Strengthening Trust in AI through Ethical Practices
In today's rapidly evolving technological landscape, the integration of artificial intelligence (AI) into various sectors has become a game changer. However, with great power comes great responsibility. The **importance of ethical practices** in AI cannot be overstated. As we embrace AI in our daily lives, from personal assistants to self-driving cars, we must ensure that these technologies operate in a fair, transparent, and accountable manner. This article delves into how ethical practices not only enhance trust among users and developers but also promote a sense of responsibility within society regarding the use of AI technologies.
Ethical AI is not just a buzzword; it’s a necessity for fostering trust and accountability in technology. Imagine handing over the keys to your car to a stranger – that’s how many feel about AI today. Ethical considerations in AI development are essential because they directly impact public perception and acceptance. If users believe that AI systems are biased, opaque, or unaccountable, they are less likely to trust or adopt these technologies. Therefore, establishing a solid ethical foundation is crucial for the long-term success of AI implementations.
Understanding the core ethical principles guiding AI development is vital. These principles serve as a foundation for responsible AI practices. Key principles include:
- Fairness: Ensuring that AI systems do not discriminate against any group.
- Transparency: Providing clear communication about AI processes and decision-making.
- Accountability: Establishing roles for developers and organizations in responsible AI usage.
These principles are not just theoretical; they have practical implications that shape the way AI technologies are developed and deployed in our society.
Fairness is a cornerstone of ethical AI. It ensures that AI systems do not discriminate against any group based on race, gender, or socioeconomic status. This can be integrated into AI algorithms by using diverse data sets that represent various demographics. Think of it like baking a cake: if you only use one ingredient, the result will be bland and unappealing. Similarly, diverse data enriches AI models, making them more robust and equitable.
Addressing biases in AI is essential for ethical practices. Various strategies can be employed to identify and mitigate bias in AI systems:
- Regular Audits: Conducting audits of AI systems to identify potential biases.
- Diverse Teams: Involving diverse teams in the development process to bring different perspectives.
- Feedback Mechanisms: Implementing systems for users to report biases and issues.
By actively working to mitigate bias, we can promote equitable outcomes for all users, ensuring that technology serves everyone fairly.
Diverse data sets play a critical role in reducing bias. Incorporating varied data sources can enhance the fairness and accuracy of AI models. For instance, if an AI model is trained predominantly on data from one demographic, it may fail to perform well for others. Just as a painter needs a palette of colors to create a masterpiece, AI models require a rich variety of data to function effectively across different populations.
Transparency is key to building trust in AI technologies. Users need to understand how AI systems work and how decisions are made. This means providing clear communication about the algorithms, data sources, and decision-making processes involved. When users feel informed, they are more likely to trust the technology. Imagine a recipe without the ingredients listed – it’s hard to trust the outcome! Similarly, transparency in AI fosters user confidence and promotes responsible usage.
Establishing accountability in AI development is essential for ethical practices. Developers and organizations must take responsibility for the AI systems they create. This includes being accountable for the outcomes of these systems and addressing any potential harms that arise. Think of it like a ship captain navigating through stormy seas – they must steer the ship carefully and take responsibility for the safety of their crew and passengers.
Regulatory frameworks provide guidelines for ethical AI practices. Existing regulations must be evaluated and updated to ensure they keep pace with the rapid advancements in AI technology. Comprehensive policies are crucial for governing AI development and deployment. Just as traffic laws help keep our roads safe, regulations can help ensure that AI operates ethically and responsibly.
Engaging stakeholders is crucial for ethical AI practices. Collaboration between developers, users, and policymakers is vital in shaping responsible AI technologies. By involving various stakeholders in the conversation, we can create a more inclusive and effective approach to AI ethics. It’s like building a community garden – when everyone pitches in, the result is more fruitful and beneficial for all.
Q: Why is ethical AI important?
A: Ethical AI is essential for building trust and ensuring that AI technologies are used responsibly and fairly.
Q: What are the key principles of ethical AI?
A: The key principles include fairness, transparency, and accountability.
Q: How can we mitigate bias in AI?
A: Strategies include regular audits, diverse teams, and feedback mechanisms.
Q: What role does diverse data play in AI?
A: Diverse data helps reduce bias and improves the accuracy and fairness of AI models.
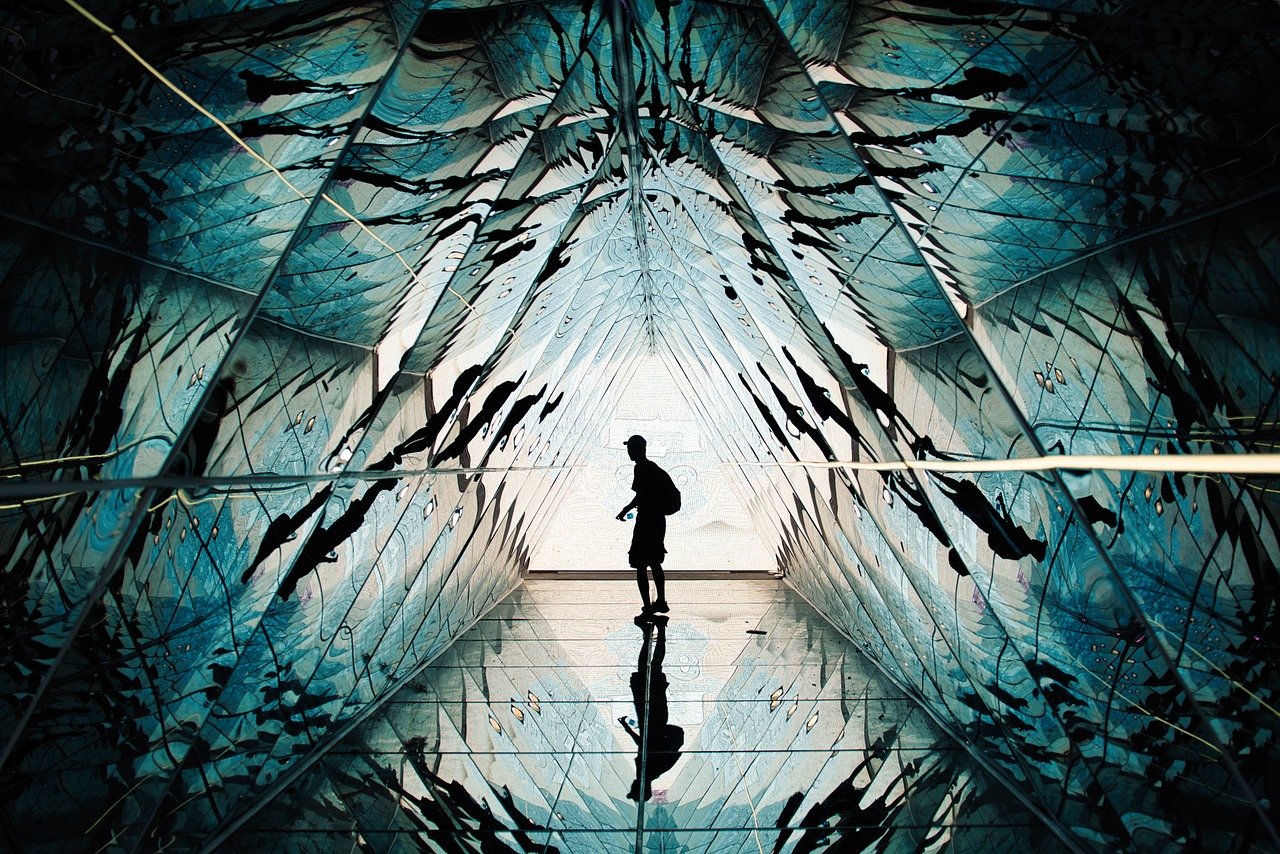
The Importance of Ethical AI
In today's rapidly evolving technological landscape, the significance of ethical AI cannot be overstated. As artificial intelligence continues to permeate various aspects of our lives, from healthcare to finance, the need for ethical considerations in its development becomes increasingly critical. Why is this so important? Simply put, ethical AI fosters trust and accountability, two pillars that are essential for ensuring that technology serves humanity rather than undermines it.
When we talk about the importance of ethical AI, we're not just discussing compliance with regulations or avoiding negative publicity. We're diving into a realm where public perception and acceptance play vital roles. Users are more likely to embrace AI technologies when they feel confident that these systems are designed with ethical principles in mind. Imagine trying to use a new app that promises to streamline your healthcare experience, but you learn that it has a history of biased outcomes and lack of transparency. Would you trust it? Probably not!
Moreover, ethical AI isn't just a nice-to-have; it's a necessity for the long-term success of AI technologies. The integration of ethical practices can lead to enhanced innovation. When developers prioritize ethics, they are more likely to create solutions that are not only effective but also beneficial for a wider audience. This approach can help prevent potential backlash from users and regulators alike, paving the way for smoother adoption and implementation of AI systems.
Let's consider some of the key reasons why ethical AI is crucial:
- Building Trust: Ethical AI practices foster trust between users and technology, making individuals feel secure in their interactions with AI systems.
- Promoting Fairness: By prioritizing ethics, developers can create AI systems that treat all users equitably, mitigating the risk of discrimination.
- Encouraging Transparency: Clear communication about how AI systems operate can demystify technology, making it more accessible and understandable for everyone.
- Ensuring Accountability: Ethical guidelines help establish who is responsible for AI outcomes, which is essential for addressing any negative impacts that may arise.
In essence, ethical AI is about more than just doing the right thing; it's about creating a future where technology enhances human life rather than complicates it. By embedding ethical practices into the core of AI development, we can ensure that these powerful tools are wielded responsibly, ultimately leading to a more equitable and just society.
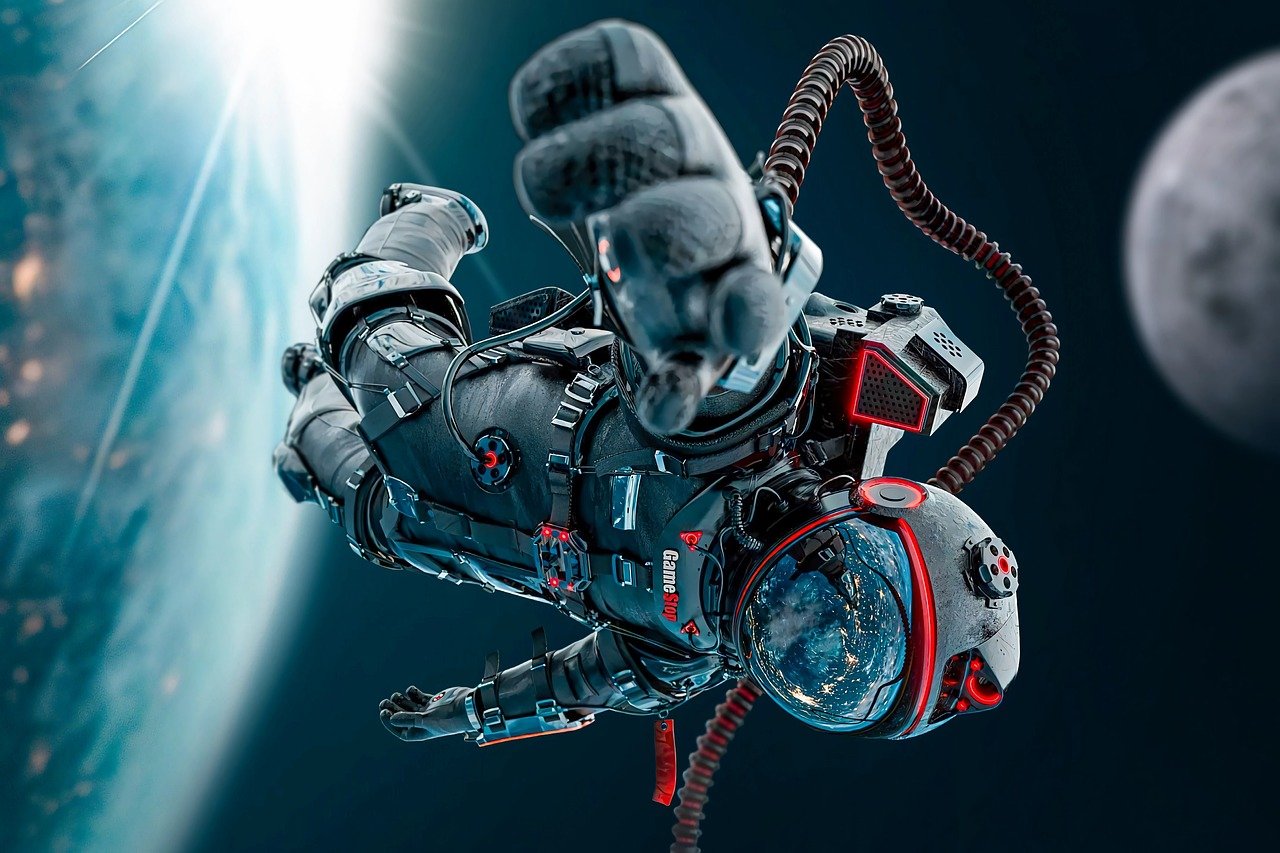
Key Ethical Principles in AI
As we dive deeper into the world of artificial intelligence, it becomes increasingly clear that the foundation of this technology is built upon a few key ethical principles. These principles not only guide the development of AI but also shape how society interacts with it. When we talk about ethical AI, we are essentially discussing the moral compass that should steer the creation and implementation of AI systems. The three primary principles that stand out are fairness, transparency, and accountability.
Fairness in AI is about ensuring that the algorithms we create do not perpetuate existing biases or create new forms of discrimination. Imagine a world where an AI system decides who gets a job or a loan based solely on biased data; that’s a nightmare scenario! To combat this, developers must actively seek to incorporate diverse data sets that reflect a wide range of experiences and backgrounds. This way, the AI can make decisions that are equitable and just for all users.
Next, we have transparency. This principle emphasizes the need for clear communication regarding how AI systems operate. Users should not feel like they are dealing with a black box. Instead, they deserve to understand the processes behind AI decision-making. For example, if an AI denies a loan application, the applicant should know the criteria used in that decision. By promoting transparency, we build trust and confidence in AI technologies, allowing users to feel more secure in their interactions with them.
Finally, we arrive at accountability. This principle is crucial as it addresses who is responsible when AI systems fail or cause harm. It’s essential for developers and organizations to establish clear lines of accountability. If an AI system makes a mistake, there should be mechanisms in place to rectify that error and to hold the responsible parties accountable. This not only helps in mitigating harm but also ensures that users feel protected and valued.
In summary, the ethical principles of fairness, transparency, and accountability serve as a guiding light for AI development. They help to ensure that AI technologies are created and used in ways that are beneficial to society. As we continue to innovate in this field, keeping these principles at the forefront will be vital in fostering trust and acceptance among users and developers alike.
- What is ethical AI? Ethical AI refers to the development and implementation of artificial intelligence systems that adhere to principles of fairness, transparency, and accountability.
- Why is fairness important in AI? Fairness is crucial to ensure that AI systems do not discriminate against any group, promoting equitable outcomes for all users.
- How does transparency build trust in AI? Transparency allows users to understand how AI systems make decisions, which fosters confidence and trust in the technology.
- Who is responsible for accountability in AI? Developers and organizations are responsible for establishing accountability in AI, ensuring that there are mechanisms to address failures or harms caused by AI systems.
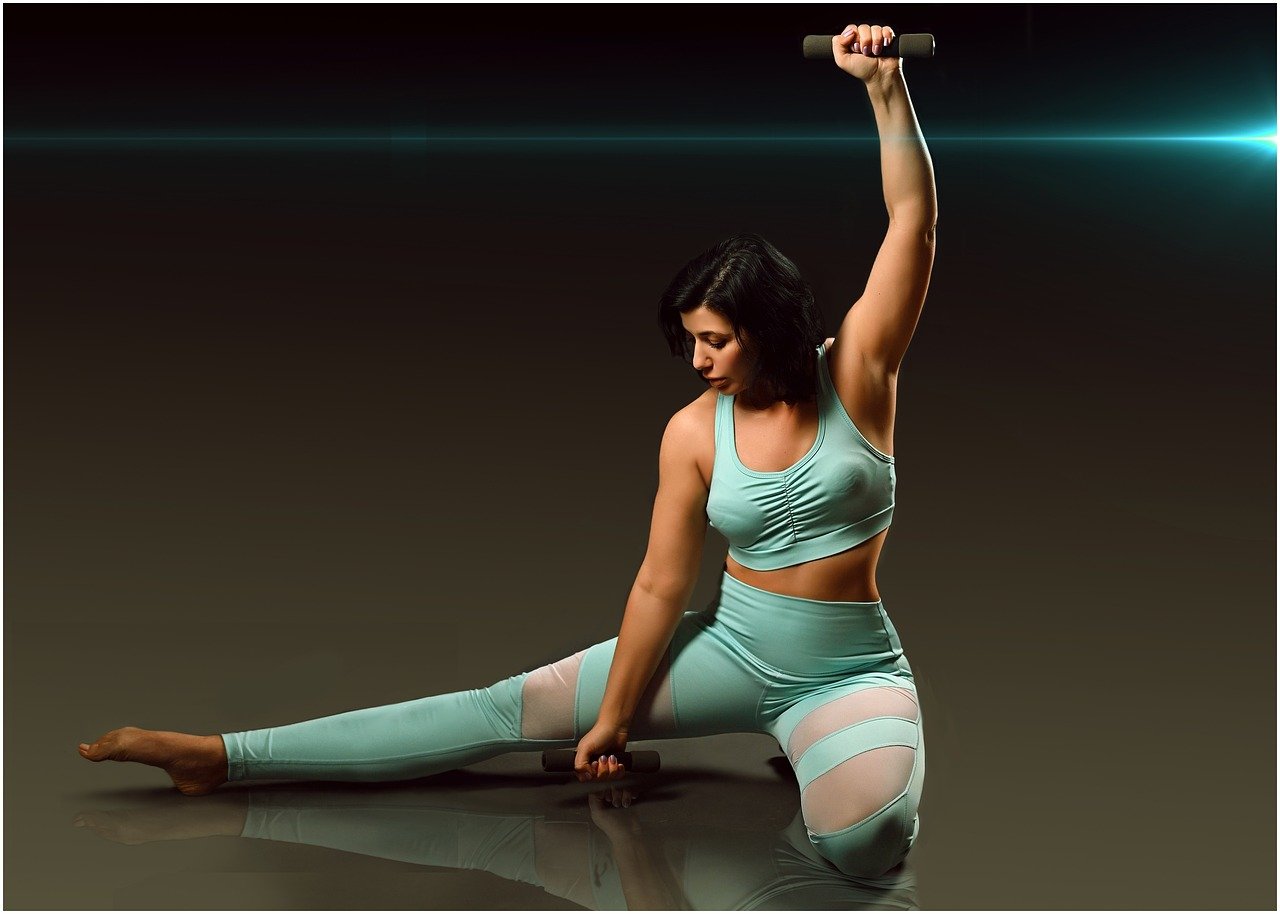
Fairness in AI Systems
In the rapidly evolving landscape of artificial intelligence, fairness has emerged as a cornerstone principle that cannot be overlooked. Imagine a world where AI systems are designed to treat everyone equally, regardless of their background. This is not just a lofty ideal but a necessary reality we must strive for. Fairness in AI systems ensures that algorithms do not discriminate against any individual or group, effectively promoting social equity. When we talk about fairness, it's essential to understand that it goes beyond mere technical adjustments; it embodies a commitment to ethical practices that resonate with the values of our society.
Integrating fairness into AI algorithms is not just a challenge; it's an opportunity to create a more just world. One way to achieve this is by utilizing diverse data sets. Think of it as cooking a delicious meal: the more varied and high-quality ingredients you use, the better the outcome. Similarly, when AI systems are trained on a rich tapestry of data that reflects different demographics, cultures, and perspectives, they become more robust and less prone to biases. This is crucial because biased algorithms can lead to unfair treatment, perpetuating existing inequalities rather than alleviating them.
To illustrate the importance of fairness, consider a scenario where a hiring algorithm is used to filter job applicants. If the algorithm is trained predominantly on data from one demographic group, it may inadvertently favor candidates from that group while disadvantaging others. This not only harms the individuals affected but also deprives organizations of diverse talent. Therefore, ensuring fairness in AI systems is not just about compliance; it's about enhancing the quality of decisions made by these systems and fostering an inclusive environment.
Furthermore, the integration of fairness in AI can be approached through several strategies, such as:
- Bias Detection: Regularly auditing algorithms for biases to ensure they operate fairly.
- Algorithmic Transparency: Making the workings of AI systems understandable to users and stakeholders.
- User Feedback: Actively seeking input from a diverse user base to identify potential biases.
By employing these strategies, developers can create AI systems that not only perform effectively but also uphold the principles of fairness and justice. In conclusion, the journey towards fair AI systems requires a concerted effort from all stakeholders involved. By embracing diverse data, actively mitigating bias, and fostering transparency, we can ensure that AI technologies contribute positively to society and enhance trust among users.
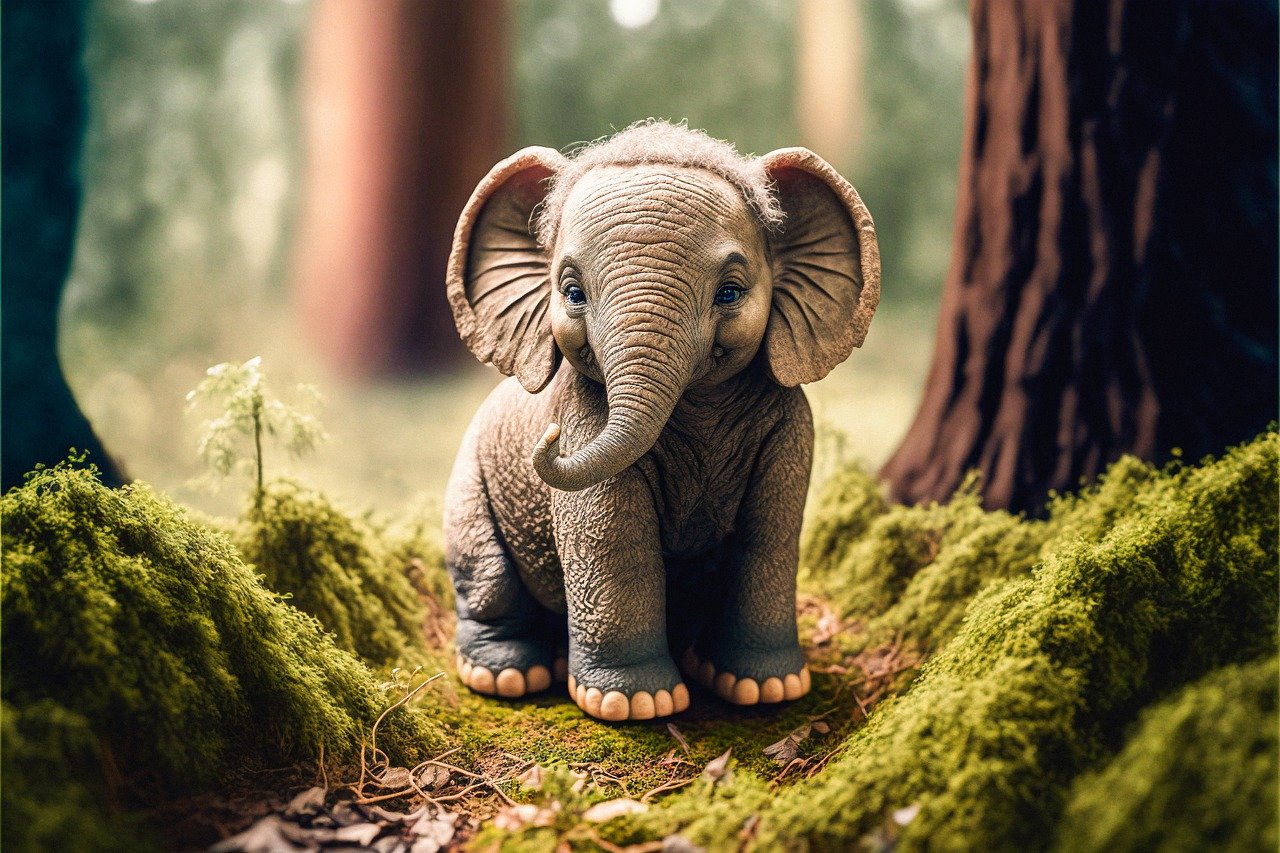
Bias Mitigation Strategies
Bias in artificial intelligence (AI) systems is a significant concern that can lead to unfair outcomes and perpetuate existing inequalities. To combat this, various have been developed. These strategies are designed to identify, reduce, and ultimately eliminate bias from AI algorithms, ensuring that all users are treated fairly and equitably. One of the first steps in this process is to conduct a thorough bias audit of the AI systems in question. This involves analyzing the data inputs and decision-making processes to uncover any hidden biases that may influence the outcomes.
Another effective strategy is to implement pre-processing techniques that modify the training data before it is fed into the AI model. This can include techniques like re-weighting the data to ensure that underrepresented groups are given more prominence or even generating synthetic data to balance the dataset. By doing this, we can create a more level playing field for all demographic groups, helping to ensure that the AI system is not skewed towards any particular group.
After the model has been trained, in-processing techniques can be utilized to adjust the model's behavior. For instance, algorithms can be designed to actively correct for biases during the decision-making process. This can involve setting fairness constraints that the algorithm must adhere to, ensuring that its predictions do not favor one group over another. Additionally, post-processing techniques can be applied after the model has made its predictions. This may include adjusting the outcomes to align with fairness criteria, thus ensuring that the final decisions made by the AI system are equitable.
Moreover, engaging with diverse stakeholders throughout the AI development process is crucial. By incorporating perspectives from various groups, developers can better understand the potential implications of their systems and identify bias that may not be immediately apparent. This collaborative approach not only enhances the fairness of AI systems but also fosters a sense of ownership and trust among users.
To summarize, bias mitigation is not a one-size-fits-all solution; it requires a multi-faceted approach that includes auditing, data manipulation, algorithm adjustments, and stakeholder engagement. By employing these strategies, we can work towards creating AI systems that are not just intelligent but also ethical and fair.
- What is bias in AI? Bias in AI refers to systematic errors in the algorithm that result in unfair outcomes for certain groups of people.
- How can bias be identified in AI systems? Bias can be identified through audits that analyze the data and decision-making processes of AI systems.
- What are some common bias mitigation strategies? Common strategies include bias audits, pre-processing, in-processing, and post-processing techniques.
- Why is stakeholder engagement important in AI? Engaging stakeholders helps to ensure that diverse perspectives are considered, which can highlight potential biases and improve the fairness of AI systems.
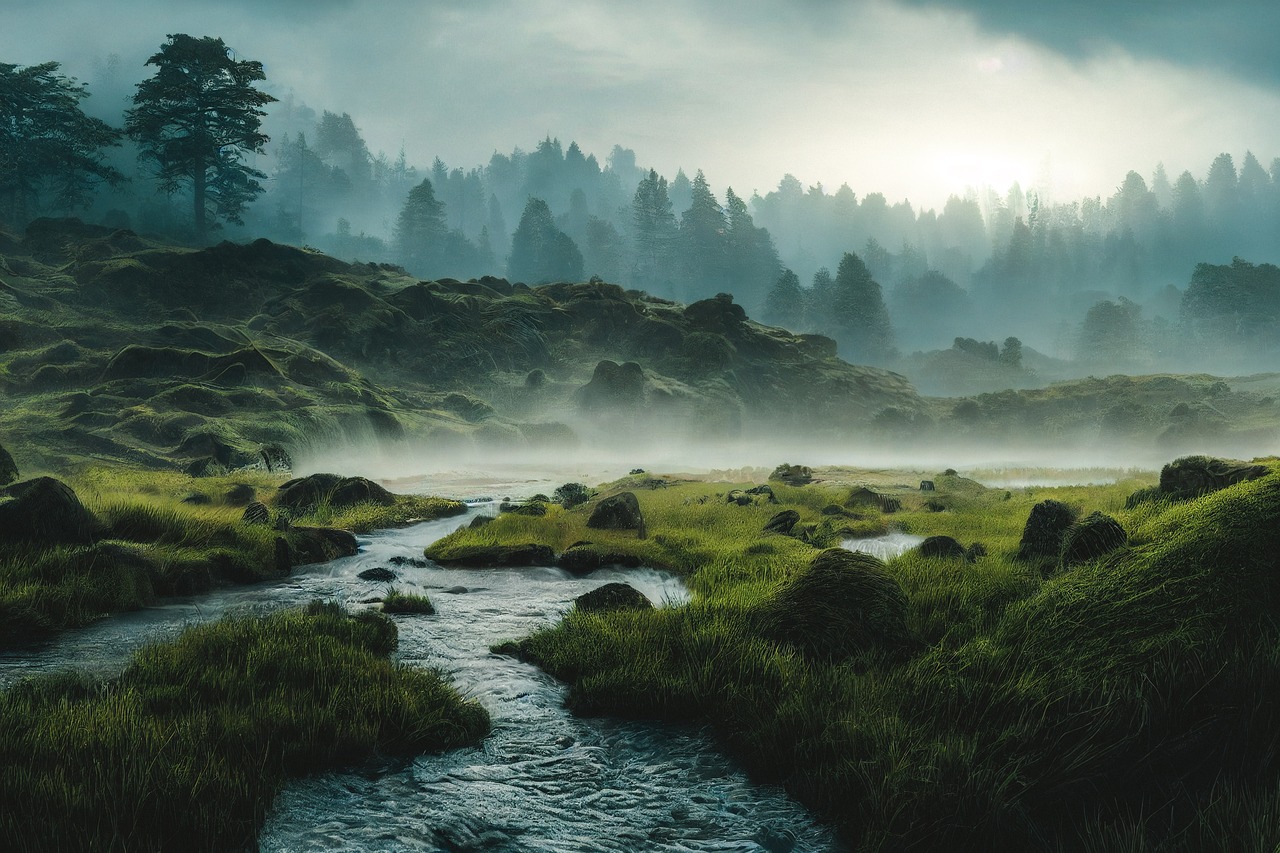
Importance of Diverse Data
When it comes to artificial intelligence, the phrase "garbage in, garbage out" rings especially true. This means that the quality and diversity of the data fed into AI systems significantly influence their performance and outcomes. Imagine trying to bake a cake with only one ingredient; it simply won’t turn out right. Similarly, AI models trained on homogeneous data sets can lead to skewed results, reinforcing existing biases and failing to represent the complexity of the real world.
Diverse data is crucial for several reasons. First and foremost, it helps to ensure that AI systems are fair. When AI is trained on a wide range of data, it can better understand and predict outcomes across different demographics, cultures, and contexts. This is particularly important in areas such as healthcare, hiring practices, and law enforcement, where biased algorithms can have serious consequences. For instance, if an AI system used for hiring is only trained on data from a specific demographic, it may unintentionally favor candidates from that group while unfairly disadvantaging others.
Moreover, diversity in data enhances the accuracy of AI models. By incorporating various data sources, AI systems can learn from a broader spectrum of information, making them more robust and reliable. This means that they can perform better in real-world applications, where scenarios can be unpredictable and varied. To illustrate, consider an AI model designed for facial recognition. If it is trained on a data set that lacks representation of certain ethnic groups, it may struggle to accurately identify individuals from those groups, leading to significant errors and potential ethical issues.
In addition to fairness and accuracy, the incorporation of diverse data also fosters innovation. When developers tap into a wide array of data sources, they can uncover new insights and trends that may not have been visible otherwise. This can lead to the creation of more innovative products and solutions that cater to a broader audience. For example, a company that uses diverse data to inform its AI-driven marketing strategies can better understand the preferences and behaviors of different customer segments, ultimately leading to more effective campaigns.
To achieve these benefits, organizations must actively seek out diverse data sources. This can include:
- Collecting data from various geographical locations
- Incorporating input from diverse demographic groups
- Utilizing data from different industries and sectors
By prioritizing diverse data, AI developers can create systems that are not only more ethical but also more effective in meeting the needs of a diverse society. As we continue to advance in the field of AI, the importance of diverse data cannot be overstated; it serves as the foundation for building trust and ensuring that technology serves all of humanity, not just a select few.
- Why is diverse data important in AI? Diverse data is essential for ensuring fairness, accuracy, and innovation in AI systems. It helps prevent biases and allows AI to better understand and represent various demographics.
- How can organizations ensure they are using diverse data? Organizations can ensure diversity by actively seeking data from varied geographical locations, demographic groups, and industries, and by continuously evaluating their data sources for inclusivity.
- What are the risks of not using diverse data in AI? Failing to use diverse data can lead to biased outcomes, inaccuracies in predictions, and a lack of representation for certain groups, which can harm individuals and society as a whole.
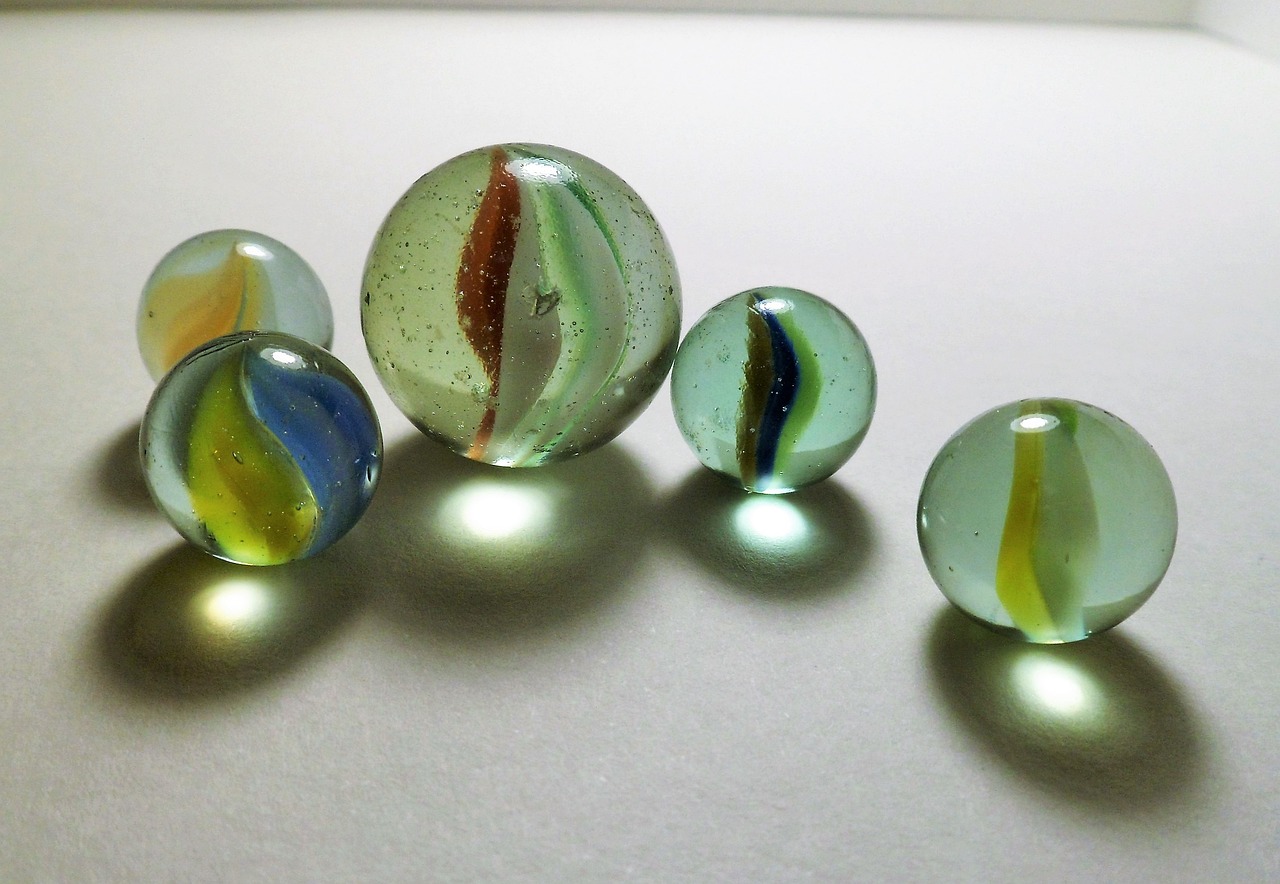
Transparency in AI Development
Transparency in AI development is not just a buzzword; it’s a fundamental aspect that can significantly influence how users perceive and trust artificial intelligence technologies. Imagine you’re using a new app that claims to be powered by AI. You’d want to know how it makes decisions, right? This need for clarity is what drives the demand for transparency in AI systems. When developers are open about their algorithms, data sources, and decision-making processes, it builds a bridge of trust between them and the users.
Moreover, transparency helps to demystify AI technologies. Many people find AI intimidating because they don’t understand how it works. By providing clear and accessible information about AI operations, developers can alleviate fears and misconceptions. For instance, if a user knows that an AI system uses a specific set of data and follows certain guidelines, they’re more likely to feel comfortable using it. Transparency transforms AI from a black box into a more understandable and approachable tool.
To achieve transparency, developers can adopt several strategies:
- Documentation: Providing comprehensive documentation that explains how AI systems function, including their limitations and potential biases.
- Open-source frameworks: Utilizing open-source platforms allows other developers and users to review, critique, and improve upon existing AI technologies.
- User feedback mechanisms: Implementing channels for users to provide feedback can help developers understand user concerns and improve their systems accordingly.
Furthermore, transparency isn't just beneficial for users; it also holds developers accountable. When AI systems are transparent, it becomes easier to identify errors or biases in their functioning. This accountability can lead to better practices and improvements in AI technologies. In essence, transparency acts as a safeguard, ensuring that developers are responsible for their creations and that they strive for ethical standards.
In summary, transparency in AI development is crucial for building trust and fostering user confidence. By making processes clearer and more understandable, developers can enhance user experience while promoting ethical practices. The more transparent the AI, the more users can engage with it without fear, paving the way for broader acceptance and responsible use of these powerful technologies.
- What does transparency in AI mean? Transparency in AI refers to the clarity and openness with which AI systems operate, including how they make decisions and the data they use.
- Why is transparency important in AI? Transparency is important because it builds trust among users, helps demystify AI technologies, and holds developers accountable for their systems.
- How can developers ensure transparency? Developers can ensure transparency by providing clear documentation, utilizing open-source frameworks, and implementing user feedback mechanisms.
- What are the benefits of transparent AI systems? The benefits include increased user trust, improved accountability, and enhanced understanding of AI functionalities, which can lead to better user experiences.
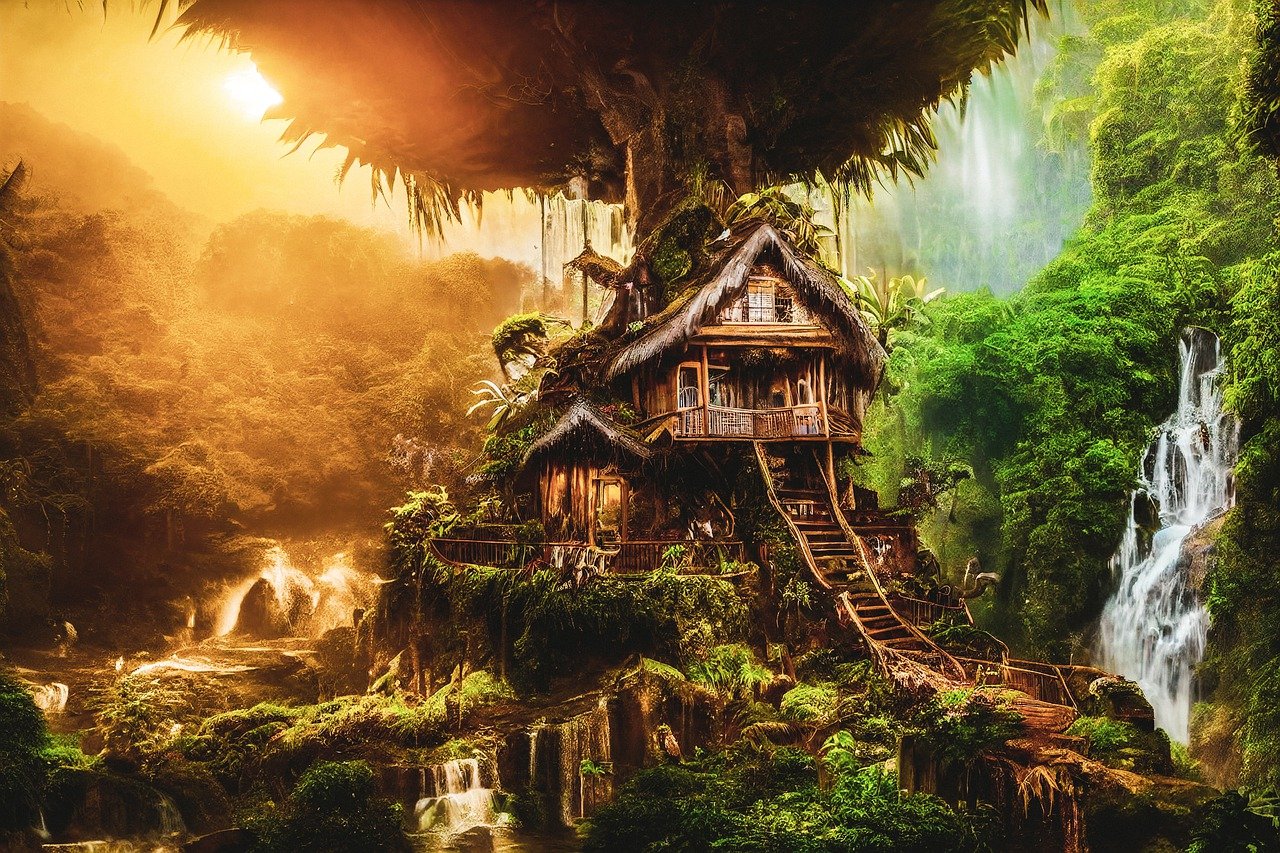
Accountability in AI Systems
In the rapidly evolving landscape of artificial intelligence, establishing accountability is not just a best practice; it’s a necessity. As AI systems become more integrated into our daily lives, the question of who is responsible for the decisions made by these systems looms larger than ever. Is it the developers, the organizations that deploy these technologies, or the AI itself? The answer is complex and multifaceted, but one thing is clear: accountability must be a cornerstone of ethical AI practices.
When we talk about accountability in AI, we’re referring to the obligation of developers and organizations to ensure that their AI systems operate within ethical boundaries and do not harm users or society at large. This involves a commitment to responsible innovation and an understanding that AI technologies can have profound impacts on individuals and communities. Developers need to ask themselves critical questions: Are we considering the potential consequences of our AI systems? Are we prepared to take responsibility for the outcomes they produce?
To foster a culture of accountability, organizations should implement clear guidelines and practices that outline the responsibilities of all stakeholders involved in AI development. This could include:
- Establishing a dedicated ethics board to oversee AI projects.
- Creating transparent processes for how AI systems are developed and deployed.
- Implementing regular audits to assess the ethical implications of AI technologies.
Moreover, regulatory frameworks play a vital role in ensuring accountability. Governments and regulatory bodies must step in to create comprehensive policies that govern the ethical use of AI. These frameworks should outline the responsibilities of developers and organizations, providing clear guidelines on how to address potential harms. For instance, regulations could mandate that AI systems undergo rigorous testing for bias and fairness before they are deployed in real-world scenarios.
Engaging stakeholders is another critical aspect of accountability in AI systems. This includes not only developers and organizations but also users, policymakers, and the communities affected by AI technologies. By fostering open dialogue among these groups, we can create a more inclusive approach to AI development that takes into account diverse perspectives and concerns. This collaboration can help identify potential risks and ensure that AI technologies are aligned with societal values.
In summary, accountability in AI systems is essential for building trust and ensuring that these technologies are used responsibly. As we move forward in this AI-driven era, it’s crucial for developers and organizations to embrace their roles as stewards of ethical AI. By doing so, they not only protect users but also contribute to a future where AI serves as a force for good in society.
- What does accountability in AI mean?
Accountability in AI refers to the obligation of developers and organizations to ensure that AI systems operate ethically and responsibly, taking responsibility for their impacts on users and society. - Why is accountability important in AI development?
Accountability is important because it fosters trust among users, ensures ethical practices, and helps mitigate potential harms caused by AI technologies. - How can organizations ensure accountability in AI?
Organizations can ensure accountability by implementing clear guidelines, engaging stakeholders, and adhering to regulatory frameworks that govern AI practices.
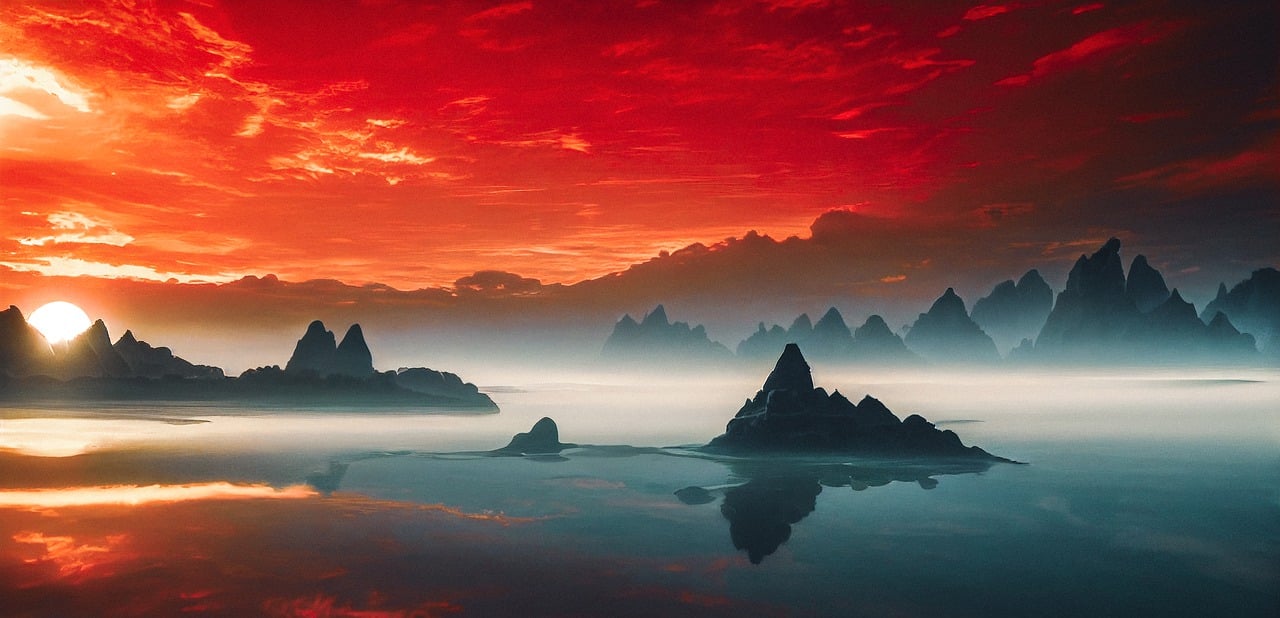
Regulatory Frameworks for AI
The rapid advancement of artificial intelligence (AI) technologies has brought forth a pressing need for regulatory frameworks that ensure ethical practices in AI development and deployment. As AI systems become increasingly integrated into our daily lives, the potential for misuse or unintended consequences grows. This is why establishing comprehensive regulations is not just beneficial; it is essential for safeguarding public interest and ensuring that AI serves humanity positively.
Regulatory frameworks provide a structured approach to managing the complexities associated with AI. They set guidelines that help developers and organizations navigate the ethical landscape, ensuring that AI technologies are not only innovative but also safe and fair. For instance, regulations can dictate how data is collected, processed, and used, which is crucial in preventing data privacy violations and ensuring compliance with laws such as the General Data Protection Regulation (GDPR).
One of the significant challenges in creating regulatory frameworks for AI is the pace at which technology evolves. Regulators often struggle to keep up with the rapid advancements, which can lead to gaps in oversight. As a result, many countries are exploring various approaches to AI regulation. Some key elements that are being considered include:
- Risk Assessment: Evaluating the potential risks associated with AI technologies before they are deployed.
- Transparency Requirements: Mandating that AI systems disclose their decision-making processes to users.
- Accountability Measures: Establishing clear lines of responsibility for developers and organizations in case of failures or harms caused by AI systems.
In addition to these elements, there is a growing consensus on the need for international cooperation in AI regulation. Since AI technologies transcend borders, a unified approach can help mitigate risks associated with fragmented regulations. For example, the European Union is actively working on the AI Act, which aims to create a comprehensive legal framework for AI across its member states. This act emphasizes the importance of human oversight and aims to classify AI systems based on their risk levels, ensuring that higher-risk applications undergo stricter scrutiny.
Moreover, stakeholder engagement is crucial in shaping effective regulatory frameworks. By involving developers, users, and policymakers in the conversation, we can create regulations that are not only practical but also reflective of societal values. This collaborative approach fosters a sense of shared responsibility and encourages innovation within ethical boundaries.
In conclusion, regulatory frameworks for AI are not merely bureaucratic hurdles; they are essential tools for fostering trust and accountability in AI technologies. As we continue to navigate the complexities of AI, it is vital that we prioritize the establishment of comprehensive, adaptable, and inclusive regulations that protect users while promoting innovation.
What are regulatory frameworks for AI?
Regulatory frameworks for AI are structured guidelines and policies designed to govern the development, deployment, and usage of artificial intelligence technologies. They aim to ensure ethical practices, protect users, and mitigate potential risks associated with AI.
Why are regulatory frameworks important?
They are important because they help establish trust in AI technologies, ensure accountability among developers, and protect users from potential harms, such as data privacy violations and biased decision-making.
How can stakeholders contribute to AI regulation?
Stakeholders, including developers, users, and policymakers, can contribute by engaging in discussions, providing feedback, and collaborating on creating regulations that reflect shared values and address real-world challenges.
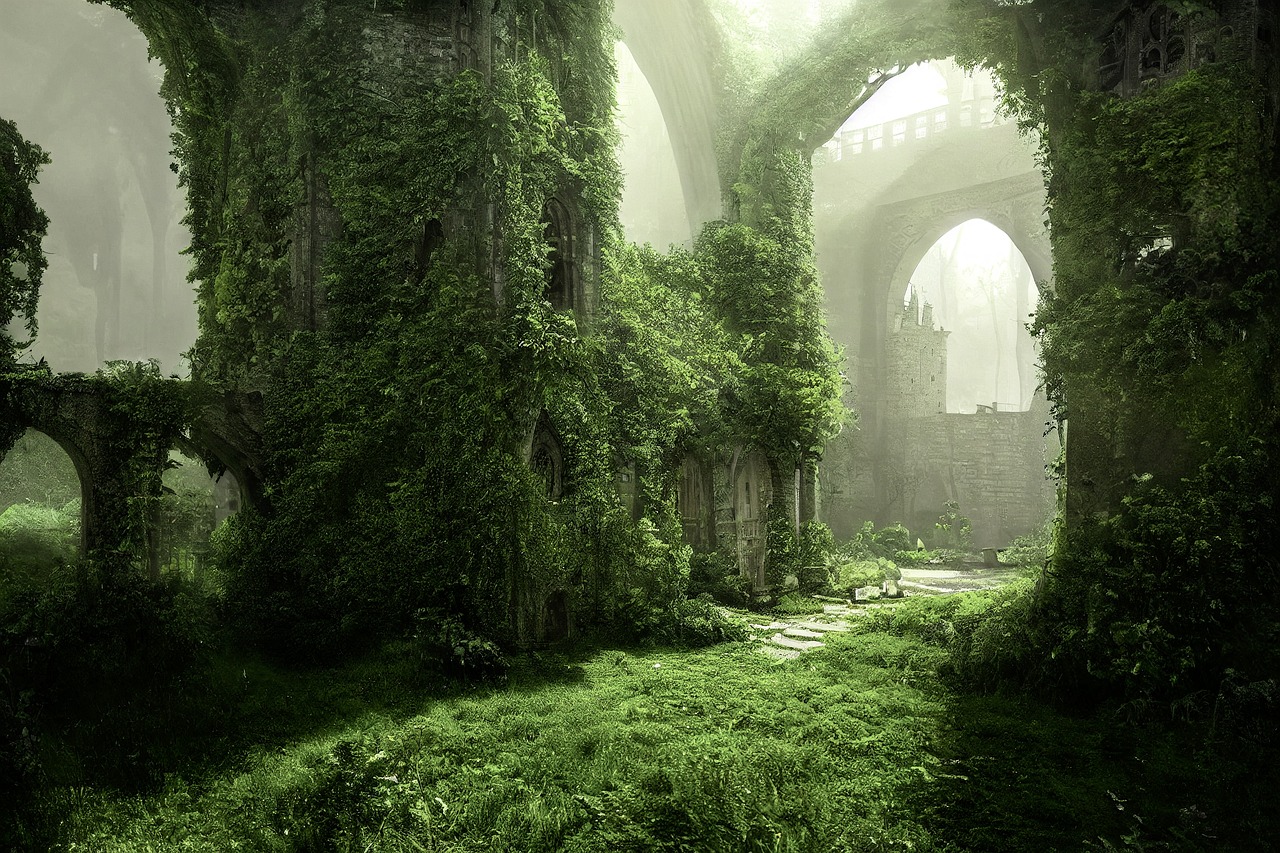
Stakeholder Engagement in AI Ethics
In the ever-evolving landscape of artificial intelligence, stakeholder engagement plays a pivotal role in shaping ethical practices. It's not just about developers crafting algorithms in isolation; rather, it's about creating a robust dialogue between various parties involved. This includes developers, users, policymakers, and even the broader community. Each of these stakeholders brings unique perspectives and insights that can significantly influence the ethical framework surrounding AI technologies.
Imagine a bustling marketplace where different voices come together to create a vibrant tapestry of ideas. This is akin to the stakeholder engagement process in AI ethics. By fostering open communication and collaboration, we can ensure that AI technologies are developed and deployed in ways that are not only innovative but also responsible and equitable.
One of the primary benefits of engaging stakeholders is the ability to identify potential ethical dilemmas early in the development process. For instance, when developers work closely with users and community representatives, they can better understand the implications of their AI systems. This collaborative approach can lead to more informed decision-making and help mitigate risks associated with bias, privacy violations, and other ethical concerns.
Moreover, stakeholder engagement can enhance public trust in AI. When users feel that their voices are heard and that they have a say in how AI technologies are shaped, they are more likely to embrace these innovations. This trust is crucial, as it lays the foundation for widespread acceptance and responsible use of AI. If stakeholders are involved in the conversation, they can contribute to the creation of guidelines and best practices that emphasize ethical considerations.
To facilitate effective stakeholder engagement, organizations can adopt several strategies:
- Inclusive Workshops: Hosting workshops that bring together diverse groups can spark meaningful discussions about ethical AI practices.
- Surveys and Feedback Mechanisms: Regularly collecting feedback from users can help developers understand their concerns and expectations.
- Partnerships with Advocacy Groups: Collaborating with organizations that focus on ethics and social justice can provide valuable insights into the societal impacts of AI.
In conclusion, stakeholder engagement is not just a checkbox in the AI development process; it is a fundamental aspect that can lead to more ethical, transparent, and accountable AI systems. By actively involving various stakeholders, we can ensure that AI technologies serve the best interests of society as a whole, paving the way for a future where innovation and ethics go hand in hand.
- What is stakeholder engagement in AI ethics?
Stakeholder engagement in AI ethics refers to the collaborative process where developers, users, policymakers, and others come together to discuss and shape the ethical implications of AI technologies. - Why is stakeholder engagement important?
It is important because it helps identify ethical dilemmas early, enhances public trust, and ensures that AI technologies are developed responsibly and equitably. - How can organizations engage stakeholders effectively?
Organizations can engage stakeholders through inclusive workshops, surveys for feedback, and partnerships with advocacy groups focused on ethics.
Frequently Asked Questions
- What is ethical AI and why is it important?
Ethical AI refers to the development and deployment of artificial intelligence technologies that prioritize fairness, transparency, and accountability. It's crucial because it helps build trust among users and society, ensuring that AI systems operate responsibly and do not perpetuate biases or discrimination.
- How can fairness be integrated into AI systems?
Fairness can be integrated into AI systems by utilizing diverse data sets and implementing algorithms designed to minimize bias. This involves actively identifying potential biases in data and ensuring that AI models are tested for equitable outcomes across different demographics.
- What are some strategies for mitigating bias in AI?
Some effective strategies for mitigating bias in AI include conducting regular audits of AI systems, employing bias detection tools, and involving diverse teams in the development process. Additionally, incorporating feedback from affected communities can help identify and address biases early on.
- Why is transparency important in AI development?
Transparency in AI development is vital because it allows users to understand how AI systems make decisions. When organizations clearly communicate their processes, it fosters user confidence and promotes accountability, ultimately leading to a more trustworthy AI landscape.
- What role do regulatory frameworks play in ethical AI?
Regulatory frameworks provide essential guidelines for ethical AI practices, helping to ensure that AI technologies are developed and deployed responsibly. They set standards for accountability, fairness, and transparency, guiding organizations in their AI initiatives and protecting users' rights.
- How can stakeholders engage in AI ethics?
Stakeholders can engage in AI ethics by collaborating on policy-making, sharing insights and experiences, and participating in discussions about ethical practices in AI. This collective effort helps shape responsible AI technologies that consider the needs and concerns of all parties involved.