The Future of AI and Machine Learning in Healthcare
The healthcare industry is on the brink of a revolutionary transformation, driven by the incredible advancements in Artificial Intelligence (AI) and Machine Learning (ML)
As we delve into the transformative potential of AI and ML, it's essential to recognize the significant advancements that have already taken place. From enhancing diagnostics to improving treatment planning, these technologies are paving the way for more personalized and efficient care solutions. The integration of AI into healthcare systems is akin to adding a turbocharger to an already powerful engine—it enhances performance and capabilities exponentially.
However, it's not all smooth sailing. The journey towards full integration of AI and ML in healthcare is fraught with challenges that must be addressed. Issues such as data privacy, algorithmic bias, and the need for informed consent are critical to ensuring that these technologies serve all patients equitably. As we explore the future of AI in healthcare, we must also consider the ethical implications and strive for solutions that benefit everyone.
In the coming sections, we will examine how advancements in AI technology are revolutionizing diagnostics, treatment protocols, and patient care. We will also highlight the ethical considerations that come with these innovations and take a glimpse into the future trends that are set to reshape the healthcare landscape. Buckle up, because the future of healthcare is not just bright; it's glowing with possibilities!
Recent breakthroughs in AI technology are reshaping healthcare, enhancing diagnostics, treatment planning, and patient monitoring, leading to more personalized and efficient care solutions. For instance, AI algorithms can analyze vast datasets to identify patterns that human practitioners might miss, enabling earlier detection of conditions like cancer or heart disease.
Machine learning algorithms are being increasingly utilized for diagnostics, improving accuracy and speed in identifying diseases. This not only enhances patient outcomes but also significantly reduces healthcare costs. Imagine a scenario where a patient's symptoms are analyzed in real-time, leading to an immediate diagnosis and treatment plan. This is the power of machine learning!
Predictive analytics powered by machine learning can forecast patient health trends, allowing for proactive interventions and tailored treatment plans, significantly improving the quality of care. For example, healthcare providers can use historical data to predict which patients are at risk for certain conditions and take preventative measures.
Developing risk stratification models helps healthcare providers identify high-risk patients and allocate resources effectively. By ensuring timely interventions, these models can reduce the likelihood of adverse health events, ultimately saving lives.
Real-time monitoring systems leverage AI to track patient vitals and health metrics. This capability enables immediate responses to critical changes, enhancing overall patient safety and outcomes. Imagine having a system that alerts your healthcare team the moment your vitals go outside the normal range!
AI-driven insights can optimize treatment protocols by analyzing vast amounts of medical data. This leads to more effective therapies and improved patient adherence to treatment plans. With AI, the days of one-size-fits-all treatment are fading, giving way to highly personalized care.
As AI becomes more integrated into healthcare, ethical considerations surrounding data privacy, algorithmic bias, and informed consent must be addressed. It is crucial to ensure equitable access to advanced medical technologies, so no patient is left behind.
Ensuring data privacy and security is paramount as healthcare organizations adopt AI solutions. Robust measures must be implemented to protect sensitive patient information from breaches and misuse. After all, trust is the foundation of the patient-provider relationship.
Addressing algorithmic bias is crucial for equitable healthcare delivery. This requires diverse datasets and ongoing evaluation to ensure AI systems serve all patient populations fairly. If we want AI to be a tool for good, we must ensure it does not perpetuate existing inequalities.
The future of AI in healthcare is poised for rapid growth. Emerging trends such as telemedicine integration, virtual health assistants, and enhanced patient engagement are shaping the landscape of medical care. These innovations promise to make healthcare more accessible and efficient.
Integrating AI with telemedicine platforms enhances remote patient monitoring and consultation. This provides timely access to care and improves health outcomes, especially in underserved areas. Imagine being able to consult your doctor from the comfort of your home, with AI assisting in real-time diagnosis!
AI-driven patient engagement tools are transforming how patients interact with healthcare providers. These tools foster better communication, education, and adherence to treatment plans for improved health management. With AI, patients can take a more active role in their healthcare journey.
- What is AI in healthcare? AI in healthcare refers to the use of artificial intelligence technologies to improve patient care, streamline processes, and enhance diagnostics.
- How does machine learning improve diagnostics? Machine learning algorithms analyze vast amounts of data to identify patterns and make predictions, leading to faster and more accurate diagnoses.
- What are the ethical concerns of AI in healthcare? Ethical concerns include data privacy, algorithmic bias, and the need for informed consent to ensure fair access to AI technologies.
- What is the future of AI in healthcare? The future includes telemedicine integration, enhanced patient engagement tools, and more personalized treatment plans driven by AI insights.
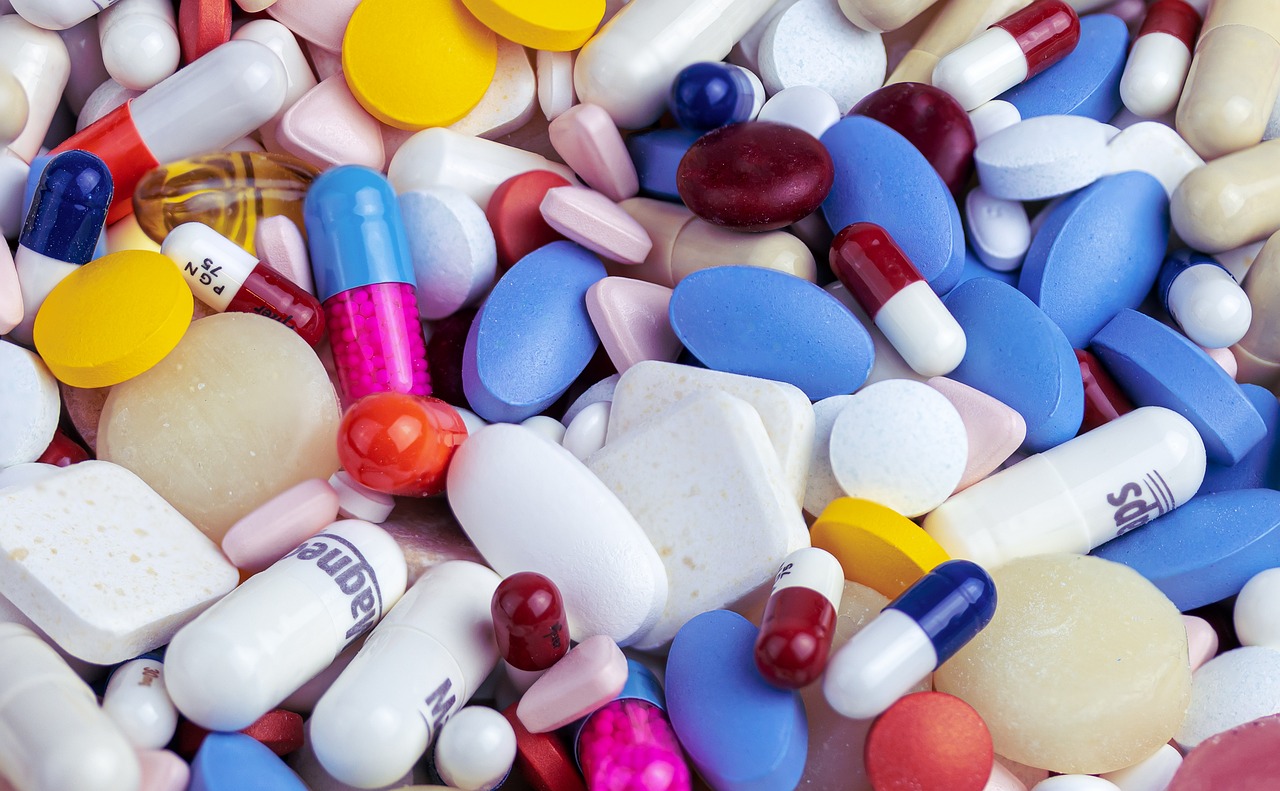
Advancements in AI Technology
The landscape of healthcare is undergoing a remarkable transformation, primarily driven by groundbreaking advancements in AI technology. These innovations are not just enhancing existing practices; they are revolutionizing the way healthcare is delivered and experienced. Imagine a world where doctors can diagnose diseases with pinpoint accuracy, tailor treatment plans to individual patients, and monitor health metrics in real-time—all thanks to the power of artificial intelligence. This isn't science fiction; it's happening right now!
One of the most significant advancements is the development of AI algorithms that can analyze vast amounts of medical data quickly and accurately. These algorithms can sift through millions of patient records, identify patterns, and generate insights that would take human analysts weeks or even months to uncover. For instance, AI can help in detecting early signs of diseases like cancer, diabetes, and heart conditions, allowing for timely interventions that can save lives. The speed and accuracy of these systems are far superior to traditional diagnostic methods, making them invaluable in today's fast-paced healthcare environment.
Moreover, AI is enhancing treatment planning through its ability to analyze patient-specific data. By considering a patient's medical history, genetic information, and even lifestyle choices, AI can recommend personalized treatment protocols that are more likely to succeed. This shift towards personalized medicine means that patients are receiving care tailored specifically to their needs, which has shown to improve outcomes significantly.
Another exciting development is the integration of AI with wearable technology. Devices like smartwatches and fitness trackers are now equipped with AI capabilities that allow them to monitor vital signs, track physical activity, and even predict health issues before they become critical. This continuous monitoring empowers patients to take charge of their health and provides healthcare providers with real-time data to make informed decisions.
In addition to diagnostics and treatment, AI is also streamlining administrative processes within healthcare systems. By automating routine tasks such as scheduling appointments, managing patient records, and billing, AI reduces the workload on healthcare professionals, allowing them to focus more on patient care. This efficiency not only enhances the patient experience but also reduces operational costs for healthcare providers.
As we look to the future, the potential of AI in healthcare seems limitless. With ongoing research and development, we can expect to see even more innovative applications that will further enhance patient care. From predictive analytics that forecast health trends to AI-driven decision support systems that assist clinicians in making better choices, the possibilities are endless. As we embrace these advancements, we must also remain vigilant about the ethical implications and ensure that these technologies are used responsibly and equitably.
In summary, the advancements in AI technology are not just reshaping the healthcare landscape; they are paving the way for a future where patient care is more effective, efficient, and personalized. As we continue to explore these innovations, the ultimate goal remains clear: to improve health outcomes and enhance the quality of life for all individuals.
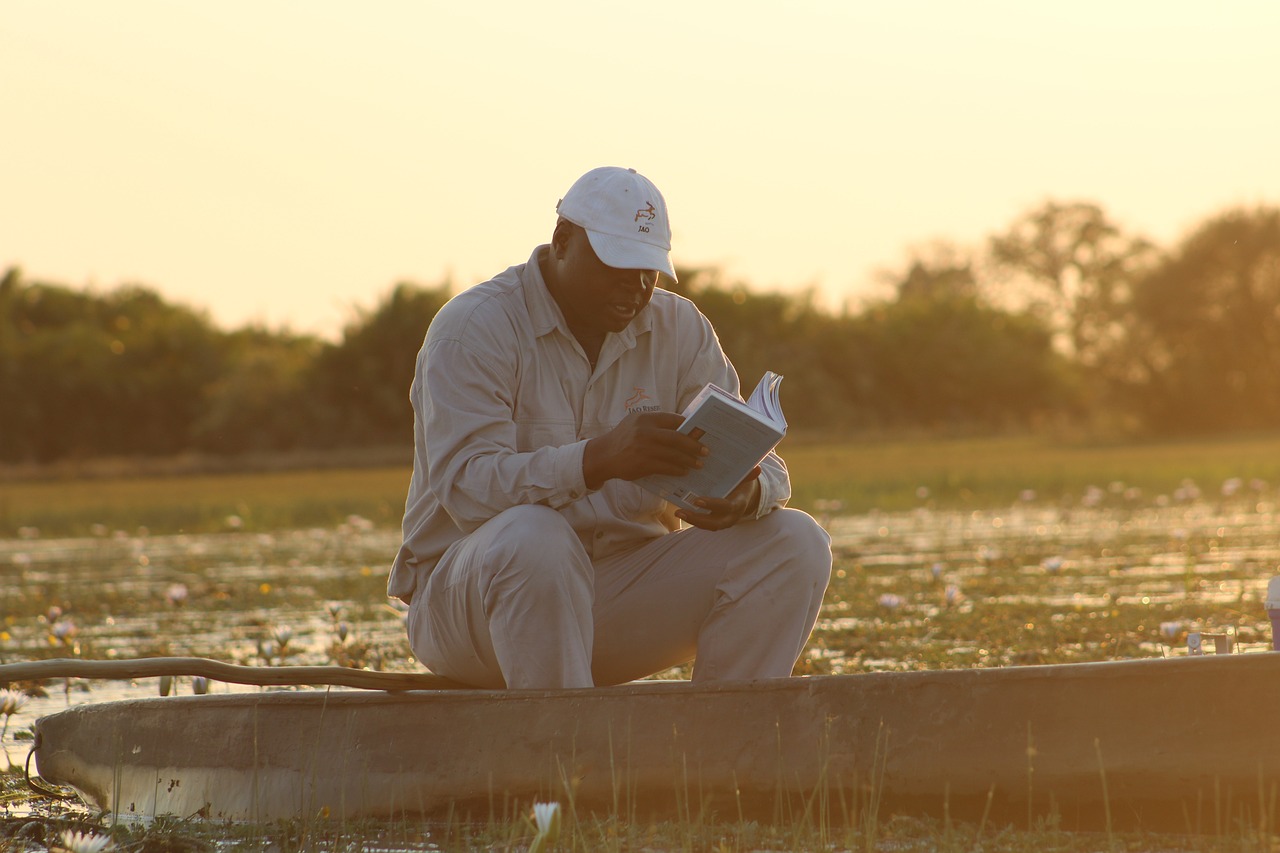
Machine Learning in Diagnostics
Machine learning is revolutionizing the field of diagnostics, and its impact cannot be overstated. Imagine having a tool that can analyze thousands of medical images or patient data points in the blink of an eye, identifying patterns that even the most trained professionals might miss. This technology is not just about speed; it's about accuracy and efficiency. By harnessing the power of algorithms, healthcare providers can now make quicker, more informed decisions, which ultimately leads to better patient outcomes.
One of the most significant advantages of machine learning in diagnostics is its ability to improve the accuracy and speed of disease identification. For instance, algorithms can be trained on vast datasets of medical images, such as X-rays, MRIs, and CT scans, allowing them to detect anomalies like tumors or fractures with remarkable precision. This can drastically reduce the time it takes for a patient to receive a diagnosis, which is crucial in situations where every second counts.
Moreover, machine learning systems can continuously learn and adapt. As they process more data, they become better at recognizing subtle signs of diseases that might have been overlooked previously. This adaptive learning process ensures that diagnostic tools remain at the cutting edge, evolving alongside advancements in medical knowledge and technology.
Let's consider a few examples of how machine learning is currently being utilized in diagnostics:
- Radiology: AI algorithms can analyze imaging data to detect conditions like pneumonia or tumors, often outperforming human radiologists in accuracy.
- Pathology: Machine learning can assist in analyzing biopsy samples, helping pathologists identify cancerous cells more reliably.
- Genomics: Algorithms can sift through genetic data to identify mutations linked to specific diseases, paving the way for personalized medicine.
However, while the benefits are clear, there are also challenges that come with implementing machine learning in diagnostics. For instance, the quality of the data used to train these algorithms is paramount. If the data is biased or incomplete, the results can be misleading, which could have serious implications for patient care. Therefore, it is essential for healthcare organizations to ensure that they are using diverse and comprehensive datasets to train their machine learning models.
In addition to improving diagnostic accuracy, machine learning can also enhance operational efficiency within healthcare settings. By automating routine tasks, such as data entry or preliminary analysis of test results, healthcare professionals can focus their time and energy on what truly matters: patient care. This shift not only improves job satisfaction for healthcare workers but also leads to a more streamlined workflow, ultimately benefiting patients.
As we look to the future, the integration of machine learning into diagnostics promises to be a game-changer. With ongoing advancements in technology, we can expect to see even more sophisticated algorithms that will further enhance the diagnostic process. The potential for early detection of diseases, reduced healthcare costs, and improved patient outcomes is enormous.
- How does machine learning improve diagnostic accuracy?
Machine learning algorithms analyze vast amounts of data to identify patterns and anomalies that may be missed by human eyes, leading to more accurate diagnoses. - What are the challenges of implementing machine learning in diagnostics?
Challenges include ensuring high-quality, unbiased data for training algorithms and addressing concerns about data privacy and security. - Can machine learning replace healthcare professionals in diagnostics?
While machine learning can significantly enhance diagnostic processes, it is intended to assist healthcare professionals rather than replace them, allowing for more informed decision-making.
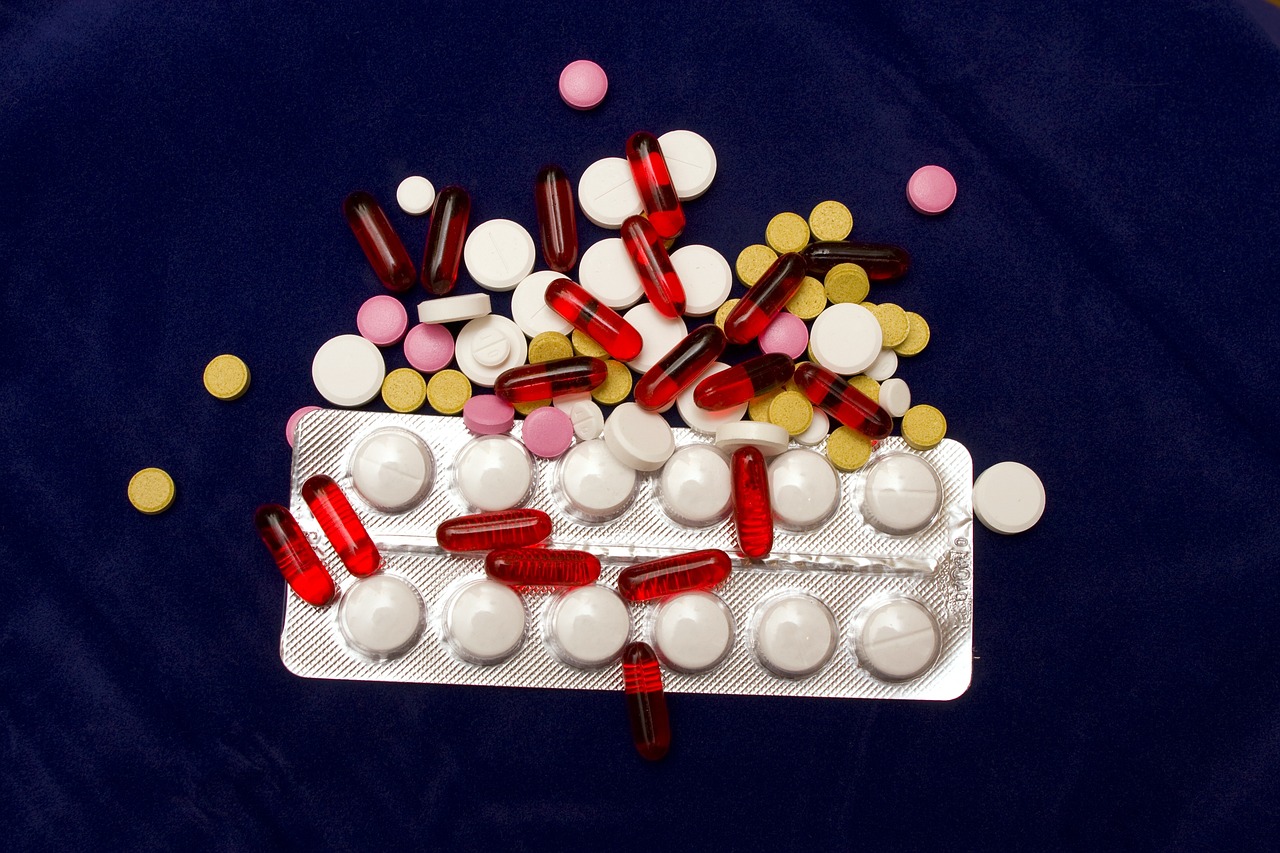
Predictive Analytics for Patient Care
Predictive analytics is revolutionizing the way healthcare providers approach patient care, acting like a crystal ball that reveals potential health trends before they become critical issues. Imagine being able to foresee a patient's health decline and intervening before it spirals out of control. This is not just a dream; it's a reality made possible by the power of machine learning algorithms that analyze vast amounts of data to identify patterns and predict outcomes. By leveraging predictive analytics, healthcare professionals can tailor treatment plans that are not only proactive but also personalized to each patient's unique health profile.
One of the most significant benefits of predictive analytics is its ability to forecast potential health risks. For instance, healthcare providers can utilize historical data to assess which patients are at a higher risk for conditions like diabetes or heart disease. This allows for early interventions, such as lifestyle modifications or preventive treatments, which can significantly improve patient outcomes. The data-driven insights derived from predictive analytics can lead to better resource allocation, ensuring that high-risk patients receive the attention they need before complications arise.
Furthermore, predictive analytics can enhance patient engagement by providing individuals with tailored health insights. When patients understand their health risks and the steps they can take to mitigate them, they become more active participants in their care. This engagement can lead to improved adherence to prescribed treatment plans, ultimately resulting in better health outcomes. For example, a patient at risk for hypertension might receive personalized alerts about dietary changes or exercise routines that can help manage their condition effectively.
To illustrate the impact of predictive analytics, consider the following table that outlines the potential benefits of implementing predictive models in patient care:
Benefit | Description |
---|---|
Improved Early Detection | Identifies health issues before they escalate, allowing for timely interventions. |
Personalized Treatment Plans | Creates customized care strategies based on individual patient data and risk factors. |
Enhanced Resource Allocation | Ensures that healthcare resources are directed towards patients who need them most. |
Increased Patient Engagement | Empowers patients with knowledge about their health, leading to better adherence to treatments. |
In conclusion, predictive analytics is not just a technological advancement; it's a fundamental shift in how we approach patient care. By harnessing the power of data, healthcare providers can not only anticipate health issues but also create a more personalized and effective healthcare experience for their patients. As we continue to explore the capabilities of predictive analytics, the potential for improved health outcomes and enhanced patient satisfaction becomes increasingly evident.
- What is predictive analytics in healthcare?
Predictive analytics in healthcare refers to the use of data analysis and machine learning techniques to predict future health outcomes based on historical data. It helps in identifying potential health risks and tailoring treatment plans accordingly. - How does predictive analytics improve patient care?
By forecasting health trends and risks, predictive analytics allows for proactive interventions and personalized treatment plans, improving the overall quality of care and patient outcomes. - What types of data are used in predictive analytics?
Predictive analytics utilizes a variety of data, including patient medical history, demographics, lifestyle factors, and clinical data to generate insights and predictions. - Are there any risks associated with predictive analytics?
While predictive analytics has numerous benefits, it also raises concerns regarding data privacy and the potential for algorithmic bias, which must be addressed to ensure equitable healthcare delivery.
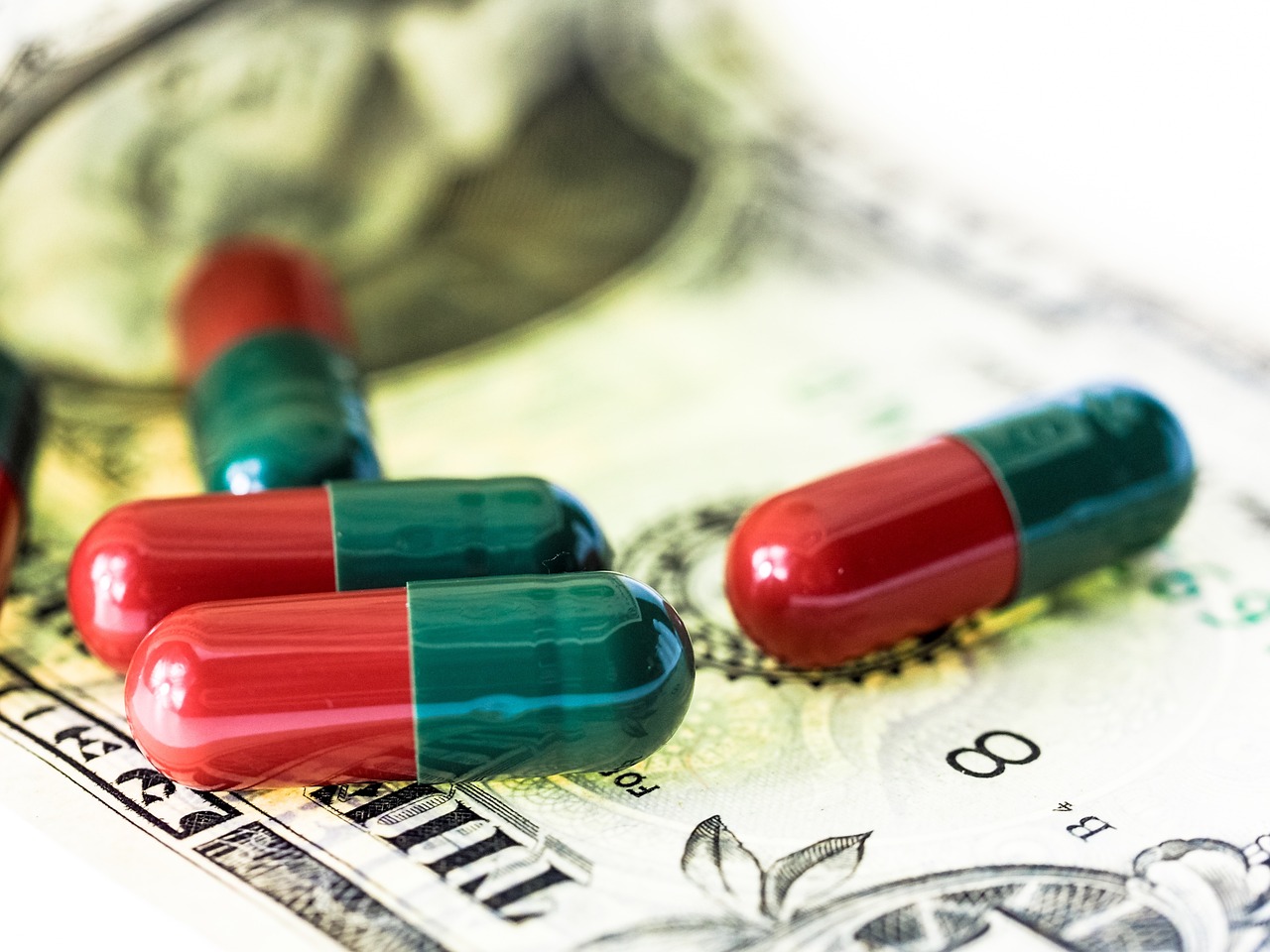
Risk Stratification Models
In the ever-evolving landscape of healthcare, stand out as a beacon of hope for improving patient outcomes. These models are designed to identify patients who are at a higher risk of developing complications or experiencing adverse health events. By leveraging data analytics and machine learning, healthcare providers can pinpoint these individuals early on, enabling them to allocate resources more effectively and tailor interventions that are both timely and appropriate.
Imagine a scenario where a hospital can predict which patients are likely to be readmitted within 30 days of discharge. With risk stratification models, healthcare professionals can intervene proactively, perhaps by arranging follow-up appointments or providing additional support services. This not only enhances patient care but also significantly reduces healthcare costs associated with avoidable readmissions.
Risk stratification models utilize a variety of data sources, including electronic health records (EHR), demographic information, and even social determinants of health. By analyzing these factors, the models can generate a comprehensive risk profile for each patient. For instance, patients with chronic conditions, such as diabetes or heart disease, may be flagged as high-risk due to their medical history, lifestyle choices, and other relevant factors.
To illustrate how risk stratification works, consider the following table that outlines common factors used in these models:
Risk Factor | Description |
---|---|
Age | Older patients generally have a higher risk of complications. |
Chronic Conditions | Conditions such as diabetes or hypertension increase risk levels. |
Previous Hospitalizations | History of hospital stays can indicate higher future risks. |
Social Determinants | Factors like socioeconomic status and access to care can influence health outcomes. |
Moreover, implementing these models requires careful consideration of the algorithms used. It's crucial to ensure that they are both accurate and fair, taking into account the diverse populations they serve. This means continuously refining the models based on new data and feedback from healthcare providers.
In conclusion, risk stratification models offer a powerful tool for healthcare systems aiming to enhance patient care and resource allocation. By identifying high-risk patients and intervening early, healthcare providers can not only improve individual health outcomes but also contribute to the overall efficiency of the healthcare system.
- What are risk stratification models? Risk stratification models are analytical tools used to identify patients at high risk for adverse health outcomes, enabling proactive care.
- How do these models work? They analyze various data points, including medical history and social determinants of health, to create risk profiles for patients.
- Why are they important in healthcare? They help healthcare providers allocate resources effectively and improve patient outcomes by enabling timely interventions.
- Are these models always accurate? While they are powerful tools, continuous evaluation and refinement are necessary to ensure their accuracy and fairness.
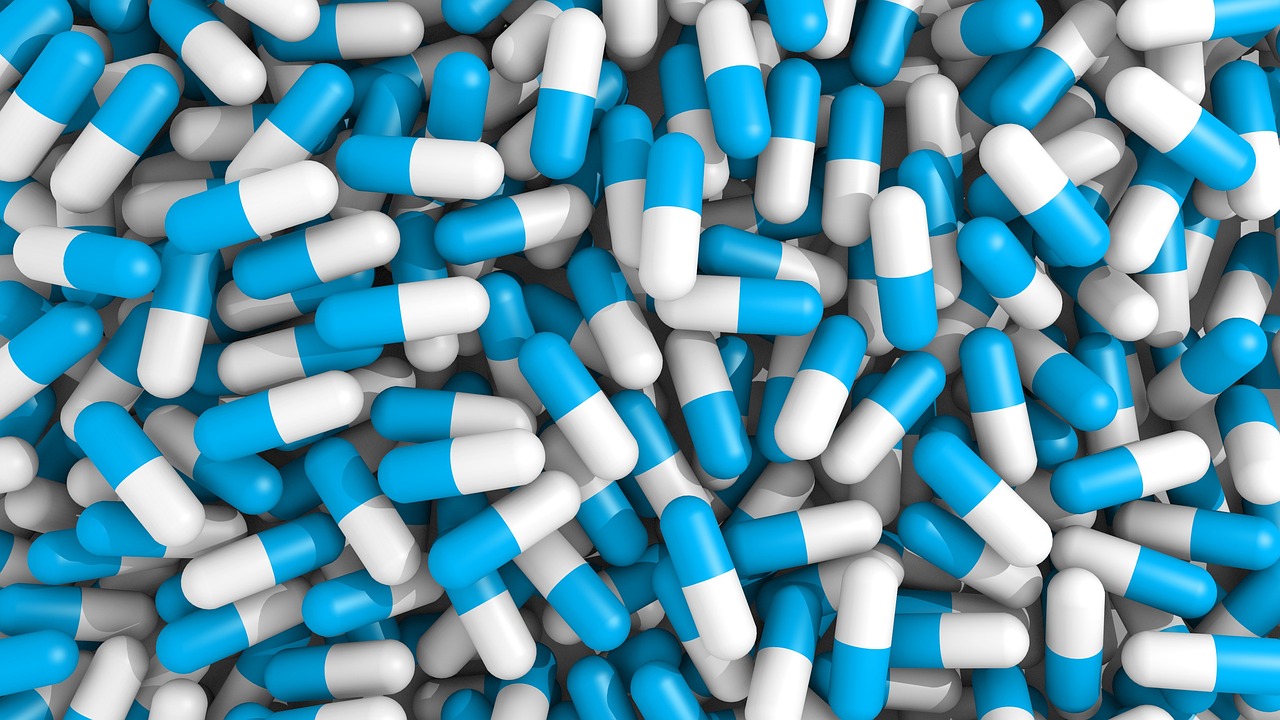
Real-time Monitoring Systems
In the fast-paced world of healthcare, are becoming a game changer. Imagine a scenario where doctors can track a patient's vital signs continuously, receiving instant alerts if anything goes awry. This isn't just a futuristic dream; it's the reality that AI and machine learning technologies are bringing to the table. These systems utilize advanced algorithms to analyze data from various sources, such as wearable devices, mobile apps, and in-hospital monitoring equipment. By doing so, they provide a comprehensive view of a patient's health status at any given moment.
One of the most significant benefits of real-time monitoring is the ability to respond promptly to critical changes. For instance, if a patient's heart rate spikes or drops unexpectedly, the system can immediately notify healthcare professionals, allowing them to intervene before a potentially life-threatening situation escalates. This proactive approach not only enhances patient safety but also significantly reduces hospital readmission rates, which is a major concern in today's healthcare landscape.
Moreover, these systems are designed to be user-friendly, enabling patients to engage actively in their health management. Many real-time monitoring solutions come equipped with mobile applications that allow patients to view their health metrics, receive reminders for medication, and even communicate directly with their healthcare providers. This level of engagement fosters a sense of empowerment among patients, making them more likely to adhere to treatment plans and lifestyle changes.
To illustrate the impact of real-time monitoring systems, consider the following table that outlines some key advantages:
Advantage | Description |
---|---|
Improved Patient Safety | Immediate alerts for critical health changes allow for prompt medical intervention. |
Enhanced Data Collection | Continuous monitoring provides a wealth of data for better diagnosis and treatment. |
Increased Patient Engagement | Patients can monitor their health metrics and communicate with providers easily. |
Cost Efficiency | Reduces hospital readmissions and emergency visits, lowering overall healthcare costs. |
However, while the benefits are evident, it's essential to consider the challenges that come with implementing real-time monitoring systems. Issues such as data privacy, the need for reliable internet connectivity, and the potential for technology overload can pose significant hurdles. As healthcare providers continue to integrate these systems into their practices, addressing these challenges will be crucial for maximizing their effectiveness.
- What is a real-time monitoring system?
A real-time monitoring system is a technology that continuously tracks patient vital signs and health metrics, providing immediate alerts to healthcare providers if any critical changes occur. - How does AI improve real-time monitoring?
AI enhances real-time monitoring by analyzing vast amounts of health data quickly and accurately, allowing for faster detection of potential health issues. - What are the benefits of real-time monitoring for patients?
Patients benefit from increased safety, better engagement in their health management, and potentially lower healthcare costs due to reduced hospital visits. - Are there any risks associated with real-time monitoring systems?
Yes, there are risks such as data privacy concerns, reliance on technology, and the potential for information overload for both patients and providers.
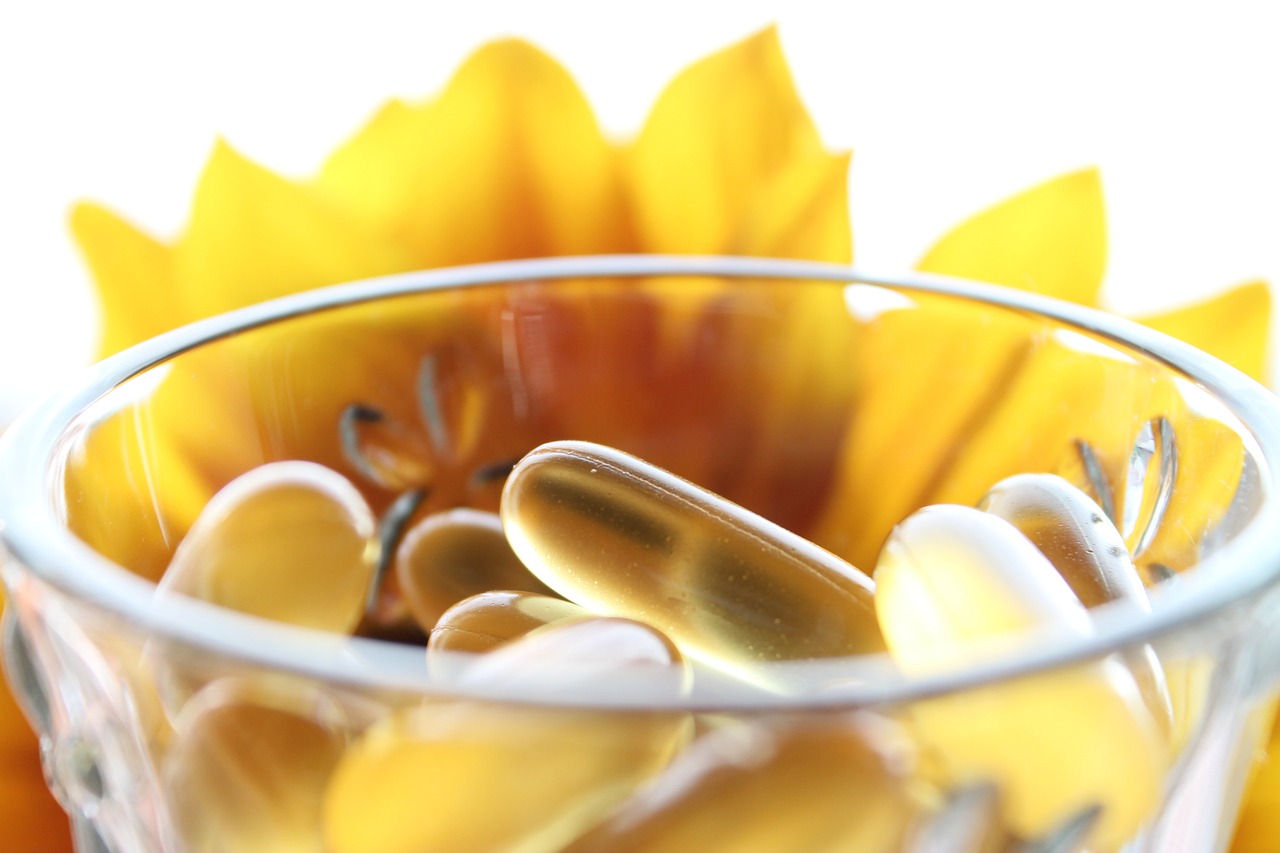
Enhancing Treatment Protocols
This article explores the transformative potential of AI and machine learning in healthcare, highlighting advancements, challenges, and future implications for patient care and medical practices.
Recent breakthroughs in AI technology are reshaping healthcare, enhancing diagnostics, treatment planning, and patient monitoring, leading to more personalized and efficient care solutions.
Machine learning algorithms are being increasingly utilized for diagnostics, improving accuracy and speed in identifying diseases, which ultimately enhances patient outcomes and reduces healthcare costs.
Predictive analytics powered by machine learning can forecast patient health trends, allowing for proactive interventions and tailored treatment plans, significantly improving the quality of care.
Developing risk stratification models helps healthcare providers identify high-risk patients and allocate resources effectively, ensuring timely interventions and reducing the likelihood of adverse health events.
Real-time monitoring systems leverage AI to track patient vitals and health metrics, enabling immediate responses to critical changes and enhancing overall patient safety.
In today's rapidly evolving healthcare environment, AI-driven insights are becoming essential for optimizing treatment protocols. By analyzing vast amounts of medical data, AI can identify patterns and correlations that may not be immediately evident to healthcare professionals. This capability allows for the development of more effective therapies tailored to individual patient needs.
For instance, imagine a scenario where a patient with diabetes is receiving treatment. Traditional methods might rely on a one-size-fits-all approach, but with AI, healthcare providers can analyze the patient's unique health data, including genetic information, lifestyle choices, and previous treatment responses. This leads to the creation of personalized treatment plans that not only enhance the efficacy of the therapy but also improve patient adherence.
Moreover, AI can facilitate the ongoing adjustment of treatment protocols based on real-time data. For example, if a patient's condition changes or they report side effects, AI systems can quickly analyze this new information and suggest modifications to the treatment plan. This dynamic approach ensures that patients receive the most appropriate care at all times, ultimately leading to better outcomes.
To illustrate the impact of AI on treatment protocols, consider the following table that summarizes key benefits:
Benefit | Description |
---|---|
Personalization | Tailored treatment plans based on individual patient data. |
Efficiency | Faster identification of effective therapies through data analysis. |
Adaptability | Real-time adjustments to treatment based on ongoing patient data. |
Improved Outcomes | Higher patient satisfaction and better health results. |
In conclusion, the integration of AI in enhancing treatment protocols is not just a trend; it’s a revolution in how we approach patient care. By leveraging the power of technology, healthcare providers can ensure that patients receive the most effective and personalized treatment possible, paving the way for a healthier future.
As AI becomes more integrated into healthcare, ethical considerations surrounding data privacy, algorithmic bias, and informed consent must be addressed to ensure equitable access to advanced medical technologies.
Ensuring data privacy and security is paramount as healthcare organizations adopt AI solutions, necessitating robust measures to protect sensitive patient information from breaches and misuse.
Addressing algorithmic bias is crucial for equitable healthcare delivery, requiring diverse datasets and ongoing evaluation to ensure AI systems serve all patient populations fairly.
The future of AI in healthcare is poised for rapid growth, with emerging trends such as telemedicine integration, virtual health assistants, and enhanced patient engagement shaping the landscape of medical care.
Integrating AI with telemedicine platforms enhances remote patient monitoring and consultation, providing timely access to care and improving health outcomes, especially in underserved areas.
AI-driven patient engagement tools are transforming how patients interact with healthcare providers, fostering better communication, education, and adherence to treatment plans for improved health management.
- What role does AI play in patient care? AI enhances patient care by providing personalized treatment plans, improving diagnostics, and enabling real-time monitoring.
- Are there risks associated with using AI in healthcare? Yes, risks include data privacy concerns and potential algorithmic bias, which need to be managed carefully.
- How can AI improve treatment adherence? By personalizing treatment plans and providing real-time feedback, AI can help patients stay engaged and adhere to their therapies.
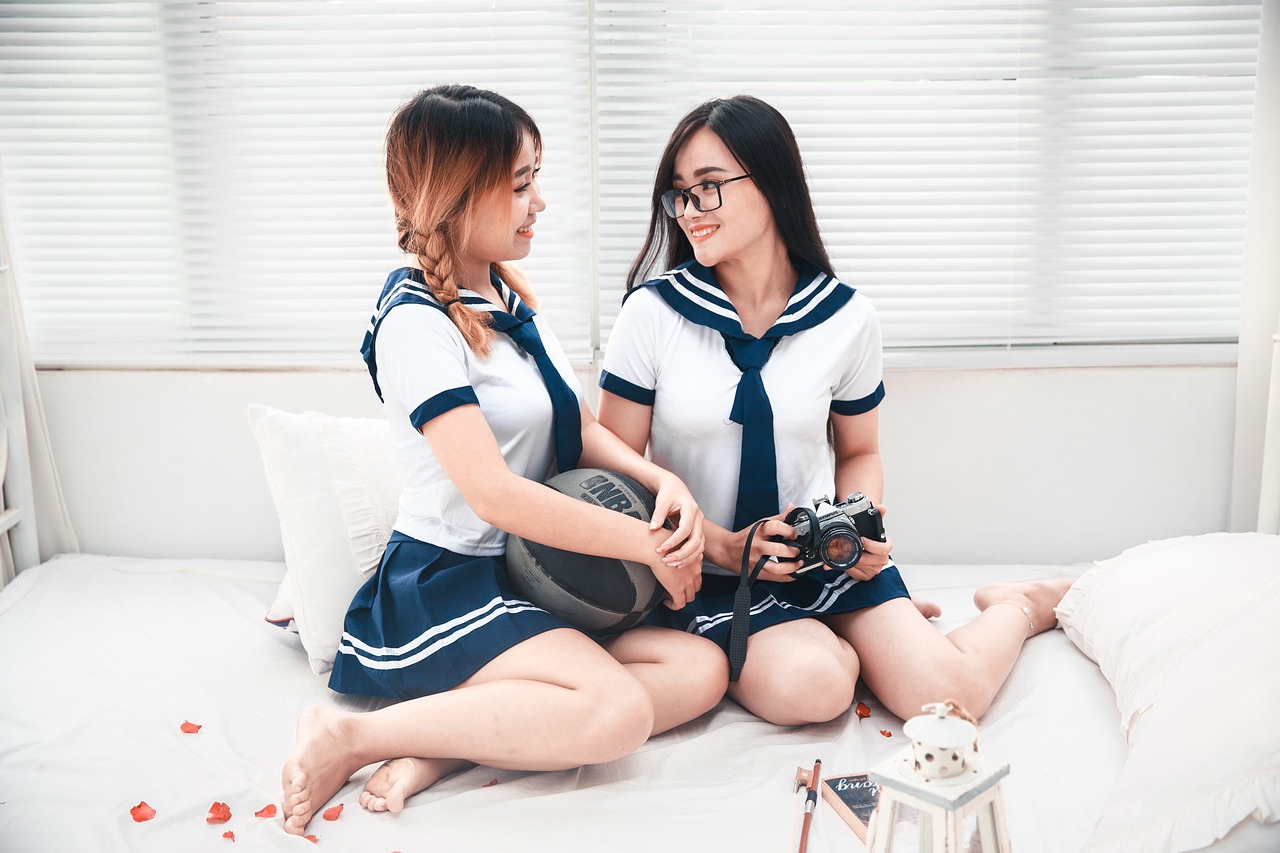
Ethical Considerations in AI
As artificial intelligence (AI) becomes more integrated into healthcare, it's essential to address the ethical considerations that arise. The rapid adoption of AI technologies brings forth complex challenges that can affect patient care and the overall healthcare landscape. One of the most pressing issues is data privacy. With the vast amounts of sensitive patient information being processed by AI systems, healthcare organizations must implement robust security measures to protect this data from breaches and misuse. Imagine your personal health data being exposed; it’s a frightening thought, isn’t it? Ensuring that patient information remains confidential is not just a regulatory requirement but a fundamental ethical obligation.
Another significant concern is algorithmic bias. AI systems learn from data, and if that data is skewed or unrepresentative, the outcomes can be detrimental. For instance, if an AI diagnostic tool is trained primarily on data from one demographic group, it may not perform accurately for patients from different backgrounds. This can lead to disparities in healthcare delivery, where certain populations do not receive the same quality of care as others. To combat this, healthcare providers must ensure that they use diverse datasets and continuously evaluate their AI systems to maintain fairness and equity in patient treatment.
Informed consent is also a vital ethical consideration. Patients should be fully aware of how AI technologies are being used in their care. This means clear communication about what data is collected, how it will be used, and the potential risks involved. Imagine going to a doctor and not knowing that an AI system is analyzing your health data; it could feel like an invasion of your privacy. Thus, healthcare organizations must prioritize transparency and patient education to foster trust and understanding.
To summarize, the ethical landscape of AI in healthcare is multifaceted, encompassing issues of data privacy, algorithmic bias, and informed consent. Addressing these challenges is not just about adhering to regulations; it’s about ensuring that all patients receive fair, safe, and effective care. As we move forward, ongoing dialogue among stakeholders—patients, providers, and policymakers—will be crucial in shaping a future where AI enhances healthcare without compromising ethical standards.
- What are the main ethical concerns regarding AI in healthcare? The main concerns include data privacy, algorithmic bias, and informed consent.
- How can healthcare organizations protect patient data? By implementing robust security measures and adhering to data protection regulations.
- Why is addressing algorithmic bias important? To ensure equitable healthcare delivery and avoid disparities in treatment outcomes.
- How can patients be informed about AI use in their healthcare? Through transparent communication and education from healthcare providers.
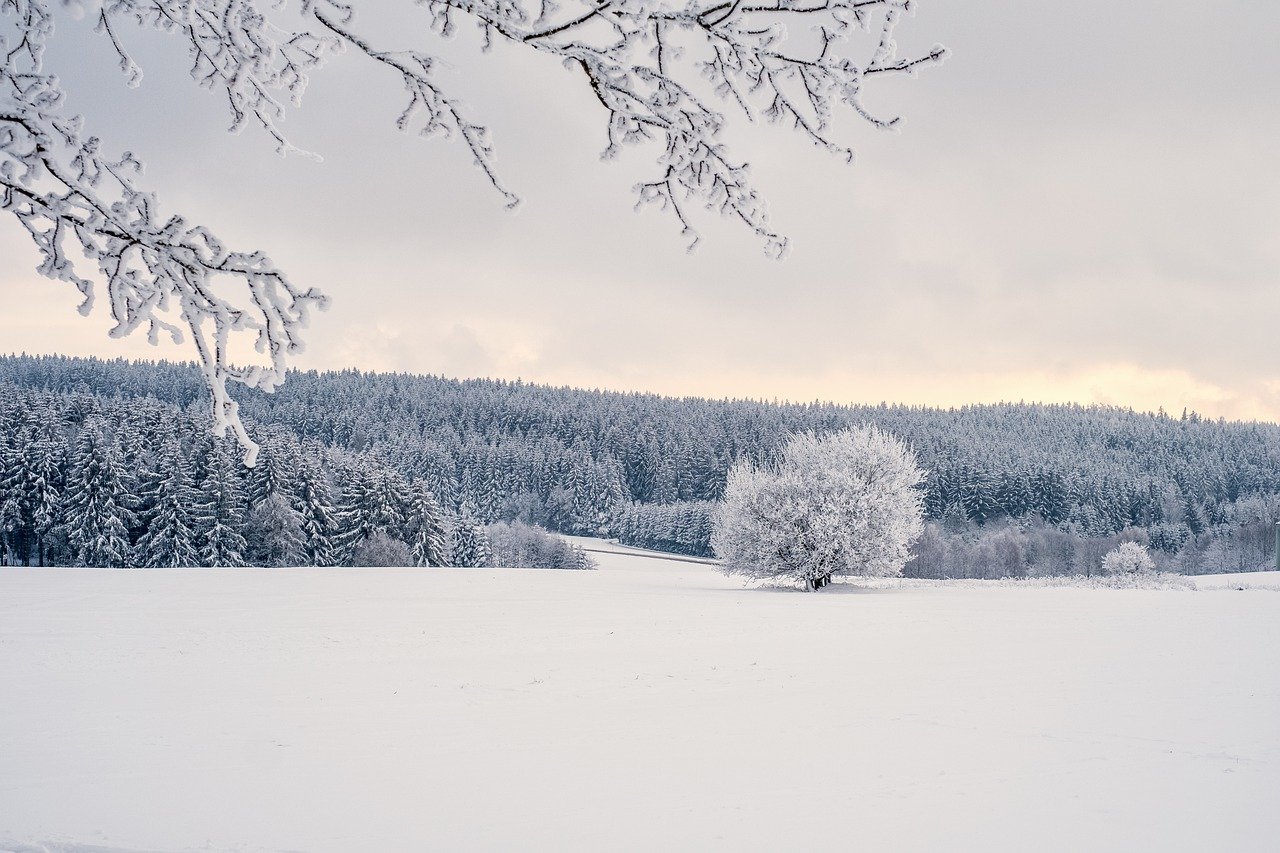
Data Privacy and Security
In the rapidly evolving landscape of healthcare, where artificial intelligence (AI) and machine learning are becoming integral to patient care, have emerged as paramount concerns. As healthcare organizations increasingly adopt AI solutions, they must navigate a complex web of regulations and ethical considerations to safeguard sensitive patient information. Imagine a world where your personal health data is as secure as a vault, yet easily accessible to those who need it for your care. This balance is crucial, and achieving it requires robust measures and a proactive approach.
One of the primary challenges in ensuring data privacy is the sheer volume of information that AI systems process. With electronic health records (EHRs) and various health apps collecting vast amounts of data, the risk of breaches increases significantly. Healthcare providers must implement stringent security protocols to protect this data from unauthorized access. This includes using advanced encryption methods, secure access controls, and regular audits to ensure compliance with regulations like HIPAA (Health Insurance Portability and Accountability Act).
Moreover, the integration of AI in healthcare often involves sharing data across multiple platforms, which can create vulnerabilities. Providers need to establish clear guidelines about data sharing and ensure that all parties involved in patient care adhere to these standards. Additionally, patients should be informed about how their data is used and the measures in place to protect it. This transparency fosters trust and encourages patients to engage more actively in their healthcare.
To illustrate the importance of data privacy and security, consider the following table that outlines key security measures healthcare organizations should adopt:
Security Measure | Description |
---|---|
Data Encryption | Converts data into a secure format that can only be read with a decryption key. |
Access Control | Restricts access to sensitive information based on user roles and responsibilities. |
Regular Audits | Conducts periodic reviews of data access and usage to identify potential vulnerabilities. |
Employee Training | Educates staff on data security best practices and the importance of protecting patient information. |
Furthermore, as AI systems learn and evolve, they can inadvertently perpetuate biases present in their training data. This brings us to another critical aspect of data privacy: the ethical use of data. Healthcare providers must ensure that the datasets used to train AI algorithms are diverse and representative of the patient population. This not only enhances the effectiveness of AI tools but also helps mitigate the risk of algorithmic bias, ensuring fair treatment for all patients.
In conclusion, as we embrace the benefits of AI in healthcare, we must remain vigilant about data privacy and security. By implementing comprehensive security measures and fostering a culture of transparency, healthcare organizations can protect sensitive patient information while leveraging the transformative power of AI. After all, when patients trust that their data is secure, they are more likely to engage with healthcare providers, leading to better health outcomes for everyone.
- What are the main risks to data privacy in healthcare AI? The main risks include unauthorized access to sensitive patient information, data breaches, and the potential misuse of data.
- How can healthcare organizations protect patient data? Organizations can protect patient data by implementing encryption, access controls, regular audits, and employee training.
- What is algorithmic bias, and why is it a concern? Algorithmic bias occurs when AI systems produce unfair outcomes due to biased training data, which can lead to inequitable healthcare delivery.
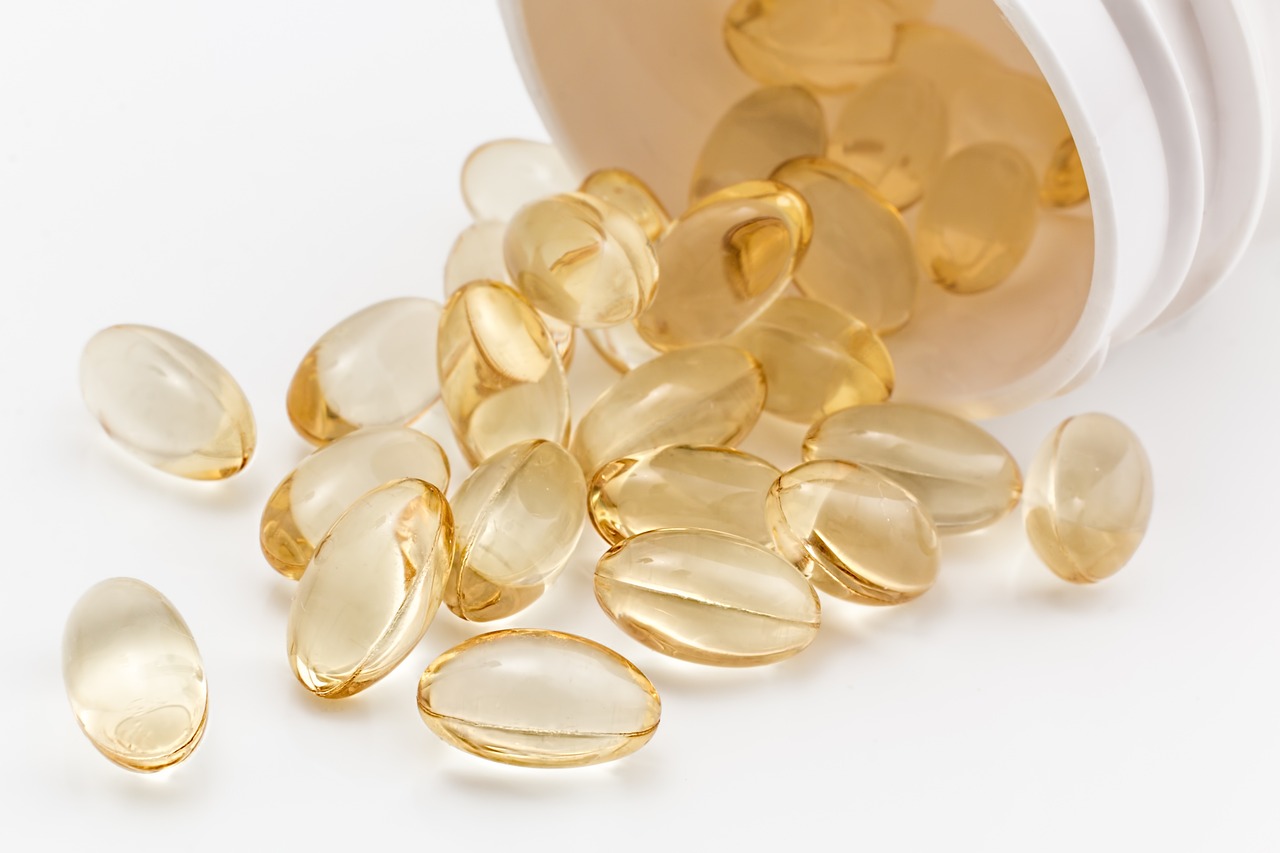
Addressing Algorithmic Bias
As we delve deeper into the world of AI in healthcare, one of the most pressing issues we face is algorithmic bias. This bias can arise from various sources, including the data sets used to train these algorithms. If the training data is not representative of the diverse population it aims to serve, the algorithms may inadvertently favor certain demographics over others. This can lead to disparities in healthcare delivery, where some groups receive subpar treatment or misdiagnosis simply because the AI was not trained on a sufficiently varied data set.
To combat this issue, healthcare organizations need to prioritize the use of diverse datasets. This means including data from a wide range of ethnicities, genders, ages, and socioeconomic backgrounds. By doing so, we can enhance the accuracy of AI predictions and ensure that all patient populations are treated equitably. Furthermore, ongoing evaluation of these AI systems is crucial. Regular audits can help identify any biases that may develop over time, allowing for timely adjustments to the algorithms.
Moreover, engaging with communities that are often underrepresented in healthcare data is essential. By incorporating feedback from these groups, AI developers can gain insights into the unique challenges they face, which can inform better data collection practices. This collaborative approach not only helps to refine the algorithms but also builds trust within the community, fostering a more inclusive healthcare environment.
Finally, it's important to implement robust regulatory frameworks that hold AI systems accountable. These frameworks should mandate transparency in how algorithms are developed and deployed, ensuring that healthcare providers can explain the decision-making processes behind AI recommendations. By addressing algorithmic bias head-on, we can pave the way for a more equitable future in healthcare.
- What is algorithmic bias? Algorithmic bias occurs when an AI system produces results that are systematically prejudiced due to erroneous assumptions in the machine learning process.
- How can we identify algorithmic bias in healthcare? Regular audits and evaluations of AI systems, along with diverse data sets, can help identify biases in healthcare algorithms.
- Why is diversity in data important for AI? Diversity in data ensures that AI systems are trained to understand and serve all demographic groups fairly, reducing the risk of biased outcomes.
- What role do regulatory frameworks play in addressing algorithmic bias? Regulatory frameworks provide guidelines and accountability for AI development, ensuring transparency and fairness in healthcare applications.
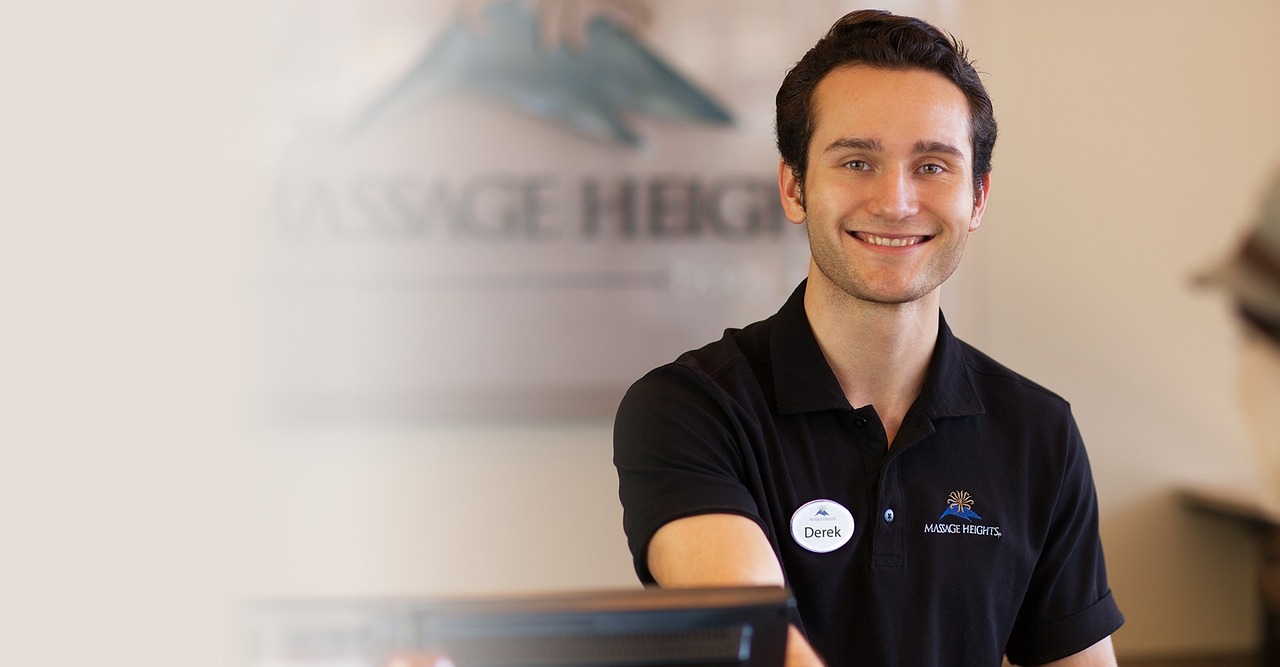
Future Trends in Healthcare AI
The future of AI in healthcare is not just a far-off dream; it's unfolding right before our eyes. With rapid advancements in technology, we are witnessing a transformation that promises to revolutionize patient care and medical practices. Imagine a world where your health data is analyzed in real-time, leading to personalized treatment plans that adapt to your unique needs. This is not science fiction; this is the future we are stepping into.
One of the most exciting trends is the integration of telemedicine with AI. This combination is a game-changer, especially for those living in remote areas where access to healthcare can be a challenge. Picture this: a patient in a rural community can consult with a specialist hundreds of miles away, with AI tools enhancing the consultation process. AI can analyze the patient’s data, suggest potential diagnoses, and even recommend treatment options, all in real-time. It's like having a personal health assistant right in your pocket!
Moreover, the emergence of AI-driven patient engagement tools is transforming the way patients interact with their healthcare providers. These tools are designed to foster better communication, making it easier for patients to understand their health conditions and treatment plans. For instance, AI chatbots can answer common health queries, schedule appointments, and send reminders for medications. This not only empowers patients but also improves adherence to treatment plans. After all, when patients feel informed and engaged, they are more likely to follow through with their healthcare recommendations.
Another trend to keep an eye on is the development of AI algorithms that focus on predictive analytics. These advanced models analyze vast amounts of health data to forecast potential health issues before they arise. Imagine your doctor being able to predict a potential health crisis based on your medical history and lifestyle choices. This proactive approach allows for timely interventions that can save lives and reduce healthcare costs. It’s like having a crystal ball that provides insights into your health, enabling you to take action before problems escalate.
However, with these advancements come challenges, particularly in the realm of data privacy and security. As healthcare organizations increasingly adopt AI solutions, the protection of sensitive patient information becomes paramount. It's essential for these organizations to implement robust security measures to safeguard data from breaches and misuse. After all, trust is the foundation of the patient-provider relationship, and any compromise can have serious ramifications.
In conclusion, the future of AI in healthcare is bright and brimming with potential. With innovations like telemedicine integration, AI-driven patient engagement tools, and predictive analytics, we are on the brink of a healthcare revolution that promises to enhance patient care and improve health outcomes. As we embrace these changes, it’s crucial to also navigate the ethical considerations that accompany them, ensuring that advancements in technology benefit all patients equitably.
- What is the role of AI in healthcare? AI plays a crucial role in enhancing diagnostics, treatment planning, and patient monitoring, leading to more personalized and efficient care.
- How does predictive analytics improve patient care? Predictive analytics helps forecast patient health trends, allowing for proactive interventions and tailored treatment plans.
- What are the ethical concerns surrounding AI in healthcare? Ethical concerns include data privacy, algorithmic bias, and ensuring informed consent among patients.
- How can telemedicine benefit from AI? AI can enhance telemedicine by facilitating remote patient monitoring and improving access to care, especially in underserved areas.
- What are AI-driven patient engagement tools? These tools help improve communication between patients and healthcare providers, fostering better understanding and adherence to treatment plans.
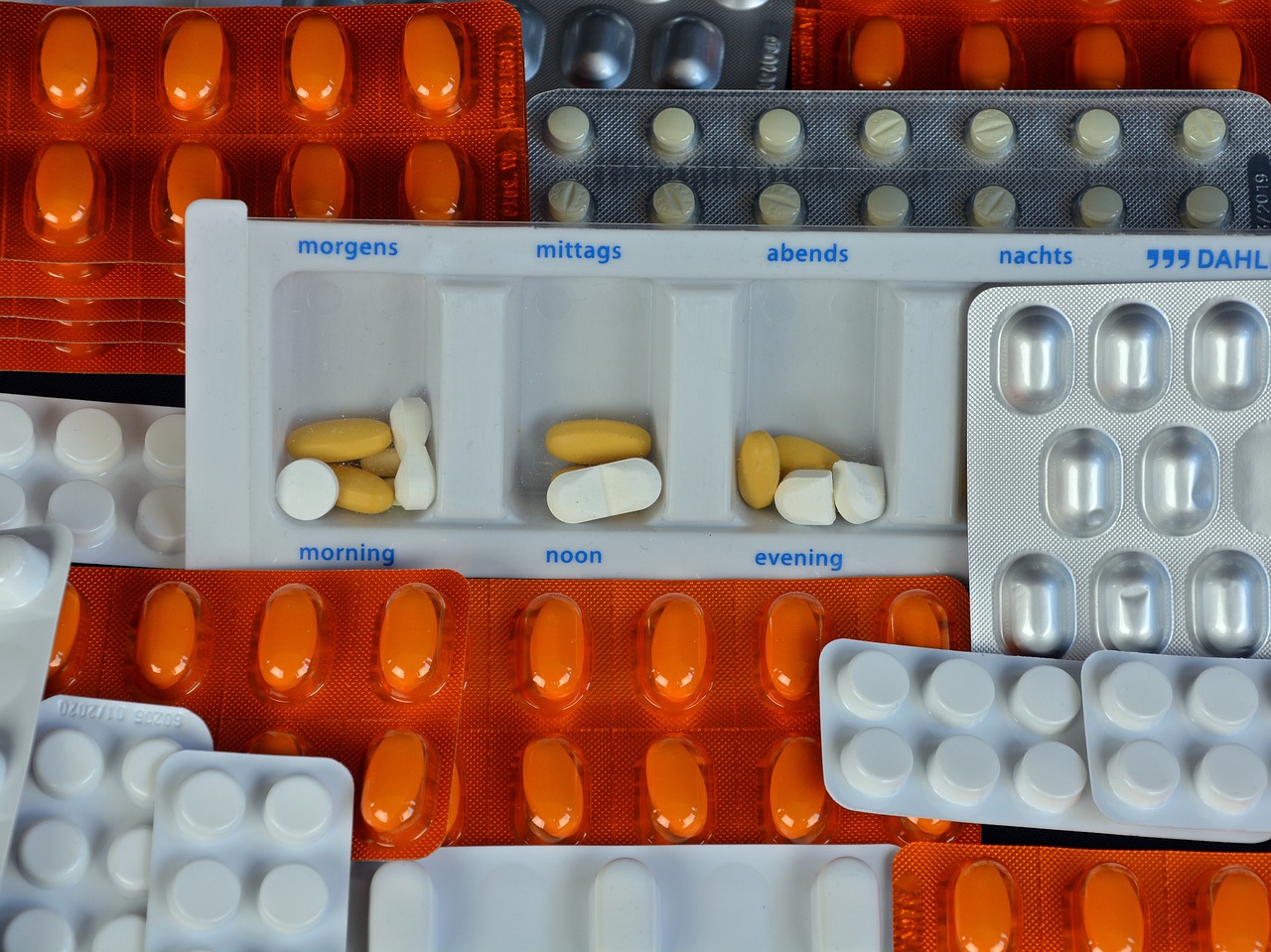
Telemedicine and AI Integration
In recent years, the convergence of telemedicine and artificial intelligence (AI) has revolutionized the way healthcare is delivered. Imagine a world where patients can receive expert medical advice from the comfort of their homes, while AI systems analyze their health data in real-time to provide personalized recommendations. This is no longer a distant dream; it's happening now, and the implications are profound.
Telemedicine has already made healthcare more accessible, especially for those in remote or underserved areas. By integrating AI into telemedicine platforms, healthcare providers can enhance the quality of care delivered remotely. For instance, AI algorithms can assist in triaging patients based on their symptoms, directing them to the appropriate level of care without unnecessary delays. This not only saves time but also reduces the burden on healthcare facilities.
One of the most exciting aspects of this integration is the ability of AI to analyze vast amounts of patient data quickly. For example, AI can monitor vital signs, medication adherence, and even patient-reported outcomes through mobile apps or wearable devices. This constant stream of data allows healthcare providers to detect potential health issues before they escalate, leading to proactive interventions. Imagine a scenario where an AI system alerts a doctor if a patient's heart rate spikes unexpectedly or if their glucose levels are trending dangerously high. Such timely alerts can be life-saving.
Moreover, AI-driven telemedicine tools can enhance patient engagement by providing tailored educational content and reminders. Patients can receive notifications about medication schedules, dietary recommendations, and upcoming appointments, all personalized to their specific health needs. This level of engagement not only empowers patients but also fosters a sense of ownership over their health.
However, the integration of AI into telemedicine is not without challenges. Issues such as data privacy, algorithmic bias, and the need for reliable internet access must be addressed to ensure equitable access to these advanced technologies. As healthcare providers navigate these challenges, the potential benefits of AI-enhanced telemedicine are too significant to ignore.
In summary, the integration of AI into telemedicine is paving the way for a more efficient, personalized, and accessible healthcare system. As technology continues to evolve, we can expect even more innovative solutions that will transform patient care and improve health outcomes.
- What is telemedicine? Telemedicine refers to the use of technology to provide medical care remotely, allowing patients to consult with healthcare providers without needing to visit a clinic or hospital.
- How does AI improve telemedicine? AI enhances telemedicine by analyzing patient data, assisting in diagnosis, and providing personalized care recommendations, ultimately leading to better health outcomes.
- Are there any challenges with AI in telemedicine? Yes, challenges include data privacy concerns, potential algorithmic bias, and ensuring all patients have reliable access to technology.
- Can telemedicine replace in-person visits? While telemedicine is a powerful tool, it may not completely replace in-person visits, especially for conditions that require physical examinations or procedures.
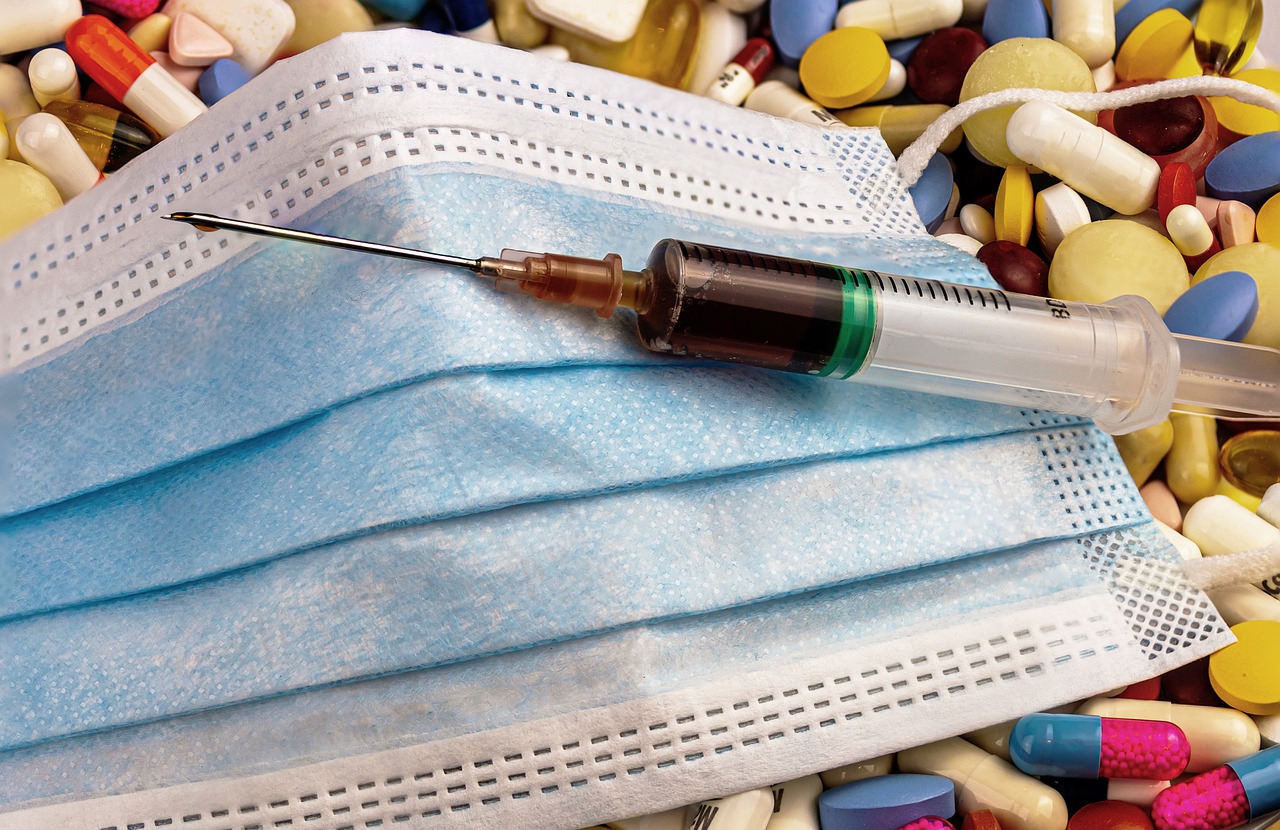
AI-driven Patient Engagement Tools
In today's fast-paced world, patient engagement is more critical than ever, and AI-driven tools are leading the charge in transforming this landscape. Imagine a world where your healthcare provider knows you so well that they can anticipate your needs even before you express them. That’s the power of AI! These tools are designed to enhance communication between patients and healthcare providers, making the entire experience more personalized and efficient.
One of the most exciting aspects of AI-driven patient engagement tools is their ability to analyze vast amounts of data to provide tailored recommendations. For instance, consider a patient managing a chronic condition like diabetes. AI can sift through their health records, lifestyle choices, and even genetic information to offer customized advice on diet, exercise, and medication adherence. This level of personalization not only empowers patients but also fosters a sense of ownership over their health.
Moreover, AI tools can facilitate seamless communication. Patients can use chatbots to ask questions about their treatment plans or medication side effects at any time, receiving instant responses that help alleviate their concerns. This 24/7 availability is especially beneficial for those living in remote areas where access to healthcare professionals might be limited. It’s like having a healthcare assistant right in your pocket!
Another remarkable feature of these tools is their capacity to remind patients about appointments, medication schedules, and even follow-up tests. By sending timely notifications via text or app alerts, AI ensures that patients remain engaged in their care journey. Think of it as a friendly nudge, helping you stay on track with your health goals.
Furthermore, AI-driven engagement tools can analyze patient feedback and satisfaction levels. By collecting and interpreting this data, healthcare providers can refine their services, addressing any gaps in care and improving overall patient experiences. It’s a continuous loop of feedback that enhances the quality of care over time.
To illustrate the impact of AI-driven patient engagement tools, let’s take a look at a table comparing traditional engagement methods with AI-enhanced approaches:
Aspect | Traditional Methods | AI-driven Methods |
---|---|---|
Communication | Phone calls and in-person visits | Chatbots and instant messaging |
Personalization | Generic advice | Customized recommendations based on data |
Availability | Limited office hours | 24/7 access via apps |
Feedback | Post-visit surveys | Real-time feedback collection and analysis |
As we look to the future, the integration of AI-driven patient engagement tools promises to revolutionize the healthcare experience. By placing patients at the center of their care, these technologies not only improve health outcomes but also foster a more collaborative relationship between patients and providers. In a world where health is paramount, these tools are paving the way for a brighter, healthier future.
- What are AI-driven patient engagement tools?
These are technologies that utilize artificial intelligence to enhance communication and interaction between patients and healthcare providers. - How do these tools improve patient care?
They provide personalized recommendations, facilitate seamless communication, and help patients manage their health more effectively. - Are AI-driven tools accessible to everyone?
While many are designed to be user-friendly, access may vary based on technology availability and patient demographics.
Frequently Asked Questions
- What are the main benefits of AI in healthcare?
AI brings a plethora of benefits to healthcare, including enhanced diagnostics, personalized treatment plans, and improved patient monitoring. By analyzing vast amounts of data, AI can identify patterns that humans may overlook, leading to faster and more accurate diagnoses.
- How does machine learning improve diagnostics?
Machine learning algorithms analyze patient data and medical images to identify diseases with greater accuracy and speed. This not only enhances patient outcomes but also reduces healthcare costs by minimizing the need for unnecessary tests and procedures.
- What role does predictive analytics play in patient care?
Predictive analytics uses historical data to forecast patient health trends, allowing healthcare providers to intervene proactively. This means that potential health issues can be addressed before they escalate, significantly improving the quality of care.
- Are there ethical concerns with AI in healthcare?
Yes, as AI becomes more integrated into healthcare, ethical concerns regarding data privacy, algorithmic bias, and informed consent arise. It's crucial for healthcare organizations to implement measures that ensure equitable access to AI technologies while safeguarding patient data.
- How can healthcare organizations ensure data privacy?
To ensure data privacy, healthcare organizations must adopt robust security measures, including encryption, access controls, and regular audits. These steps help protect sensitive patient information from breaches and misuse.
- What is algorithmic bias and why is it important?
Algorithmic bias occurs when AI systems produce unfair outcomes due to biased training data. Addressing this bias is vital to ensure that AI serves all patient populations fairly and equitably, ultimately leading to better health outcomes for everyone.
- What future trends can we expect in healthcare AI?
The future of healthcare AI looks promising, with trends like telemedicine integration, virtual health assistants, and AI-driven patient engagement tools on the rise. These advancements are set to reshape how patients interact with healthcare providers and access medical care.
- How does AI enhance telemedicine?
AI enhances telemedicine by improving remote patient monitoring and facilitating timely consultations. This integration ensures that patients, especially in underserved areas, receive prompt and effective care, ultimately improving health outcomes.
- What are AI-driven patient engagement tools?
AI-driven patient engagement tools are technologies that foster better communication between patients and healthcare providers. They help educate patients about their health, encourage adherence to treatment plans, and empower individuals to take an active role in their healthcare journey.