The Role of AI in Predictive Analysis
In today's fast-paced world, the ability to predict future trends and behaviors is more crucial than ever. Artificial Intelligence (AI) has emerged as a game-changer in the realm of predictive analysis, enabling organizations to make informed decisions based on data-driven insights. Imagine having a crystal ball that not only forecasts outcomes but also enhances the accuracy of those predictions. That's the power of AI! By leveraging advanced algorithms and vast amounts of data, AI transforms the way we interpret information, making it an invaluable tool across various sectors.
At its core, predictive analysis is about using historical data to anticipate future events. Think of it as a detective piecing together clues to solve a mystery. AI enhances this process by automating complex calculations and uncovering hidden patterns that humans might overlook. This not only speeds up the analysis but also improves its accuracy, leading to better decision-making. For instance, in the healthcare sector, AI can predict patient outcomes based on previous cases, while in finance, it can forecast market trends by analyzing consumer behavior. The implications are vast and exciting!
One of the most fascinating aspects of AI in predictive analysis is its ability to continuously learn and adapt. As more data becomes available, AI systems refine their algorithms, leading to increasingly accurate predictions over time. This is akin to a chef perfecting a recipe; the more they cook, the better they understand the ingredients and techniques needed to create a masterpiece. By harnessing machine learning and natural language processing, AI is not just a tool but a partner in innovation, driving organizations toward success.
However, it's important to recognize that while AI offers tremendous advantages, it also comes with its own set of challenges. Issues such as data quality and ethical considerations must be addressed to fully realize its potential. After all, a powerful tool is only as good as the data it processes. As we delve deeper into the world of AI-driven predictive analysis, we will explore its transformative capabilities, real-world applications, and the hurdles that need to be overcome.
So, what does the future hold for AI in predictive analysis? As technology continues to evolve, we can expect even more sophisticated algorithms and tools that will revolutionize how we approach data interpretation. The integration of AI into predictive analysis is not just a trend; it's a fundamental shift in how we understand and leverage data for decision-making. Buckle up, because the journey into the world of AI-enhanced predictive analysis is just beginning!
- What is predictive analysis?
Predictive analysis involves using statistical techniques and algorithms to forecast future outcomes based on historical data. - How does AI improve predictive analysis?
AI enhances predictive analysis by automating processes, uncovering hidden patterns, and continuously learning from new data. - What industries benefit from AI in predictive analysis?
Industries such as healthcare, finance, marketing, and retail utilize AI-driven predictive analysis to improve decision-making and operational efficiency. - What are the challenges of using AI in predictive analysis?
Challenges include data quality issues and ethical concerns, such as privacy and bias in algorithmic decision-making.
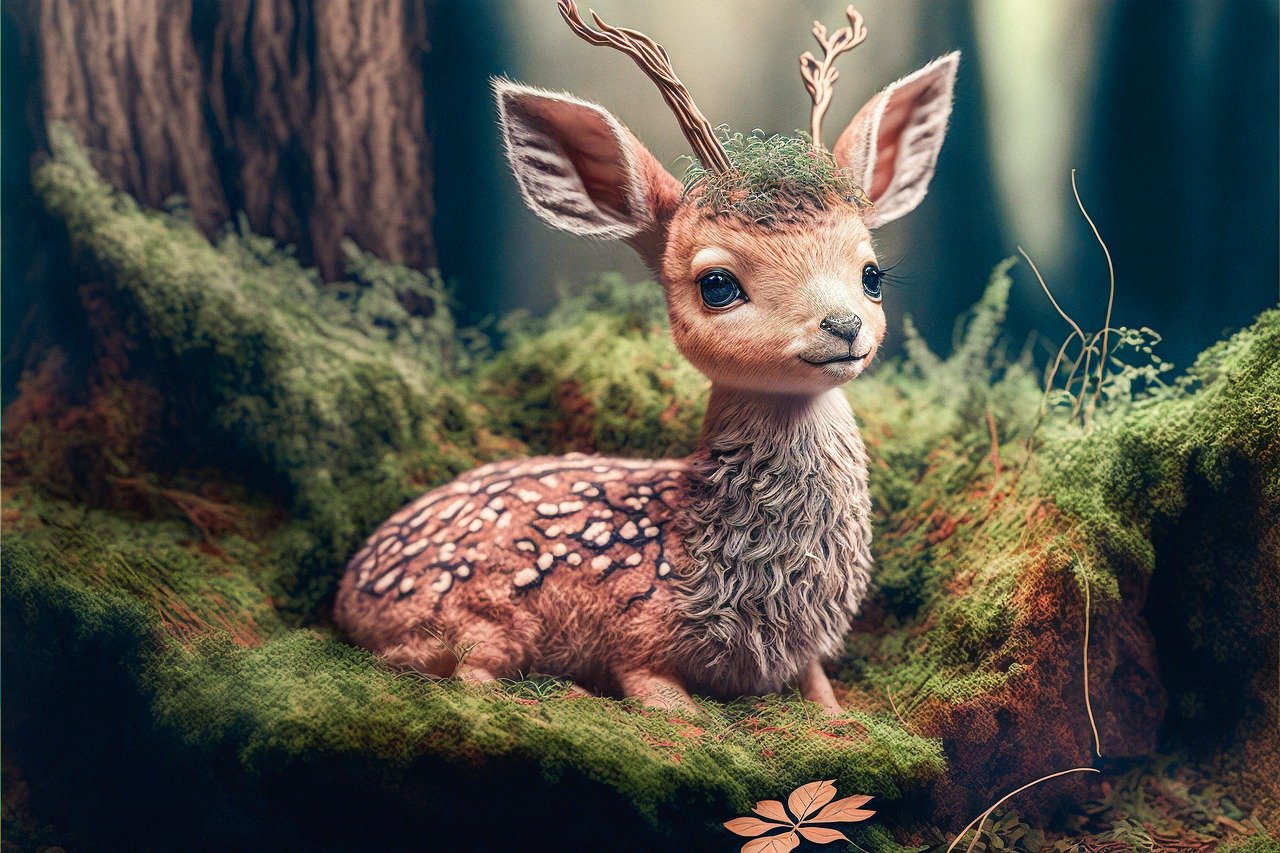
Understanding Predictive Analysis
Predictive analysis is a fascinating field that combines statistics, data mining, and machine learning to forecast future outcomes based on historical data. Imagine trying to predict the weather; you gather data from previous weather patterns, analyze it, and then use that information to make informed guesses about what might happen next. This is essentially what predictive analysis does, but on a much grander scale and across various industries.
The importance of predictive analysis cannot be overstated. In today's data-driven world, organizations are inundated with vast amounts of information. Predictive analysis acts like a compass, helping businesses navigate through this sea of data to make better decisions. By identifying trends and patterns, companies can anticipate customer behaviors, optimize operations, and ultimately drive profitability. For instance, a retail company can predict which products will be in demand based on past sales data, allowing them to stock efficiently and reduce waste.
Applications of predictive analysis are diverse and span across multiple sectors. Here are a few examples:
- Healthcare: Predictive analysis can identify potential health risks in patients, allowing for proactive interventions.
- Finance: Financial institutions use predictive models to assess credit risk and detect fraudulent activities.
- Marketing: Companies leverage predictive analytics to tailor their marketing strategies, ensuring they reach the right audience with the right message.
The process of predictive analysis typically involves several stages, including data collection, data cleaning, and model building. Data collection can come from various sources, such as customer interactions, sales transactions, and even social media. However, raw data is often messy and unstructured, which is why data cleaning is critical. This step ensures that the data is accurate and relevant, laying a solid foundation for the predictive models.
Once the data is prepared, analysts employ various statistical techniques and algorithms to build models that can predict future outcomes. These models are then validated and tested to ensure their accuracy. The end goal is to create a reliable tool that organizations can use to make informed decisions. The beauty of predictive analysis lies in its ability to transform raw data into actionable insights, paving the way for smarter strategies and improved outcomes.
In conclusion, understanding predictive analysis is essential for anyone looking to harness the power of data in today's fast-paced environment. It unlocks the potential to foresee trends, anticipate challenges, and seize opportunities, making it an invaluable tool across industries.
- What is predictive analysis? Predictive analysis is the practice of using data, statistical algorithms, and machine learning techniques to identify the likelihood of future outcomes based on historical data.
- How does predictive analysis work? It involves collecting and cleaning data, building predictive models, and validating these models to forecast future trends.
- What industries benefit from predictive analysis? Industries such as healthcare, finance, marketing, and retail utilize predictive analysis to enhance decision-making and operational efficiency.
- What are the challenges associated with predictive analysis? Common challenges include data quality issues and ethical concerns related to privacy and bias in AI models.

How AI Transforms Data Interpretation
When it comes to making sense of the massive amounts of data generated every day, traditional methods can feel like trying to find a needle in a haystack. This is where artificial intelligence (AI) steps in, revolutionizing the way we interpret data. By automating complex processes and uncovering hidden patterns, AI not only enhances the accuracy of predictions but also significantly boosts the efficiency of data analysis.
Imagine a detective sifting through countless pieces of evidence to solve a case. Without the right tools, it can take ages to piece everything together. Similarly, AI acts as a powerful magnifying glass, allowing analysts to zoom in on critical data points that might otherwise go unnoticed. This transformative capability is primarily driven by advanced algorithms that learn from historical data, making it possible to spot trends and anomalies quickly.
One of the most significant advantages of AI in data interpretation is its ability to process data at an unprecedented scale. Traditional methods often struggle with large datasets, but AI can analyze terabytes of information in mere seconds. This speed not only accelerates decision-making processes but also provides businesses with a competitive edge. For instance, companies can respond to market changes in real-time, adjusting their strategies based on the latest insights.
Moreover, AI enhances data interpretation through machine learning, a subset of AI that allows systems to learn from data without being explicitly programmed. Machine learning algorithms adapt and improve over time, making them incredibly effective at identifying patterns in complex datasets. Here’s a quick breakdown of how machine learning contributes to predictive analysis:
Machine Learning Technique | Description | Application in Predictive Analysis |
---|---|---|
Supervised Learning | Trains models on labeled datasets | Used for forecasting sales or predicting customer behavior |
Unsupervised Learning | Identifies patterns without labeled data | Useful for market segmentation and anomaly detection |
Additionally, AI's ability to leverage Natural Language Processing (NLP) allows it to interpret unstructured data, such as customer reviews or social media posts. This capability is crucial for businesses aiming to understand customer sentiment and preferences. By analyzing textual data, AI can provide insights that help companies tailor their products and marketing strategies to better meet consumer needs.
In summary, AI is not just a tool; it’s a game-changer in the realm of data interpretation. By automating complex processes, identifying hidden patterns, and processing vast amounts of information, AI empowers organizations to make informed decisions faster and more accurately. As we continue to embrace AI technologies, we can expect even greater advancements in how we interpret and utilize data in predictive analysis.
- How does AI improve data accuracy? AI algorithms analyze data patterns more effectively than traditional methods, leading to more accurate predictions.
- What industries benefit most from AI in data interpretation? Industries such as healthcare, finance, and marketing are seeing significant improvements in decision-making processes due to AI.
- Can AI replace human analysts? While AI significantly enhances data interpretation, human analysts are still crucial for contextual understanding and ethical considerations.

Machine Learning Techniques
Machine learning is a fascinating subset of artificial intelligence that empowers systems to learn from data and improve their performance over time without being explicitly programmed. When it comes to predictive analysis, machine learning techniques play a pivotal role in transforming raw data into actionable insights. These techniques can be broadly categorized into two types: supervised learning and unsupervised learning. Each of these approaches has its unique strengths and applications, making them indispensable tools in the predictive analytics toolkit.
Let's first dive into supervised learning. This technique involves training a model on a labeled dataset, meaning that the data used for training includes both the input features and the corresponding output labels. For instance, if we were predicting house prices, the input features could include the size of the house, the number of bedrooms, and the location, while the output label would be the actual price. Supervised learning algorithms, such as linear regression, decision trees, and support vector machines, learn to map input data to the correct output by minimizing the error between predicted and actual values. This method is particularly effective in scenarios where historical data is available, allowing organizations to make reliable predictions.
On the other hand, we have unsupervised learning, which is all about discovering hidden patterns in data without any labeled outcomes. Imagine you have a collection of customer data but no specific insights about their purchasing behavior. Unsupervised learning techniques, such as clustering and dimensionality reduction, can help identify groups of similar customers or reduce the complexity of the data while retaining its essential characteristics. This approach is invaluable for businesses looking to uncover trends, anomalies, or segments within their data that they might not have previously considered. For example, clustering algorithms can segment customers based on their buying habits, enabling targeted marketing strategies.
Moreover, there are also hybrid approaches that combine both supervised and unsupervised learning to leverage the strengths of each. For instance, a common technique is to use unsupervised learning to preprocess the data and discover patterns, followed by supervised learning to make predictions based on those patterns. This synergy can lead to more accurate and robust predictive models.
In summary, the power of machine learning techniques in predictive analysis lies in their ability to adapt and learn from data, whether through supervised or unsupervised methods. By harnessing these techniques, organizations can significantly enhance their decision-making processes, improve operational efficiency, and ultimately achieve better outcomes.
- What is the difference between supervised and unsupervised learning?
Supervised learning uses labeled data to train models, while unsupervised learning finds patterns in data without labels. - Can machine learning improve predictive accuracy?
Yes, machine learning techniques can enhance predictive accuracy by learning from historical data and adapting to new information. - What industries benefit from machine learning in predictive analysis?
Industries such as healthcare, finance, marketing, and retail utilize machine learning for predictive analysis to improve decision-making.

Supervised Learning
Supervised learning is a cornerstone of predictive analysis, acting like a seasoned coach guiding athletes to reach their peak performance. In this approach, models are trained on labeled datasets, which means that each input data point is paired with the correct output. Think of it as teaching a child to recognize animals: you show them pictures of cats and dogs, telling them which is which, until they can independently identify them. This process helps machines learn from past experiences, enabling them to make accurate predictions about new, unseen data.
One of the key benefits of supervised learning in predictive analysis is its ability to enhance accuracy and reliability. With a well-labeled dataset, algorithms can learn the underlying patterns and relationships between input features and the target outcome. For instance, in a healthcare setting, supervised learning can be applied to predict patient diagnoses based on historical medical records. By analyzing data such as symptoms, lab results, and previous diagnoses, the model can identify which factors are most predictive of specific conditions.
Moreover, supervised learning encompasses various algorithms, each with its unique strengths. Some of the most commonly used algorithms include:
- Linear Regression: Ideal for predicting continuous outcomes, like sales figures or temperatures.
- Logistic Regression: Perfect for binary classification tasks, such as determining whether a customer will churn or not.
- Decision Trees: Great for visualizing decisions and outcomes, making them easy to interpret.
- Random Forests: An ensemble method that builds multiple decision trees for improved accuracy.
Each of these algorithms has its own use cases and can be selected based on the specific needs of the analysis. For example, if you're working with a large dataset with many features, a random forest might be your best bet due to its ability to handle complexity and reduce overfitting.
However, supervised learning isn't without its challenges. The quality of the labeled data is paramount; if the data is biased or inaccurate, the model's predictions will likely be flawed. Thus, ensuring high-quality data collection and labeling processes is crucial for the success of any supervised learning initiative.
In summary, supervised learning is a powerful tool in predictive analysis that leverages labeled data to train algorithms for accurate predictions. By understanding the relationships within the data and applying the right algorithms, businesses and organizations can make informed decisions that lead to better outcomes.

Unsupervised Learning
Unsupervised learning is a fascinating branch of machine learning that stands out for its ability to identify patterns and structures within data without the need for labeled outcomes. Imagine diving into a vast ocean of information, where the data points are like fish swimming freely. Instead of having a guide to tell you which fish belong to which species (like in supervised learning), you are left to explore and categorize these fish based on their characteristics. This approach allows for a more organic discovery of insights, making it incredibly valuable in predictive analysis.
The core idea behind unsupervised learning is to enable machines to learn from data without explicit instructions. This method is particularly useful in scenarios where we lack predefined labels or outcomes. For instance, businesses can utilize unsupervised learning to segment customers based on purchasing behavior, leading to more targeted marketing strategies. By analyzing patterns in customer data, companies can discover distinct groups and tailor their offerings to meet the specific needs of each segment.
One of the key techniques used in unsupervised learning is clustering. Clustering algorithms group similar data points together, allowing analysts to identify natural clusters within the data. For example, a retail company might use clustering to identify different customer segments based on their shopping habits. The table below illustrates some common clustering algorithms and their applications:
Algorithm | Description | Applications |
---|---|---|
K-Means | Partitions data into K distinct clusters based on distance from the centroid. | Customer segmentation, market research |
Hierarchical Clustering | Builds a tree of clusters by either merging or splitting them. | Social network analysis, gene analysis |
DBSCAN | Groups together points that are closely packed while marking outliers as noise. | Geospatial data analysis, anomaly detection |
Another important aspect of unsupervised learning is dimensionality reduction. This technique simplifies complex datasets by reducing the number of variables under consideration. By doing so, it helps in visualizing high-dimensional data and can enhance the performance of other machine learning algorithms. For instance, Principal Component Analysis (PCA) is a popular method used to reduce dimensions while retaining the most significant features of the data.
Ultimately, unsupervised learning plays a crucial role in predictive analysis by enabling organizations to uncover hidden insights and trends. It empowers businesses to make data-driven decisions based on the patterns they discover, leading to improved strategies and outcomes. As we continue to harness the power of AI and machine learning, the potential of unsupervised learning will only grow, providing even deeper insights into complex datasets.
- What is the main difference between supervised and unsupervised learning?
Supervised learning uses labeled data to train models, while unsupervised learning identifies patterns in unlabeled data. - Can unsupervised learning be used for predictive analysis?
Yes, unsupervised learning can help identify trends and groupings that inform predictive models. - What are some common applications of unsupervised learning?
Applications include customer segmentation, anomaly detection, and market basket analysis.

Natural Language Processing
Natural Language Processing (NLP) is a fascinating branch of artificial intelligence that bridges the gap between human communication and computer understanding. Imagine trying to explain a complex idea to a friend who speaks a different language; that’s somewhat akin to what NLP aims to achieve with machines. By enabling computers to comprehend, interpret, and generate human language, NLP opens up a world of possibilities for predictive analysis. It allows businesses and organizations to analyze vast amounts of text data, extracting valuable insights that can inform decision-making and strategy.
One of the most prominent applications of NLP in predictive analysis is sentiment analysis. This technique involves evaluating text—such as social media posts, customer reviews, or survey responses—to determine the emotional tone behind the words. For instance, a company can analyze customer feedback to gauge public sentiment about a new product launch. By employing NLP techniques, businesses can swiftly categorize feedback as positive, negative, or neutral, enabling them to respond proactively to customer concerns or capitalize on positive sentiment.
Moreover, NLP enhances customer insights by analyzing communication patterns and preferences. For example, chatbots powered by NLP can interact with customers in real-time, understanding their queries and providing relevant responses. This not only improves customer satisfaction but also generates data that can be analyzed to predict future customer behavior. As these systems learn from interactions, they become increasingly adept at identifying trends and making predictions based on historical data.
However, it's essential to recognize that NLP is not without its challenges. The complexity of human language—filled with nuances, idioms, and cultural references—can make it difficult for machines to interpret text accurately. Additionally, the vast diversity of languages and dialects adds another layer of complexity. To address these challenges, researchers are continually developing advanced algorithms and models that can better understand context and meaning in human language.
In conclusion, the role of NLP in predictive analysis is transformative. By leveraging the power of language, organizations can uncover insights that were previously hidden in unstructured data. As technology advances, the potential for NLP to enhance predictive analytics will only grow, leading to more informed decision-making and improved outcomes across various sectors.
- What is Natural Language Processing?
NLP is a field of artificial intelligence that focuses on the interaction between computers and humans through natural language. It enables machines to understand, interpret, and generate human language.
- How does NLP benefit predictive analysis?
NLP allows for the analysis of large volumes of text data to extract insights, enabling organizations to understand customer sentiment and behavior patterns, which can inform strategic decisions.
- What are some challenges associated with NLP?
Challenges include the complexity of human language, the need for context understanding, and the diversity of languages and dialects, which can hinder accurate interpretation.

Real-World Applications of AI in Predictive Analysis
The impact of artificial intelligence on predictive analysis is nothing short of revolutionary. Across various sectors, AI-driven predictive analysis is transforming the way organizations make decisions and strategize for the future. Imagine having the ability to foresee market trends, patient needs, or even customer preferences before they manifest. This is not science fiction; it’s the reality that AI brings to the table. From healthcare to finance and marketing, let’s dive into some compelling examples that illustrate how AI is reshaping predictive analysis in real-world applications.
In the realm of healthcare, AI-powered predictive analysis tools are being utilized to enhance patient outcomes significantly. For instance, predictive algorithms analyze historical patient data to forecast potential health issues, allowing healthcare providers to intervene early. This proactive approach not only improves patient care but also optimizes resource allocation within hospitals. A case in point is the use of AI in predicting hospital readmission rates. By analyzing patterns in patient records, healthcare professionals can identify those at high risk and implement tailored discharge plans, effectively reducing readmissions.
Similarly, in the finance sector, AI plays a crucial role in enhancing financial forecasting and risk assessment. Financial institutions leverage predictive analytics to analyze market trends and consumer behavior, which informs investment strategies and risk management practices. For example, banks use AI to assess the creditworthiness of loan applicants by evaluating their financial history and predicting future behavior. This not only speeds up the approval process but also minimizes the risk of defaults. Moreover, AI algorithms can analyze vast amounts of market data in real-time, allowing traders to make informed decisions based on predictive insights.
In marketing, businesses are harnessing the power of AI to predict customer behavior and preferences. By analyzing past purchasing data, social media interactions, and online browsing habits, companies can forecast which products will resonate with their target audience. This predictive capability enables marketers to tailor their campaigns more effectively, ensuring a higher return on investment. For instance, e-commerce platforms utilize AI to recommend products to users based on their previous purchases, thereby enhancing the shopping experience and driving sales.
To summarize, the applications of AI in predictive analysis are vast and varied. Below is a brief overview of how AI is making waves in different sectors:
Sector | Application | Benefits |
---|---|---|
Healthcare | Predicting patient outcomes and readmissions | Improved patient care, optimized resource allocation |
Finance | Risk assessment and market trend analysis | Faster decision-making, reduced risk of defaults |
Marketing | Customer behavior prediction and targeted campaigns | Enhanced ROI, improved customer satisfaction |
As we can see, AI is not just a buzzword; it’s a game-changer that is redefining predictive analysis across multiple industries. By leveraging data-driven insights, organizations can make more informed decisions, ultimately leading to better outcomes and increased efficiency.
- What is predictive analysis? Predictive analysis involves using statistical techniques and algorithms to identify future outcomes based on historical data.
- How does AI enhance predictive analysis? AI automates complex data interpretation processes, uncovering hidden patterns and improving accuracy and efficiency.
- What are some real-world applications of AI in predictive analysis? AI is used in healthcare for patient outcomes, in finance for risk assessment, and in marketing for predicting customer behavior.
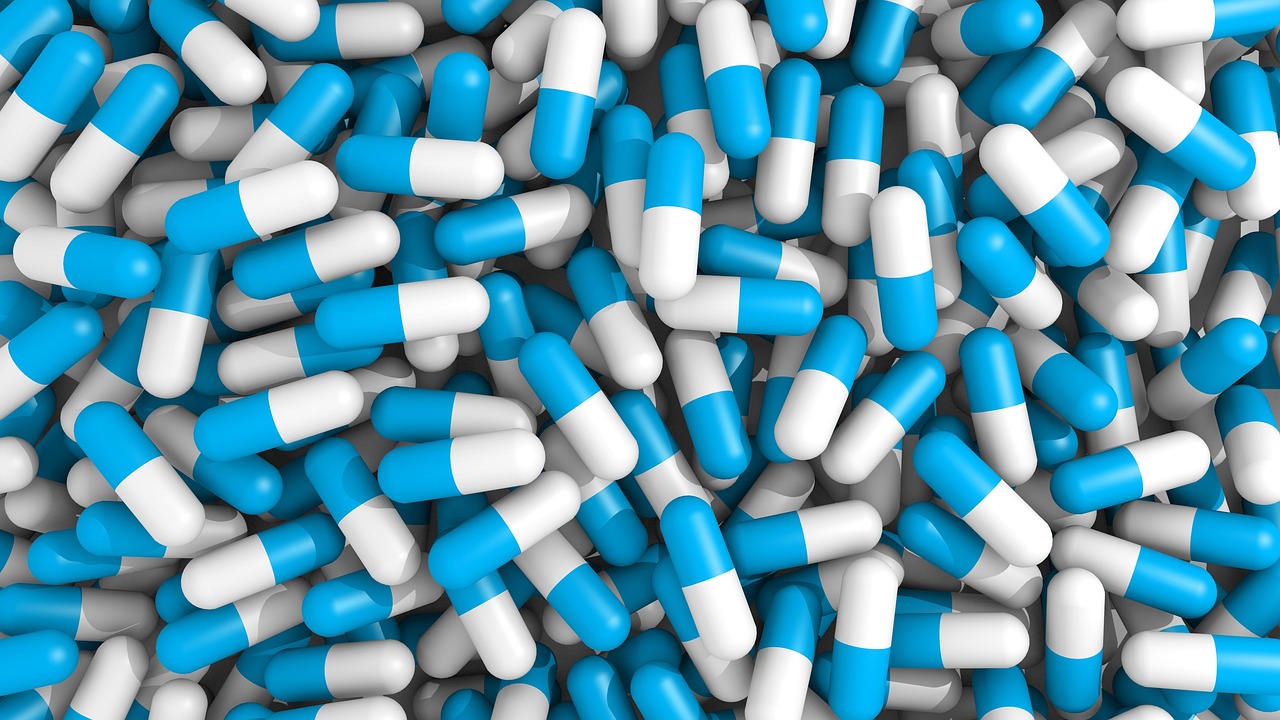
Healthcare Innovations
In the realm of healthcare, the integration of artificial intelligence into predictive analysis is nothing short of revolutionary. Imagine a world where doctors can anticipate health issues before they arise, allowing for proactive rather than reactive treatment. This is not just a dream; it is becoming a reality thanks to AI-powered predictive analytics. By leveraging vast amounts of patient data, AI systems can identify patterns and trends that human analysts might overlook. For instance, algorithms can analyze historical patient records to predict the likelihood of diseases such as diabetes or heart conditions, enabling healthcare providers to tailor preventative measures for at-risk individuals.
One of the most significant innovations in healthcare predictive analysis is the use of machine learning algorithms to enhance diagnostic accuracy. These algorithms can sift through thousands of medical images, such as X-rays and MRIs, to detect anomalies that may indicate the early stages of cancer. In a recent study, AI systems demonstrated an accuracy rate that surpassed human radiologists, showcasing the potential for machines to assist in diagnostics and improve patient outcomes.
Furthermore, AI-driven predictive analysis can optimize treatment plans by analyzing patient responses to previous treatments. By understanding which therapies have been most effective for similar patients, healthcare providers can make informed decisions that enhance recovery rates. This data-driven approach not only improves individual patient care but also contributes to the overall efficiency of healthcare systems.
To illustrate the impact of AI in healthcare, consider the following table that highlights some of the key applications and their benefits:
Application | Description | Benefits |
---|---|---|
Predictive Diagnostics | Using historical data to predict disease risk. | Early intervention and personalized care. |
Treatment Optimization | Analyzing treatment efficacy based on patient data. | Improved recovery rates and reduced costs. |
Operational Efficiency | Streamlining hospital operations through predictive modeling. | Reduced wait times and better resource allocation. |
The potential of AI in healthcare is vast, but it also raises important questions about data privacy and security. As healthcare providers collect and analyze sensitive patient information, they must ensure that robust measures are in place to protect this data from breaches. Moreover, the ethical implications of relying on AI for critical healthcare decisions cannot be overlooked. Ensuring that these systems are transparent and free from bias is paramount to maintaining trust in AI-driven healthcare solutions.
In conclusion, the innovations brought about by AI in predictive analysis are transforming the healthcare landscape. By improving diagnostic accuracy, optimizing treatment plans, and enhancing operational efficiency, AI is paving the way for a future where healthcare is more personalized, proactive, and effective. As we continue to embrace these technologies, it is essential to address the accompanying challenges to fully realize the benefits of AI in healthcare.
- What is predictive analysis in healthcare? Predictive analysis in healthcare involves using data and algorithms to forecast potential health outcomes, enabling proactive patient care.
- How does AI improve patient outcomes? AI enhances patient outcomes by providing accurate predictions for disease risk, optimizing treatment plans, and streamlining operations.
- Are there ethical concerns with AI in healthcare? Yes, ethical concerns include data privacy, security, and potential biases in AI algorithms that could affect patient care.
- Can AI replace doctors? While AI can assist in diagnostics and treatment planning, it is not intended to replace doctors but to enhance their capabilities.

Financial Forecasting
In the fast-paced world of finance, predictive analysis powered by artificial intelligence has become a game changer. It enhances financial forecasting by analyzing vast amounts of data to identify market trends and consumer behaviors that would be impossible for humans to discern alone. Imagine having a crystal ball that not only predicts future market movements but also helps financial institutions make informed decisions. This is precisely what AI brings to the table.
One of the key advantages of AI in financial forecasting is its ability to process and analyze data at lightning speed. Traditional forecasting methods often rely on historical data and basic statistical models, which can be limiting. In contrast, AI utilizes advanced algorithms and machine learning techniques to consider a multitude of factors, including economic indicators, social media sentiment, and even geopolitical events. This comprehensive approach leads to more accurate predictions and better risk management.
For instance, AI can analyze consumer spending patterns by sifting through transaction data, social media interactions, and online behavior. By doing so, it can predict shifts in demand for products or services. Financial institutions can leverage these insights to tailor their investment strategies, optimize their portfolios, and mitigate risks. The result? Enhanced profitability and a competitive edge in the market.
Moreover, AI-driven forecasting models can adapt and learn over time. They can refine their predictions based on new data inputs, making them increasingly precise. This adaptability is crucial in a volatile market environment where changes can occur rapidly. For example, during economic downturns or unexpected global events, AI can quickly recalibrate its models to reflect the new reality, ensuring that financial institutions are not caught off guard.
However, while AI offers powerful tools for financial forecasting, it is essential to acknowledge the challenges that come with it. Data quality is paramount; if the data fed into these models is flawed or biased, the predictions will be too. Additionally, ethical considerations around data privacy and algorithmic bias must be addressed to ensure that AI is used responsibly in financial forecasting.
To illustrate the impact of AI in financial forecasting, consider the following table that highlights some notable applications:
Application | Description | Impact |
---|---|---|
Risk Assessment | AI models analyze historical data to evaluate potential risks in investments. | Improved risk management strategies and reduced losses. |
Market Trend Analysis | AI analyzes various data sources to predict market shifts. | More accurate forecasting leads to better investment decisions. |
Customer Insights | AI examines consumer behavior and preferences. | Enhanced product offerings and targeted marketing strategies. |
In conclusion, AI is revolutionizing financial forecasting by providing deeper insights and more accurate predictions. As financial institutions continue to embrace this technology, they will not only enhance their decision-making processes but also gain a significant advantage in an increasingly competitive landscape.
- What is financial forecasting? Financial forecasting is the process of estimating future financial outcomes based on historical data and analysis.
- How does AI improve financial forecasting? AI enhances forecasting accuracy by analyzing large datasets and identifying patterns that traditional methods might miss.
- What challenges does AI face in financial forecasting? Key challenges include data quality, ethical considerations, and the need for transparency in AI algorithms.
- Can AI predict economic downturns? While AI can identify trends that may indicate a downturn, predictions are never guaranteed due to the unpredictable nature of markets.

Challenges and Limitations
While the integration of AI in predictive analysis has revolutionized how we interpret data and make decisions, it is not without its challenges and limitations. One of the most pressing issues is data quality. Predictive models rely heavily on historical data, and if this data is flawed, incomplete, or biased, the predictions generated can lead to erroneous conclusions. For instance, if a healthcare model is trained on data that lacks diversity, it may not be effective across different demographics, potentially leading to harmful outcomes.
Moreover, the sheer volume of data can be overwhelming. Organizations often struggle to manage and clean their data effectively. This leads to a scenario where analysts spend more time preparing data than actually analyzing it. To illustrate, consider a financial institution that collects vast amounts of transaction data. If they fail to address inconsistencies, such as duplicate entries or missing values, the predictive models they develop will likely yield unreliable results.
Another significant challenge is the ethical considerations surrounding AI in predictive analysis. As algorithms become more sophisticated, questions arise about privacy and bias. For example, if a model is trained on biased data, it may perpetuate existing inequalities. This is particularly concerning in sectors like law enforcement or hiring, where biased predictions can have serious repercussions. Organizations must prioritize the development of ethical frameworks to ensure that AI tools are used responsibly and transparently.
To address these challenges, companies can implement several strategies:
- Data Governance: Establishing clear policies for data collection, storage, and processing can enhance data quality.
- Bias Mitigation: Regular audits of AI models can help identify and correct biases in predictions.
- Stakeholder Engagement: Involving diverse groups in the development of predictive models can ensure that multiple perspectives are considered.
In summary, while AI in predictive analysis offers tremendous potential, organizations must navigate the complexities of data quality and ethical considerations. By addressing these challenges proactively, they can harness the full power of AI to make informed decisions and drive positive outcomes.
Q: What are the main challenges of using AI in predictive analysis?
A: The main challenges include data quality issues, ethical considerations regarding bias and privacy, and the sheer volume of data that can complicate analysis.
Q: How can organizations improve data quality for predictive analysis?
A: Organizations can improve data quality by implementing data governance policies, regularly auditing their data sources, and ensuring that their datasets are diverse and representative.
Q: Why is ethical consideration important in AI predictive analysis?
A: Ethical considerations are crucial to prevent bias in predictions, protect individual privacy, and ensure that AI systems are used responsibly and transparently.

Data Quality Issues
When it comes to predictive analysis, the quality of data is paramount. Think of data as the raw material for a chef; if the ingredients are subpar, the dish will inevitably suffer. This analogy holds true in the realm of AI-driven analytics, where poor data quality can skew results, leading to inaccurate predictions and misguided decisions. The challenges surrounding data quality can be categorized into several key issues, each of which can significantly impact the effectiveness of predictive models.
One of the most common issues is incomplete data. Imagine trying to solve a puzzle with missing pieces; you can guess what the picture looks like, but you’ll never truly know. Incomplete datasets can arise from various sources, such as human error during data entry or system malfunctions. This lack of completeness can lead to biased predictions, as the model may not have enough information to make accurate assessments.
Another critical factor is data inconsistency. This occurs when the same data is recorded in different formats or values across various datasets. For instance, a customer’s name might be spelled differently in two separate databases, leading to confusion and misinterpretation. Such inconsistencies can create significant hurdles in predictive analysis, as they can distort the underlying patterns that algorithms rely on. To mitigate this, organizations must implement strict data governance policies and standardize data entry processes.
Moreover, data accuracy is essential for reliable predictions. Inaccurate data can stem from outdated information, erroneous entries, or even deliberate manipulation. For example, if a financial institution uses outdated credit scores for predictive modeling, it might make poor lending decisions, which could result in significant financial losses. Therefore, regular data audits and updates are crucial to maintain accuracy.
Lastly, data relevance cannot be overlooked. Even if data is complete, consistent, and accurate, it must also be pertinent to the predictive model at hand. Using irrelevant data is akin to trying to drive a car with an engine that doesn’t fit; it simply won’t work effectively. Organizations must ensure that the data they use aligns with the specific objectives of their predictive analysis.
In summary, addressing data quality issues is vital for the success of predictive analysis. By focusing on completeness, consistency, accuracy, and relevance, organizations can enhance their predictive models and make more informed decisions. Ultimately, high-quality data is the foundation upon which effective predictive insights are built, paving the way for better outcomes across various sectors.
- What is data quality, and why is it important in predictive analysis?
Data quality refers to the condition of a dataset based on factors like accuracy, completeness, consistency, and relevance. In predictive analysis, high-quality data ensures that the predictions made by AI models are reliable and actionable. - How can organizations improve their data quality?
Organizations can enhance data quality by implementing stringent data governance practices, conducting regular audits, standardizing data entry processes, and ensuring that data remains current and relevant. - What are the consequences of poor data quality in predictive analysis?
Poor data quality can lead to inaccurate predictions, misguided business decisions, financial losses, and a lack of trust in data-driven insights.

Ethical Considerations
As we dive deeper into the realm of artificial intelligence and predictive analysis, we can't ignore the that come into play. With great power comes great responsibility, and the capabilities of AI to analyze vast amounts of data raise significant questions about privacy, bias, and accountability. It's like giving a teenager the keys to a sports car; while they might be thrilled, it’s essential to ensure they drive responsibly.
One of the primary concerns is data privacy. As AI systems collect and analyze personal data to make predictions, there's a risk that sensitive information could be misused or inadequately protected. Imagine a healthcare system that predicts patient outcomes based on personal health data; if that data is leaked or exploited, it could have dire consequences for individuals. Thus, implementing robust data protection measures is crucial.
Another pressing ethical issue is algorithmic bias. AI systems learn from historical data, and if this data reflects existing societal biases, the predictions made can perpetuate and even worsen these biases. For instance, if a predictive model used in hiring processes has been trained on biased data, it may unfairly disadvantage certain groups. This scenario can create a ripple effect, leading to systemic inequalities. It's essential to continuously audit and refine these algorithms to ensure they promote fairness and inclusivity.
Transparency is also a vital aspect of ethical AI usage. Stakeholders need to understand how predictions are made and the reasoning behind them. Imagine receiving a loan denial without any explanation; it leaves individuals feeling powerless and frustrated. By fostering transparency, organizations can build trust with users and ensure that AI systems are held accountable for their decisions.
Lastly, it's important to consider the ethical frameworks guiding the development and implementation of AI technologies. Organizations should establish guidelines that prioritize ethical considerations in predictive analysis. These frameworks can help navigate the complexities of AI deployment, ensuring that the technology is used responsibly and ethically. Just like a compass guides a traveler, these frameworks can steer AI development in the right direction.
In summary, while AI has the potential to revolutionize predictive analysis, it’s imperative to address these ethical considerations. Protecting privacy, mitigating bias, ensuring transparency, and establishing ethical guidelines are not just best practices; they are essential for creating a future where AI serves humanity positively and equitably.
- What are the main ethical concerns with AI and predictive analysis?
The primary concerns include data privacy, algorithmic bias, transparency, and the need for ethical frameworks to guide AI development.
- How can organizations ensure data privacy in AI?
Organizations can implement strong data protection measures, such as encryption, access controls, and regular audits to safeguard personal information.
- What is algorithmic bias, and why is it a problem?
Algorithmic bias occurs when AI systems reflect existing societal biases due to biased training data, leading to unfair outcomes and perpetuating inequalities.
- Why is transparency important in AI?
Transparency helps build trust between users and organizations, allowing individuals to understand how decisions are made and ensuring accountability.
Frequently Asked Questions
- What is predictive analysis?
Predictive analysis is a statistical technique that utilizes historical data and algorithms to forecast future outcomes. It's like looking into a crystal ball, but instead of magic, it relies on data-driven insights to help businesses and organizations make informed decisions.
- How does AI enhance predictive analysis?
AI enhances predictive analysis by automating complex data processes and revealing hidden patterns that humans might overlook. Think of AI as a super-smart assistant that can sift through mountains of data in a fraction of the time, improving both accuracy and efficiency in predictions.
- What are the main types of machine learning used in predictive analysis?
The two main types of machine learning in predictive analysis are supervised and unsupervised learning. Supervised learning works with labeled datasets to train models, while unsupervised learning identifies patterns in data without labels, making it useful for discovering trends and anomalies.
- Can AI be used in healthcare predictive analysis?
Absolutely! AI-driven predictive analysis in healthcare can aid in patient diagnosis and treatment planning. It helps healthcare providers anticipate patient needs and improve operational efficiency, ultimately leading to better patient outcomes.
- What challenges does AI face in predictive analysis?
AI in predictive analysis encounters challenges such as data quality issues and ethical concerns. Poor data quality can lead to inaccurate predictions, while ethical issues often revolve around privacy and bias in AI algorithms, making it essential to establish robust ethical frameworks.
- How does natural language processing (NLP) fit into predictive analysis?
NLP allows machines to comprehend human language, making it a powerful tool in predictive analysis. It is particularly useful for sentiment analysis and extracting customer insights, helping businesses understand consumer behavior and preferences better.