Artificial Intelligence: The Future of Cognitive Computing
Artificial Intelligence (AI) has emerged as a transformative force in the realm of cognitive computing, reshaping how we interact with technology and each other. Imagine a world where machines not only process information but also understand and respond to human emotions, intentions, and nuances. This is not just a futuristic dream; it is rapidly becoming our reality. As we delve into the intricate relationship between AI and cognitive computing, we will uncover the vast potential applications, the challenges that lie ahead, and the exciting future landscape that these advanced technologies are shaping.
The integration of AI into cognitive computing is akin to adding a brain to a computer. It enables machines to learn from experiences, adapt to new inputs, and perform tasks that typically require human intelligence. From self-driving cars to virtual assistants, AI is infiltrating various facets of our daily lives, enhancing efficiency and creating new opportunities. But what does this mean for the future? Are we prepared for the ethical dilemmas and challenges that come with such powerful technologies? In this article, we will explore these questions and more.
As we navigate this journey, we will also highlight key technologies that are driving cognitive computing, such as natural language processing (NLP), machine learning, and neural networks. Each of these components plays a crucial role in enhancing AI capabilities, allowing for more sophisticated interactions between humans and machines. By understanding these technologies, we can better appreciate how they contribute to the evolution of AI and its applications in various industries.
Furthermore, we will address the ethical considerations that arise from the deployment of AI systems. Issues such as bias, privacy, and accountability are paramount as we integrate these technologies into our lives. How can we ensure that AI serves everyone fairly and ethically? This is a question that we must grapple with as we move forward.
In the subsequent sections, we will explore the evolution of AI, the technologies that fuel cognitive computing, and the implications for our future. We will also delve into specific applications of AI in healthcare and business, highlighting how these advancements can revolutionize patient care and improve operational efficiency. The future of cognitive computing is not just about technology; it’s about enhancing human capabilities and creating a better world for everyone.
- What is cognitive computing? Cognitive computing refers to systems that learn and adapt to human behavior, mimicking human thought processes to solve complex problems.
- How does AI differ from traditional computing? Unlike traditional computing, which follows set instructions, AI can learn from data and improve its performance over time.
- What are the ethical implications of AI? Ethical implications include concerns about bias in algorithms, privacy issues, and the accountability of AI systems in decision-making.
- How is AI impacting healthcare? AI is revolutionizing healthcare through predictive analytics, personalized medicine, and improved diagnostic tools, ultimately enhancing patient care.
- What role does machine learning play in cognitive computing? Machine learning is a subset of AI that enables systems to learn from data without explicit programming, making it essential for cognitive computing applications.
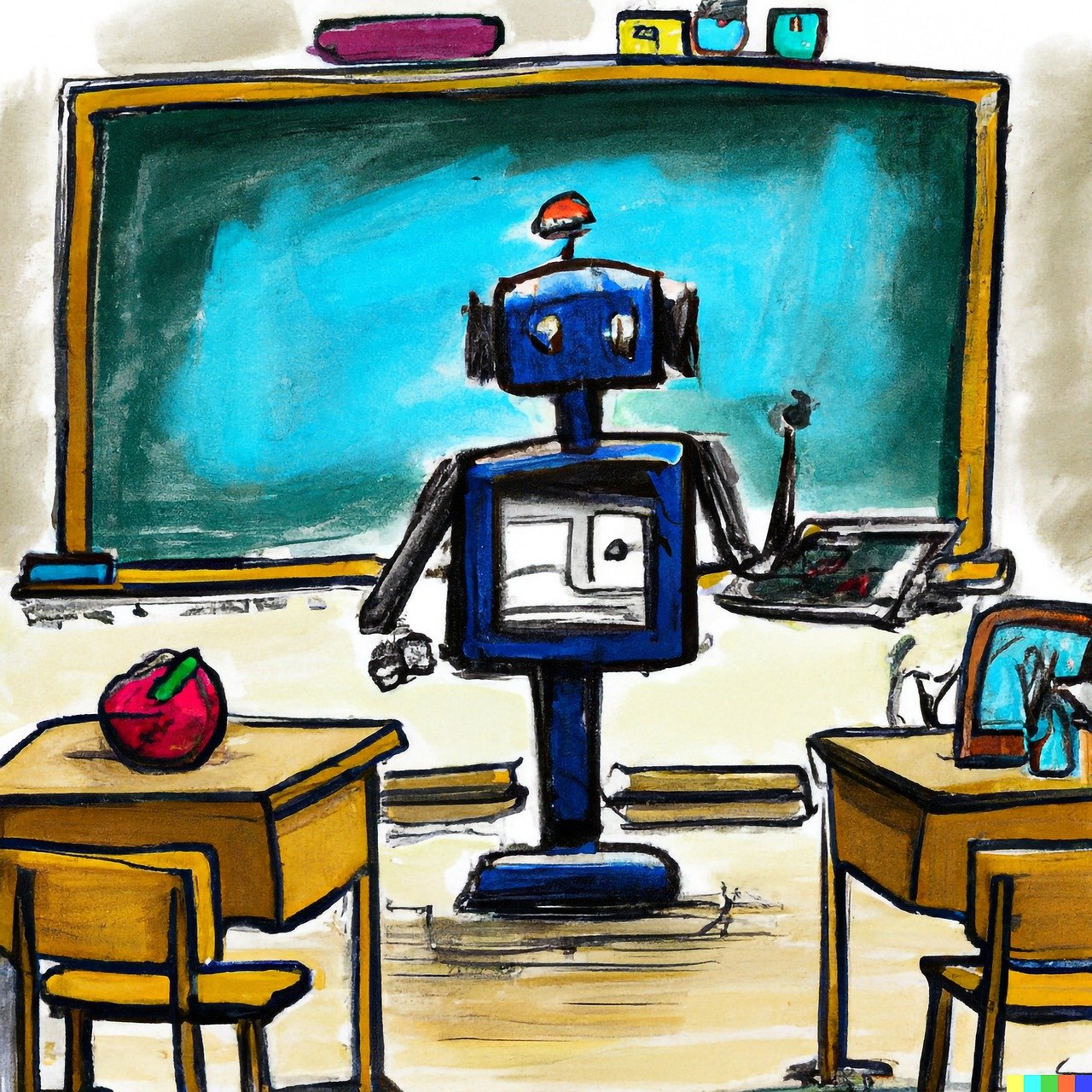
The Evolution of Artificial Intelligence
The journey of artificial intelligence (AI) is nothing short of fascinating. It’s like watching a child grow into a brilliant adult, filled with potential and promise. The roots of AI can be traced back to ancient history, where myths and stories hinted at the creation of intelligent beings. However, the formal study began in the mid-20th century, marking the dawn of a new era in technology. In 1956, at the Dartmouth Conference, the term "artificial intelligence" was coined, setting the stage for decades of research and innovation.
Throughout the years, there have been several significant milestones that have propelled AI into the forefront of technology. The 1960s saw the development of early AI programs, such as ELIZA, a natural language processing computer program that simulated conversation. This was a pivotal moment, as it demonstrated that machines could mimic human interaction, albeit in a very simplistic way.
Fast forward to the 1980s, and we witness the rise of expert systems, which were designed to solve complex problems by mimicking human expertise in specific domains. These systems laid the groundwork for more advanced AI applications, showcasing the potential for machines to assist in decision-making processes across various fields.
As technology advanced, the 1990s introduced a new wave of AI development with the advent of machine learning. Unlike previous AI systems that relied heavily on pre-programmed rules, machine learning allowed computers to learn from data and improve their performance over time. This shift was revolutionary, as it opened the door to more sophisticated applications, such as image recognition and predictive analytics.
Today, we stand on the precipice of a new era in AI, characterized by the rise of deep learning and neural networks. These technologies are inspired by the human brain's structure and function, enabling machines to process vast amounts of information and recognize patterns with remarkable accuracy. The implications are profound, as AI continues to evolve and integrate into our daily lives, transforming industries and reshaping our understanding of what machines can achieve.
In summary, the evolution of artificial intelligence has been marked by significant milestones that reflect both technological advancements and shifts in our understanding of intelligence itself. From its humble beginnings in the 1950s to the sophisticated systems we see today, AI has come a long way. As we look to the future, it’s exciting to imagine where this journey will take us next.
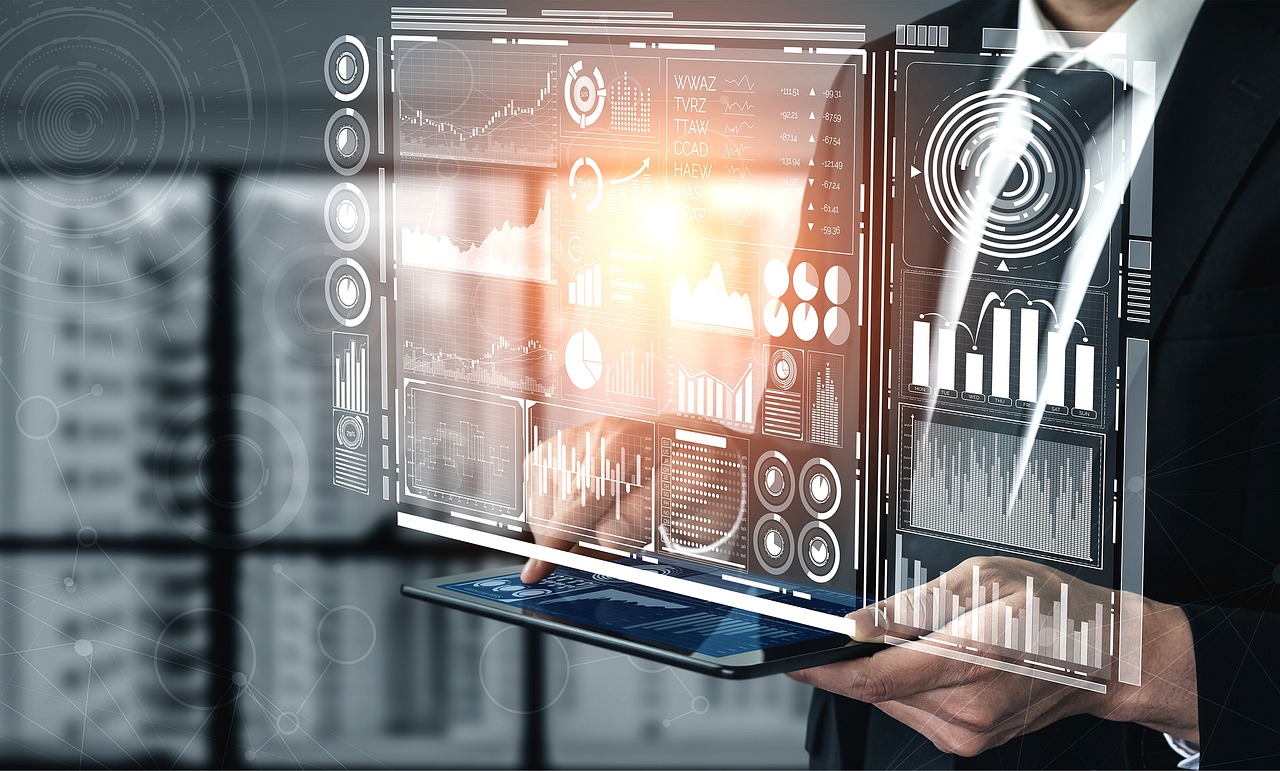
Key Technologies in Cognitive Computing
The landscape of cognitive computing is illuminated by several groundbreaking technologies that enable machines to mimic human thought processes. At the heart of this transformation are natural language processing (NLP), machine learning, and neural networks. These technologies not only enhance the capabilities of artificial intelligence but also pave the way for innovative applications across various sectors.
Natural language processing is one of the most fascinating aspects of cognitive computing. It allows machines to understand, interpret, and respond to human language in a way that feels natural and intuitive. Imagine chatting with a computer that can understand your emotions and respond accordingly! This capability is not just a futuristic dream; it is already being utilized in applications like chatbots and virtual assistants. NLP's ability to analyze text and speech patterns is revolutionizing how businesses interact with their customers, making communication seamless and efficient.
Next, we have machine learning, a subset of artificial intelligence that focuses on the development of algorithms that enable computers to learn from and make predictions based on data. Think of machine learning as a child learning to recognize animals: the more examples it sees, the better it gets at identifying them. This technology is crucial for tasks such as image recognition, recommendation systems, and predictive analytics. By continuously learning from new data, machine learning algorithms can adapt and improve over time, providing more accurate insights and solutions.
Another vital component of cognitive computing is neural networks. These systems are designed to simulate the way human brains operate. They consist of interconnected nodes (or "neurons") that process information in layers. When exposed to vast amounts of data, neural networks can identify patterns and relationships that might be invisible to traditional algorithms. This capability is particularly powerful in areas such as computer vision and speech recognition. As neural networks evolve, they are becoming increasingly adept at handling complex tasks, opening doors to new possibilities in AI.
To better understand the interplay between these technologies, consider the following table that outlines their key features:
Technology | Key Features | Applications |
---|---|---|
Natural Language Processing | Language understanding, Sentiment analysis | Chatbots, Virtual assistants, Translation services |
Machine Learning | Data-driven learning, Predictive analytics | Recommendation systems, Fraud detection, Image recognition |
Neural Networks | Pattern recognition, Deep learning | Computer vision, Speech recognition, Autonomous systems |
As we dive deeper into the world of cognitive computing, it becomes clear that these key technologies are not just standalone entities; they are interwoven, each enhancing the other. For instance, NLP relies on machine learning to improve its understanding of language nuances, while neural networks can enhance machine learning algorithms by providing more sophisticated pattern recognition capabilities. This synergy creates a robust framework for developing intelligent systems that can tackle real-world challenges.
In conclusion, the key technologies in cognitive computing are reshaping our interaction with machines and have the potential to revolutionize industries. As these technologies continue to evolve, they will undoubtedly lead us toward a future where artificial intelligence is not just a tool, but a collaborative partner in our everyday lives.
- What is cognitive computing? Cognitive computing refers to systems that simulate human thought processes in complex situations, using AI technologies to learn and adapt.
- How does natural language processing work? NLP involves the use of algorithms to interpret and generate human language, enabling machines to understand context, sentiment, and intent.
- What are neural networks used for? Neural networks are used for tasks such as image and speech recognition, where they can learn from vast amounts of data to improve accuracy over time.
- Can machine learning be applied in everyday life? Absolutely! Machine learning is used in various applications, from recommendation systems on streaming platforms to fraud detection in banking.
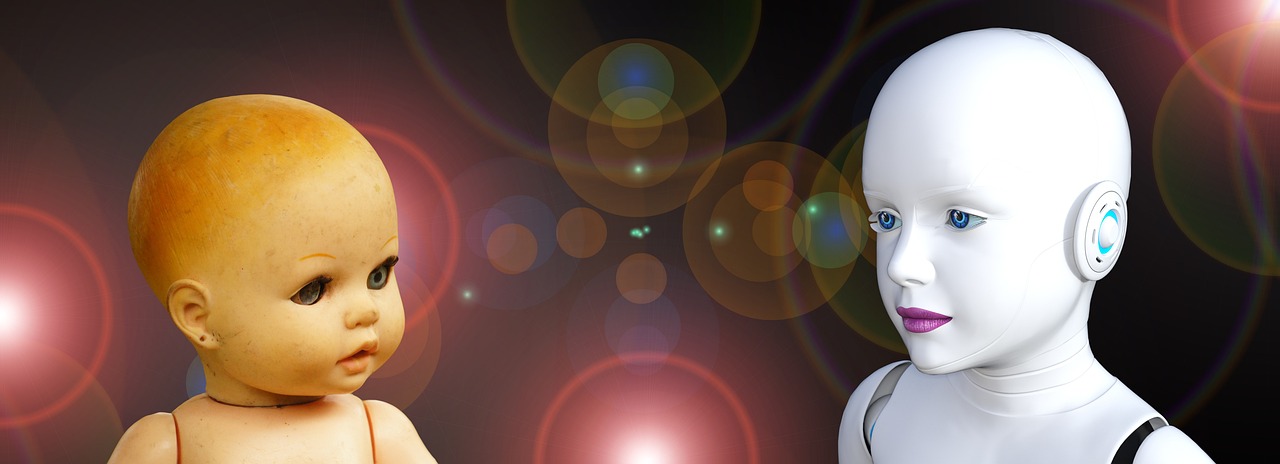
Natural Language Processing
Natural Language Processing, or NLP, is one of the most fascinating branches of artificial intelligence that focuses on the interaction between computers and humans through natural language. Imagine your computer not just being a tool, but a conversational partner that understands your words, nuances, and even emotions. This is not science fiction; it’s the reality that NLP is making possible. By leveraging algorithms and machine learning techniques, NLP enables machines to comprehend, interpret, and generate human language in a way that is both meaningful and contextually relevant.
At its core, NLP aims to bridge the gap between human communication and computer understanding. This involves several complex processes, including syntax analysis, semantic analysis, and contextual understanding. For instance, when you ask your smartphone a question, it doesn’t just recognize the words; it analyzes the structure of your sentence, understands the intent behind your inquiry, and provides a relevant answer. This intricate dance of understanding is what makes NLP so essential in today's digital landscape.
The applications of NLP are vast and varied, transforming how we interact with technology. From chatbots that provide customer support to sentiment analysis tools that gauge public opinion on social media, the impact of NLP is profound. Consider the following applications:
- Chatbots: These AI-powered tools can handle customer inquiries 24/7, providing instant responses and improving user satisfaction.
- Sentiment Analysis: Businesses use NLP to analyze customer feedback, allowing them to gauge public sentiment and adjust their strategies accordingly.
- Language Translation: Tools like Google Translate utilize NLP to break down language barriers, making communication seamless across different cultures.
However, with great power comes great responsibility. NLP is not without its challenges. One of the major hurdles is ambiguity in language. Words can have multiple meanings depending on the context, which can lead to misunderstandings. For example, the word "bank" could refer to a financial institution or the side of a river. Additionally, cultural nuances can complicate NLP systems. What might be a common phrase in one culture could be entirely foreign in another, leading to misinterpretations.
Moreover, the development of sophisticated language models is hindered by the need for extensive and diverse datasets. The more varied the data, the better the model can learn and adapt to different linguistic patterns. This is where ethical considerations come into play, as biases in data can lead to skewed interpretations and unfair outcomes. As we continue to advance in the field of NLP, addressing these challenges will be crucial to harnessing its full potential.
In summary, Natural Language Processing is not just a technological innovation; it is a gateway to more intuitive, human-like interactions with machines. As we continue to refine these systems, the possibilities for enhancing user experience and operational efficiency are limitless. The future of NLP is bright, and its role in shaping the landscape of cognitive computing is undeniable.
Q: What is Natural Language Processing (NLP)?
A: NLP is a field of artificial intelligence that focuses on the interaction between computers and humans through natural language, enabling machines to understand and respond to human language in a meaningful way.
Q: What are some common applications of NLP?
A: Common applications include chatbots, sentiment analysis, language translation, and voice recognition systems, all of which enhance user interaction and operational efficiency.
Q: What challenges does NLP face?
A: NLP faces challenges such as ambiguity in language, cultural nuances, and the need for extensive and diverse datasets to train effective models.
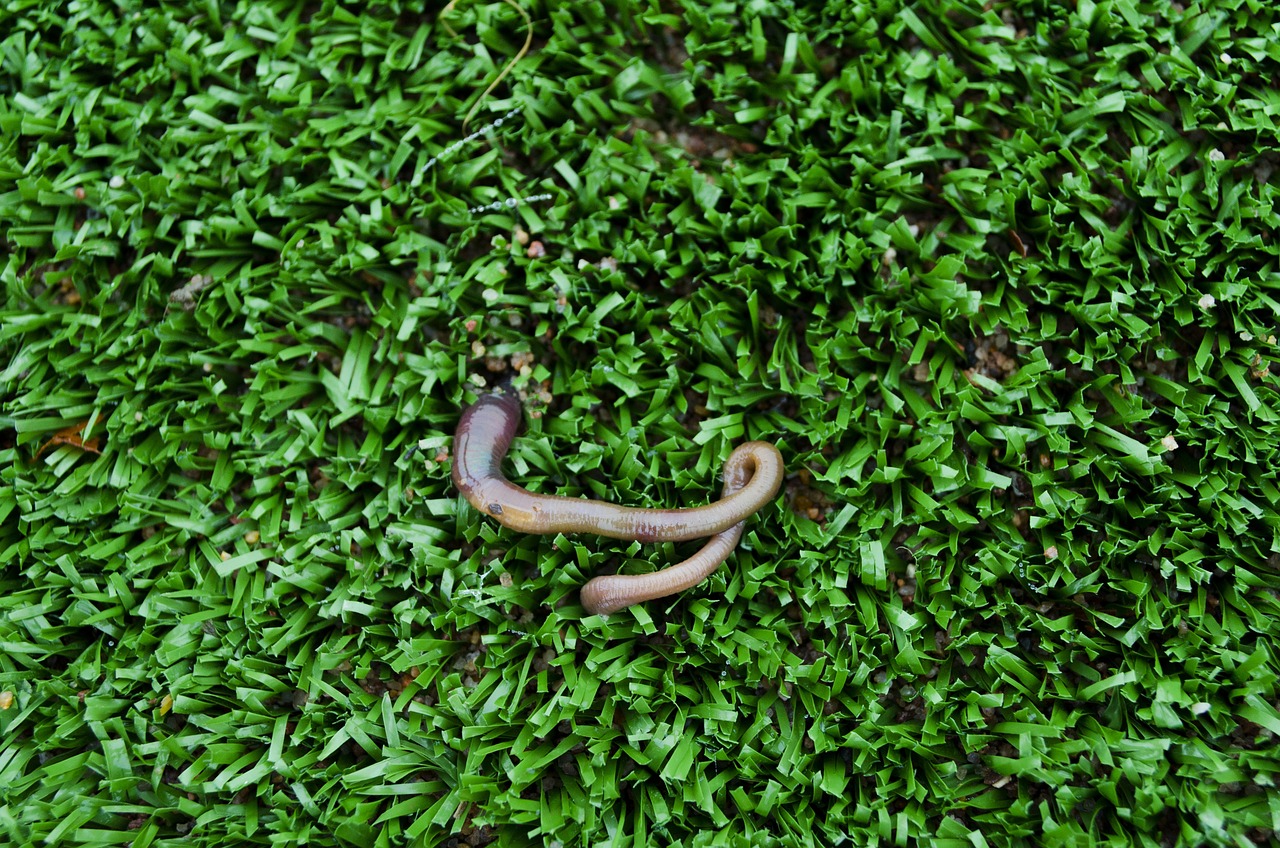
Applications of NLP
Natural Language Processing, or NLP, has become a cornerstone of modern technology, seamlessly integrating into various applications that enhance our daily lives. Imagine chatting with a virtual assistant that understands your commands as if it were a human; that’s the magic of NLP at work. From chatbots that provide customer support to sophisticated systems that analyze sentiments in social media posts, NLP is revolutionizing how we interact with machines and each other.
One of the most prominent applications of NLP is in chatbots. These AI-driven tools are designed to simulate human conversation, allowing businesses to provide 24/7 customer service without the need for human intervention. Imagine a customer visiting your website at 2 AM, seeking help with a product. A well-designed chatbot can respond instantly, answering questions and guiding users through troubleshooting processes, thus enhancing user experience and operational efficiency.
Another exciting application of NLP is in sentiment analysis. Companies are increasingly using NLP algorithms to gauge public opinion on their products or services by analyzing social media posts, reviews, and feedback. By understanding the emotions behind the words, businesses can adjust their strategies accordingly. For instance, if a product receives negative feedback, companies can quickly address the issues, turning potential crises into opportunities for improvement.
Language translation is yet another area where NLP shines. With tools like Google Translate, users can communicate across language barriers, making the world feel smaller and more connected. These translation systems use sophisticated algorithms to understand context and provide accurate translations, allowing for smoother interactions in our globalized society. However, it’s essential to recognize that while NLP can facilitate communication, it’s not without its challenges, especially when dealing with idiomatic expressions or cultural nuances.
Moreover, NLP is making strides in content creation and summarization. Automated writing assistants can help generate articles, reports, and even creative writing pieces by analyzing existing content and producing new material that maintains the original intent. This technology not only saves time but also helps users overcome writer's block. Imagine having a brainstorming partner that can generate ideas and suggestions based on your prompts!
In the healthcare sector, NLP is being utilized to analyze patient records and extract meaningful insights. By processing vast amounts of unstructured data, such as medical notes and research papers, NLP helps healthcare professionals make informed decisions quickly. This capability can lead to improved patient care and more efficient healthcare systems.
In summary, the applications of NLP are vast and varied, impacting numerous sectors and enhancing our interactions with technology. As NLP continues to evolve, we can expect even more innovative solutions that will shape our future in unimaginable ways. The potential is limitless, and we are only scratching the surface of what NLP can achieve.
- What is Natural Language Processing (NLP)?
NLP is a branch of artificial intelligence that focuses on the interaction between computers and humans through natural language. It enables machines to understand, interpret, and respond to human language in a valuable way.
- How is NLP used in customer service?
NLP is commonly used in chatbots that provide instant responses to customer inquiries, helping businesses enhance customer satisfaction and operational efficiency.
- Can NLP analyze sentiments?
Yes, NLP can analyze sentiments by evaluating text data from social media, reviews, and feedback to determine the emotional tone behind the words.
- What are the challenges of NLP?
Challenges in NLP include understanding context, handling ambiguity, and addressing cultural nuances, which can complicate language processing tasks.
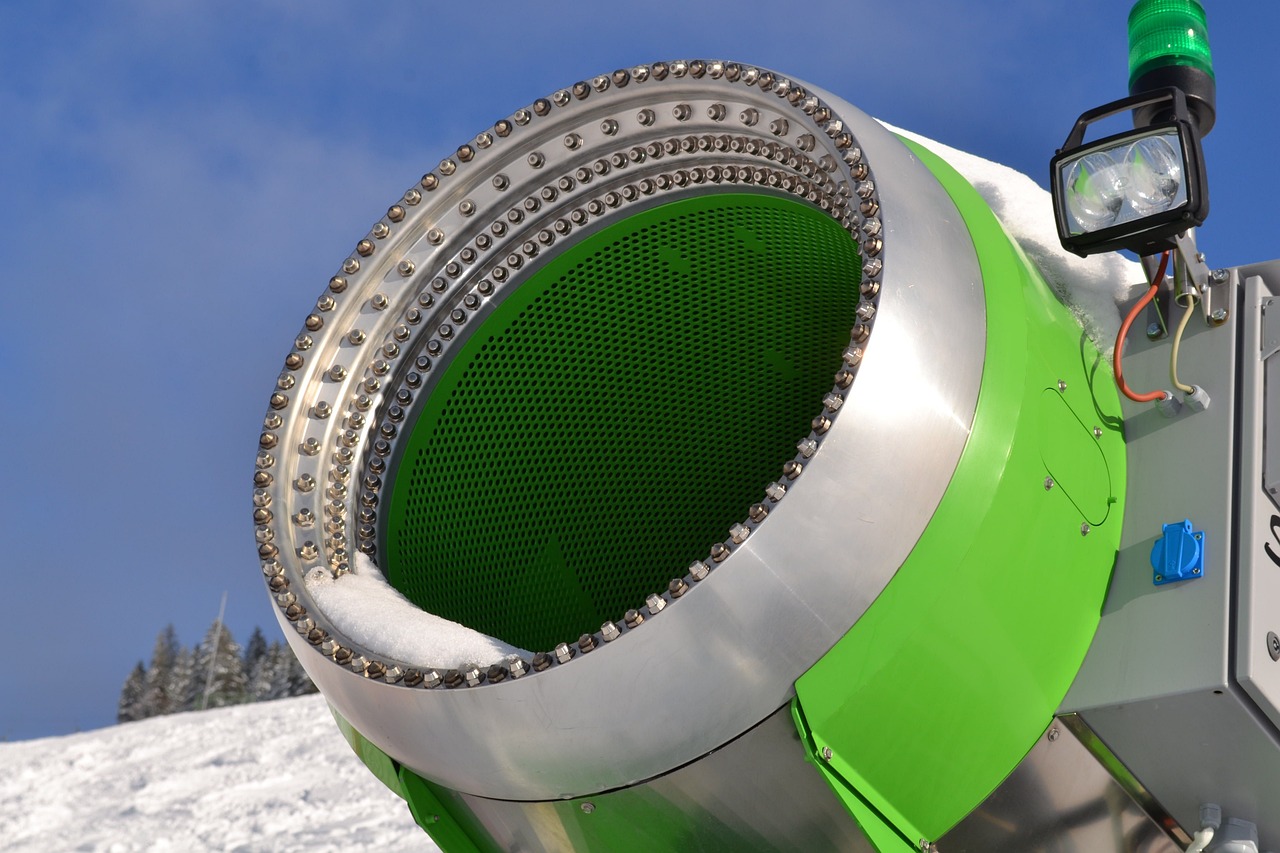
Challenges in NLP
Natural Language Processing (NLP) has made significant strides in recent years, but it still faces a myriad of challenges that can hinder its effectiveness and reliability. One of the primary obstacles is ambiguity. Human language is inherently complex and often vague; words can have multiple meanings depending on context. For example, consider the word "bank." It could refer to a financial institution or the side of a river. This ambiguity can confuse NLP systems, leading to misunderstandings and incorrect interpretations.
Another challenge lies in context understanding. Humans are adept at picking up on subtle cues and context that inform meaning, but machines struggle with this aspect. Take a simple sentence like, "I saw her duck." Without context, it's unclear whether "duck" refers to the action of lowering one's head or the bird itself. NLP systems need to develop a more nuanced understanding of context to accurately process and respond to human language.
Moreover, cultural nuances pose a significant hurdle. Language is not just about words; it reflects the culture and experiences of its speakers. Idioms, slang, and cultural references can vary widely across different regions and communities. For instance, the phrase "kick the bucket" means to die in English-speaking cultures, but it may not translate well in other languages or contexts. This cultural disconnect can lead to misinterpretations and ineffective communication.
Additionally, there is the issue of data quality. NLP systems rely heavily on large datasets for training, and if these datasets contain biases or inaccuracies, the resulting models will likely reflect those flaws. For example, if an NLP system is trained predominantly on text from a specific demographic, it may struggle to understand or accurately process language from other groups. This can lead to skewed results and perpetuate existing biases.
Lastly, the rapid evolution of language, especially with the rise of social media and digital communication, presents a continuous challenge. New slang, abbreviations, and even entirely new languages (like emojis) emerge frequently, and keeping NLP systems up to date with these changes can be a daunting task. As language evolves, so must the algorithms that process it, making ongoing training and adaptation essential.
In summary, while NLP has made remarkable progress, it is essential to address these challenges to enhance its capabilities further. By improving ambiguity resolution, context understanding, cultural sensitivity, data quality, and adaptability to language evolution, we can pave the way for more effective and sophisticated NLP systems that can truly understand and interact with human language.
- What is NLP? Natural Language Processing (NLP) is a branch of artificial intelligence that focuses on the interaction between computers and humans through natural language.
- What are the main challenges of NLP? The main challenges include ambiguity, context understanding, cultural nuances, data quality, and the rapid evolution of language.
- How does ambiguity affect NLP? Ambiguity can lead to misinterpretations in NLP systems, as words can have multiple meanings depending on the context in which they are used.
- Why is cultural nuance important in NLP? Cultural nuances are important because they influence how language is used and understood, and failing to account for them can lead to miscommunication.
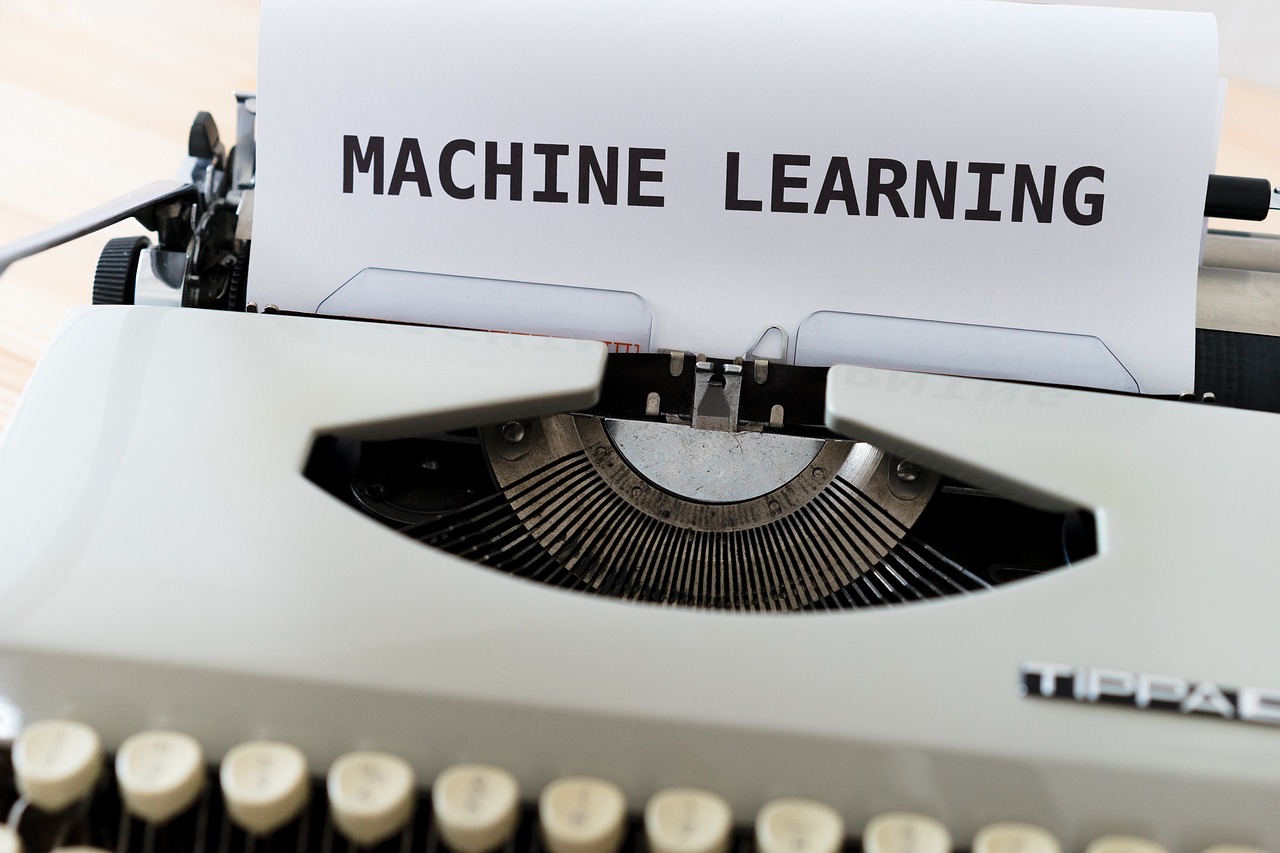
Machine Learning Techniques
Machine learning, a subset of artificial intelligence, is revolutionizing the way we process and analyze data. It empowers systems to learn from data patterns and improve their performance over time without explicit programming. Imagine teaching a child to recognize animals: initially, they might struggle to differentiate between a dog and a cat, but with exposure to various examples, they gradually learn to identify them accurately. This is the essence of machine learning—using data to train algorithms to make informed decisions.
There are several key techniques within machine learning that serve as the backbone of cognitive computing. These techniques can be broadly categorized into three main types: supervised learning, unsupervised learning, and reinforcement learning. Each technique has its unique approach to learning from data, and understanding these can help us appreciate the vast potential of machine learning in various applications.
Supervised learning is like having a teacher guiding the learning process. In this technique, algorithms are trained on labeled data, meaning that the input data is paired with the correct output. For instance, if we want to train a model to recognize images of cats and dogs, we would provide it with a dataset of labeled images indicating which are cats and which are dogs. The algorithm learns from these examples, enabling it to predict the labels of new, unseen images. This method is widely used in applications such as spam detection, fraud detection, and image classification.
On the other hand, unsupervised learning operates without labeled outputs. Here, the algorithm is tasked with identifying patterns and structures in the data on its own. Think of it as exploring a new city without a map; you wander around and gradually learn the layout based on your observations. This technique is particularly useful for clustering similar data points and discovering hidden patterns. Common applications include customer segmentation in marketing, anomaly detection in network security, and even recommendation systems that suggest products based on user behavior.
Lastly, reinforcement learning is akin to training a pet with rewards and punishments. In this approach, an agent learns to make decisions by interacting with its environment, receiving feedback in the form of rewards or penalties. The goal is to maximize cumulative rewards over time. This technique has gained significant traction in areas such as robotics, gaming, and autonomous vehicles. For example, in a game, the algorithm learns to play by receiving points for winning and losing points for mistakes, continuously refining its strategy to improve performance.
The potential of machine learning techniques is vast and ever-expanding. As we continue to develop more sophisticated algorithms and harness the power of big data, the applications of these techniques will only grow. From enhancing customer experiences to driving innovations in healthcare, machine learning is at the forefront of the cognitive computing revolution.
- What is the difference between supervised and unsupervised learning? Supervised learning uses labeled data to train algorithms, while unsupervised learning identifies patterns in unlabeled data.
- How does reinforcement learning work? Reinforcement learning involves an agent that learns to make decisions by receiving rewards or penalties based on its actions.
- What are some common applications of machine learning? Machine learning is used in various fields, including finance for fraud detection, healthcare for predictive analytics, and marketing for customer segmentation.
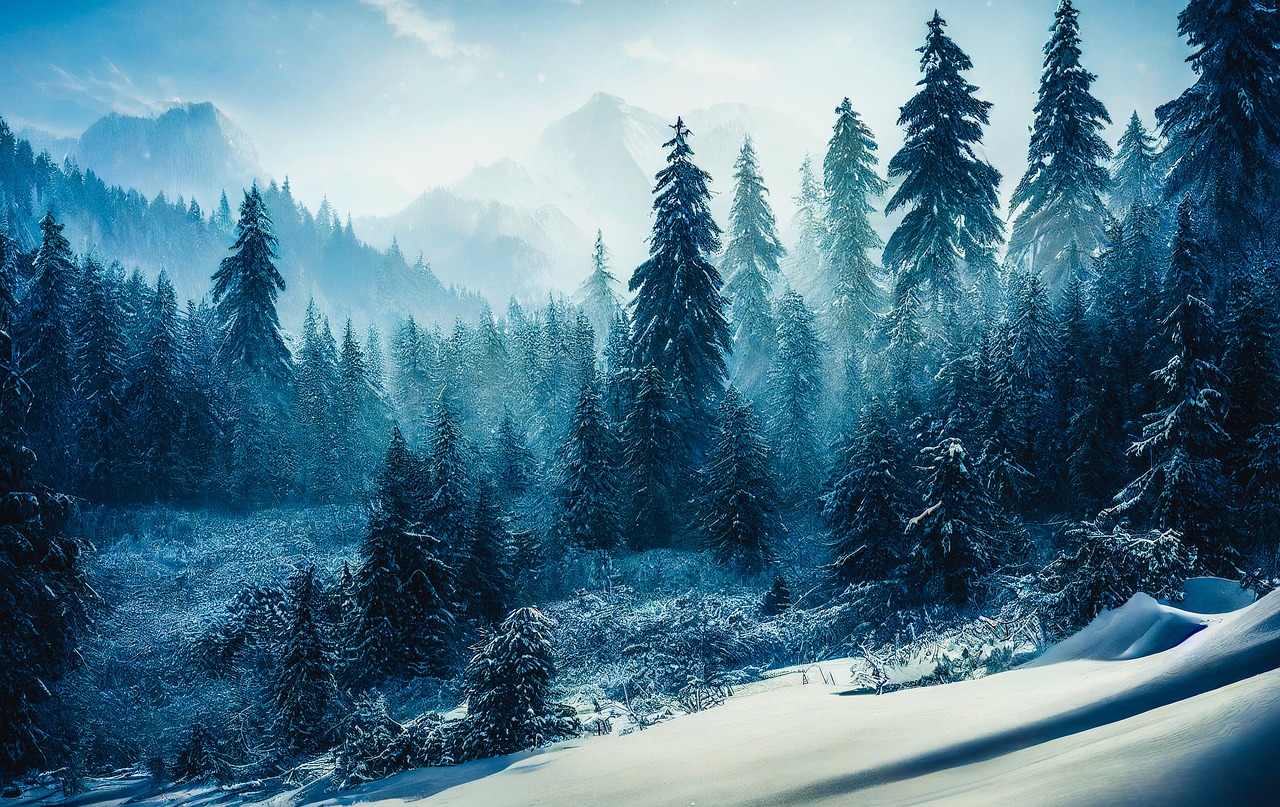
Ethical Considerations in AI
As we leap into the age of artificial intelligence, the conversation surrounding its ethical implications is more crucial than ever. With great power comes great responsibility, and AI is no exception. The deployment of cognitive computing systems raises several ethical concerns that we must address to ensure a future where technology serves humanity positively.
One of the most pressing issues is the potential for bias in AI algorithms. Bias can creep into these systems through various channels, including the data used for training and the design of the algorithms themselves. For instance, if an AI system is trained on data that reflects existing societal biases, it can perpetuate and even exacerbate those biases in its decision-making processes. This is particularly concerning in fields like hiring, law enforcement, and lending, where biased decisions can have significant real-world consequences.
To combat this, it's essential to create fair and unbiased systems. This involves:
- Regularly auditing AI systems for bias.
- Ensuring diverse datasets that represent various demographics.
- Incorporating transparency in how algorithms make decisions.
Another critical ethical consideration revolves around privacy and data security. As AI systems often rely on vast amounts of personal data to function effectively, the risks associated with data breaches and misuse become a significant concern. Users must trust that their information is handled responsibly and securely. Therefore, organizations must implement robust frameworks to protect user data, ensuring compliance with regulations like GDPR and CCPA.
Moreover, the issue of accountability in AI systems cannot be overlooked. When AI systems make decisions that lead to negative outcomes, who is responsible? Is it the developers, the organizations that deploy the technology, or the AI itself? This ambiguity can lead to complications in legal and ethical accountability. Establishing clear guidelines and frameworks is essential to address these questions and ensure responsible AI usage.
In conclusion, as we harness the power of artificial intelligence, we must tread carefully, ensuring that we address these ethical considerations head-on. By fostering a culture of responsibility and transparency, we can build AI systems that not only advance technology but also uphold our shared values and ethics.
- What are the main ethical concerns regarding AI?
The primary concerns include bias in algorithms, privacy and data security, and accountability for AI-driven decisions. - How can we mitigate bias in AI systems?
Regular audits, diverse datasets, and transparency in algorithms can help reduce bias. - Why is data privacy important in AI?
AI systems often require personal data, and protecting this information is crucial for maintaining user trust and compliance with regulations. - Who is responsible for AI decisions?
Accountability can be complex; it often involves developers, organizations, and sometimes the AI itself, necessitating clear guidelines.
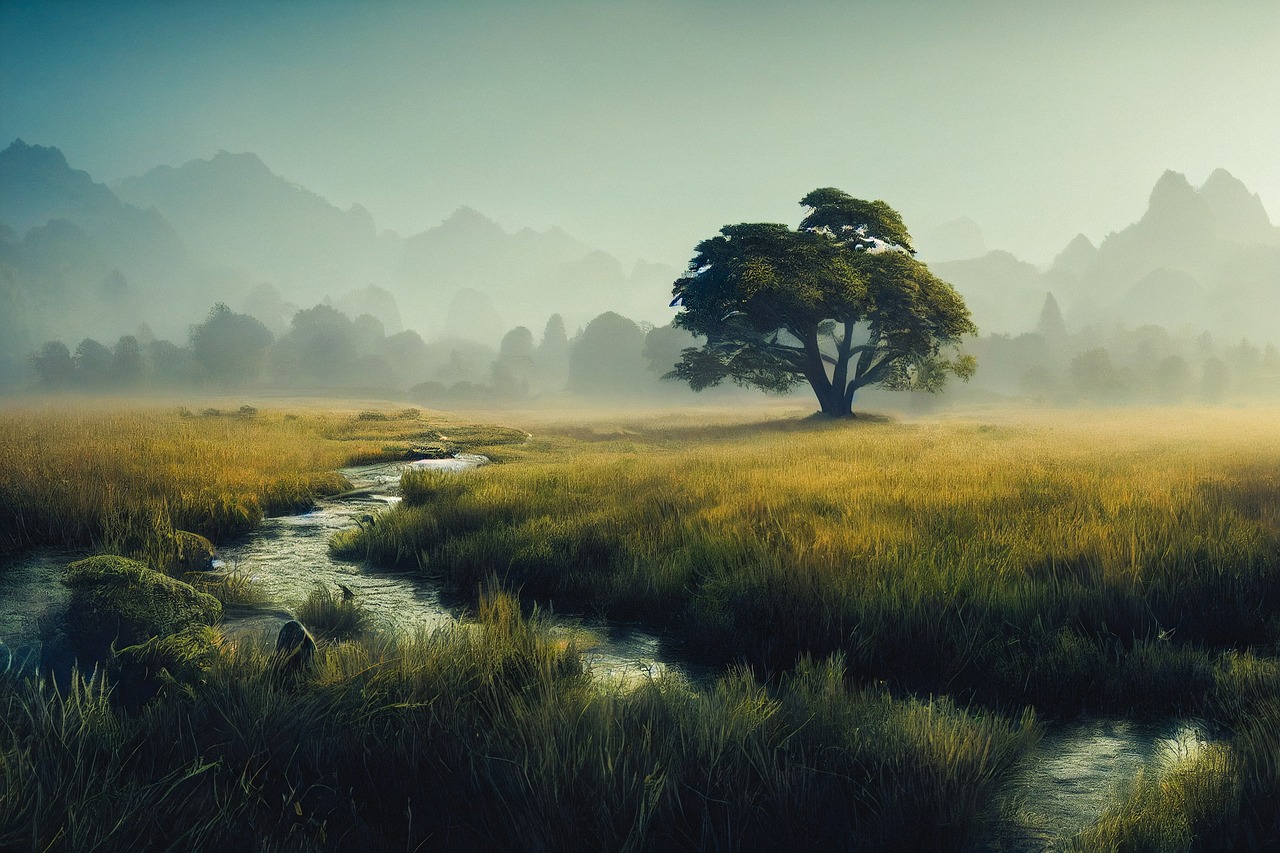
Bias in AI Algorithms
As artificial intelligence continues to evolve and integrate into various sectors, one of the most pressing issues we face is the presence of . This bias can arise from a multitude of sources, including the data used to train these systems, the design of the algorithms themselves, and even the societal norms that inform the development process. The implications of biased AI can be profound, leading to unfair treatment in critical areas such as hiring, lending, and law enforcement. Imagine a world where a machine, programmed to make decisions, inadvertently perpetuates historical prejudices. This is not just a theoretical concern; it is a reality that we must confront.
One key factor contributing to bias in AI is the data used for training. If the training data reflects existing inequalities or is not representative of the broader population, the AI will likely learn and replicate these biases. For example, a hiring algorithm trained predominantly on data from a specific demographic may favor candidates from that group, leading to discrimination against others. To illustrate this point, consider the following table:
Training Data Source | Potential Bias | Impact |
---|---|---|
Historical hiring records | Gender and racial bias | Exclusion of qualified candidates from underrepresented groups |
Facial recognition datasets | Racial bias | Higher error rates for individuals of certain races |
Medical datasets | Gender bias | Misdiagnosis or inadequate treatment recommendations for women |
Moreover, the algorithms themselves can introduce bias through their design. Developers may unintentionally embed their own biases into the algorithms, which can amplify existing societal disparities. This is particularly concerning in applications like criminal justice, where biased algorithms can lead to disproportionate sentencing or surveillance of specific communities. The challenge here is not only technical but also ethical, as it raises questions about accountability and responsibility.
To combat bias in AI algorithms, several strategies can be employed:
- Diverse Data Collection: Ensuring that training datasets are representative of the entire population can help mitigate bias.
- Bias Detection Tools: Implementing tools that can identify and rectify biases within algorithms is crucial for fair AI.
- Ethical Guidelines: Establishing clear ethical standards for AI development can guide developers in creating fair systems.
As we move forward, it is essential for stakeholders—developers, businesses, and policymakers—to collaborate in addressing these biases. By fostering a culture of transparency and accountability, we can create AI systems that not only function effectively but also uphold the principles of fairness and justice. The future of AI should not just be about efficiency and profitability; it must also prioritize equity and inclusion, ensuring that technology serves the best interests of all.
1. What is bias in AI algorithms?
Bias in AI algorithms refers to systematic errors that result in unfair treatment of certain groups, often due to skewed training data or flawed algorithm design.
2. How does bias affect decision-making in AI?
Bias can lead to incorrect or unjust outcomes in decision-making processes, such as hiring, lending, or law enforcement, ultimately perpetuating existing inequalities.
3. What can be done to reduce bias in AI?
Strategies such as diverse data collection, bias detection tools, and ethical guidelines can help mitigate bias in AI systems.
4. Why is it important to address bias in AI?
Addressing bias is crucial for ensuring that AI technologies are fair, equitable, and trustworthy, which is essential for their acceptance and effectiveness in society.
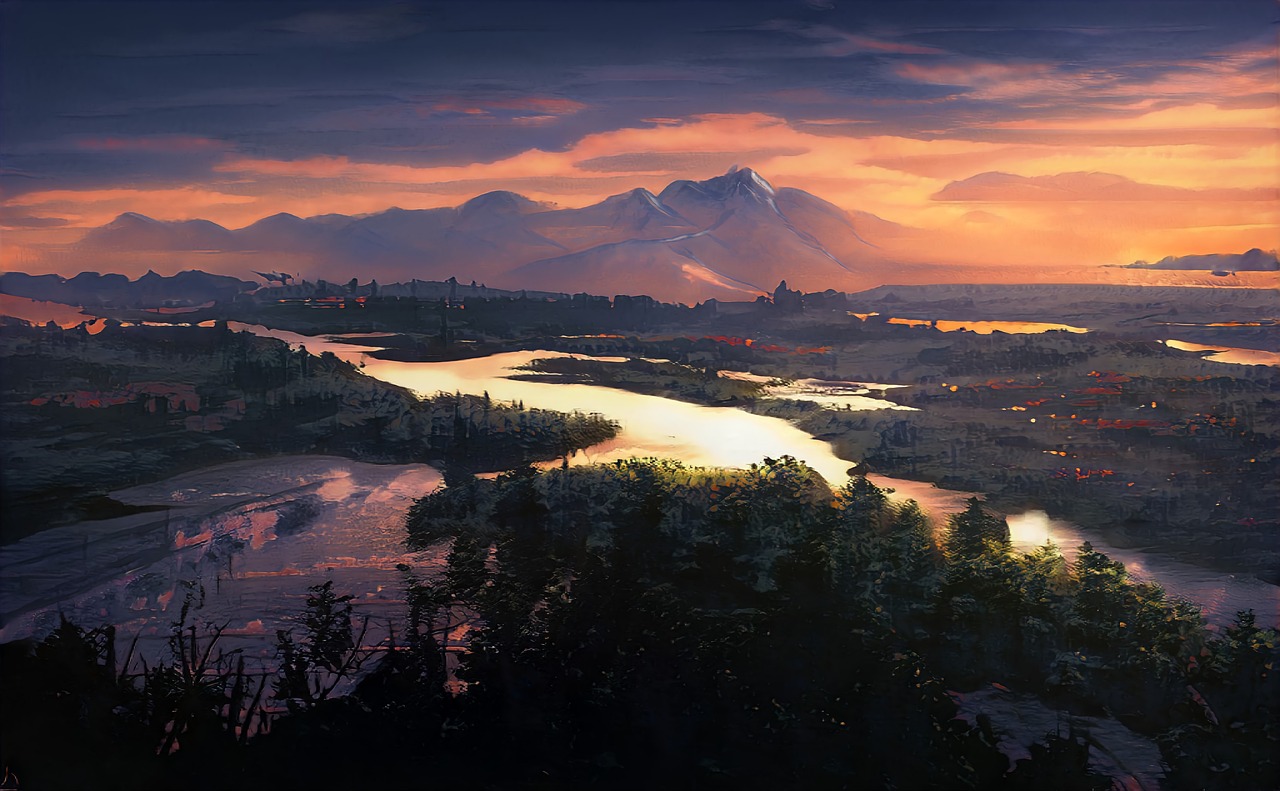
Privacy and Data Security
In our increasingly digital world, the importance of privacy and data security cannot be overstated, especially when it comes to artificial intelligence (AI) applications. As AI systems become more integrated into our daily lives, they collect vast amounts of data, raising significant concerns about how this information is used and protected. Imagine handing over your personal diary to a stranger; that’s how it feels when we share our data without fully understanding the implications.
One of the primary challenges in ensuring privacy is the sheer volume of data AI systems require. These systems analyze user behavior, preferences, and even biometric data to deliver personalized experiences. However, this data collection can lead to vulnerabilities that could be exploited by malicious actors. For instance, a breach in a healthcare AI system could expose sensitive patient records, leading to identity theft or discrimination.
To address these challenges, organizations must implement robust frameworks that prioritize data protection. This includes adopting strong encryption methods, conducting regular security audits, and ensuring compliance with regulations such as the General Data Protection Regulation (GDPR). These measures not only protect user data but also build trust with consumers, who are more likely to engage with AI systems that demonstrate a commitment to privacy.
Moreover, educating users about their rights and the potential risks associated with AI technologies is essential. Users should be aware of how their data is being used and have the ability to opt-out of data collection practices. Transparency is key; companies should provide clear explanations about their data handling processes.
As we move forward, the dialogue around privacy and data security in AI will continue to evolve. Here are some critical elements to consider:
- Data Minimization: Collect only the data that is necessary for the intended purpose, reducing the risk of exposure.
- Access Control: Limit access to sensitive data to only those who need it, implementing strict user authentication protocols.
- Regular Audits: Conduct frequent assessments of data security practices to identify vulnerabilities and ensure compliance with regulations.
As AI technology advances, so too must our approaches to privacy and data security. Organizations must remain vigilant and proactive, adapting to new challenges as they arise. The future of AI depends not just on innovation but also on our ability to safeguard the personal information of individuals.
What are the main privacy concerns associated with AI?
Privacy concerns primarily revolve around data collection, storage, and usage. AI systems often require access to large datasets, which can include sensitive personal information. Without proper safeguards, this data can be misused or exposed during a security breach.
How can individuals protect their privacy when using AI applications?
Individuals can protect their privacy by being cautious about the information they share, using privacy settings on applications, and opting out of data collection where possible. It's also crucial to stay informed about the privacy policies of the services they use.
Are there regulations in place to protect data privacy in AI?
Yes, regulations like the GDPR in Europe and various state-level laws in the U.S. aim to protect data privacy. These laws require organizations to be transparent about their data practices and provide individuals with rights regarding their personal information.
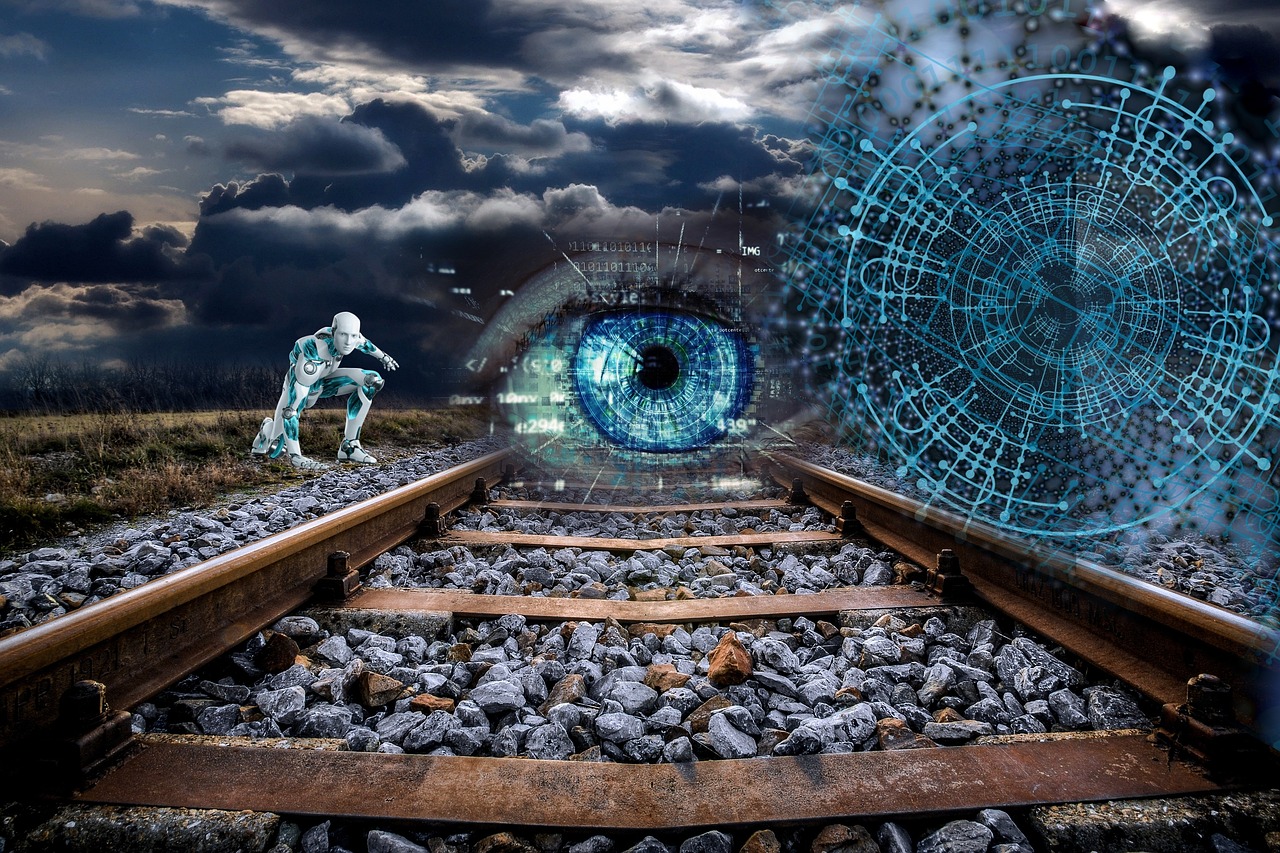
The Future of Cognitive Computing
The future of cognitive computing is not just a distant dream; it's unfolding right before our eyes, and it's nothing short of exhilarating. As we stand on the brink of this technological revolution, we can only imagine the myriad of possibilities that artificial intelligence (AI) will bring to our lives. Cognitive computing, powered by AI, is set to redefine how we interact with technology, solve complex problems, and make decisions. Imagine a world where machines not only process data but also understand context, emotions, and nuances of human interaction. This is the future we are heading towards!
One of the most exciting aspects of cognitive computing is its potential to integrate seamlessly into our daily lives. From smart homes that anticipate our needs to virtual assistants that understand our preferences, the applications are endless. For instance, consider how AI can revolutionize the way we manage our health. With advancements in predictive analytics, cognitive computing can analyze vast amounts of health data to predict potential health issues before they arise. This proactive approach can lead to better health outcomes, ultimately saving lives.
Moreover, the impact of cognitive computing on various industries is profound. In healthcare, AI can assist doctors in diagnosing diseases more accurately by analyzing medical images and patient data with incredible precision. In finance, cognitive computing can enhance fraud detection systems, making transactions safer and more secure. The retail sector is also set to benefit, with AI-driven analytics providing insights into consumer behavior that can lead to personalized shopping experiences. The potential is enormous, and businesses that embrace these technologies will likely gain a competitive edge.
However, with great power comes great responsibility. As we explore the future of cognitive computing, it is crucial to consider the ethical implications of these advancements. How do we ensure that AI systems are fair and unbiased? What measures can we take to protect user privacy and data security? These are not just theoretical questions; they are challenges that we must address as we move forward. Establishing robust frameworks for accountability and transparency in AI deployment will be essential to building trust with users.
In summary, the future of cognitive computing is bright and full of potential. As we continue to innovate and integrate AI into various aspects of our lives, we must remain vigilant about the ethical implications and strive to create systems that enhance human capabilities while safeguarding our values. The journey ahead is filled with surprises and opportunities, and it is up to us to shape it wisely.
- What is cognitive computing? Cognitive computing refers to systems that simulate human thought processes in complex situations, using technologies like AI, machine learning, and natural language processing.
- How will AI impact the job market? While AI may automate certain tasks, it will also create new job opportunities in tech development, data analysis, and more, requiring a shift in skills and education.
- What are the ethical concerns surrounding AI? Ethical concerns include bias in algorithms, privacy issues, and the need for accountability in AI decision-making processes.
- How is AI being used in healthcare? AI is used in healthcare for predictive analytics, personalized medicine, and improving diagnostic accuracy, ultimately enhancing patient care.
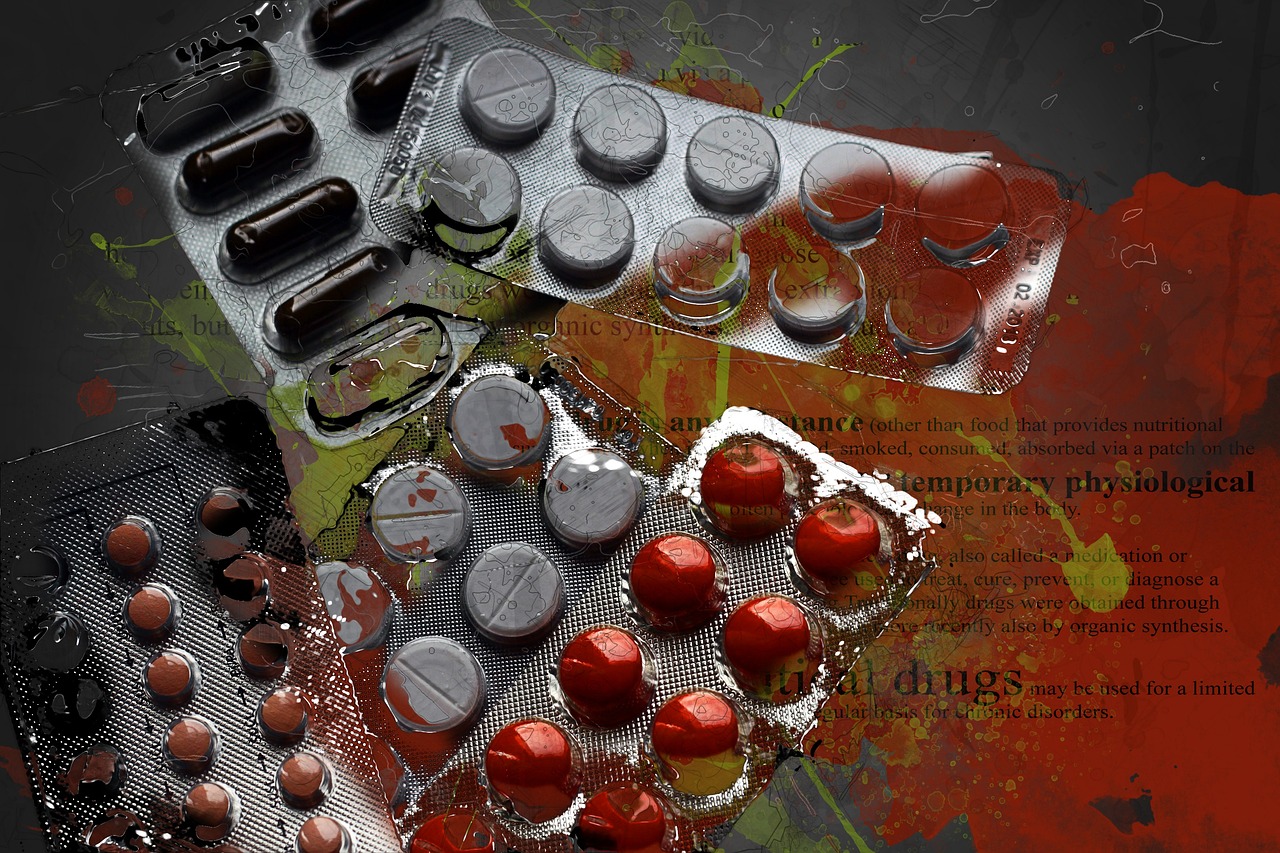
AI in Healthcare
Artificial Intelligence (AI) is not just a buzzword; it’s a game changer in the healthcare industry. Imagine a world where your doctor is augmented by a super-intelligent assistant that can analyze vast amounts of data in seconds. Sounds like science fiction? Well, it’s happening right now! The integration of AI in healthcare is transforming how we diagnose, treat, and manage diseases. From predictive analytics to personalized medicine, AI is paving the way for a future where healthcare is not only more efficient but also more tailored to individual needs.
One of the most exciting applications of AI in healthcare is predictive analytics. By analyzing historical patient data, AI algorithms can identify patterns and predict potential health risks before they become critical. For instance, AI can assess a patient’s medical history, lifestyle choices, and genetic factors to forecast the likelihood of developing conditions like diabetes or heart disease. This proactive approach allows healthcare providers to intervene early, potentially saving lives.
Another area where AI shines is in personalized medicine. Every patient is unique, and AI helps in creating treatment plans that are specifically tailored to the individual. By analyzing data from various sources—such as genetic tests, lab results, and even lifestyle factors—AI can recommend the most effective therapies for each patient. This not only enhances treatment efficacy but also minimizes the risk of adverse effects, making healthcare safer and more effective.
Furthermore, AI is revolutionizing diagnostics. Traditional diagnostic methods can be time-consuming and sometimes inaccurate. However, AI systems can analyze medical images, such as X-rays or MRIs, with incredible precision. For example, AI algorithms can detect anomalies that may be missed by the human eye, leading to earlier and more accurate diagnoses of conditions like cancer. This capability not only streamlines the diagnostic process but also significantly improves patient outcomes.
Despite these advancements, the integration of AI in healthcare is not without its challenges. Issues such as data privacy, algorithmic bias, and the need for rigorous testing and validation of AI systems must be addressed to ensure that these technologies are both safe and effective. The healthcare industry must navigate these hurdles while also ensuring that AI complements the work of healthcare professionals, rather than replacing the human touch that is so vital in patient care.
In summary, AI is set to revolutionize healthcare in ways we are just beginning to understand. By enhancing predictive analytics, personalizing treatment plans, and improving diagnostic accuracy, AI is making healthcare more efficient and effective. As we continue to embrace these technologies, the potential for improved patient care is limitless. The future of healthcare is bright, and AI is at the forefront of this transformation!
- What are the main benefits of AI in healthcare? AI enhances predictive analytics, personalizes treatment plans, and improves diagnostic accuracy.
- Are there any risks associated with AI in healthcare? Yes, challenges include data privacy, algorithmic bias, and the need for thorough validation of AI systems.
- How does AI improve patient outcomes? By providing early diagnosis and tailored treatment plans, AI can lead to better health outcomes for patients.
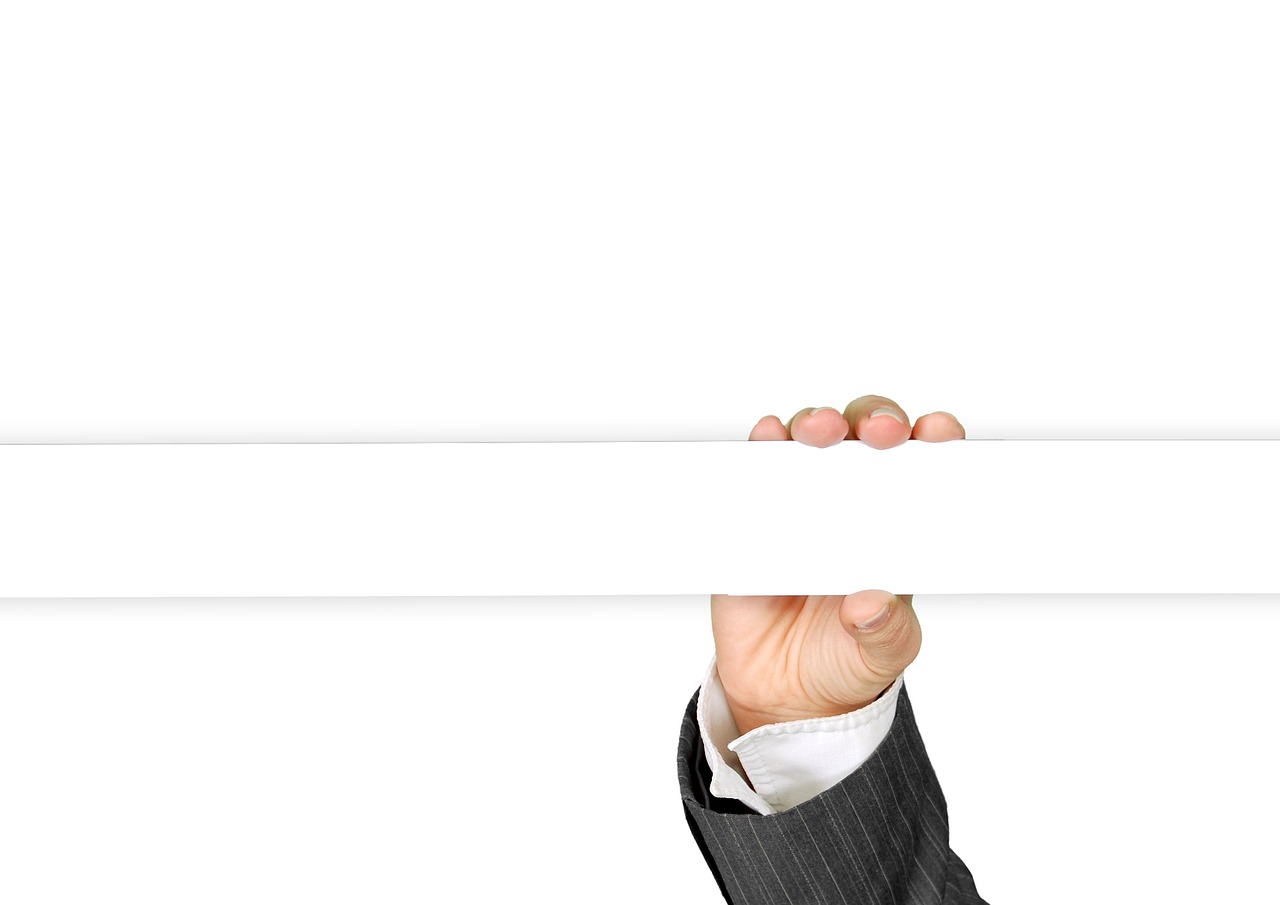
AI in Business
Artificial Intelligence (AI) is not just a buzzword; it’s a transformative force reshaping the way businesses operate. Imagine running a company where mundane tasks are automated, allowing employees to focus on creativity and innovation. That’s the reality AI is creating today. From small startups to large corporations, AI is being integrated into various business functions, enhancing efficiency and driving growth.
One of the most significant impacts of AI in business is in the realm of data-driven decision-making. Businesses generate enormous amounts of data daily, and AI technologies, particularly machine learning algorithms, analyze this data to uncover trends and insights that human analysts might miss. This capability enables companies to make informed decisions quickly, adapting to market changes and customer preferences with unprecedented agility.
Additionally, AI enhances customer experiences through personalized interactions. For instance, AI-powered chatbots are now commonplace on websites, providing instant support to customers 24/7. These chatbots can handle inquiries, resolve issues, and even recommend products based on individual customer behavior. Not only does this improve customer satisfaction, but it also reduces operational costs by minimizing the need for extensive customer service teams.
Moreover, AI plays a pivotal role in automation. Tasks that once required hours of manual labor can now be completed in minutes with the help of AI. For example, AI can automate inventory management, streamline supply chain processes, and enhance marketing strategies through predictive analytics. This level of automation not only boosts productivity but also allows businesses to allocate resources more efficiently, ultimately leading to increased profitability.
However, the integration of AI in business is not without its challenges. Companies must navigate the complexities of implementing AI solutions, which often require significant investment in technology and training. Furthermore, there are ethical considerations to keep in mind, such as ensuring that AI systems are transparent and do not perpetuate bias. Businesses must commit to developing fair and responsible AI practices to maintain trust with their customers and stakeholders.
As we look to the future, the potential for AI in business seems limitless. Industries such as finance, retail, and healthcare are already witnessing significant transformations due to AI technologies. For instance, in finance, AI algorithms are being used for fraud detection, while in retail, personalized shopping experiences are becoming the norm. The question is not whether AI will change business, but rather how quickly and effectively companies can adapt to these changes.
In conclusion, AI is revolutionizing the business landscape by enhancing efficiency, improving customer experiences, and enabling data-driven decision-making. As businesses continue to embrace these technologies, the potential for innovation and growth is boundless. The key lies in leveraging AI responsibly and strategically to unlock its full potential.
- What are the main benefits of using AI in business? AI enhances efficiency, improves customer experiences, and enables data-driven decision-making.
- How can AI improve customer service? AI-powered chatbots provide instant support and can handle inquiries, improving customer satisfaction.
- What challenges do businesses face when implementing AI? Challenges include investment costs, the need for training, and ethical considerations regarding bias and transparency.
- Which industries are most impacted by AI? Industries such as finance, retail, and healthcare are experiencing significant transformations due to AI technologies.
Frequently Asked Questions
- What is artificial intelligence (AI)?
Artificial intelligence (AI) refers to the simulation of human intelligence in machines that are programmed to think and learn like humans. It encompasses a variety of technologies, including machine learning, natural language processing, and neural networks, which allow computers to perform tasks that typically require human intelligence.
- How does cognitive computing differ from traditional computing?
Cognitive computing goes beyond traditional computing by mimicking human thought processes in complex situations. While traditional computing relies on pre-defined algorithms and rules, cognitive computing uses AI to analyze data, learn from it, and make decisions based on that learning, enabling it to handle ambiguity and adapt to new information.
- What are the main applications of natural language processing (NLP)?
NLP has numerous applications, including chatbots for customer service, sentiment analysis for understanding public opinion, and language translation services. These applications enhance user interaction and improve operational efficiency by enabling machines to understand and respond to human language effectively.
- What challenges does natural language processing face?
Some of the major challenges in NLP include understanding context, handling ambiguity in language, and recognizing cultural nuances. These issues can lead to misinterpretations and affect the performance of NLP systems, making ongoing research and development crucial for improvement.
- What are the ethical considerations surrounding AI?
Ethical considerations in AI include concerns about bias in algorithms, privacy issues, and accountability for decisions made by AI systems. It's essential to address these concerns to create fair, transparent, and trustworthy AI applications that respect user rights and promote equity.
- How can AI impact the healthcare industry?
AI has the potential to revolutionize healthcare through predictive analytics, personalized medicine, and enhanced diagnostics. By analyzing vast amounts of data, AI can help healthcare professionals make informed decisions, leading to better patient outcomes and more efficient healthcare delivery.
- What role does AI play in business operations?
AI plays a significant role in business by automating processes, enabling data-driven decision-making, and enhancing customer experiences. This leads to increased efficiency, reduced operational costs, and improved service delivery, allowing businesses to stay competitive in a rapidly evolving market.