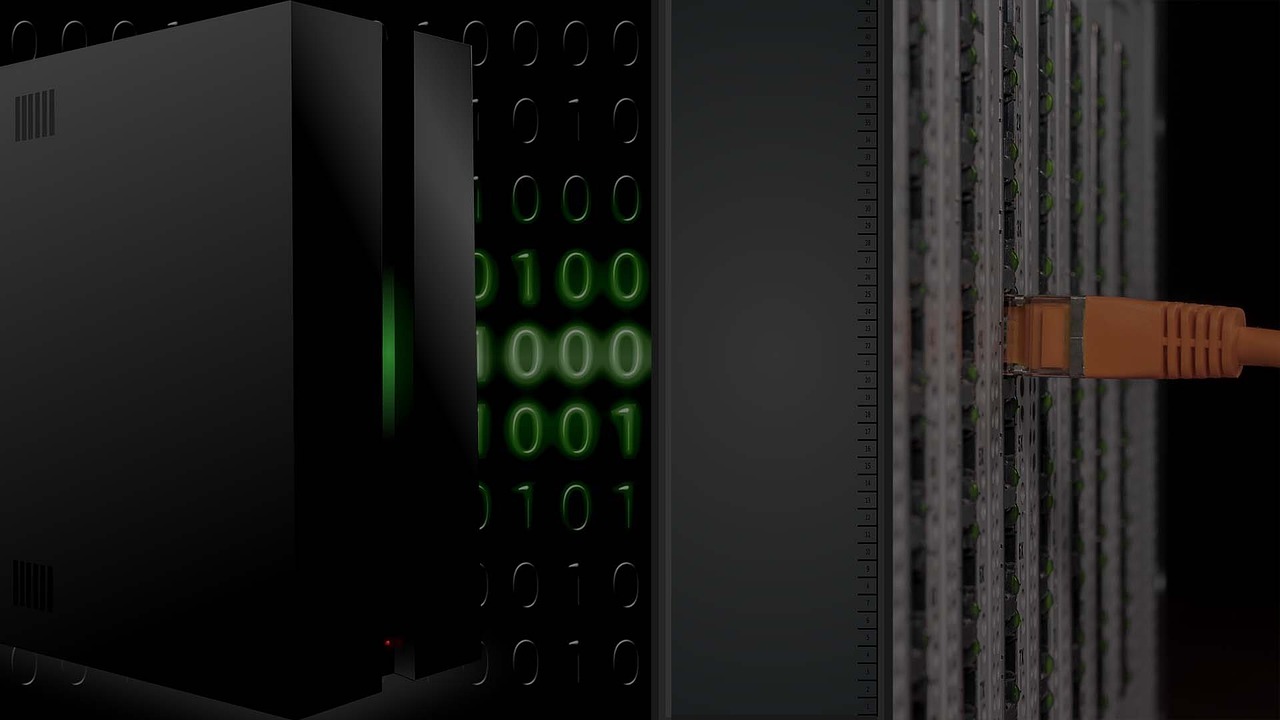
Storytelling with Data
In today's fast-paced digital world, data is everywhere, but the challenge lies in making it meaningful. This is where the art of comes into play. Imagine trying to explain a complex concept without a narrative—it's like trying to navigate a maze without a map. Data storytelling transforms raw numbers and statistics into engaging narratives that resonate with audiences, making it easier to grasp insights and take action.
Effective data storytelling combines visual elements with a narrative structure, creating a compelling experience for the audience. Think of it as a bridge that connects the data to the human experience. It involves weaving together facts, figures, and visuals to create a story that not only informs but also captivates. For instance, instead of just presenting a chart showing sales figures over the years, a data storyteller might highlight how those figures correlate with customer satisfaction and market trends, providing a richer context.
To enhance the impact of data storytelling, consider the following key elements:
- Clarity: The story should be straightforward and easy to understand. Avoid jargon and complex terms that might confuse the audience.
- Relevance: Tailor the narrative to the audience's interests and needs. What matters to them? What questions do they have?
- Visual Appeal: Use engaging visuals like graphs, charts, and infographics to complement the narrative. This not only makes the data more digestible but also keeps the audience's attention.
- Emotion: Connect with the audience on an emotional level. Use anecdotes or real-life examples that illustrate the data's significance.
By focusing on these elements, businesses can create powerful narratives that drive action. For example, a company might present its annual performance data by sharing stories of individual employees who contributed to that success. This not only highlights the data but also showcases the human side of the organization, making the information more relatable.
Moreover, storytelling with data is not just about presenting past performance; it can also be a tool for forecasting and strategic planning. By illustrating trends and patterns through a narrative lens, organizations can better anticipate future challenges and opportunities. This approach allows stakeholders to visualize potential scenarios and make informed decisions based on a comprehensive understanding of the data.
In conclusion, the power of storytelling with data lies in its ability to transform numbers into narratives that engage, inform, and inspire action. As we continue to harness the capabilities of AI and data analysis, the importance of effective storytelling will only grow, making it an essential skill for anyone looking to make an impact in their field.
- What is data storytelling? Data storytelling is the practice of using narrative techniques combined with data visualizations to communicate insights and engage an audience effectively.
- Why is storytelling important in data analysis? Storytelling helps to contextualize data, making it more relatable and easier for audiences to understand, thus driving better decision-making.
- How can I improve my data storytelling skills? Focus on clarity, relevance, visual appeal, and emotional connection while practicing presenting data in narrative formats.
- What tools can assist in data storytelling? Tools like Tableau, Power BI, and Google Data Studio provide robust features for creating visualizations that enhance data storytelling.
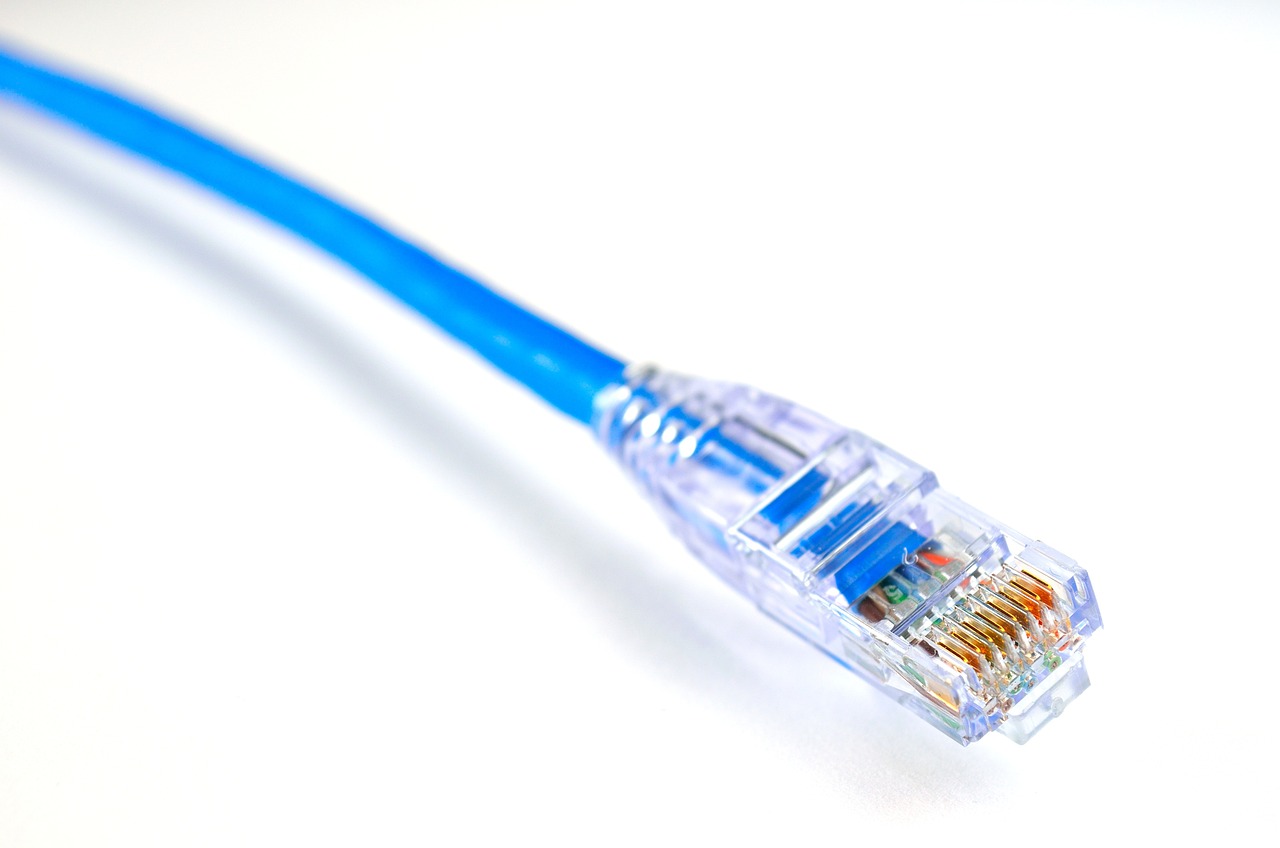
The Future of AI in Data Analysis
As we gaze into the horizon of technological advancements, it's clear that the future of artificial intelligence (AI) in data analysis is not just bright; it's dazzling! The evolution of AI is set to redefine how organizations interpret and utilize data, ushering in an era of unprecedented insights and operational efficiency. Imagine a world where data analysis is not a cumbersome task but a seamless, intuitive process that empowers businesses to make lightning-fast decisions. This is not a distant dream; it’s on the cusp of becoming reality!
One of the most exciting developments on the horizon is the integration of automated machine learning (AutoML). This technology will allow non-experts to harness the power of machine learning without needing extensive technical knowledge. Picture a marketing manager being able to input data and receive predictive insights without having to consult a data scientist. This democratization of data analysis will enable organizations of all sizes to leverage AI tools, leveling the playing field in competitive industries.
Moreover, as AI algorithms become more sophisticated, we can expect them to analyze data in real-time, providing businesses with immediate insights. This capability will be crucial in sectors like finance, healthcare, and e-commerce, where timely decision-making can significantly impact outcomes. For instance, real-time data analysis can help retailers adjust their inventory based on current shopping trends, ensuring that they meet customer demands without overstocking.
In addition to real-time capabilities, the future of AI in data analysis will also see a surge in explainable AI (XAI). As organizations rely more on AI-driven insights, the need for transparency in how decisions are made becomes paramount. XAI will allow businesses to understand the rationale behind AI predictions, fostering trust among stakeholders. This transparency will be essential, especially in industries such as finance and healthcare, where decisions can have significant ethical implications.
Furthermore, the rise of edge computing will revolutionize data analysis by processing data closer to its source, rather than relying solely on centralized cloud systems. This shift will enhance the speed and efficiency of data analysis, particularly for Internet of Things (IoT) devices that generate massive amounts of data. Imagine smart cities where traffic patterns are analyzed in real-time to optimize traffic flow—this is the kind of future we are heading towards!
As we look forward, we must also consider the implications of AI on job roles within data analysis. While some fear that AI will replace human jobs, the reality is that it will augment human capabilities. Data analysts will transition from performing repetitive tasks to focusing on strategic decision-making and creative problem-solving. In this sense, AI will act as a powerful assistant, enabling analysts to derive deeper insights from data.
In conclusion, the future of AI in data analysis is not just about technology; it's about transforming the way we think about data. With advancements in automated machine learning, real-time analysis, explainable AI, and edge computing, we are on the brink of a data revolution. Organizations that embrace these changes will not only thrive but will also lead their industries into a new era of data-driven decision-making.
- What is the role of AI in data analysis? AI enhances data analysis by automating processes, uncovering patterns, and providing predictive insights, making data interpretation faster and more accurate.
- How will automated machine learning impact businesses? AutoML will enable non-experts to leverage machine learning, allowing businesses of all sizes to gain insights without needing extensive technical expertise.
- What is explainable AI and why is it important? Explainable AI provides transparency in AI decision-making processes, helping businesses and stakeholders understand the reasoning behind predictions, thus fostering trust.
- Will AI replace jobs in data analysis? While AI will automate certain tasks, it will also augment human capabilities, allowing data analysts to focus on strategic decision-making and creative problem-solving.
Frequently Asked Questions
- What is the role of AI in data analysis?
AI plays a crucial role in data analysis by automating processes, identifying patterns, and providing insights that were previously hard to uncover. With techniques like machine learning and natural language processing, AI helps organizations make smarter decisions by analyzing vast amounts of data quickly and accurately.
- How does machine learning enhance data analysis?
Machine learning enhances data analysis by enabling algorithms to learn from data and improve over time. This capability allows businesses to identify trends and make predictions, which can significantly improve decision-making processes across various sectors, from finance to healthcare.
- What is natural language processing (NLP) and why is it important?
NLP is a branch of AI that focuses on the interaction between computers and human language. It is important because it allows machines to understand and interpret unstructured data, such as customer feedback and social media posts, turning it into actionable insights for businesses.
- Can you explain sentiment analysis?
Sentiment analysis is a technique that uses NLP to determine the emotional tone behind a series of words. This is particularly useful for businesses to gauge public opinion and tailor their strategies based on customer sentiment, ultimately leading to better engagement and satisfaction.
- How does predictive analytics impact business decisions?
Predictive analytics uses historical data to forecast future trends, helping organizations make proactive decisions. By anticipating potential outcomes, businesses can optimize their operations, manage risks effectively, and allocate resources more efficiently.
- What are the benefits of data visualization in AI?
Data visualization enhances the interpretability of complex data, making it easier for stakeholders to understand insights derived from AI analysis. It allows users to explore data dynamically through interactive dashboards and storytelling techniques, which can drive strategic initiatives.
- What does the future hold for AI in data analysis?
The future of AI in data analysis looks promising, with continuous advancements expected in tools and methodologies. As AI technology evolves, it will further revolutionize how organizations interpret and utilize data, leading to more sophisticated insights and improved decision-making processes.