AI Innovations in Fraud Detection: An Overview
In today's fast-paced digital world, the threat of fraud looms larger than ever. Organizations across various sectors—be it banking, e-commerce, or insurance—are constantly battling against deceptive practices that can lead to significant financial losses and tarnished reputations. Fortunately, the rise of artificial intelligence (AI) has ushered in a new era of fraud detection, enabling businesses to stay one step ahead of fraudsters. This article delves into the latest advancements in AI technologies that are revolutionizing the way fraud is detected and prevented.
At its core, fraud detection is about identifying and mitigating deceptive activities that can harm businesses and consumers alike. Traditional methods often rely on rule-based systems that can be slow, cumbersome, and prone to errors. However, with the integration of AI, organizations can leverage powerful machine learning algorithms and sophisticated data analytics to enhance their fraud detection capabilities. The result? A more robust, efficient, and proactive approach to combating fraud.
As we explore the innovations in AI for fraud detection, we will uncover key techniques that are making waves in the industry, examine the myriad benefits these technologies offer, and discuss the challenges organizations face in implementing AI solutions. Furthermore, we will take a glimpse into the future trends that will shape the landscape of fraud prevention. So, buckle up as we embark on this journey through the fascinating world of AI innovations in fraud detection!
Fraud detection involves identifying and preventing deceptive activities in various industries. This section outlines the importance of fraud detection systems and their impact on businesses and consumers alike.
AI technologies significantly enhance fraud detection capabilities. This section discusses how machine learning algorithms and data analytics are transforming traditional fraud detection methods.
Machine learning algorithms play a crucial role in identifying patterns and anomalies in large datasets. This subsection explains the different types of algorithms used in fraud detection and their effectiveness.
Supervised learning techniques utilize labeled datasets to train models for fraud detection. This part delves into how these techniques improve accuracy and reduce false positives in fraud detection.
Unsupervised learning techniques analyze data without pre-existing labels, identifying hidden patterns. This section highlights their importance in discovering new types of fraud that traditional methods might miss.
Data analytics is essential for effective fraud detection. This subsection covers the various analytical methods employed to scrutinize transaction data and detect suspicious activities.
Implementing AI in fraud detection offers numerous advantages, including improved accuracy, speed, and efficiency. This section discusses how these benefits enhance overall security and customer trust.
AI-driven fraud detection systems can significantly reduce costs associated with fraud losses and manual investigations. This part elaborates on the financial benefits for organizations adopting these technologies.
AI technologies not only prevent fraud but also enhance customer experience by minimizing false declines. This section explores how businesses can maintain customer satisfaction while ensuring security.
Despite its advantages, implementing AI solutions for fraud detection poses challenges. This section addresses common hurdles organizations face when integrating AI technologies into their systems.
Data privacy remains a significant concern in AI applications. This subsection discusses the ethical implications and regulations surrounding data usage in fraud detection systems.
Integrating AI solutions with legacy systems can be complex. This part examines the technical challenges and strategies for seamless integration to maximize the effectiveness of fraud detection efforts.
The landscape of AI in fraud detection is constantly evolving. This section highlights emerging trends and technologies that will shape the future of fraud prevention and detection strategies.
- What is AI in fraud detection? AI in fraud detection refers to the use of artificial intelligence technologies, such as machine learning and data analytics, to identify and prevent fraudulent activities.
- How does machine learning improve fraud detection? Machine learning algorithms analyze large datasets to identify patterns and anomalies, improving accuracy and reducing false positives in fraud detection.
- What are the challenges of implementing AI in fraud detection? Common challenges include data privacy concerns, integration with existing systems, and the need for skilled personnel to manage AI technologies.
- What are the benefits of using AI for fraud detection? Benefits include improved accuracy, faster detection times, cost reduction, and enhanced customer experience.
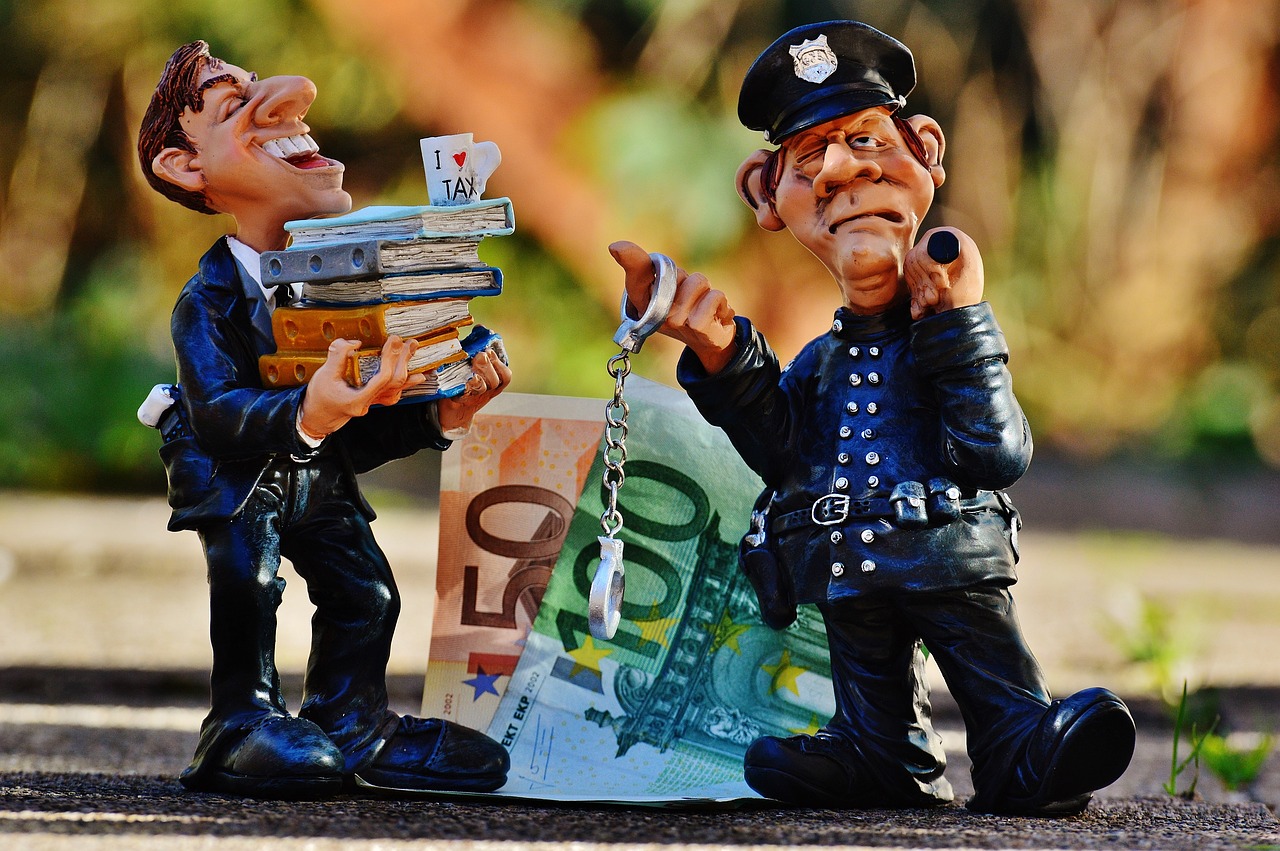
Understanding Fraud Detection
Fraud detection is a critical aspect of modern business operations. In a world where transactions occur at lightning speed, identifying and preventing deceptive activities has become more important than ever. Organizations across various sectors, from banking to e-commerce, face the constant threat of fraud, which can lead to significant financial losses and damage to reputation. The importance of fraud detection systems cannot be overstated, as they play a vital role in safeguarding both businesses and consumers.
At its core, fraud detection involves the use of sophisticated technologies and methodologies to identify suspicious activities that deviate from the norm. With the rise of digital transactions, the landscape of fraud has evolved, leading to increasingly complex schemes that are difficult to detect. This is where advanced fraud detection systems come into play, utilizing a combination of statistical methods, machine learning algorithms, and data analytics to uncover fraudulent behavior.
Fraud detection systems not only protect organizations from financial losses but also enhance consumer trust. When consumers know that robust fraud detection measures are in place, they feel more secure in their transactions. This trust is crucial for businesses that rely on customer loyalty and repeat purchases. In fact, a study by the Association of Certified Fraud Examiners found that organizations with effective fraud detection systems can save up to 50% on fraud-related losses compared to those without such systems.
In addition to financial implications, the impact of fraud extends to legal and regulatory consequences. Organizations that fail to adequately protect against fraud may face penalties and legal action, further compounding their losses. Therefore, investing in effective fraud detection systems is not just a smart business move; it's a necessary step to ensure compliance and protect the integrity of the organization.
In summary, understanding fraud detection is essential for any organization operating in today’s digital landscape. By implementing effective fraud detection systems, businesses can not only protect their bottom line but also build a reputation for security and reliability. As we delve deeper into the role of artificial intelligence in fraud detection, we'll uncover how these technologies are revolutionizing the way organizations combat fraud.
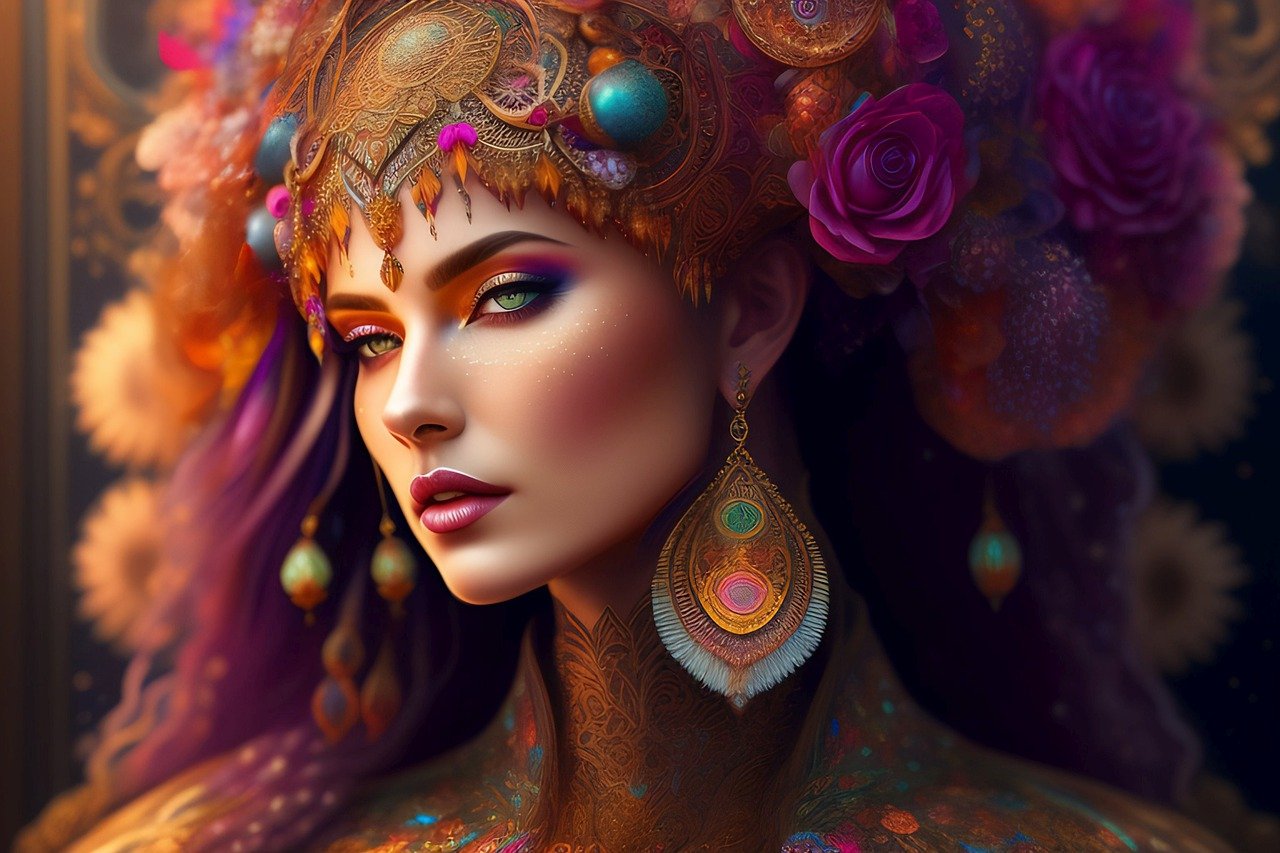
The Role of AI in Fraud Detection
In today's fast-paced digital world, where transactions happen at lightning speed, the role of artificial intelligence (AI) in fraud detection has become crucial. Traditional methods of fraud detection often rely on static rules and historical data, which can quickly become outdated. Enter AI, a game-changer that leverages machine learning algorithms and data analytics to enhance the accuracy and efficiency of fraud detection systems. By utilizing AI, organizations can not only keep pace with fraudsters but also stay one step ahead of them.
One of the most significant advantages of AI in fraud detection is its ability to process vast amounts of data in real-time. Imagine trying to find a needle in a haystack; now imagine having a magnet that can pull out that needle almost instantly. This is what AI does for fraud detection. It analyzes transaction patterns, user behaviors, and other relevant data points to identify anomalies that could indicate fraudulent activities. This capability is especially beneficial in sectors like banking, e-commerce, and insurance, where the volume of transactions is enormous and the stakes are high.
Moreover, AI technologies utilize both supervised and unsupervised learning techniques to refine their predictive capabilities. With supervised learning, models are trained on labeled datasets, allowing them to recognize known fraud patterns. Conversely, unsupervised learning explores data without labels, uncovering hidden patterns and potential new types of fraud that might not have been previously identified. This dual approach equips organizations with a comprehensive toolkit for combating fraud.
Another remarkable aspect of AI in fraud detection is its ability to adapt and improve over time. As AI systems are exposed to more data, they learn from past incidents and continuously refine their algorithms. This means that the longer an organization uses AI for fraud detection, the more effective it becomes. In contrast, traditional methods may require constant manual updates and adjustments, which can be both time-consuming and prone to human error.
However, while AI offers promising solutions, it is essential to remember that it is not a silver bullet. Organizations must remain vigilant and combine AI technologies with other fraud prevention strategies. This holistic approach ensures that they are not solely reliant on technology but are also implementing best practices, employee training, and robust policies to safeguard against fraud.
In summary, the role of AI in fraud detection is transformative. By leveraging advanced algorithms and data analytics, businesses can enhance their fraud detection capabilities, reduce false positives, and ultimately protect their bottom line. As we look to the future, it is clear that AI will continue to shape the landscape of fraud detection, making it an indispensable tool for organizations across various sectors.
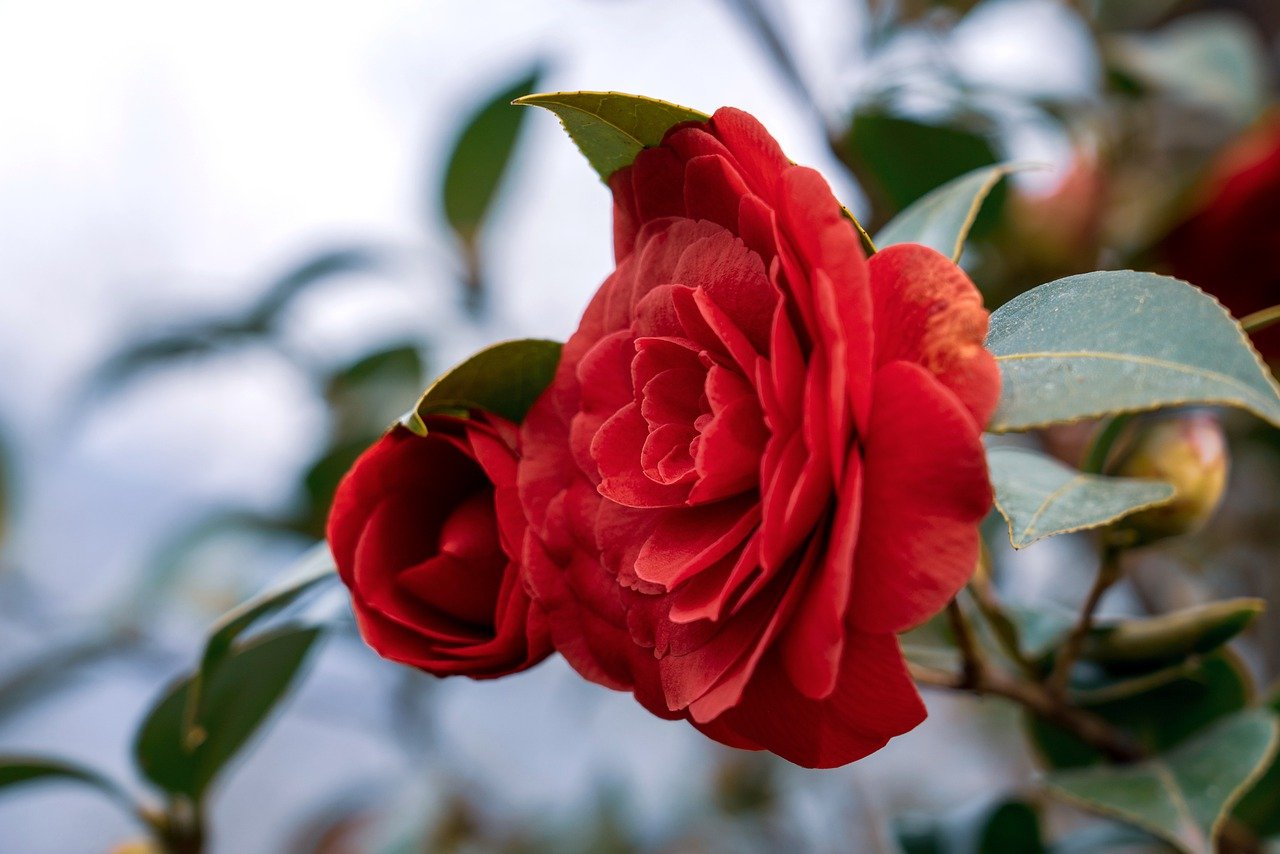
Machine Learning Algorithms
Machine learning algorithms are at the forefront of revolutionizing fraud detection. These algorithms enable systems to learn from data, identify patterns, and make decisions with minimal human intervention. Imagine having a vigilant watchdog that never sleeps, constantly analyzing vast amounts of data to catch suspicious activities—this is precisely what machine learning does in the realm of fraud detection.
There are several types of machine learning algorithms that play a crucial role in this process. Each algorithm has its unique strengths and applications, making them suitable for different fraud detection scenarios. Here’s a brief overview of the primary types:
- Supervised Learning: This approach involves training a model on a labeled dataset, which means that the data includes examples of both fraudulent and legitimate transactions. The model learns to differentiate between the two, improving its accuracy over time.
- Unsupervised Learning: Unlike supervised learning, this technique works with unlabeled data. It identifies hidden patterns and anomalies that may indicate fraud. This is particularly useful for detecting new types of fraud that haven’t been encountered before.
- Reinforcement Learning: This algorithm learns through trial and error, receiving feedback from its actions. It’s like teaching a dog new tricks—rewarding it when it does something right. In fraud detection, reinforcement learning can adapt strategies based on the success or failure of previous actions.
These algorithms are not just theoretical concepts; they are actively used in various industries, including finance, e-commerce, and insurance. For instance, in the banking sector, supervised learning algorithms analyze transaction histories to flag potentially fraudulent transactions before they escalate. On the other hand, e-commerce platforms often employ unsupervised learning to detect unusual purchasing behaviors that could indicate fraud.
The effectiveness of these machine learning algorithms lies in their ability to process and analyze large datasets quickly. Traditional methods of fraud detection often rely on manual reviews and rule-based systems, which can be time-consuming and prone to human error. In contrast, machine learning algorithms can analyze thousands of transactions in seconds, providing real-time insights and alerts.
However, it’s essential to note that while machine learning algorithms significantly enhance fraud detection capabilities, they are not foolproof. They require continuous training and updating to adapt to new fraud tactics. Additionally, the effectiveness of these algorithms heavily depends on the quality and quantity of data available for training. Poor data can lead to inaccurate predictions, which could result in false positives or negatives—issues that can harm customer trust and business operations.
In conclusion, machine learning algorithms are transforming the landscape of fraud detection, offering powerful tools for identifying and preventing fraudulent activities. By leveraging these technologies, organizations can not only enhance their security measures but also foster greater trust and satisfaction among their customers.
- What are machine learning algorithms? Machine learning algorithms are computational methods that enable systems to learn from data, identify patterns, and make predictions or decisions without being explicitly programmed.
- How do machine learning algorithms help in fraud detection? They analyze large datasets to identify unusual patterns and anomalies that may indicate fraudulent activity, allowing for quicker and more accurate detection compared to traditional methods.
- What is the difference between supervised and unsupervised learning? Supervised learning uses labeled datasets to train models, while unsupervised learning works with unlabeled data to discover hidden patterns.
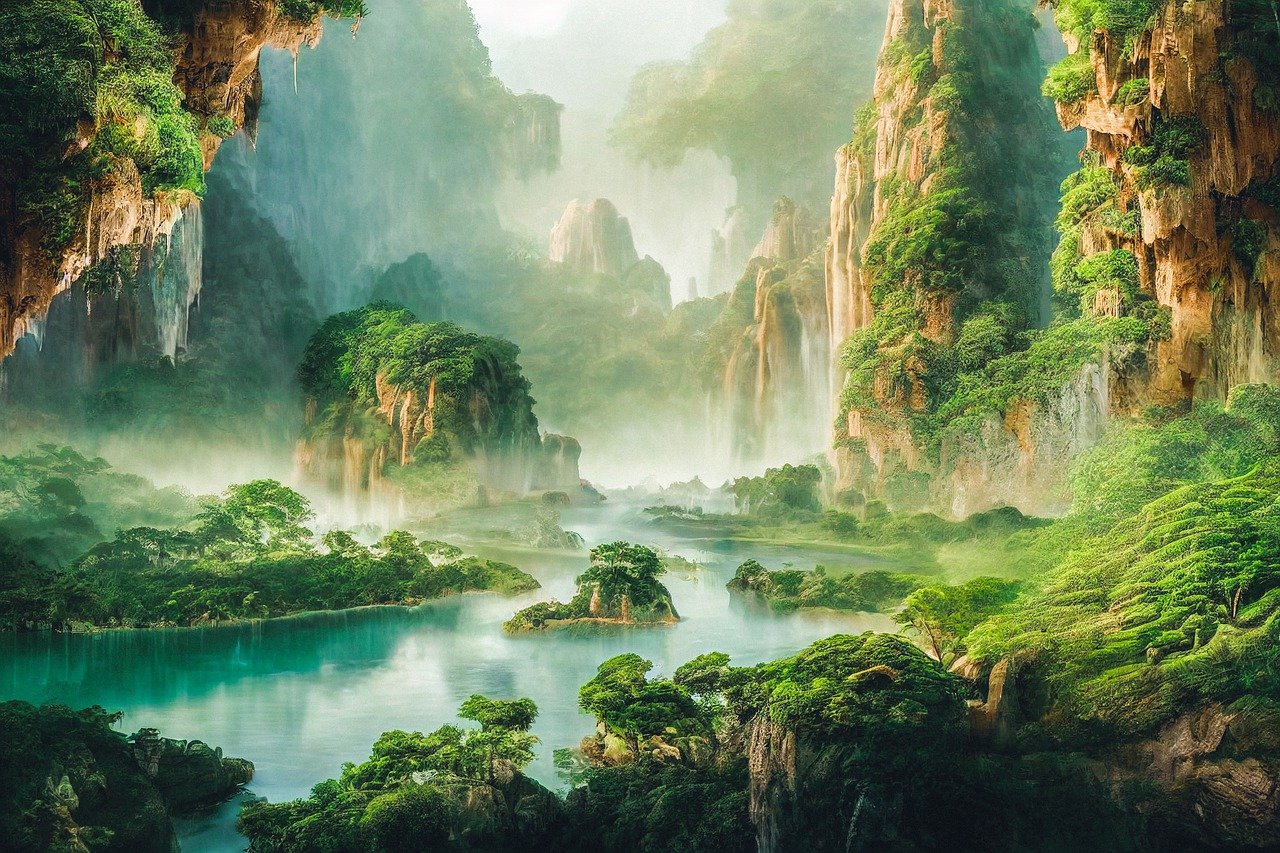
Supervised Learning Techniques
Supervised learning techniques are a cornerstone of modern fraud detection systems. By utilizing labeled datasets, these methods train algorithms to recognize patterns associated with fraudulent activities. Imagine teaching a child to identify apples and oranges by showing them examples; similarly, supervised learning feeds data into a model, allowing it to learn the characteristics of legitimate transactions versus fraudulent ones. This approach not only improves the accuracy of fraud detection but also significantly reduces the rate of false positives, which can frustrate customers and lead to lost business opportunities.
In the realm of supervised learning, various algorithms are employed, each with its unique strengths. Some of the most common include:
- Decision Trees: These algorithms split data into branches based on decision rules, making it easy to visualize the decision-making process.
- Random Forest: An ensemble method that combines multiple decision trees to enhance prediction accuracy and control overfitting.
- Support Vector Machines (SVM): These models find the hyperplane that best separates different classes of data, effectively distinguishing between fraudulent and non-fraudulent transactions.
- Neural Networks: Inspired by the human brain, these models can learn complex patterns and are particularly effective in high-dimensional spaces.
The effectiveness of supervised learning techniques in fraud detection is often measured using metrics like precision, recall, and F1 score. These metrics help organizations understand how well their models are performing. For instance, high precision indicates that when the model predicts a transaction as fraudulent, it is likely correct, whereas high recall shows that the model successfully identifies a significant portion of actual fraud cases. Striking the right balance between these metrics is crucial. A model that is too conservative may miss real fraud cases, while one that is too aggressive may flag too many legitimate transactions, leading to customer dissatisfaction.
Moreover, as fraudsters become more sophisticated, the need for continuous learning is paramount. Supervised learning models must be regularly updated with new data to adapt to evolving fraud patterns. This dynamic nature of fraud detection means that organizations must invest in ongoing model training and validation. By doing so, they not only maintain their defenses against fraud but also enhance their overall operational efficiency. In conclusion, supervised learning techniques are invaluable in the fight against fraud, providing a systematic approach to identifying and mitigating risks while fostering a safer environment for consumers and businesses alike.
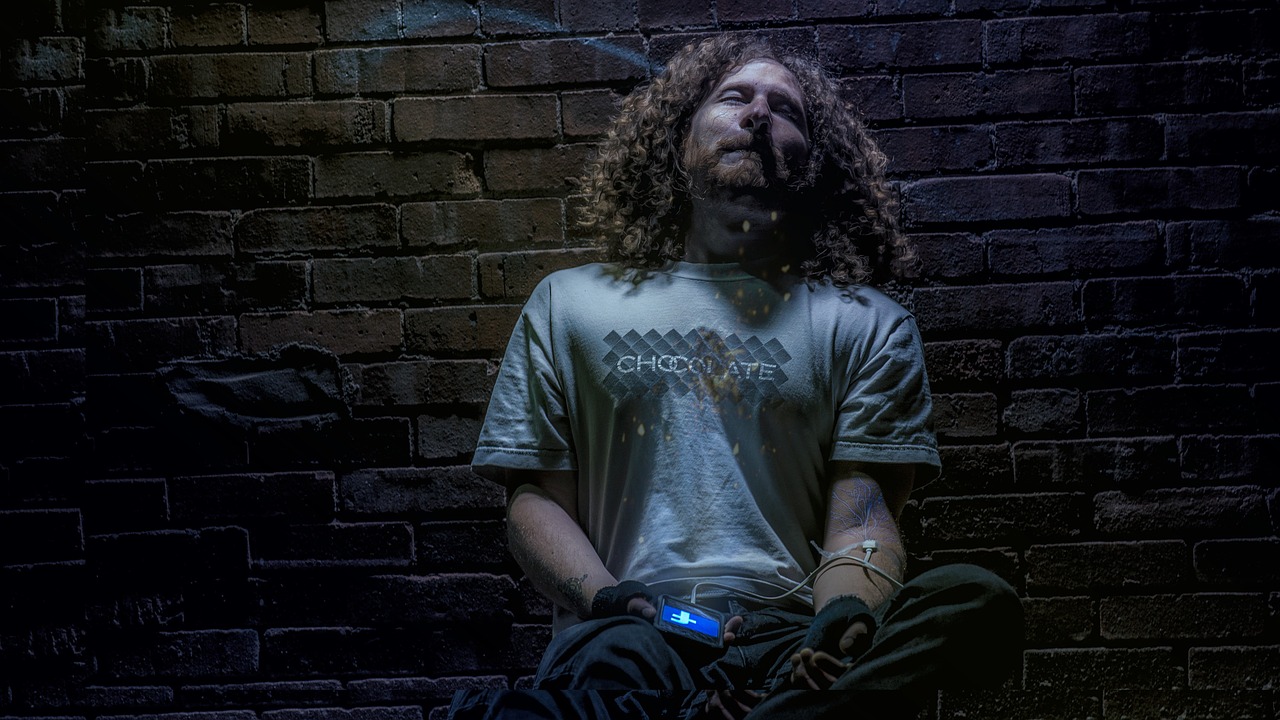
Unsupervised Learning Techniques
Unsupervised learning techniques are a game changer in the realm of fraud detection, primarily because they allow systems to analyze vast amounts of data without needing pre-labeled outcomes. Imagine walking into a crowded room and trying to find someone you know without any hints about their appearance. It’s a daunting task, right? Now, think of unsupervised learning as a smart assistant that helps you identify patterns in the crowd, even when you don’t know who you’re looking for. This powerful capability is crucial in detecting fraudulent activities that may not have been previously recognized by traditional methods.
These techniques excel in identifying hidden patterns and anomalies in transactional data, which could signify fraudulent behavior. For instance, when a financial institution analyzes thousands of transactions, unsupervised learning algorithms can highlight unusual spending patterns that deviate from a user’s typical behavior. By clustering similar transactions and flagging those that fall outside the norm, these algorithms can uncover new types of fraud that might escape the notice of supervised models.
Some common unsupervised learning techniques used in fraud detection include:
- Clustering: This method groups similar data points together, allowing the identification of outliers that may indicate fraud.
- Anomaly Detection: This approach focuses on identifying rare items, events, or observations that raise suspicions by differing significantly from the majority of the data.
- Dimensionality Reduction: Techniques like Principal Component Analysis (PCA) simplify complex datasets, making it easier to visualize and analyze transaction behaviors.
One of the most significant advantages of unsupervised learning is its ability to adapt and learn over time. As new fraud patterns emerge, these systems can continuously refine their models, ensuring that organizations stay ahead of fraudsters. This adaptability is crucial in an environment where fraud techniques evolve rapidly.
However, it's important to note that while unsupervised learning techniques are powerful, they are not without challenges. One of the main hurdles is the potential for high false-positive rates. Since these models do not rely on labeled data, they may flag legitimate transactions as fraudulent, causing unnecessary disruptions for customers. Thus, organizations must strike a balance between vigilance and customer experience.
In conclusion, unsupervised learning techniques represent a frontier in the fight against fraud. By leveraging these innovative methods, businesses can uncover hidden insights and enhance their fraud detection capabilities, ultimately leading to a more secure environment for consumers and organizations alike.
Q1: What is unsupervised learning in fraud detection?
A1: Unsupervised learning involves analyzing data without pre-existing labels, enabling systems to identify hidden patterns and anomalies that may indicate fraud.
Q2: How does clustering work in fraud detection?
A2: Clustering groups similar transactions together, allowing the identification of outliers that could signify fraudulent activity.
Q3: What are the challenges of using unsupervised learning techniques?
A3: One major challenge is the potential for high false-positive rates, where legitimate transactions may be incorrectly flagged as fraudulent.
Q4: Can unsupervised learning adapt to new fraud patterns?
A4: Yes, unsupervised learning techniques can continuously refine their models to adapt to emerging fraud patterns, making them highly effective in dynamic environments.
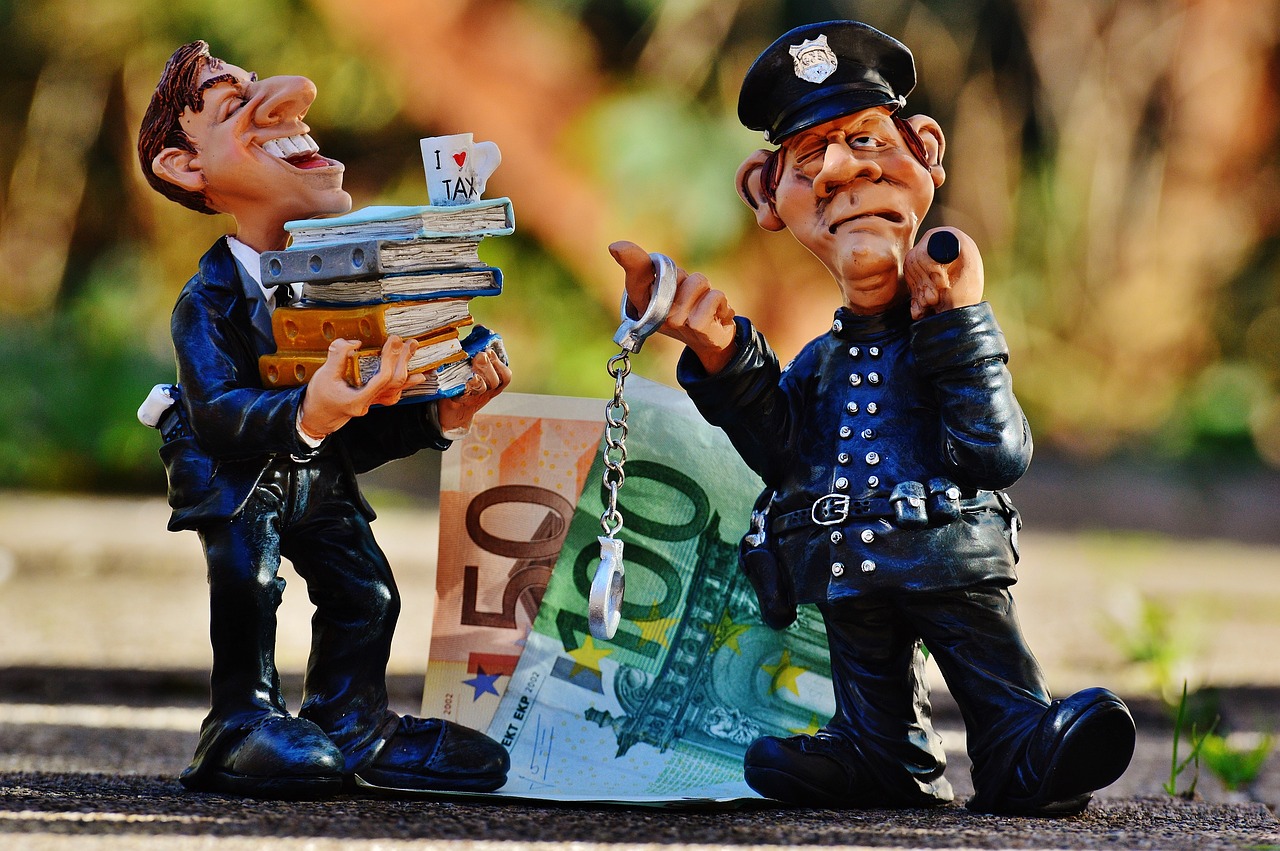
Data Analytics in Fraud Detection
Data analytics is the backbone of modern fraud detection systems, acting like a vigilant watchdog that tirelessly scans through vast amounts of transaction data. In a world where fraudsters are becoming increasingly sophisticated, relying on traditional methods is akin to using a paper map in a GPS-driven world. With the sheer volume of transactions happening every second, manual monitoring simply isn't feasible. Instead, businesses are harnessing the power of data analytics to uncover suspicious activities that might otherwise go unnoticed.
One of the most effective analytical methods employed in fraud detection is transaction monitoring. By continuously analyzing transaction patterns in real-time, organizations can quickly identify anomalies that deviate from established norms. For instance, if a customer's spending suddenly spikes or if a transaction occurs in a location far from their usual habits, these red flags can trigger alerts for further investigation.
Moreover, predictive analytics plays a pivotal role in forecasting potential fraud scenarios before they even occur. By leveraging historical data, machine learning models can predict the likelihood of fraudulent activity based on various factors such as transaction type, amount, and frequency. This proactive approach allows businesses to take preventive measures, reducing the chances of fraud before it manifests.
Another essential aspect of data analytics is the use of network analysis. This technique examines the relationships and interactions between different entities, such as users and transactions. By visualizing these connections, organizations can uncover hidden networks of fraudsters who might be collaborating to execute scams. This method is invaluable, as it not only helps in identifying existing fraud but also in predicting future fraudulent behaviors.
Data visualization tools further enhance the effectiveness of fraud detection analytics. By presenting complex data in an easily digestible format, these tools allow analysts to quickly grasp the situation and make informed decisions. For example, heat maps can highlight regions with high fraud incidents, enabling companies to allocate resources more effectively and target their fraud prevention efforts where they are needed most.
In conclusion, the integration of data analytics into fraud detection systems is not just a trend; it's a necessity in today’s digital landscape. As fraud tactics evolve, so must the strategies to combat them. By leveraging advanced analytics, organizations can not only enhance their fraud detection capabilities but also foster a culture of security and trust among their customers.
- What is the role of data analytics in fraud detection?
Data analytics helps in monitoring transactions, predicting potential fraud, and uncovering hidden networks of fraudsters. - How can predictive analytics prevent fraud?
By analyzing historical data, predictive analytics can forecast the likelihood of fraudulent activities, allowing businesses to take preventive measures. - What are some common techniques used in data analytics for fraud detection?
Common techniques include transaction monitoring, predictive analytics, and network analysis. - Why is real-time monitoring important in fraud detection?
Real-time monitoring allows businesses to quickly identify and respond to suspicious activities, reducing potential losses.
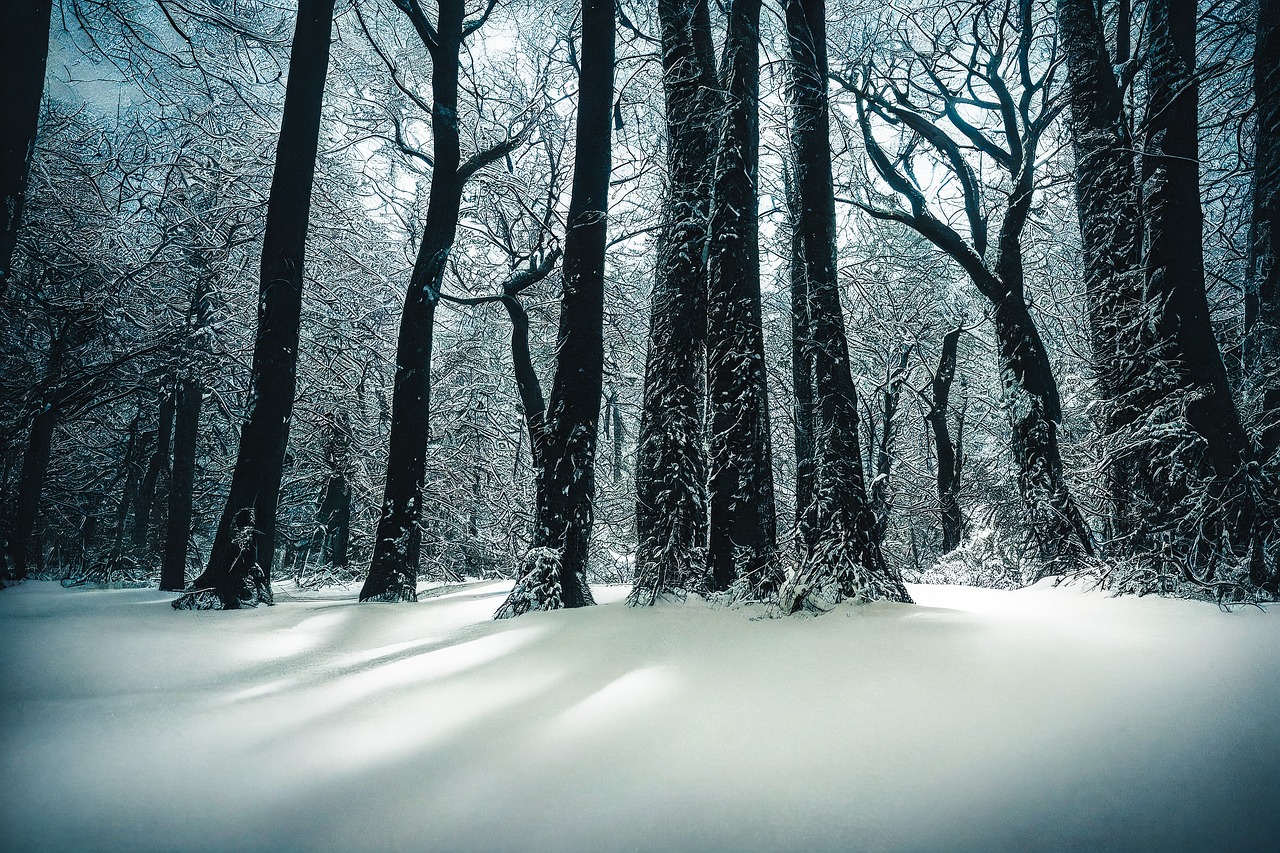
Benefits of AI in Fraud Detection
Implementing artificial intelligence (AI) in fraud detection brings a plethora of advantages that can significantly enhance the security landscape for businesses and their customers. One of the most notable benefits is the improved accuracy of fraud detection systems. Traditional methods often rely on predefined rules that can miss subtle or emerging fraudulent patterns. In contrast, AI systems leverage advanced algorithms to analyze vast amounts of data, identifying anomalies that human analysts might overlook. This leads to a more robust defense against fraud, ensuring that organizations can act swiftly and effectively.
Another remarkable benefit of AI in fraud detection is the speed at which suspicious activities can be identified and addressed. Machine learning models can process data in real-time, allowing businesses to respond to potential fraud attempts almost instantaneously. This rapid response capability not only helps in mitigating losses but also fosters a sense of security among customers, enhancing their trust in the organization. As a result, businesses can maintain a competitive edge in the market.
Furthermore, the efficiency gained from AI-driven systems cannot be overstated. By automating the detection process, organizations can significantly reduce the time and resources spent on manual investigations. This efficiency translates into cost savings, as businesses can allocate their resources more effectively, focusing on growth and innovation rather than constantly battling fraud. According to recent studies, companies that have integrated AI into their fraud detection processes have seen a reduction in fraud-related losses by up to 30%.
To illustrate the financial impact of AI on fraud detection, consider the following table:
Aspect | Traditional Methods | AI-Driven Methods |
---|---|---|
Fraud Detection Speed | Hours to Days | Real-Time |
False Positive Rate | High (up to 25%) | Low (below 5%) |
Cost of Investigations | High | Significantly Reduced |
Moreover, AI technologies not only focus on preventing fraud but also play a crucial role in enhancing the customer experience. One of the common pain points for customers is the frustration that arises from false declines during transactions. AI systems can analyze user behavior and transaction patterns more accurately, allowing legitimate transactions to go through while still flagging suspicious ones. This balance between security and customer satisfaction is vital for businesses aiming to build long-term relationships with their clients.
In summary, the benefits of AI in fraud detection are multifaceted, encompassing improved accuracy, speed, efficiency, and enhanced customer experiences. These advantages not only help protect organizations from financial losses but also bolster consumer trust, ultimately leading to a more secure and reliable marketplace for all.
- What is the primary benefit of using AI in fraud detection? The primary benefit is improved accuracy in identifying fraudulent activities, which helps organizations respond more effectively.
- How does AI enhance customer experience? AI reduces false declines in transactions, ensuring legitimate customers can complete their purchases without unnecessary interruptions.
- Are AI-driven fraud detection systems cost-effective? Yes, they can significantly reduce costs associated with fraud losses and manual investigations.
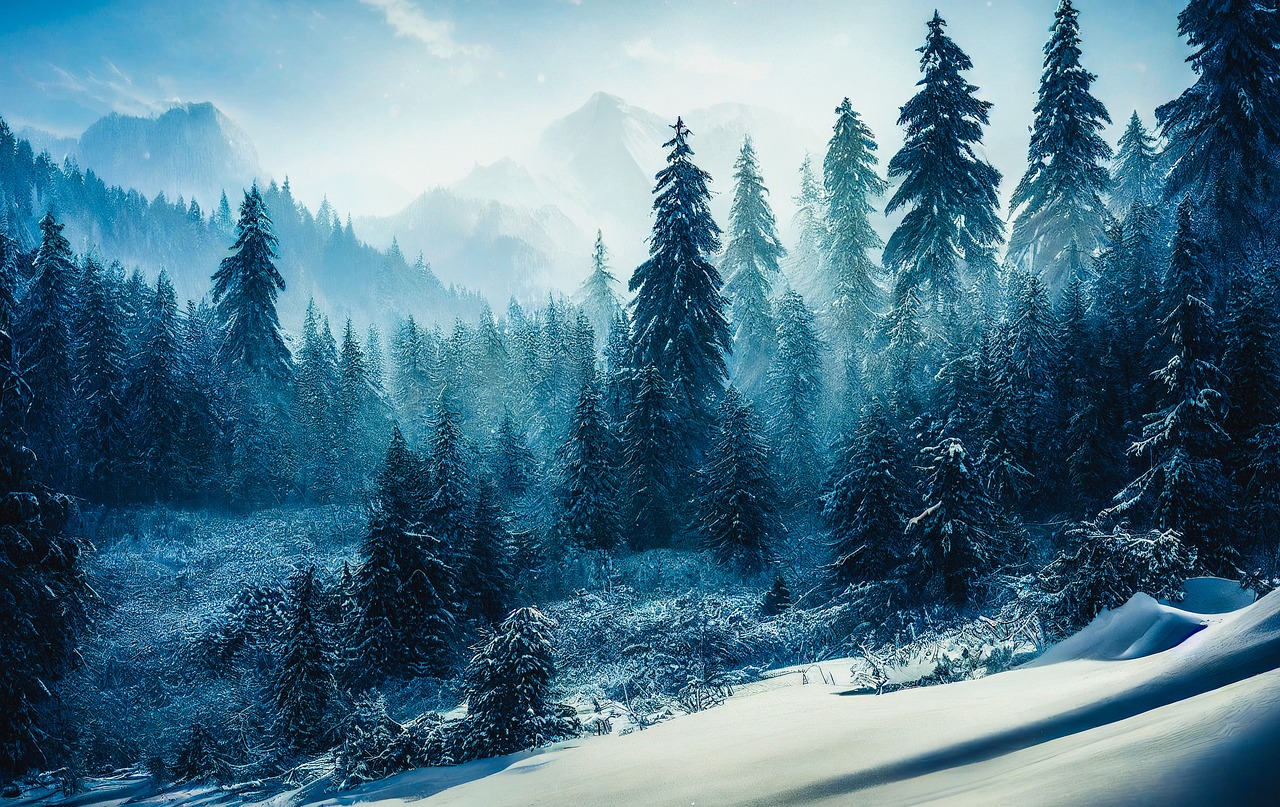
Cost Reduction
In today's fast-paced business environment, where every penny counts, organizations are increasingly turning to AI-driven fraud detection systems to mitigate financial losses associated with fraudulent activities. These advanced technologies not only help in identifying and preventing fraud but also play a crucial role in significantly reducing operational costs. How do they achieve this? Well, let’s break it down.
First and foremost, AI systems can analyze vast amounts of data at lightning speed, far surpassing the capabilities of human analysts. This efficiency means that organizations can detect fraudulent transactions in real-time, preventing potential losses before they escalate. For instance, consider a retail company that experiences a surge in credit card chargebacks due to fraudulent purchases. By implementing an AI system, the company can reduce the time spent on manual investigations, thereby saving on labor costs associated with fraud detection.
Moreover, the implementation of AI technologies often leads to a reduction in false positives. Traditional fraud detection methods frequently flag legitimate transactions as suspicious, leading to unnecessary declines and customer dissatisfaction. This not only frustrates customers but can also result in lost sales. AI systems, particularly those utilizing machine learning algorithms, continuously learn from historical data, refining their models to distinguish between genuine and fraudulent transactions with greater accuracy. This improvement translates into fewer false alarms, allowing businesses to maintain revenue streams while ensuring customer trust.
Additionally, the cost associated with fraud losses can be staggering. According to recent studies, organizations lose approximately $3.5 trillion annually due to fraud. By proactively employing AI solutions, businesses can reduce these losses significantly. For example, a financial institution that adopts AI-driven fraud detection might see a 30% decrease in fraud-related losses within the first year of implementation. This not only enhances their bottom line but also contributes to a more secure financial ecosystem.
To illustrate this further, consider the following table that outlines the potential cost savings associated with AI fraud detection:
Cost Factor | Traditional Method | AI-Driven Method | Estimated Savings |
---|---|---|---|
Fraud Losses | $1,000,000 | $700,000 | $300,000 |
Operational Costs | $500,000 | $300,000 | $200,000 |
False Positives | $200,000 | $50,000 | $150,000 |
Total Savings | $1,700,000 | $1,050,000 | $650,000 |
As illustrated, the transition from traditional methods to AI-driven solutions can lead to substantial cost reductions. This not only impacts the financial health of organizations but also enhances their ability to invest in other critical areas, such as customer service and innovation.
In conclusion, the integration of AI in fraud detection is not just a trend; it’s a game-changer. By reducing costs associated with fraud losses, operational inefficiencies, and false positives, organizations can create a more robust and secure environment for both themselves and their customers. As businesses continue to navigate the complexities of fraud, those who adopt AI technologies will likely find themselves at a significant advantage.
- What are the main benefits of AI in fraud detection? AI enhances accuracy, reduces false positives, and speeds up transaction analysis, leading to significant cost savings.
- How does AI reduce false positives? AI systems learn from historical data, improving their ability to distinguish between legitimate and fraudulent transactions.
- Can small businesses benefit from AI fraud detection? Absolutely! AI solutions are scalable and can provide significant advantages to businesses of all sizes.
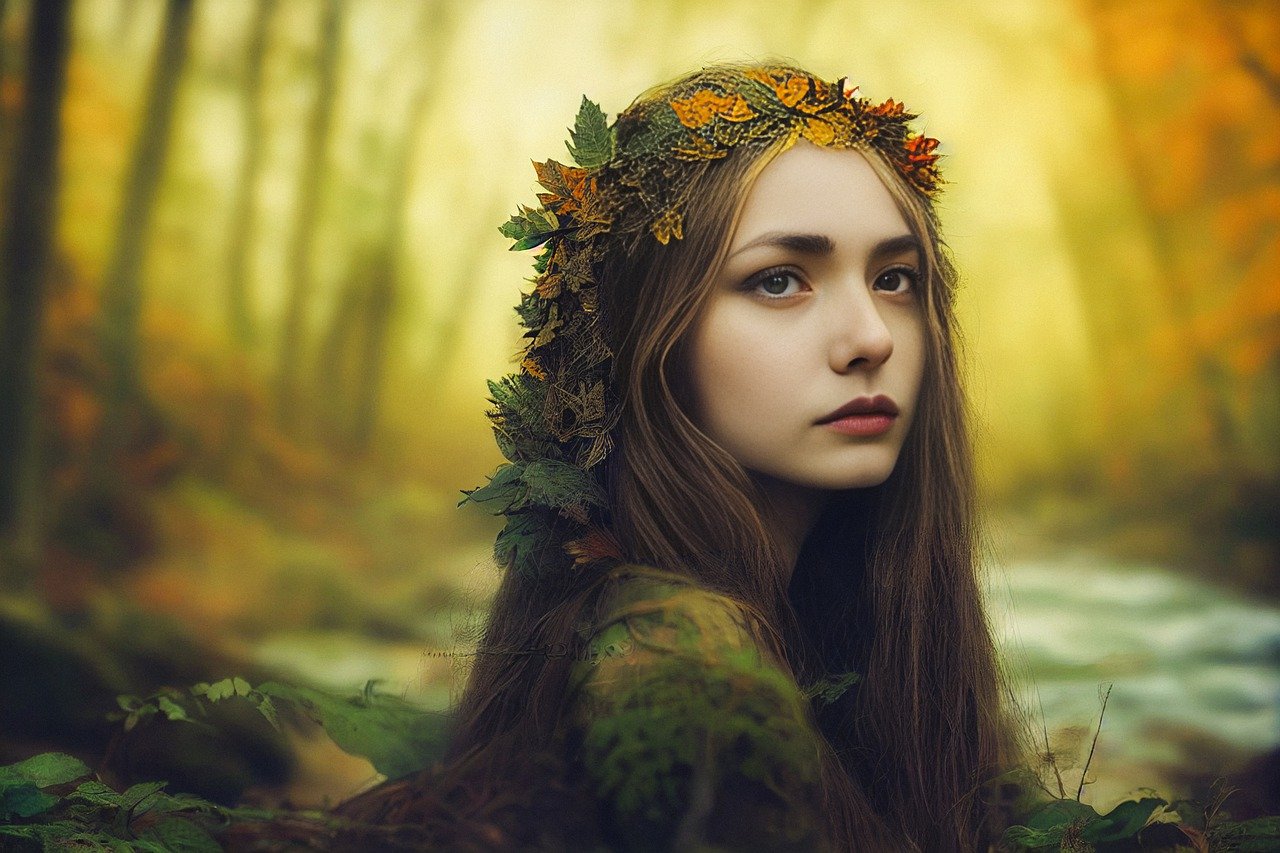
Enhanced Customer Experience
In the fast-paced world of digital transactions, ensuring a seamless and secure customer experience is paramount. With the integration of AI technologies in fraud detection, businesses are not only enhancing their security protocols but also significantly improving the overall customer experience. Imagine walking into a store where the staff knows your preferences and can serve you without delays. This is akin to how AI works in the background, making transactions smoother and more efficient.
One of the most notable advantages of AI in fraud detection is its ability to minimize false declines. These are instances where legitimate transactions are mistakenly flagged as fraudulent, leading to unnecessary frustration for customers. By utilizing advanced machine learning algorithms, AI systems can accurately assess the risk level of transactions in real-time, ensuring that genuine customers can complete their purchases without hindrance. This not only boosts customer satisfaction but also fosters a sense of trust between the consumer and the business.
Moreover, AI-driven systems can learn from past transactions, adapting and evolving to recognize patterns associated with both legitimate and fraudulent activities. This adaptability is crucial in today's ever-changing digital landscape, where fraudsters continuously devise new tactics. By staying one step ahead, businesses can provide a secure shopping experience, allowing customers to shop with confidence.
In addition to reducing false positives, AI technologies can also enhance customer interactions through personalized experiences. For instance, when a customer is flagged for unusual activity, AI systems can quickly verify the identity of the customer through various means, such as biometric recognition or behavioral analysis. This swift verification process not only saves time but also ensures that customers feel valued and understood.
Furthermore, the implementation of AI in fraud detection can lead to significant cost savings for businesses. By reducing the number of false declines and improving the efficiency of fraud investigations, companies can allocate more resources towards enhancing customer service initiatives. This creates a positive feedback loop: as businesses save money, they can invest more in customer experience improvements, leading to higher satisfaction rates and customer loyalty.
In conclusion, the integration of AI in fraud detection systems is a game-changer for enhancing customer experience. By reducing false declines, personalizing interactions, and optimizing operational costs, businesses can create a secure and enjoyable shopping environment. As we move forward, it will be exciting to see how these technologies continue to evolve and reshape the landscape of customer service.
- How does AI reduce false declines in fraud detection? AI algorithms analyze transaction patterns in real-time, distinguishing between legitimate and fraudulent activities, thereby minimizing errors.
- Can AI improve customer interactions? Yes, AI can personalize customer interactions by quickly verifying identities and adapting to customer preferences.
- What are the cost benefits of using AI in fraud detection? AI reduces the costs associated with fraud losses and manual investigations, allowing businesses to invest in better customer service.
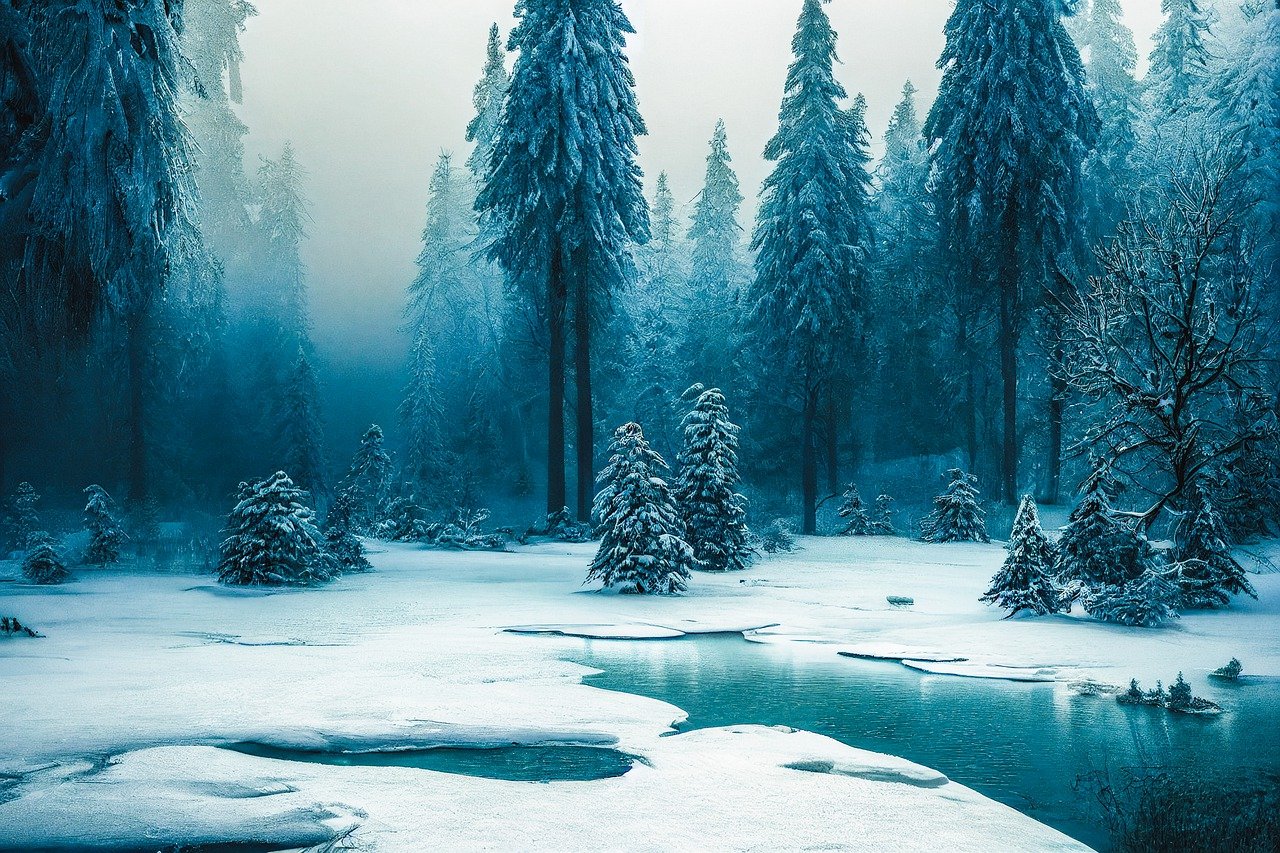
Challenges in Implementing AI Solutions
Implementing AI solutions for fraud detection is not without its hurdles. While the benefits of AI are substantial, organizations often find themselves grappling with a variety of challenges that can hinder the effective deployment of these technologies. One of the most pressing issues is data privacy concerns. As AI systems rely heavily on vast amounts of data to function effectively, the ethical implications surrounding data usage become paramount. Organizations must navigate complex regulations, such as the General Data Protection Regulation (GDPR) in Europe, which governs how personal data is collected, stored, and used. Failure to comply with these regulations can lead to hefty fines and damage to a company’s reputation.
Another significant challenge is the integration with existing systems. Many businesses operate on legacy systems that may not be compatible with modern AI technologies. Integrating AI solutions into these outdated infrastructures can be complex and time-consuming. Organizations often face technical hurdles, such as data silos and incompatible software, that can complicate the integration process. To overcome these challenges, companies may need to invest in upgrading their systems or developing custom solutions that bridge the gap between old and new technologies.
Additionally, there is a skills gap in the workforce. Implementing AI solutions requires specialized knowledge and expertise that many organizations may lack. The demand for data scientists and AI specialists is soaring, but the supply is limited. This shortage can lead to delays in project timelines and can increase the likelihood of errors during implementation. Companies must either invest in training their existing staff or seek external talent, both of which can be costly and time-consuming.
Moreover, cost considerations cannot be overlooked. While AI can lead to long-term savings, the initial investment in technology and talent can be substantial. Organizations must weigh the potential return on investment against the upfront costs. This can be particularly challenging for smaller businesses that may not have the budget to invest in sophisticated AI solutions. A detailed cost-benefit analysis should be conducted to ensure that the investment is justified and aligns with the company’s strategic goals.
Finally, the need for continuous monitoring and maintenance of AI systems is crucial. Once implemented, AI solutions require ongoing oversight to ensure they function correctly and adapt to evolving fraud patterns. This involves regularly updating algorithms, retraining models, and maintaining data integrity. Without proper maintenance, AI systems can become less effective over time, leading to missed fraudulent activities and increased risk for the organization.
In summary, while AI presents exciting opportunities for enhancing fraud detection, organizations must be prepared to tackle these challenges head-on. By addressing data privacy concerns, ensuring seamless integration with existing systems, bridging the skills gap, considering costs, and committing to ongoing maintenance, businesses can position themselves to leverage the full potential of AI in fraud detection.
- What are the main challenges in implementing AI solutions for fraud detection?
The main challenges include data privacy concerns, integration with legacy systems, a skills gap in the workforce, cost considerations, and the need for continuous monitoring and maintenance.
- How can organizations address data privacy concerns?
Organizations can address data privacy concerns by adhering to regulations like GDPR, implementing robust data governance practices, and ensuring transparency in how data is used.
- Why is integration with existing systems a challenge?
Integration is challenging because many businesses use outdated legacy systems that may not be compatible with modern AI technologies, leading to data silos and compatibility issues.
- What is the importance of continuous monitoring of AI systems?
Continuous monitoring is crucial to ensure AI systems remain effective in detecting fraud, as they need regular updates and maintenance to adapt to new fraudulent patterns.
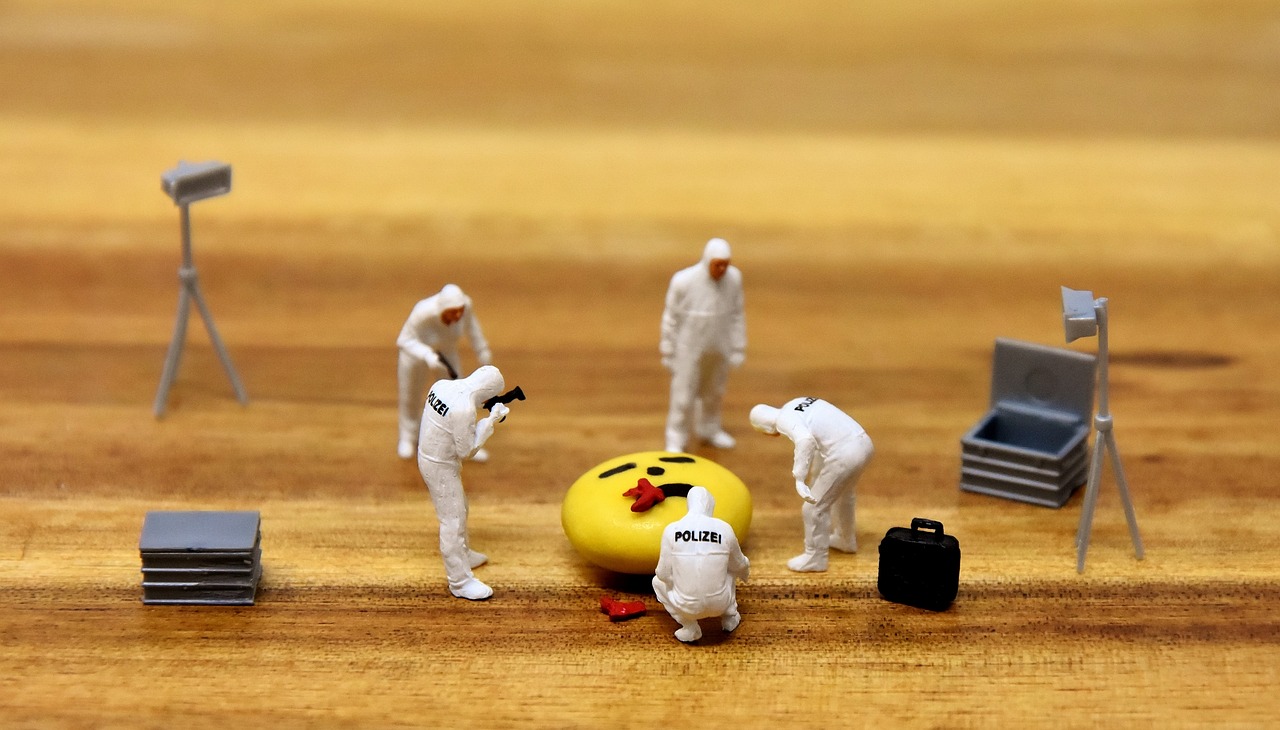
Data Privacy Concerns
As we dive deeper into the world of artificial intelligence and its applications in fraud detection, one crucial aspect that cannot be overlooked is data privacy. With the increasing reliance on AI technologies, organizations are tasked with navigating a complex landscape of ethical considerations and regulatory requirements. The very nature of fraud detection involves analyzing vast amounts of data, often including sensitive personal information. This raises significant questions about how this data is collected, stored, and utilized.
For instance, organizations must ensure that they are compliant with regulations such as the General Data Protection Regulation (GDPR) in Europe and the California Consumer Privacy Act (CCPA) in the United States. These laws impose strict guidelines on data usage, requiring companies to obtain explicit consent from individuals before processing their data. Failure to comply can lead to hefty fines and damage to a company’s reputation.
Moreover, the ethical implications of using AI for fraud detection are profound. While AI can enhance the ability to detect fraudulent activities, it can also lead to potential biases if the algorithms are not designed carefully. For example, if the data used to train these algorithms contains biases, the AI systems may inadvertently target specific demographics, leading to unfair treatment of certain groups. This not only undermines the effectiveness of fraud detection efforts but also erodes trust among consumers.
Organizations must also consider the implications of data breaches. With cyberattacks becoming increasingly sophisticated, the risk of sensitive data falling into the wrong hands is a significant concern. A breach can result in not only financial losses but also a loss of consumer trust that can take years to rebuild. Therefore, implementing robust security measures to protect data is paramount.
To address these concerns, companies can adopt several best practices, including:
- Data Minimization: Collect only the data necessary for fraud detection to reduce exposure.
- Transparency: Clearly communicate to customers how their data will be used and obtain their consent.
- Regular Audits: Conduct regular assessments of data handling practices to ensure compliance with regulations.
- Robust Security Protocols: Implement advanced security measures to protect sensitive information from breaches.
In conclusion, while AI offers tremendous potential for enhancing fraud detection, it also brings forth significant data privacy concerns. Organizations must strike a balance between leveraging AI technologies and protecting consumer data. By prioritizing ethical considerations and adhering to regulatory requirements, businesses can foster a safer environment for their customers while effectively combating fraud.
Q1: What are the main data privacy regulations affecting AI in fraud detection?
A1: The General Data Protection Regulation (GDPR) and the California Consumer Privacy Act (CCPA) are two significant regulations that impact how organizations handle personal data in AI applications.
Q2: How can organizations ensure they are compliant with data privacy laws?
A2: Organizations can ensure compliance by obtaining explicit consent from individuals, conducting regular audits, and implementing data minimization practices.
Q3: What are the risks associated with data breaches in AI fraud detection?
A3: Data breaches can lead to financial losses, legal penalties, and a significant loss of consumer trust, which can take years to recover.
Q4: How can AI systems be biased in fraud detection?
A4: If the training data used for AI systems contains biases, the algorithms may unfairly target specific demographics, leading to discriminatory practices.
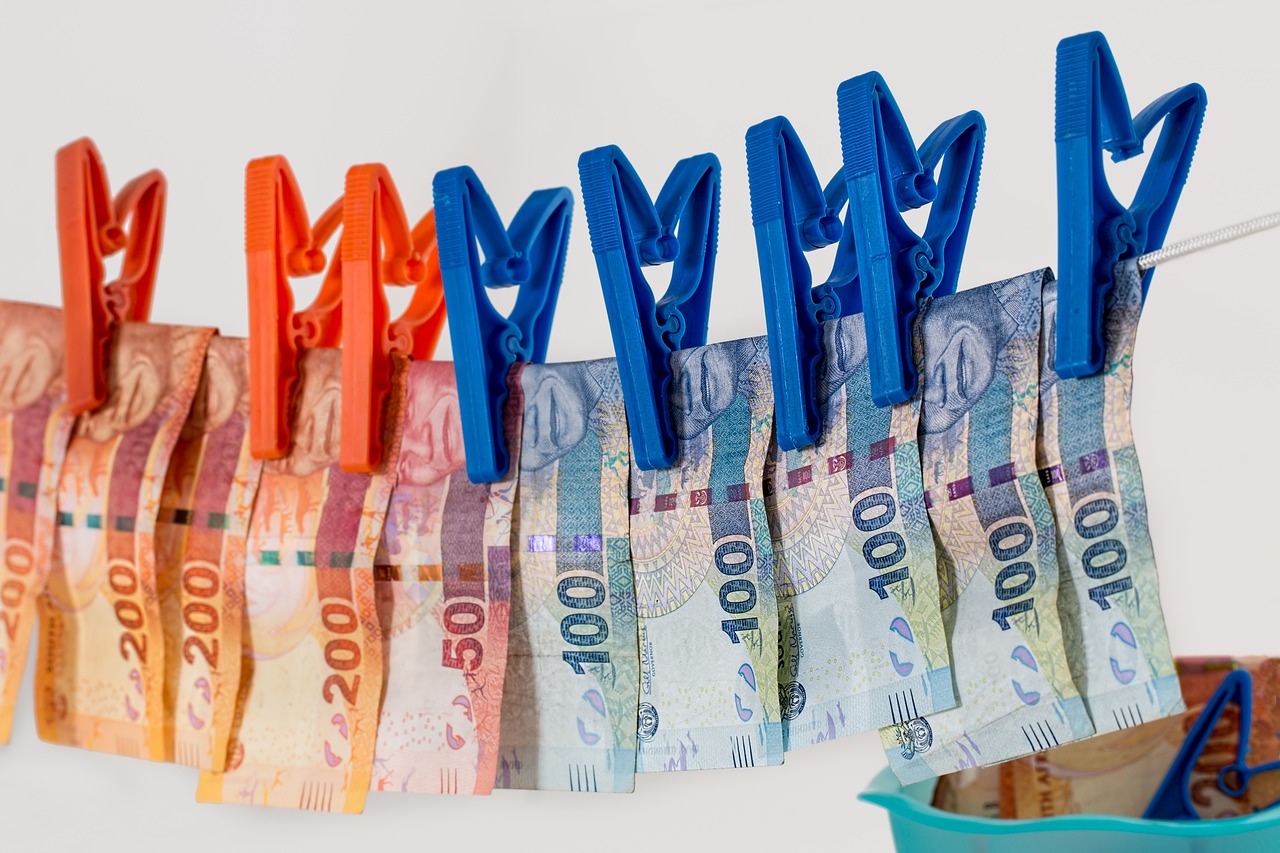
Integration with Existing Systems
Integrating AI solutions with existing systems is like trying to fit a square peg into a round hole—it's not always straightforward, but with the right approach, it can be done effectively. Many organizations face significant challenges when they attempt to incorporate advanced AI technologies into their legacy systems. These legacy systems, often built on outdated technology and processes, can create barriers that hinder the seamless adoption of new AI-driven fraud detection tools.
One of the primary hurdles is the compatibility between new AI solutions and existing infrastructure. Organizations may find that their current systems lack the necessary architecture to support the advanced analytics and processing power required by AI technologies. This can lead to a situation where the AI tools are underutilized or, worse, ineffective. To mitigate this, businesses often need to invest in upgrading their current systems or implementing middleware solutions that can bridge the gap between old and new technologies.
Another challenge is the data silos that many organizations inadvertently create. When data is stored in isolated systems, it becomes difficult for AI algorithms to access and analyze the comprehensive datasets needed for effective fraud detection. Organizations must work to break down these silos by integrating their data sources, ensuring that AI systems have access to a holistic view of transactions and customer behavior.
Moreover, training staff to work with new AI tools is crucial. Employees must be equipped with the skills and knowledge to leverage these technologies effectively. This often requires comprehensive training programs and a cultural shift within the organization to embrace AI as a valuable asset rather than a threat to job security. As organizations navigate these challenges, they must also consider the cost implications of integration. Budget constraints can limit the extent to which companies can upgrade their systems or invest in new technologies.
To address these integration challenges, organizations can adopt a phased approach. This involves implementing AI solutions incrementally, allowing for adjustments and refinements based on real-world performance. By starting small, companies can minimize disruptions to their existing operations while gradually building up their AI capabilities. Additionally, collaboration with technology partners who specialize in AI integration can provide the expertise needed to navigate these complex waters.
In conclusion, while integrating AI solutions with existing systems presents challenges, it is a necessary step for organizations aiming to enhance their fraud detection capabilities. By focusing on compatibility, breaking down data silos, training staff, and adopting a phased approach, businesses can successfully incorporate AI technologies and reap the benefits of improved fraud detection and prevention.
- What are the main challenges of integrating AI into existing systems?
Some of the main challenges include compatibility issues, data silos, training staff, and budget constraints. - How can organizations overcome data silo issues?
Organizations can integrate their data sources to ensure AI systems have access to a comprehensive view of transactions and customer behavior. - What is the benefit of a phased approach to AI integration?
A phased approach allows organizations to implement AI solutions incrementally, minimizing disruptions and allowing for adjustments based on performance.
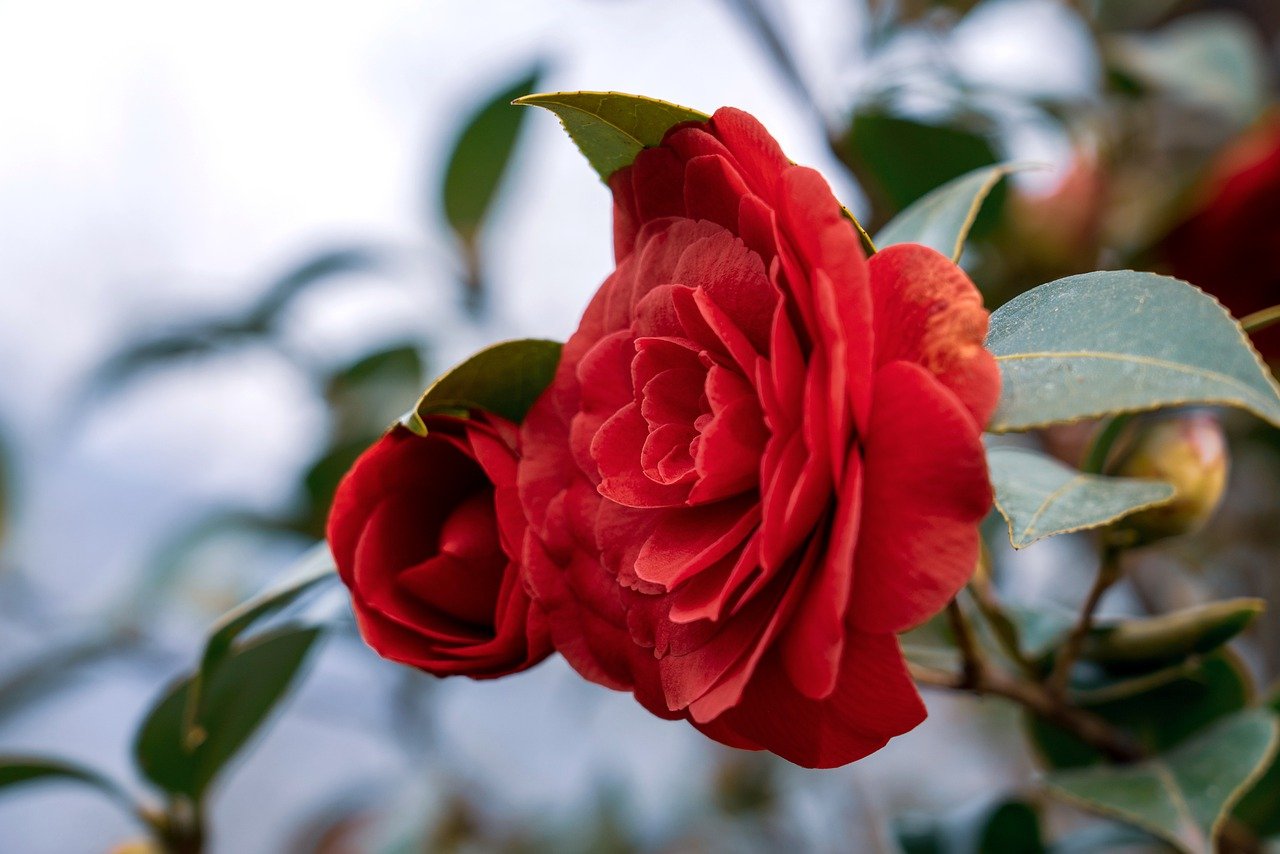
Future Trends in AI Fraud Detection
The landscape of AI in fraud detection is rapidly evolving, driven by advancements in technology and an ever-changing threat environment. As businesses seek to stay one step ahead of fraudsters, several emerging trends are set to redefine the way organizations approach fraud prevention and detection. One of the most significant trends is the increasing use of real-time analytics. With the proliferation of digital transactions, the ability to analyze data as it occurs is becoming crucial. Real-time analytics allows businesses to detect suspicious activities instantly, enabling them to respond swiftly and effectively to potential threats.
Another trend that is gaining traction is the integration of AI with blockchain technology. Blockchain's decentralized and immutable nature can enhance the security of transactions, while AI can analyze patterns within the blockchain to identify anomalies. This combination not only improves fraud detection but also provides a transparent and verifiable method of tracking transactions. As more industries adopt blockchain, the synergy between AI and blockchain will likely become a cornerstone of fraud prevention strategies.
Moreover, the rise of predictive analytics is set to revolutionize how organizations anticipate and mitigate fraud. By leveraging historical data and machine learning algorithms, businesses can forecast potential fraud scenarios and implement proactive measures. Predictive models can analyze consumer behavior, transaction patterns, and even external factors to identify vulnerabilities before they are exploited. This forward-thinking approach not only enhances security but also fosters a culture of vigilance within organizations.
Additionally, the implementation of biometric authentication is on the rise. As traditional password systems become increasingly vulnerable, organizations are turning to biometric solutions such as fingerprint scanning, facial recognition, and voice recognition. These methods not only provide a higher level of security but also improve the overall user experience by streamlining the authentication process.
As we look to the future, the importance of collaboration and data sharing among organizations cannot be overstated. By sharing insights and data regarding fraud attempts, businesses can create a more robust defense system against fraud. Collaborative efforts, such as industry-wide databases that track fraudulent activities, can lead to quicker identification of emerging threats and more effective countermeasures.
Finally, as AI technologies continue to advance, the focus on explainable AI will become increasingly important. Stakeholders want to understand how AI systems make decisions, especially in sensitive areas like fraud detection. By developing transparent AI models, organizations can build trust with consumers and regulatory bodies, ensuring that their fraud detection systems are not only effective but also ethical.
- What is the role of AI in fraud detection?
AI enhances fraud detection by analyzing large datasets to identify patterns and anomalies that may indicate fraudulent activities. - How does predictive analytics help in fraud prevention?
Predictive analytics uses historical data to forecast potential fraud scenarios, allowing businesses to implement proactive measures. - What are the benefits of using biometric authentication?
Biometric authentication provides a higher level of security and improves user experience by simplifying the authentication process. - Why is collaboration important in fraud detection?
Collaboration allows organizations to share insights and data, leading to quicker identification of fraud attempts and more effective defense strategies. - What is explainable AI?
Explainable AI refers to AI systems that provide transparency in their decision-making processes, helping to build trust with users and stakeholders.
Frequently Asked Questions
- What is fraud detection?
Fraud detection is the process of identifying and preventing deceptive activities across various industries. It plays a vital role in protecting businesses and consumers by ensuring that fraudulent transactions are caught early, thus minimizing potential losses.
- How does AI enhance fraud detection?
AI enhances fraud detection by utilizing advanced machine learning algorithms and data analytics to identify patterns and anomalies in large datasets. This technology significantly improves the accuracy and speed of detecting fraudulent activities compared to traditional methods.
- What are supervised learning techniques?
Supervised learning techniques involve training models on labeled datasets to improve their ability to identify fraudulent transactions. By learning from past examples, these models can reduce false positives and enhance the overall accuracy of fraud detection systems.
- What is the significance of unsupervised learning in fraud detection?
Unsupervised learning is crucial for discovering hidden patterns in data without pre-existing labels. This technique can uncover new types of fraud that traditional methods may overlook, making it an essential tool in the evolving landscape of fraud detection.
- What benefits does AI bring to fraud detection?
Implementing AI in fraud detection offers numerous benefits, including improved accuracy, faster response times, and increased efficiency. These advantages not only enhance security but also help in building customer trust by minimizing false declines.
- How can AI reduce costs for organizations?
AI-driven fraud detection systems can significantly reduce costs associated with fraud losses and manual investigations. By automating the detection process, organizations can allocate resources more effectively and lower their overall operational costs.
- What challenges do organizations face when implementing AI solutions?
Organizations often encounter challenges such as data privacy concerns and the complexity of integrating AI solutions with existing legacy systems. Addressing these hurdles is crucial for maximizing the effectiveness of fraud detection efforts.
- What are the future trends in AI fraud detection?
The future of AI in fraud detection is shaped by emerging technologies and trends, such as enhanced predictive analytics, real-time monitoring, and the incorporation of blockchain technology. These advancements will continue to evolve fraud prevention strategies in various sectors.