Debating the Moral Dimensions of AI
As we stand on the brink of a technological revolution, the rise of artificial intelligence (AI) is both exhilarating and daunting. The rapid development of AI technologies has sparked a heated debate about their moral implications and how they will shape our society. Are we ready to embrace the changes that AI brings, or do we need to take a step back and consider the ethical ramifications? This article delves into the complexities of AI, exploring the responsibilities of developers and users in crafting a future that prioritizes ethical considerations.
When we talk about the ethics of AI, it’s essential to recognize that there are multiple frameworks through which we can analyze these technologies. Three of the most significant frameworks are consequentialism, deontology, and virtue ethics. Each of these perspectives offers unique insights into how AI should be developed and utilized.
Consequentialism focuses on the outcomes of actions. In the context of AI, this means evaluating whether the benefits of AI technologies outweigh the potential harms. On the other hand, deontology emphasizes the importance of adhering to rules and duties. This perspective argues that certain actions, like infringing on privacy or perpetuating bias, are inherently wrong, regardless of the outcomes. Lastly, virtue ethics stresses the character and intentions behind actions. It asks us to consider what kind of society we want to foster and how AI contributes to that vision.
One of the most pressing ethical issues surrounding AI is the presence of bias in algorithms. AI systems are trained on data that may reflect existing societal biases, leading to outcomes that can reinforce inequality and discrimination. It’s crucial to understand how bias manifests in AI, as developers hold a moral responsibility to address these issues before they escalate.
Bias in AI can take many forms. Here are a few critical types:
- Racial Bias: Algorithms trained on biased data may lead to unfair treatment of individuals based on race.
- Gender Bias: AI can perpetuate stereotypes that disadvantage specific genders, particularly women.
- Socioeconomic Bias: Systems may discriminate against individuals from lower socioeconomic backgrounds, further entrenching inequality.
Each of these biases has real-world implications, affecting everything from hiring practices to law enforcement. For instance, if an AI system used in hiring is trained on data that favors male candidates, it may continue to overlook qualified female applicants, perpetuating gender inequality in the workplace.
The consequences of deploying biased AI systems can be dire. In sectors like healthcare, biased algorithms can result in unequal treatment, where certain demographics receive subpar care. In the criminal justice system, biased predictive policing tools can lead to over-policing in minority neighborhoods, exacerbating existing tensions and mistrust. These outcomes highlight the urgent need for developers to scrutinize their algorithms and the data they rely on.
Addressing bias in AI is not just a moral obligation; it’s essential for creating fair and effective systems. Here are some strategies that can help:
- Diverse Data Collection: Actively seek out diverse datasets to train AI models, ensuring representation across various demographics.
- Algorithmic Audits: Regularly review and test algorithms for bias, making necessary adjustments to mitigate any identified issues.
By implementing these strategies, developers can take significant steps toward creating more equitable AI systems that serve all members of society.
As AI continues to evolve, the question of accountability becomes increasingly complex. Who is responsible when an AI system makes a harmful decision? Is it the developer, the user, or the organization that deployed the AI? These questions highlight the need for clear ethical guidelines and frameworks that delineate the responsibilities of all parties involved in AI development and usage.
The integration of AI into our daily lives also raises significant privacy concerns. With AI technologies capable of processing vast amounts of personal data, the potential for privacy infringements is alarming. It's essential to investigate how AI can infringe upon personal privacy and the ethical implications of such actions.
Understanding the data collection practices employed by AI systems is crucial for maintaining ethical standards. Many AI systems rely on user data to function effectively, but this raises ethical considerations surrounding consent and transparency. Users should be informed about how their data is being used and have the option to opt-out, ensuring that their privacy is respected.
The development of regulatory frameworks is vital for the ethical deployment of AI technologies. Existing regulations, such as the General Data Protection Regulation (GDPR) in Europe, set a precedent for how organizations should handle data. However, more comprehensive policies are needed to address the unique challenges posed by AI. Future regulations should focus on safeguarding ethical standards, ensuring accountability, and protecting individuals' rights in an increasingly automated world.
Q: What are the most significant ethical concerns regarding AI?
A: The most significant concerns include bias in algorithms, privacy infringements, and accountability for AI decisions.
Q: How can developers ensure their AI systems are ethical?
A: Developers can implement strategies such as diverse data collection and regular algorithmic audits to reduce bias and uphold ethical standards.
Q: What role do users play in the ethical use of AI?
A: Users can advocate for transparency and responsible data usage, ensuring that their rights are respected in AI applications.
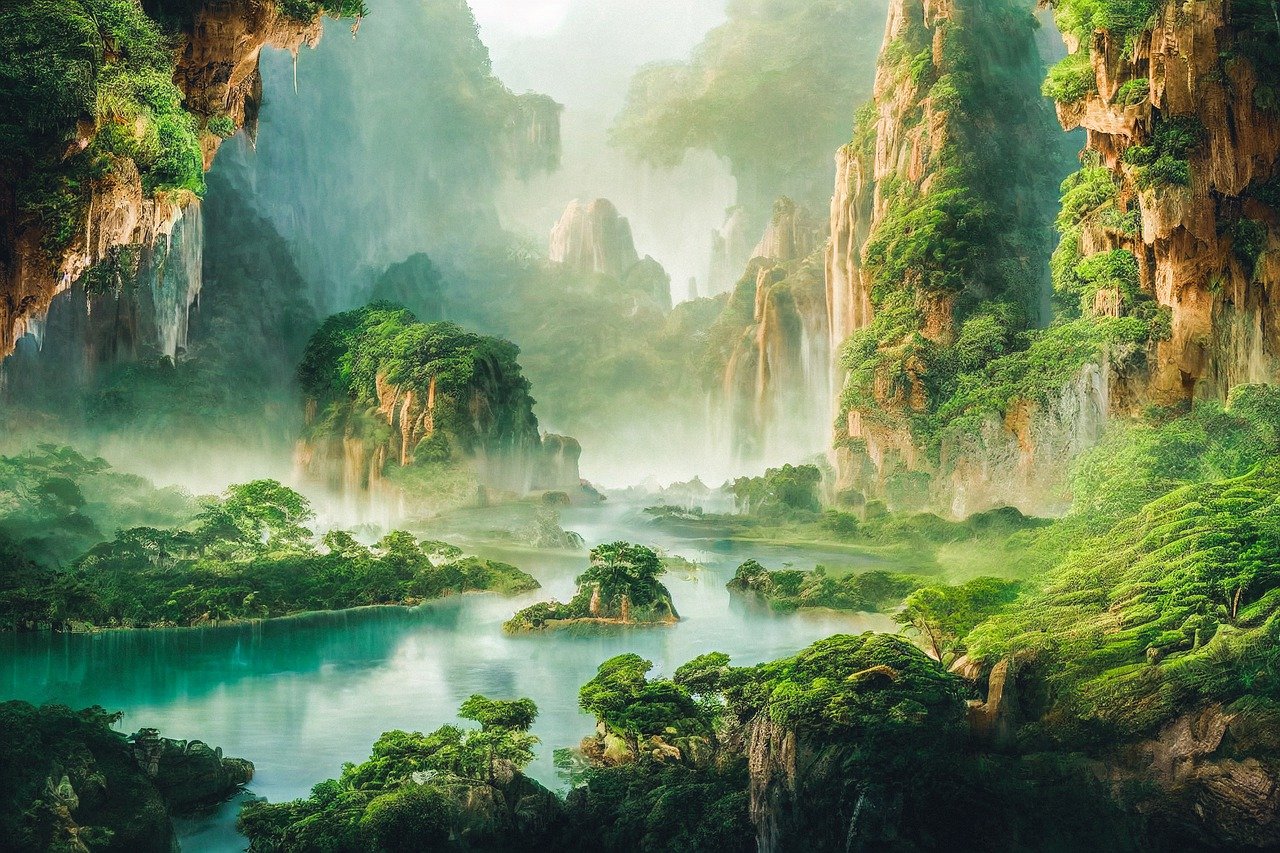
The Ethical Frameworks of AI
When we dive into the world of artificial intelligence (AI), we quickly realize that it's not just about algorithms and data; it’s also about the ethical frameworks that guide its development and implementation. Imagine AI as a powerful tool, much like a double-edged sword—it can bring about tremendous benefits or cause significant harm, depending on how we wield it. To navigate this complex landscape, we need to explore various ethical perspectives that inform our decisions regarding AI.
One of the most prominent ethical frameworks is consequentialism, which posits that the morality of an action is determined by its outcomes. In the context of AI, this means evaluating the potential consequences of deploying an AI system. For example, if an AI algorithm improves healthcare outcomes for thousands of patients but also leads to job losses in the medical field, how do we weigh these outcomes? The challenge lies in predicting these consequences accurately and ensuring that the benefits outweigh any potential harms.
On the other hand, we have deontology, which focuses on the morality of actions themselves rather than their consequences. This framework emphasizes duties and rules. For instance, if an AI system is designed to make hiring decisions, a deontological perspective would stress the importance of fairness and transparency in the decision-making process, regardless of the outcomes. Developers must adhere to ethical guidelines that prioritize human rights and dignity, ensuring that AI systems do not violate fundamental principles.
Then, there’s virtue ethics, which encourages us to consider the character and intentions of the developers and users of AI. This perspective asks questions like: What kind of society do we want to create with AI? Are we fostering innovation that aligns with our values? Virtue ethics emphasizes the need for individuals and organizations to cultivate virtues such as honesty, integrity, and responsibility in their AI-related endeavors. In essence, it’s about creating a culture of ethical awareness and accountability within the AI community.
Understanding these ethical frameworks is crucial as we develop and deploy AI technologies. Each framework offers valuable insights that can help us navigate the moral dilemmas we face. By combining these perspectives, we can create a more holistic approach to AI ethics, ensuring that our innovations serve humanity positively. As we move forward, it’s essential for developers, users, and policymakers to engage in ongoing dialogue about these ethical considerations, fostering a shared understanding of the responsibilities that come with creating intelligent systems.
In summary, the ethical frameworks of AI—consequentialism, deontology, and virtue ethics—provide us with essential tools to evaluate our choices in the realm of artificial intelligence. By considering the outcomes of our actions, adhering to ethical principles, and cultivating virtuous practices, we can strive to shape a future where AI benefits everyone. So, as we stand at the crossroads of technology and morality, let’s ask ourselves: How can we ensure that our AI systems reflect the best of our human values?
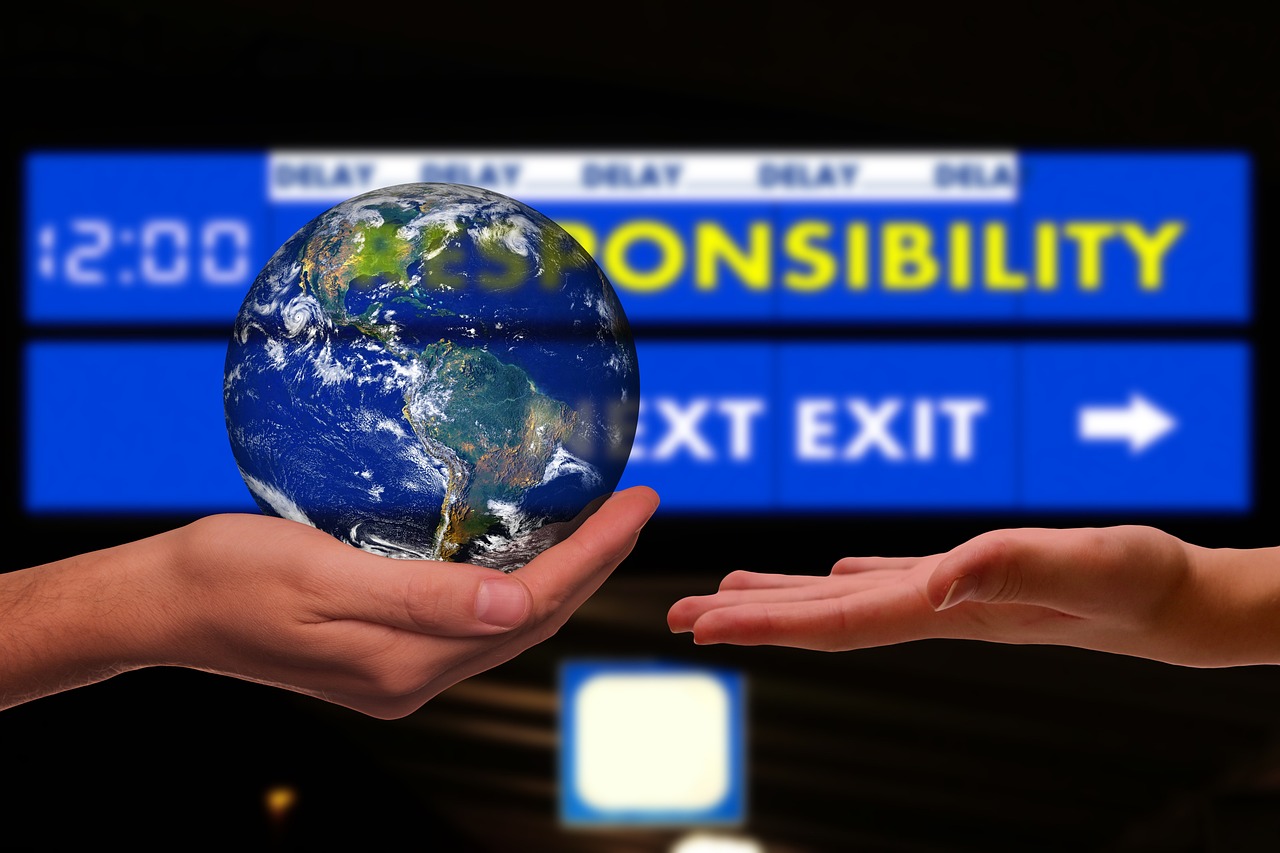
The Role of Bias in AI
Artificial Intelligence (AI) is rapidly becoming an integral part of our lives, but with its rise comes a significant concern: bias. Bias in AI can be likened to a double-edged sword; while it has the potential to streamline processes and enhance decision-making, it can also perpetuate and even amplify existing inequalities in society. The algorithms that power AI systems are trained on vast datasets, which often reflect the biases present in our world. This means that if the data is flawed, the AI's decisions can be flawed too. But how does this bias manifest, and what are the implications for society?
To truly grasp the role of bias in AI, we need to understand that it can seep into various stages of AI development. From data collection to algorithm design, bias can infiltrate every aspect. For instance, if an AI system is trained on historical hiring data from a company that has predominantly hired male candidates, it may learn to favor male applicants over equally qualified females. This not only impacts job opportunities but also reinforces gender stereotypes in the workforce.
Furthermore, bias in AI isn't just a theoretical concern; it has real-world consequences that can affect millions. For example, biased algorithms in criminal justice systems can lead to disproportionate sentencing for minority groups, while biased healthcare algorithms might overlook critical health indicators for certain demographics. The stakes are high, and as AI continues to evolve, so too does the urgency to address these biases.
Understanding the different types of bias is crucial to mitigating their effects. Here are some common types:
- Racial Bias: This occurs when algorithms favor one racial group over another, often due to historical data that reflects systemic inequalities.
- Gender Bias: Similar to racial bias, this type can arise when AI systems are trained on data that predominantly features one gender, leading to skewed outcomes.
- Socioeconomic Bias: AI can inadvertently discriminate against individuals from lower socioeconomic backgrounds if the training data lacks representation from these groups.
As developers and organizations recognize the potential for bias, it becomes imperative to implement strategies to mitigate these issues. This includes diversifying training datasets, conducting regular algorithmic audits, and involving a diverse group of stakeholders in the AI development process. By being proactive, we can create AI systems that are not only effective but also fair.
In summary, the role of bias in AI is complex and multifaceted. It can have profound implications for society, affecting everything from hiring practices to criminal justice outcomes. As we continue to navigate the evolving landscape of AI, it is crucial to remain vigilant and committed to addressing these biases head-on. By doing so, we can harness the power of AI while ensuring that it serves as a tool for equality rather than a perpetuator of injustice.
- What is bias in AI? Bias in AI refers to the tendency of algorithms to produce unfair outcomes due to flawed training data or design.
- How can bias in AI be mitigated? Bias can be mitigated through diverse data collection, algorithmic audits, and involving diverse stakeholders in the development process.
- Why is addressing bias in AI important? Addressing bias is crucial to ensure fairness and equality in AI decision-making, preventing discrimination in various sectors.
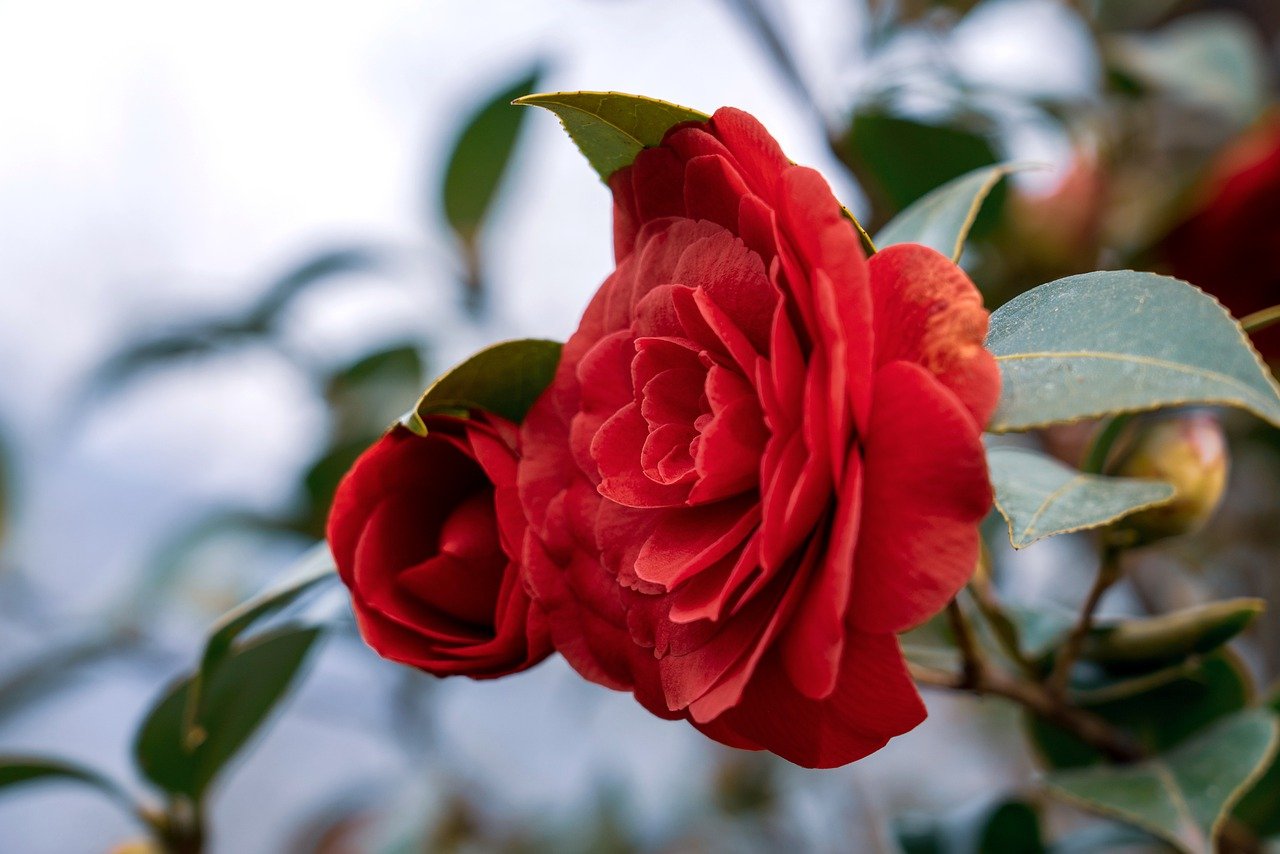
Types of Bias in AI
The world of artificial intelligence is as fascinating as it is complex, especially when we dive into the murky waters of bias. Just like a prism that refracts light into various colors, AI can reflect the biases inherent in the data used to train it. Understanding the different types of bias is crucial for developers and users alike, as it enables us to recognize the potential pitfalls and take proactive steps to mitigate them. Bias in AI can manifest in several forms, including racial bias, gender bias, and societal bias. Each type can have serious implications, affecting everything from hiring practices to law enforcement. Let’s take a closer look at these biases and their impact.
Racial Bias is perhaps one of the most discussed forms of bias in AI. For instance, facial recognition systems have shown a troubling tendency to misidentify individuals from minority groups at a significantly higher rate than their white counterparts. This can lead to wrongful accusations or even arrests, creating a ripple effect of injustice. A well-known example is the 2018 study by MIT Media Lab, which found that commercial facial recognition systems misclassified darker-skinned women 34% of the time, compared to just 1% for lighter-skinned men. This stark disparity raises serious questions about the ethical implications of deploying such technologies without proper scrutiny.
Gender Bias also plays a major role in shaping AI outcomes. Consider how voice recognition systems often perform poorly with female voices compared to male voices. This can be traced back to the training data, which may predominantly feature male voices. As a result, women may find themselves frustrated when their devices fail to understand their commands. This not only affects user experience but can also perpetuate stereotypes about women being less authoritative or less capable of being understood in professional settings.
Socioeconomic Bias is another critical aspect to consider. AI systems trained on data from affluent neighborhoods may not perform well in lower-income areas. For example, predictive policing algorithms that rely on historical crime data may unfairly target communities that are already over-policed, further entrenching cycles of discrimination and inequality. This type of bias can lead to a skewed understanding of crime and safety, which can have devastating effects on marginalized communities.
In summary, recognizing the various types of bias in AI is not just an academic exercise; it's a moral imperative. By understanding how these biases manifest, we can work towards creating more equitable AI systems. Addressing these issues requires a multi-faceted approach, including diverse data collection, transparent algorithms, and a commitment to ongoing evaluation and improvement. Only through these efforts can we hope to harness the true potential of AI while minimizing its risks.
- What is bias in AI? Bias in AI refers to the systematic favoritism or discrimination that arises from the data used to train AI systems, leading to unfair outcomes.
- How can bias in AI be mitigated? Bias can be mitigated through diverse data collection, regular audits of algorithms, and implementing ethical guidelines during development.
- Why is it important to address bias in AI? Addressing bias is crucial to ensure fairness, accountability, and transparency in AI applications, preventing discrimination and promoting equality.
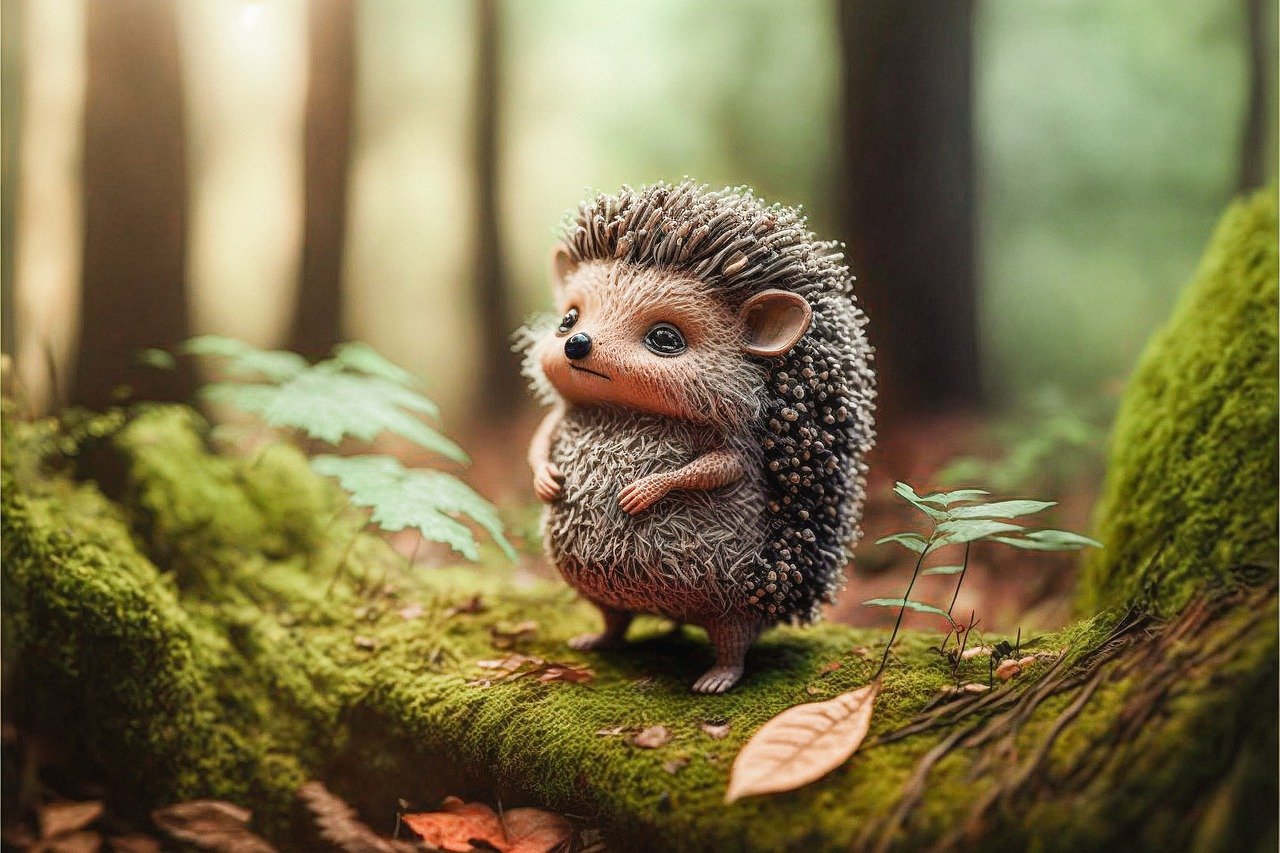
Consequences of Biased AI
When we think about the implications of biased AI, it’s almost like peering into a funhouse mirror—what we see can often be distorted, unfair, and downright alarming. The consequences of deploying biased algorithms can ripple through society, affecting everything from hiring practices to law enforcement. Imagine a world where job applicants are overlooked not because of their qualifications, but due to the biases embedded in the AI systems assessing them. This is not just a hypothetical scenario; it’s a reality that many individuals face today.
One of the most troubling aspects of biased AI is its potential to perpetuate systemic inequalities. For instance, in the criminal justice system, algorithms used to predict recidivism have been shown to disproportionately target individuals from minority backgrounds. This can lead to harsher sentencing and increased surveillance, effectively entrenching existing social disparities. The consequences here are not just theoretical; they manifest in real lives, affecting families and communities.
Moreover, biased AI can lead to significant economic repercussions. Businesses that rely on these flawed systems may find themselves making poor decisions that can cost them dearly. For example, if an AI system used in recruitment favors candidates from certain demographics over others, companies could miss out on diverse talent that brings fresh perspectives and innovation. This not only stifles creativity but also limits market competitiveness.
In healthcare, biased algorithms can have dire consequences. If AI systems are trained on data that is not representative of the entire population, they may fail to provide accurate diagnoses or treatment recommendations for underrepresented groups. This can lead to inadequate care and worsen health disparities. A study by the National Institute of Health found that algorithms used in healthcare disproportionately favored white patients, raising serious ethical concerns about fairness and justice in medical treatment.
To illustrate the potential fallout from biased AI, consider the following table:
Sector | Consequences of Bias |
---|---|
Employment | Job discrimination based on biased hiring algorithms. |
Criminal Justice | Increased incarceration rates for minorities due to biased risk assessment tools. |
Healthcare | Inaccurate treatment recommendations leading to worsening health outcomes. |
Finance | Unfair loan approvals or denials based on biased credit scoring models. |
As we navigate this complex landscape, it’s crucial to recognize that the consequences of biased AI are not just technical failures; they are moral failures that affect lives. Developers and organizations must take a proactive approach to identify and rectify biases within their systems. This involves ongoing audits, diverse data sets, and a commitment to ethical standards that prioritize fairness and equality.
In conclusion, the consequences of biased AI are profound and far-reaching. They challenge our values and demand that we take responsibility for the technologies we create and deploy. If we fail to address these issues, we risk building a future where technology amplifies existing injustices rather than alleviating them.
- What is biased AI? Biased AI refers to artificial intelligence systems that produce unfair or prejudiced outcomes due to flawed training data or algorithm design.
- How can we mitigate bias in AI? Strategies include using diverse data sets, conducting regular algorithmic audits, and involving a diverse group of stakeholders in the development process.
- What are the real-world impacts of biased AI? Biased AI can lead to discrimination in hiring, unfair treatment in healthcare, and unjust legal outcomes, among other societal issues.
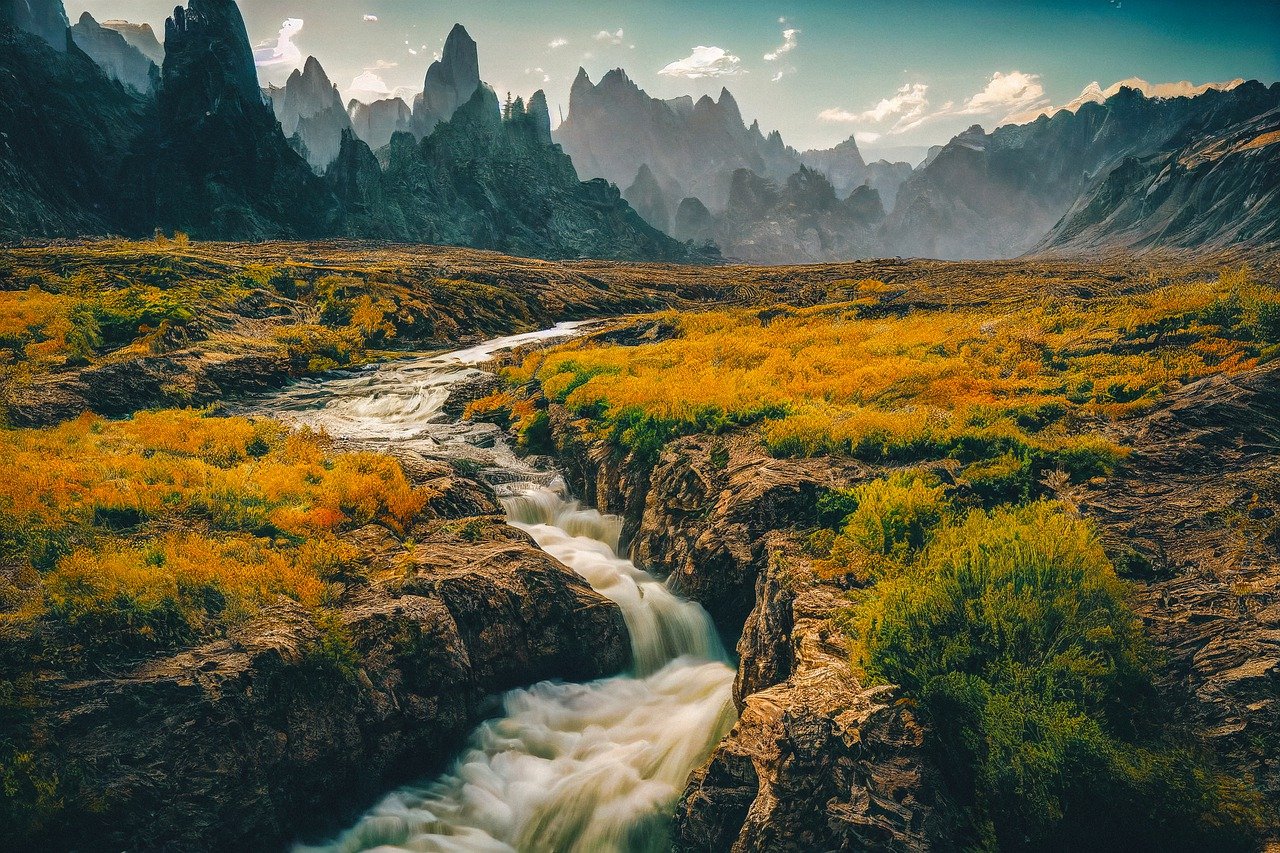
Strategies to Mitigate Bias
Mitigating bias in artificial intelligence is not just a technical challenge; it's a moral imperative that demands our attention. As we delve into the strategies to address bias, we must recognize that the journey towards equitable AI is multifaceted. One of the most effective approaches is to ensure diverse data collection. By including a wide range of demographics in the training datasets, we can better reflect the complexities of the real world. This means actively seeking out data from underrepresented groups to create a more balanced perspective.
Another crucial strategy is conducting algorithmic audits. These audits serve as a check-up for AI systems, allowing developers to identify and rectify biases that may have slipped through the cracks during the initial development stages. Regular assessments can help maintain the integrity of AI applications and ensure they operate fairly across different populations. Additionally, implementing techniques such as adversarial debiasing can help in training models that are less sensitive to biased inputs, making them more robust and equitable.
Moreover, fostering a culture of awareness and education among developers and stakeholders is essential. Workshops and training sessions focused on recognizing and addressing bias can empower teams to make informed decisions throughout the AI lifecycle. It's not enough to just build AI; we must also build a community that values ethical considerations and holds itself accountable for the outcomes of its technologies.
To further illustrate these strategies, consider the following table that summarizes key approaches and their benefits:
Strategy | Description | Benefits |
---|---|---|
Diverse Data Collection | Gathering data from a wide range of demographics | Reduces representation bias, enhances fairness |
Algorithmic Audits | Regular reviews of AI systems for bias detection | Ensures ongoing fairness, identifies issues early |
Adversarial Debiasing | Training models to minimize bias sensitivity | Creates more robust AI systems, improves equity |
Awareness and Education | Training developers on bias recognition | Empowers ethical decision-making, promotes accountability |
In conclusion, the fight against bias in AI requires a comprehensive approach that combines technical solutions with a commitment to ethical practices. By implementing these strategies, we can work towards creating AI systems that not only function effectively but also uphold the values of fairness and justice in our society.
- What is the most effective way to mitigate bias in AI?
There is no one-size-fits-all solution, but strategies such as diverse data collection, algorithmic audits, and adversarial debiasing are among the most effective.
- How can organizations ensure their AI systems are fair?
Organizations can implement regular audits, involve diverse teams in the development process, and prioritize transparency in their data collection practices.
- Why is bias in AI a moral issue?
Bias in AI can lead to unfair treatment of individuals and groups, perpetuating existing inequalities in society. Addressing it is crucial for ethical AI deployment.

Accountability and Responsibility
When it comes to artificial intelligence, the question of accountability is as murky as a foggy morning. Who is responsible when an AI system makes a mistake? Is it the developers who created the algorithm, the users who deployed it, or the organizations that benefit from its outcomes? This intricate web of responsibility is crucial to untangle, especially as AI becomes more embedded in our daily lives. After all, when a self-driving car gets into an accident, pointing fingers isn't as straightforward as it seems.
One of the primary challenges of AI accountability lies in the complexity of these systems. Unlike traditional software, AI algorithms learn from data, which means their decision-making processes can be opaque. This opacity raises significant ethical concerns. If an AI makes a biased decision, can we trace back the roots of that bias? Can developers be held accountable for the unintended consequences of their creations? These questions are essential for fostering a responsible AI ecosystem.
Moreover, the concept of moral responsibility extends beyond just developers. Users of AI technologies also carry a share of the responsibility. They must understand the tools they are using and the potential implications of their decisions. For instance, if a company uses an AI-powered hiring tool that inadvertently discriminates against candidates from certain backgrounds, the organization must evaluate its role in perpetuating that bias. This dual-layer of responsibility—developers and users—creates a more comprehensive framework for accountability.
To illustrate this point, consider the following table that outlines the different parties involved in AI accountability:
Party | Responsibilities |
---|---|
Developers | Design ethical algorithms, ensure transparency, and mitigate biases during the development process. |
Users | Understand AI capabilities and limitations, apply AI responsibly, and monitor outcomes. |
Organizations | Implement policies for ethical AI use, provide training for users, and take responsibility for AI outcomes. |
In addition to these responsibilities, there is a pressing need for regulatory frameworks that delineate accountability in AI usage. Governments and regulatory bodies must step in to establish clear guidelines that define who is responsible when AI systems cause harm. This could involve creating laws that hold organizations accountable for the outcomes of their AI systems, thereby pushing them to prioritize ethical considerations in their AI strategies.
Ultimately, accountability in AI is not just about assigning blame; it’s about fostering a culture of responsibility and ethical awareness among all stakeholders. By promoting transparency, encouraging open dialogue about the implications of AI, and ensuring that developers and users alike understand their roles, we can pave the way for a future where AI technologies are used ethically and responsibly.
- Who is responsible for biased AI decisions? - Both developers and users share responsibility. Developers must create ethical algorithms, while users must apply them wisely.
- What can organizations do to ensure AI accountability? - Organizations should implement ethical guidelines, conduct regular audits, and provide training to users on the responsible use of AI.
- Are there laws governing AI accountability? - While some regulations exist, the field is still developing, and more comprehensive laws are needed to address accountability in AI.
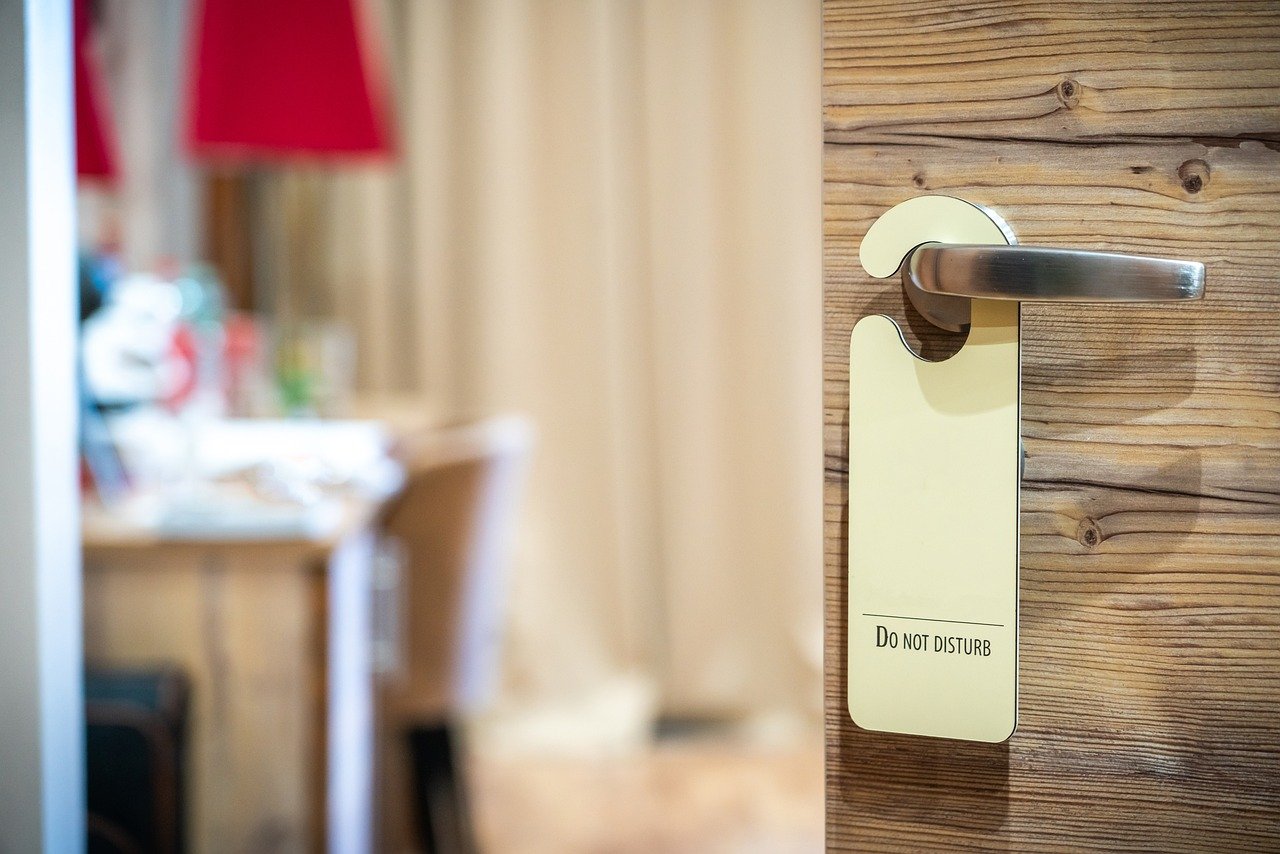
AI and Privacy Concerns
As artificial intelligence continues to weave itself into the fabric of our daily lives, the concerns surrounding privacy have surged to the forefront of public discourse. Imagine a world where your every move is monitored, analyzed, and stored by algorithms designed to predict your behavior. Sounds like a scene straight out of a dystopian novel, right? But the reality is that AI technologies, from smart assistants to facial recognition systems, are increasingly capable of infringing on our personal privacy in ways we might not fully comprehend.
At the heart of these privacy concerns is the way AI systems collect and utilize data. With the vast amounts of information generated daily, AI thrives on data—your preferences, habits, and even your location. This data is often harvested without explicit consent, raising ethical questions about ownership and transparency. For instance, when you interact with an AI-driven application, do you truly understand what data is being collected and how it will be used? The lack of clarity in data collection practices can lead to a significant erosion of trust between users and technology.
Moreover, the implications of AI on privacy extend beyond mere data collection. Consider the following scenarios:
- Surveillance: AI-powered surveillance systems can track individuals in real-time, leading to potential misuse by governments or corporations.
- Data Breaches: The more data AI systems accumulate, the more attractive they become to hackers, risking personal information exposure.
- Behavioral Targeting: AI can analyze your online behavior to create detailed profiles, which can be used for targeted advertising or even manipulation.
These scenarios underscore the urgent need for a robust regulatory framework that addresses the ethical implications of AI in relation to privacy. Currently, various countries are grappling with how to legislate AI technologies. For instance, the General Data Protection Regulation (GDPR) in Europe sets stringent rules on data protection and privacy, yet many argue that it may not be sufficient to keep pace with the rapid advancements in AI. The challenge lies not only in creating regulations but also in ensuring that they are enforced effectively and adapted to the evolving landscape of technology.
In light of these concerns, developers and organizations have a moral responsibility to prioritize user privacy. This can be achieved through practices such as:
- Transparency: Clearly informing users about what data is collected and how it will be used.
- Consent: Obtaining explicit permission from users before collecting their data.
- Data Minimization: Collecting only the data necessary for the intended purpose.
As we navigate this complex intersection of AI and privacy, it is crucial for users to stay informed and advocate for their rights. After all, in a world where data is the new currency, understanding your privacy rights is more important than ever. The conversation about AI and privacy should not be limited to experts in the field; it should include every individual who interacts with technology. Are we ready to take a stand for our privacy, or will we let the machines dictate the terms?
Q1: How does AI collect personal data?
AI systems often collect personal data through user interactions, online activities, and third-party data sources. This data is then used to improve AI algorithms and personalize user experiences.
Q2: What are the risks associated with AI and privacy?
The risks include unauthorized data collection, surveillance, data breaches, and behavioral targeting, which can compromise individual privacy and lead to misuse of personal information.
Q3: How can I protect my privacy when using AI technologies?
You can protect your privacy by being aware of the data collection practices of the AI systems you use, adjusting privacy settings, and opting out of data sharing whenever possible.
Q4: Are there regulations in place to protect my privacy?
Yes, regulations like the GDPR in Europe aim to protect user privacy, but the effectiveness of these regulations can vary by region and industry.
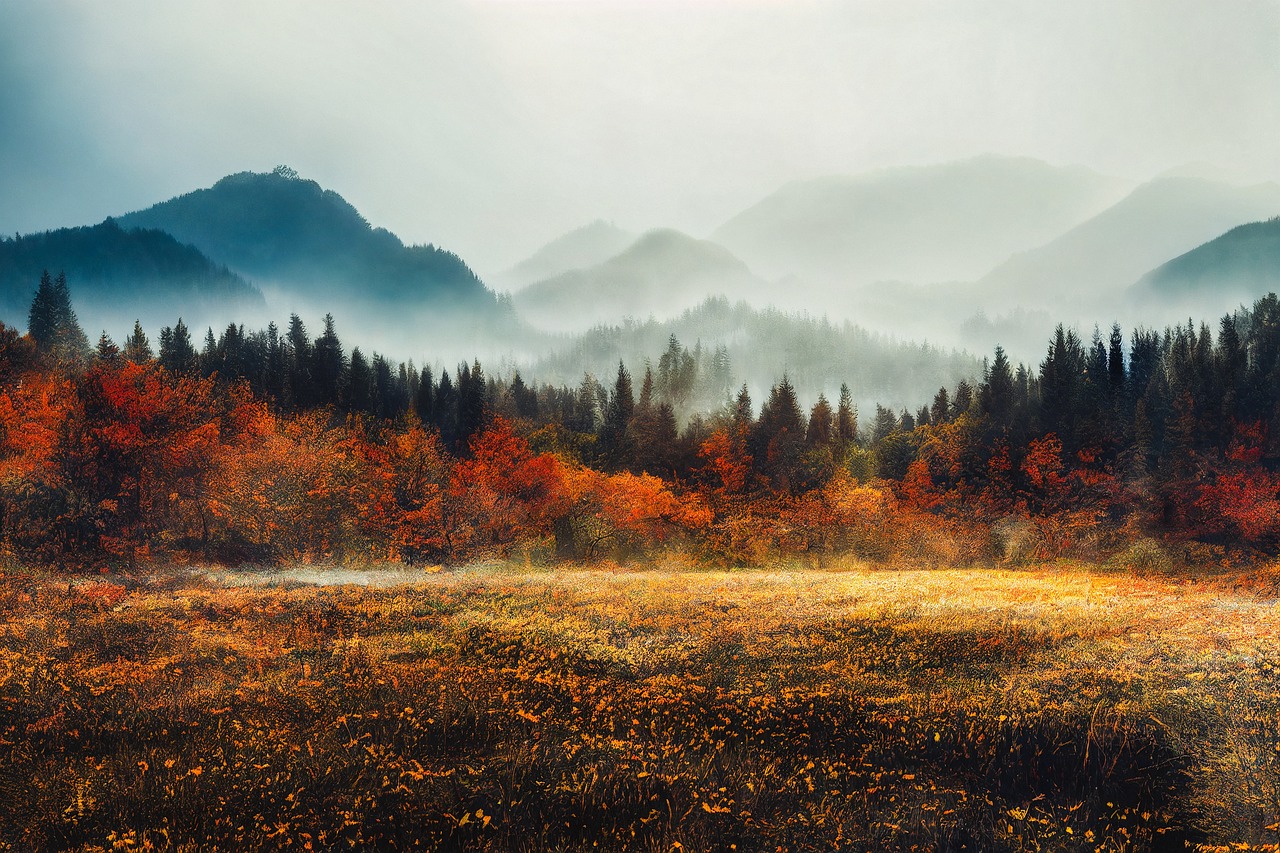
Data Collection Practices
In today's digital age, the collection of data has become a cornerstone of artificial intelligence (AI) development. AI systems thrive on data, using it to learn, adapt, and make decisions. However, the practices surrounding data collection raise significant ethical questions. Are users fully aware of what data is being collected? Do they understand how their information will be used? These questions are crucial, as the answers can determine the level of trust users place in AI technologies.
One of the most pressing issues in data collection is the concept of informed consent. In an ideal world, users would be fully informed about the data being collected, how it will be processed, and the implications of its use. Unfortunately, many companies use convoluted privacy policies that are difficult for the average person to understand. This lack of transparency can lead to a sense of betrayal when users discover that their data has been used in ways they never agreed to.
Moreover, the methods of data collection can vary widely. Some AI systems gather data through direct user interaction, such as through apps or websites where users voluntarily provide information. Others may employ more covert methods, like tracking user behavior online through cookies or monitoring social media activity. Understanding these practices is essential, as they can significantly impact user privacy and trust.
Here are some common data collection practices used by AI systems:
- Active Data Collection: This involves users providing information directly, such as filling out forms or surveys.
- Passive Data Collection: This method collects data without user intervention, often through tracking technologies like cookies or device sensors.
- Third-Party Data Sharing: Companies may share user data with third parties, raising concerns about how that data will be used.
As we navigate the complexities of AI and data collection, it's vital to consider the ethical implications of these practices. For instance, how do we ensure that data collection is equitable? Are certain groups being disproportionately affected by invasive data practices? These questions highlight the need for ethical guidelines that prioritize user privacy and consent.
Furthermore, regulatory frameworks are starting to emerge to address these issues. Laws like the General Data Protection Regulation (GDPR) in Europe set strict guidelines on how data can be collected, stored, and used. Such regulations aim to protect users and ensure that companies are held accountable for their data practices. However, compliance can vary, and many companies may still find loopholes, making it essential for users to remain vigilant about their data rights.
In conclusion, the practices surrounding data collection in AI are multifaceted and fraught with ethical dilemmas. As users, we must educate ourselves about these practices and advocate for transparency and fairness in how our data is handled. Only then can we foster a relationship of trust between AI technologies and the individuals they serve.
- What is informed consent in data collection?
Informed consent refers to the process of ensuring that users are fully aware of what data is being collected and how it will be used before they agree to provide their information. - How can I protect my data when using AI technologies?
You can protect your data by carefully reviewing privacy policies, adjusting your privacy settings on platforms, and being mindful of the information you share online. - What are some regulations governing data collection practices?
Regulations like the GDPR in Europe and the California Consumer Privacy Act (CCPA) in the United States set standards for how companies can collect and use personal data.
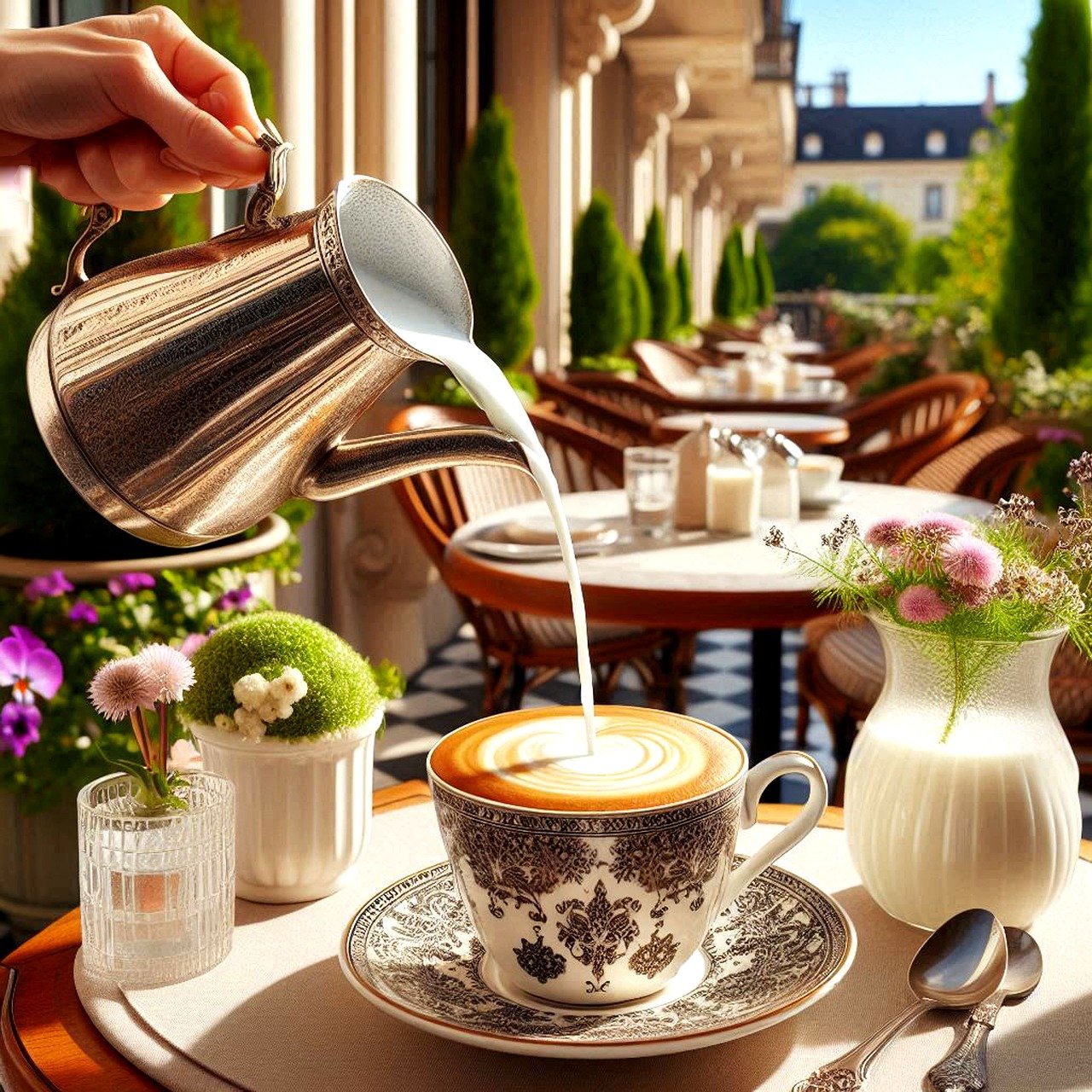
Regulatory Frameworks for AI
The rapid advancement of artificial intelligence (AI) technologies has sparked a critical conversation about the need for robust regulatory frameworks to ensure ethical deployment. As AI systems become increasingly integrated into our daily lives, the potential for misuse and unintended consequences grows. Regulatory frameworks serve as essential guidelines that can help balance innovation with ethical considerations, safeguarding public interests while promoting technological progress.
One of the primary challenges in developing effective regulatory frameworks is the dynamic nature of AI technologies. Unlike traditional industries, AI evolves at a breakneck speed, making it difficult for regulations to keep pace. To address this, regulatory bodies must adopt a proactive approach, focusing on principles rather than rigid rules. This allows for flexibility and adaptability in the face of rapid technological changes. Key principles often include transparency, accountability, and fairness.
Globally, various regions are beginning to establish their own regulatory measures. For instance, the European Union has proposed the AI Act, which categorizes AI systems based on their risk levels. This legislation aims to impose stricter requirements on high-risk AI applications, such as those used in healthcare or law enforcement, while allowing lower-risk applications to operate with less oversight. The goal is to create a balanced environment where innovation can flourish without compromising ethical standards.
In addition to regional regulations, it is crucial for international cooperation to take place. AI is a global phenomenon, and its implications transcend borders. Countries must work together to establish a cohesive regulatory framework that addresses ethical issues universally. This could involve creating international treaties or agreements that set baseline standards for AI development and usage, ensuring that all nations adhere to shared ethical principles.
Moreover, stakeholder engagement is vital in the regulatory process. Developers, users, ethicists, and policymakers should collaborate to create comprehensive regulations that reflect diverse perspectives. This collaborative approach can help identify potential risks and ethical dilemmas associated with AI technologies, leading to more informed decision-making.
As we look ahead, the importance of continuous evaluation and adaptation of regulatory frameworks cannot be overstated. AI technologies will evolve, and so too must the regulations that govern them. Regular assessments and updates to these frameworks will ensure they remain relevant and effective in addressing emerging challenges. By fostering an environment of ongoing dialogue and adaptation, we can create a future where AI is not only innovative but also ethically aligned with societal values.
- What are regulatory frameworks for AI? Regulatory frameworks for AI are guidelines and rules designed to ensure the ethical development and deployment of artificial intelligence technologies.
- Why are regulatory frameworks important? They are crucial for protecting public interests, ensuring accountability, and promoting fairness in AI applications.
- How do different countries approach AI regulation? Countries vary in their regulatory approaches; for example, the EU has proposed the AI Act, while other nations may have different guidelines or no formal regulations yet.
- What role do stakeholders play in AI regulation? Stakeholders, including developers, users, and policymakers, contribute diverse perspectives that help shape effective regulations.
Frequently Asked Questions
- What are the main ethical frameworks used to evaluate AI?
The primary ethical frameworks include consequentialism, which focuses on the outcomes of actions; deontology, which emphasizes duties and rules; and virtue ethics, which considers the character of the moral agent. Each framework offers a unique lens through which to examine the implications of AI technology on society.
- How does bias manifest in AI systems?
Bias in AI often stems from the data used to train these systems. If the training data contains racial, gender, or socioeconomic biases, the AI can perpetuate these biases in its decision-making processes. This can lead to unfair treatment and discrimination, impacting individuals and communities.
- What are the consequences of biased AI?
Biased AI can result in serious consequences, such as unjust hiring practices, discriminatory law enforcement, and unequal access to services. These outcomes not only harm individuals but can also erode trust in technology and institutions.
- What strategies can be implemented to mitigate bias in AI?
To reduce bias, developers can employ strategies like diverse data collection, ensuring representation across different demographics, and conducting algorithmic audits to identify and rectify biases. These approaches are essential for creating fairer AI systems.
- Who is responsible for the ethical use of AI?
Accountability in AI is complex and shared among developers, users, and organizations. Each party has a role in ensuring that AI is used ethically, from responsible development practices to informed usage and oversight.
- What privacy concerns arise with AI integration?
The integration of AI into daily life raises significant privacy issues, such as the potential for unauthorized data collection and surveillance. It's crucial to consider how AI technologies can infringe on personal privacy and the ethical implications of these actions.
- How are data collection practices regulated in AI?
Regulatory frameworks are being developed to address ethical concerns around data collection in AI. These regulations aim to ensure transparency, consent, and the responsible use of personal data, safeguarding individuals' privacy rights.