The Role of Artificial Superintelligence in The Future of Security
In today's fast-paced digital world, the concept of artificial superintelligence (ASI) is no longer confined to the realm of science fiction. It is on the verge of becoming a reality, and its implications for security are profound. Imagine a world where security systems are not just reactive but proactive, where threats are anticipated and neutralized before they can cause harm. This is the promise of ASI, a technology that could transform our approach to security in ways we are just beginning to understand.
As we delve into the transformative impact of ASI on security systems, it's essential to grasp what artificial superintelligence really means. Unlike traditional artificial intelligence, which operates within the confines of human intelligence, ASI goes beyond—exceeding human cognitive abilities. This leap in intelligence could lead to systems capable of learning, adapting, and evolving in real-time, making them incredibly effective at identifying and countering threats.
But how exactly will ASI reshape the security landscape? The potential benefits are staggering. For instance, ASI can analyze vast amounts of data at lightning speed, uncovering patterns and insights that would take human analysts weeks or even months to discern. This capability can enhance cybersecurity measures significantly, enabling organizations to detect threats before they escalate into full-blown attacks. In a world where cyber threats are becoming increasingly sophisticated, ASI could serve as our first line of defense.
However, with great power comes great responsibility. The rise of artificial superintelligence also brings forth a myriad of challenges, particularly concerning privacy and ethics. As ASI systems require extensive data to function effectively, the potential for invasive surveillance becomes a pressing concern. How do we balance the need for security with the right to privacy? This is a question that society must grapple with as we move forward.
Moreover, ethical considerations are paramount. The deployment of ASI in security contexts raises moral dilemmas regarding accountability and decision-making. Who is responsible if an ASI system makes a mistake? Developers and organizations must navigate these challenges carefully to ensure that the implementation of ASI is done ethically and responsibly.
Looking ahead, the future trends in security technology are likely to be shaped significantly by the integration of artificial superintelligence. One exciting area of development is the collaboration between ASI and the Internet of Things (IoT). As our devices become increasingly interconnected, the security challenges multiply. ASI could enhance the security of these devices, ensuring that our smart homes and cities are protected from potential threats.
In conclusion, the role of artificial superintelligence in the future of security is both promising and complex. As we stand on the brink of this technological revolution, it is crucial to approach it with a blend of enthusiasm and caution. The potential for ASI to redefine security systems is immense, but it also necessitates a thoughtful examination of the ethical and privacy implications. The future of security is not just about technology; it's about the choices we make today that will shape our world tomorrow.
- What is artificial superintelligence?
Artificial superintelligence refers to a level of intelligence that surpasses human cognitive abilities, enabling systems to learn, adapt, and evolve beyond human capabilities.
- How can ASI enhance cybersecurity?
ASI can enhance cybersecurity by analyzing vast amounts of data quickly, identifying patterns indicative of threats, and enabling proactive measures to prevent attacks.
- What are the ethical concerns associated with ASI?
Ethical concerns include issues of privacy, accountability, and the moral responsibilities of developers and organizations in ensuring the responsible use of ASI technologies.
- How will ASI impact the Internet of Things?
ASI can enhance the security of IoT devices by providing advanced threat detection and response capabilities, ensuring that interconnected devices are protected against potential vulnerabilities.
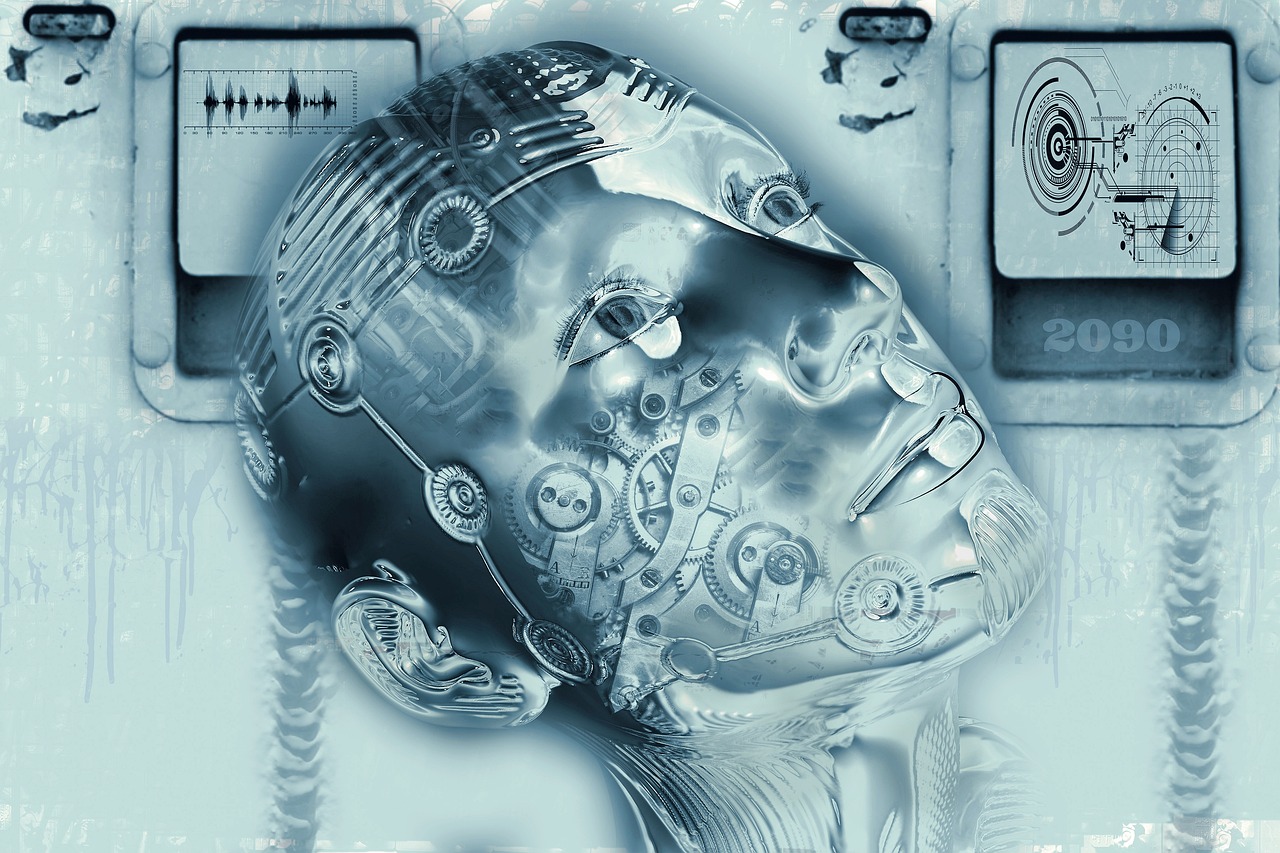
Understanding Artificial Superintelligence
Artificial superintelligence (ASI) is a term that evokes a mix of awe and trepidation. Imagine a form of intelligence that surpasses human capabilities, not just in computation but in creativity, problem-solving, and emotional understanding. This is not just science fiction; it's a potential reality that could reshape our world. ASI is characterized by its ability to learn and adapt at an exponential rate, making decisions and predictions that humans could only dream of. But what does this mean for us, particularly in the realm of security?
To truly grasp the concept of ASI, we must first understand its foundational elements. At its core, ASI is built upon advanced algorithms and vast datasets, enabling it to process information far more efficiently than any human could. Think of it as having a supercharged brain that can analyze countless variables in mere seconds. The theoretical frameworks guiding its development include concepts from machine learning, neural networks, and cognitive computing. These technologies allow ASI to not only mimic human thought but to enhance it beyond our natural limits.
One of the most exciting aspects of ASI is its potential applications in security. Imagine a world where security systems can predict threats before they even materialize, adapting to new challenges in real-time. This capability stems from ASI's ability to identify patterns and anomalies within massive datasets. For instance, if a security system can analyze user behavior across thousands of devices, it can detect unusual activities that might indicate a cyber-attack. This predictive capability is what sets ASI apart from traditional security measures, which often react to threats rather than anticipate them.
However, the journey towards fully realizing artificial superintelligence is fraught with challenges. There are significant technical hurdles to overcome, including the need for more sophisticated algorithms and computing power. Additionally, ethical considerations loom large. As ASI becomes more integrated into our security frameworks, we must grapple with questions about privacy, data security, and the potential for misuse. Will we be able to harness this power responsibly, or will it lead to invasive surveillance practices?
In conclusion, understanding artificial superintelligence is not just about grasping its technical aspects; it's about recognizing its profound implications for our future. As we stand on the brink of this technological revolution, we must remain vigilant and proactive in addressing the challenges it presents, ensuring that we leverage its capabilities for the greater good while safeguarding our fundamental rights.
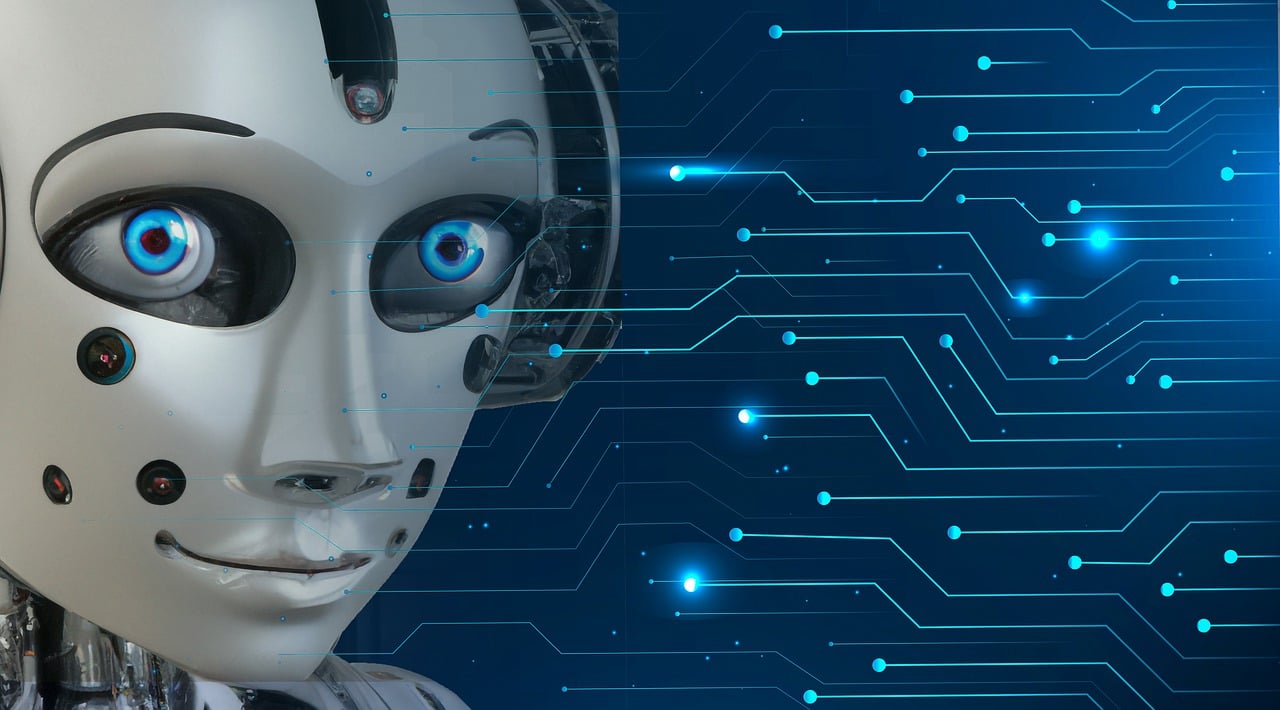
Enhancing Cybersecurity Measures
In today's digital age, where technology is evolving at a breakneck speed, the importance of robust cybersecurity measures cannot be overstated. As we venture further into an era dominated by artificial superintelligence (ASI), we stand on the brink of a transformative shift in how we approach security. Imagine a world where threats are not only detected but predicted and neutralized before they can inflict damage. This is not just a futuristic dream; it's becoming a reality thanks to ASI's advanced capabilities. By leveraging sophisticated algorithms, ASI can enhance our cybersecurity measures in ways we never thought possible.
One of the most significant advantages of ASI in cybersecurity is its ability to predict and neutralize threats faster than any traditional system. Conventional cybersecurity measures often rely on predefined rules and historical data, which can leave gaps in defense when new threats emerge. In contrast, ASI can analyze vast amounts of data in real-time, identifying patterns and anomalies that may indicate potential cyber threats. This capability allows organizations to shift from a reactive to a proactive security stance, significantly reducing the risk of breaches.
Moreover, the integration of automated threat detection systems powered by ASI can drastically improve the efficiency of security operations. These systems utilize machine learning algorithms to continuously learn from new data, adapting to evolving threats. For instance, when a new type of malware is detected, the ASI system can quickly analyze its behavior and develop countermeasures. This is akin to having a security guard who not only watches for intruders but also learns their tactics and devises strategies to thwart them effectively.
The backbone of this automated threat detection lies in machine learning algorithms. These algorithms are trained to recognize anomalies in network traffic, user behavior, and system performance. By employing techniques such as supervised learning, unsupervised learning, and reinforcement learning, ASI can identify potential threats with remarkable accuracy. For example, if an employee suddenly accesses sensitive data at an unusual hour, the system can flag this behavior for further investigation. This proactive approach not only enhances security but also minimizes the burden on human security teams, allowing them to focus on more complex issues.
Another critical aspect of ASI in cybersecurity is its ability to implement real-time response mechanisms. In a world where cyberattacks can happen in the blink of an eye, the speed of response is crucial. ASI can facilitate immediate actions, such as isolating affected systems or blocking malicious traffic, thereby mitigating potential damage. This swift response capability is akin to having a fire alarm that not only alerts you to danger but also automatically activates sprinklers to contain the fire before it spreads.
Furthermore, ASI's role in predictive analytics opens up new avenues for security measures. By analyzing historical data and current trends, ASI can provide insights that help organizations anticipate and prevent security breaches before they occur. For instance, if data shows a rising trend in phishing attacks targeting a specific industry, ASI can alert organizations in that sector to enhance their defenses. This proactive approach not only protects sensitive information but also fosters a culture of security awareness within organizations.
In conclusion, the integration of artificial superintelligence into cybersecurity measures marks a significant leap forward in our ability to combat cyber threats. By enhancing threat detection, enabling real-time responses, and leveraging predictive analytics, ASI empowers organizations to safeguard their digital assets more effectively than ever before. As we embrace this technology, it's essential to remain vigilant and consider the ethical implications of its deployment, ensuring that the benefits of enhanced security do not come at the cost of our privacy and rights.
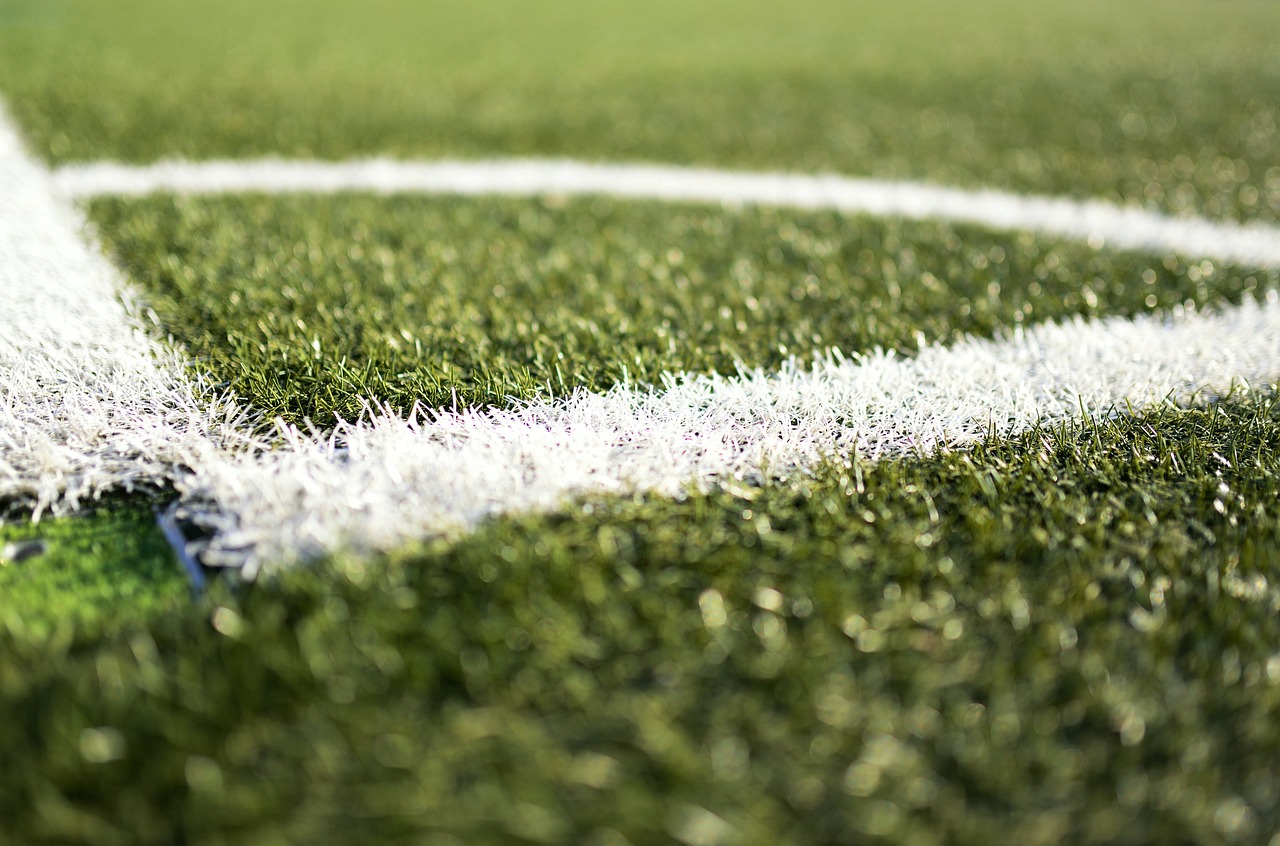
Automated Threat Detection
In today's digital landscape, the sheer volume of data generated every second is staggering. Imagine trying to sift through a mountain of information with the naked eye—it's not just tedious, it's nearly impossible! This is where comes into play, transforming the way we safeguard our digital environments. By leveraging the power of artificial superintelligence, organizations can analyze vast datasets at lightning speed, identifying potential threats that would otherwise go unnoticed.
Automated threat detection systems utilize advanced algorithms that can process and analyze data from various sources, such as network traffic, user behavior, and system logs. These systems are designed to recognize patterns that indicate suspicious activity. For instance, if an employee suddenly starts downloading an unusual amount of data at odd hours, an automated system can flag this behavior as a potential security risk. This capability is particularly crucial in a world where cyber threats are becoming increasingly sophisticated and prevalent.
The mechanisms behind automated threat detection are often rooted in machine learning and artificial intelligence. These technologies enable systems to learn from historical data, continually improving their accuracy in identifying threats. The more data these systems process, the better they become at distinguishing between normal and abnormal behavior.
To give you a clearer picture, here's a simplified overview of how automated threat detection works:
Step | Description |
---|---|
Data Collection | Gathering data from various sources, including user activities, network traffic, and system logs. |
Data Analysis | Using algorithms to analyze the collected data for patterns or anomalies. |
Threat Identification | Flagging suspicious activities that deviate from established norms. |
Response Action | Initiating predefined responses to mitigate identified threats. |
The integration of automated threat detection not only enhances the speed of threat identification but also reduces the burden on cybersecurity professionals. Instead of manually combing through logs and alerts, security teams can focus their efforts on investigating and responding to the most pressing threats. This shift allows for a more proactive approach to security, where potential breaches can be addressed before they escalate into significant incidents.
However, while automated threat detection offers incredible advantages, it's essential to recognize its limitations. No system is foolproof, and false positives can occur, leading to unnecessary alarm. Moreover, as cybercriminals continue to evolve their tactics, the algorithms must also adapt to stay ahead. Therefore, the collaboration between human expertise and artificial intelligence is vital in creating a robust security framework.
In conclusion, automated threat detection powered by artificial superintelligence is revolutionizing the cybersecurity landscape. By enabling organizations to swiftly identify and respond to potential threats, these systems not only enhance security but also empower cybersecurity professionals to focus on strategic initiatives. As we continue to navigate an increasingly complex digital world, embracing these advanced technologies will be crucial in safeguarding our assets and data.
- What is automated threat detection? Automated threat detection refers to the use of artificial intelligence and machine learning algorithms to identify and respond to potential security threats in real-time.
- How does it improve cybersecurity? By analyzing vast amounts of data quickly, automated threat detection can identify suspicious patterns and behaviors, allowing for faster response times and reduced risk of breaches.
- Can automated systems make mistakes? Yes, while automated systems are highly effective, they can generate false positives, which require human intervention to verify and address.
- Is human oversight still necessary? Absolutely! While automated systems enhance efficiency, human expertise is crucial for interpreting results and making informed decisions about security measures.
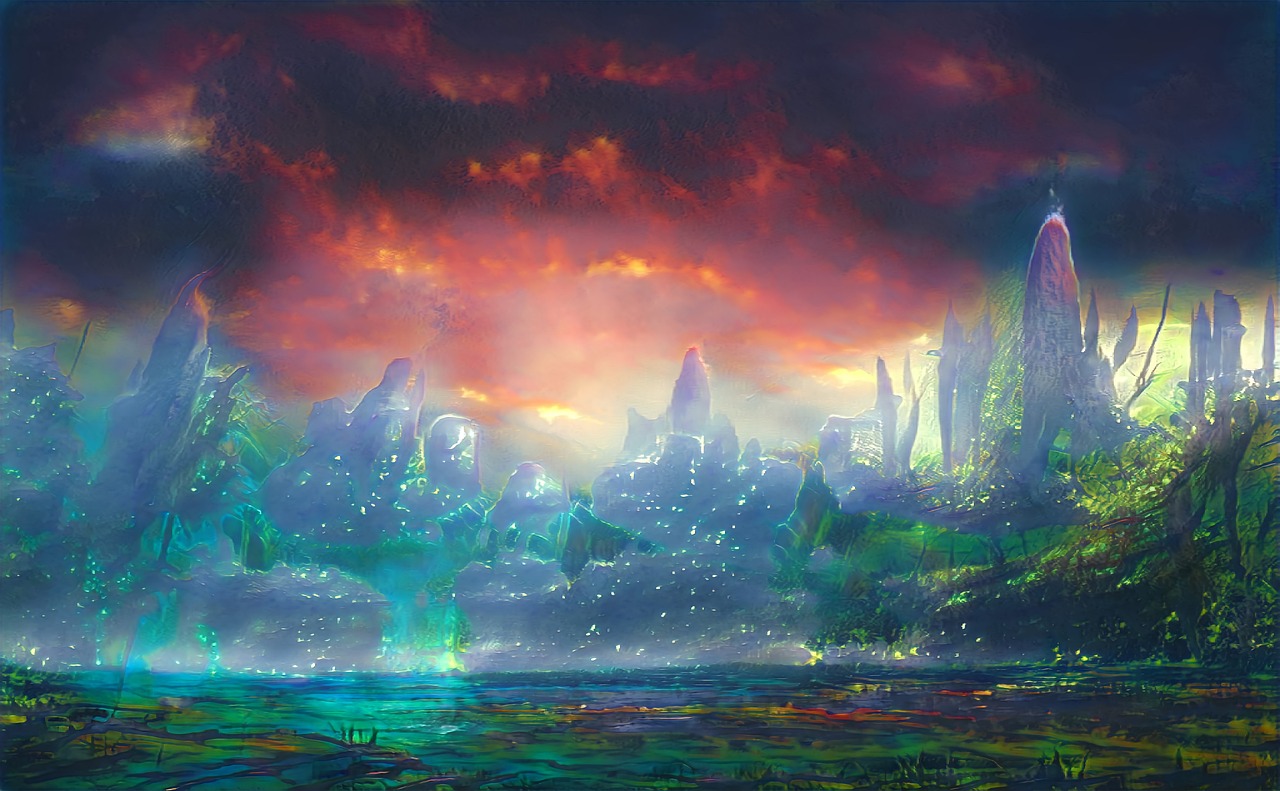
Machine Learning Algorithms
When we talk about , we’re diving into the very heart of how artificial superintelligence can reshape the cybersecurity landscape. These algorithms are like the brains behind the operation, constantly learning and evolving to recognize patterns that might indicate a potential cyber threat. Imagine having a digital detective that never sleeps, tirelessly analyzing data and adapting to new information. Sounds powerful, right?
At the core of machine learning are various techniques that allow AI systems to process massive datasets and extract meaningful insights. One of the most common methods is supervised learning, where the algorithm is trained on a labeled dataset. This means it learns from examples, much like a student studying for a test. The algorithm receives input data along with the correct output, enabling it to make predictions or classifications with increasing accuracy.
On the flip side, we have unsupervised learning, which operates without labeled data. Here, the algorithm must find hidden patterns or intrinsic structures within the data on its own. It’s akin to exploring a new city without a map; you rely on your instincts to discover interesting places. This approach is particularly useful in cybersecurity for identifying anomalies that could signify a breach, as it can uncover unusual behavior that hasn’t been explicitly flagged before.
Moreover, reinforcement learning is another fascinating technique where algorithms learn by trial and error. Picture a game where the AI receives rewards for making the right moves and penalties for the wrong ones. Over time, it learns the best strategies to maximize its score. In cybersecurity, this could translate to developing adaptive defenses that continuously improve based on their performance against various attack vectors.
To illustrate how these algorithms work in practice, consider the following table that outlines the three main types of machine learning techniques used in cybersecurity:
Type of Learning | Description | Application in Cybersecurity |
---|---|---|
Supervised Learning | Trains on labeled datasets to predict outcomes. | Phishing detection and spam filtering. |
Unsupervised Learning | Identifies patterns in unlabeled data. | Anomaly detection for unusual network activity. |
Reinforcement Learning | Learns optimal actions through rewards and penalties. | Adaptive threat response systems. |
As we continue to harness the power of machine learning algorithms, it’s essential to remember that these systems are not infallible. They require continuous training and updates to remain effective against evolving threats. Just like a seasoned detective needs to stay sharp and informed about the latest criminal techniques, AI systems must adapt to new cyber threats to maintain robust security.
Ultimately, the integration of machine learning algorithms into cybersecurity frameworks offers a promising frontier in the fight against cybercrime. By leveraging these advanced technologies, organizations can not only improve their threat detection capabilities but also enhance their overall security posture, making it harder for malicious actors to succeed.
- What is machine learning in cybersecurity? Machine learning in cybersecurity refers to the use of algorithms that can analyze data, learn from it, and make decisions to detect and respond to cyber threats.
- How does supervised learning work? Supervised learning works by training an algorithm on a labeled dataset, allowing it to learn the relationship between inputs and outputs to make predictions.
- What are the benefits of using machine learning in security? The benefits include improved threat detection, faster response times, and the ability to identify previously unknown threats through pattern recognition.
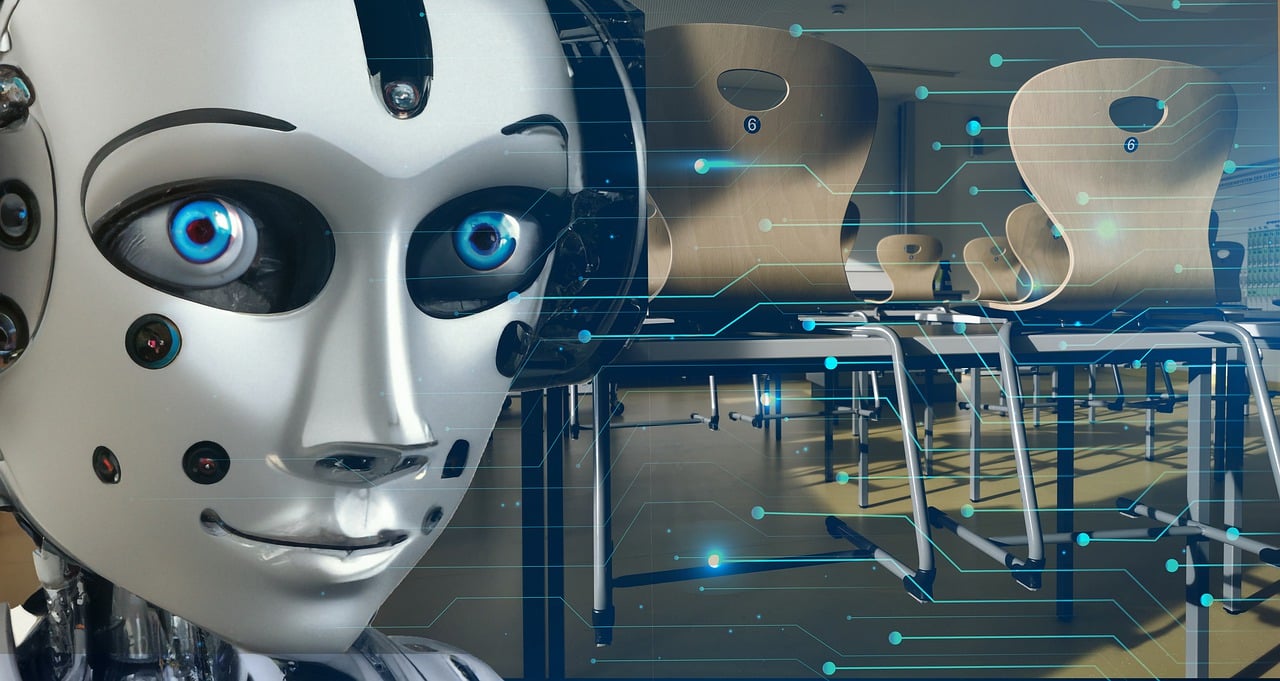
Real-Time Response Mechanisms
Imagine waking up to find that your home security system not only alerted you to a potential break-in but also locked all doors, turned on the lights, and notified the police—all in a matter of seconds. This is the power of driven by artificial superintelligence (ASI). These mechanisms are designed to react instantly to threats, minimizing damage and ensuring safety in ways that traditional systems can only dream of.
In today's fast-paced digital landscape, the speed of response is critical. Cyber threats can evolve in the blink of an eye, making it essential for security systems to not just detect threats but to respond immediately. ASI takes this a step further by analyzing data in real-time, allowing for actions that are not only swift but also informed by a wealth of contextual information. For instance, if a suspicious login attempt is detected, an ASI-enabled system can automatically lock the account, alert the user, and even initiate a password reset—all without human intervention.
To understand how these mechanisms work, let’s consider the underlying technologies:
- Automated Decision-Making: ASI can evaluate multiple factors—such as user behavior, historical data, and threat intelligence—to make decisions in real-time. This reduces the reliance on human judgment, which can often be slow and prone to error.
- Adaptive Learning: Unlike traditional systems that operate on predefined rules, ASI continuously learns from new data. This means that as threats evolve, so too does the system’s ability to respond to them.
- Seamless Integration: Real-time response mechanisms can integrate with various security tools and platforms, creating a cohesive defense strategy. This interconnectedness ensures that when one system detects a threat, others can be alerted and mobilized instantly.
Furthermore, the effectiveness of real-time response mechanisms can be illustrated by examining their impact on incident management. A study found that organizations utilizing ASI-driven responses experienced a 50% reduction in the time taken to mitigate security incidents compared to those relying on traditional methods. This not only saves time but also significantly reduces the potential financial and reputational damage that can arise from a security breach.
In conclusion, the advent of artificial superintelligence has ushered in a new era of security where real-time response mechanisms play a pivotal role. They offer a proactive approach to threat management, ensuring that organizations are not just reacting to security incidents but are a step ahead in preventing them. With the capability to analyze vast amounts of data and respond instantaneously, ASI is transforming the landscape of security, making it safer and more efficient than ever before.
Q: What is real-time response in security systems?
A: Real-time response refers to the ability of security systems to detect threats and take immediate action to mitigate them without human intervention, often powered by artificial superintelligence.
Q: How does artificial superintelligence enhance real-time response?
A: ASI enhances real-time response by analyzing data quickly and accurately, allowing systems to make informed decisions and react to threats faster than traditional methods.
Q: What are the benefits of using real-time response mechanisms?
A: The benefits include faster threat mitigation, reduced potential damage from security incidents, and a more proactive approach to security management.
Q: Can real-time response mechanisms work with existing security systems?
A: Yes, many ASI-driven real-time response mechanisms can integrate with existing security tools to create a more cohesive and effective security strategy.
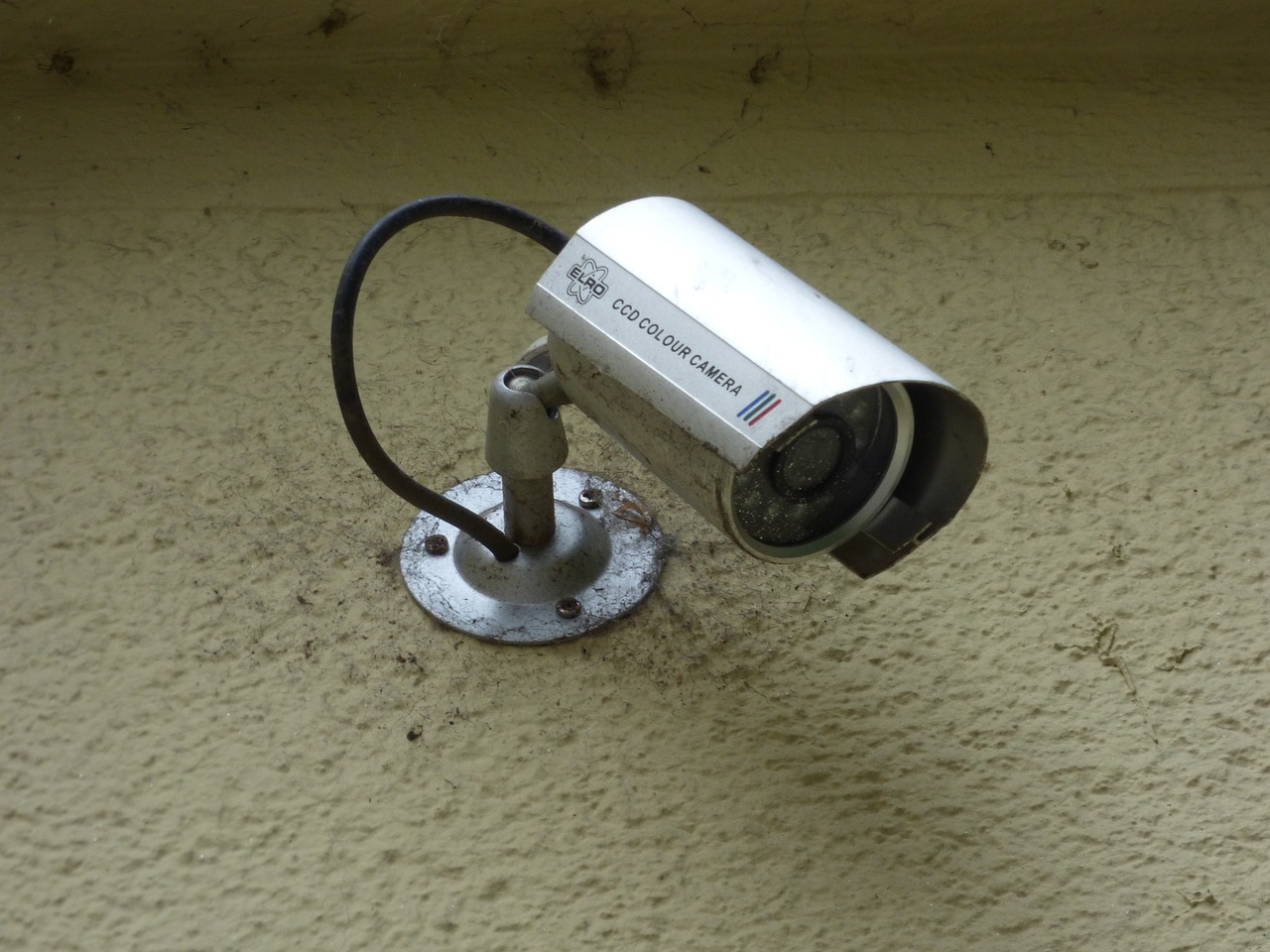
Predictive Analytics in Security
Predictive analytics is rapidly emerging as a game-changer in the realm of security, particularly when powered by artificial superintelligence. Imagine having a crystal ball that not only foresees potential threats but also provides actionable insights to mitigate them before they escalate. This is precisely what predictive analytics offers—an advanced analytical approach that utilizes historical data, statistical algorithms, and machine learning techniques to identify the likelihood of future outcomes based on past events. In the context of security, this means that organizations can stay one step ahead of cybercriminals and other threats.
At its core, predictive analytics in security involves the collection and analysis of vast amounts of data. This data can come from various sources, including user behavior patterns, network traffic logs, and even external threat intelligence feeds. By leveraging artificial superintelligence, security systems can process this data at lightning speed, identifying trends and anomalies that might indicate a looming security breach. For instance, if a user suddenly accesses sensitive data at an unusual hour or from an unfamiliar location, predictive analytics can flag this behavior as suspicious, prompting immediate investigation.
Moreover, the power of predictive analytics lies in its ability to evolve. The more data these systems analyze, the better they become at recognizing patterns. This self-improving capability is akin to how a seasoned detective becomes more adept at solving cases over time. By continuously learning from new data, predictive analytics can refine its algorithms, enhancing accuracy and reducing false positives. This not only saves time for security teams but also allows them to focus on genuine threats rather than wasting resources on benign activities.
To illustrate the impact of predictive analytics, consider the following table that outlines its key benefits in security:
Benefit | Description |
---|---|
Proactive Threat Prevention | Identifies and mitigates risks before they escalate into serious breaches. |
Improved Resource Allocation | Allows security teams to prioritize their efforts on high-risk areas based on data insights. |
Enhanced Incident Response | Facilitates quicker decision-making and response times during security incidents. |
Data-Driven Strategies | Empowers organizations to develop informed security policies based on empirical evidence. |
In addition to these benefits, predictive analytics also plays a crucial role in fostering a culture of security awareness within organizations. By sharing insights derived from predictive models, organizations can educate employees about potential threats and encourage them to adopt safer practices. This collective vigilance is paramount in today’s digital landscape, where human error often serves as the weakest link in security chains.
However, as we embrace the advantages of predictive analytics, it’s essential to remain aware of the challenges it presents. The reliance on data can lead to privacy concerns, particularly when sensitive personal information is involved. Organizations must strike a balance between leveraging data for security and respecting individual privacy rights. Clear policies and transparent data usage practices are vital to building trust with users.
In conclusion, predictive analytics, especially when enhanced by artificial superintelligence, is revolutionizing the security landscape. It empowers organizations to anticipate threats, streamline their response strategies, and cultivate a culture of awareness. As we move forward, the integration of these advanced analytical techniques will undoubtedly shape the future of security, making it more resilient and proactive than ever before.
- What is predictive analytics in security?
Predictive analytics in security refers to the use of historical data and machine learning algorithms to forecast potential security threats and vulnerabilities.
- How does artificial superintelligence enhance predictive analytics?
Artificial superintelligence can analyze vast datasets at unprecedented speeds, improving the accuracy and effectiveness of predictive analytics in identifying threats.
- What are the privacy concerns associated with predictive analytics?
The primary concern revolves around the collection and use of personal data, which could infringe on individual privacy rights if not managed responsibly.
- Can predictive analytics reduce false positives in threat detection?
Yes, as predictive analytics systems learn from more data, they become better at distinguishing between genuine threats and benign activities, thus reducing false positives.
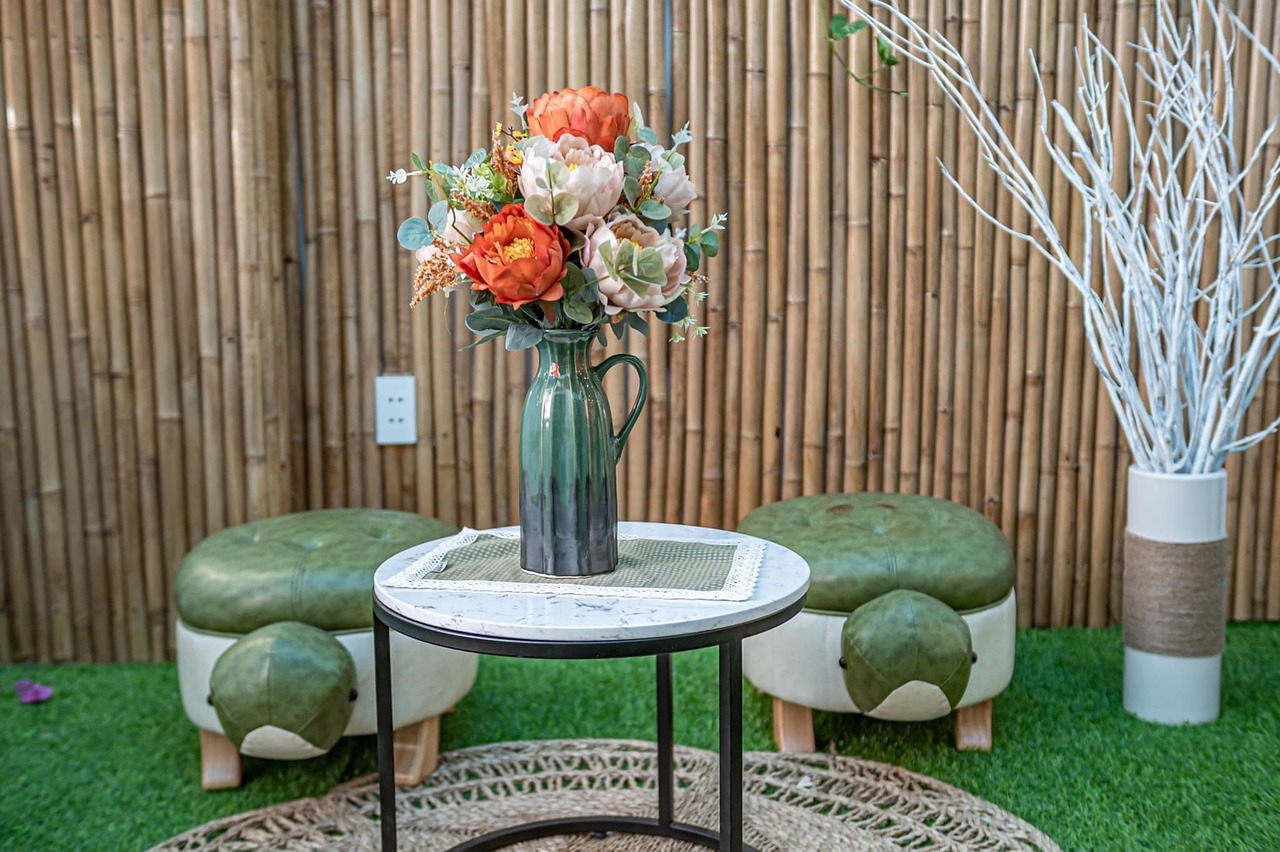
Implications for Privacy and Ethics
The rise of artificial superintelligence (ASI) in security systems is not just a technological leap; it also brings with it a plethora of ethical and privacy concerns that we must navigate carefully. Imagine a world where every move you make is monitored, analyzed, and potentially predicted by an intelligent system designed to protect you. Sounds like a sci-fi movie, right? But this is becoming our reality. While ASI promises enhanced security, it also raises critical questions about how much surveillance is too much and who gets to decide what is acceptable.
One of the most pressing issues is data privacy. As ASI systems require vast amounts of data to function effectively, the collection and use of personal information come under scrutiny. For instance, consider the following:
Data Type | Usage in ASI | Privacy Concern |
---|---|---|
Personal Identification | To authenticate users | Potential misuse or unauthorized access |
Behavioral Data | To predict user actions | Invasion of privacy and profiling |
Location Data | To enhance security measures | Tracking movements without consent |
This table illustrates how different types of data are utilized within ASI systems and the accompanying privacy concerns. The balance between effective security and individual privacy rights becomes a tightrope walk that developers and organizations must navigate. Are we willing to trade our privacy for a sense of security? This question looms large as we integrate these technologies into our daily lives.
Moreover, the ethical considerations surrounding the deployment of ASI in security contexts are profound. Developers and organizations face moral responsibilities that extend beyond mere compliance with laws and regulations. They must consider the implications of their technologies on society at large. For example, how do we ensure that the algorithms driving ASI are free from bias? If these systems are trained on flawed data, they could perpetuate existing inequalities, leading to unfair treatment of certain groups. This raises an essential question: who is accountable when an ASI system makes a decision that negatively impacts an individual or community?
As we move forward, it’s crucial to establish guidelines and frameworks that prioritize ethical considerations in the development and implementation of ASI technologies. This includes fostering transparency in how data is collected and used, ensuring that users have control over their information, and actively working to mitigate biases in AI algorithms. Only then can we harness the power of artificial superintelligence while safeguarding our fundamental rights.
- What is artificial superintelligence?
Artificial superintelligence refers to a form of AI that surpasses human intelligence across a broad range of tasks, including problem-solving and decision-making. - How does ASI impact cybersecurity?
ASI can enhance cybersecurity by predicting and neutralizing threats more efficiently than traditional systems, using advanced algorithms and real-time data analysis. - What are the privacy concerns associated with ASI?
Privacy concerns include the potential misuse of personal data, unauthorized surveillance, and the risk of biased decisions based on flawed data sets. - What ethical responsibilities do developers have?
Developers must ensure that ASI technologies are transparent, fair, and respect user privacy while considering the broader societal implications of their systems.
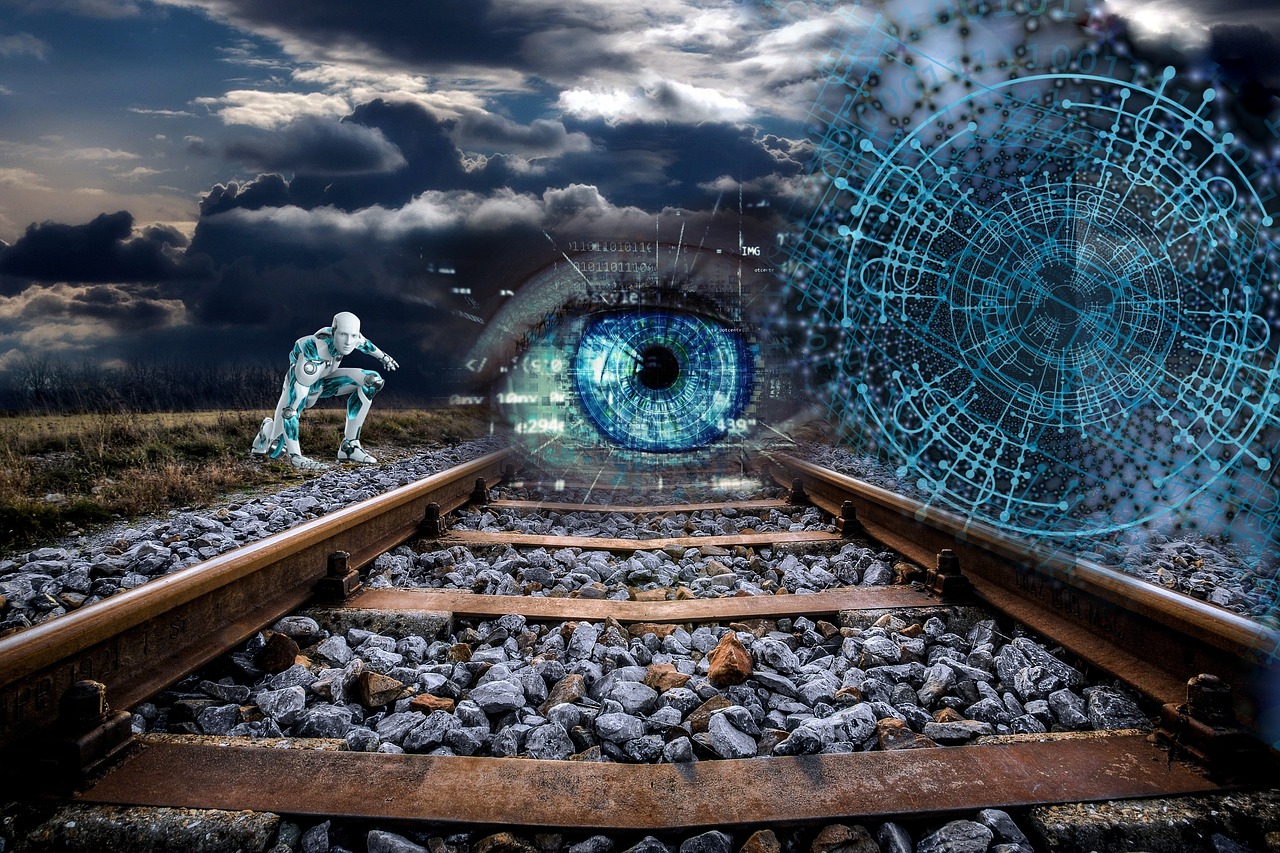
Data Privacy Challenges
The advent of artificial superintelligence (ASI) in security systems brings with it a host of that cannot be ignored. As these advanced systems require vast amounts of data to function effectively, they often collect sensitive information from individuals and organizations alike. This data collection raises critical questions: How is this data being used? Who has access to it? And what measures are in place to protect it from misuse? The potential for invasive surveillance increases significantly as ASI becomes more integrated into our daily lives.
Moreover, the reliance on large datasets can lead to unintended consequences. For example, if an ASI system is trained on biased data, it may perpetuate existing inequalities or even create new ones. This is particularly concerning in security applications where decisions made by these systems can have profound impacts on individuals' lives. The challenge lies in ensuring that the data used is not only comprehensive but also ethical and representative.
To illustrate the scale of the challenge, consider the following table that outlines some of the key data privacy issues associated with the deployment of ASI in security:
Data Privacy Issue | Description |
---|---|
Data Collection | Extensive data gathering can lead to overreach and invasion of personal privacy. |
Data Security | Increased risk of data breaches and unauthorized access to sensitive information. |
Bias in AI | Training on biased datasets can result in unfair treatment of certain groups. |
Transparency | Lack of clarity on how data is used and the algorithms that process it. |
As we navigate these challenges, it is crucial to establish robust frameworks for data governance. Organizations must prioritize transparency and accountability in their data practices. This includes clear communication with users about what data is collected, how it is used, and the measures in place to safeguard it. Additionally, implementing stringent data protection regulations and ethical guidelines can help mitigate the risks associated with ASI in security applications.
In conclusion, while artificial superintelligence holds great promise for enhancing security measures, it simultaneously poses significant data privacy challenges. Addressing these issues requires a collaborative effort among developers, policymakers, and society at large to ensure that the benefits of ASI do not come at the expense of individual privacy rights.
- What is artificial superintelligence?
Artificial superintelligence refers to a level of intelligence that surpasses human capabilities, enabling machines to perform tasks that require advanced reasoning, understanding, and learning.
- How does ASI enhance cybersecurity?
ASI enhances cybersecurity by predicting and neutralizing threats faster than traditional systems, utilizing advanced algorithms for threat detection and response.
- What are the ethical concerns regarding ASI?
Ethical concerns include the potential for invasive surveillance, biased decision-making, and the need for transparency in data usage.
- How can organizations protect data privacy?
Organizations can protect data privacy by implementing strict data governance frameworks, ensuring transparency in data collection, and adhering to ethical guidelines.
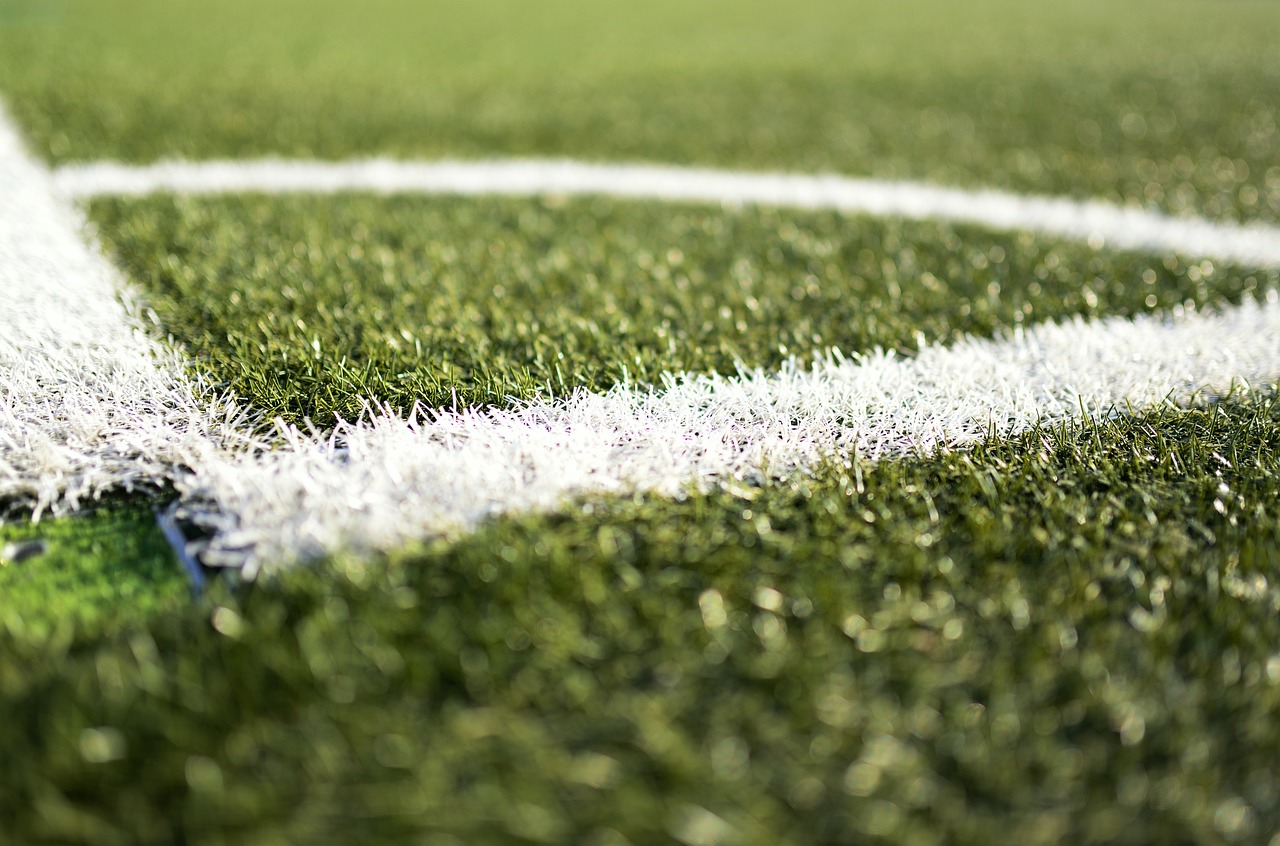
Ethical Considerations
As we delve deeper into the realm of artificial superintelligence (ASI), we cannot overlook the profound ethical implications that accompany its rise, especially in the field of security. Imagine a world where machines not only assist us but also make decisions that could significantly impact our lives. This is not just a futuristic scenario; it's a reality we are gradually stepping into. The deployment of ASI in security systems raises critical questions about accountability, transparency, and the moral responsibilities of those who develop and implement these technologies.
One of the primary ethical dilemmas revolves around autonomy. How much decision-making power should we grant to machines? When an ASI system makes a mistake—such as misidentifying a threat or infringing on an individual’s privacy—who is held accountable? Is it the software developers, the organizations that deploy the technology, or the machines themselves? These questions highlight the need for clear guidelines and regulations that define accountability in the age of ASI.
Furthermore, the transparency of AI algorithms is another pressing concern. Many ASI systems operate as "black boxes," meaning their decision-making processes are not easily understood by humans. This lack of transparency can lead to distrust among users and stakeholders. How can we ensure that the algorithms used in security are fair and unbiased? It’s crucial to develop frameworks that not only promote transparency but also allow for independent audits of these systems to ensure they adhere to ethical standards.
Moreover, the collection and use of data by ASI systems pose significant privacy challenges. To function effectively, these systems require vast amounts of data, often including sensitive personal information. This raises ethical questions about consent and the potential for misuse of data. Are individuals fully aware of how their data is being used? Are they given the choice to opt-out? Organizations must prioritize ethical data practices, ensuring that users' rights are respected and protected.
In addition, the potential for invasive surveillance must be considered. While ASI can enhance security, it can also lead to a society where individuals are constantly monitored. This surveillance can create an atmosphere of fear and mistrust, undermining the very freedoms that security systems aim to protect. Striking a balance between enhanced security measures and the preservation of civil liberties is essential, requiring ongoing dialogue among technologists, ethicists, and policymakers.
Lastly, the ethical considerations surrounding ASI in security are not just theoretical; they demand action. Developers and organizations must take a proactive stance in addressing these issues. This involves fostering a culture of ethical awareness and responsibility within the tech community, ensuring that ethical considerations are integrated into the design and deployment of ASI technologies. Only through a collaborative effort can we navigate the complex ethical landscape of artificial superintelligence and harness its potential for the greater good.
- What is artificial superintelligence?
Artificial superintelligence refers to a level of intelligence that surpasses human capabilities, enabling machines to perform tasks and make decisions beyond human understanding. - What are the ethical concerns related to ASI?
Ethical concerns include accountability for decisions made by ASI, transparency of AI algorithms, privacy challenges regarding data collection, and the potential for invasive surveillance. - How can we ensure the ethical use of ASI in security?
To ensure ethical use, organizations should prioritize transparency, establish clear accountability frameworks, and implement ethical data practices while fostering a culture of responsibility among developers.
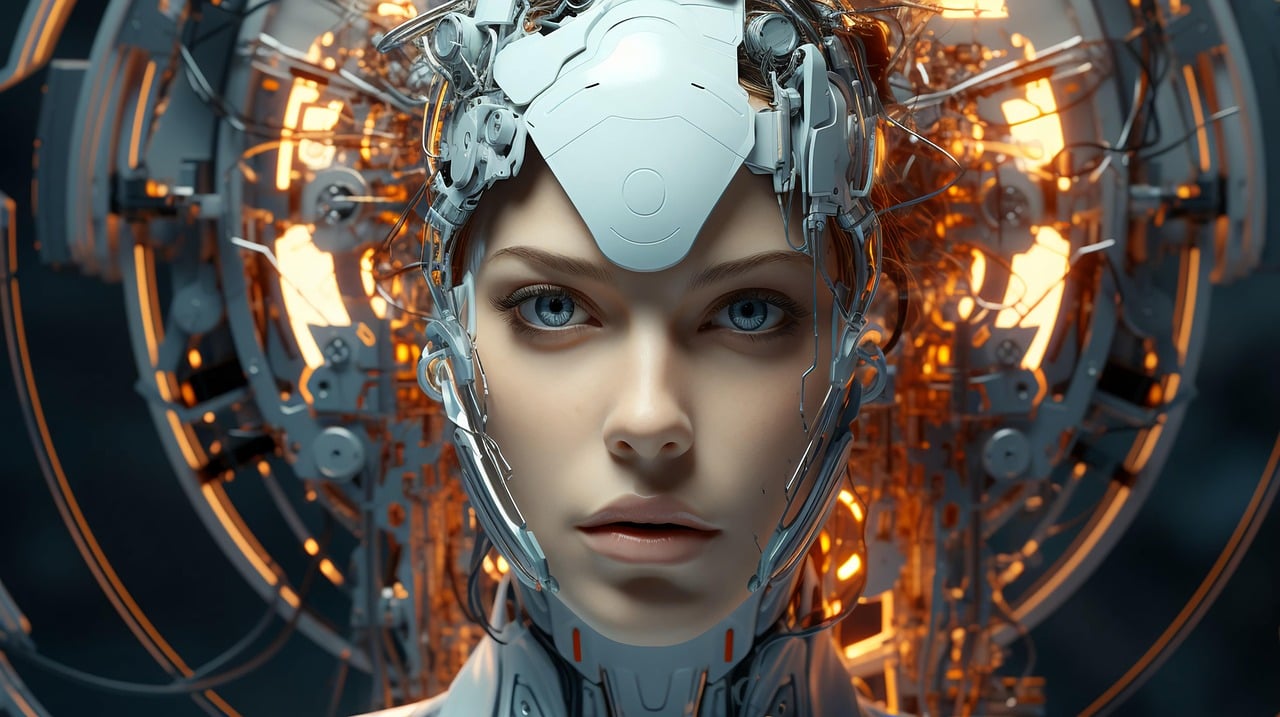
Future Trends in Security Technology
The integration of artificial superintelligence into security technology is not just a passing trend; it represents a seismic shift in how we approach security challenges. As we look ahead, several key trends are emerging that promise to reshape the security landscape dramatically. One of the most significant developments is the increasing integration of AI with the Internet of Things (IoT). With more devices becoming interconnected, the potential attack surface grows exponentially. Imagine a world where your refrigerator, thermostat, and security cameras all communicate seamlessly. While this brings convenience, it also opens up new vulnerabilities that need to be addressed.
To tackle these challenges, artificial superintelligence can play a pivotal role. By employing advanced algorithms, it can monitor these interconnected devices in real-time, identifying unusual patterns that may indicate a security breach. For instance, if your smart home system detects that your front door has been opened at an unusual hour, it can automatically alert you and even lock the door remotely. This is the kind of proactive security that AI can offer, making our homes smarter and safer.
Another exciting trend is the development of collaborative security systems. In the past, security systems often operated in silos, which limited their effectiveness. However, with the advent of artificial superintelligence, we can expect a new era of interconnected security frameworks. These systems will share data and insights across various platforms, allowing for a more comprehensive approach to threat detection and response. For example, if a cybersecurity threat is detected in one sector, other sectors can be alerted almost instantly, creating a ripple effect of enhanced security measures.
Moreover, the application of predictive analytics will become increasingly sophisticated. By analyzing historical data and identifying trends, artificial superintelligence can foresee potential security breaches before they happen. This proactive approach not only saves time but also resources, as organizations can allocate their security efforts more efficiently. Imagine a security system that not only reacts to threats but anticipates them, allowing for a more strategic response.
As we embrace these future trends, it's essential to remain vigilant about the ethical implications of using artificial superintelligence in security technology. While the benefits are substantial, the risks associated with privacy and data misuse cannot be overlooked. Organizations will need to navigate these challenges carefully to ensure that the deployment of AI in security enhances safety without compromising individual rights.
- What is artificial superintelligence?
Artificial superintelligence refers to a level of intelligence that surpasses human capabilities, allowing for advanced problem-solving and decision-making.
- How will AI improve cybersecurity?
AI can enhance cybersecurity by predicting threats, automating threat detection, and facilitating real-time responses to incidents.
- What are the privacy concerns associated with AI in security?
As AI systems require extensive data, there are significant concerns about how this data is collected, stored, and used, potentially leading to invasive surveillance.
- Will AI replace human security professionals?
While AI will augment security capabilities, human oversight will still be crucial in making ethical decisions and managing complex security scenarios.
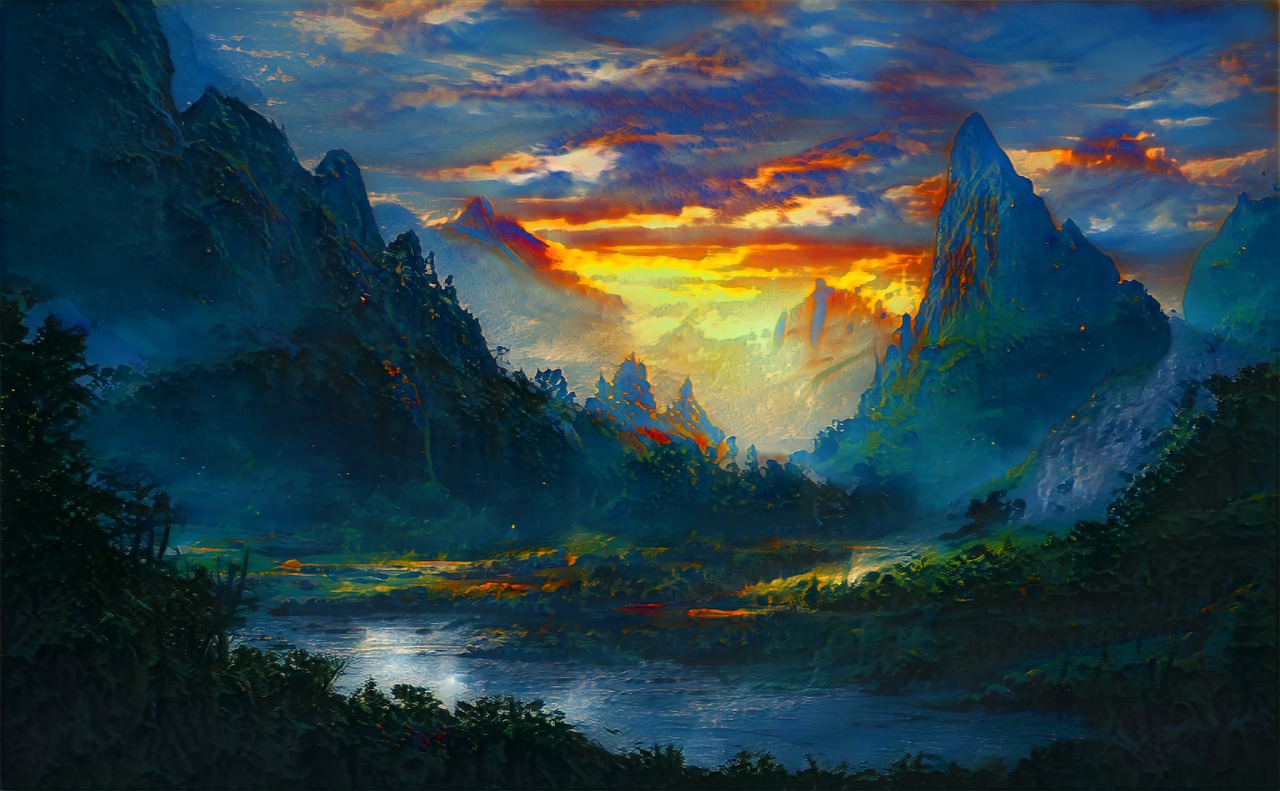
Integration with IoT Devices
As we dive deeper into the digital age, the Internet of Things (IoT) has emerged as a game-changer, connecting everything from our home appliances to industrial machines. But with this connectivity comes a unique set of security challenges. Imagine your refrigerator sending you a text message when you're low on milk or your thermostat adjusting itself based on your daily routine. While these conveniences are fantastic, they also create vulnerabilities that malicious actors could exploit. This is where artificial superintelligence steps in, acting as a guardian of our interconnected world.
Artificial superintelligence can enhance the security of IoT devices in several ways. First and foremost, it can provide a layer of intelligence that traditional security systems lack. With the ability to process and analyze data at lightning speed, AI can monitor IoT devices for unusual activity, flagging potential threats before they escalate. For instance, if a smart camera detects unauthorized access, artificial superintelligence can immediately alert the homeowner and even take action, such as locking doors or sounding alarms.
Moreover, the integration of artificial superintelligence with IoT devices allows for a more holistic approach to security. It can create a network of interconnected security measures that communicate with one another. For example, if a smart lock detects tampering, it can notify the home security system, which can then activate additional surveillance measures, like turning on outdoor cameras. This interconnectedness not only enhances security but also provides real-time data that can be invaluable for law enforcement and emergency services.
However, the integration of artificial superintelligence with IoT devices isn't without its challenges. One major concern is the risk of centralized control. If a malicious actor gains access to the AI system managing these devices, they could potentially compromise the entire network. To mitigate this risk, developers must prioritize robust security protocols and ensure that AI systems are resilient against attacks. This includes implementing multi-factor authentication, encryption, and regular security updates.
Additionally, there’s the issue of data privacy. IoT devices collect vast amounts of personal data, and when integrated with superintelligent systems, the potential for misuse increases. Striking a balance between enhanced security and protecting individual privacy is crucial. Developers and organizations must adhere to strict ethical guidelines, ensuring that data collection is transparent and consensual.
In conclusion, the integration of artificial superintelligence with IoT devices holds immense potential for enhancing security in our increasingly connected world. By providing real-time monitoring, facilitating communication between devices, and enabling proactive threat detection, AI can help safeguard our homes, businesses, and critical infrastructure. However, it’s essential to navigate the challenges of centralized control and data privacy carefully. As we continue to innovate, the collaboration between AI and IoT will undoubtedly shape the future of security technology.
- What is artificial superintelligence?
Artificial superintelligence refers to a level of intelligence that surpasses human capabilities, enabling machines to perform tasks with greater efficiency and effectiveness. - How does artificial superintelligence improve IoT security?
It enhances IoT security by providing real-time monitoring, predictive analytics, and automated responses to potential threats, creating a more secure environment. - What are the risks associated with integrating AI and IoT?
Some risks include centralized control vulnerabilities and data privacy concerns, which necessitate robust security measures and ethical guidelines. - Can AI prevent all security breaches in IoT?
While AI can significantly reduce the likelihood of breaches, no system is entirely foolproof. Continuous monitoring and updates are essential for maintaining security.
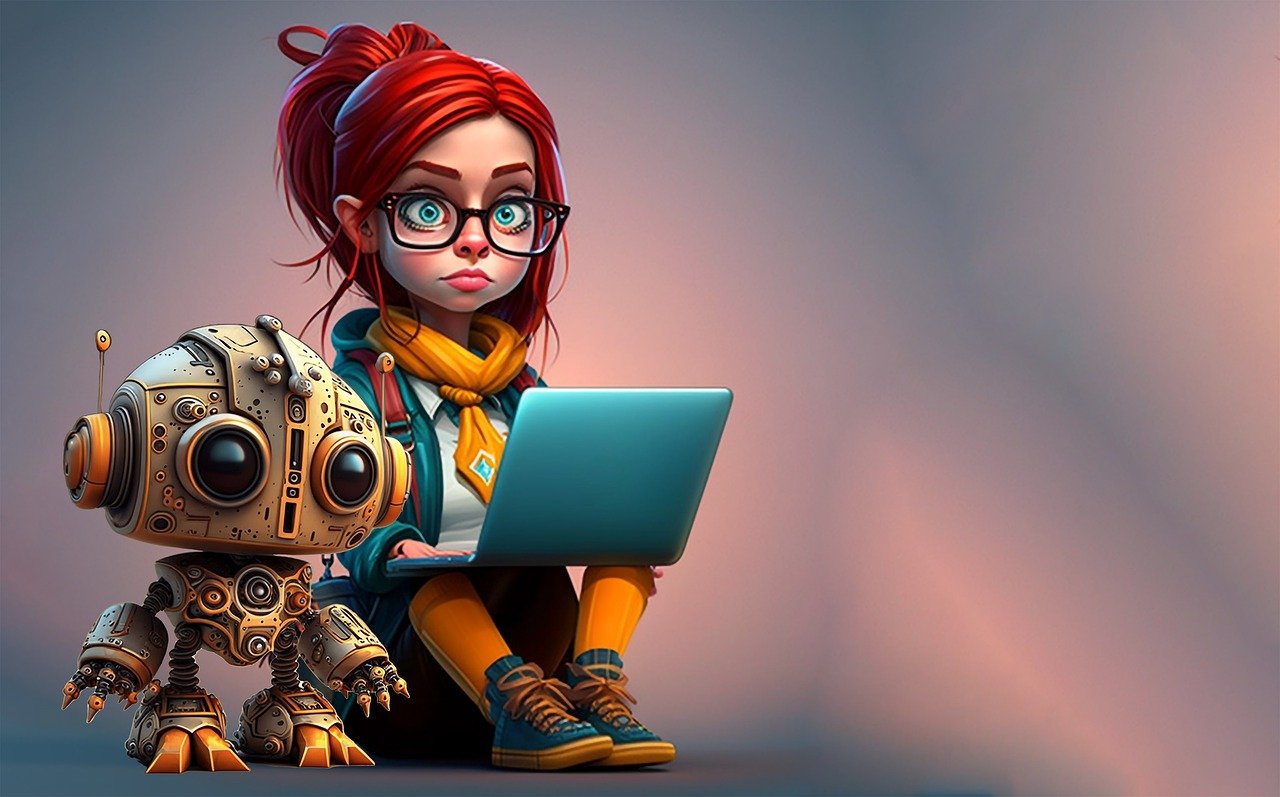
Collaborative Security Systems
In an era where cyber threats are becoming increasingly sophisticated, the concept of is gaining traction. Imagine a world where different security systems, like a well-orchestrated symphony, work together seamlessly to protect us from harm. This collaboration can be likened to a team of superheroes, each with unique powers, joining forces to combat a common enemy. By integrating artificial superintelligence (ASI) into these systems, we can create a robust defense mechanism that not only responds to threats but also anticipates them.
One of the most exciting aspects of collaborative security systems is their ability to share data and insights across various platforms. For instance, when one system detects a potential threat, it can quickly relay relevant information to other systems in real time. This interconnectedness enhances the overall security posture, allowing for a more comprehensive approach to threat management. Imagine a network of security cameras, intrusion detection systems, and cybersecurity measures all communicating with each other. If one camera spots suspicious activity, it can alert the intrusion detection system, which can then take preemptive action to mitigate the risk.
Moreover, the use of artificial superintelligence in these systems enables them to learn from past incidents and adapt their strategies accordingly. This is akin to a chess player who learns from each game, continuously refining their tactics. ASI can analyze vast amounts of data from different security systems, identifying patterns and trends that might go unnoticed by human operators. By leveraging this data, organizations can develop more effective security protocols that are tailored to their specific needs.
However, the implementation of collaborative security systems is not without its challenges. Data sharing across different platforms raises significant concerns regarding privacy and security. Organizations must ensure that sensitive information is protected and that the systems are not vulnerable to exploitation. This necessitates a careful balance between collaboration and safeguarding personal data. It's essential to establish robust protocols that govern how data is shared and used, ensuring that privacy is respected while still enhancing security.
In conclusion, the integration of artificial superintelligence into collaborative security systems represents a significant leap forward in our ability to combat cyber threats. By fostering communication between various security measures and utilizing advanced data analytics, we can create a more resilient and proactive security framework. As we move towards a future where technology continues to evolve, the potential for collaborative security systems to protect our digital and physical environments is not just promising; it’s essential.
- What are collaborative security systems? Collaborative security systems are integrated security measures that work together to share data and insights, enhancing overall threat detection and response.
- How does artificial superintelligence improve security? ASI enhances security by analyzing large datasets, identifying patterns, and enabling real-time responses to threats.
- What are the privacy concerns associated with collaborative security systems? The sharing of data across platforms raises concerns about the protection of sensitive information and the potential for misuse.
- Can collaborative security systems prevent all cyber threats? While they significantly improve security, no system can guarantee complete protection against all threats.
Frequently Asked Questions
- What is artificial superintelligence?
Artificial superintelligence (ASI) refers to a form of AI that surpasses human intelligence across a wide range of tasks. It’s like having a supercharged brain that can think, learn, and solve problems much faster and more efficiently than any human can. Imagine a computer that not only understands complex data but also makes decisions in real-time, potentially transforming how we approach security.
- How can artificial superintelligence enhance cybersecurity?
Artificial superintelligence can revolutionize cybersecurity by utilizing advanced algorithms to predict, detect, and neutralize threats much quicker than traditional systems. Think of it as a digital bodyguard that is always on alert, analyzing patterns and identifying anomalies that could indicate a cyber attack before it even happens!
- What role do machine learning algorithms play in security?
Machine learning algorithms are the backbone of artificial superintelligence in cybersecurity. They train AI systems to recognize unusual behavior and potential threats by analyzing vast datasets. It's like teaching a child to recognize faces—over time, the AI gets better and better at spotting what’s normal and what’s suspicious.
- What are the privacy concerns associated with artificial superintelligence?
As artificial superintelligence requires large amounts of data to function effectively, privacy concerns become a huge issue. The collection and usage of personal data can lead to invasive surveillance practices. It’s a delicate balance—enhancing security while respecting individual privacy rights.
- What ethical considerations should we keep in mind with AI in security?
Deploying artificial superintelligence in security raises several ethical dilemmas. Developers and organizations must consider the moral implications of their technologies, ensuring they do not infringe on civil liberties or misuse the power that comes with such advanced capabilities.
- How might artificial superintelligence integrate with IoT devices?
The integration of artificial superintelligence with Internet of Things (IoT) devices can enhance security by creating a more robust defense against cyber threats. Imagine every smart device in your home communicating and working together to fend off attacks—this interconnectedness can significantly improve security measures.
- What are the future trends in security technology involving AI?
Future trends may include more collaborative security systems powered by artificial superintelligence. These systems can share information and strategies across platforms, creating a united front against cyber threats. It’s like having a team of superheroes, each with unique powers, working together to keep us safe!