The Advancements in AI-Powered Speech Recognition
In today's fast-paced world, the way we communicate is evolving at an astonishing rate. AI-powered speech recognition technology is at the forefront of this evolution, transforming how we interact with machines and each other. But what exactly does this mean for us? Imagine speaking to your device and having it understand you perfectly, regardless of your accent or the background noise. Sounds like something out of a sci-fi movie, right? Well, it's happening now, and the implications are profound.
From voice-activated assistants like Siri and Alexa to sophisticated transcription services used in corporate environments, AI-driven speech recognition is making waves across various industries. The ability to convert spoken language into text with remarkable accuracy is not just a technical feat; it's a game changer for productivity and accessibility. In fact, the global speech recognition market is expected to reach $26.8 billion by 2025, reflecting the growing demand for this technology.
But what fuels these advancements? At its core, AI speech recognition relies on a combination of machine learning, natural language processing, and neural networks. These technologies work in tandem to enhance the accuracy and usability of speech recognition systems. As we delve deeper into this article, we'll explore how these core technologies are reshaping the landscape of communication.
Moreover, the applications of AI speech recognition are vast and varied. From healthcare to customer service, the versatility of this technology is evident. Imagine doctors dictating patient notes while still engaging with their patients, or customer service agents providing instant support through voice commands. The potential is limitless, and as the technology continues to evolve, so too will its applications.
However, it's essential to recognize that the journey is not without its challenges. Issues such as accent variability and background noise can hinder the effectiveness of speech recognition systems. As we explore these challenges, we will also discuss the ongoing research and innovations aimed at overcoming these hurdles.
As we look towards the future, the possibilities for AI speech recognition are exciting. With continuous advancements in technology, we can expect even greater accuracy, broader language support, and seamless integration into our daily lives. So, buckle up as we take a closer look at the historical context, core technologies, applications, challenges, and the bright future of AI-powered speech recognition!
- What is AI-powered speech recognition? - It's a technology that enables machines to understand and process human speech, converting it into text or commands.
- How does speech recognition work? - It uses algorithms and models trained on vast amounts of voice data to recognize and interpret spoken language.
- What are the main applications of AI speech recognition? - Applications include healthcare documentation, customer service automation, virtual assistants, and more.
- What challenges does AI speech recognition face? - Challenges include handling different accents, background noise, and the need for continuous learning to improve accuracy.
- What does the future hold for speech recognition technology? - The future looks promising with advancements likely to enhance accuracy, expand language support, and integrate into more everyday applications.

Historical Context of Speech Recognition
The journey of speech recognition technology is nothing short of fascinating. It all began in the 1950s when researchers first attempted to create systems that could understand human speech. Early systems, like IBM's Shoebox, could recognize a mere 16 words, which seems like child's play compared to today's standards. Imagine trying to have a conversation with a machine that could only respond to "yes" or "no"—frustrating, right? This rudimentary technology laid the groundwork for what was to come.
As the decades rolled on, significant advancements were made. The 1970s and 1980s saw the introduction of hidden Markov models (HMM), which revolutionized the field by providing a statistical framework for modeling speech. This was akin to giving machines a pair of glasses to see the world more clearly. Suddenly, they could understand not just isolated words but also the flow of speech, paving the way for more complex applications.
Fast forward to the 1990s, when the internet began to take off. The rise of machine learning and the availability of vast amounts of data transformed speech recognition from a theoretical concept into a practical tool. Researchers started using large datasets to train models, significantly improving accuracy. It's like teaching a child to speak by exposing them to conversations from various contexts; the more they hear, the better they get at understanding and responding.
Today, we stand at the pinnacle of this technological evolution. Modern AI-powered speech recognition systems employ deep learning techniques, such as recurrent neural networks (RNNs) and convolutional neural networks (CNNs), allowing them to process audio data with astonishing precision. These advancements have not only improved accuracy but also expanded the range of languages and dialects recognized by machines. It's as if we've handed over the reins to a highly intelligent assistant that learns and adapts in real time.
To summarize the historical context of speech recognition, here’s a quick table outlining key milestones:
Year | Milestone | Description |
---|---|---|
1952 | IBM Shoebox | First speech recognition system capable of recognizing 16 words. |
1970s | Hidden Markov Models | Statistical models introduced, improving flow and context understanding. |
1990s | Machine Learning | Large datasets utilized for training, enhancing accuracy. |
2010s-Present | Deep Learning | Advanced algorithms enable high-precision audio processing and language support. |
As we look back at this incredible journey, it’s clear that the evolution of speech recognition is a testament to human ingenuity and technological progress. Each advancement has brought us closer to a future where machines can understand and interact with us as seamlessly as another human would. The next sections will delve deeper into the core technologies that have made these advancements possible.
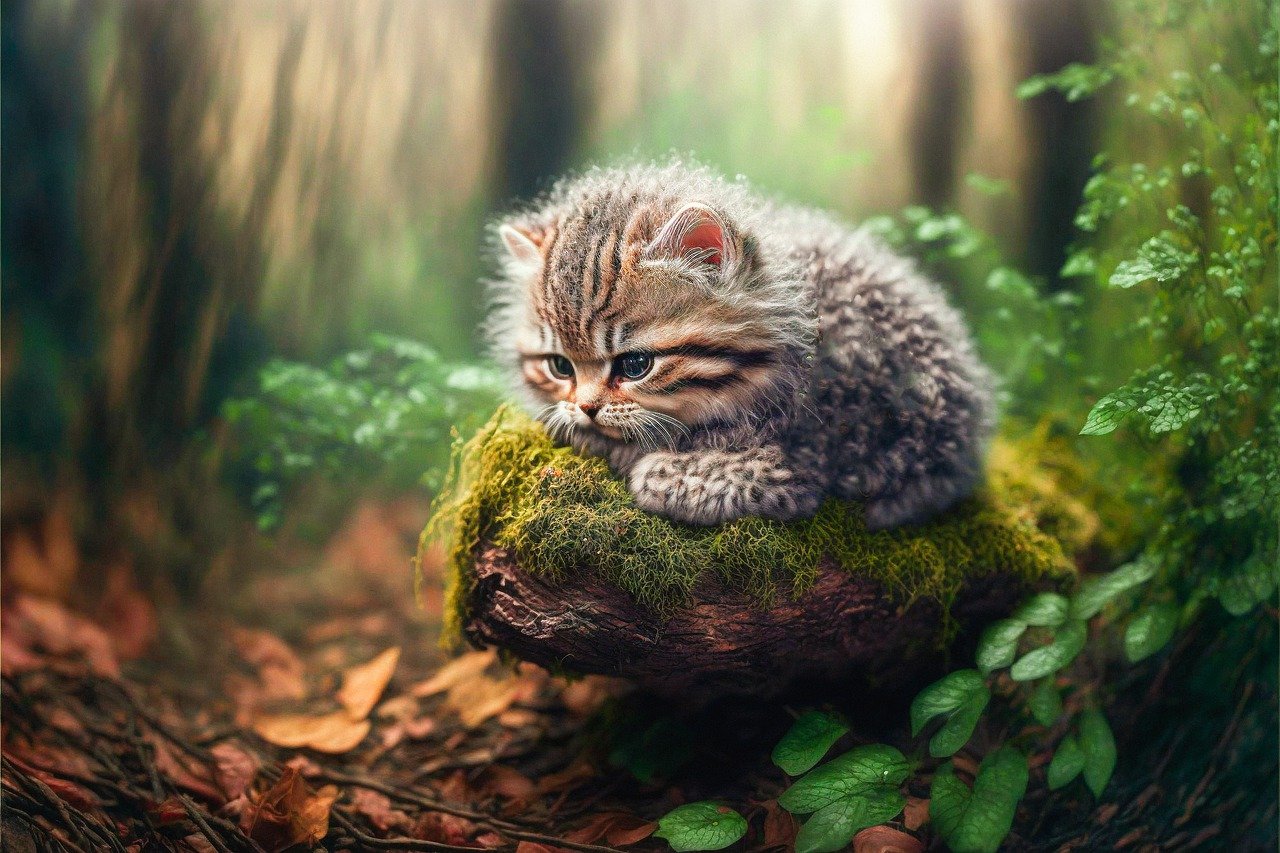
Core Technologies Behind AI Speech Recognition
The world of AI-powered speech recognition is nothing short of fascinating. At its core, this technology relies on a blend of several advanced fields that work together to decode human speech. Imagine trying to understand a foreign language while also interpreting the emotions behind the words—that's what these technologies aim to achieve! The primary components include machine learning, natural language processing (NLP), and neural networks. Each of these plays a pivotal role in transforming raw audio into comprehensible text and meaningful interactions.
Machine learning algorithms are the backbone of speech recognition systems. These algorithms are designed to learn from vast datasets, improving their accuracy over time. They analyze countless hours of spoken language, identifying patterns, phonetics, and contextual clues that humans naturally pick up on. For instance, when you speak to your virtual assistant, it doesn’t just recognize words; it learns from each interaction, adapting to your unique voice and speech patterns. This continuous training is what sets modern systems apart from their predecessors.
Within the realm of machine learning, there are two primary types of learning that are crucial for speech recognition: supervised learning and unsupervised learning. In supervised learning, models are trained on labeled datasets, meaning they learn from examples where the correct output is already known. This is akin to a student learning from a textbook, where they can see the correct answers and understand the reasoning behind them.
On the other hand, unsupervised learning involves training on data without explicit labels. This method is more like exploring a new city without a map—you gather experiences and insights without predefined routes. This distinction is vital, as it influences how models generalize to new, unseen data during real-time applications. The combination of both methods allows for a more robust understanding of language nuances.
Deep learning techniques, particularly recurrent neural networks (RNNs) and convolutional neural networks (CNNs), have revolutionized the speech recognition landscape. RNNs are particularly adept at handling sequences, making them ideal for processing audio data where the order of sounds matters. They can remember previous inputs, allowing the system to maintain context over longer conversations. In contrast, CNNs excel at extracting features from audio signals, enabling the system to recognize phonemes with remarkable precision.
Natural Language Processing (NLP) complements these technologies by enabling machines to grasp the context, intent, and emotional undertones of human language. Imagine having a conversation where your tone and choice of words convey deeper meanings—NLP helps machines decipher these intricacies, leading to more accurate transcriptions and enhanced user interactions. By understanding the subtleties of language, AI can provide responses that feel more human-like and engaging.
In summary, the core technologies behind AI speech recognition are a blend of sophisticated algorithms and models that mimic human learning and understanding. As these technologies continue to evolve, we can expect even more seamless and intuitive interactions with machines, making our lives easier and more connected.
- What is AI speech recognition?
AI speech recognition is a technology that allows machines to understand and interpret human speech, converting it into text or executing commands based on voice input. - How does machine learning improve speech recognition?
Machine learning improves speech recognition by allowing systems to learn from large datasets, continuously refining their accuracy and ability to understand different accents and dialects. - What role does natural language processing play?
NLP enhances speech recognition by helping systems understand the context and nuances of spoken language, leading to more accurate transcriptions and interactions. - What are the challenges faced by AI speech recognition?
Challenges include variability in accents, background noise interference, and the complexity of human language, which can affect the system's performance.
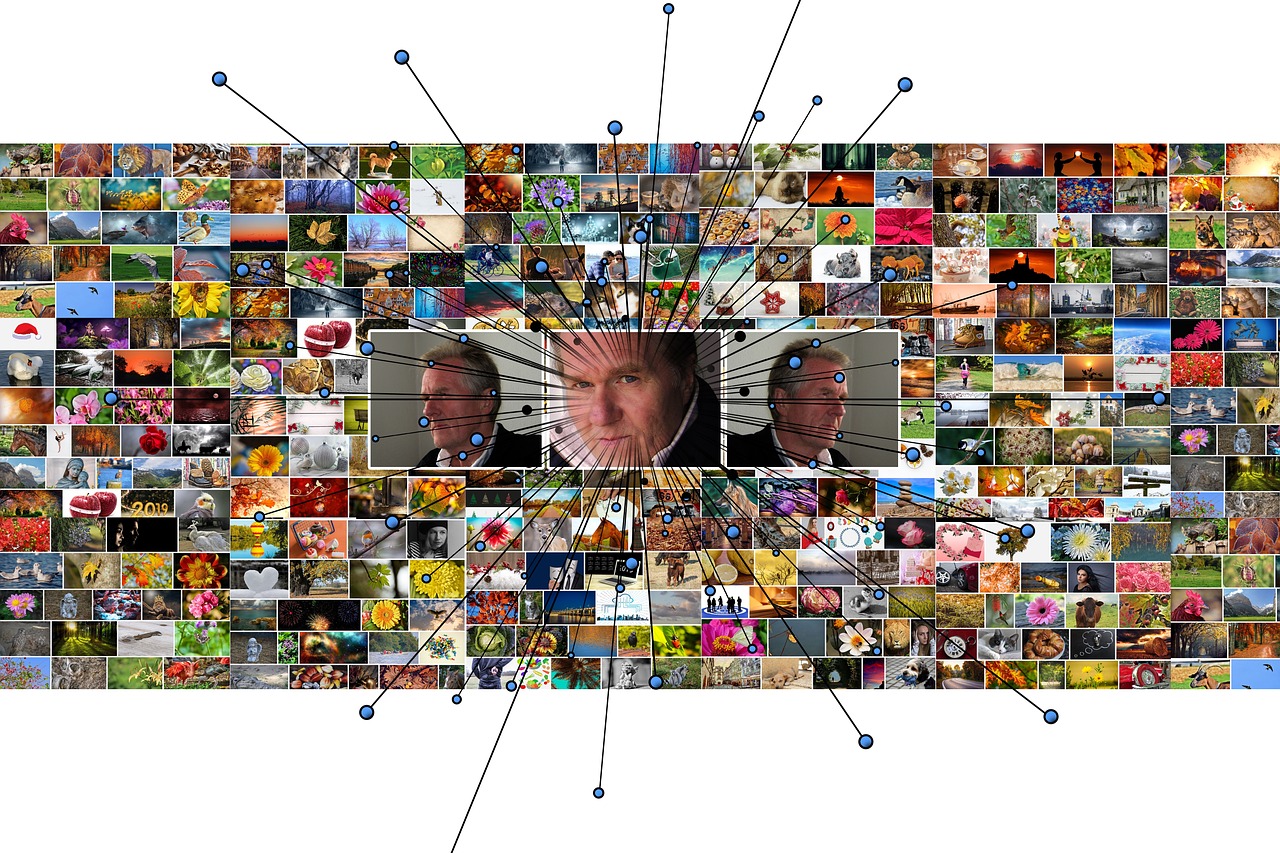
Machine Learning Algorithms
Machine learning algorithms are the backbone of modern AI-powered speech recognition systems. They act like the brain behind the scenes, tirelessly analyzing vast amounts of data to improve the accuracy and efficiency of voice recognition. Imagine teaching a child to recognize different animals by showing them countless pictures; similarly, these algorithms learn from extensive datasets containing audio samples and their corresponding transcriptions. This process allows them to adapt and refine their understanding over time, making them more proficient at recognizing speech patterns and nuances.
At the core of these algorithms lies the concept of training. During the training phase, the algorithms are exposed to labeled data, which includes both the audio input and the correct text output. This is where the magic happens. The algorithms learn to identify key features in the audio, such as phonemes and intonations, which are essential for accurate transcription. As they process more data, they become better at distinguishing between similar-sounding words and phrases, ultimately improving their performance in real-world applications.
There are several types of machine learning algorithms used in speech recognition, each with its unique strengths and weaknesses. Here’s a quick overview:
- Supervised Learning: In this approach, the algorithm is trained on labeled datasets. It learns to map input (audio) to output (text) by minimizing the error between its predictions and the actual labels.
- Unsupervised Learning: This method allows the algorithm to identify patterns in data without labeled outputs. It’s particularly useful for clustering similar audio samples, which can enhance the recognition process.
- Reinforcement Learning: This technique teaches algorithms to make decisions by rewarding them for correct actions and penalizing them for mistakes, enabling them to learn from their experiences.
One of the most exciting developments in machine learning for speech recognition is the advent of deep learning. This subset of machine learning utilizes artificial neural networks to model complex patterns in data. By employing architectures like recurrent neural networks (RNNs) and convolutional neural networks (CNNs), deep learning algorithms can process audio data more effectively than traditional methods. RNNs, for instance, are particularly adept at handling sequential data, making them ideal for speech recognition tasks where the order of words matters significantly.
As these algorithms continue to evolve, they are becoming increasingly capable of handling diverse languages and dialects, making speech recognition more accessible to users around the globe. However, it's essential to acknowledge that the journey is ongoing. The field is constantly pushing the boundaries of what’s possible, striving for systems that can understand not just the words we say but also the context and emotion behind them.
- What are machine learning algorithms? Machine learning algorithms are computational methods that allow systems to learn from data and improve their performance without being explicitly programmed.
- How do these algorithms improve speech recognition? They analyze large datasets of audio and text to identify patterns, allowing them to recognize speech more accurately over time.
- What is deep learning? Deep learning is a subset of machine learning that uses neural networks to model complex patterns in data, significantly enhancing the capabilities of speech recognition systems.
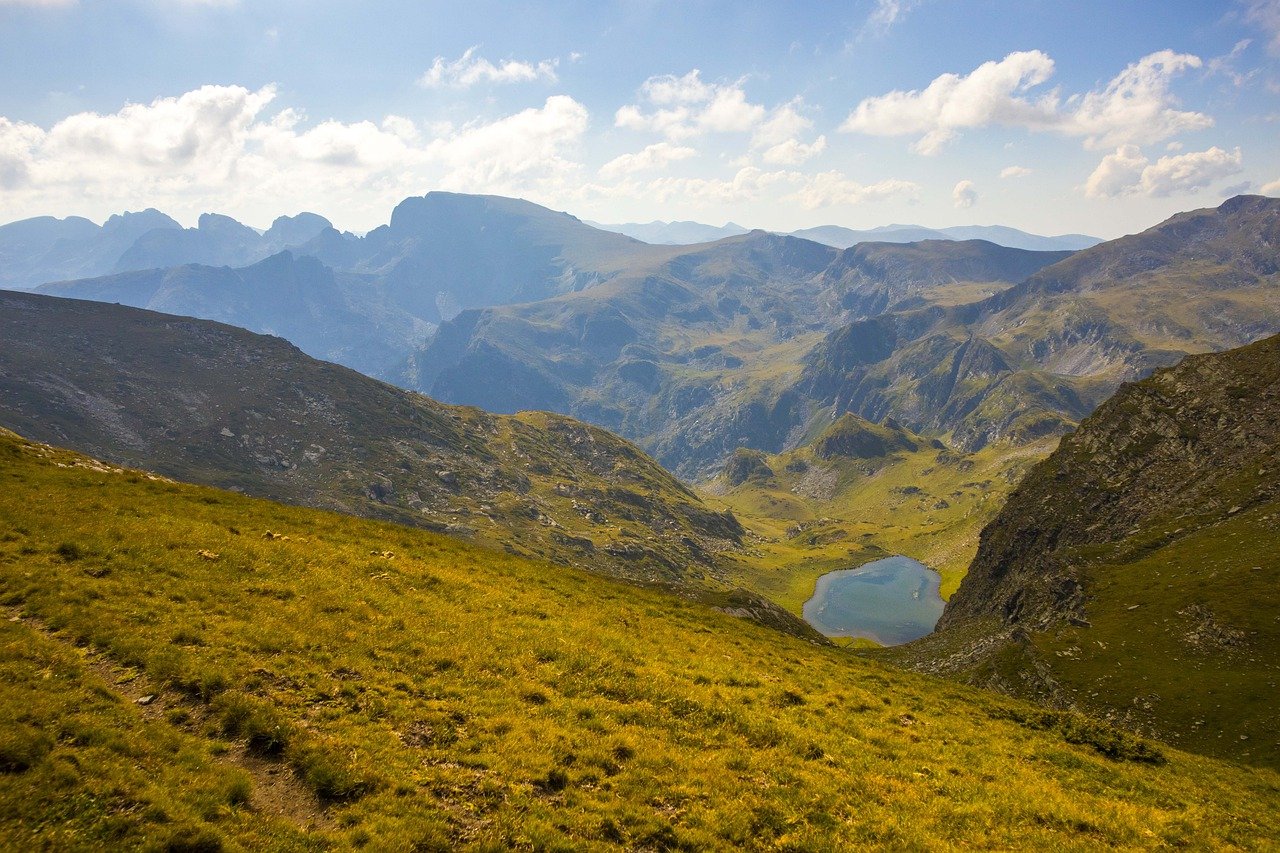
Supervised vs. Unsupervised Learning
When it comes to training AI speech recognition systems, understanding the difference between supervised and unsupervised learning is crucial. These two approaches represent different methodologies for teaching machines how to recognize and interpret human speech. In supervised learning, the model is trained on a labeled dataset, which means that each input (like an audio clip) is paired with the correct output (the corresponding text). This method allows the system to learn from examples, making it highly effective in achieving high accuracy in speech recognition tasks. For instance, if a system is trained with thousands of audio recordings of people saying "hello," along with their transcriptions, it can learn to recognize that particular phrase in future audio inputs.
On the other hand, unsupervised learning takes a different approach. In this scenario, the model is provided with data without explicit instructions on what to do with it. This means that the system must identify patterns and relationships within the data on its own. While this method can be less accurate initially, it has the advantage of being able to adapt to new and unseen data without requiring constant retraining. For example, an unsupervised learning model might analyze a vast collection of audio recordings and start to cluster similar sounds or phrases together, even if it doesn’t know what those sounds mean. This can be particularly useful in identifying new dialects or accents that were not part of the training dataset.
To illustrate the key differences, consider the following table:
Aspect | Supervised Learning | Unsupervised Learning |
---|---|---|
Data Type | Labeled data | Unlabeled data |
Goal | Predict outcomes based on input | Discover patterns and relationships |
Accuracy | High accuracy with sufficient data | Lower accuracy initially, adapts over time |
Use Cases | Speech-to-text transcription, sentiment analysis | Clustering, anomaly detection |
In the realm of AI speech recognition, both learning methods have their place. Supervised learning is often preferred when high accuracy is paramount, such as in medical transcription or legal documentation, where errors can have serious implications. Conversely, unsupervised learning shines in scenarios where the data is vast and varied, allowing the system to evolve and improve its understanding of language over time.
Ultimately, the choice between supervised and unsupervised learning in speech recognition systems depends on the specific application and the nature of the data available. As technology continues to advance, we may also see hybrid approaches that combine the strengths of both methods, leading to even more robust and adaptable speech recognition solutions.
- What is the main difference between supervised and unsupervised learning?
Supervised learning uses labeled data for training, while unsupervised learning uses unlabeled data to identify patterns. - Can unsupervised learning be used for speech recognition?
Yes, unsupervised learning can help identify new patterns in speech and adapt to different accents and dialects. - Which learning method is more accurate?
Supervised learning typically achieves higher accuracy with sufficient labeled data, while unsupervised learning may require more time to adapt.
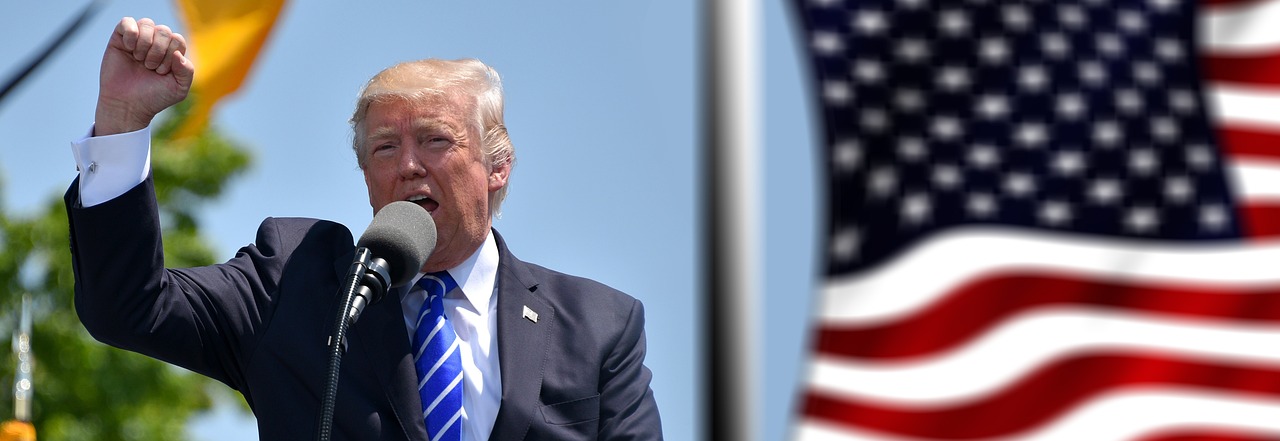
Deep Learning Techniques
Deep learning techniques have brought about a seismic shift in the realm of speech recognition. At the heart of this transformation are two primary architectures: Recurrent Neural Networks (RNNs) and Convolutional Neural Networks (CNNs). These advanced models allow systems to not only process audio data but also to understand and interpret the complex patterns within that data. Think of RNNs as a type of neural network designed to recognize sequences, much like how we, as humans, process language in a flow—where the meaning of a word is often influenced by the words that come before it.
RNNs are particularly effective in handling time-series data, making them ideal for tasks such as speech-to-text conversion. By maintaining a memory of previous inputs, RNNs can predict the next word in a sentence, significantly enhancing the accuracy of transcription. However, traditional RNNs can struggle with long sequences due to the vanishing gradient problem, which is where Long Short-Term Memory (LSTM) networks come into play. LSTMs are a specialized form of RNNs that can retain information over longer periods, making them highly effective for processing spoken language.
On the other hand, Convolutional Neural Networks (CNNs) excel in extracting spatial features from audio spectrograms, which are visual representations of the spectrum of frequencies in a sound signal as it varies with time. CNNs can analyze these spectrograms to detect phonetic elements, allowing the system to understand the nuances of speech better. The combination of RNNs and CNNs in a hybrid model can lead to even greater performance, as they leverage the strengths of both architectures to improve overall speech recognition capabilities.
To further illustrate the impact of these deep learning techniques, consider the following table that outlines their respective strengths:
Technique | Strengths |
---|---|
Recurrent Neural Networks (RNNs) | Ideal for sequential data, maintains contextual information, suitable for real-time applications. |
Long Short-Term Memory (LSTM) | Overcomes vanishing gradient problem, retains information over long sequences. |
Convolutional Neural Networks (CNNs) | Excellent at feature extraction, processes audio spectrograms effectively, captures phonetic nuances. |
As we continue to refine these deep learning techniques, the potential for more accurate and efficient speech recognition systems becomes increasingly clear. The marriage of RNNs and CNNs is just the beginning; future innovations may introduce even more sophisticated architectures that can further enhance our interactions with technology. Imagine a world where your voice is the key to unlocking seamless communication with machines, making everyday tasks easier and more intuitive. With deep learning leading the charge, that world is not far off.
- What is deep learning in speech recognition? Deep learning refers to the use of neural networks with many layers to analyze and interpret complex data, such as audio signals, improving the accuracy of speech recognition systems.
- Why are RNNs important for speech recognition? RNNs are crucial because they can process sequences of data, allowing them to remember previous spoken words and improve contextual understanding.
- How do CNNs contribute to speech recognition? CNNs help by analyzing audio spectrograms, identifying patterns and features that enhance the system's ability to understand spoken language.
- What are some challenges faced by deep learning in speech recognition? Challenges include dealing with diverse accents, background noise, and the need for large datasets to train the models effectively.
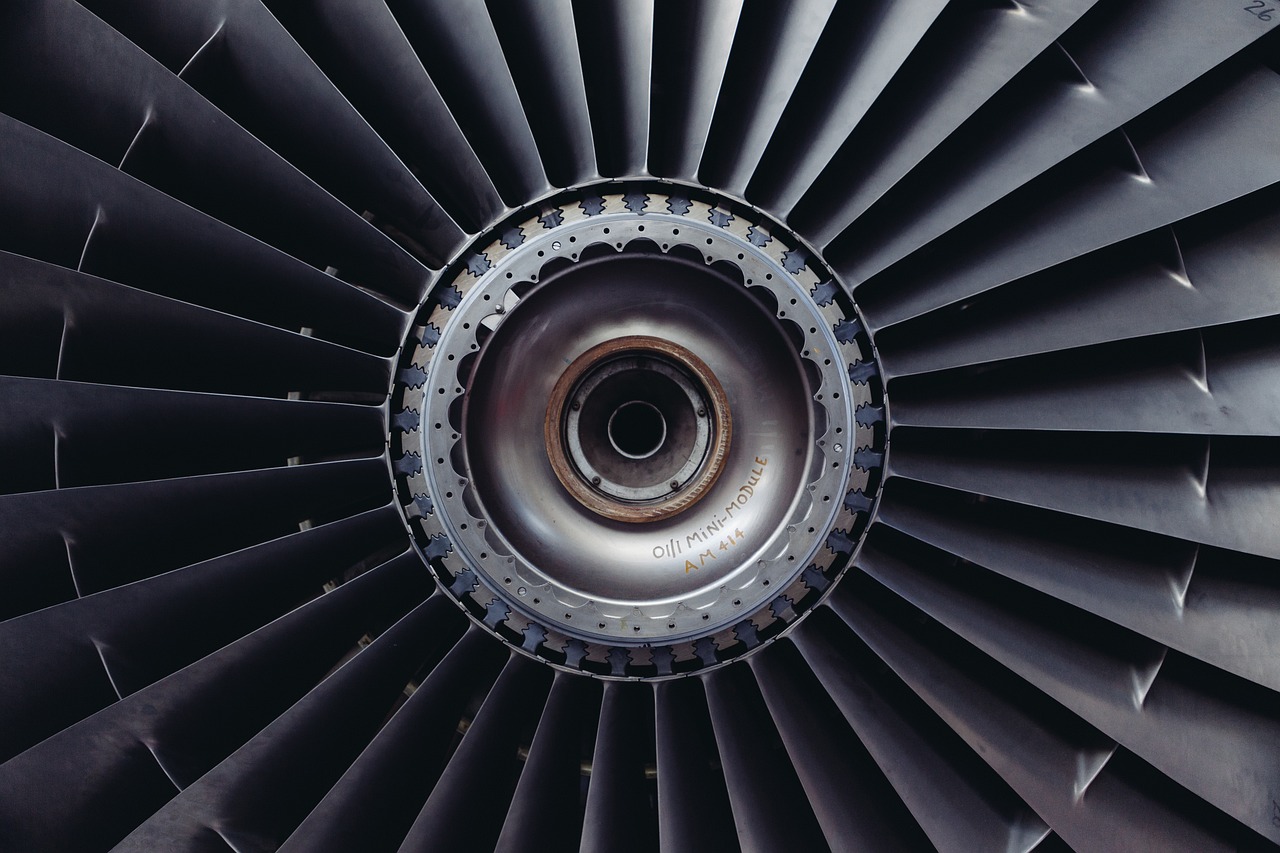
Natural Language Processing
Natural Language Processing, or NLP, is like the magic wand that helps machines understand human language, bridging the gap between man and machine. Imagine talking to your computer as if you were chatting with a friend, and it actually gets what you're saying! That's the beauty of NLP. This technology enables computers to process and analyze vast amounts of natural language data, allowing them to comprehend context, intent, and even the subtle nuances of human communication.
At its core, NLP combines several disciplines, including linguistics, computer science, and artificial intelligence, to create systems that can understand and respond to human language in a meaningful way. It’s not just about converting speech to text; it’s about understanding what the text means. NLP algorithms can analyze sentence structures, identify parts of speech, and even discern the sentiment behind a statement, which is crucial for effective communication.
The applications of NLP are diverse and impactful. For instance, in customer service, chatbots powered by NLP can understand customer inquiries and provide relevant responses, enhancing user experience. Similarly, in healthcare, NLP can assist in transcribing doctor-patient conversations, ensuring that important information is captured accurately. Here are some key areas where NLP shines:
- Sentiment Analysis: Understanding emotions behind words, helping businesses gauge customer satisfaction.
- Machine Translation: Translating languages in real-time, breaking down language barriers.
- Text Summarization: Condensing large amounts of text into concise summaries for quick understanding.
However, NLP is not without its challenges. The complexity of human language, with its idioms, slang, and regional dialects, can make it difficult for machines to interpret meanings accurately. Moreover, context plays a crucial role; the same word can have different meanings based on how it’s used. For instance, the word "bank" could refer to a financial institution or the side of a river. Thus, ongoing research is essential to refine NLP models, making them more robust and versatile.
In summary, Natural Language Processing is a pivotal technology that enables machines to interact with humans in an intuitive way. As advancements continue, we can expect even more sophisticated applications that will further enhance our interactions with technology, making it feel as natural as conversing with another person.
To wrap up, let's address some common questions about Natural Language Processing and its role in AI speech recognition.
- What is the main goal of NLP? The primary goal of NLP is to enable machines to understand, interpret, and generate human language in a way that is both meaningful and useful.
- How does NLP improve speech recognition? NLP enhances speech recognition by providing context and understanding nuances, which leads to more accurate transcriptions and interactions.
- Can NLP understand multiple languages? Yes, many NLP systems are designed to handle multiple languages, although their effectiveness can vary based on the complexity of the language.
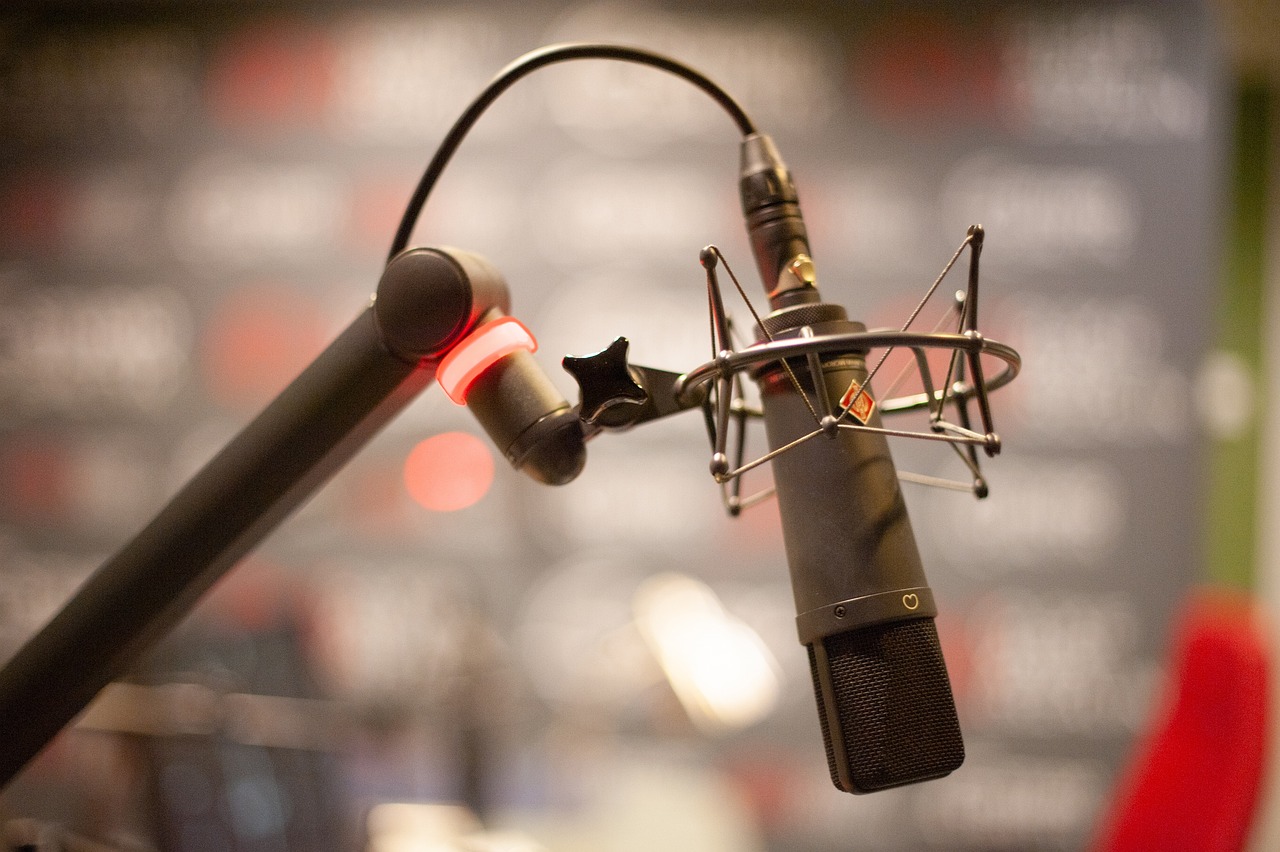
Applications of AI Speech Recognition
AI-powered speech recognition technology is not just a futuristic concept; it has become an integral part of our daily lives, influencing various sectors in profound ways. From healthcare to customer service, the applications of this technology are vast and varied, driving efficiency and enhancing user experiences. Imagine a world where you can interact with your devices and services using just your voice—this is the reality that AI speech recognition offers.
One of the most significant applications of AI speech recognition is in the healthcare sector. Medical professionals often find themselves bogged down by tedious documentation processes. With AI speech recognition, doctors can dictate notes and patient interactions directly into electronic health records, streamlining their workflow. This not only saves time but also improves the accuracy of patient records, as voice commands can capture details more quickly than traditional typing methods. Moreover, this technology allows healthcare providers to focus more on patient care rather than paperwork, which is a win-win for both doctors and patients alike.
In the realm of customer service, AI speech recognition is revolutionizing how businesses interact with their customers. Imagine calling a support line and instead of waiting for a representative, you can simply ask your question to an AI-powered system that understands and processes your request in real-time. This technology enables voice-activated support systems that provide instant responses, significantly improving response times and customer satisfaction. Furthermore, these systems can learn from previous interactions, allowing for a more personalized experience each time a customer reaches out for help.
Another area where AI speech recognition is making waves is in education. With the rise of online learning platforms, educators are leveraging this technology to create more interactive and engaging learning experiences. For instance, students can use voice commands to navigate through lessons, ask questions, or even participate in discussions, making learning more accessible and inclusive. Additionally, AI speech recognition can assist in language learning by providing real-time feedback on pronunciation and fluency, helping learners improve their skills more effectively.
Furthermore, the entertainment industry is also benefiting from AI speech recognition. From voice-controlled smart devices to interactive gaming experiences, users can engage with content in ways that were previously unimaginable. Imagine playing a video game where you can issue commands simply by speaking—this not only enhances the gaming experience but also makes it more immersive and enjoyable.
To summarize, AI speech recognition technology is not just a trend; it is a transformative force across multiple industries. Its ability to streamline processes, enhance user interaction, and improve efficiency is paving the way for a future where voice will be the primary mode of communication with machines. As this technology continues to evolve, we can expect to see even more innovative applications that will further integrate AI speech recognition into our everyday lives.
- What is AI speech recognition?
AI speech recognition is a technology that enables machines to understand and process human speech, allowing for voice commands and dictation. - How is AI speech recognition used in healthcare?
In healthcare, it streamlines documentation, allowing professionals to dictate notes directly into patient records, thus improving efficiency and accuracy. - Can AI speech recognition understand different accents?
While advancements have been made, understanding different accents remains a challenge, and ongoing research is focused on improving this aspect. - What are the future prospects of AI speech recognition?
The future looks promising, with expected advancements in accuracy, language support, and seamless integration into everyday applications.
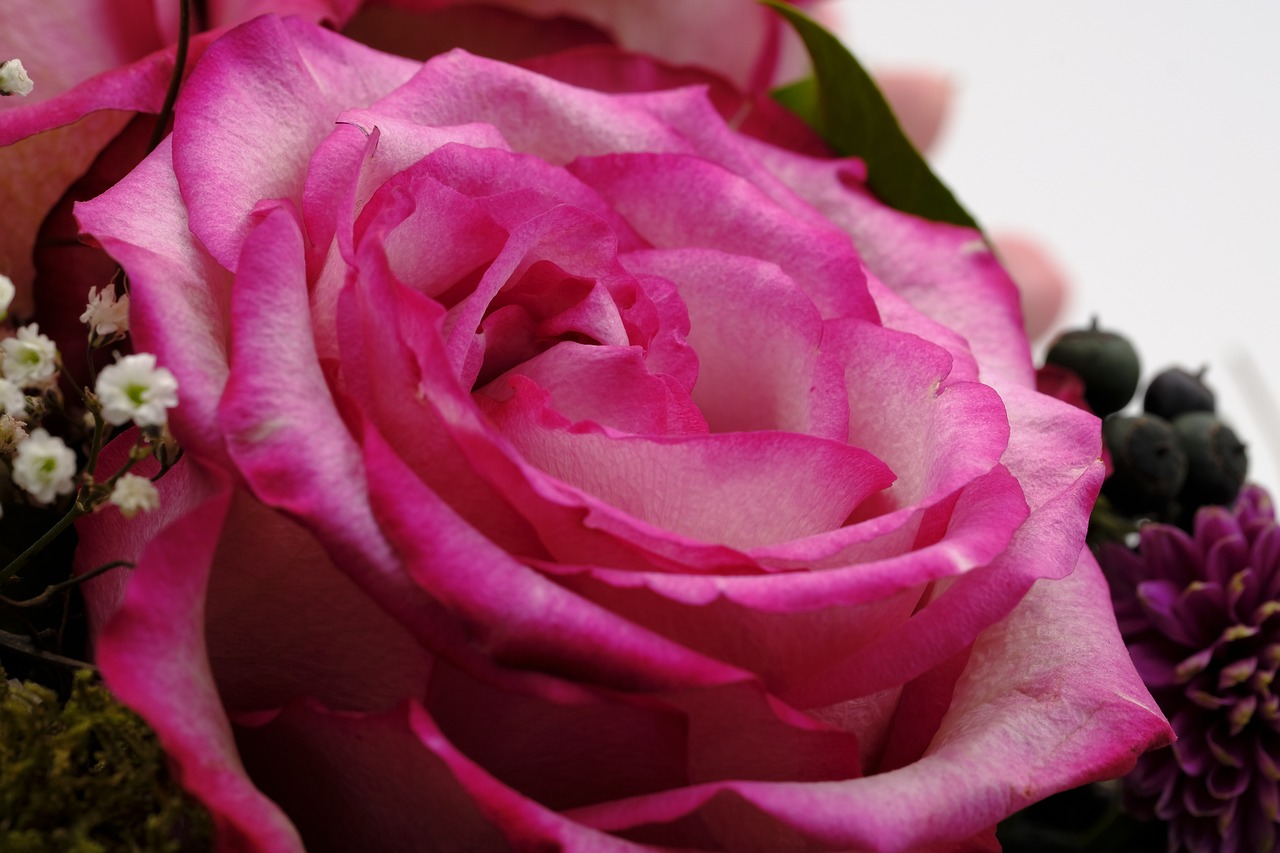
Healthcare Innovations
In the rapidly evolving world of healthcare, AI-powered speech recognition is making waves by revolutionizing how medical professionals document patient interactions and manage records. Imagine a bustling hospital where doctors are juggling patient care, paperwork, and endless notes. Now, picture them dictating their observations and treatment plans directly into a system that accurately captures their words in real-time. This is not just a dream; it’s a reality made possible by advancements in speech recognition technology.
One of the most significant benefits of AI speech recognition in healthcare is its ability to streamline documentation processes. Traditionally, healthcare providers spent countless hours filling out forms and entering data into electronic health records (EHRs). This not only consumed valuable time that could be spent with patients but also contributed to burnout among healthcare workers. With AI speech recognition, physicians can now use voice commands to dictate notes, which are then transcribed accurately and swiftly into the system. This means they can focus more on patient care rather than paperwork.
Moreover, the integration of AI speech recognition into healthcare systems enhances accuracy and efficiency. By utilizing advanced algorithms, these systems can learn medical terminology and adapt to the specific language used in healthcare settings. This leads to fewer errors in documentation, which is crucial for patient safety and compliance with regulations. For instance, a study revealed that hospitals using AI speech recognition saw a significant reduction in documentation errors, leading to improved patient outcomes.
Another exciting aspect of AI in healthcare is its ability to facilitate telemedicine. As more healthcare providers offer virtual consultations, the need for efficient communication tools has grown. AI speech recognition allows doctors to conduct remote consultations while documenting the conversation simultaneously. This ensures that all relevant information is captured without interrupting the flow of the consultation, making virtual visits as effective as in-person appointments.
However, while the benefits are clear, it’s essential to acknowledge the challenges that come with implementing AI speech recognition in healthcare. For example, variations in accents and dialects can affect the system's accuracy. Additionally, some healthcare professionals may be hesitant to adopt new technologies, fearing that they may disrupt their workflow. To address these concerns, ongoing training and support are crucial to ensure that all staff members feel comfortable using these innovative tools.
In conclusion, the innovations brought by AI-powered speech recognition in healthcare are nothing short of transformative. By enhancing documentation processes, improving accuracy, and facilitating telemedicine, this technology is paving the way for a more efficient and patient-centered healthcare system. As we look to the future, it's exciting to think about how these advancements will continue to shape the way healthcare is delivered, ultimately leading to better patient care and outcomes.
- What is AI-powered speech recognition?
AI-powered speech recognition refers to the technology that enables machines to understand and transcribe spoken language into text using artificial intelligence algorithms. - How does AI speech recognition benefit healthcare?
It streamlines documentation, reduces errors, enhances telemedicine, and allows healthcare professionals to focus more on patient care. - What challenges does AI speech recognition face in healthcare?
Challenges include variations in accents, background noise, and resistance to adopting new technologies among healthcare professionals.
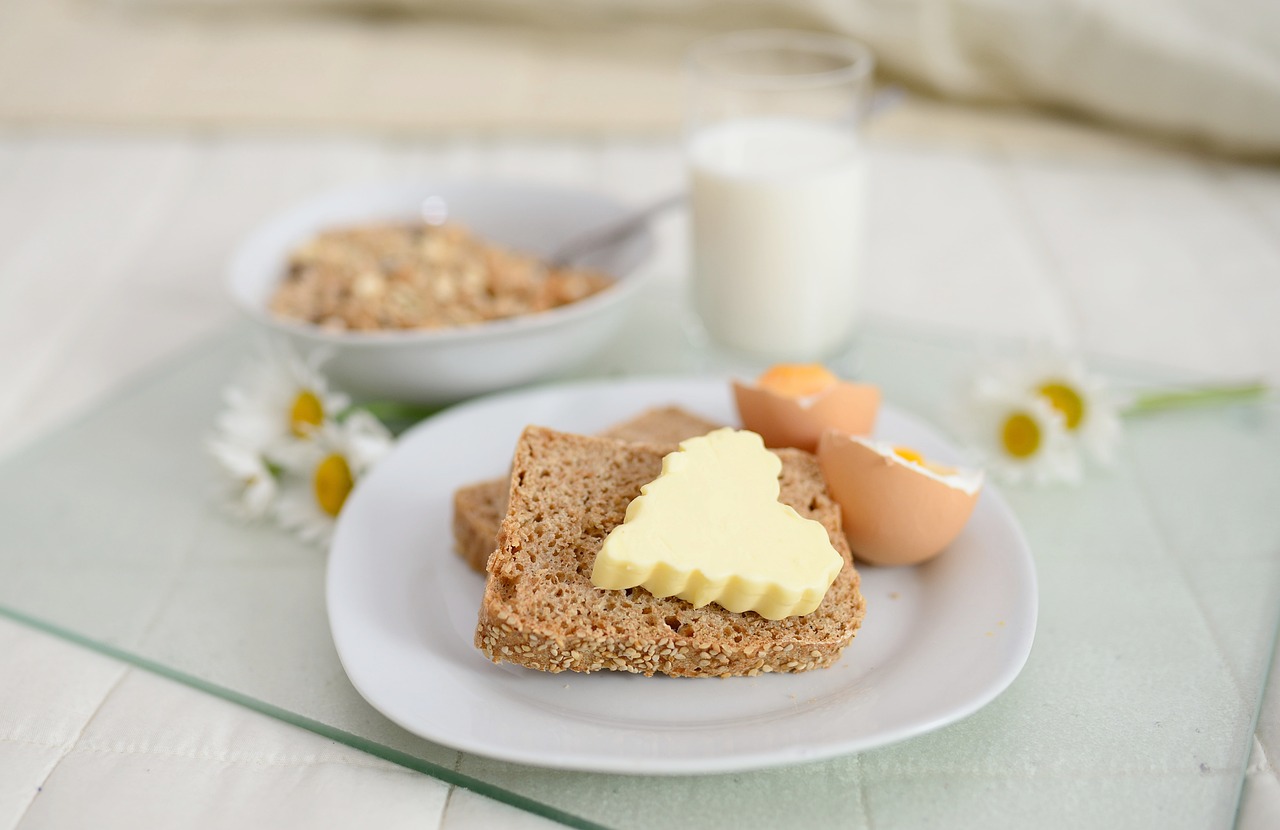
Customer Service Enhancements
In today's fast-paced world, customer service is more critical than ever. With the rise of AI-powered speech recognition, businesses are transforming the way they interact with customers. Imagine walking into a store or calling a service hotline, and instead of waiting on hold or dealing with complicated menus, you can simply speak your request. This is not just a dream—it's a reality made possible by advanced speech recognition technologies.
AI speech recognition systems are revolutionizing customer service by providing voice-activated support systems. These systems allow customers to engage with businesses in a more natural, conversational manner. For instance, instead of navigating through a series of automated prompts, customers can directly ask questions or state their needs. This leads to faster response times and a more satisfying experience overall.
Furthermore, the integration of AI in customer service enhances personalization. By using data analytics alongside speech recognition, businesses can tailor their responses based on previous interactions. When a customer calls, the AI can recognize their voice and pull up their history, allowing for a seamless and personalized experience. This not only improves customer satisfaction but also fosters loyalty, as customers feel valued and understood.
However, the benefits of AI speech recognition in customer service go beyond just speed and personalization. These systems can also handle a higher volume of inquiries simultaneously, reducing wait times significantly. For example, during peak hours, while human agents may be overwhelmed, AI can effectively manage multiple requests, ensuring that no customer is left waiting.
Moreover, AI-powered chatbots are becoming increasingly sophisticated. They can now understand complex queries and provide relevant responses, often resolving issues without the need for human intervention. This not only saves time for customers but also allows human agents to focus on more intricate problems that require a personal touch. The result? A more efficient and effective customer service operation.
To illustrate the impact of AI speech recognition in customer service, consider the following table:
Feature | Traditional Customer Service | AI-Powered Customer Service |
---|---|---|
Response Time | Long wait times | Instant responses |
Personalization | Generic responses | Tailored interactions |
Volume Handling | Limited capacity | High capacity |
Issue Resolution | Human intervention required | Automated solutions available |
As we move forward, it's clear that AI speech recognition is not just a trend; it’s a fundamental shift in how businesses approach customer service. By embracing these technologies, companies can provide a level of service that was previously unimaginable. Customers can expect a more intuitive, responsive, and personalized experience, which ultimately leads to greater satisfaction and loyalty.
- What is AI-powered speech recognition?
AI-powered speech recognition refers to technology that enables computers to understand and process human speech, allowing for voice-activated interactions.
- How does AI improve customer service?
AI enhances customer service by providing instant responses, personalizing interactions, and handling a higher volume of inquiries without long wait times.
- Can AI systems understand different accents?
While AI systems are improving in this area, challenges remain with certain accents and dialects, necessitating ongoing advancements in technology.
- Are AI chatbots capable of resolving complex issues?
AI chatbots are becoming increasingly sophisticated and can handle many inquiries independently, but complex issues may still require human intervention.
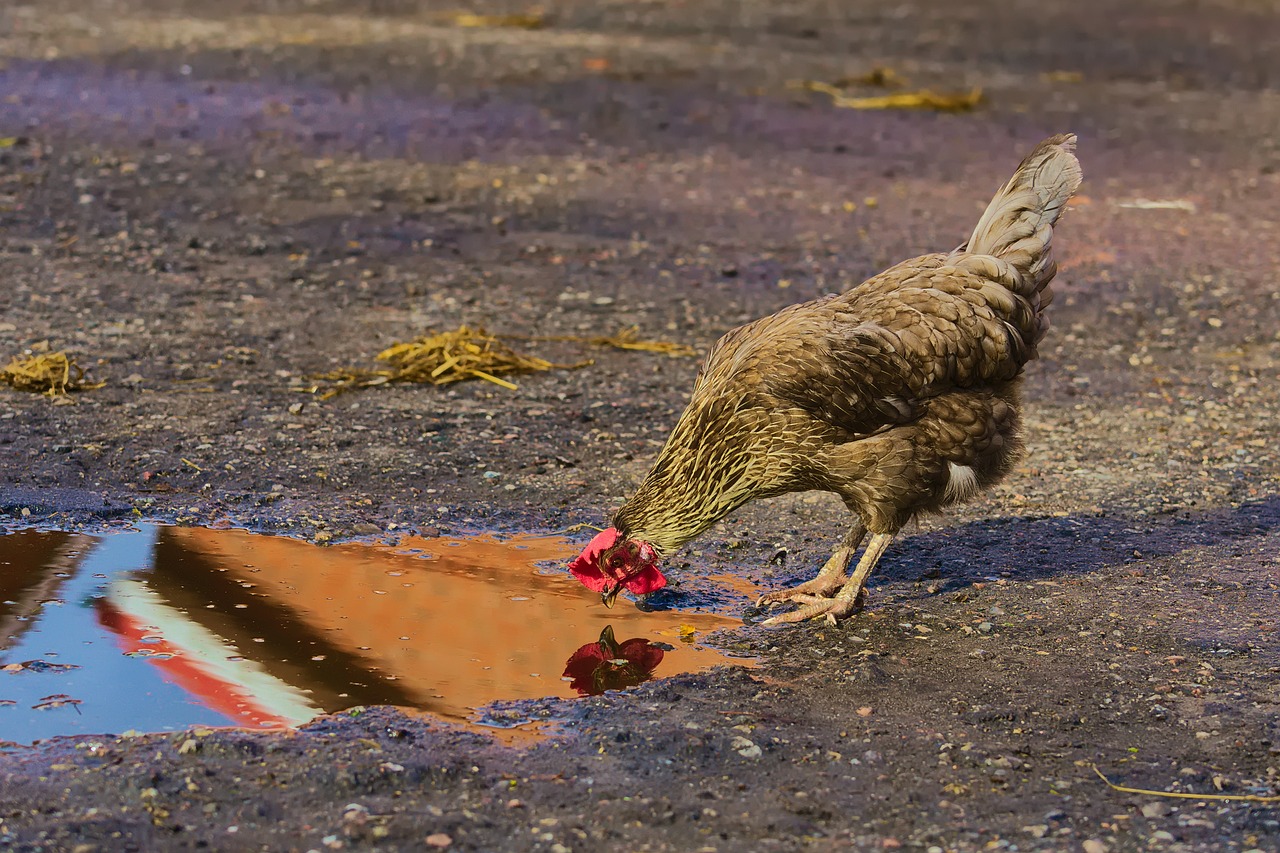
Challenges and Limitations
Despite the remarkable advancements in AI-powered speech recognition technology, it is not without its . One of the most significant hurdles is the variability in accents and dialects. With a world filled with diverse languages and regional pronunciations, AI systems often struggle to accurately interpret speech from different speakers. This can lead to frustrating experiences for users, particularly in multilingual environments where clear communication is essential. Imagine trying to have a conversation with a friend who speaks a different dialect; you might find yourself constantly asking them to repeat themselves. This is the reality for many users of speech recognition technology.
Another critical challenge is the impact of background noise. In real-world scenarios, conversations rarely take place in perfectly quiet environments. Whether in a bustling café, a busy office, or even at home with family members chatting in the background, noise can significantly hinder the performance of AI speech recognition systems. As a result, developers are continuously working on advanced noise-cancellation techniques and improved algorithms to enhance clarity and accuracy in such conditions. For instance, using directional microphones and sophisticated filtering methods can help mitigate these issues, but they are not foolproof.
Furthermore, the diversity of languages poses another layer of complexity. While many speech recognition systems are proficient in widely spoken languages like English, Spanish, or Mandarin, they often fall short when it comes to less common languages or dialects. This limitation can alienate users who speak these languages, creating a gap in accessibility and usability. To bridge this gap, ongoing research and development are crucial, focusing on expanding language support and ensuring that speech recognition technology is inclusive and effective for all users.
In summary, while AI-powered speech recognition has transformed how we interact with technology, it still faces significant challenges that require attention and innovation. Addressing issues related to accents, background noise, and language diversity is essential for enhancing the user experience and ensuring that this technology is truly universal. As we look to the future, it is clear that ongoing research and development will be vital in overcoming these hurdles and unlocking the full potential of speech recognition systems.
- What are the main challenges faced by AI speech recognition technology?
AI speech recognition technology faces challenges such as accent and dialect variability, background noise interference, and limitations in language support. - How does background noise affect speech recognition?
Background noise can distort audio signals, making it difficult for AI systems to accurately transcribe spoken language, leading to misunderstandings and errors. - What advancements are being made to improve speech recognition accuracy?
Developers are focusing on advanced noise-cancellation techniques, improved algorithms, and expanding language support to enhance the accuracy and usability of speech recognition technology.
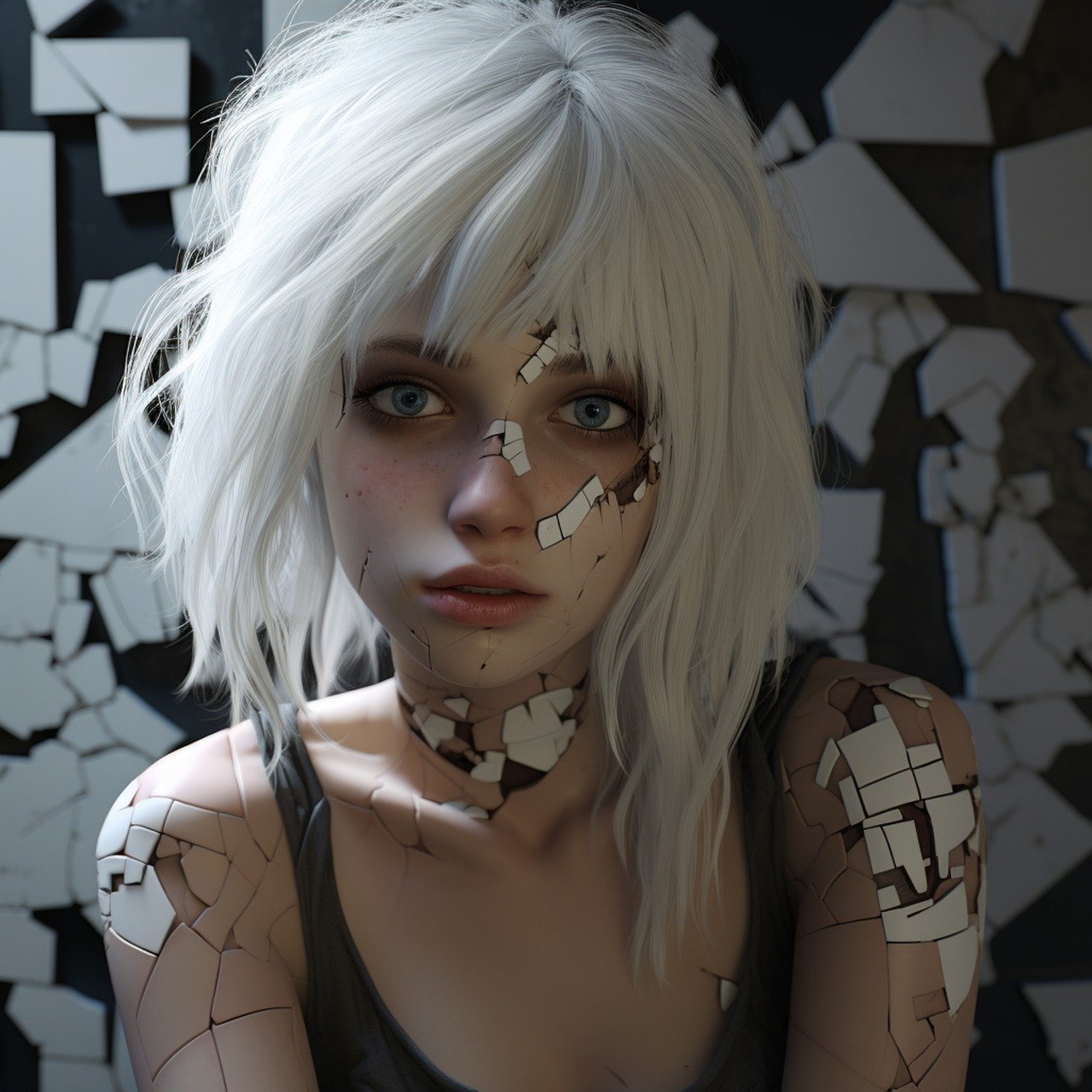
Accent and Dialect Variability
One of the most fascinating yet challenging aspects of AI-powered speech recognition is its ability to understand accents and dialects. Imagine trying to decipher a conversation in a crowded café where multiple languages and accents blend into a symphony of sounds. For AI systems, this is not just a challenge; it’s a complex puzzle that requires sophisticated algorithms and extensive training data. Accents can vary significantly not just from country to country but also within regions of the same country. For instance, the way someone speaks in New York can be quite different from someone in Texas, both of whom are speaking English. This variability can lead to misunderstandings and errors in transcription, which can be particularly problematic in critical applications such as healthcare or legal settings.
To illustrate the impact of accent and dialect variability, consider the following table that highlights some common English accents and their unique characteristics:
Accent | Region | Characteristics |
---|---|---|
British | United Kingdom | Received Pronunciation, distinct vowel sounds |
American | United States | Rhotic accent, flat 'a' sounds |
Australian | Australia | Broad vowel sounds, unique slang |
Indian | India | Influenced by native languages, syllable-timed rhythm |
As this table shows, each accent carries its own set of phonetic nuances that can throw off even the most advanced speech recognition systems. The challenge lies in the fact that traditional models often rely on a limited dataset that may not encompass the vast array of accents present in the world today. This limitation can lead to a higher error rate when the system encounters speakers with unfamiliar pronunciations. To combat this, many developers are now focusing on creating more inclusive datasets that represent a wider variety of speech patterns.
Moreover, the challenge of dialect variability extends beyond mere pronunciation. Different regions might use different words or phrases to describe the same object or action. For example, what is known as a "soda" in the Midwest might be referred to as "pop" in the North or "coke" in the South. This lexical diversity can further complicate the AI's ability to accurately interpret spoken language.
In response to these challenges, researchers are employing advanced techniques such as transfer learning and data augmentation to create more adaptable models. These methods allow AI systems to learn from a broader range of speech patterns, thereby improving their ability to recognize and adapt to various accents and dialects. The goal is to develop a system that not only understands the words being spoken but also grasps the context and intent behind them, making interactions more natural and effective.
In summary, while the journey toward mastering accent and dialect variability in AI speech recognition is fraught with challenges, ongoing research and development hold the promise of more inclusive and accurate systems. As we continue to push the boundaries of technology, the hope is that AI will one day understand every voice, no matter how unique, creating a seamless bridge between humans and machines.
- What is accent variability in speech recognition?
Accent variability refers to the differences in pronunciation and speech patterns among speakers from different regions or backgrounds, which can affect how well AI systems understand spoken language. - How does dialect variability impact speech recognition?
Dialect variability includes differences in vocabulary and expressions used in different regions, which can lead to misinterpretations by AI systems if not properly trained on diverse datasets. - What are some solutions to improve speech recognition accuracy?
Solutions include using larger and more diverse datasets, employing advanced machine learning techniques, and continuously training models to adapt to new accents and dialects.
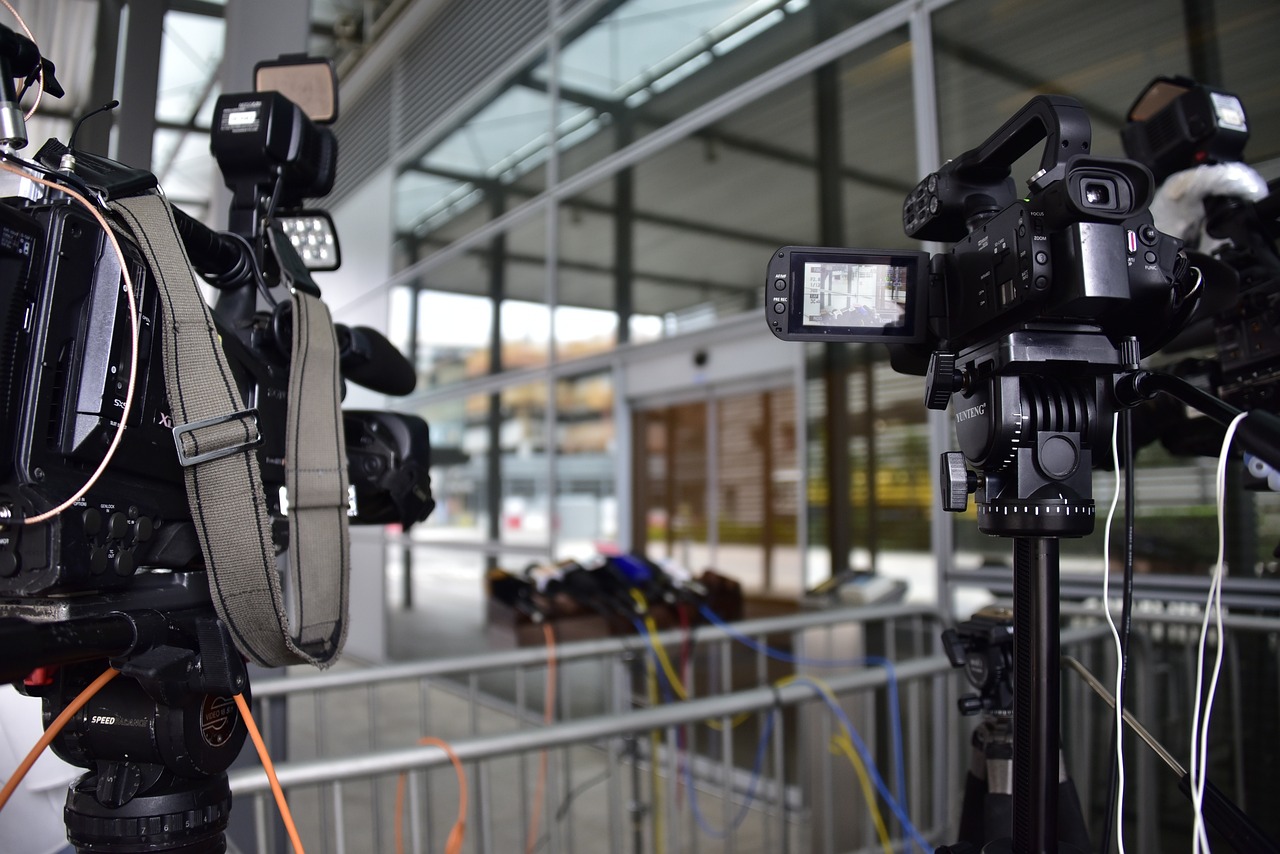
Background Noise Interference
Background noise interference is one of the most challenging obstacles facing AI-powered speech recognition systems today. Imagine trying to have a conversation in a crowded café, where the clinking of cups, chatter of patrons, and the hum of music all compete for your attention. This scenario mirrors the difficulties faced by speech recognition technologies when they attempt to accurately interpret spoken words amidst various distracting sounds. The presence of background noise can significantly degrade the performance of these systems, leading to misinterpretations and errors in transcription.
To combat this issue, developers are continuously working on advanced noise-cancellation techniques and improved algorithms. These innovations aim to filter out unwanted sounds and enhance the clarity of the primary voice input. For instance, techniques such as beamforming utilize multiple microphones to focus on the speaker's voice while minimizing surrounding noise. Additionally, machine learning models are being trained on diverse datasets that include various noise conditions, allowing them to learn how to distinguish between relevant speech and disruptive sounds.
Furthermore, the effectiveness of a speech recognition system in noisy environments can be influenced by several factors, including:
- Type of Noise: Different types of noise, such as white noise, chatter, or mechanical sounds, can affect recognition accuracy in unique ways.
- Volume Levels: The volume of background noise in relation to the speaker's voice plays a critical role; louder noises can completely overshadow speech.
- Distance from Microphone: The distance between the speaker and the microphone can also impact how well the system captures voice input amidst background noise.
Despite these challenges, the future of AI speech recognition remains bright. Researchers are optimistic that ongoing advancements in technology will lead to systems that can effectively operate in even the noisiest environments. This transformation will not only enhance user experience but also broaden the applicability of speech recognition technology in various fields, from customer service to healthcare.
- What is background noise interference?
Background noise interference refers to the sounds surrounding a person speaking, which can disrupt the accuracy of speech recognition systems. - How do AI systems combat background noise?
AI systems employ techniques such as beamforming and advanced algorithms to filter out background noise and focus on the speaker's voice. - Can speech recognition work in noisy environments?
While it is challenging, ongoing advancements in technology are improving the ability of speech recognition systems to operate effectively in noisy settings.
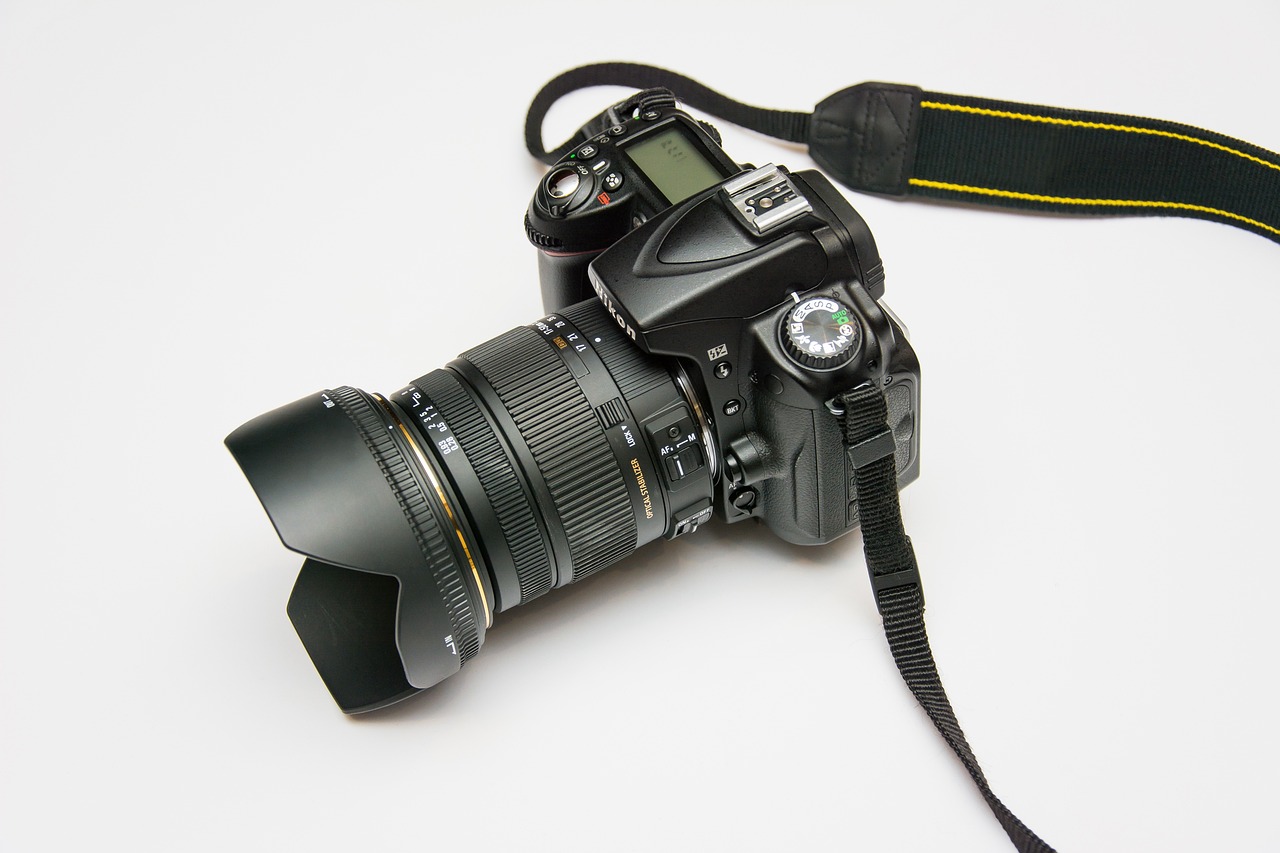
The Future of AI Speech Recognition
As we gaze into the horizon of technological advancements, the future of AI speech recognition appears not only promising but also exhilarating. Imagine a world where our interactions with machines are as natural as conversing with a friend. With the rapid evolution of AI technologies, we are on the brink of achieving just that. The integration of speech recognition into our daily lives is set to become more seamless, efficient, and intuitive.
One of the most exciting prospects is the enhancement of accuracy. Current systems are already impressive, but as algorithms continue to improve, we can expect speech recognition to become nearly flawless. This leap in accuracy will be driven by advancements in machine learning and deep learning techniques, which will enable systems to learn from a wider array of voices and accents. Think about it: a virtual assistant that understands you perfectly, no matter your accent or dialect. This is not just a dream; it’s a reality that’s inching closer.
Furthermore, the future will see a significant expansion in language support. Today, many speech recognition systems are limited to a handful of languages. However, as AI continues to evolve, we can anticipate a broader range of languages and dialects being supported, making technology accessible to more people around the globe. This inclusivity will not only enhance user experience but also foster communication across cultures, breaking down language barriers.
Integration into everyday applications is another area where AI speech recognition is set to shine. From smart home devices to customer service bots, the ability to interact with technology through voice commands will become a standard expectation rather than a novelty. Picture walking into your home and simply saying, “Turn on the lights” or “Play my favorite playlist,” and having your devices respond instantly and accurately. This level of convenience will revolutionize how we manage our daily tasks.
Moreover, as the technology matures, we can expect to see contextual understanding becoming a hallmark of AI speech recognition. Machines will not only recognize words but will also grasp the meaning behind them. This means that your virtual assistant could understand whether you’re making a joke or asking a serious question, allowing for more human-like interactions. Imagine asking your assistant to find a restaurant and it suggesting options based on your mood or previous choices—now that’s a game changer!
However, with great power comes great responsibility. As we advance, ethical considerations surrounding privacy and data security will be paramount. Users will need assurance that their voice data is handled securely and responsibly. The future will likely see stricter regulations and enhanced security measures to protect user information, ensuring that while we embrace this technology, we also safeguard our privacy.
In conclusion, the future of AI speech recognition is not just about improved accuracy or expanded language support. It’s about creating a more intuitive, inclusive, and secure interaction between humans and machines. As we stand on the cusp of this transformation, one can only wonder: how will our lives change when we can communicate effortlessly with the technology around us? The possibilities are endless, and the journey has only just begun.
- What advancements are expected in AI speech recognition?
Future advancements include improved accuracy, broader language support, and enhanced contextual understanding. - How will AI speech recognition impact daily life?
It will enable more natural interactions with technology, making tasks easier and more efficient. - Are there privacy concerns with AI speech recognition?
Yes, data security and user privacy will be critical issues that need to be addressed as the technology evolves.
Frequently Asked Questions
- What is AI-powered speech recognition?
AI-powered speech recognition refers to technology that enables computers to understand and process human speech using artificial intelligence. This technology has advanced significantly, allowing for more accurate transcriptions and better understanding of context and nuances in language.
- How has speech recognition technology evolved over the years?
The evolution of speech recognition technology has been remarkable. From its early days of simple voice command systems, it has progressed to sophisticated AI-driven solutions that utilize machine learning and natural language processing to enhance accuracy and usability.
- What are the core technologies behind AI speech recognition?
The core technologies include machine learning algorithms, natural language processing (NLP), and deep learning techniques. These technologies work together to improve the performance of speech recognition systems by enabling them to learn from data and understand human language better.
- What are some common applications of AI speech recognition?
AI speech recognition is used in various sectors such as healthcare, where it streamlines documentation, and customer service, where it enhances user interactions through voice-activated systems. It's also finding its way into education and entertainment, making it a versatile technology.
- What challenges does AI speech recognition face?
Despite its advancements, AI speech recognition grapples with challenges like accent and dialect variability, background noise interference, and the diversity of languages. These factors can hinder the accuracy and usability of the technology, prompting ongoing research to address these issues.
- How does background noise affect speech recognition accuracy?
Background noise can significantly interfere with the ability of speech recognition systems to accurately interpret spoken language. This necessitates the development of advanced noise-cancellation techniques and improved algorithms to ensure clear communication in noisy environments.
- What does the future hold for AI speech recognition?
The future of AI speech recognition looks bright! With continuous advancements in technology, we can expect enhanced accuracy, expanded language support, and seamless integration into everyday applications, fundamentally changing how we interact with machines.