The Intersection of AI and Quantum Physics
The convergence of artificial intelligence (AI) and quantum physics is not just a fascinating topic; it’s a thrilling ride into the future of technology and science. Imagine a world where machines can learn from quantum data, unraveling complexities that were once thought to be unsolvable. This intersection is akin to combining the powers of a supercomputer with the mysteries of the universe, creating a synergy that could redefine our understanding of everything from computational efficiency to drug discovery.
At its core, this relationship is built on the principles of quantum mechanics, which govern the behavior of particles at the smallest scales. Quantum physics challenges our traditional notions of reality, introducing concepts like superposition and entanglement. These principles offer a rich playground for AI algorithms, allowing them to process and analyze data in ways that classical systems simply cannot. As we delve deeper into this topic, we’ll explore how AI enhances quantum computing capabilities, paving the way for groundbreaking advancements across various domains.
Furthermore, the implications of merging these two fields extend far beyond theoretical discussions. Industries are already beginning to harness the power of AI and quantum physics, leading to innovations that could revolutionize everything from finance to pharmaceuticals. The potential applications are as vast as they are exciting, and as we stand at this crossroads, the possibilities are virtually limitless.
In this article, we’ll unpack the nuances of this intersection, examining how AI not only complements quantum physics but also propels it into new realms of possibility. We’ll look at the challenges that lie ahead, the potential applications in industries, and speculate on the future developments that await us. Buckle up, because this journey promises to be nothing short of exhilarating!
Before diving deeper into the intersection of AI and quantum physics, it’s essential to grasp the foundational concepts of quantum physics itself. At its essence, quantum physics is the study of matter and energy at the smallest scales—think atoms and subatomic particles. Unlike classical physics, which operates on predictable laws, quantum physics introduces a level of uncertainty and probability that can be mind-boggling.
Key principles of quantum physics include:
- Superposition: Particles can exist in multiple states at once until measured.
- Entanglement: Particles can become interconnected, such that the state of one instantly influences the state of another, regardless of distance.
- Quantum tunneling: Particles can pass through energy barriers that would be insurmountable in classical physics.
These principles are not just theoretical; they have profound implications for technology. Quantum physics is the backbone of quantum computing, which uses quantum bits or qubits to perform calculations at speeds unimaginable with classical computers. As we explore the role of AI in enhancing quantum computing, we begin to see how these two cutting-edge fields can work together to solve some of the most complex problems in science and technology.
Artificial intelligence plays a crucial role in maximizing the potential of quantum computing. The combination of these two fields allows researchers to tackle challenges that were previously insurmountable. For instance, AI algorithms can optimize quantum algorithms, improving their efficiency and accuracy. This is particularly important in error correction methods, which are vital for maintaining the integrity of quantum computations.
As we delve deeper into this topic, we will explore specific machine learning algorithms designed for quantum systems, such as:
- Quantum Support Vector Machines: These algorithms leverage quantum properties to enhance classification tasks.
- Variational Quantum Eigensolver: A hybrid approach that combines classical and quantum computing to find ground state energies of molecules.
By integrating AI with quantum computing, we not only enhance computational capabilities but also open doors to new applications that could revolutionize industries. The future is bright, and the intersection of these fields is a key driver of innovation.
Q: What is the significance of quantum physics in modern technology?
A: Quantum physics underpins many modern technologies, including semiconductors and lasers, and is crucial for the development of quantum computing, which promises to solve complex problems faster than classical computers.
Q: How does AI improve quantum computing?
A: AI enhances quantum computing by optimizing algorithms, improving error correction, and enabling more efficient data processing, making quantum technologies more practical and accessible.
Q: What industries can benefit from the AI and quantum physics intersection?
A: Industries such as pharmaceuticals, finance, and materials science are poised to benefit significantly from advancements at the intersection of AI and quantum physics, leading to innovations in drug discovery, financial modeling, and more.
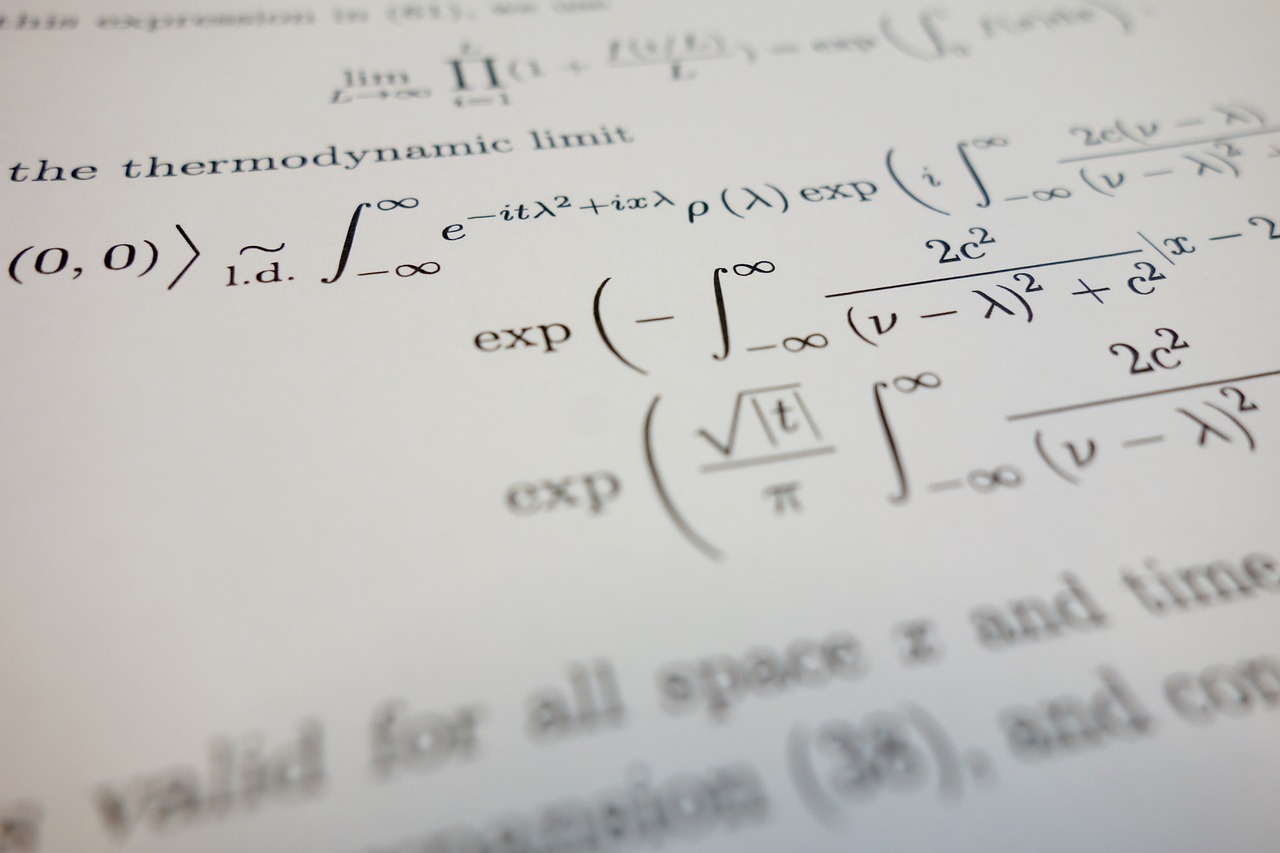
Understanding Quantum Physics
Quantum physics, often described as the science of the very small, delves into the behavior of matter and energy at the atomic and subatomic levels. Imagine a world where particles can exist in multiple states at once, where they can be entangled across vast distances, and where the act of observing something can fundamentally change its state. This is the strange yet fascinating realm of quantum mechanics, a field that has reshaped our understanding of the universe.
At its core, quantum physics challenges our classical intuitions. Traditional physics operates under the assumption that objects have definite positions and velocities, but quantum mechanics introduces the concept of superposition—the idea that particles can exist in multiple states simultaneously until measured. This principle is famously illustrated by Schrödinger's cat thought experiment, where a cat in a box can be both alive and dead until someone opens the box to observe it. This paradox highlights the counterintuitive nature of quantum phenomena.
Another key principle is quantum entanglement, where pairs of particles become interconnected in such a way that the state of one instantly influences the state of the other, no matter how far apart they are. This phenomenon baffled even Einstein, who referred to it as "spooky action at a distance." Such properties not only challenge our understanding of locality and causality but also pave the way for revolutionary technologies like quantum computing and quantum cryptography.
The significance of quantum physics extends far beyond theoretical musings; it has practical applications that are reshaping modern technology. For instance, the development of semiconductors, lasers, and MRI machines all stem from principles of quantum mechanics. Furthermore, as we stand on the brink of the quantum computing revolution, understanding these principles becomes essential for leveraging their potential in fields such as artificial intelligence.
In summary, quantum physics is not just a niche area of study but a fundamental aspect of our universe that influences various scientific disciplines. It challenges our perceptions, provokes our imaginations, and holds the key to future technological advancements. As we explore the intersection of quantum physics and artificial intelligence, we must first grasp these core concepts to appreciate the profound implications they hold for the future of technology and science.
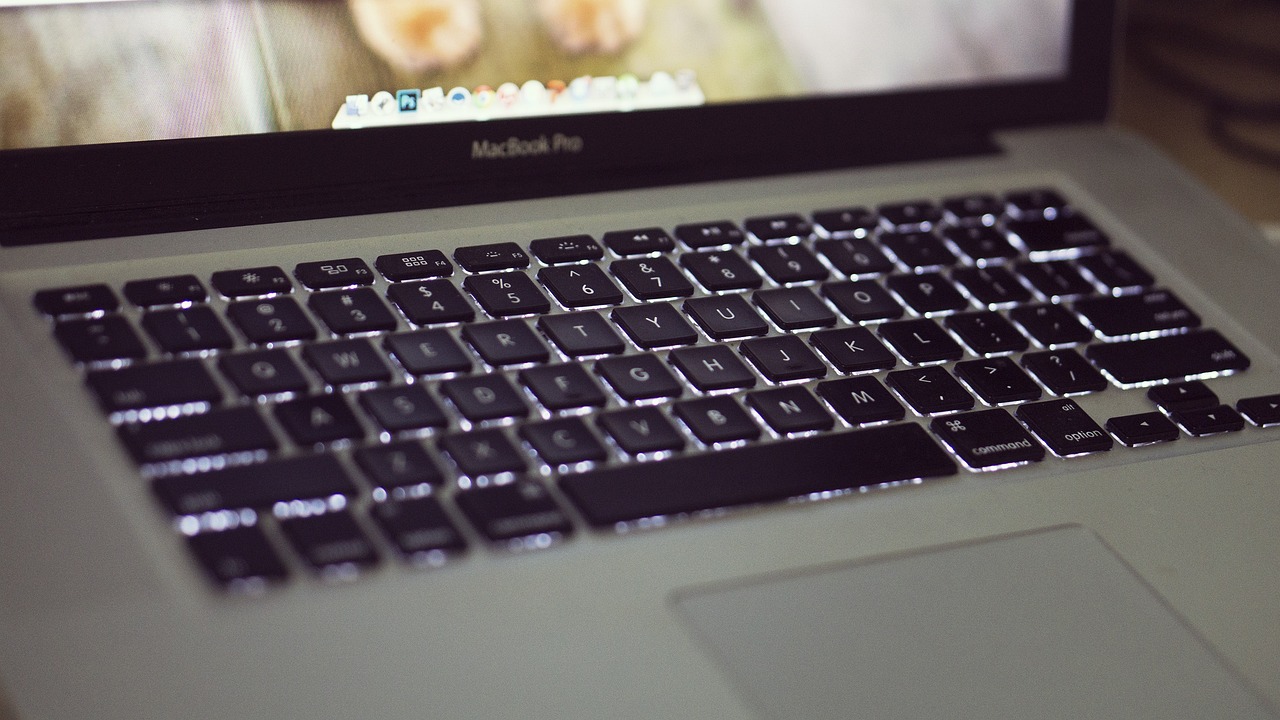
The Role of AI in Quantum Computing
Artificial Intelligence (AI) is revolutionizing numerous fields, and its impact on quantum computing is nothing short of extraordinary. Imagine a world where computers can solve complex problems at lightning speed, far beyond the capabilities of traditional machines. This is not just a fantasy; it's becoming a reality thanks to the synergy between AI and quantum computing. In this section, we will delve into how AI enhances quantum computing capabilities, focusing on algorithm optimization and error correction methods that significantly improve computational efficiency.
First, let’s talk about algorithm optimization. Quantum computers operate on principles that are fundamentally different from classical computers. They leverage quantum bits, or qubits, which can exist in multiple states simultaneously, allowing for an exponential increase in processing power. However, harnessing this power requires sophisticated algorithms. Here, AI steps in as a game-changer. By employing machine learning techniques, researchers can develop algorithms that are not only faster but also more efficient in navigating the complex landscapes of quantum states.
For instance, AI can analyze vast datasets generated by quantum experiments to identify patterns and optimize the parameters of quantum algorithms. This capability is akin to having a highly skilled navigator guiding a ship through treacherous waters, ensuring that the journey is not only swift but also safe. In this case, the waters are the intricate and often unpredictable realms of quantum mechanics.
Moreover, error correction is another critical area where AI shines. Quantum systems are notoriously prone to errors due to decoherence and other environmental factors. Traditional error correction methods can be cumbersome and inefficient. However, with AI's ability to learn and adapt, it can develop more effective strategies for error detection and correction. Imagine a skilled mechanic who can not only fix a car but also predict potential issues before they occur—this is the essence of AI in quantum error correction.
To give you a clearer picture, let’s consider a few key roles AI plays in quantum computing:
- Optimization of Quantum Algorithms: AI helps in fine-tuning algorithms to maximize their performance on quantum hardware.
- Error Correction: AI techniques enhance the robustness of quantum computations by minimizing errors.
- Resource Management: AI can efficiently allocate resources in quantum systems, ensuring optimal performance during computations.
As we explore the intersection of AI and quantum computing, we also encounter fascinating innovations such as quantum neural networks. These networks are designed to mimic the architecture of classical neural networks but operate within the quantum realm, leveraging the power of superposition and entanglement. This opens up new avenues for solving complex problems that were previously deemed unsolvable.
Furthermore, AI-driven quantum simulations are paving the way for groundbreaking research. By utilizing AI, researchers can simulate quantum phenomena that are incredibly challenging to observe directly. This capability not only accelerates the pace of scientific discovery but also enriches our understanding of the universe at a fundamental level.
In conclusion, the role of AI in quantum computing is multifaceted and transformative. From optimizing algorithms to enhancing error correction and facilitating advanced simulations, AI is the catalyst that propels quantum computing into new frontiers. As we continue to explore this exciting intersection, we can only imagine the myriad of possibilities that lie ahead.
Q: How does AI improve quantum computing?
A: AI enhances quantum computing by optimizing algorithms, improving error correction methods, and facilitating advanced simulations, thereby increasing computational efficiency.
Q: What are quantum neural networks?
A: Quantum neural networks are a type of neural network that operates on quantum principles, allowing them to solve complex problems more effectively than classical neural networks.
Q: Can AI help in drug discovery using quantum computing?
A: Yes, the combination of AI and quantum computing can significantly accelerate drug discovery processes by modeling molecular interactions with greater accuracy and speed.
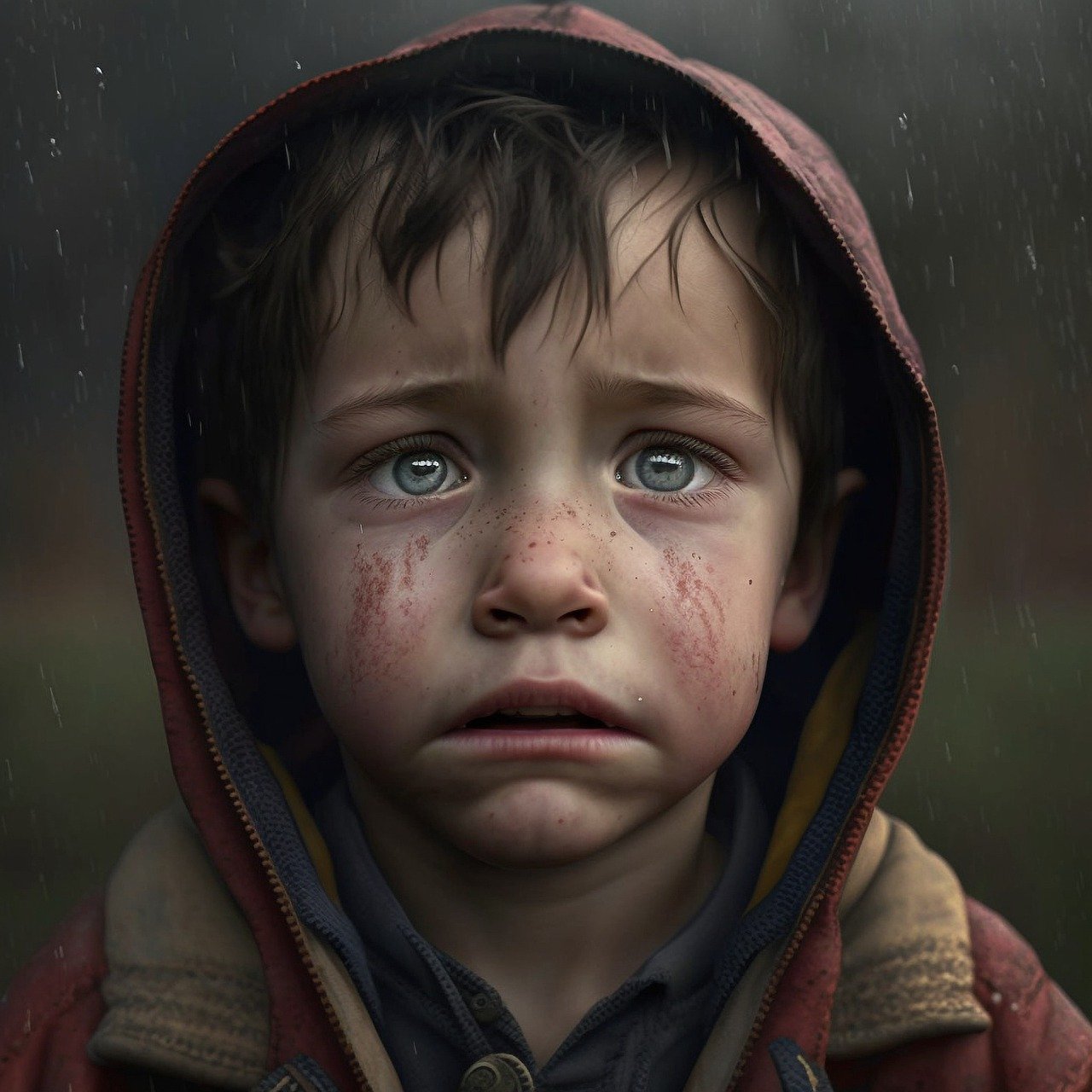
Machine Learning Algorithms in Quantum Systems
When we think about the future of technology, the fusion of machine learning and quantum systems stands out as a groundbreaking frontier. Imagine a world where computers can not only process information at unimaginable speeds but also learn from that data in ways we've only begun to understand. This is where machine learning algorithms come into play, specifically tailored for the unique challenges posed by quantum physics.
At its core, machine learning involves teaching computers to recognize patterns and make decisions based on data. In quantum systems, this concept becomes even more intricate due to the principles of superposition and entanglement. These principles allow quantum bits, or qubits, to exist in multiple states simultaneously, which means that traditional algorithms often fall short. Instead, we need algorithms that can harness the power of quantum mechanics to solve complex problems more efficiently.
One of the most promising approaches is the development of quantum-enhanced machine learning algorithms. These algorithms leverage the unique properties of quantum systems to improve learning processes. For instance, quantum algorithms can process vast amounts of data faster than classical counterparts, making them ideal for tasks such as:
- Classification: Identifying categories within data, which is crucial in fields like image recognition and natural language processing.
- Regression: Predicting outcomes based on input variables, essential for forecasting in various industries.
- Clustering: Grouping similar data points, which can help in market segmentation and customer analysis.
Moreover, specific machine learning models, such as quantum support vector machines and quantum Boltzmann machines, have been tailored to exploit quantum phenomena. These models can provide solutions to problems that are currently intractable for classical computers. For example, a quantum support vector machine can classify data points in a high-dimensional space more effectively than its classical counterpart, allowing for better accuracy in predictions.
However, the integration of machine learning into quantum systems is not without its challenges. Quantum data is often noisy and subject to errors, which can hinder the learning process. To combat this, researchers are developing quantum error correction techniques that aim to enhance the reliability of data processed by quantum algorithms. Additionally, the scarcity of quantum data presents another hurdle; machine learning thrives on large datasets, and the quantum realm is still relatively uncharted territory.
Nevertheless, the potential applications of these advanced algorithms are vast and varied. From optimizing logistics in supply chains to enhancing the accuracy of weather predictions, the intersection of machine learning and quantum systems promises to revolutionize numerous fields. As we continue to explore this exciting frontier, the collaboration between physicists, computer scientists, and engineers will be crucial in overcoming the challenges and unlocking the full potential of these technologies.
Q: What are quantum machine learning algorithms?
A: Quantum machine learning algorithms are specialized algorithms designed to leverage the principles of quantum mechanics to enhance the learning process and solve complex problems more efficiently than classical algorithms.
Q: How do quantum algorithms differ from classical algorithms?
A: Quantum algorithms can process information in ways that classical algorithms cannot, due to the properties of qubits, such as superposition and entanglement, allowing them to handle larger datasets and perform complex computations faster.
Q: What are some applications of machine learning in quantum systems?
A: Applications include classification, regression, and clustering tasks across various industries, such as finance, healthcare, and logistics, where enhanced data processing can lead to better insights and decision-making.
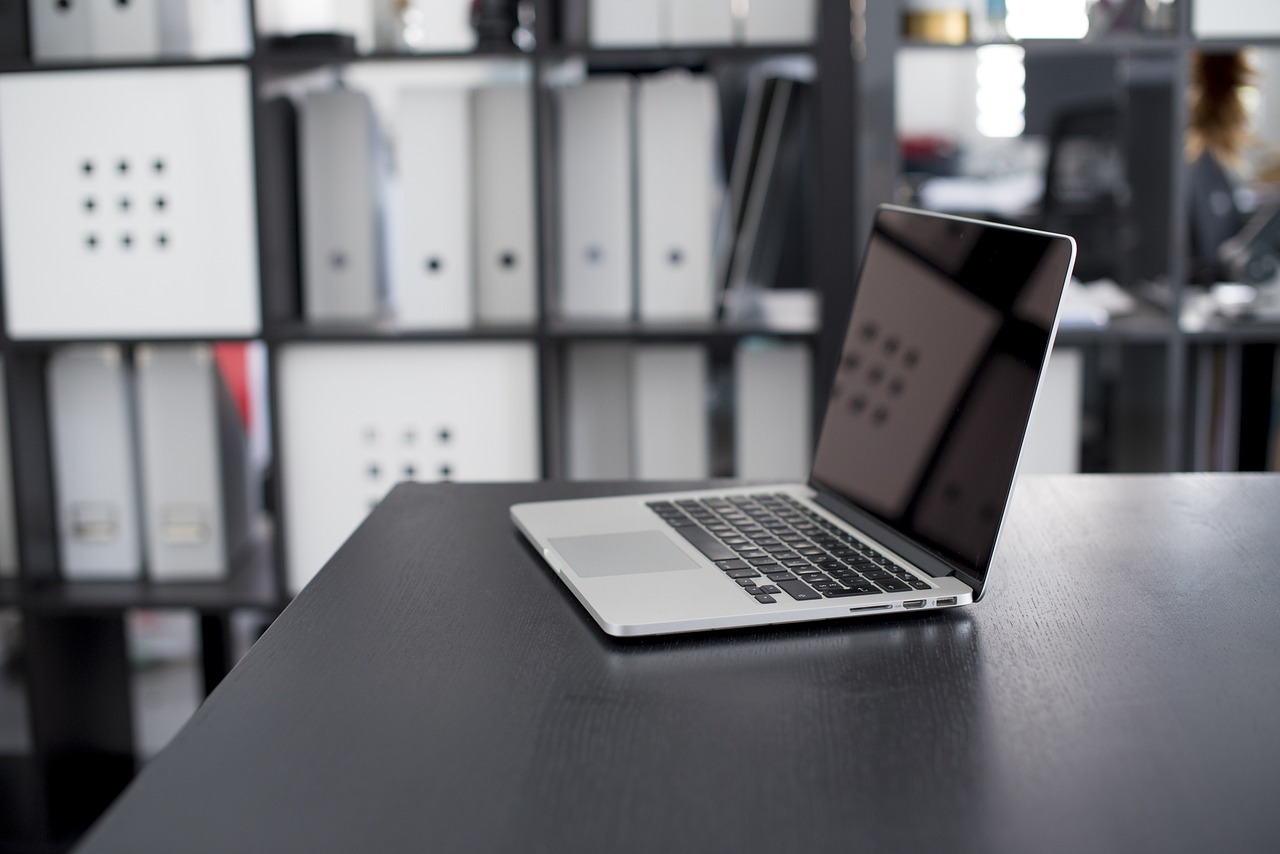
Quantum Neural Networks
When we talk about , we're diving into a realm where the laws of quantum physics blend seamlessly with the principles of machine learning. Imagine a neural network, the backbone of many AI applications, but supercharged with the capabilities of quantum mechanics. This fusion opens up a world of possibilities, allowing us to tackle complex problems that were once deemed impossible. But what exactly makes quantum neural networks so special?
At their core, quantum neural networks leverage the unique properties of quantum bits, or qubits, which can exist in multiple states simultaneously, thanks to a phenomenon known as superposition. This is a stark contrast to classical bits, which can only be either 0 or 1. By utilizing superposition, quantum neural networks can process vast amounts of information at unprecedented speeds. Think of it like having a multi-lane highway instead of a single lane; the traffic can flow much more efficiently, allowing for quicker data processing and analysis.
Moreover, quantum neural networks incorporate another quantum property called entanglement. This allows qubits that are entangled to be correlated with each other, no matter the distance separating them. In practical terms, this means that changes to one qubit can instantaneously affect another, which could lead to enhanced learning capabilities and faster convergence in training processes. Picture a group of friends who can communicate telepathically—while they may be far apart, they still manage to share ideas instantly. This is the kind of potential quantum entanglement brings to the table.
Now, you might be wondering how these networks differ from classical neural networks. While traditional neural networks rely on layers of interconnected nodes to process data, quantum neural networks can implement quantum gates that manipulate qubits in ways that classical gates cannot. This allows for a more complex representation of data and could lead to breakthroughs in areas like image recognition, natural language processing, and even optimization problems.
However, the journey to fully realize the potential of quantum neural networks is not without its challenges. The technology is still in its infancy, and creating stable quantum systems that can reliably perform computations is a significant hurdle. Additionally, developing algorithms that can effectively harness the power of quantum mechanics requires a deep understanding of both quantum physics and machine learning, making it a highly interdisciplinary field.
In conclusion, quantum neural networks represent a thrilling frontier in both AI and quantum computing. As researchers continue to explore and innovate in this space, we can anticipate a future where these networks not only enhance our understanding of quantum phenomena but also revolutionize practical applications across various industries. The possibilities are indeed as vast as the quantum realm itself!
- What are quantum neural networks? Quantum neural networks are a type of neural network that utilizes quantum bits (qubits) to process information, leveraging the principles of quantum mechanics.
- How do quantum neural networks differ from classical neural networks? Quantum neural networks use quantum gates to manipulate qubits, allowing for more complex data representation compared to classical neural networks that rely on interconnected nodes.
- What are the advantages of using quantum neural networks? They offer faster data processing, enhanced learning capabilities, and the potential to solve complex problems more efficiently due to superposition and entanglement.
- What challenges do quantum neural networks face? The main challenges include the stability of quantum systems, the development of effective algorithms, and the need for interdisciplinary collaboration.
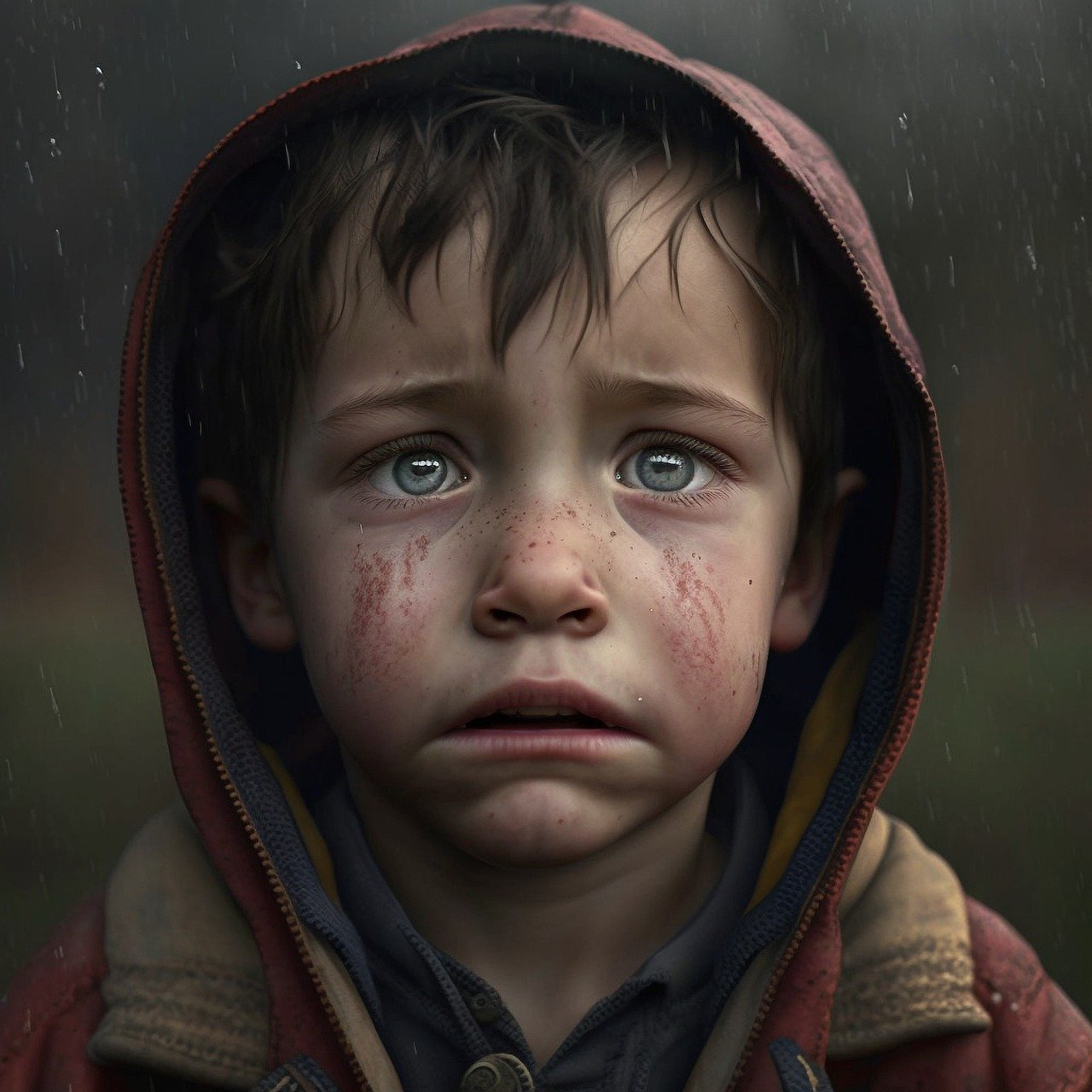
AI-Driven Quantum Simulations
In the rapidly evolving world of technology, the fusion of artificial intelligence (AI) and quantum physics has opened up a treasure trove of possibilities, particularly in the realm of simulations. Imagine trying to predict the behavior of a complex quantum system—it's like attempting to navigate a maze without a map. Traditional methods often fall short, but with AI, we can create a virtual compass that not only helps us find our way but also reveals hidden paths we never knew existed.
AI-driven quantum simulations are transforming how researchers model and understand quantum phenomena. By leveraging the vast computational power of quantum computers, combined with the analytical prowess of AI, scientists can simulate intricate systems that were previously deemed too complex to analyze. This synergy allows for the exploration of new materials, the understanding of fundamental particles, and the prediction of chemical reactions with unprecedented accuracy.
One of the most exciting aspects of AI-driven quantum simulations is their ability to handle massive datasets that typical computational methods struggle with. For instance, traditional simulations may take weeks or even months, but AI algorithms can process and analyze data in a fraction of the time. This speed not only accelerates research but also enhances the accuracy of results, allowing scientists to make informed decisions based on reliable data.
Moreover, AI can optimize the parameters of quantum simulations, ensuring that researchers can focus on the most relevant aspects of their studies. This optimization process is akin to fine-tuning a musical instrument; just as a musician adjusts their strings for the perfect sound, AI helps researchers adjust their simulations for optimal performance. By identifying the most critical variables and relationships, AI algorithms can significantly reduce the computational resources required, making quantum simulations more accessible to a broader range of researchers.
To illustrate the impact of AI-driven quantum simulations, consider the following table that highlights some key applications:
Application | Description | Benefits |
---|---|---|
Material Science | Simulating the properties of new materials at the quantum level. | Faster discovery of materials with desired properties. |
Chemical Reactions | Predicting the outcomes of complex chemical reactions. | More accurate predictions leading to efficient synthesis. |
Quantum Chemistry | Understanding molecular interactions and behaviors. | Enhanced drug design and development. |
As we delve deeper into the realm of AI-driven quantum simulations, it's essential to recognize the challenges that lie ahead. While the potential is enormous, integrating AI with quantum computing is not without its hurdles. Issues such as data scarcity, the need for interdisciplinary collaboration, and the technical limitations of current quantum computers must be addressed to fully harness this technology.
In conclusion, AI-driven quantum simulations represent a groundbreaking frontier in scientific research. They not only enhance our understanding of the quantum world but also pave the way for innovations across various fields, from materials science to pharmaceuticals. As we continue to explore this intersection, the future looks bright, with the promise of discoveries that could redefine our understanding of the universe.
- What are AI-driven quantum simulations? They are simulations that utilize artificial intelligence to model complex quantum systems, enhancing accuracy and speed.
- How do AI and quantum physics work together? AI optimizes quantum simulations, processes large datasets, and helps researchers focus on critical variables.
- What industries benefit from AI-driven quantum simulations? Industries such as pharmaceuticals, materials science, and finance stand to gain significantly from these advancements.
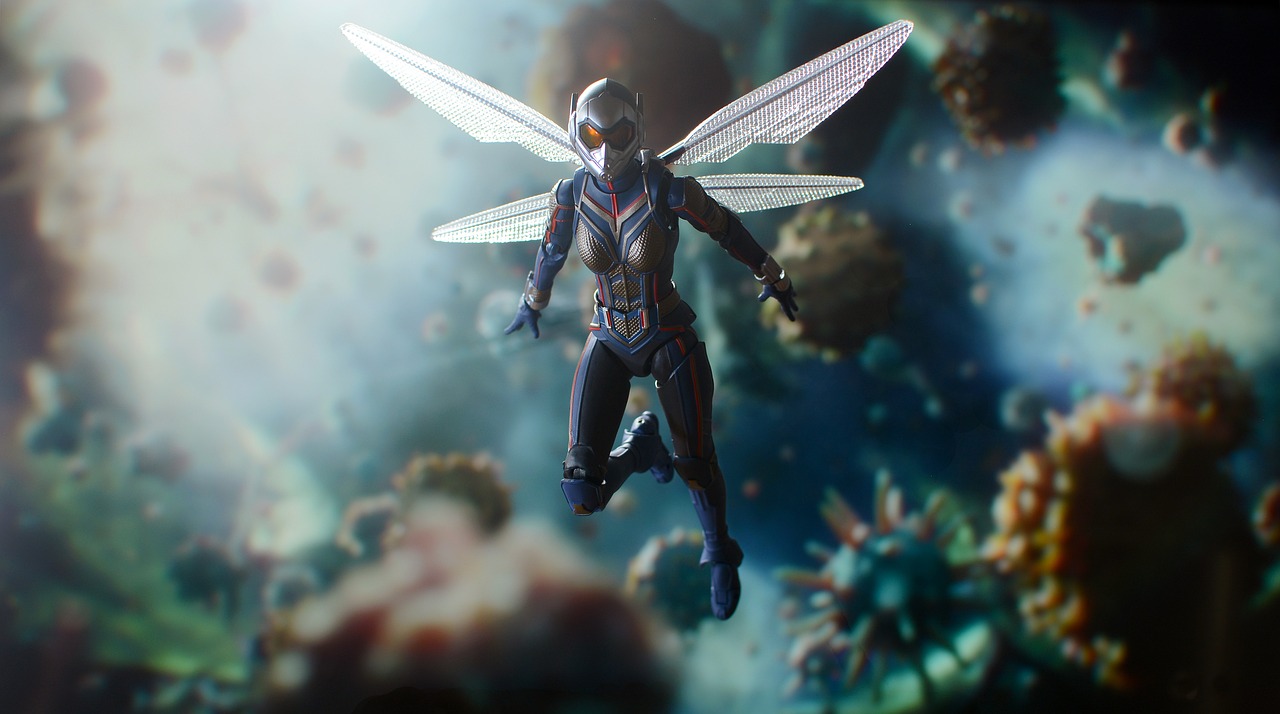
Challenges at the Intersection
The intersection of artificial intelligence (AI) and quantum physics is not without its hurdles. As these two groundbreaking fields converge, they bring with them a plethora of challenges that researchers and developers must navigate. One of the most significant barriers is the technical limitations inherent in both domains. Quantum computing, for instance, is still in its infancy, and many of the algorithms that drive AI need further refinement to work effectively with quantum systems. This is akin to trying to fit a square peg into a round hole; without the right adjustments, the synergy between AI and quantum physics may not achieve its full potential.
Another challenge lies in the realm of data scarcity. Quantum systems operate on principles that are often counterintuitive and complex, making it difficult to gather sufficient data for training AI models. The limited amount of reliable quantum data can lead to overfitting, where the AI learns too much from a small dataset and fails to generalize effectively to new scenarios. Imagine trying to teach a child about the world using only a handful of pictures; their understanding will be severely limited. In the same way, AI's ability to learn from quantum data is hampered by the lack of comprehensive datasets.
Moreover, the integration of AI with quantum physics necessitates a high degree of interdisciplinary collaboration. Professionals from fields as diverse as computer science, physics, and mathematics must work together to bridge the gap between these two areas. This can often lead to communication barriers, as experts may use jargon or concepts that are not easily understood by those outside their field. Without effective collaboration, the potential innovations at this intersection could remain unrealized. It's like trying to build a bridge between two islands without a common language; the connection will always be tenuous.
In addition to these challenges, there are also ethical considerations that must be addressed. As AI systems become more integrated with quantum computing, questions around data privacy and security will arise. How do we ensure that sensitive information is protected when utilizing advanced technologies? The potential for misuse or unintended consequences is a concern that cannot be overlooked. As we push the boundaries of what is possible, we must also remain vigilant about the implications of our innovations.
Ultimately, while the challenges at the intersection of AI and quantum physics are significant, they are not insurmountable. By fostering collaboration, addressing data limitations, and prioritizing ethical considerations, we can pave the way for groundbreaking advancements that could transform our understanding of both fields. The journey may be fraught with obstacles, but the potential rewards make it a pursuit worth undertaking.
- What are the main challenges of integrating AI with quantum computing?
The main challenges include technical limitations, data scarcity, the need for interdisciplinary collaboration, and ethical considerations related to data privacy and security.
- How does data scarcity affect AI in quantum physics?
Data scarcity can lead to overfitting, where AI models learn too much from a limited dataset and struggle to generalize to new situations.
- Why is interdisciplinary collaboration important?
Interdisciplinary collaboration is crucial because it brings together experts from various fields, helping to bridge knowledge gaps and foster innovation.
- What ethical concerns arise from AI and quantum physics integration?
Ethical concerns include data privacy and security issues, as well as the potential for misuse of advanced technologies.
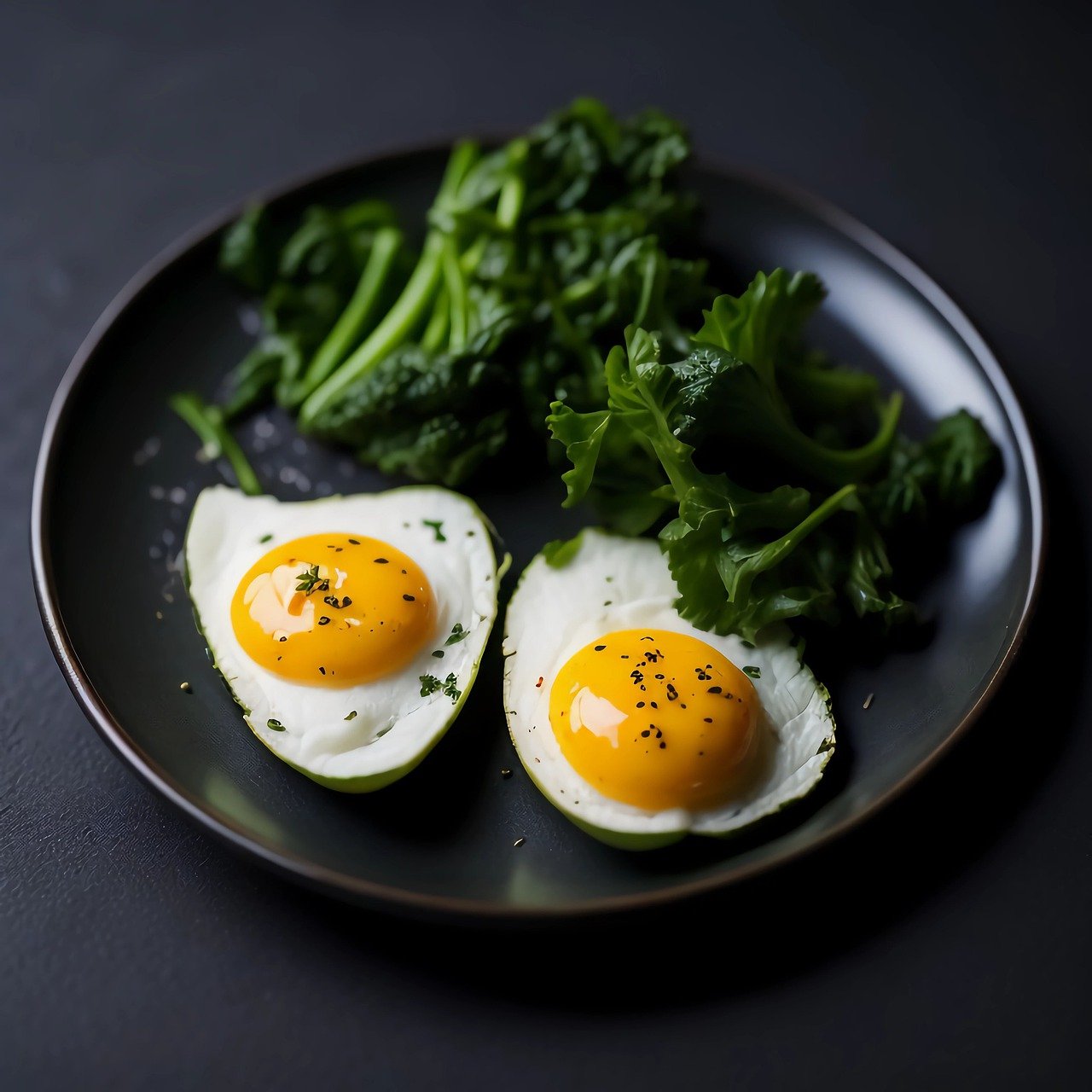
Potential Applications in Industry
The intersection of artificial intelligence (AI) and quantum physics is not just a theoretical playground; it has real-world implications that could revolutionize various industries. Imagine a world where complex problems are solved in seconds, where drug discoveries happen at lightning speed, and financial risks are assessed with unparalleled accuracy. This is not science fiction; this is the potential that AI and quantum physics hold when combined. Let’s dive into some of the most promising applications across different sectors.
In the realm of pharmaceuticals, the integration of AI and quantum computing can drastically change the landscape of drug discovery. Traditional methods can take years, if not decades, to bring a new drug to market. However, with the power of quantum computing, researchers can simulate molecular interactions at an unprecedented scale and speed. AI algorithms can analyze vast datasets to identify potential drug candidates, predict their efficacy, and even reduce side effects. This synergy not only accelerates the discovery process but also leads to more targeted and personalized medicine.
Another industry poised for transformation is finance. Financial institutions are constantly grappling with complex models that predict market trends and assess risks. By leveraging quantum computing, these institutions can process and analyze massive datasets far more efficiently than classical computers. AI can enhance these capabilities by optimizing algorithms that predict market fluctuations, ultimately leading to better investment strategies and risk management. For instance, a quantum algorithm could analyze thousands of variables in real-time, providing insights that were previously unimaginable.
To illustrate the potential applications in these industries, consider the following table:
Industry | Application | Benefits |
---|---|---|
Pharmaceuticals | Drug Discovery | Faster development, increased accuracy, personalized medicine |
Finance | Financial Modeling | Improved risk assessment, better investment strategies |
Logistics | Supply Chain Optimization | Enhanced efficiency, reduced costs |
Telecommunications | Network Optimization | Improved connectivity, reduced latency |
Moreover, industries like logistics and telecommunications are also beginning to harness this powerful duo. For logistics, quantum computing can optimize supply chains by analyzing numerous variables such as demand fluctuations, transportation routes, and inventory levels. AI can help in making real-time decisions, ensuring that goods are delivered in the most efficient manner possible. In telecommunications, quantum algorithms can improve network performance by optimizing data flow and reducing latency, which is crucial for maintaining high-quality service.
As we look to the future, the applications of AI and quantum physics are boundless. The potential for innovation is immense, and as these technologies continue to evolve, they will undoubtedly reshape industries and create new opportunities. The collaboration between AI and quantum physics is not just about improving existing processes; it’s about reimagining what’s possible and paving the way for breakthroughs that will define the next era of technological advancement.
- What is the significance of combining AI and quantum physics? The combination enhances computational capabilities, allowing for faster problem-solving and more accurate predictions across various industries.
- How will AI change drug discovery? AI can analyze large datasets to identify potential drug candidates and predict their effectiveness, significantly speeding up the discovery process.
- Can quantum computing improve financial risk assessment? Yes, quantum computing can process complex datasets more efficiently, leading to better risk management and investment strategies.
- What other industries can benefit from this intersection? Industries such as logistics and telecommunications are also exploring AI and quantum physics for optimization and efficiency improvements.
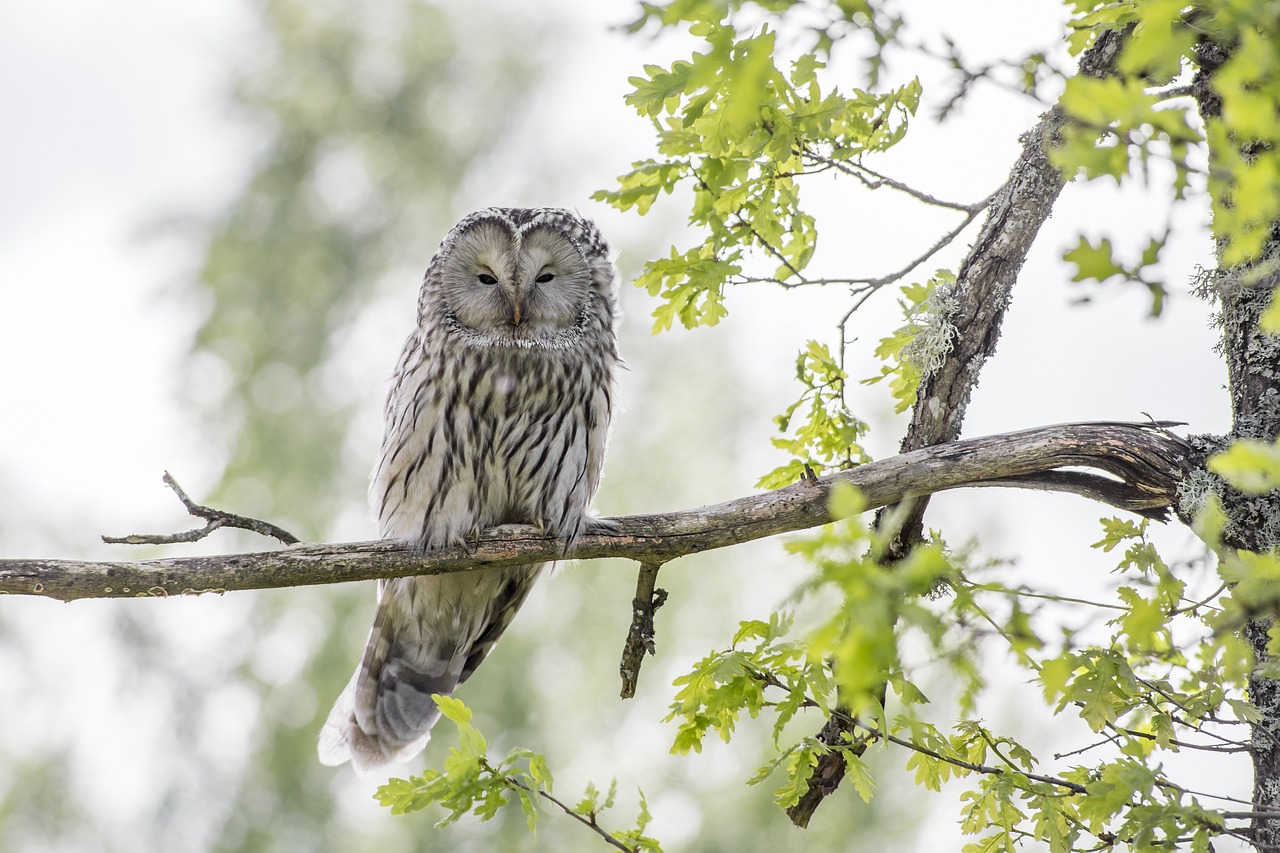
Advancements in Drug Discovery
In the ever-evolving landscape of pharmaceuticals, the integration of artificial intelligence (AI) and quantum computing is ushering in a new era of drug discovery. Traditionally, the process of discovering new drugs has been a tedious and time-consuming journey, often taking years, if not decades, before a viable candidate reaches the market. However, with the advent of AI and quantum technologies, researchers are now able to accelerate this process significantly, leading to faster and more accurate results.
One of the most remarkable advancements is the ability of AI to analyze vast datasets at lightning speed. This capability allows for the identification of potential drug candidates by predicting how different compounds will interact with biological targets. Imagine a researcher sifting through millions of chemical compounds—this would take an eternity without AI. By using machine learning algorithms, AI can quickly narrow down the candidates to a select few that show the most promise.
Moreover, quantum computing enhances this process by providing the computational power needed to simulate complex molecular interactions. While classical computers struggle with the intricacies of quantum phenomena, quantum computers can model these interactions more accurately, leading to better predictions about how a new drug might perform in the body. This synergy between AI and quantum computing is akin to having a supercharged engine that not only drives faster but also navigates through the most complex terrains effortlessly.
To illustrate the impact of these advancements, consider the following table that summarizes the traditional drug discovery process versus the AI and quantum-enhanced approach:
Aspect | Traditional Drug Discovery | AI & Quantum-Enhanced Drug Discovery |
---|---|---|
Timeframe | 10-15 years | 1-3 years |
Cost | $2.6 billion | $500 million |
Success Rate | 1 in 10,000 | 1 in 1,000 |
Data Analysis | Manual and time-consuming | Automated and rapid |
As we delve deeper into the specifics, AI algorithms can also help in the design of new molecules tailored to target specific diseases, effectively personalizing medicine. This not only enhances efficacy but also reduces side effects, making treatments safer for patients. Additionally, quantum simulations can provide insights into the stability and reactivity of these new compounds, thereby informing researchers about the likelihood of success in clinical trials.
Furthermore, the collaborative efforts between AI and quantum computing are paving the way for innovative approaches to tackling diseases that have long been deemed challenging, such as cancer and neurodegenerative disorders. By leveraging the predictive capabilities of AI alongside the computational prowess of quantum technology, researchers can explore uncharted territories in drug discovery, potentially leading to breakthroughs that were once thought to be impossible.
In conclusion, the advancements in drug discovery driven by the intersection of AI and quantum physics are not just incremental improvements; they represent a paradigm shift in how we approach the development of new therapies. As these technologies continue to evolve, we can expect to see a future where diseases are tackled with unprecedented speed and precision, ultimately improving health outcomes for countless individuals around the globe.
- How does AI improve drug discovery? AI enhances drug discovery by analyzing large datasets quickly, predicting interactions, and identifying promising drug candidates.
- What role does quantum computing play in drug discovery? Quantum computing provides the computational power necessary to simulate complex molecular interactions more accurately than classical computers.
- Can AI and quantum computing work together? Yes, their combination allows for faster and more efficient drug discovery processes, leading to innovative treatments.
- What diseases can benefit from these advancements? Diseases like cancer and neurodegenerative disorders are among those that could see significant benefits from AI and quantum-enhanced drug discovery.
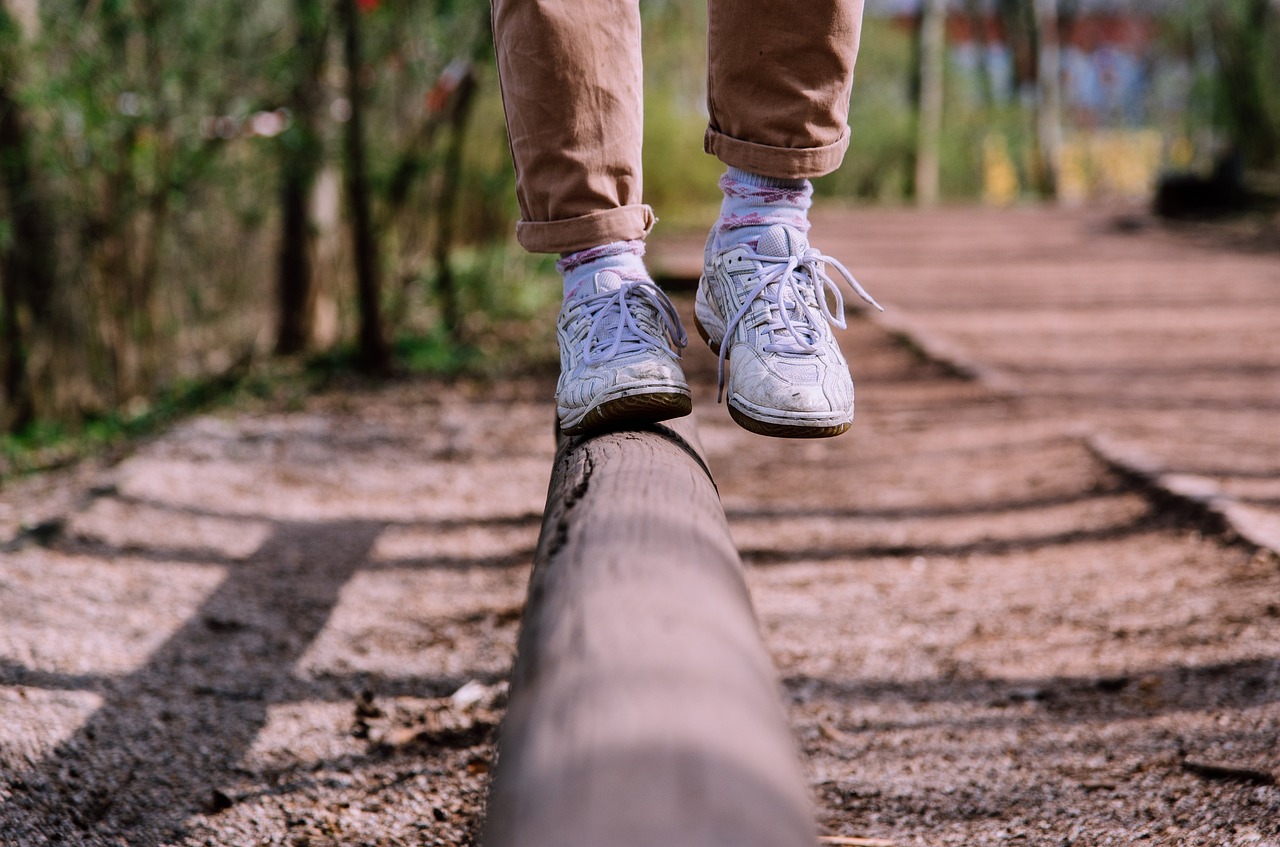
Financial Modeling and Risk Assessment
The financial landscape is constantly evolving, and with the advent of artificial intelligence (AI) and quantum physics, we are witnessing a transformative shift in how financial modeling and risk assessment are conducted. Imagine a world where complex financial scenarios can be simulated with unprecedented accuracy and speed. This is not just a dream; it’s becoming a reality as we merge these two groundbreaking fields. AI enhances our ability to process vast amounts of data, while quantum computing allows us to tackle problems that were previously deemed unsolvable.
At the core of this synergy is the ability to analyze risk more effectively. Traditional financial models often rely on historical data and linear assumptions, which can lead to significant errors in predictions. However, with the integration of AI and quantum computing, we can develop models that account for a multitude of variables and their interdependencies in real-time. This means that financial institutions can not only predict market trends more accurately but also assess risks with a level of granularity that was previously unimaginable.
For instance, consider the way AI algorithms can analyze market sentiment by scanning news articles, social media, and economic indicators. When combined with quantum computing's capacity to process this information at lightning speed, financial analysts can gain insights into market movements almost instantaneously. This dual approach allows for a more dynamic and responsive financial strategy, enabling firms to adjust their portfolios in real-time based on emerging data.
Moreover, one of the most exciting applications of this technology is in stress testing. Financial institutions are required to conduct stress tests to evaluate their resilience against economic shocks. With AI-powered quantum simulations, these tests can be conducted more thoroughly and efficiently. Financial institutions can simulate thousands of potential economic scenarios, incorporating various factors such as sudden market drops, changes in interest rates, and geopolitical events. The results can then guide decision-making, ensuring that firms are better prepared for potential crises.
Here’s a brief overview of how AI and quantum physics can enhance financial modeling and risk assessment:
Aspect | Traditional Approach | AI & Quantum Approach |
---|---|---|
Data Processing | Linear analysis of historical data | Real-time analysis of vast data sets |
Risk Prediction | Static models with limited variables | Dynamic models considering multiple scenarios |
Stress Testing | Manual simulations | Automated, complex simulations |
Decision Making | Slow response to market changes | Instantaneous adjustments based on data |
As we look towards the future, the potential applications of AI and quantum physics in finance are vast. From improving fraud detection systems to enhancing customer service through intelligent chatbots, the possibilities are endless. The integration of these technologies will not only streamline operations but also foster a culture of innovation within financial institutions.
In conclusion, the intersection of AI and quantum physics is revolutionizing financial modeling and risk assessment. It’s empowering financial professionals to make more informed decisions, ultimately leading to a more stable and resilient financial ecosystem. As these technologies continue to evolve, we can expect even greater advancements that will reshape the way we approach finance.
- What is the role of AI in financial modeling?
AI enhances data analysis, allowing for more accurate predictions and risk assessments by processing vast amounts of information quickly. - How does quantum computing improve risk assessment?
Quantum computing allows for complex simulations of various financial scenarios, enabling institutions to better prepare for potential economic shocks. - Can AI and quantum physics be used in other industries?
Yes, the integration of these technologies has applications across various sectors, including healthcare, logistics, and manufacturing.
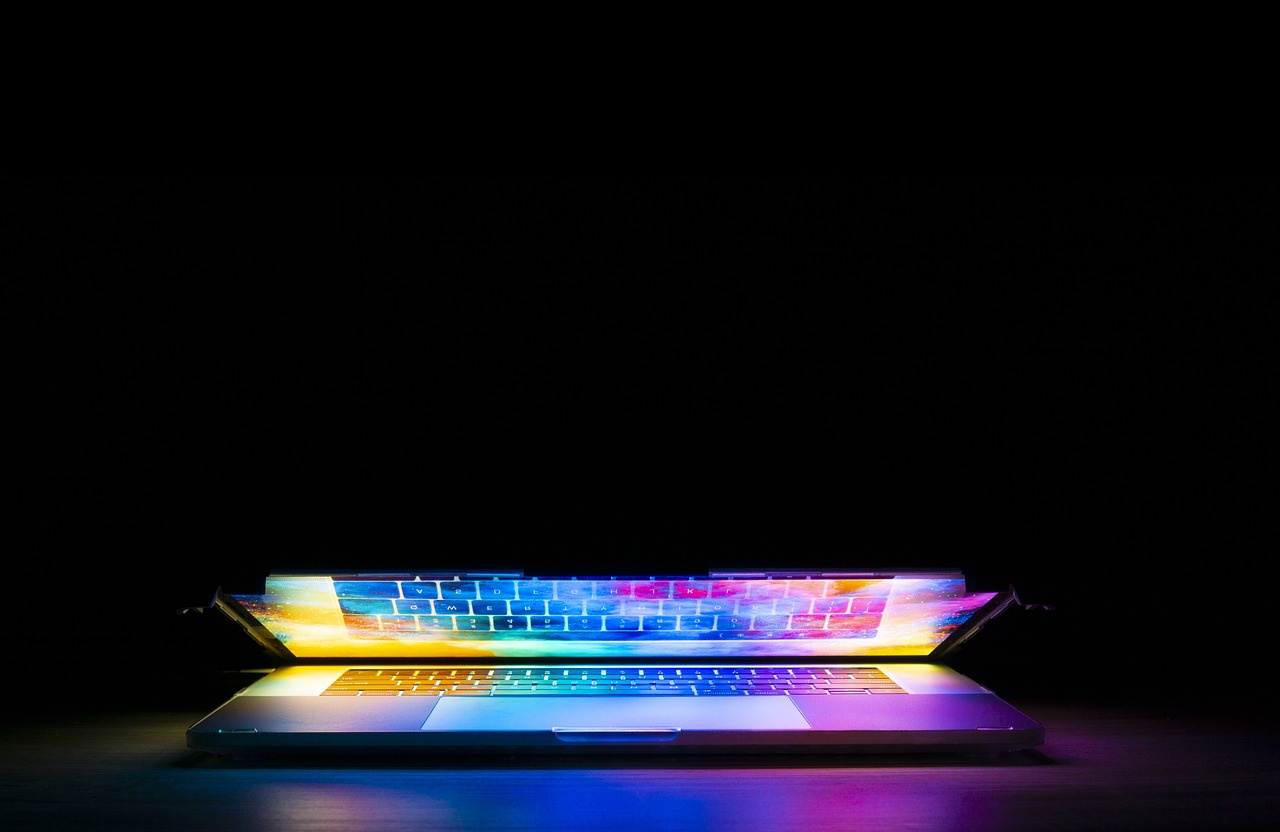
The Future of AI and Quantum Physics
The convergence of artificial intelligence and quantum physics paints a thrilling picture for the future of technology and science. Imagine a world where computers can solve problems that are currently deemed impossible, all thanks to the synergy of these two groundbreaking fields. As we stand on the brink of this technological revolution, the possibilities seem endless. Will we see AI algorithms that can predict quantum phenomena with unprecedented accuracy? Or perhaps quantum computers that can learn and adapt in ways we never thought possible? The future is indeed bright, but it also comes with its own set of challenges and considerations.
One of the most exciting prospects is the potential for quantum-enhanced AI. By harnessing the unique properties of quantum mechanics, such as superposition and entanglement, we could develop AI systems that are exponentially more powerful than their classical counterparts. This could lead to breakthroughs in various fields, from healthcare to climate modeling. For instance, imagine an AI that can analyze complex datasets related to climate change and provide solutions that are not only innovative but also viable. This is not just a dream; it's a possibility that could be realized in the near future.
However, with great power comes great responsibility. As we venture deeper into the integration of AI and quantum physics, ethical considerations must be at the forefront. How do we ensure that these powerful tools are used for the greater good? What safeguards can we put in place to prevent misuse? These questions are crucial as we navigate this uncharted territory.
Furthermore, the future will likely see a greater emphasis on interdisciplinary collaboration. The intersection of AI and quantum physics requires expertise from various fields, including computer science, physics, mathematics, and ethics. By fostering a collaborative environment, we can accelerate advancements and ensure that we address the multifaceted challenges that arise. For instance, universities and research institutions are already beginning to create programs that combine these disciplines, preparing the next generation of innovators to tackle the complex problems of tomorrow.
As we look ahead, it's essential to consider the potential applications of this fusion in real-world scenarios. Industries such as pharmaceuticals, finance, and energy are likely to be transformed by the capabilities that arise from AI and quantum computing. For example, in drug discovery, the combination of AI's predictive power and quantum computing's ability to model molecular interactions could lead to the rapid development of new therapies. In finance, quantum algorithms could revolutionize risk assessment and portfolio optimization, enabling more informed decision-making.
In summary, the future of AI and quantum physics is not just about technological advancements; it's about how we choose to harness these advancements for the benefit of society. As we embrace this exciting frontier, we must also remain vigilant, ensuring that our innovations serve to enhance human life and address the pressing challenges we face. The journey ahead is filled with possibilities, and it's up to us to navigate it wisely.
- What is the significance of AI in quantum computing?
AI enhances quantum computing by optimizing algorithms and improving error correction methods, which increases computational efficiency. - How can quantum neural networks differ from classical neural networks?
Quantum neural networks leverage quantum mechanics principles to process information, potentially offering greater computational power and efficiency. - What industries could benefit from the AI and quantum physics integration?
Industries such as pharmaceuticals, finance, and energy are poised to gain significantly from this integration, leading to advancements in drug discovery, financial modeling, and more.
Frequently Asked Questions
- What is the relationship between AI and quantum physics?
The relationship between AI and quantum physics is a fascinating one, where both fields intersect to enhance each other's capabilities. AI can optimize quantum algorithms and improve error correction methods, making quantum computing more efficient. In turn, quantum physics provides a rich framework for developing advanced AI models, especially in areas like quantum machine learning.
- How does AI improve quantum computing?
AI improves quantum computing by optimizing algorithms, which allows for faster and more accurate computations. Techniques like machine learning can help in error correction, making quantum systems more reliable. This synergy enables researchers to tackle complex problems that classical computers struggle with, pushing the boundaries of what technology can achieve.
- What are quantum neural networks?
Quantum neural networks are a novel approach that combines the principles of quantum mechanics with artificial neural networks. Unlike classical neural networks, quantum neural networks leverage quantum bits (qubits) to process information in ways that classical systems can't. This unique architecture allows them to solve problems more efficiently, especially in fields like optimization and data analysis.
- Can AI help in drug discovery?
Absolutely! The combination of AI and quantum computing can revolutionize drug discovery by speeding up the process of identifying and validating potential drug candidates. AI algorithms can analyze vast datasets to predict how different compounds interact, while quantum computing can model complex molecular interactions more accurately than traditional methods.
- What challenges exist at the intersection of AI and quantum physics?
Several challenges exist, including technical limitations in current quantum hardware, the scarcity of data for training AI models, and the need for interdisciplinary collaboration among experts in both fields. Addressing these challenges is crucial for unlocking the full potential of AI and quantum physics together.
- What industries can benefit from AI and quantum physics integration?
Industries such as pharmaceuticals, finance, materials science, and logistics stand to gain immensely from the integration of AI and quantum physics. For instance, in finance, improved risk assessment and financial modeling can lead to better investment strategies, while in pharmaceuticals, faster drug discovery can save time and resources.
- What does the future hold for AI and quantum physics?
The future of AI and quantum physics is filled with exciting possibilities. As both fields continue to evolve, we can expect breakthroughs that will transform technology and research landscapes. Innovations in quantum algorithms, enhanced AI models, and new applications across various industries are just a few of the developments on the horizon.