Emerging AI Trends in Cybersecurity
In today's digital landscape, the intersection of artificial intelligence (AI) and cybersecurity has become a hot topic, sparking conversations about how these technologies can work together to fend off increasingly sophisticated cyber threats. As organizations around the globe grapple with the rising tide of cyberattacks, the integration of AI into their security frameworks is not just an option; it’s a necessity. Imagine a world where your security systems are not just reactive, but proactive, anticipating threats before they materialize. This is the promise of AI in cybersecurity, and it’s reshaping how we think about digital safety.
The rapid advancements in AI technologies have opened up new avenues for combating cyber threats effectively. From real-time threat detection to automated incident response, AI is enhancing the way organizations protect their sensitive data. With the ability to analyze massive datasets at lightning speed, AI-driven solutions can identify unusual patterns and potential vulnerabilities that human analysts might miss. This capability is crucial in a landscape where cybercriminals are constantly evolving their tactics, making it imperative for security measures to keep pace.
Furthermore, the integration of machine learning algorithms allows systems to continuously learn and adapt from new data, improving their accuracy over time. This means that the more they are used, the better they become at spotting threats. It’s like having a security guard who learns from every incident and becomes more vigilant with each passing day. As organizations harness the power of AI, they are not only enhancing their defenses but also gaining a competitive edge in the battle against cybercrime.
In this article, we will delve into the various AI-driven trends reshaping cybersecurity, exploring how they are implemented and the profound impact they have on protecting sensitive information. We will also take a closer look at how AI is enhancing data privacy, preventing phishing attacks, and streamlining incident response processes. With the stakes higher than ever, understanding these trends is vital for any organization looking to fortify its cybersecurity posture.
- What is AI's role in cybersecurity? AI helps in identifying and mitigating threats by analyzing data patterns and automating responses to incidents.
- How does machine learning improve threat detection? Machine learning algorithms learn from historical data, allowing them to predict and identify new threats more effectively.
- Can AI prevent phishing attacks? Yes, AI technologies can analyze emails and detect potential phishing attempts before they reach users.
- Is AI enough to secure an organization? While AI significantly enhances security measures, it should be part of a comprehensive cybersecurity strategy that includes human oversight and other technologies.
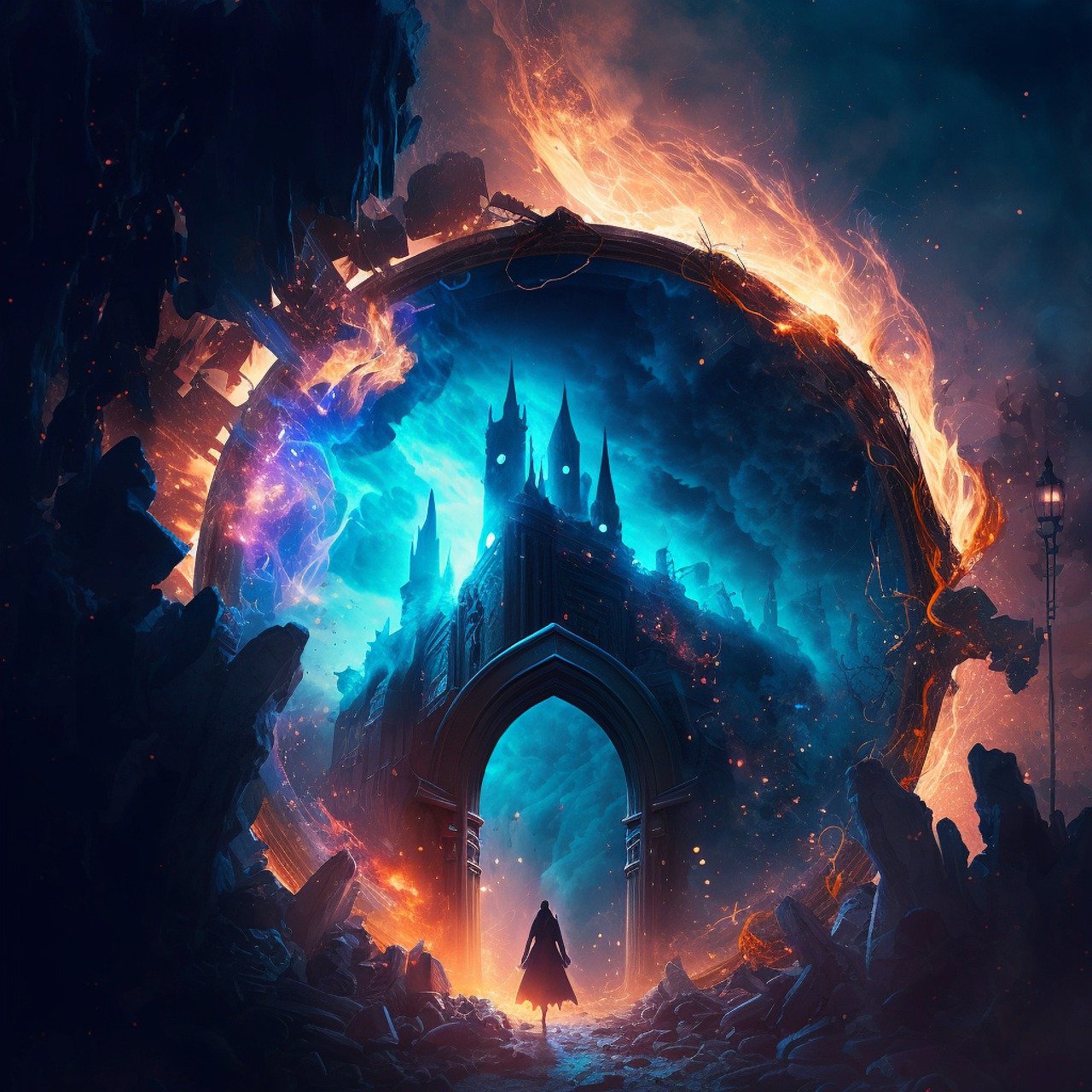
AI-Driven Threat Detection
In today's digital landscape, the stakes are higher than ever when it comes to cybersecurity. With cyber threats evolving at an alarming rate, organizations are turning to as a powerful ally in their defense strategies. Imagine having a vigilant guard that never sleeps, tirelessly analyzing vast amounts of data in real time. This is precisely what AI technologies bring to the table. By leveraging advanced algorithms and machine learning, companies can identify potential security breaches before they escalate into full-blown crises.
AI's ability to process and analyze data at lightning speed allows it to spot patterns and anomalies that might elude human analysts. For instance, traditional methods of threat detection often rely on static rules and signatures, which can become outdated as attackers develop new tactics. In contrast, AI systems continuously learn from new data, adapting to the ever-changing landscape of cyber threats. This proactive approach not only enhances the speed of detection but also significantly reduces the risk of false positives, allowing security teams to focus on genuine threats.
Furthermore, AI-driven threat detection can be integrated with existing security infrastructures, creating a comprehensive defense mechanism. This integration empowers organizations to harness the full potential of their security tools, making them more efficient and effective. As cybercriminals become increasingly sophisticated, relying solely on traditional methods is akin to bringing a knife to a gunfight. Organizations must evolve, and AI is the key to staying one step ahead.
To illustrate the impact of AI in threat detection, consider the following table outlining the differences between traditional and AI-driven approaches:
Feature | Traditional Threat Detection | AI-Driven Threat Detection |
---|---|---|
Speed | Slower response times | Real-time analysis |
Adaptability | Static rules | Dynamic learning |
False Positives | Higher rates | Significantly lower rates |
Integration | Limited | Seamless integration with existing tools |
In conclusion, AI-driven threat detection is not just a trend; it is a necessary evolution in the fight against cybercrime. By harnessing the power of artificial intelligence, organizations can fortify their defenses, ensuring they are well-equipped to tackle the challenges of tomorrow. As we continue to witness advancements in AI technologies, the potential for even more innovative threat detection methods is boundless, paving the way for a more secure digital future.
- What is AI-driven threat detection? AI-driven threat detection involves using artificial intelligence technologies to analyze data in real-time, identifying potential cyber threats before they escalate.
- How does AI improve threat detection? AI improves threat detection by continuously learning from new data patterns, adapting to evolving attack strategies, and reducing the risk of false positives.
- Can AI be integrated with existing security systems? Yes, AI-driven threat detection can seamlessly integrate with existing security infrastructures, enhancing their effectiveness.
- What are the benefits of using AI in cybersecurity? Benefits include faster response times, dynamic learning capabilities, lower false positive rates, and improved overall security posture.
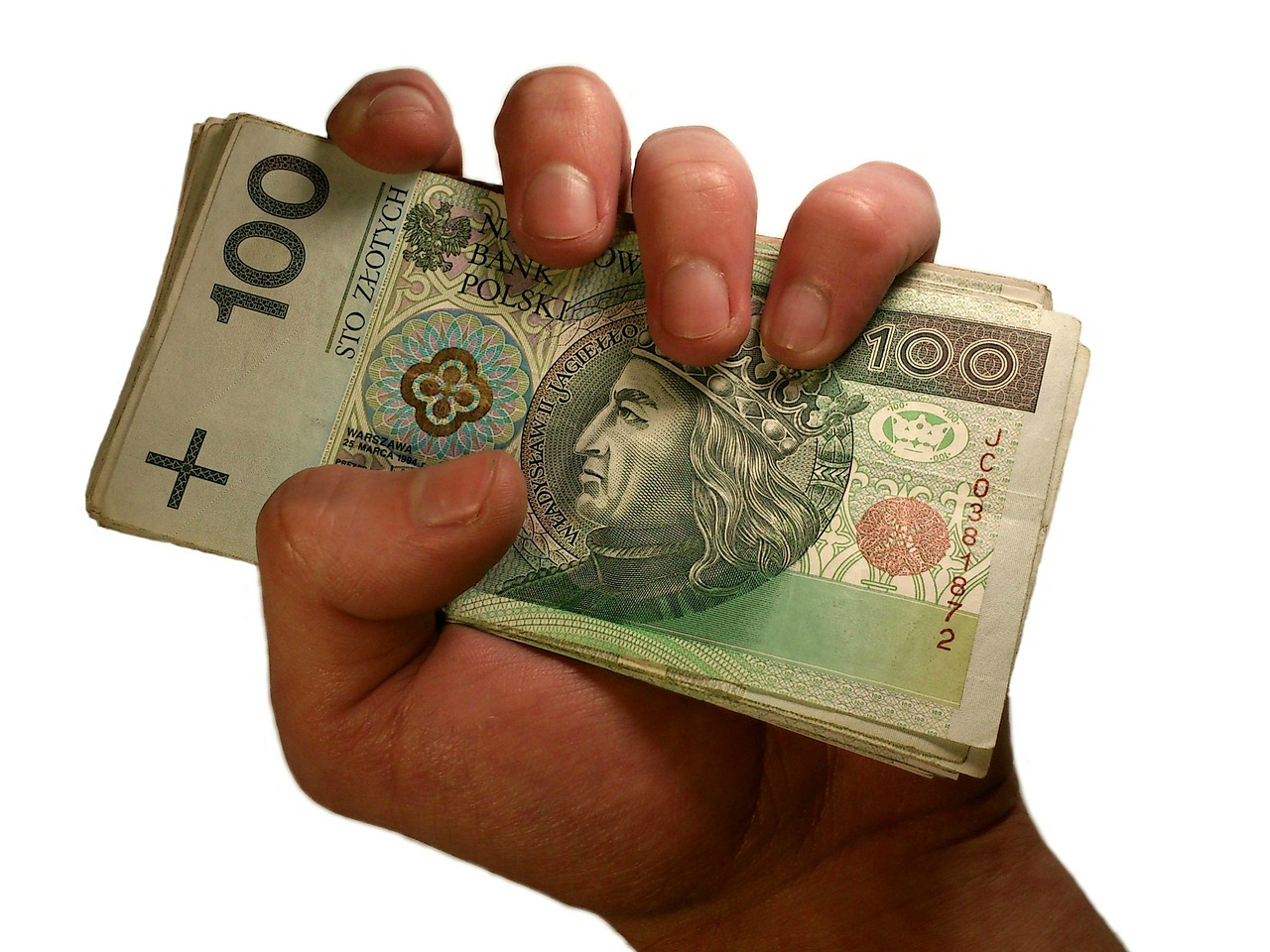
Automated Incident Response
In today's fast-paced digital landscape, where cyber threats lurk around every corner, the need for swift and efficient incident response has never been more critical. Automated incident response systems are emerging as a game-changer, revolutionizing how organizations tackle cyberattacks. Imagine having a virtual security guard that never sleeps, constantly monitoring your digital environment, ready to spring into action at a moment's notice. That's the essence of automation in cybersecurity.
By leveraging the power of artificial intelligence, these automated systems can analyze vast amounts of data in real time, allowing them to detect threats and respond to incidents much faster than a human could. This not only minimizes the potential damage caused by a breach but also frees up valuable resources, enabling security teams to focus on more complex tasks that require human intervention.
One of the most compelling features of automated incident response is its ability to execute predefined actions based on specific triggers. For instance, if a suspicious login attempt is detected, the system can automatically lock the user account, notify the security team, and even initiate a password reset—all without human input. This rapid response can be the difference between a minor incident and a full-blown security breach.
Moreover, automation allows for consistent responses to incidents, reducing the likelihood of human error. Security teams can establish a set of protocols that the automated system follows, ensuring that every incident is handled uniformly. This consistency is crucial, especially in high-pressure situations where every second counts.
However, while automation brings numerous benefits, it’s essential to remember that it’s not a silver bullet. Organizations must implement a well-rounded strategy that combines automated systems with human expertise. For example, while the automated system can handle initial responses, human analysts are still needed to investigate the root cause of incidents and develop long-term solutions.
To illustrate the impact of automated incident response, consider the following table that compares traditional incident response methods with automated approaches:
Aspect | Traditional Response | Automated Response |
---|---|---|
Speed | Slower, dependent on human availability | Instantaneous, 24/7 monitoring |
Consistency | Varies by individual response | Uniform, based on predefined protocols |
Resource Allocation | High, requires significant human involvement | Low, frees up human resources for strategic tasks |
Error Rate | Higher, prone to human mistakes | Lower, follows programmed responses |
In conclusion, automated incident response systems represent a significant advancement in the fight against cyber threats. By combining the speed and accuracy of AI with human oversight, organizations can create a robust defense against potential breaches. As technology continues to evolve, embracing these automated solutions will be crucial for maintaining security in an increasingly complex digital world.
- What is automated incident response? Automated incident response refers to the use of AI-driven systems to detect and respond to cybersecurity incidents without human intervention.
- How does it improve cybersecurity? By enabling faster response times, reducing human error, and ensuring consistent actions during incidents.
- Can automated systems replace human security teams? No, while automation enhances efficiency, human expertise is still vital for investigating incidents and developing strategies.
- What are some examples of automated responses? Actions like locking accounts, resetting passwords, and alerting security teams can be automated based on predefined triggers.
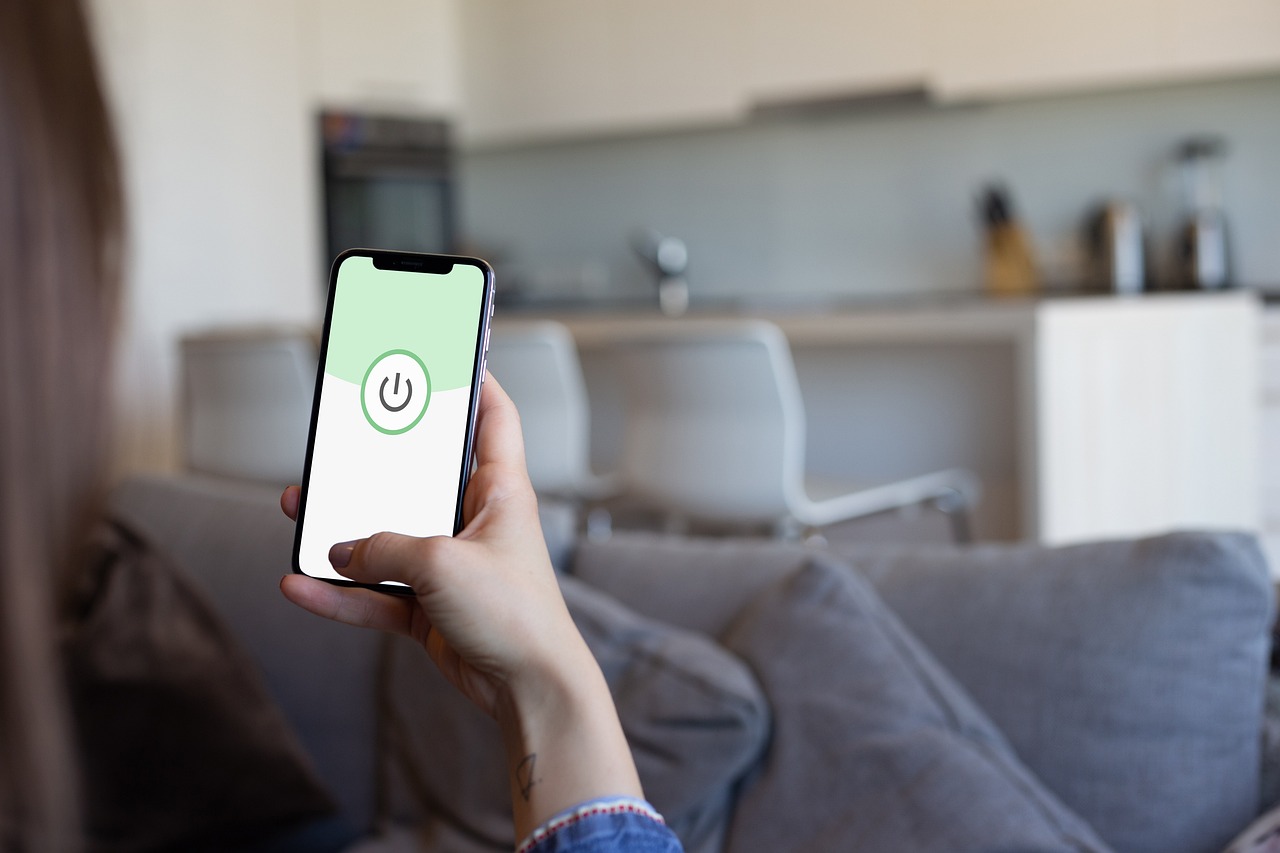
Machine Learning Algorithms
In the ever-evolving landscape of cybersecurity, have emerged as a game-changer, transforming the way organizations defend against cyber threats. These algorithms are not just simple programs; they are sophisticated systems that learn from data and adapt over time. Imagine having a security guard who not only watches for intruders but also learns from every incident to become more effective at their job. That’s precisely what machine learning does for cybersecurity.
At the heart of machine learning is the ability to process and analyze vast amounts of data at lightning speed. This capability allows organizations to identify patterns and anomalies that would be impossible for humans to detect in real time. For instance, a machine learning model can sift through millions of network logs, user activities, and system alerts to pinpoint unusual behaviors that may indicate a security breach. This proactive approach means that potential threats can be identified before they escalate into full-blown attacks, saving organizations time, money, and reputational damage.
One of the most significant advantages of machine learning in cybersecurity is its predictive capability. By continuously learning from new data, these algorithms can adapt to evolving attack strategies. For example, if a new type of malware emerges, a well-trained machine learning model can quickly recognize its characteristics and update its detection methods accordingly. This adaptability is crucial in a world where cybercriminals are constantly innovating and finding new ways to exploit vulnerabilities.
Moreover, machine learning can enhance threat detection through various techniques, such as:
- Supervised Learning: This involves training models on labeled datasets, where the algorithm learns to classify data based on known outcomes. For example, it can identify whether an email is spam or legitimate.
- Unsupervised Learning: Unlike supervised learning, this method does not rely on labeled data. Instead, it finds hidden patterns or groupings in data, which can be particularly useful for identifying previously unknown threats.
- Reinforcement Learning: This approach uses feedback from previous actions to improve future decision-making. It’s akin to teaching a dog new tricks by rewarding it for good behavior—over time, the algorithm learns the most effective ways to respond to threats.
To illustrate the impact of machine learning algorithms in cybersecurity, consider the following table that highlights key capabilities and benefits:
Capability | Benefit |
---|---|
Real-time Analysis | Immediate identification of threats as they occur. |
Pattern Recognition | Ability to detect complex attack vectors and behaviors. |
Adaptive Learning | Continuous improvement in threat detection accuracy. |
Scalability | Ability to handle growing amounts of data without compromising performance. |
In summary, machine learning algorithms are revolutionizing the cybersecurity landscape by providing organizations with the tools they need to detect, analyze, and respond to threats more effectively. By leveraging these advanced technologies, businesses can not only enhance their security posture but also stay one step ahead of cybercriminals. As we forge ahead into an increasingly digital future, the integration of machine learning in cybersecurity will undoubtedly become a cornerstone of effective threat management strategies.
Q: What is machine learning in cybersecurity?
A: Machine learning in cybersecurity refers to the use of algorithms that can learn from data to identify patterns and detect potential threats in real time.
Q: How does machine learning improve threat detection?
A: By analyzing vast amounts of data and continuously adapting to new information, machine learning enhances the ability to recognize unusual behaviors that may indicate cyber threats.
Q: Can machine learning algorithms keep up with evolving cyber threats?
A: Yes, machine learning algorithms are designed to learn and adapt, allowing them to stay current with new attack strategies and techniques used by cybercriminals.
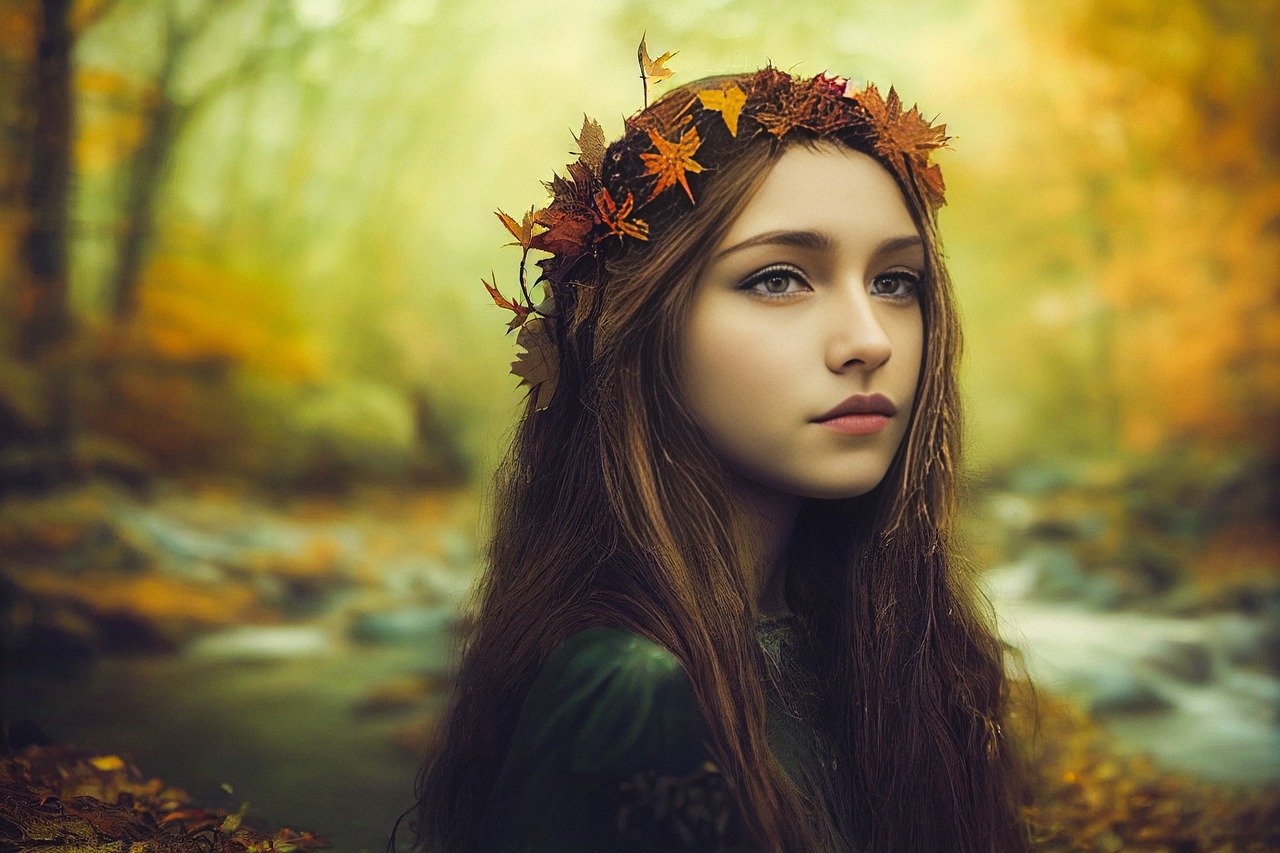
Behavioral Analysis
Behavioral analysis is rapidly becoming a cornerstone of modern cybersecurity strategies. Imagine a security system that learns about its users just like a trusted friend would. This is precisely what behavioral analysis aims to achieve. By monitoring user activities and identifying patterns, AI can detect anomalies that could indicate a potential security breach. For instance, if an employee suddenly accesses sensitive files at odd hours or from an unusual location, the system can flag this behavior for further investigation. This proactive approach not only helps in identifying threats but also significantly reduces the time it takes to respond to them.
One of the most compelling aspects of behavioral analysis is its ability to adapt and evolve. As cyber threats become increasingly sophisticated, traditional security measures often fall short. However, with AI-driven behavioral analysis, organizations can stay one step ahead. The system continuously learns from new data, refining its understanding of what constitutes "normal" behavior for each user. This means that even if a new type of attack emerges, the system can quickly adjust its parameters to detect it.
Furthermore, the implementation of behavioral analysis does not require a complete overhaul of existing security frameworks. Instead, it can be integrated into current systems, enhancing their capabilities. Organizations can utilize behavioral analysis in conjunction with other security measures, creating a multi-layered defense strategy. This synergy is crucial because, in the world of cybersecurity, relying on a single method can be akin to leaving a door wide open for intruders.
To illustrate the effectiveness of behavioral analysis, consider a scenario where an employee’s account is compromised. The attacker might begin by mimicking the employee's typical activities, making it challenging for traditional security systems to detect the intrusion. However, a behavioral analysis system would notice deviations from the established pattern, such as unusual login times or access to unfamiliar data. This early detection capability can be the difference between a minor incident and a full-blown data breach.
Overall, behavioral analysis represents a significant advancement in the fight against cyber threats. By focusing on user behavior, organizations can enhance their security posture and better protect sensitive information. As we continue to navigate the complexities of the digital landscape, embracing such innovative techniques will be essential for maintaining robust cybersecurity defenses.
- What is behavioral analysis in cybersecurity?
Behavioral analysis involves monitoring user activities to identify patterns and detect anomalies that may indicate security threats. - How does AI improve behavioral analysis?
AI enhances behavioral analysis by continuously learning from data, allowing it to adapt to new threats and refine its detection capabilities. - Can behavioral analysis be integrated with existing security systems?
Yes, behavioral analysis can complement and enhance existing security frameworks without requiring a complete overhaul. - Why is behavioral analysis important for organizations?
It helps organizations detect potential security breaches early, reducing the risk of data loss and ensuring better protection of sensitive information.
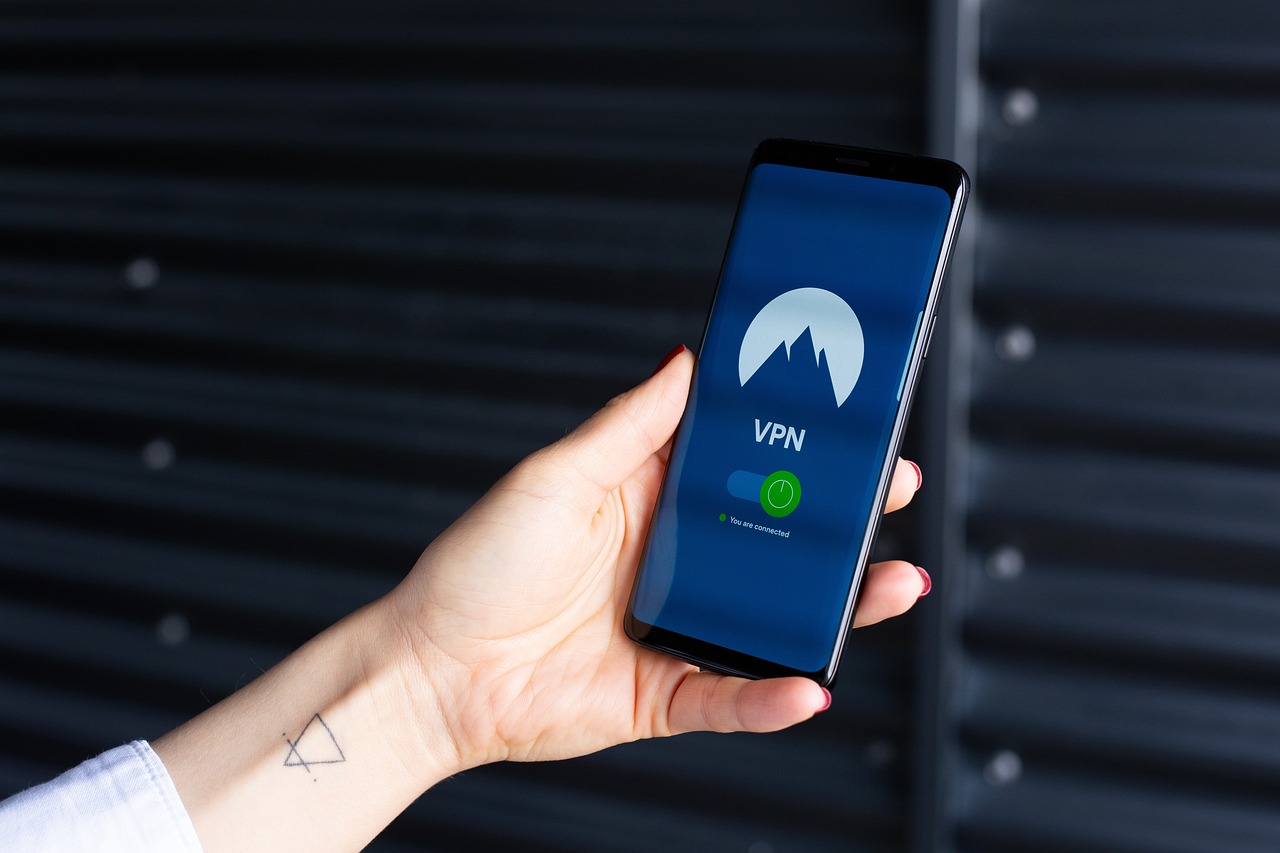
Anomaly Detection Systems
Anomaly detection systems are a vital component in the arsenal of modern cybersecurity strategies. These systems leverage the power of artificial intelligence to monitor network activities continuously, identifying irregular patterns that could signify a potential threat. Imagine having a security guard who not only watches the door but also knows the usual flow of people and can spot when something feels off. That's precisely what anomaly detection systems do—they learn the normal behavior of users and systems, so they can quickly flag anything that deviates from the norm.
One of the most exciting aspects of these systems is their ability to adapt over time. As they process more data, they improve their understanding of what constitutes normal behavior, which enhances their detection capabilities. This is especially important in today’s rapidly evolving cyber landscape, where attackers are constantly refining their techniques. For instance, if a user typically logs in from a specific location and suddenly attempts to access the network from an unfamiliar country, the system can raise an alert, prompting security teams to investigate further.
To illustrate how anomaly detection systems work, consider the following table that outlines the key components involved in their operation:
Component | Description |
---|---|
Data Collection | Gathering data from various sources such as user activity logs, network traffic, and system performance metrics. |
Data Processing | Analyzing the collected data to establish a baseline of normal behavior. |
Detection Algorithms | Utilizing machine learning algorithms to identify deviations from the established baseline. |
Alerting Mechanism | Notifying security teams of potential anomalies for further investigation. |
Moreover, the implementation of anomaly detection systems can significantly reduce the time it takes to identify and respond to threats. Instead of relying solely on traditional methods, which often involve manual monitoring and analysis, these systems provide a much-needed layer of automation. By doing so, they free up valuable resources within security teams, allowing them to focus on more strategic tasks rather than being bogged down by routine monitoring.
However, it’s important to note that while anomaly detection systems are powerful tools, they are not foolproof. False positives can occur, where legitimate user activities are flagged as suspicious. To mitigate this, organizations must continuously refine their algorithms and incorporate feedback from security analysts to improve the system's accuracy. This ongoing process ensures that the anomaly detection systems remain effective in a landscape where cyber threats are becoming increasingly sophisticated.
In conclusion, anomaly detection systems represent a significant advancement in the field of cybersecurity. By harnessing the capabilities of AI, these systems not only enhance the detection of potential threats but also improve the overall efficiency of security operations. As organizations continue to face a barrage of cyber threats, investing in robust anomaly detection systems will be crucial in safeguarding sensitive information and maintaining trust with clients and stakeholders.
- What is an anomaly detection system? An anomaly detection system is an AI-powered tool that monitors network activities to identify unusual patterns that may indicate a security threat.
- How do anomaly detection systems improve over time? They continuously learn from new data, refining their understanding of normal behavior and enhancing their ability to detect deviations.
- Can anomaly detection systems generate false positives? Yes, these systems can sometimes flag legitimate activities as suspicious, so it's essential to regularly update and refine their algorithms.
- Why are anomaly detection systems important for cybersecurity? They provide real-time monitoring and rapid detection of potential threats, enabling organizations to respond promptly and minimize damage.
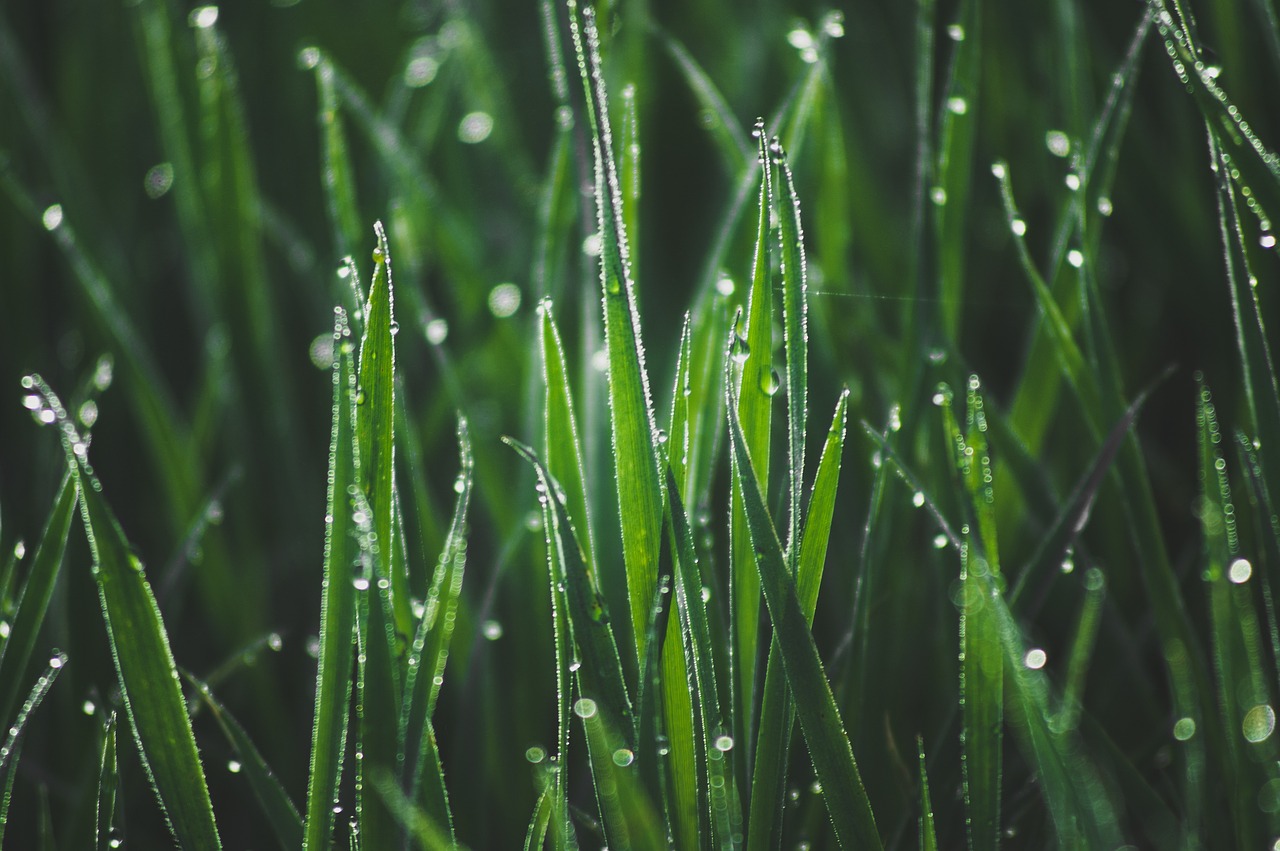
Predictive Analytics
In the ever-evolving landscape of cybersecurity, has emerged as a vital tool for organizations aiming to stay one step ahead of cybercriminals. By leveraging the power of artificial intelligence, predictive analytics allows businesses to analyze historical data and identify patterns that can indicate potential future threats. Imagine having a crystal ball that not only reveals past incidents but also forecasts what might happen next—this is the essence of predictive analytics in the realm of cybersecurity.
At its core, predictive analytics involves the use of sophisticated algorithms that sift through massive datasets to uncover trends and correlations. This process is akin to piecing together a complex puzzle; every data point contributes to a clearer picture of what vulnerabilities exist and how they might be exploited. For instance, if a particular type of attack has been on the rise in a specific sector, predictive analytics can help organizations anticipate similar attacks on their own systems, thereby allowing them to fortify their defenses in advance.
Furthermore, predictive analytics doesn't operate in a vacuum. It continuously learns and adapts based on new data inputs, much like how a seasoned detective refines their methods after each case. By incorporating machine learning techniques, these systems can evolve alongside emerging threats, ensuring that organizations are not just reactive but proactive in their security measures. This adaptability is crucial, as cyber threats are becoming increasingly sophisticated and varied.
To illustrate the potential of predictive analytics, consider the following table that outlines its key benefits:
Benefit | Description |
---|---|
Proactive Threat Mitigation | Identifies vulnerabilities before they can be exploited. |
Resource Optimization | Helps allocate security resources more effectively based on predicted threats. |
Enhanced Decision-Making | Provides data-driven insights for informed strategic planning. |
Reduced Incident Response Time | Enables quicker reactions to potential threats, minimizing damage. |
As organizations increasingly adopt predictive analytics, they also benefit from improved collaboration between security teams and data scientists. This partnership fosters a culture of continuous improvement, where feedback loops help refine predictive models and enhance overall security posture. In this way, predictive analytics not only bolsters defenses but also cultivates a more informed and agile cybersecurity workforce.
In conclusion, predictive analytics stands as a game-changer in the cybersecurity arena, offering organizations the foresight needed to combat the ever-present threat of cyberattacks. By harnessing the power of AI and machine learning, businesses can anticipate potential risks and fortify their defenses, ultimately creating a more secure digital environment.
- What is predictive analytics in cybersecurity? Predictive analytics involves using historical data and machine learning algorithms to forecast potential cyber threats and vulnerabilities.
- How does predictive analytics improve security? It helps organizations identify and mitigate risks before they materialize, allowing for proactive security measures.
- Can predictive analytics adapt to new threats? Yes, predictive analytics systems continuously learn from new data, enabling them to adapt to evolving cyber threats.
- What are the benefits of using predictive analytics? Benefits include proactive threat mitigation, resource optimization, enhanced decision-making, and reduced incident response times.
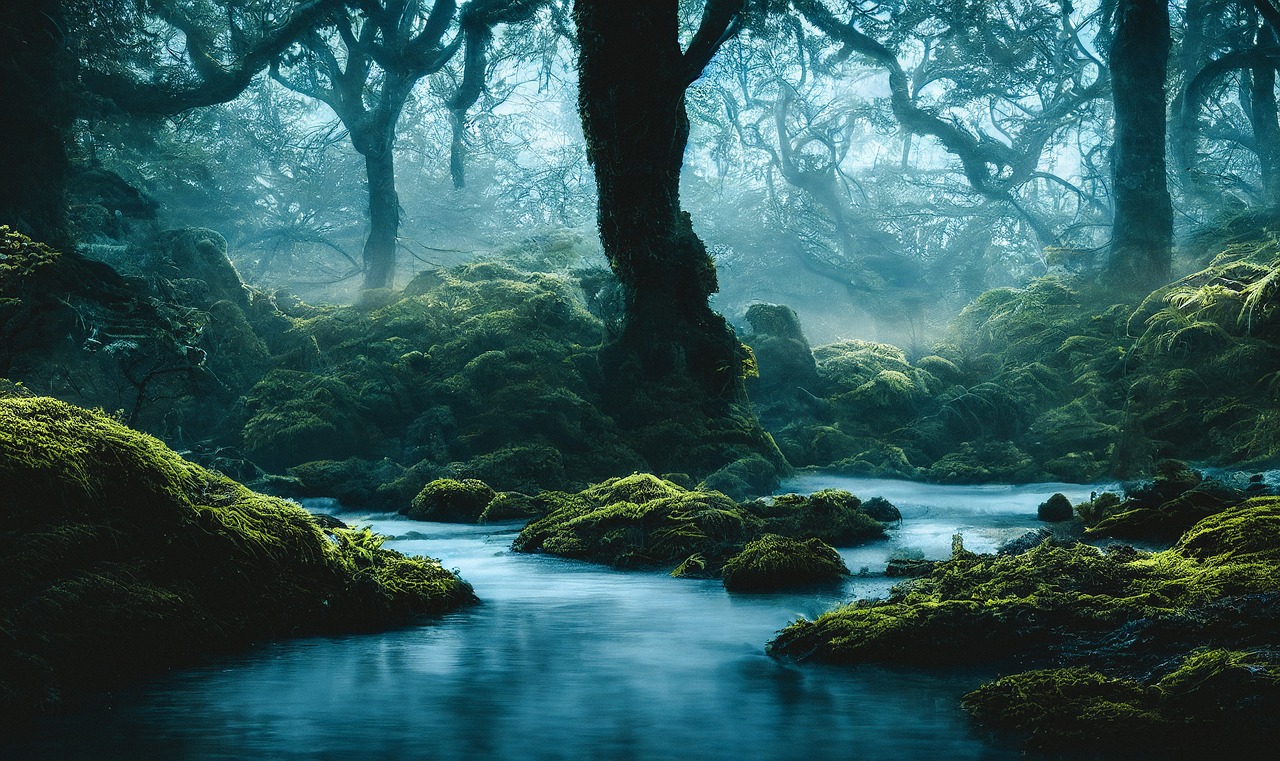
Enhanced Data Privacy
In today's digital landscape, where data breaches are as common as morning coffee, the importance of cannot be overstated. With the rapid advancement of technology, particularly in the realm of artificial intelligence, organizations are now better equipped to protect sensitive information from unauthorized access. This is not just about compliance with regulations; it's about safeguarding the trust that customers place in businesses. Imagine your personal data as a treasure chest; without proper locks and guards, it’s just waiting to be plundered.
AI is playing a pivotal role in this protective gear. Through sophisticated algorithms, it enables organizations to implement data encryption techniques that are more robust than ever. These advanced methods not only secure data at rest but also during transmission. Picture this: when you send a message, it’s like putting it in a secure vault that only the intended recipient can open. This ensures that even if cybercriminals intercept the message, they find nothing but gibberish.
Moreover, the development of privacy-preserving AI models has taken data privacy a step further. These models are designed to analyze data without compromising the personal information contained within it. Think of it as a chef who can create a delicious dish without revealing the secret ingredients. This capability allows organizations to leverage AI for insights while adhering to stringent privacy standards, such as GDPR and CCPA.
To illustrate the impact of these technologies, consider the following table that outlines the key features of modern data privacy solutions:
Feature | Description |
---|---|
Data Encryption | Transforms data into a secure format that can only be read by authorized users. |
Privacy-Preserving Models | Allows data analysis without exposing personal information. |
Real-Time Monitoring | Continuously checks for unauthorized access or anomalies in data usage. |
Regulatory Compliance | Ensures that data handling practices meet legal standards. |
As organizations continue to navigate the complexities of data privacy, the integration of AI technologies will be crucial. Not only do these advancements help in protecting sensitive information, but they also foster a culture of transparency and trust. After all, in a world where data is the new oil, ensuring its safety is akin to fortifying a fortress. The future of cybersecurity is not just about defending against attacks; it's about creating a safe environment where data can thrive without fear.
- What is enhanced data privacy? Enhanced data privacy refers to improved measures and technologies used to protect sensitive information from unauthorized access and breaches.
- How does AI contribute to data privacy? AI enhances data privacy through advanced encryption techniques and privacy-preserving models that analyze data without compromising personal information.
- Why is data encryption important? Data encryption is crucial as it secures sensitive information by converting it into a format that unauthorized users cannot read, thus protecting it from cyber threats.
- What are privacy-preserving AI models? These models allow organizations to utilize AI for data analysis while ensuring that personal data remains confidential and complies with privacy regulations.
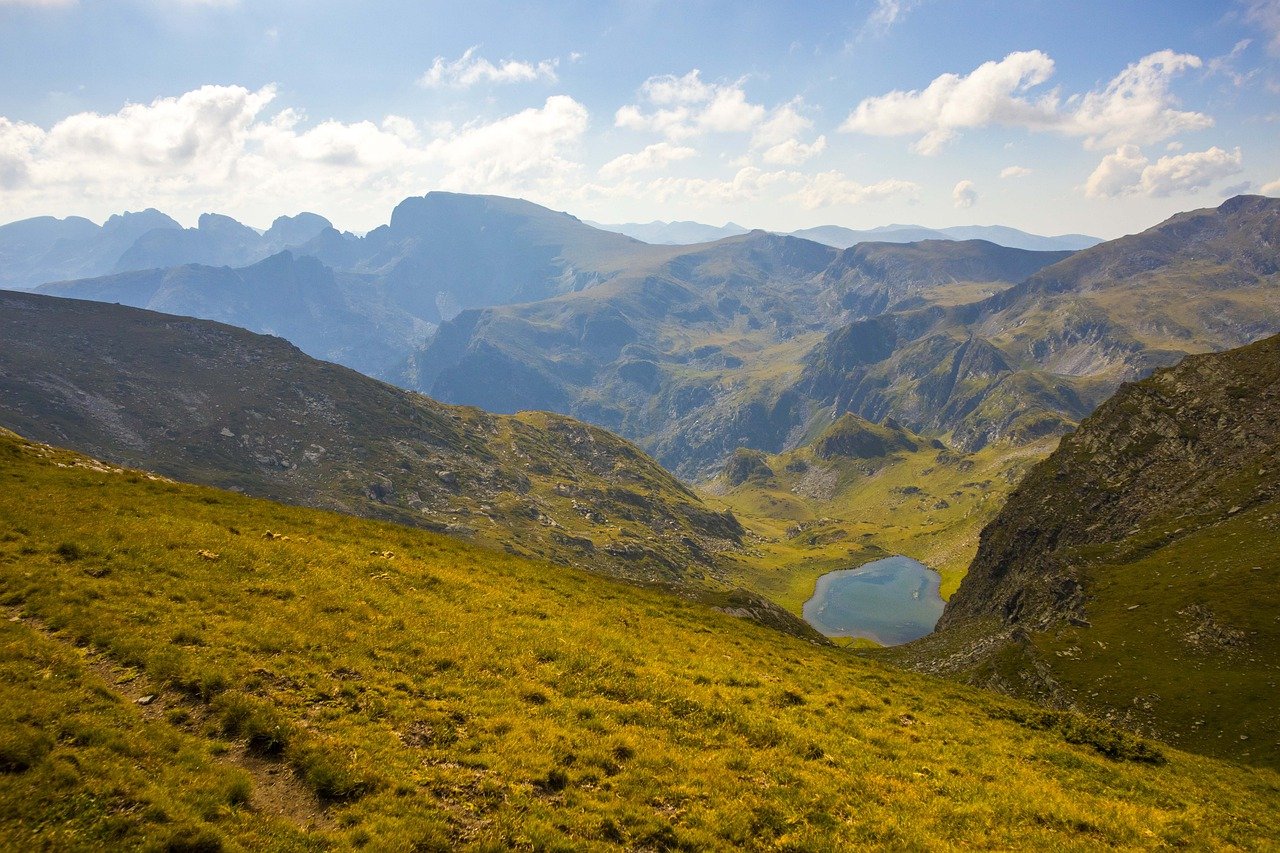
Data Encryption Techniques
In today's digital landscape, where data breaches and cyber threats loom large, have emerged as a cornerstone of cybersecurity. These techniques act like a fortress, safeguarding sensitive information from prying eyes. Imagine sending a secret message that only the intended recipient can read; that's essentially what encryption does for your data. It transforms your information into a code, making it unreadable to anyone who doesn't possess the key to decrypt it. This transformation is crucial, especially for organizations handling sensitive data such as personal identification information, financial records, and intellectual property.
There are various encryption methods, each with its own strengths and weaknesses. The most commonly used techniques include Symmetric Encryption and Asymmetric Encryption. Symmetric encryption uses a single key for both encryption and decryption, which makes it fast and efficient. However, the challenge lies in securely sharing that key. On the other hand, asymmetric encryption employs a pair of keys—a public key for encryption and a private key for decryption. This method is more secure for sharing data over the internet, as the private key never leaves the sender's device.
To illustrate the differences, take a look at the following table:
Encryption Type | Key Usage | Speed | Security |
---|---|---|---|
Symmetric Encryption | Single Key | Fast | Requires secure key sharing |
Asymmetric Encryption | Key Pair (Public and Private) | Slower | More secure for data sharing |
Moreover, advanced AI algorithms are being developed to enhance these encryption techniques. For instance, machine learning can help in identifying patterns and anomalies in data access, allowing for dynamic adjustments to encryption methods based on real-time threats. This adaptive approach ensures that even if a particular encryption method is compromised, organizations can pivot quickly to a more secure alternative.
In addition to traditional encryption methods, there's a rising trend in quantum encryption, which leverages the principles of quantum mechanics to secure data. This method promises unprecedented levels of security, as it makes it nearly impossible for hackers to intercept and decode information without detection. It's like trying to sneak a peek at a magician's trick—if you try to observe it, the very act of observation changes the outcome!
As organizations strive to comply with stringent data protection regulations, such as GDPR and HIPAA, the need for robust encryption techniques becomes even more critical. These regulations mandate that sensitive data must be protected with appropriate safeguards, and encryption is often a key component of those safeguards. In essence, implementing effective data encryption not only protects sensitive information but also fosters trust among customers and stakeholders.
In conclusion, as cyber threats evolve, so too must our approach to data security. By embracing advanced data encryption techniques and integrating them with AI technologies, organizations can build a resilient defense against potential breaches. This proactive stance not only protects sensitive information but also reinforces the integrity and reputation of the organization in the digital realm.
- What is data encryption? Data encryption is the process of converting information into a code to prevent unauthorized access.
- Why is encryption important? Encryption is crucial for protecting sensitive data from breaches and ensuring compliance with data protection regulations.
- What are the common types of encryption? The most common types are symmetric encryption and asymmetric encryption.
- How does AI enhance encryption? AI can identify patterns in data access and adapt encryption methods in real-time to respond to threats.
- What is quantum encryption? Quantum encryption uses quantum mechanics principles to secure data, offering high levels of security against interception.
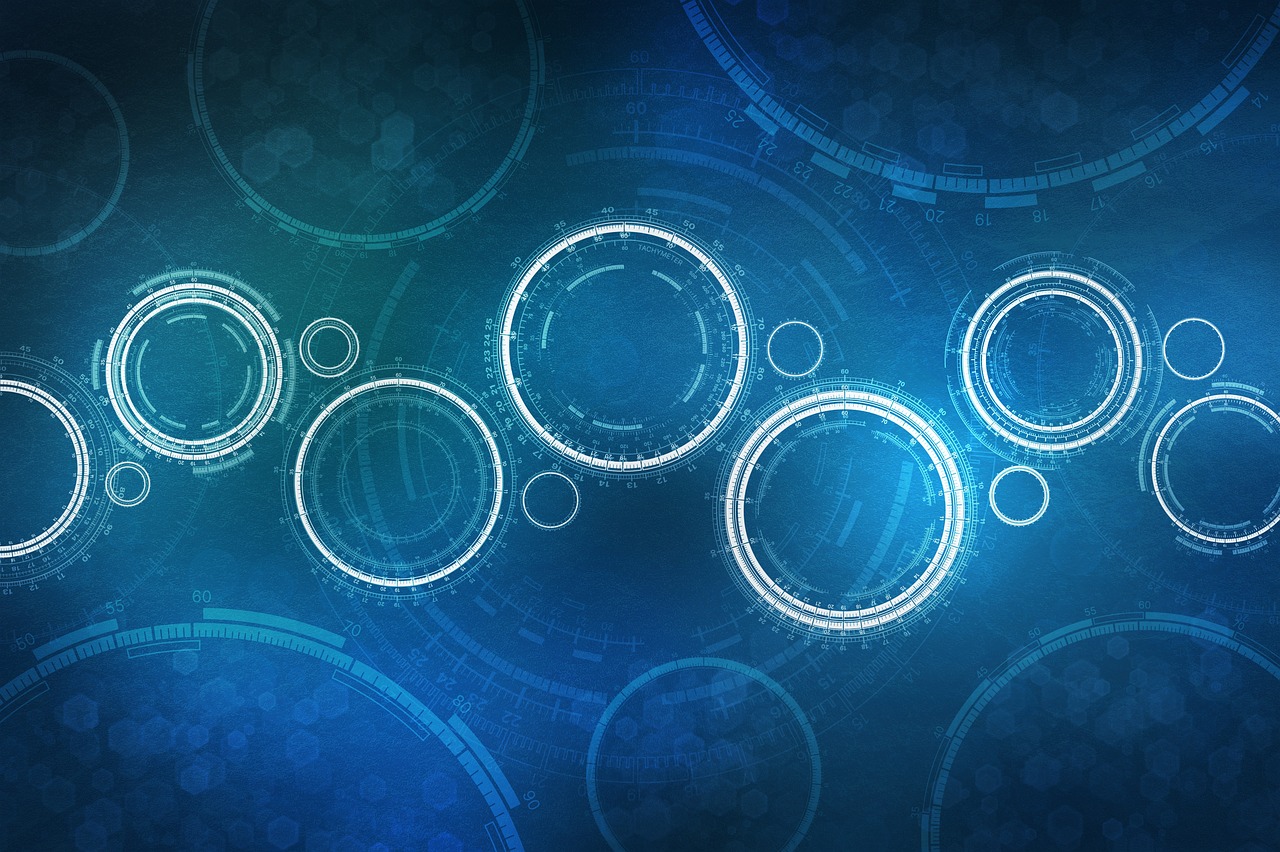
Privacy-Preserving AI Models
In today's digital age, where data is the new oil, are becoming increasingly vital. These models are designed to analyze data without compromising the privacy of individuals, ensuring that sensitive information remains protected even during complex analyses. Imagine a world where you can harness the power of AI while keeping your personal data safe—this is the promise of privacy-preserving AI.
One of the key techniques used in these models is federated learning. Instead of sending all data to a central server for processing, federated learning allows algorithms to learn from data stored on local devices. This means that the raw data never leaves the device, significantly reducing the risk of data breaches. It's like having a team of chefs who can learn new recipes without ever sharing their secret ingredients!
Moreover, privacy-preserving AI models often utilize homomorphic encryption. This advanced form of encryption allows computations to be performed on encrypted data without needing to decrypt it first. As a result, organizations can analyze data while keeping it secure. Think of it as being able to read a book without ever opening it—an impressive feat that maintains confidentiality!
Another exciting aspect of these models is their ability to comply with data protection regulations, such as the General Data Protection Regulation (GDPR) in Europe. By ensuring that personal data is anonymized and protected during analysis, organizations can avoid hefty fines and build trust with their customers. In a world where data breaches can lead to significant financial and reputational damage, implementing privacy-preserving AI is not just a good practice; it’s a necessity.
In summary, privacy-preserving AI models are revolutionizing how organizations handle sensitive information. They provide a robust framework for leveraging AI's capabilities while respecting individuals' privacy rights. As we move forward, the integration of these models will be crucial in creating a safe and secure digital landscape.
- What are privacy-preserving AI models?
Privacy-preserving AI models are designed to analyze data while ensuring that sensitive information remains protected, often using techniques like federated learning and homomorphic encryption. - Why are these models important?
They are essential for maintaining data privacy, complying with regulations, and protecting organizations from data breaches. - How does federated learning work?
Federated learning allows AI algorithms to learn from data stored on local devices without transferring the raw data to a central server. - What is homomorphic encryption?
Homomorphic encryption enables computations to be performed on encrypted data, allowing for data analysis without exposing sensitive information.
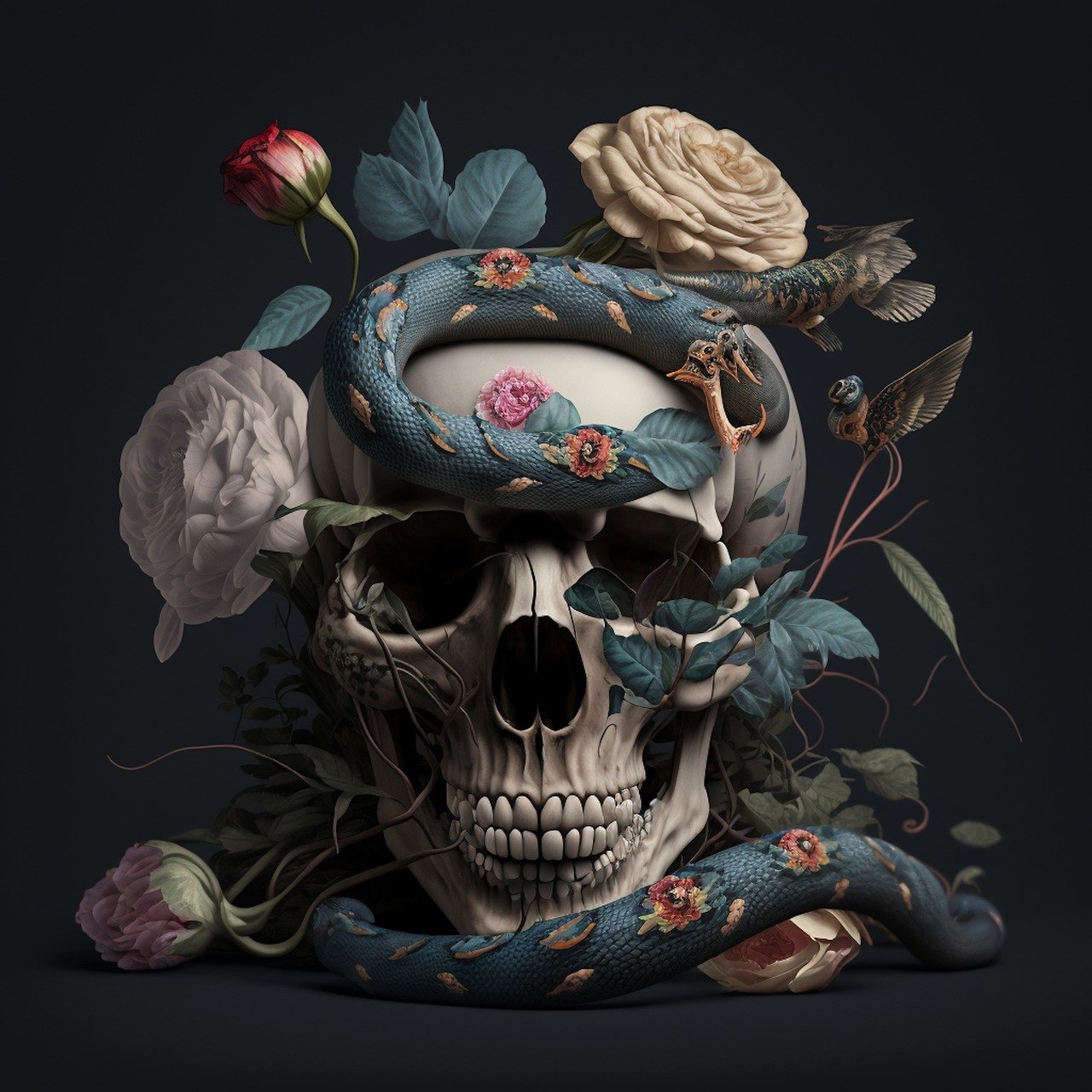
AI in Phishing Prevention
In today’s digital landscape, phishing attacks have become a prevalent threat, often masquerading as legitimate communications to trick unsuspecting users. The rise of artificial intelligence has ushered in a new era of cybersecurity, particularly in the realm of phishing prevention. With AI technologies, organizations are now equipped to combat these deceptive tactics more effectively than ever before. Imagine having a digital sentinel that not only identifies threats but also learns and adapts to new phishing strategies—this is the promise of AI in the fight against cybercrime.
AI technologies are increasingly being utilized to combat phishing attacks by identifying and blocking malicious emails before they reach end-users. These systems employ advanced algorithms that analyze patterns in email metadata, content, and sender behavior to determine the legitimacy of incoming messages. For instance, they can scrutinize the language used in an email, looking for telltale signs of phishing attempts, such as urgent requests for personal information or suspicious links. This level of scrutiny is akin to having a highly trained guard at the entrance of a secure facility, only allowing trusted individuals through the gate.
One of the most significant advancements in this area is the development of intelligent email filtering systems. These systems utilize machine learning to continuously improve their detection capabilities. As they process more data, they learn to distinguish between legitimate communications and potential phishing attempts with remarkable accuracy. The result is a robust defense mechanism that not only filters out harmful emails but also reduces the burden on IT departments by decreasing the number of false positives. This means that employees can focus on their work without the constant interruption of worrying about phishing threats.
Moreover, AI-driven platforms are also being developed to educate users about phishing tactics. These platforms raise awareness and equip individuals with the knowledge to recognize and report suspicious activities. For example, they may simulate phishing attacks in a controlled environment, allowing users to practice identifying threats without the risk of actual harm. This proactive approach is akin to fire drills in a building; by preparing individuals for potential threats, organizations can foster a culture of vigilance and readiness.
To further illustrate the impact of AI in phishing prevention, consider the following table that highlights key features of AI-driven phishing prevention systems:
Feature | Description |
---|---|
Real-time Analysis | AI systems analyze emails as they arrive, providing immediate threat assessment. |
Adaptive Learning | Machine learning algorithms adapt to new phishing tactics over time. |
User Education | Interactive training modules teach users how to identify phishing attempts. |
Threat Intelligence | Integration with global threat intelligence feeds for up-to-date information. |
In conclusion, the integration of AI in phishing prevention is not just a technological advancement; it's a necessary evolution in the fight against cyber threats. By leveraging AI’s capabilities, organizations can create a formidable defense against phishing attacks, protecting sensitive data and maintaining the trust of their users. As cybercriminals continue to refine their tactics, the adaptation and innovation offered by AI will be critical in staying one step ahead of potential threats.
- What is phishing? Phishing is a cyber attack where attackers impersonate legitimate entities to steal sensitive information from individuals.
- How does AI help in preventing phishing? AI analyzes email patterns and content to identify potential phishing attempts and block them before reaching users.
- Can AI completely eliminate phishing threats? While AI significantly reduces the risk of phishing, it cannot guarantee complete elimination due to the constantly evolving nature of cyber threats.
- Is user education still necessary with AI systems in place? Yes, user education is crucial as it empowers individuals to recognize and respond to phishing attempts effectively.
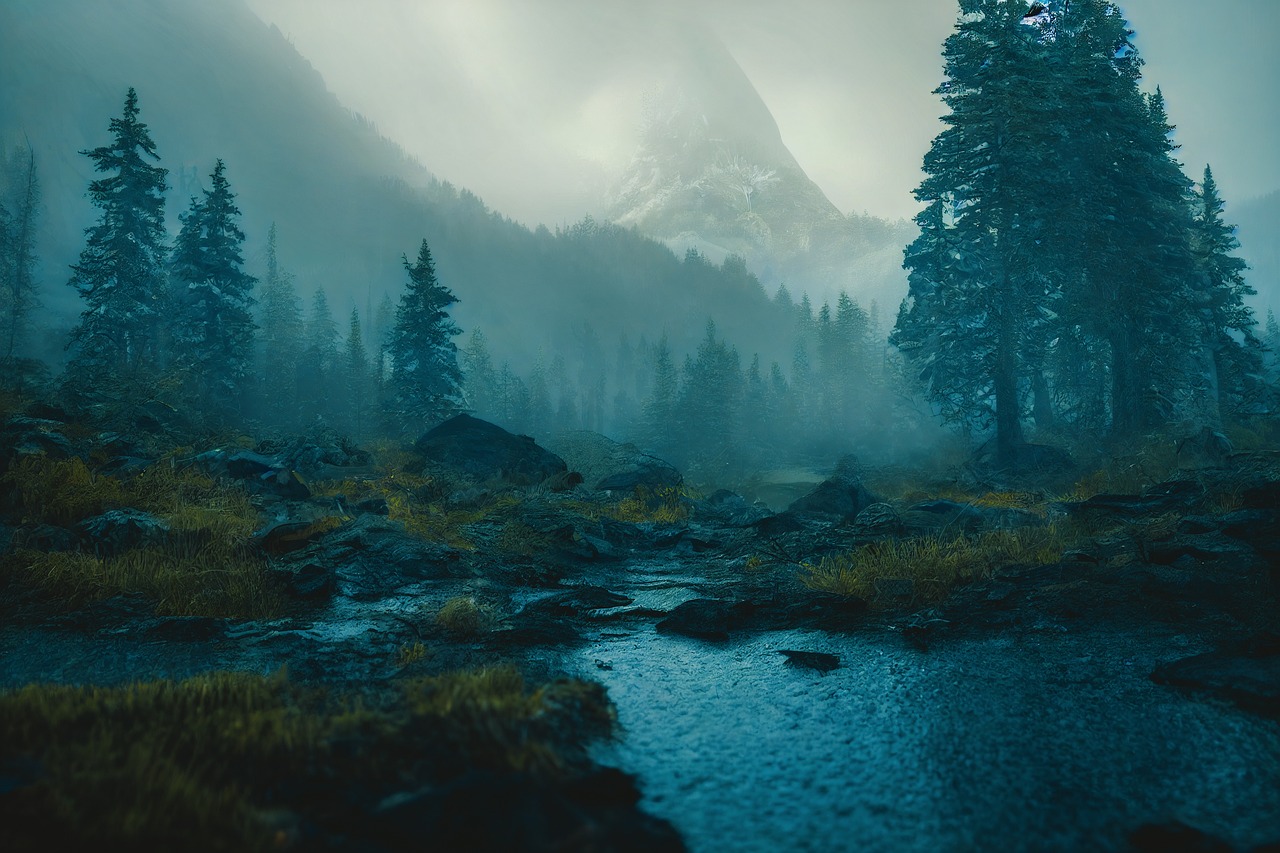
Intelligent Email Filtering
In today's digital landscape, where cyber threats lurk around every corner, has emerged as a critical line of defense against phishing attacks. Imagine your inbox as a bustling marketplace, where legitimate vendors try to sell their goods, but there are also con artists lurking, ready to pounce on unsuspecting shoppers. Just like a savvy shopper who can spot a fake product from a distance, intelligent email filtering systems are designed to sift through the noise and identify potentially harmful emails before they reach your inbox.
At the heart of these filtering systems lies artificial intelligence, which employs sophisticated algorithms to analyze incoming messages. These algorithms assess various factors such as the sender's reputation, the content of the email, and even the structure of the message. Think of it as a digital bouncer at a club, checking IDs and ensuring that only the right people get in. The result? A significant reduction in the number of phishing emails that manage to slip through the cracks.
One of the most impressive aspects of intelligent email filtering is its ability to learn and adapt over time. As more data is fed into the system, it becomes increasingly proficient at distinguishing between legitimate communications and malicious attempts. This is akin to a seasoned detective who, after years of experience, can spot a fraudulent scheme from a mile away. The more the system encounters different phishing tactics, the better it becomes at identifying them, which is a game changer for organizations trying to protect sensitive information.
Moreover, intelligent email filtering doesn't just stop at identifying threats; it also plays a crucial role in educating users. Many systems are now integrated with user-friendly dashboards that provide insights into the types of threats being blocked. This transparency empowers users to understand the risks associated with phishing and equips them with the knowledge to recognize suspicious emails in the future. It's like having a personal cybersecurity coach who not only protects you but also teaches you how to defend yourself.
To illustrate how intelligent email filtering works, consider the following table that outlines the key features and benefits of these systems:
Feature | Benefit |
---|---|
Real-time Analysis | Identifies threats as they arrive, minimizing response time. |
Machine Learning | Continuously improves detection accuracy based on new data. |
User Education | Empowers users with knowledge to spot phishing attempts. |
Customizable Filters | Allows organizations to tailor filtering criteria to their specific needs. |
In conclusion, as phishing attacks become increasingly sophisticated, the role of intelligent email filtering cannot be overstated. By leveraging the power of AI, organizations can not only shield themselves from cyber threats but also cultivate a culture of awareness among their users. After all, in the fight against cybercrime, knowledge is just as powerful as technology.
- What is intelligent email filtering?
Intelligent email filtering uses AI algorithms to analyze incoming emails, identifying and blocking phishing attempts before they reach the user's inbox. - How does it improve over time?
The system learns from new data and user interactions, continuously enhancing its ability to detect and block malicious emails. - Can users customize the filtering criteria?
Yes, many intelligent email filtering systems allow organizations to tailor the filtering settings to fit their specific security needs. - What are the benefits of using intelligent email filtering?
Benefits include real-time threat detection, improved accuracy through machine learning, user education, and customizable features.
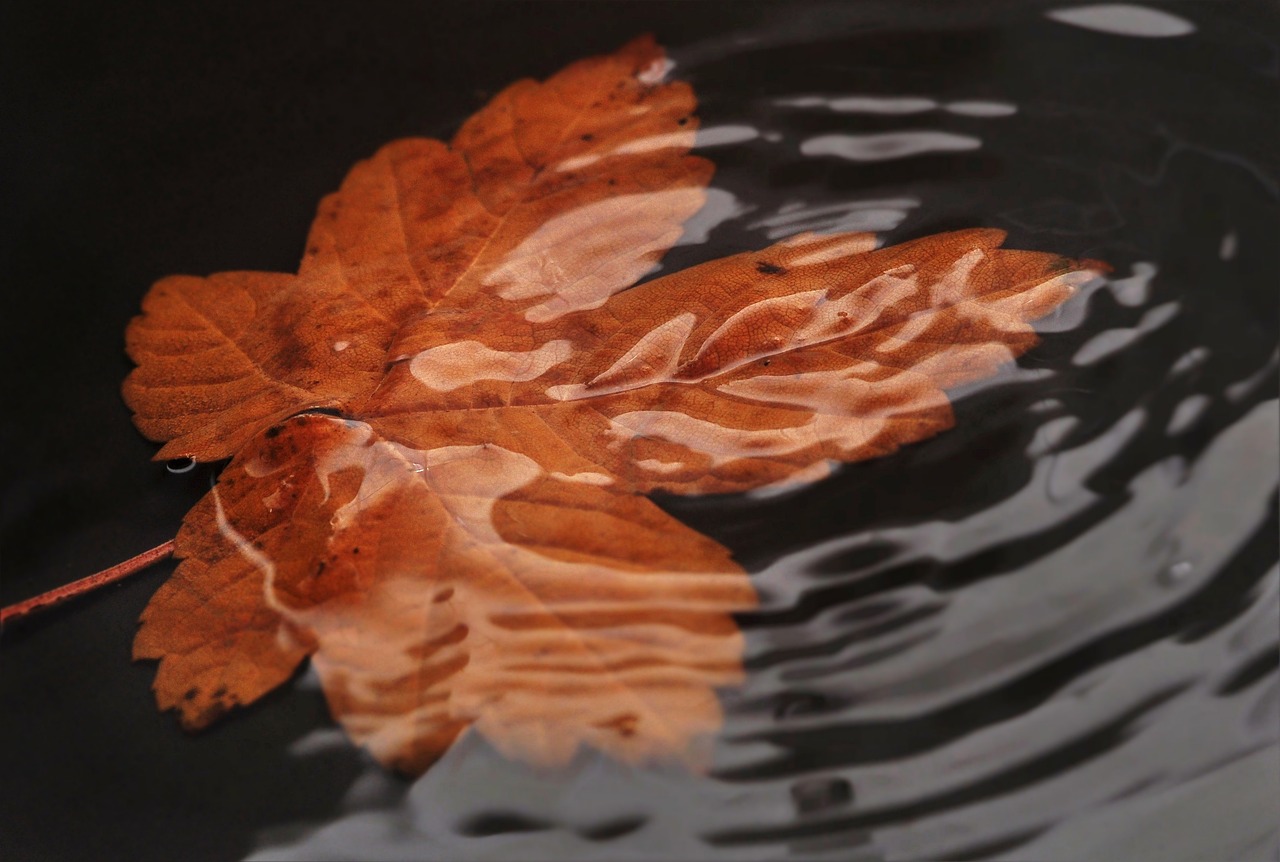
User Education and Awareness
User education and awareness are crucial components in the fight against cyber threats, particularly phishing attacks. As cybercriminals become more sophisticated, the tactics they employ to deceive users also evolve. This is where the power of artificial intelligence comes into play. AI-driven platforms are being designed not only to identify and block phishing attempts but also to educate users about the various tactics employed by cybercriminals. Imagine your email inbox as a bustling marketplace, where every message is a vendor trying to sell you something. Some are legitimate, while others are merely traps set to catch the unwary. AI acts as your personal guide through this chaotic environment, helping you discern the trustworthy vendors from the deceitful ones.
One of the significant advantages of AI in user education is its ability to tailor learning experiences. By analyzing user behavior and interaction patterns, AI can deliver personalized training sessions that address specific vulnerabilities. For example, if a user frequently clicks on suspicious links, the AI can provide targeted resources and training modules to raise awareness about the dangers of phishing. This personalized approach not only enhances learning but also fosters a more proactive mindset among users. Instead of waiting for the next phishing attempt to occur, users are empowered to recognize and report suspicious activities before they escalate.
Moreover, AI-driven educational platforms can simulate phishing attacks in a safe environment. These simulations allow users to practice identifying phishing attempts without the risk of actual data loss. Think of it as a fire drill, where employees learn how to respond to emergencies without facing real danger. This hands-on experience is invaluable, as it prepares users to react appropriately when faced with genuine threats. The more familiar they become with recognizing the signs of phishing, the more confident they will be in their ability to protect themselves and their organizations.
To further enhance user awareness, organizations can implement ongoing training programs that evolve alongside emerging threats. Just as cybercriminals continuously adapt their strategies, user education must also be dynamic. Regular updates and refresher courses can keep employees informed about the latest phishing trends and techniques. For instance, a quarterly training session could cover recent phishing scams that have targeted similar organizations, making the information relevant and actionable.
In addition to formal training sessions, organizations can leverage AI to create a culture of security awareness. This can be achieved through various communication channels, such as newsletters, intranet posts, or even gamified learning experiences. By fostering an environment where security is a shared responsibility, organizations can significantly reduce the likelihood of successful phishing attacks. After all, a well-informed user base acts as the first line of defense against cyber threats.
In conclusion, user education and awareness, bolstered by AI technologies, are essential in the ongoing battle against phishing attacks. By providing tailored training, simulating real-world scenarios, and fostering a culture of security, organizations can empower their employees to become vigilant defenders against cyber threats. The more knowledgeable users are about the tactics employed by cybercriminals, the less likely they are to fall victim to their schemes. It’s not just about implementing technology; it’s about creating a well-informed community that stands strong against potential threats.
- What is the role of AI in user education? AI enhances user education by providing personalized training experiences and simulating phishing attempts to improve recognition skills.
- How can organizations implement ongoing training? Organizations can conduct regular training sessions and updates to keep employees informed about the latest phishing tactics and trends.
- Why is user awareness important in cybersecurity? User awareness is crucial because it empowers individuals to recognize and report suspicious activities, acting as the first line of defense against cyber threats.
Frequently Asked Questions
- What are the main benefits of using AI in cybersecurity?
AI enhances cybersecurity by providing real-time threat detection, automating incident response, and improving data privacy measures. It can analyze vast amounts of data quickly, allowing organizations to identify potential threats before they escalate into serious breaches.
- How does AI-driven threat detection work?
AI-driven threat detection utilizes machine learning algorithms to analyze patterns in data traffic. By continuously learning from new data, these systems can identify anomalies and potential security breaches, enabling organizations to respond swiftly and effectively.
- What role does predictive analytics play in cybersecurity?
Predictive analytics uses historical data to forecast future cyber threats. By analyzing past incidents, organizations can proactively strengthen their defenses against emerging risks, making it a crucial aspect of a robust cybersecurity strategy.
- How does AI improve data privacy?
AI improves data privacy through advanced encryption techniques and privacy-preserving models. These technologies help organizations protect sensitive information and comply with regulations, ensuring that personal data remains secure even in the event of a cyber breach.
- Can AI help prevent phishing attacks?
Absolutely! AI technologies are increasingly used to combat phishing by analyzing incoming emails and identifying potential threats. Intelligent email filtering systems can block malicious messages before they reach users, significantly reducing the risk of data breaches.
- What is behavioral analysis in cybersecurity?
Behavioral analysis leverages AI to monitor user activities and identify any anomalies that may indicate a security breach. This additional layer of defense helps organizations detect unusual behavior patterns, allowing for quicker responses to potential threats.
- How can organizations educate users about phishing?
AI-driven platforms can be developed to provide training and resources for users, helping them recognize phishing tactics and suspicious activities. By raising awareness, organizations can empower their employees to act as the first line of defense against cyber threats.