Observing Ethical Boundaries in AI Innovation
In today's rapidly evolving technological landscape, the development of artificial intelligence (AI) presents both incredible opportunities and daunting challenges. As we stand on the brink of a new era, where machines can learn, adapt, and even make decisions, the question arises: how do we ensure that this powerful tool is used ethically? The concept of ethical boundaries in AI innovation is not just a theoretical discussion; it is a critical necessity for the well-being of society. Without proper ethical considerations, we risk creating systems that may reinforce biases, invade privacy, or operate without accountability.
Imagine a world where AI systems make decisions that directly affect our lives—like hiring choices, loan approvals, or even medical diagnoses. The implications are profound. If these systems are not held to ethical standards, they could perpetuate inequalities and injustices rather than alleviate them. Thus, the importance of observing ethical boundaries cannot be overstated. It is about ensuring that technological advancements serve to enhance human dignity and societal welfare.
Furthermore, as we delve deeper into the realm of AI, we must recognize the role of public trust. People are increasingly aware of how technology impacts their lives, and they demand transparency and accountability from the organizations that develop these systems. By adhering to ethical principles, AI innovators can build trust with users, fostering a relationship that is not only beneficial but also respectful of individual rights and societal norms.
To navigate these complex waters, we must embrace a framework of ethical guidelines that encompasses various aspects of AI development. This includes recognizing and addressing bias in algorithms, ensuring transparency in AI operations, establishing accountability measures, and respecting user privacy. Each of these areas plays a crucial role in shaping the future of AI innovation, paving the way for a responsible and ethical approach to technology.
In this article, we will explore these ethical considerations in detail, examining the fundamental principles that guide AI innovation and the practical steps we can take to uphold these standards. From identifying biases in data to establishing clear accountability frameworks, we will uncover the essential elements that contribute to ethical AI development. By doing so, we aim to inspire a collective commitment to responsible innovation that not only embraces technological advancement but also prioritizes the well-being of society.
- What are the main ethical concerns in AI development? The primary concerns include bias in algorithms, lack of transparency, accountability issues, and privacy violations.
- How can organizations ensure fairness in AI? Organizations can implement diverse data sets, conduct regular audits, and promote inclusive practices to mitigate bias.
- Why is transparency important in AI? Transparency fosters trust and allows users to understand how AI systems make decisions, which is crucial for ethical engagement.
- What role do regulations play in ethical AI? Regulations help establish standards and guidelines that promote ethical practices, ensuring that AI development aligns with societal values.
- How can user privacy be protected in AI applications? Effective data protection measures, informed consent, and respecting user autonomy are key to safeguarding privacy in AI.
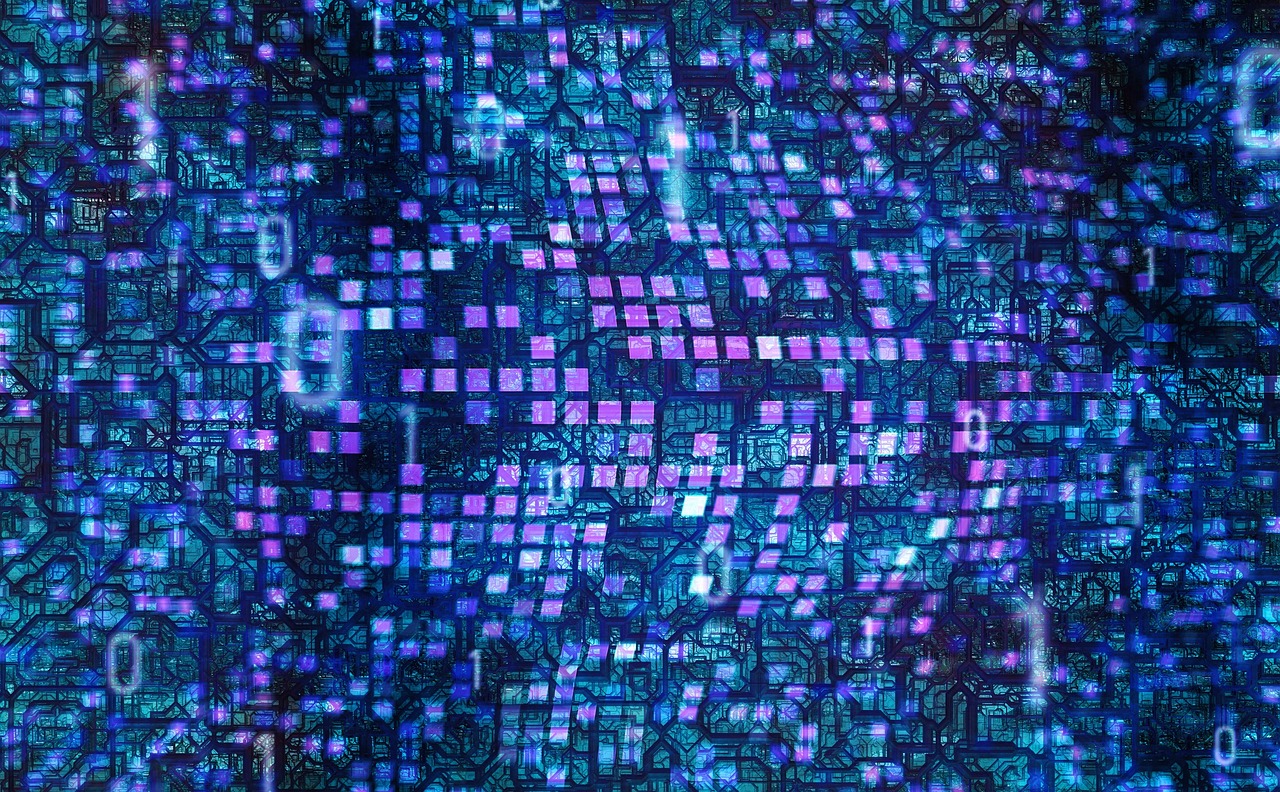
The Importance of Ethics in AI
In today's rapidly evolving digital landscape, the significance of ethics in artificial intelligence (AI) cannot be overstated. As we integrate AI into various aspects of our lives—from healthcare to transportation—it's crucial to understand that ethical considerations are not just an afterthought; they are the bedrock upon which responsible innovation is built. Why is this so important? Well, imagine a world where AI systems operate without ethical guidelines. The potential for misuse and harm could be staggering, leading to a society that mistrusts technology rather than embraces it.
Ethics in AI serves as a compass, guiding developers and organizations toward creating solutions that prioritize human welfare. By addressing ethical concerns proactively, we can mitigate risks associated with AI deployment, such as bias, privacy violations, and unintended consequences. This not only enhances public trust in technology but also ensures that innovations are aligned with societal values. Think of it as a safety net—one that catches potential pitfalls before they become catastrophic failures.
Moreover, ethical AI fosters inclusivity and fairness. When we incorporate diverse perspectives and backgrounds in the development process, we create systems that cater to a broader range of needs and experiences. For example, consider how biased algorithms can perpetuate inequality. By prioritizing ethics, we can work towards eliminating such biases, ensuring that AI serves everyone equitably. This is not just about fairness; it's about creating technology that uplifts society as a whole.
In summary, the importance of ethics in AI lies in its ability to:
- Mitigate risks and prevent harm
- Enhance public trust and acceptance
- Promote fairness and inclusivity
- Align innovations with societal values
As we move forward, it’s essential to remember that ethical considerations are not static. They evolve as technology advances and societal norms shift. Therefore, ongoing dialogue and collaboration among stakeholders—including developers, policymakers, and the public—are necessary to ensure that AI continues to benefit humanity. The stakes are high, but with a strong ethical foundation, we can harness the full potential of AI for good.
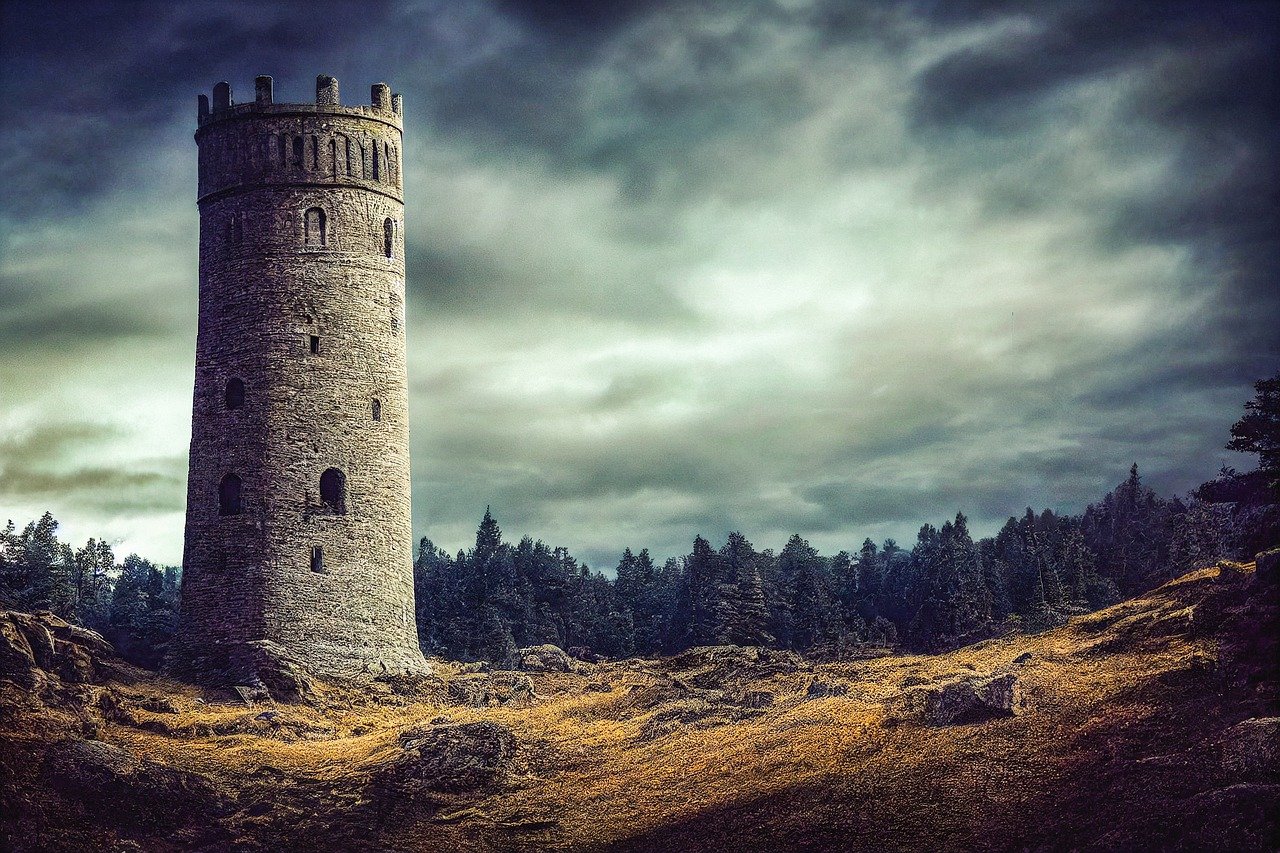
Key Ethical Principles
When we dive into the world of artificial intelligence, it's crucial to anchor our innovations in strong ethical principles. These principles serve as the compass guiding developers and organizations through the murky waters of technology. Without them, we risk creating systems that could harm society or exacerbate existing inequalities. The key ethical principles that should guide AI innovation include fairness, transparency, accountability, and privacy. Each of these principles plays a vital role in shaping a future where technology benefits everyone rather than a select few.
First up is fairness. Imagine a world where AI systems make decisions that impact lives—like hiring, lending, or even law enforcement. If these systems are biased, they can perpetuate inequalities. Therefore, it’s essential to ensure that AI algorithms are designed to promote equity, not discrimination. This involves scrutinizing data sources and algorithms to identify and mitigate biases. Developers must ask themselves: Are we treating all individuals fairly? Are we reinforcing stereotypes?
Next, we have transparency. In a time when technology is often viewed as a black box, transparency is more important than ever. Users should have a clear understanding of how AI systems work and the rationale behind their decisions. This is where explainable AI comes into play. By making AI processes understandable, we can foster trust and encourage users to embrace technology rather than fear it. Imagine trying to navigate a maze without knowing where the exits are; that’s how users feel when they don’t understand AI systems!
Moving on to accountability, it’s essential that developers and organizations are held responsible for the societal impacts of their technologies. This means establishing clear frameworks that outline who is accountable when things go wrong. If an AI system makes a harmful decision, who is to blame? Understanding this is crucial for building public trust and ensuring that developers prioritize ethical considerations in their work.
Lastly, we cannot forget about privacy. In an age where data is the new oil, protecting user information is paramount. Organizations must implement robust data protection measures to safeguard personal data from breaches or misuse. This includes adhering to regulations and being transparent about how user data is collected, stored, and utilized. Furthermore, obtaining informed consent from users is not just a legal obligation; it’s a moral one. Users should feel empowered to control their own data and understand how it’s being used in AI applications.
In summary, these key ethical principles—fairness, transparency, accountability, and privacy—are not just buzzwords; they are the bedrock of responsible AI innovation. By adhering to these principles, we can ensure that the technology we create serves humanity positively, paving the way for a future where AI and society coexist harmoniously.
- What is the significance of ethical principles in AI? Ethical principles guide the development of AI technologies to ensure they are fair, transparent, accountable, and respect user privacy.
- How can bias be identified in AI algorithms? Bias can be identified by analyzing the datasets used for training AI and checking for representation across different demographics.
- What does explainable AI mean? Explainable AI refers to AI systems that can provide clear explanations for their decisions, enhancing user trust and understanding.
- Why is accountability important in AI? Accountability ensures that developers and organizations are responsible for the impacts of their technologies, fostering trust and ethical practices.
- How can user privacy be protected in AI? Organizations can protect user privacy by implementing strong data protection measures and obtaining informed consent from users.
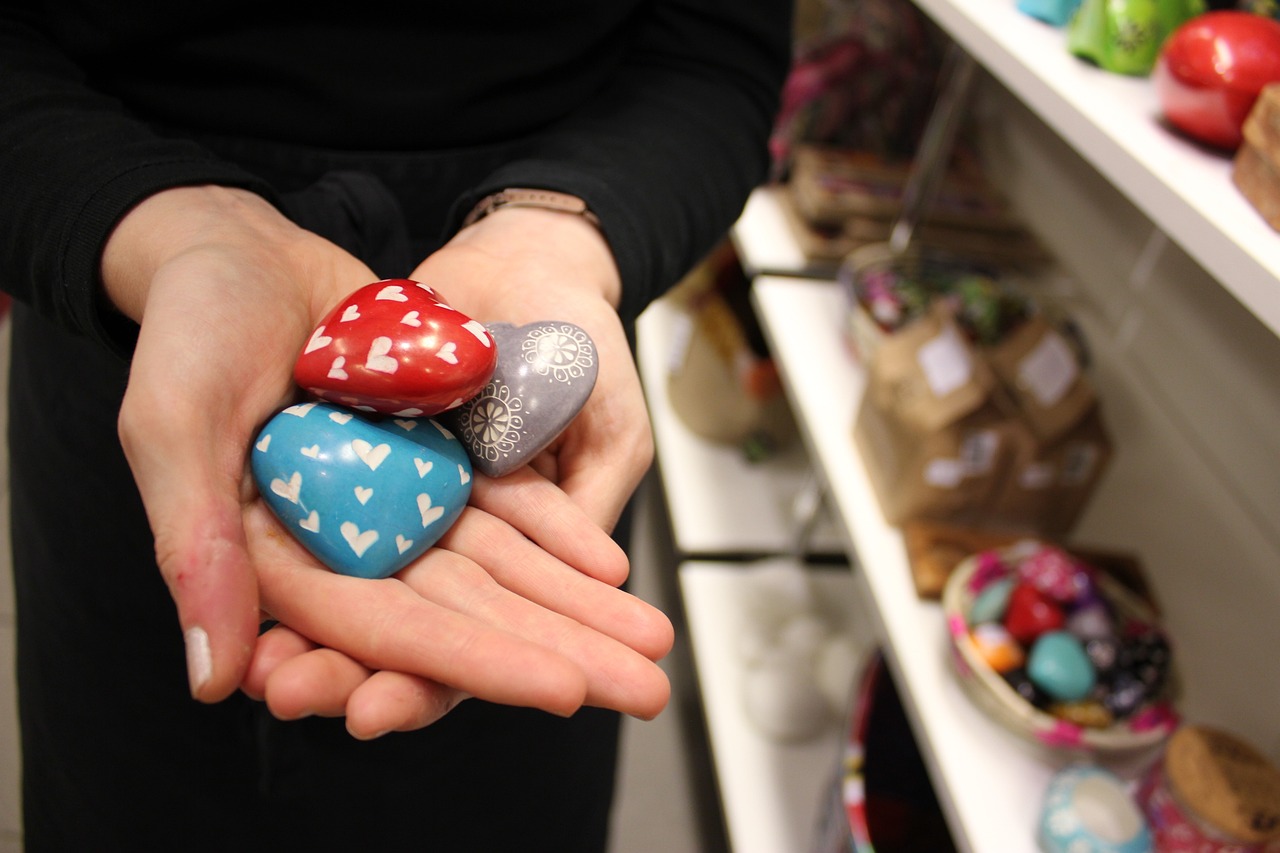
Fairness in AI Algorithms
When we talk about , we step into a complex realm where technology meets ethics. Imagine a world where decisions that impact our lives—from job applications to loan approvals—are made by machines. Sounds efficient, right? But what if those machines are biased? That's the crux of the issue. Bias in AI can stem from various sources, often leading to unfair outcomes that can disproportionately affect certain groups. It’s like having a referee in a game who only sees one side of the field; the results will undoubtedly be skewed.
To truly understand fairness in AI, we need to recognize how bias can creep into algorithms. Bias often starts at the data level. If the data used to train an AI model is skewed or unrepresentative, the outcomes will reflect those disparities. For instance, if an AI system is trained primarily on data from one demographic group, it may fail to accurately assess or serve others. This can result in significant consequences, such as reinforcing stereotypes or perpetuating inequalities. Therefore, identifying bias in data is critical.
Identifying bias is not merely a technical challenge; it's a societal imperative. We can use several methods to detect bias within datasets:
- Statistical Analysis: Employing statistical tests can help identify disparities in how different groups are represented.
- Diverse Data Sources: Ensuring that the data comes from a variety of sources can help paint a more accurate picture.
- Regular Audits: Conducting audits of AI systems can reveal hidden biases that may not be immediately apparent.
Once we identify bias, the next step is to mitigate it. This is where the real work begins. It’s not enough to just point out the problem; we need actionable strategies to rectify it.
There are several strategies and techniques that developers can implement to reduce bias in AI algorithms:
- Regular Audits: Frequent audits can help ensure that algorithms are functioning as intended and not perpetuating biases.
- Algorithmic Transparency: By making algorithms more transparent, developers can invite scrutiny and feedback, which can help catch biases early.
- Diverse Development Teams: Having a diverse team working on AI projects can bring different perspectives and reduce the risk of bias.
Ultimately, achieving fairness in AI algorithms requires a concerted effort from everyone involved in the development process. It’s about fostering an environment where ethical considerations are at the forefront of innovation. When developers prioritize fairness, they not only enhance the credibility of AI technologies but also contribute to a more equitable society. In a world increasingly influenced by AI, ensuring fairness isn't just a technical requirement; it's a moral obligation.
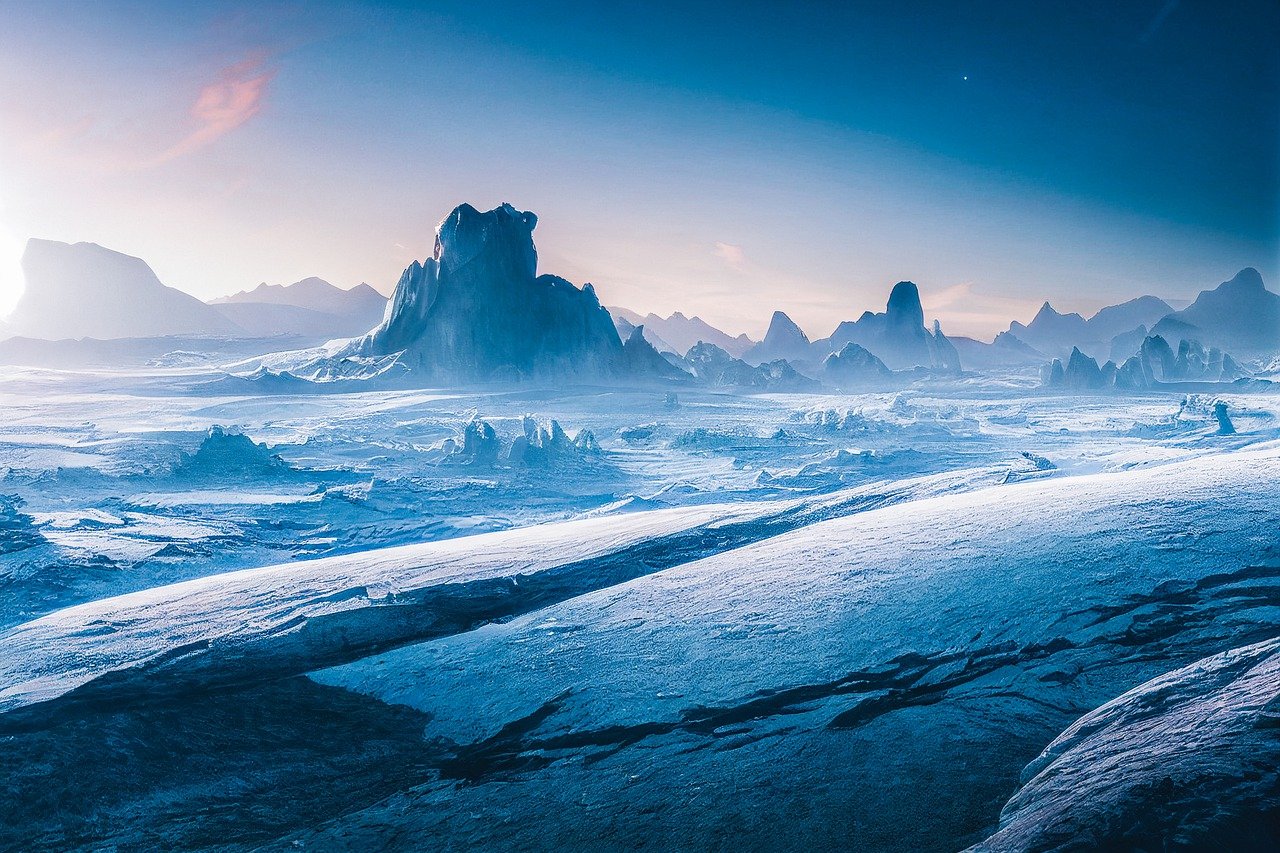
Identifying Bias in Data
In the realm of artificial intelligence, the foundation of any robust AI system is its data. However, the presence of bias in this data can lead to skewed outcomes, reinforcing stereotypes and perpetuating inequality. So, how do we actually identify bias in data? It’s not just about looking for discrepancies; it’s about understanding the broader context of the data we’re working with.
First and foremost, it’s essential to recognize that bias can manifest in various forms. It might be a result of historical inequalities, where certain groups have been underrepresented or misrepresented in the data. For instance, if an AI system is trained primarily on data from a specific demographic, it may not perform well for individuals outside that group. This is why having a diverse and representative dataset is crucial.
To effectively identify bias, organizations can implement several strategies:
- Data Audits: Conducting thorough audits of datasets can reveal patterns of bias. This involves analyzing the data for representation across different demographics, such as race, gender, and socio-economic status.
- Statistical Analysis: Employing statistical methods to assess the fairness of the data can help pinpoint imbalances. Techniques like disparity analysis can highlight whether certain groups are disadvantaged by the dataset.
- Stakeholder Involvement: Engaging with community stakeholders who represent diverse perspectives can provide valuable insights into potential biases that may not be immediately apparent from the data alone.
Moreover, it’s important to consider the sources of the data. Are they reliable? Were they collected ethically? Understanding the origins of the data can shed light on inherent biases. For example, if data is sourced from social media, it may reflect the biases of its users, who may not represent the entire population.
Another critical aspect of identifying bias is to regularly revisit and update datasets. As society evolves, so do the norms and values that shape our understanding of fairness and equality. What was considered an acceptable representation a decade ago may no longer hold true today. Therefore, ongoing evaluation and adjustment of datasets are imperative for maintaining relevance and fairness in AI systems.
In conclusion, identifying bias in data is a multifaceted process that requires diligence, transparency, and a commitment to ethical practices. By actively seeking out and addressing biases, we can work towards creating AI systems that are not only innovative but also just and equitable for all.
- What is data bias?
Data bias occurs when the data used to train AI systems is skewed or unrepresentative of the broader population, leading to unfair outcomes. - How can organizations ensure their data is unbiased?
Organizations can conduct data audits, involve diverse stakeholders, and regularly update their datasets to ensure fairness and representation. - Why is it important to identify bias in AI?
Identifying bias in AI is crucial to prevent perpetuating inequalities and to ensure that AI systems benefit all segments of society.
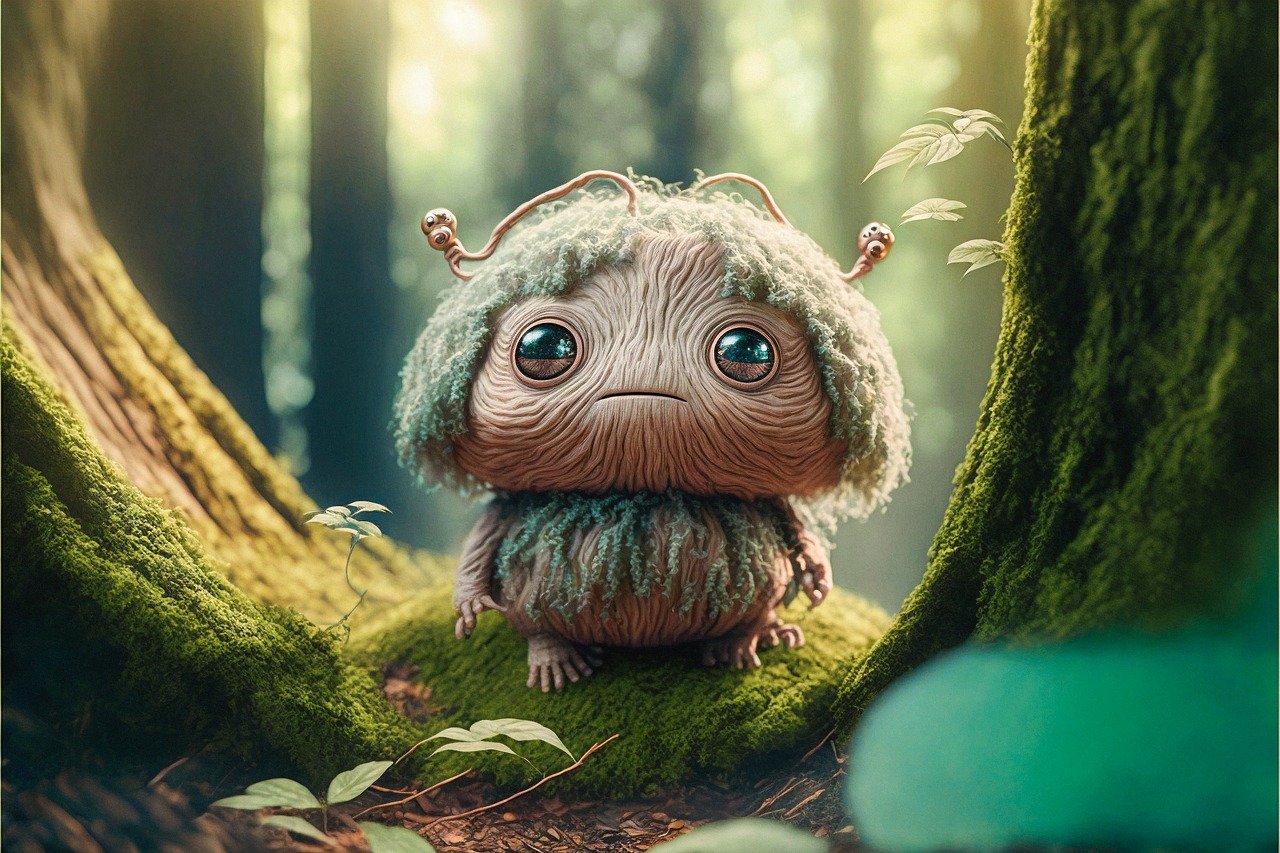
Mitigating Algorithmic Bias
In the rapidly evolving landscape of artificial intelligence, algorithmic bias poses a significant challenge that can undermine the very foundations of fairness and equity in AI-driven decisions. To combat this issue, developers and organizations must adopt a proactive approach in identifying and mitigating biases that can creep into AI algorithms. But what does this actually entail? It’s not just about tweaking a few lines of code; it’s a comprehensive strategy that requires a deep understanding of the data, the algorithms, and the societal implications of their deployment.
One of the most effective ways to mitigate algorithmic bias is through regular audits of AI systems. These audits serve as a critical checkpoint, allowing developers to assess the performance of their algorithms and identify any unintended biases that may have emerged during the training process. Imagine driving a car without checking the fuel gauge; you might run out of gas unexpectedly. Similarly, failing to audit AI systems can lead to unforeseen consequences that affect real lives.
Another crucial strategy involves algorithmic transparency. This means making the workings of AI algorithms understandable to users and stakeholders. When people can see how decisions are made, they are more likely to trust the technology. Transparency can be enhanced by implementing explainable AI techniques, which break down complex algorithms into simpler, more understandable components. For instance, using visualizations to illustrate how an algorithm arrived at a decision can demystify the process and foster greater trust among users.
Moreover, organizations should prioritize the use of diverse and representative datasets when training AI algorithms. Bias often stems from unbalanced datasets that fail to capture the full spectrum of human experience. By ensuring that training data includes a wide variety of demographics, experiences, and perspectives, developers can create algorithms that are more equitable and less prone to bias. This is akin to cooking a stew; if you only add a few ingredients, the flavor will be one-dimensional. A rich, diverse mix yields a much more satisfying outcome.
Lastly, fostering a culture of ethical awareness within organizations is essential. By training teams on the implications of algorithmic bias and the importance of fairness in AI, companies can cultivate an environment where ethical considerations are at the forefront of innovation. This cultural shift is not just about compliance; it’s about creating a mindset that values responsibility and accountability, ensuring that the technologies developed are beneficial for all.
- What is algorithmic bias? Algorithmic bias refers to systematic and unfair discrimination that occurs when algorithms produce biased outcomes based on the data they are trained on.
- How can organizations identify bias in their AI systems? Organizations can identify bias by conducting regular audits, analyzing algorithm results across different demographic groups, and using fairness metrics.
- What role does transparency play in mitigating algorithmic bias? Transparency helps users understand how decisions are made, fostering trust and allowing for scrutiny that can highlight biases in the algorithms.
- Why is diverse data important in AI development? Diverse data helps ensure that AI systems are trained on a wide range of experiences and perspectives, reducing the risk of biased outcomes.
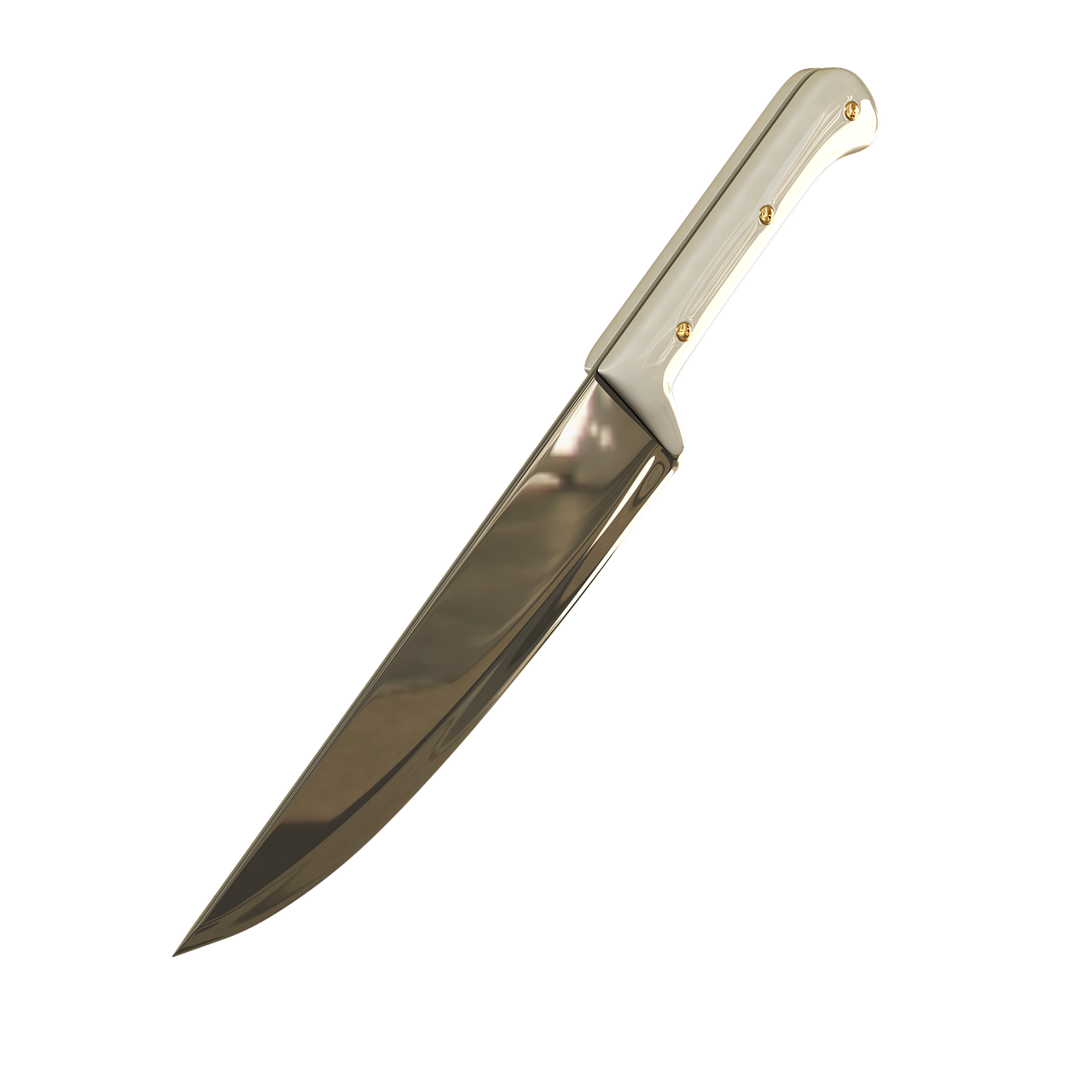
Transparency and Explainability
In the rapidly evolving landscape of artificial intelligence (AI), transparency and explainability serve as the bedrock for fostering trust between technology and its users. Imagine trying to navigate a complex maze without a map; that’s akin to using AI systems that operate as black boxes. Users often find themselves unsure of how decisions are made, leading to skepticism and mistrust. To bridge this gap, it is crucial that AI systems are designed with transparency at their core, enabling users to understand not just the outcomes but also the reasoning behind them. This understanding is vital for users to feel comfortable and secure in their interactions with AI technologies.
One of the primary challenges in achieving transparency lies in the intricate nature of many AI algorithms, particularly those based on deep learning. These models can process vast amounts of data and identify patterns that are not immediately apparent to humans. However, the complexity can lead to situations where even developers struggle to explain how a decision was reached. This is where the need for explainable AI (XAI) comes into play. XAI aims to create AI systems that are not only effective but also comprehensible. By providing insights into the decision-making processes, users can gain confidence in the technology, ultimately enhancing its acceptance and integration into society.
To facilitate transparency, organizations can adopt several strategies:
- Clear Communication: Companies should openly share information about how their AI systems work, including the data sources and algorithms used.
- Visualization Tools: Implementing tools that visually represent how decisions are made can help demystify AI processes for end-users.
- User-Centric Design: Designing AI systems with the end-user in mind ensures that explanations are accessible and relevant, catering to a diverse audience.
Moreover, the significance of transparency extends beyond user trust; it also plays a critical role in accountability. When AI systems are transparent, it becomes easier to identify and rectify errors or biases, ensuring that the technology serves its intended purpose without causing harm. For instance, if an AI system makes a biased hiring decision, transparency allows stakeholders to trace back through the decision-making process, identifying the source of the bias and addressing it effectively.
In conclusion, the journey towards ethical AI innovation is paved with the principles of transparency and explainability. By prioritizing these elements, developers can create AI systems that not only perform well but also earn the trust of users. As we continue to integrate AI into various aspects of life, understanding and clarity will be paramount in ensuring that these technologies enhance human experience rather than complicate it.
What is transparency in AI?
Transparency in AI refers to the clarity with which users can understand how AI systems operate, including the data and algorithms used in decision-making processes.
Why is explainability important in AI?
Explainability is crucial because it helps users comprehend the rationale behind AI decisions, fostering trust and ensuring accountability in the technology.
How can organizations improve transparency?
Organizations can improve transparency by clearly communicating how their AI systems work, using visualization tools, and designing user-centric interfaces that make explanations accessible.
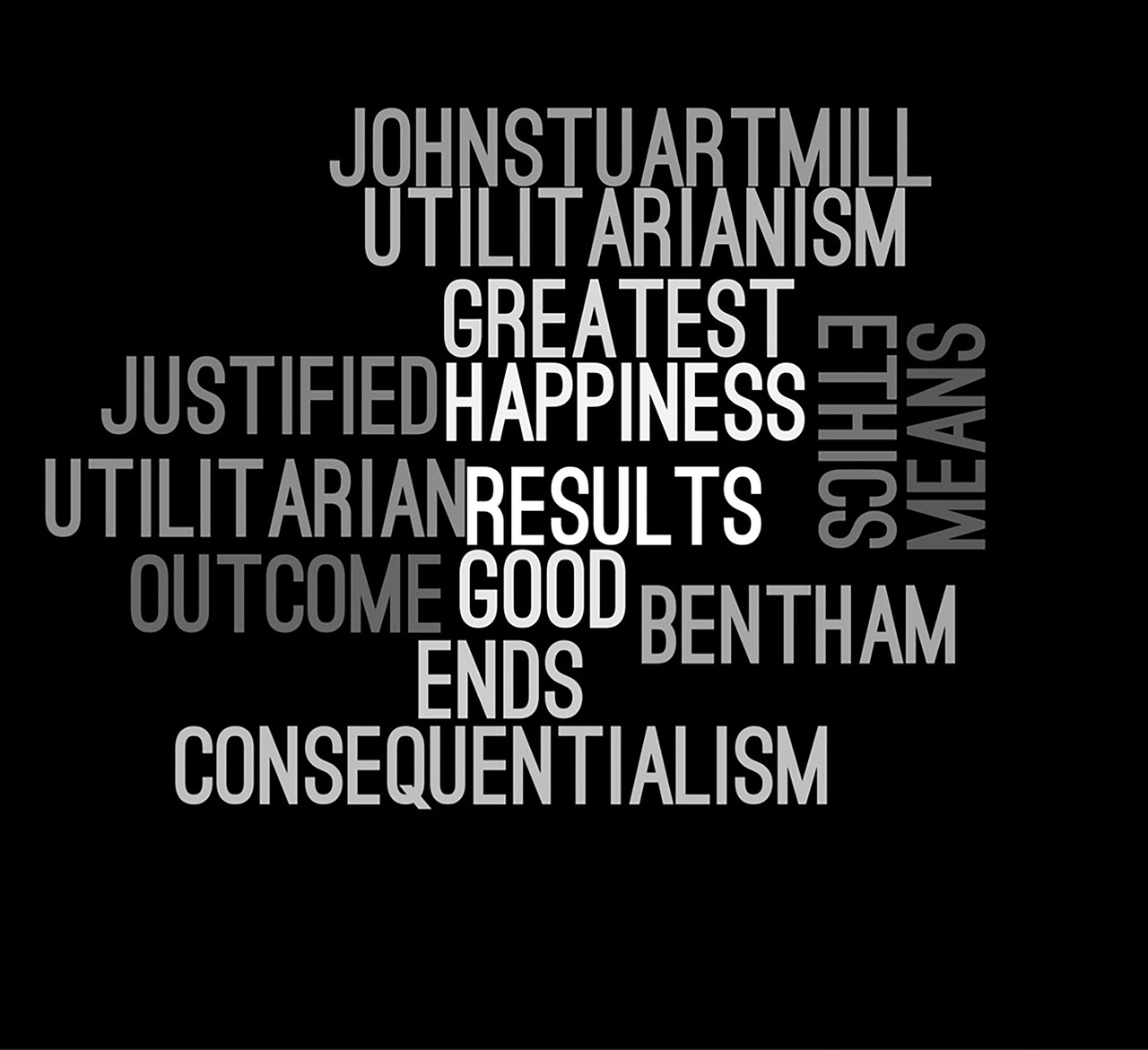
Accountability in AI Development
In the fast-paced world of artificial intelligence (AI), where innovation often outstrips regulation, the concept of accountability is more critical than ever. As AI systems become increasingly integrated into our daily lives, the need to establish clear lines of responsibility for their development and deployment cannot be overstated. Imagine a self-driving car making a mistake that leads to an accident; who is to blame—the manufacturer, the software developer, or the user? This ambiguity highlights the urgent need for accountability frameworks that clearly define the roles and responsibilities of all parties involved in AI innovation.
One of the primary challenges in ensuring accountability in AI development is the complexity of the technology itself. AI systems often operate as black boxes, making it difficult to trace back decisions to specific actions or inputs. This lack of transparency can lead to a situation where harmful outcomes occur without anyone being held responsible. Therefore, establishing a framework of accountability is not just about assigning blame; it is about creating a culture of responsibility that prioritizes ethical considerations throughout the development process.
To effectively implement accountability in AI, several key components must be considered:
- Regulatory Frameworks: Governments and regulatory bodies must develop comprehensive guidelines that outline the responsibilities of AI developers and organizations. These regulations should address issues such as safety, ethical standards, and consumer protection.
- Internal Accountability Measures: Organizations should establish internal policies that promote accountability among their teams. This includes regular audits, performance reviews, and the establishment of ethics committees to oversee AI projects.
- Public Engagement: Engaging with the public and stakeholders is essential for building trust. Organizations should be open about their AI practices and encourage feedback to foster a sense of community involvement in ethical AI development.
Moreover, the establishment of ethical guidelines plays a crucial role in promoting accountability. By providing a clear set of principles for AI practitioners, these guidelines can help ensure that all stakeholders are aware of their responsibilities. Ethical guidelines should encompass various aspects of AI, including:
Aspect | Description |
---|---|
Fairness | Ensuring that AI systems do not perpetuate bias or discrimination. |
Transparency | Making AI decision-making processes understandable and accessible. |
Accountability | Establishing clear lines of responsibility for AI outcomes. |
Privacy | Protecting user data and ensuring informed consent. |
In conclusion, accountability in AI development is not just a regulatory requirement; it is a moral imperative. As we navigate the complexities of AI technology, it is essential to create robust accountability frameworks that ensure developers and organizations are held responsible for the societal impacts of their innovations. By prioritizing accountability, we can foster a culture of ethical AI development that benefits everyone while minimizing risks.
Q1: Why is accountability important in AI development?
A1: Accountability is crucial in AI development to ensure that developers and organizations are responsible for the outcomes of their technologies, fostering trust and ethical practices.
Q2: What role do regulatory frameworks play in AI accountability?
A2: Regulatory frameworks help establish guidelines and responsibilities for AI developers, ensuring compliance with ethical standards and protecting public interests.
Q3: How can organizations promote accountability internally?
A3: Organizations can promote accountability by implementing internal policies, regular audits, and establishing ethics committees to oversee AI projects.
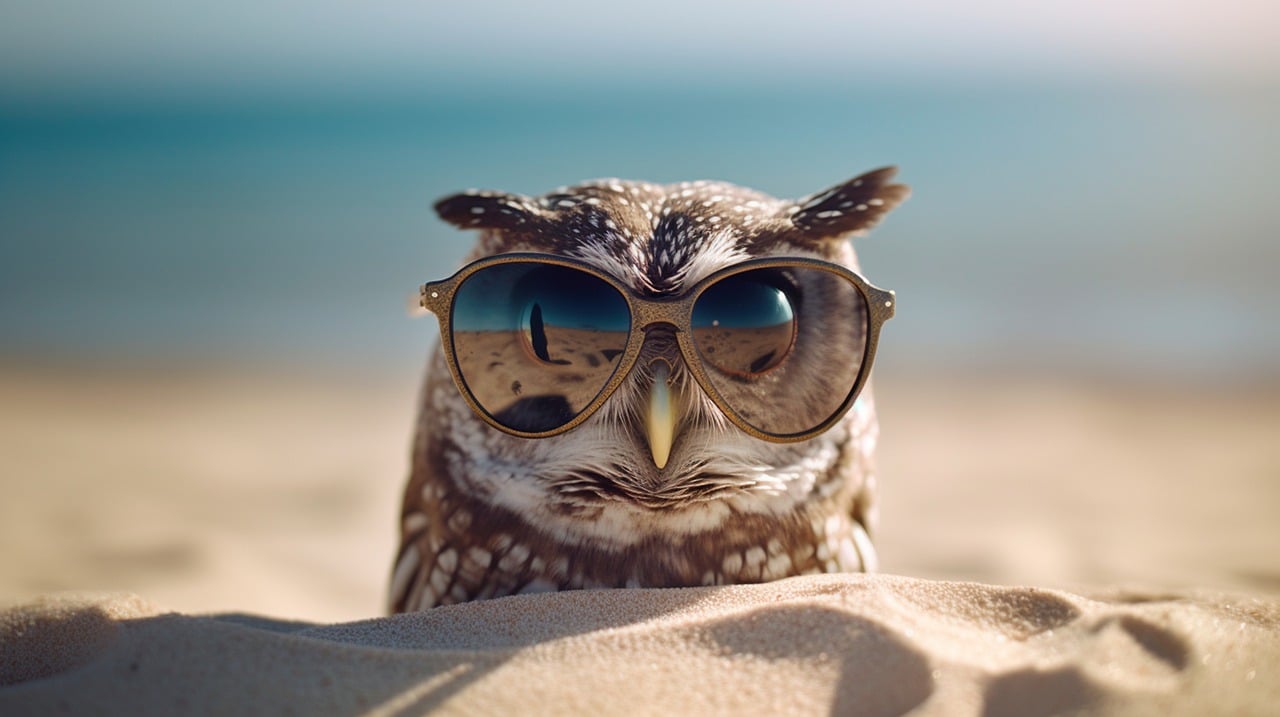
Regulatory Frameworks
In the rapidly evolving landscape of artificial intelligence, the establishment of robust is not just beneficial but essential. These frameworks serve as the backbone for ethical AI practices, ensuring that developers and organizations adhere to standards that prioritize public interest. Without these regulations, the potential for misuse of AI technologies could lead to significant societal harm. Imagine a world where AI systems operate without oversight—chaos would ensue, similar to a ship sailing without a captain.
Regulatory frameworks provide a structured approach to managing the complexities of AI. They outline the responsibilities of developers and organizations, ensuring that they are held accountable for the societal impacts of their technologies. This accountability is crucial, as it fosters trust among users, encouraging them to embrace AI innovations rather than fear them. Transparency and compliance with these regulations are vital in safeguarding public interest, as they create a clear set of expectations for all stakeholders involved.
One significant challenge in establishing these frameworks is the need to keep pace with the rapid advancements in AI technology. Regulations must be adaptable, allowing for flexibility in response to new developments while still maintaining core ethical principles. For instance, consider the GDPR (General Data Protection Regulation) in Europe, which has set a precedent for data protection laws worldwide. It provides a comprehensive approach to user privacy, ensuring that individuals have control over their personal information. Similarly, AI regulations should evolve to address emerging challenges, such as bias in algorithms and data security.
Moreover, the role of international collaboration cannot be understated. AI operates on a global scale, and thus, regulatory frameworks should ideally be harmonized across borders. This would prevent a patchwork of regulations that could confuse developers and stifle innovation. A unified approach could facilitate a smoother implementation of ethical AI practices, ensuring that all organizations, regardless of location, adhere to similar standards. In this context, the establishment of international bodies dedicated to AI ethics and regulation could be a game-changer, promoting best practices and sharing knowledge across nations.
Ultimately, the goal of these regulatory frameworks is to create an environment where AI can flourish while minimizing risks to individuals and society. By fostering collaboration between governments, industry leaders, and ethicists, we can develop a comprehensive set of regulations that not only protect users but also encourage responsible innovation. This proactive approach will help us navigate the complexities of AI development, ensuring that technology serves as a force for good rather than a source of potential harm.
- What are regulatory frameworks in AI?
Regulatory frameworks are sets of guidelines and rules established to govern the ethical development and deployment of artificial intelligence technologies. - Why are regulatory frameworks important?
They are crucial for ensuring accountability, transparency, and public trust in AI systems, helping to mitigate risks associated with misuse or unethical practices. - How can regulatory frameworks keep pace with AI advancements?
By being adaptable and flexible, allowing for updates and revisions as new technologies and challenges emerge in the AI landscape. - Is international collaboration necessary for AI regulations?
Yes, a unified approach across borders can help ensure consistent ethical standards and practices, fostering innovation while protecting users globally.
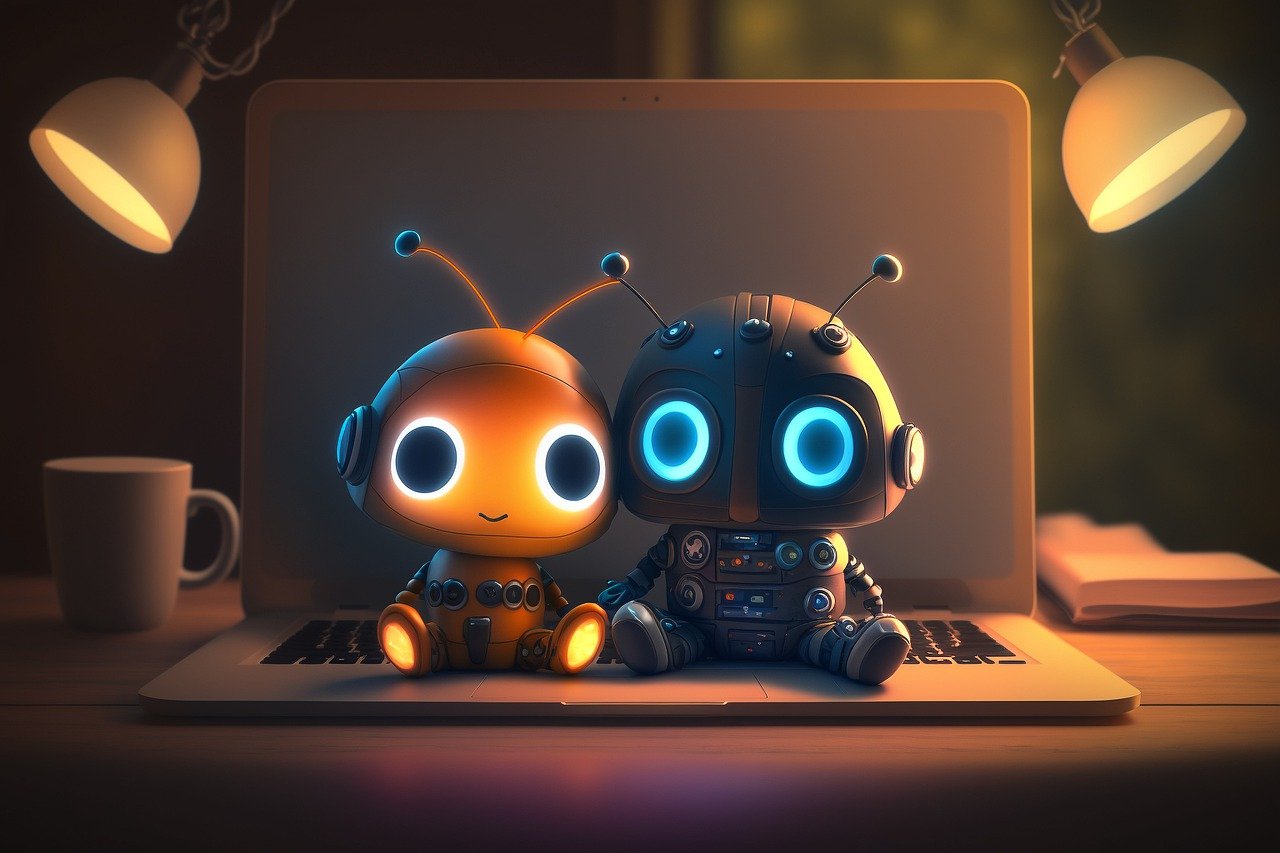
Establishing Ethical Guidelines
In the rapidly evolving world of artificial intelligence, has become more crucial than ever. These guidelines serve as a roadmap for developers, organizations, and policymakers, ensuring that AI technologies are designed and implemented in ways that prioritize human values and societal well-being. But what exactly does it mean to have ethical guidelines in AI? Think of it as a compass that keeps us on course, steering us away from potential pitfalls that could arise from unchecked innovation.
First and foremost, ethical guidelines in AI should emphasize responsibility. This means that developers and organizations must take ownership of the technologies they create and the impacts these technologies have on society. By fostering a culture of accountability, we can ensure that AI innovations are not just about profit margins but also about enhancing the quality of life for everyone. Imagine a world where AI is used to solve pressing issues like climate change or healthcare accessibility; this vision can only be realized if ethical considerations are at the forefront of development.
Moreover, these guidelines should promote collaboration among various stakeholders. This includes not only AI developers but also ethicists, sociologists, and representatives from diverse communities. By engaging a broad spectrum of voices, we can create AI systems that are more inclusive and considerate of different perspectives. It’s like assembling a team for a complex project; each member brings unique insights that contribute to a more robust outcome. This collaborative approach can help identify potential biases and blind spots that a singular viewpoint might overlook.
Another key aspect of establishing ethical guidelines is the necessity for continuous evaluation. The landscape of AI is constantly changing, and what may have been considered ethical yesterday might not hold true today. Therefore, guidelines should not be static documents but rather dynamic frameworks that evolve with technological advancements and societal needs. This is akin to maintaining a garden; it requires regular attention and care to ensure that it thrives. Regular audits and assessments can help in identifying areas needing improvement and adapting to new challenges as they arise.
To illustrate how ethical guidelines can be structured, consider the following table that outlines essential components:
Component | Description |
---|---|
Responsibility | Developers must be accountable for the societal impacts of their AI technologies. |
Collaboration | Engage diverse stakeholders to gather a wide range of insights and perspectives. |
Continuous Evaluation | Regularly update guidelines to adapt to technological and societal changes. |
Transparency | Ensure that AI processes and decisions are understandable and accessible to users. |
Fairness | Implement measures to detect and mitigate biases in AI systems. |
In conclusion, establishing ethical guidelines in AI is not just an optional add-on; it is a fundamental necessity for responsible innovation. By focusing on responsibility, collaboration, continuous evaluation, transparency, and fairness, we can create a framework that not only guides AI development but also builds public trust. After all, the ultimate goal of AI should be to enhance human life, not complicate it. Let’s ensure that the future of technology is one that we can all be proud of.
- What are ethical guidelines in AI? Ethical guidelines in AI are frameworks that help developers and organizations ensure their technologies are designed and implemented responsibly, prioritizing human values and societal well-being.
- Why is collaboration important in establishing ethical guidelines? Collaboration brings diverse perspectives and insights, helping to create more inclusive and considerate AI systems that address potential biases and blind spots.
- How often should ethical guidelines be evaluated? Ethical guidelines should be continuously evaluated and updated to adapt to technological advancements and changing societal needs.
- What role does transparency play in AI ethics? Transparency ensures that AI processes and decisions are understandable, fostering trust and accountability among users.
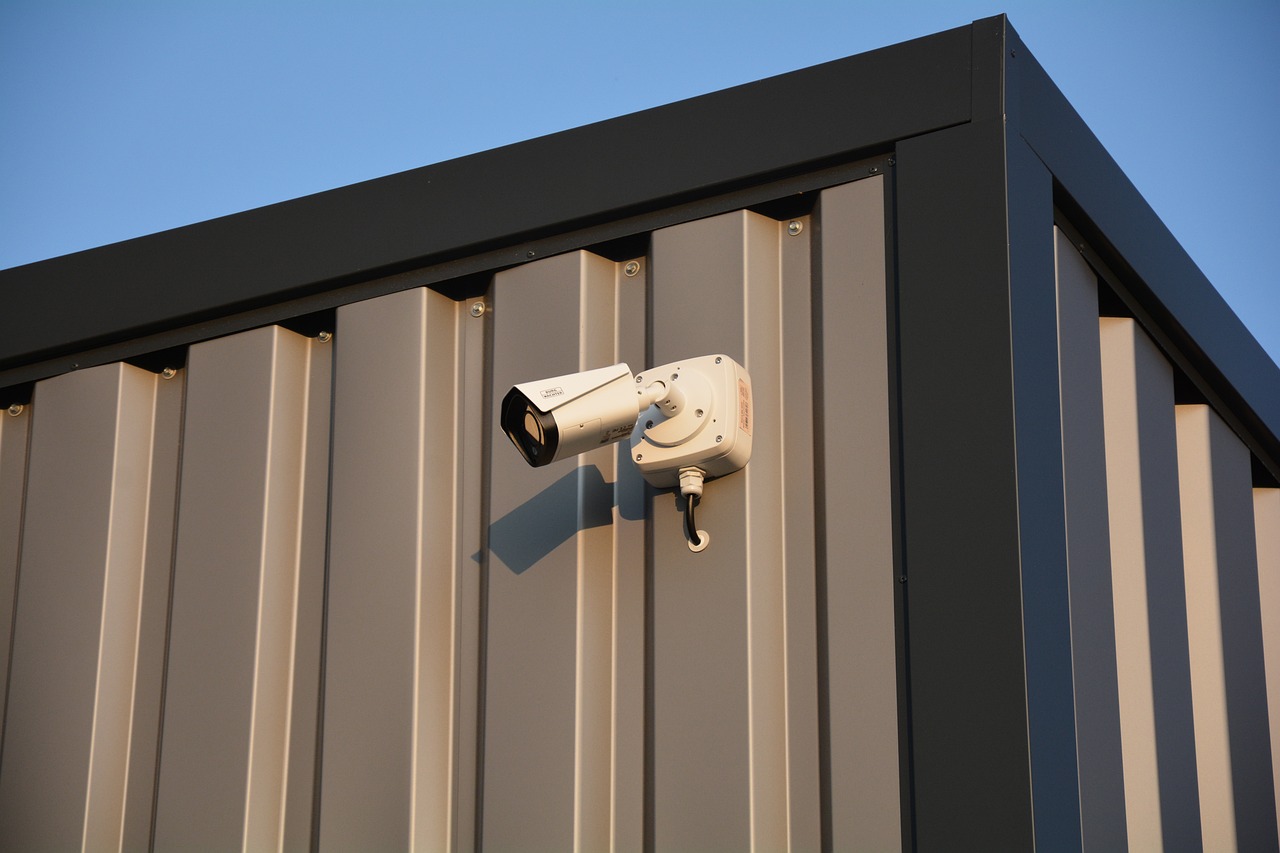
Privacy Concerns in AI
In today’s digital age, where artificial intelligence (AI) is becoming increasingly integrated into our daily lives, privacy concerns are at the forefront of discussions about technology. The ability of AI systems to process vast amounts of personal data raises critical questions about how this information is collected, stored, and utilized. Are we sacrificing our privacy for the sake of convenience? This is a question that many users grapple with as they navigate the digital landscape.
AI technologies often rely on data collection to function effectively, but this can lead to significant privacy implications. For instance, when we use voice assistants, smart devices, or even social media platforms, we unknowingly share sensitive information that can be analyzed and used by algorithms. The challenge lies in balancing the benefits of AI with the need to protect individual rights. As such, organizations must implement robust data protection measures to ensure that user information is handled responsibly.
One of the most pressing issues is the need for user consent. Users should have a clear understanding of what data is being collected and how it will be used. This means organizations must prioritize transparency and actively communicate their data practices. When users are informed and can make choices regarding their data, they feel more empowered and secure in their interactions with AI systems. This is where the concept of informed consent becomes crucial, as it respects users' autonomy and builds trust.
Moreover, organizations should consider implementing the following data protection strategies:
- Data Minimization: Collect only the data that is necessary for the intended purpose.
- Anonymization: Remove personally identifiable information from datasets to protect user identities.
- Access Controls: Limit access to sensitive data to only those who need it.
- Regular Audits: Conduct audits to ensure compliance with privacy policies and regulations.
As we delve deeper into the implications of AI on privacy, it’s essential to recognize that the responsibility doesn’t solely lie with organizations. Users also play a vital role in safeguarding their privacy. By being aware of their data rights and actively managing their digital footprints, individuals can take steps to protect themselves. This includes reviewing privacy settings on applications, opting out of data sharing where possible, and being cautious about the information they share online.
In conclusion, while AI offers remarkable advancements, it is crucial to address the associated privacy concerns. By fostering a culture of transparency and accountability, we can ensure that AI technologies develop in a way that respects individual rights and promotes societal benefit. As we move forward, the conversation around privacy in AI must continue, engaging both developers and users in a collaborative effort to create a safer digital environment.
- What are the main privacy concerns associated with AI? Privacy concerns include data collection without consent, misuse of personal information, and potential data breaches.
- How can organizations protect user privacy in AI? Organizations can implement data protection measures such as data minimization, anonymization, and regular audits.
- Why is user consent important in AI? User consent is crucial because it empowers individuals to control their personal information and builds trust in AI technologies.
- What can users do to protect their privacy? Users can review privacy settings, opt-out of data sharing, and be cautious about the information they share online.
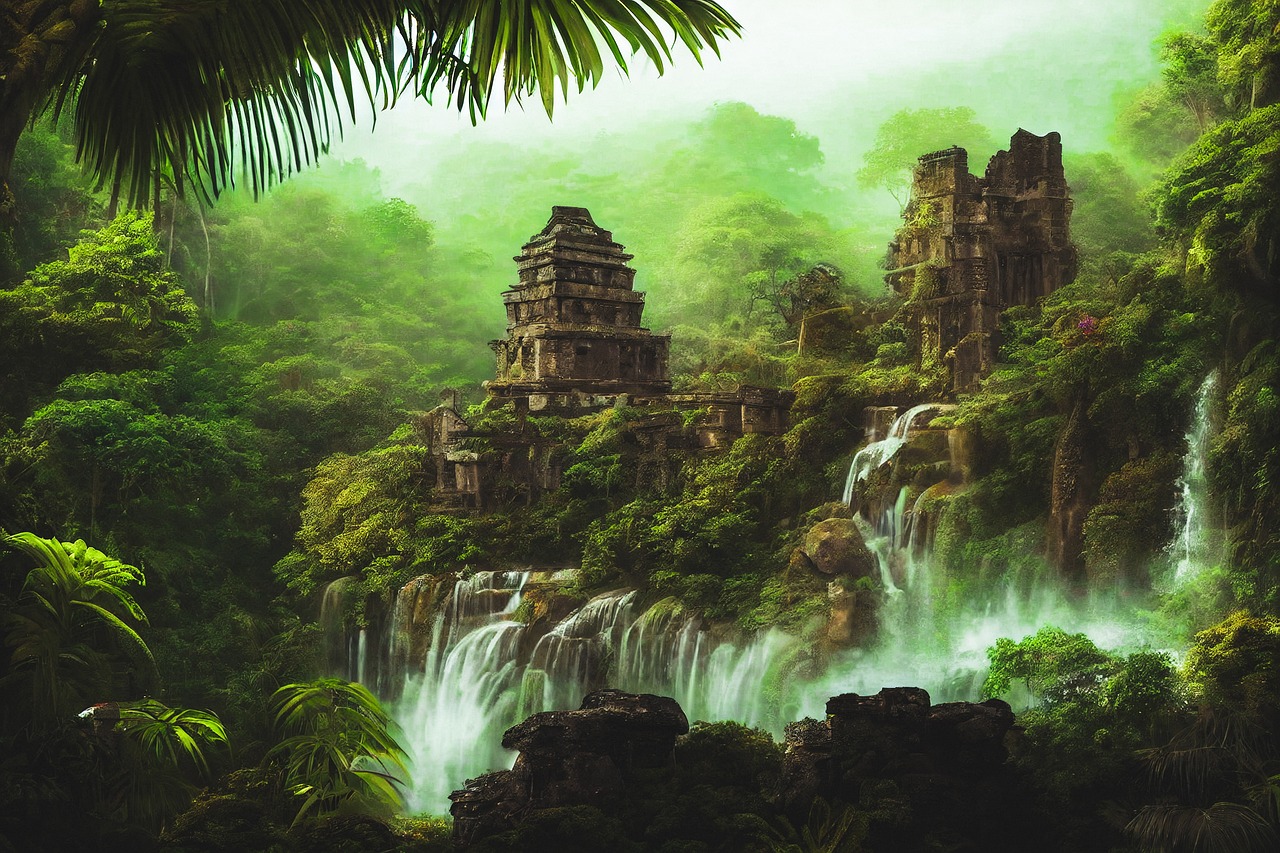
Data Protection Measures
In the rapidly evolving world of artificial intelligence, data protection has emerged as a cornerstone of ethical practice. As AI systems increasingly rely on vast amounts of personal data, ensuring that this information is handled responsibly is not just a regulatory requirement but a moral imperative. Organizations must implement robust measures to protect user data from unauthorized access, breaches, and misuse. This involves not only technical safeguards but also a commitment to ethical principles that prioritize user privacy.
One effective strategy for data protection is the implementation of encryption techniques. By encrypting sensitive data both in transit and at rest, organizations can significantly reduce the risk of data breaches. Encryption acts as a shield, making it nearly impossible for unauthorized parties to decipher the information, even if they manage to gain access to it. Additionally, organizations should adopt strict access controls, ensuring that only authorized personnel have access to sensitive data. This not only minimizes the risk of internal breaches but also fosters a culture of accountability within the organization.
Moreover, regular security audits are essential in identifying vulnerabilities within data systems. By conducting these audits, organizations can proactively address potential threats and reinforce their data protection strategies. It’s also crucial for organizations to stay updated with the latest cybersecurity trends and technologies, as cyber threats are constantly evolving. Investing in advanced security solutions, such as intrusion detection systems and firewalls, can provide an additional layer of defense against malicious attacks.
Another critical aspect of data protection is the principle of data minimization. Organizations should collect only the data that is absolutely necessary for their operations. This not only reduces the amount of sensitive information at risk but also aligns with the ethical principle of respecting user privacy. By minimizing data collection, organizations can enhance their reputation and build trust with their users.
Furthermore, organizations must prioritize user consent and transparency regarding data usage. Users should be informed about how their data will be collected, stored, and utilized. This transparency fosters trust and empowers users to make informed decisions about their data. Organizations can implement user-friendly consent management systems that allow individuals to easily opt-in or opt-out of data collection practices.
To summarize, effective data protection measures are vital for ethical AI practices. By incorporating encryption, conducting security audits, practicing data minimization, and ensuring transparency with users, organizations can create a safer digital environment. As technology continues to advance, maintaining a strong focus on data protection will not only safeguard individual rights but also enhance the overall integrity of AI systems.
- What are the main data protection measures organizations should implement?
Organizations should focus on encryption, access controls, security audits, data minimization, and transparency regarding data usage. - How does encryption protect user data?
Encryption makes data unreadable to unauthorized users, ensuring that even if data is intercepted, it cannot be accessed without the proper decryption key. - Why is user consent important in AI?
User consent is crucial as it respects individual autonomy and builds trust between users and organizations regarding how their data is used. - What is data minimization?
Data minimization is the practice of collecting only the data that is necessary for a specific purpose, reducing the risk of data breaches.
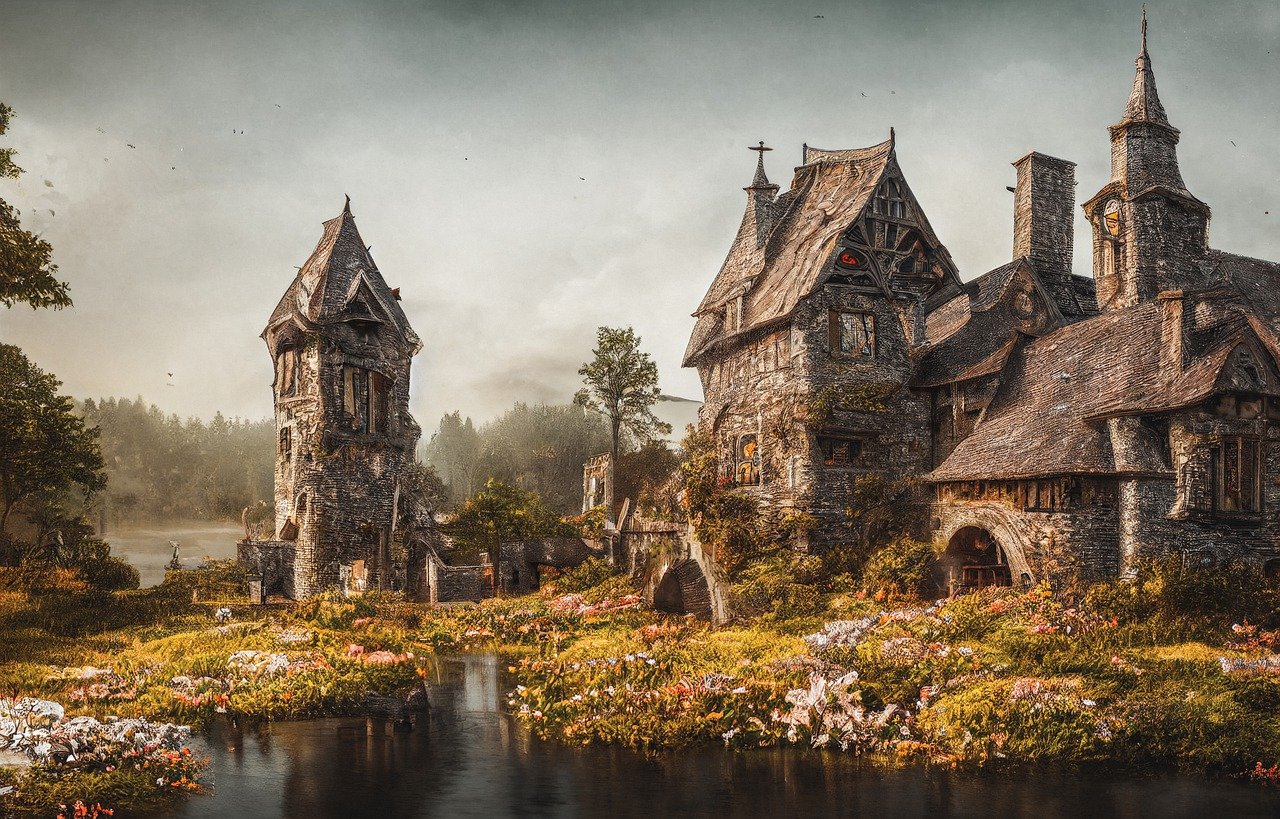
User Consent and Autonomy
In the rapidly evolving landscape of artificial intelligence, the concept of user consent and autonomy has emerged as a critical focal point. As AI systems become increasingly integrated into our daily lives, understanding how these technologies interact with personal data is essential. User consent is not merely a checkbox to be ticked; it represents a fundamental right that empowers individuals to control their own information. Imagine handing over your house keys to a stranger without knowing their intentions—this is akin to what happens when users unknowingly share their data with AI systems.
Moreover, autonomy in this context means that users should have the ability to make informed choices about how their data is used. This necessitates a clear understanding of what data is being collected, how it will be used, and who will have access to it. To achieve this, organizations must adopt transparent practices that prioritize user education. Providing concise and comprehensible information about data practices can significantly enhance user trust in AI technologies.
For instance, consider the following key points regarding user consent:
- Informed Consent: Users should be fully aware of what they are consenting to. This includes understanding the specific data being collected and its intended use.
- Revocation of Consent: Users should have the right to withdraw their consent at any time, ensuring they maintain control over their personal information.
- Granular Consent: Rather than a blanket agreement, users should be able to provide consent for specific uses of their data, allowing for a more tailored approach to privacy.
To illustrate the importance of user consent and autonomy, let’s take a brief look at how various industries handle these concepts:
Industry | Data Usage Practices | User Consent Mechanisms |
---|---|---|
Healthcare | Patient data for research and treatment | Explicit consent required for data sharing |
Finance | Transaction data for fraud detection | Opt-in consent for marketing purposes |
Social Media | User-generated content and interactions | Default settings often require users to opt-out |
As we can see, the approach to user consent varies significantly across industries. The healthcare sector tends to prioritize explicit consent, recognizing the sensitivity of personal health information. On the other hand, social media platforms often adopt an opt-out model, which can lead to users unwittingly sharing more data than they intended. This disparity highlights the necessity for a more standardized approach to user consent across all sectors.
Ultimately, fostering a culture of consent and respect for autonomy is not just a legal obligation but a moral imperative. By prioritizing user rights and ensuring that consent is informed and respected, organizations can build stronger, more trusting relationships with their users. This, in turn, can lead to a more ethical and responsible implementation of AI technologies, benefiting both society and the individuals who interact with these systems.
Q1: Why is user consent important in AI?
User consent is crucial because it empowers individuals to control their personal information and ensures that organizations use data responsibly and ethically.
Q2: How can organizations ensure informed consent?
Organizations can ensure informed consent by providing clear, concise information about data collection and usage, allowing users to make educated decisions.
Q3: Can users revoke their consent once given?
Yes, users should always have the right to revoke their consent at any time, allowing them to maintain control over their data.
Q4: What does autonomy mean in the context of AI?
Autonomy refers to the ability of users to make informed choices about how their data is used, ensuring they are not manipulated or coerced into sharing their information.
Frequently Asked Questions
- What are the main ethical considerations in AI development?
The main ethical considerations in AI development include fairness, transparency, accountability, and privacy. These principles are essential to ensure that AI technologies are developed responsibly and serve the public good, minimizing risks associated with bias and misuse.
- How can bias affect AI algorithms?
Bias can seep into AI algorithms through skewed training data, leading to unfair outcomes. For instance, if an AI system is trained on data that lacks diversity, it might produce results that favor one group over another, perpetuating inequality. Identifying and addressing this bias is crucial for fairness.
- What steps can be taken to mitigate algorithmic bias?
To mitigate algorithmic bias, organizations can implement regular audits of their AI systems, use diverse and representative datasets, and promote algorithmic transparency. These strategies help ensure that AI technologies make equitable decisions and foster public trust.
- Why is transparency important in AI systems?
Transparency is vital in AI systems because it allows users to understand how decisions are made. Explainable AI fosters trust and helps users feel more comfortable with technology, ensuring they know what data is being used and how it impacts outcomes.
- What is the role of accountability in AI development?
Accountability ensures that developers and organizations are responsible for the societal impacts of their AI technologies. Establishing clear accountability frameworks helps hold parties responsible for any negative consequences that arise from AI applications.
- How do regulations shape ethical AI practices?
Regulations play a crucial role in shaping ethical AI practices by setting standards that organizations must adhere to. Compliance with these regulations helps protect public interest and ensures that AI technologies are developed and deployed responsibly.
- What are effective data protection measures for AI?
Effective data protection measures include implementing encryption, access controls, and regular security audits. Organizations should also ensure that personal data is collected and processed in compliance with privacy laws to safeguard user information.
- Why is user consent important in AI interactions?
User consent is important because it respects individuals' autonomy and rights regarding their personal data. Obtaining informed consent ensures that users are aware of how their data will be used, fostering trust and encouraging responsible AI interactions.