Importance of AI in Big Data Analysis
In today's digital landscape, the sheer volume of data generated is nothing short of astounding. Every second, countless bytes of information are created, and this influx of data presents both a challenge and an opportunity for businesses and organizations. Enter artificial intelligence (AI), a game-changing technology that is revolutionizing how we analyze and interpret big data. By leveraging AI, companies can sift through mountains of information to uncover actionable insights, streamline processes, and enhance decision-making.
Imagine trying to find a needle in a haystack. That's what traditional data analysis often feels like when dealing with vast datasets. However, with AI, this process becomes more like using a powerful magnet that attracts and identifies relevant information in an instant. AI technologies are designed to automate data processing, making it faster and more efficient. This is crucial because timely insights can be the difference between seizing an opportunity and watching it slip away.
Moreover, the integration of AI into big data analysis is not just about speed; it's also about enhancing the quality of insights. AI algorithms can detect patterns and trends that might go unnoticed by the human eye. By analyzing historical data, AI can help organizations predict future behaviors and outcomes. This predictive capability is invaluable in today's competitive landscape, where businesses must make informed strategic decisions to stay ahead of the curve.
As we delve deeper into the role of AI in big data analysis, it's essential to recognize the various techniques and methodologies that drive this innovation. From machine learning algorithms that uncover hidden patterns to natural language processing (NLP) that enables the analysis of human language, AI is transforming the way we interact with data. Each of these components plays a vital role in enhancing the overall effectiveness of data analysis, ensuring that organizations can leverage their data assets to their fullest potential.
In conclusion, the importance of AI in big data analysis cannot be overstated. As organizations continue to navigate the complexities of vast datasets, AI stands out as a powerful ally. By embracing AI technologies, businesses can unlock new opportunities, drive innovation, and make data-driven decisions that propel them forward in an ever-evolving marketplace.
- What is the role of AI in big data analysis? AI streamlines data processing, uncovers patterns, and enhances decision-making by providing actionable insights.
- How does predictive analytics work with AI? AI analyzes historical data to forecast trends and behaviors, allowing organizations to make informed strategic decisions.
- What are machine learning algorithms? These are AI techniques that identify patterns in data, facilitating automated predictions and improving analysis accuracy.
- What is natural language processing? NLP allows AI to interpret human language, enabling businesses to extract insights from customer feedback and social media.
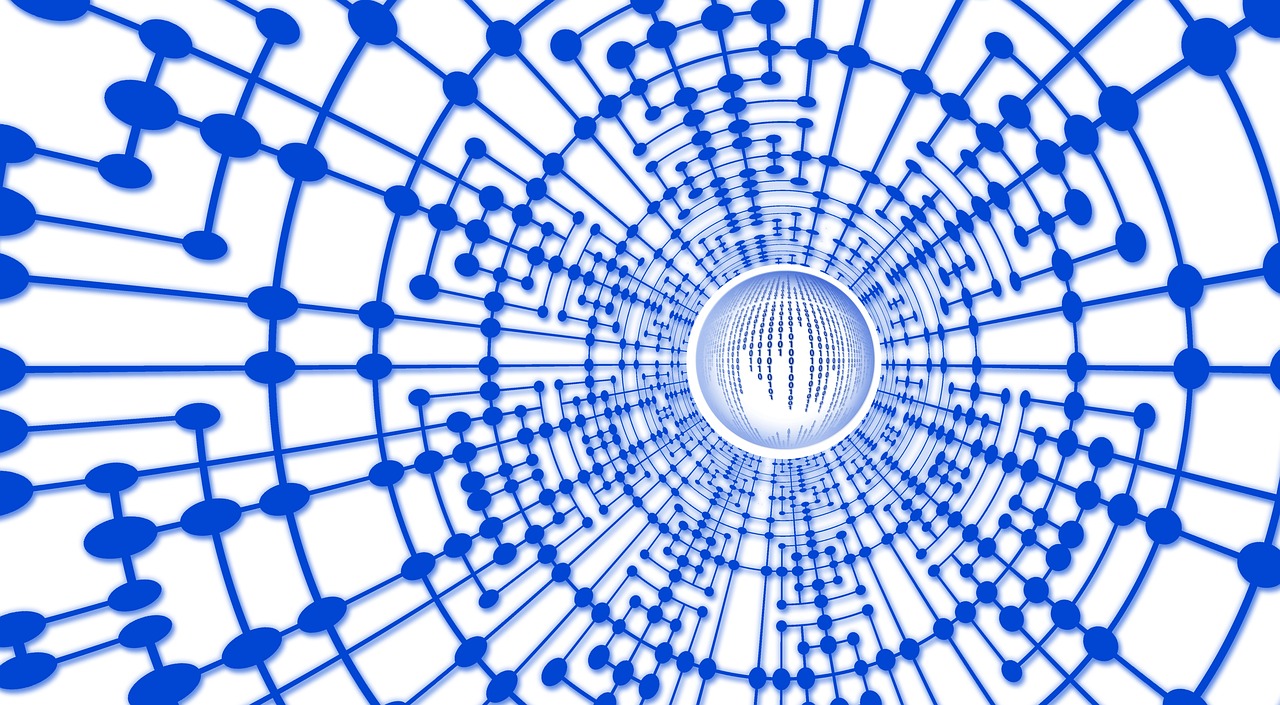
The Role of AI in Data Processing
In today's digital age, the sheer volume of data generated every second is staggering. From social media interactions to online transactions, the amount of information available is growing exponentially. This is where artificial intelligence (AI) steps in, revolutionizing the way we handle and process data. Imagine trying to sift through a mountain of sand to find a few precious grains; that's what data analysts face without AI. By automating and streamlining data processing workflows, AI empowers organizations to manage vast datasets with remarkable speed and accuracy.
AI technologies, such as machine learning and neural networks, are designed to enhance efficiency and reduce human error in data processing. For instance, traditional data processing methods can be time-consuming and prone to inaccuracies, but AI systems can analyze data at lightning speed, identifying trends and anomalies that might go unnoticed. This capability is crucial for businesses that rely on timely insights for strategic decision-making. With AI, organizations can transform raw data into actionable insights, enabling them to stay ahead of the competition.
Moreover, AI's ability to learn from data means that the more it processes, the better it becomes. This self-improving aspect of AI not only enhances the accuracy of data analysis but also allows for continuous refinement of processes. For example, in sectors like finance, AI can analyze transaction patterns to detect fraudulent activities, adapting its algorithms in real-time to improve detection rates. This adaptability is akin to having a personal assistant who learns your preferences over time, becoming increasingly effective at anticipating your needs.
Another vital component of AI in data processing is its ability to integrate various data sources. Organizations often deal with data from multiple channels, which can lead to inconsistencies and data silos. AI can seamlessly merge these disparate data sources, providing a holistic view of information that is crucial for comprehensive analysis. This integration not only saves time but also ensures that decision-makers have access to the most relevant and up-to-date information.
To illustrate the impact of AI on data processing, consider the following table that highlights key benefits:
Benefit | Description |
---|---|
Speed | AI processes large datasets in a fraction of the time compared to traditional methods. |
Accuracy | Reduces human error and enhances the reliability of data analysis. |
Scalability | AI systems can easily scale to handle growing volumes of data. |
Integration | Combines data from various sources for a comprehensive view. |
In summary, the role of AI in data processing is pivotal. It not only accelerates the handling of large datasets but also enhances accuracy, integrates diverse data sources, and continuously improves its capabilities. As organizations continue to navigate the complexities of big data, leveraging AI will be essential for unlocking valuable insights and driving innovation.
- What is AI's primary function in data processing? AI automates and enhances the efficiency of analyzing large datasets, providing timely insights for decision-making.
- How does AI improve accuracy in data analysis? AI reduces human error by utilizing algorithms that learn from data, improving their predictions and analyses over time.
- Can AI integrate data from different sources? Yes, AI can seamlessly merge data from various channels, providing a comprehensive view for better analysis.
- What industries benefit most from AI in data processing? Industries such as finance, healthcare, marketing, and retail significantly benefit from AI-enhanced data processing.
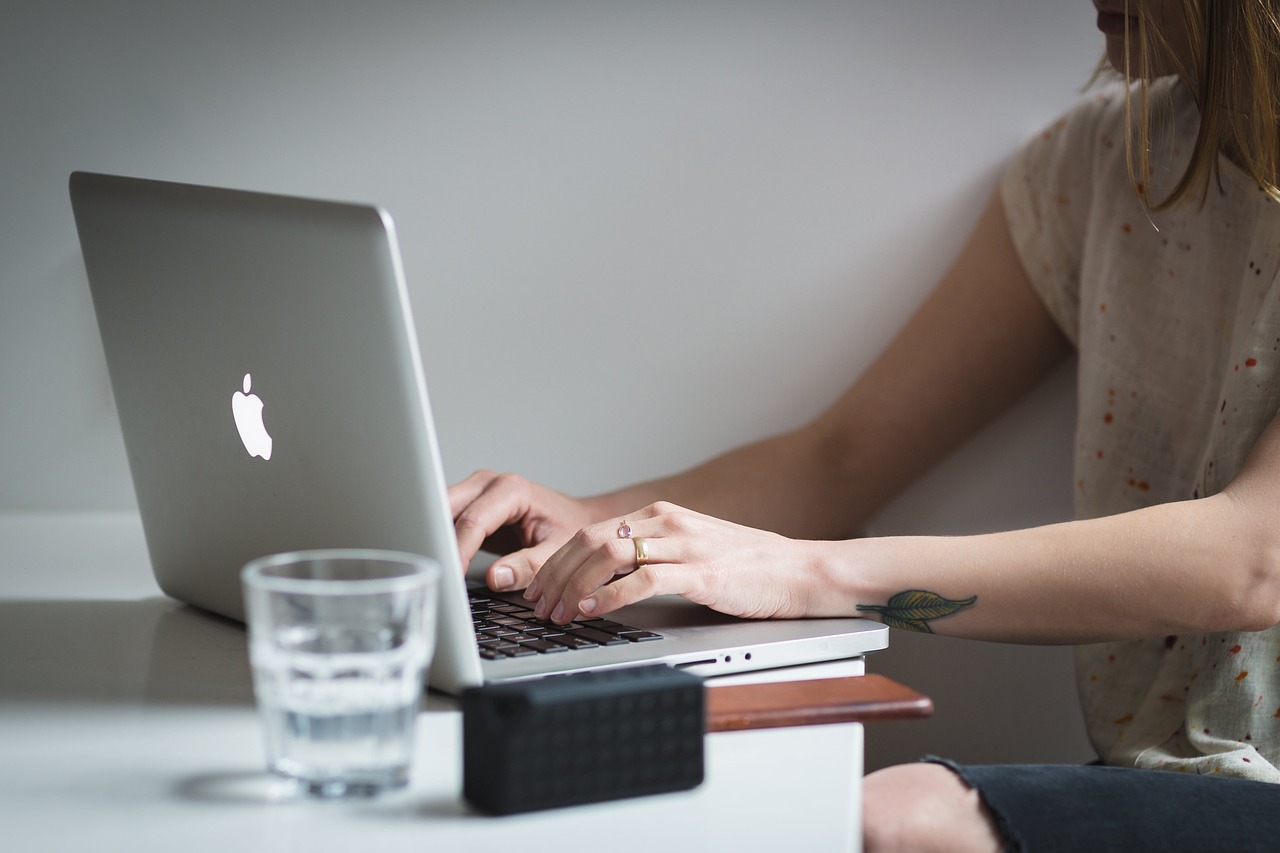
Predictive Analytics and AI
In today's fast-paced business environment, the integration of Artificial Intelligence (AI) with predictive analytics has become a game-changer for organizations aiming to stay ahead of the curve. Imagine having the ability to foresee trends, consumer behaviors, and market shifts before they happen! This is precisely what predictive analytics powered by AI offers. By analyzing historical data and identifying patterns, AI enables businesses to make informed strategic decisions that can significantly enhance their competitive edge.
One of the most exciting aspects of this integration is its ability to transform raw data into actionable insights. AI algorithms can sift through vast amounts of data, uncovering hidden patterns that might go unnoticed by human analysts. For instance, retailers can predict inventory needs based on seasonal trends and customer preferences, while financial institutions can assess risks and opportunities in real time. This not only leads to improved efficiency but also helps in allocating resources more effectively.
To illustrate the impact of predictive analytics in various sectors, consider the following examples:
- Healthcare: AI-driven predictive models can forecast patient admissions, allowing hospitals to optimize staffing and resource allocation.
- Marketing: Businesses can tailor their marketing strategies by predicting customer responses to campaigns, resulting in higher conversion rates.
- Manufacturing: Predictive maintenance powered by AI helps in anticipating equipment failures, reducing downtime and maintenance costs.
However, the true power of predictive analytics lies in its ability to adapt and learn from new data. AI systems continuously refine their models, ensuring that predictions remain accurate as market dynamics evolve. This is where Machine Learning (ML) comes into play. By employing various machine learning algorithms, organizations can enhance their predictive capabilities, making them not just reactive but also proactive in their decision-making processes.
Moreover, the combination of AI and predictive analytics fosters a culture of data-driven decision-making within organizations. When teams have access to reliable forecasts, they can confidently pursue innovative strategies, allocate budgets wisely, and mitigate risks effectively. This leads to a significant transformation in how businesses operate, ultimately driving growth and innovation.
In summary, the synergy between AI and predictive analytics is not just a technological advancement; it is a fundamental shift in how organizations approach decision-making. By leveraging these tools, companies can navigate the complexities of the modern market landscape with greater agility and foresight, setting the stage for long-term success.
- What is predictive analytics? Predictive analytics involves using historical data and statistical algorithms to identify the likelihood of future outcomes based on past behaviors.
- How does AI enhance predictive analytics? AI enhances predictive analytics by automating data analysis, uncovering complex patterns, and continuously improving predictions through machine learning.
- What industries benefit from predictive analytics? Industries such as healthcare, finance, retail, and manufacturing significantly benefit from predictive analytics to improve decision-making and operational efficiency.
- Can predictive analytics predict customer behavior? Yes, predictive analytics can forecast customer behavior by analyzing past interactions and identifying trends that influence purchasing decisions.
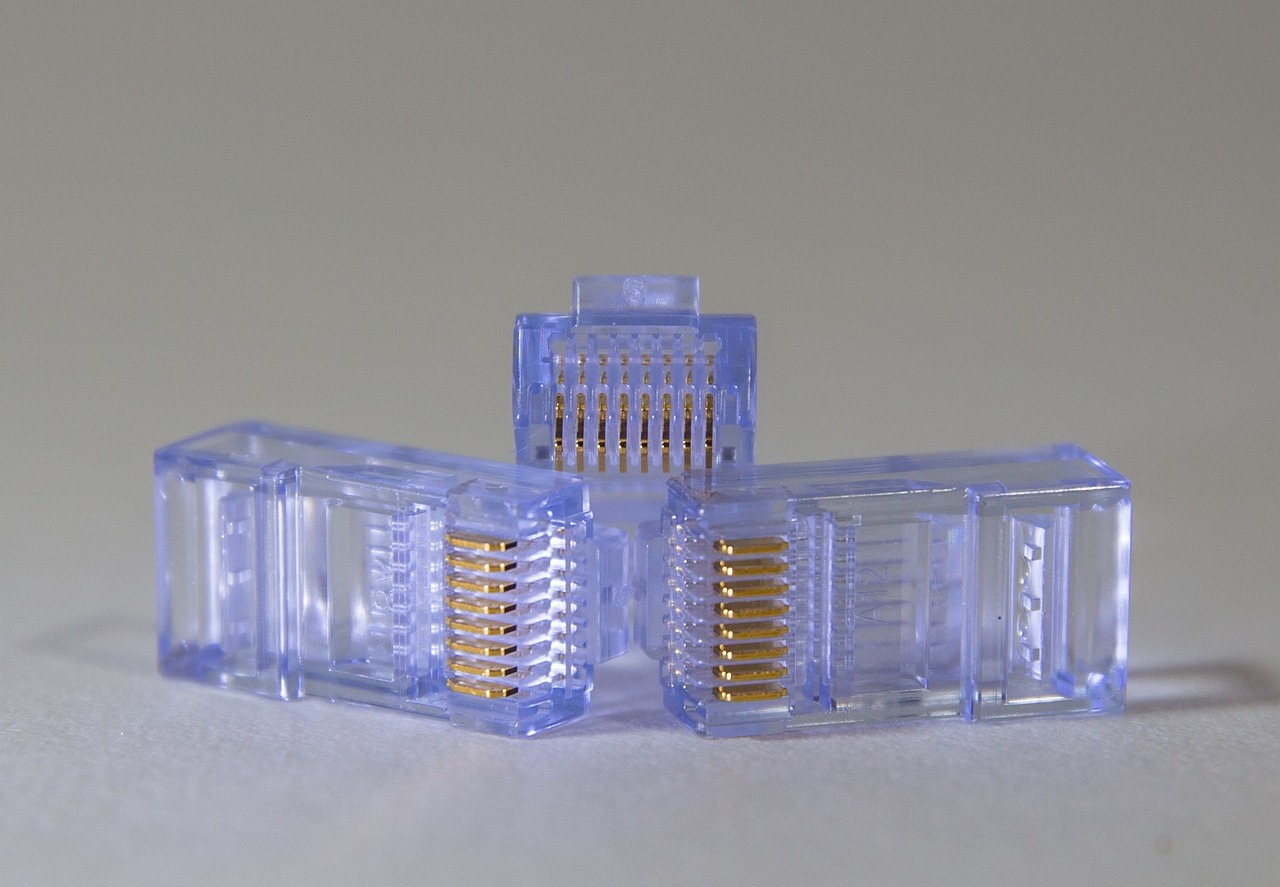
Machine Learning Algorithms
Machine learning algorithms are at the heart of how artificial intelligence (AI) interacts with big data. Imagine trying to find a needle in a haystack, but instead of just searching blindly, you have a smart assistant that learns from every attempt you make. This is essentially what machine learning does; it helps organizations sift through vast amounts of data to uncover hidden patterns and insights that would otherwise remain obscured. By employing these algorithms, businesses can automate predictions and improve the accuracy of their data analysis, ultimately leading to better decision-making.
There are several types of machine learning algorithms, each with its unique strengths and applications. Broadly speaking, they can be categorized into three main types: supervised learning, unsupervised learning, and reinforcement learning.
Type of Learning | Description | Common Algorithms |
---|---|---|
Supervised Learning | Involves training a model on labeled data, where the desired output is known. | Linear Regression, Decision Trees, Support Vector Machines |
Unsupervised Learning | Used for clustering and association problems, where the model learns from unlabeled data. | K-Means Clustering, Hierarchical Clustering, Apriori Algorithm |
Reinforcement Learning | A type of learning where an agent learns to make decisions by receiving rewards or penalties. | Q-Learning, Deep Q-Networks, Proximal Policy Optimization |
Each of these algorithms has its own set of applications and is suited for different types of data analysis tasks. For instance, supervised learning is often used in scenarios where historical data is available, such as predicting customer churn or sales forecasting. On the other hand, unsupervised learning shines in exploratory data analysis, helping businesses identify natural groupings in their data, like segmenting customers based on purchasing behavior.
Furthermore, reinforcement learning is gaining traction in fields like robotics and game development, where an agent learns to navigate environments by trial and error. This adaptability is crucial for tasks that require a high level of decision-making under uncertainty.
In summary, machine learning algorithms serve as powerful tools for unlocking the potential of big data. They not only enhance the accuracy of predictions but also empower organizations to make data-driven decisions that can lead to significant competitive advantages. By leveraging these algorithms, businesses can transform their data into actionable insights that drive innovation and growth.
- What is the difference between supervised and unsupervised learning?
Supervised learning uses labeled data to train algorithms, while unsupervised learning works with unlabeled data to find hidden patterns. - Can machine learning algorithms work with small datasets?
While they can, machine learning typically performs better with larger datasets where patterns can be more easily identified. - How do I choose the right machine learning algorithm?
Choosing the right algorithm depends on the problem at hand, the nature of the data, and the desired outcome. It often requires experimentation and validation.
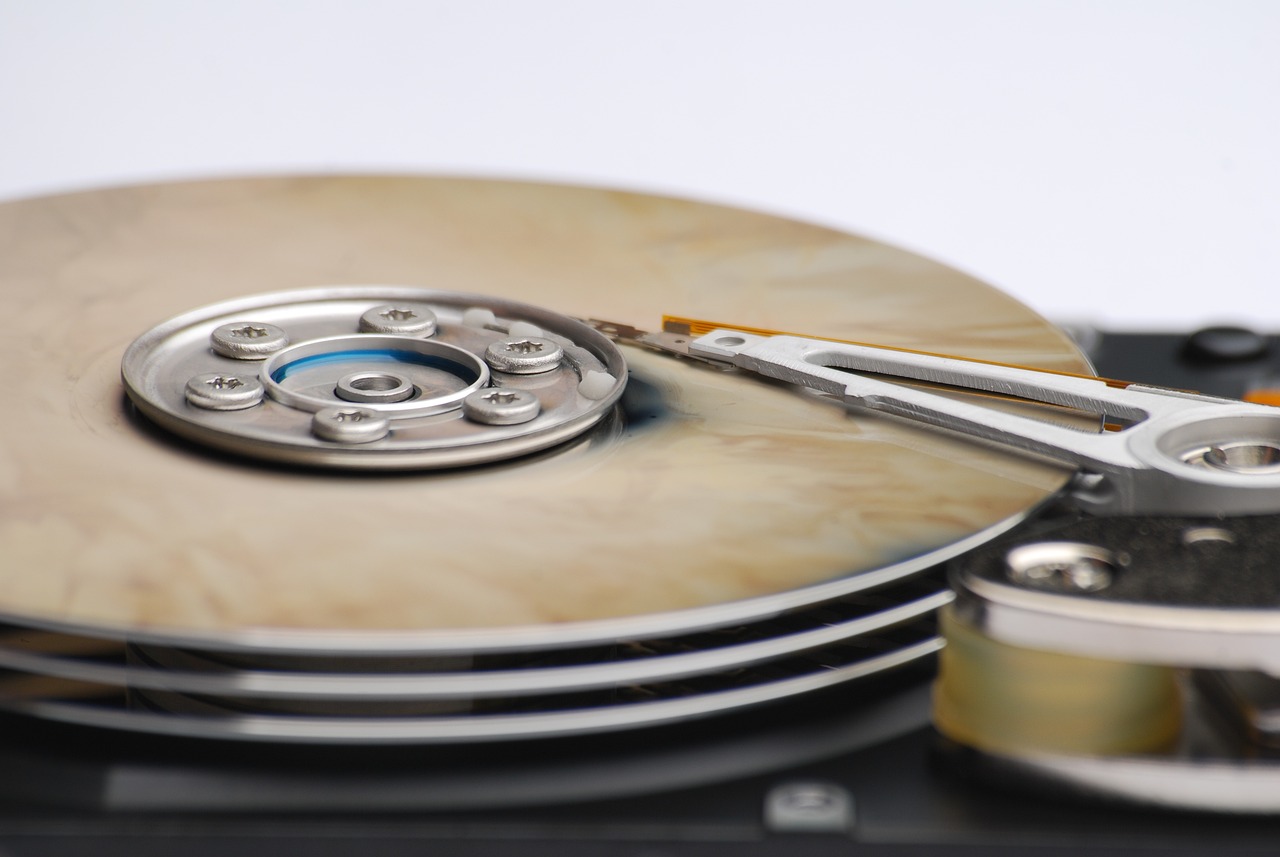
Supervised vs. Unsupervised Learning
When diving into the world of machine learning, one of the first distinctions you’ll encounter is between supervised and unsupervised learning. Think of supervised learning as a teacher guiding students through lessons, while unsupervised learning is like a student exploring the world without any guidance. In supervised learning, the model is trained on a labeled dataset, meaning that each input data point is paired with the correct output. This approach is particularly useful when you have clear objectives and outcomes in mind. For example, if a company wants to predict customer churn, it can utilize historical data where the outcomes (whether a customer left or stayed) are already known. This allows the model to learn from past examples and make accurate predictions for future cases.
On the other hand, unsupervised learning operates in a different realm. Here, the model is fed data without explicit instructions on what to do with it. The goal is to identify patterns and relationships within the data itself. This method is akin to discovering hidden treasures in a vast ocean of information. For instance, a retail company might use unsupervised learning to segment its customers into distinct groups based on purchasing behavior, without pre-defined labels. This can unveil insights that were previously hidden, such as identifying a new customer demographic that prefers organic products.
To help clarify the differences, let’s break it down into a simple comparison:
Feature | Supervised Learning | Unsupervised Learning |
---|---|---|
Data Type | Labeled data | Unlabeled data |
Objective | Predict outcomes | Discover patterns |
Examples | Classification, Regression | Clustering, Association |
Use Cases | Email spam detection, Credit scoring | Market segmentation, Anomaly detection |
Both methods have their unique advantages and can be chosen based on the specific needs of the analysis. Supervised learning shines when the desired outcome is clear and data is plentiful, whereas unsupervised learning is invaluable when exploring unknown territories within a dataset. In many cases, organizations might even employ a combination of both approaches to gain a comprehensive understanding of their data landscape.
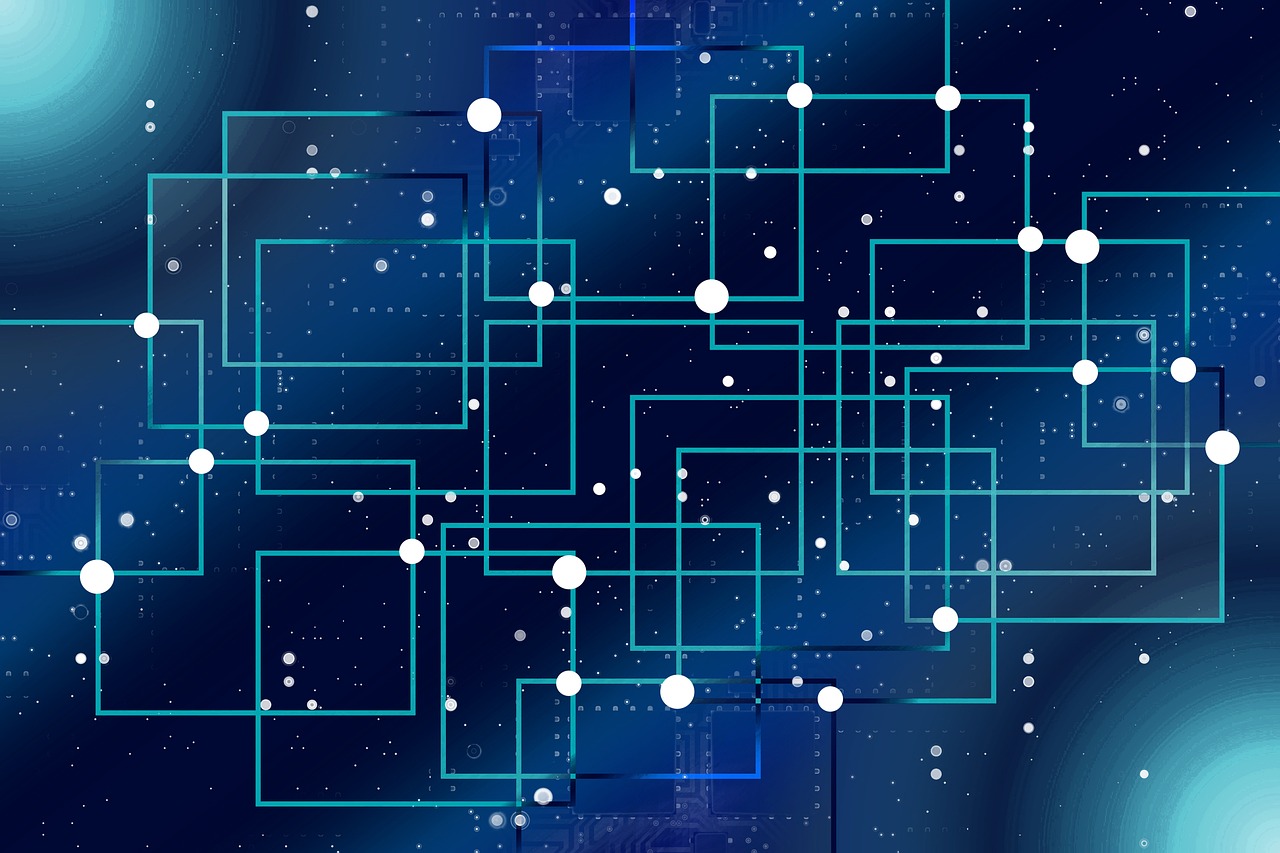
Deep Learning Techniques
Deep learning techniques have emerged as a game-changer in the realm of big data analysis, particularly when it comes to processing unstructured data. Imagine trying to make sense of thousands of images, audio files, or text documents. Traditional data processing methods can feel like searching for a needle in a haystack, but deep learning acts like a powerful magnet, efficiently pulling out the relevant insights.
At its core, deep learning is a subset of machine learning that utilizes neural networks with many layers—hence the term "deep." These networks are designed to mimic the way humans think and learn, making them exceptionally adept at recognizing patterns. For instance, when analyzing images, a deep learning model can identify objects, faces, and even emotions with remarkable accuracy. This capability is invaluable for businesses looking to enhance customer experience through personalized interactions.
One of the standout features of deep learning is its ability to automatically improve its performance as it processes more data. This characteristic is akin to how we humans learn from experience; the more we practice, the better we become. In practical terms, this means that as organizations feed their deep learning models with more data, the models become increasingly sophisticated, leading to richer and more actionable insights.
Furthermore, deep learning techniques excel in areas such as:
- Image Recognition: Automating the identification of objects and scenes in images.
- Speech Recognition: Converting spoken language into text, enabling voice-activated systems.
- Natural Language Processing: Understanding and generating human language for applications like chatbots and sentiment analysis.
However, implementing deep learning isn't without its challenges. Organizations must ensure they have access to large datasets, as deep learning thrives on vast amounts of information. Additionally, the computational power required can be significant, necessitating investments in advanced hardware. But the rewards are often worth the effort, as deep learning can unlock insights that were previously hidden, driving innovation and strategic decision-making.
In conclusion, deep learning techniques represent a frontier in big data analysis, offering unparalleled capabilities in understanding complex data. As businesses continue to harness the power of these techniques, they position themselves at the forefront of their industries, ready to tackle the challenges of tomorrow.
Q: What is deep learning?
A: Deep learning is a subset of machine learning that uses neural networks with many layers to analyze data and make predictions, particularly effective in processing unstructured data like images and text.
Q: How does deep learning differ from traditional machine learning?
A: While traditional machine learning often requires manual feature extraction, deep learning automates this process, allowing models to learn directly from raw data.
Q: What are some applications of deep learning?
A: Deep learning is used in various applications, including image and speech recognition, natural language processing, and autonomous vehicles.
Q: Is deep learning suitable for small datasets?
A: Deep learning typically requires large datasets to perform effectively. For smaller datasets, traditional machine learning methods may be more appropriate.
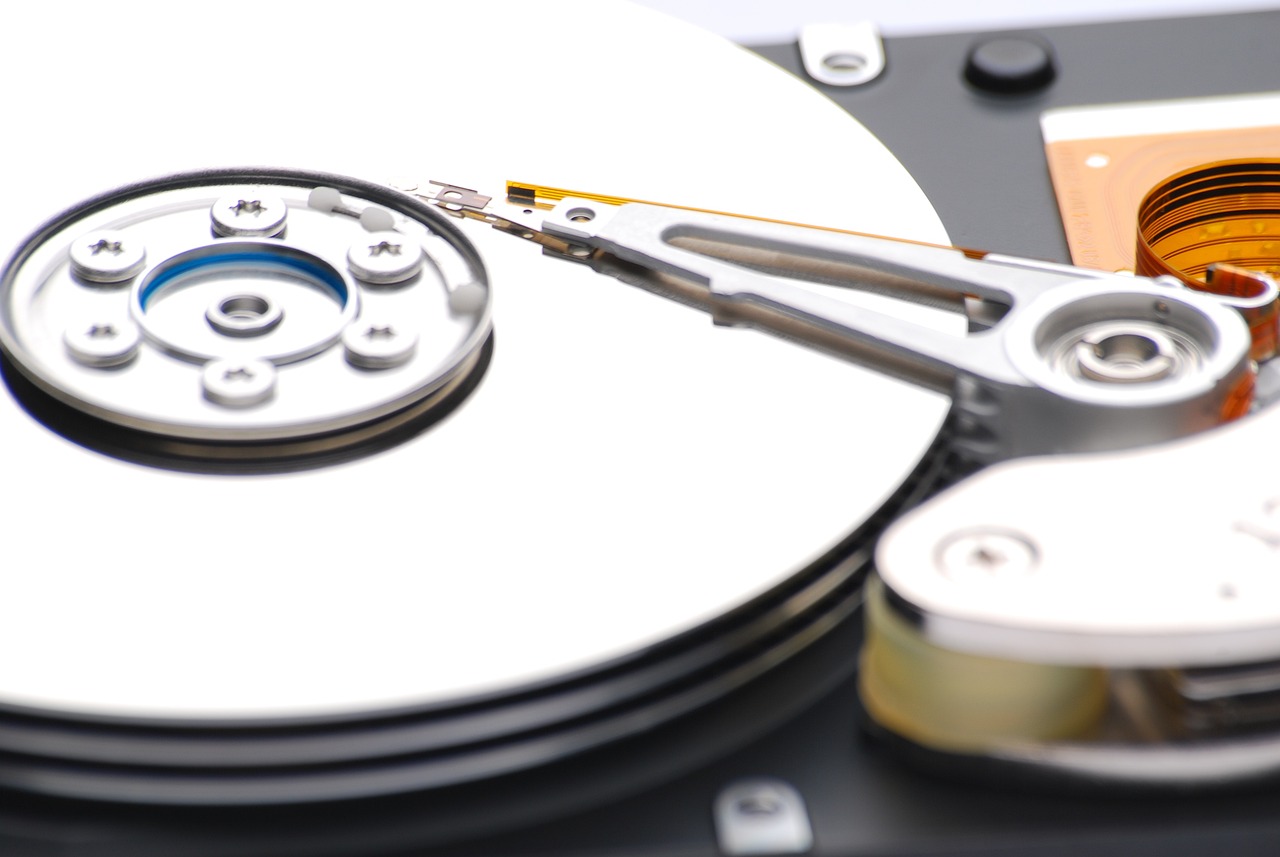
Natural Language Processing in Big Data
Natural Language Processing, or NLP, is one of the most exciting fields in artificial intelligence, particularly when it comes to analyzing big data. Imagine having the power to understand and interpret human language at scale—this is what NLP brings to the table. In a world overflowing with unstructured data, such as social media posts, customer reviews, and online articles, NLP acts as a bridge, transforming raw text into actionable insights. By leveraging NLP, businesses can tap into the sentiments, opinions, and trends that emerge from the vast sea of human communication.
Consider this: every day, millions of people express their thoughts and feelings online. From tweets to blog posts, the potential for extracting valuable insights from this data is enormous. However, the challenge lies in the sheer volume and complexity of the language used. This is where NLP shines. It employs various techniques to analyze text, including tokenization, sentiment analysis, and entity recognition. These methods allow organizations to decode what customers are really saying about their products or services.
For instance, through sentiment analysis, businesses can gauge public opinion about a new product launch or marketing campaign. By categorizing sentiments as positive, negative, or neutral, companies can adjust their strategies accordingly. This not only enhances customer satisfaction but also drives better business outcomes. It's like having a pulse on your audience's feelings, enabling you to respond proactively rather than reactively.
Another fascinating aspect of NLP is its ability to automate responses and interactions. With the integration of chatbots and virtual assistants, companies can provide real-time support to customers, answering queries and resolving issues without human intervention. This not only improves operational efficiency but also enhances the overall customer experience. Imagine chatting with a bot that understands your needs and preferences just as well as a human would—this is the future that NLP is paving.
Moreover, the applications of NLP extend beyond customer service. In industries like healthcare, NLP can sift through medical records and research papers to identify trends in patient care or emerging health issues. In finance, it can analyze news articles to predict market movements. The versatility of NLP makes it an indispensable tool for any organization looking to leverage big data effectively.
However, as powerful as NLP is, it’s important to acknowledge its limitations. Language is nuanced and context-dependent, which means that NLP systems must continuously evolve to understand idioms, slang, and cultural references. This ongoing development is crucial for ensuring that NLP remains relevant and effective in deciphering human language.
In conclusion, Natural Language Processing is revolutionizing the way businesses interact with and analyze big data. By transforming unstructured text into structured insights, NLP empowers organizations to make informed decisions, enhance customer engagement, and drive innovation. As technology continues to advance, the potential for NLP in big data analysis is boundless, promising a future where understanding human language is as intuitive as a conversation over coffee.
- What is Natural Language Processing?
NLP is a branch of artificial intelligence that focuses on the interaction between computers and humans through natural language. It enables machines to understand, interpret, and respond to human language in a valuable way.
- How does NLP benefit businesses?
NLP helps businesses analyze customer feedback, automate responses, and gain insights from unstructured data, leading to improved decision-making and enhanced customer experiences.
- What are some common applications of NLP?
Common applications include sentiment analysis, chatbots, language translation, and extracting information from large text datasets.
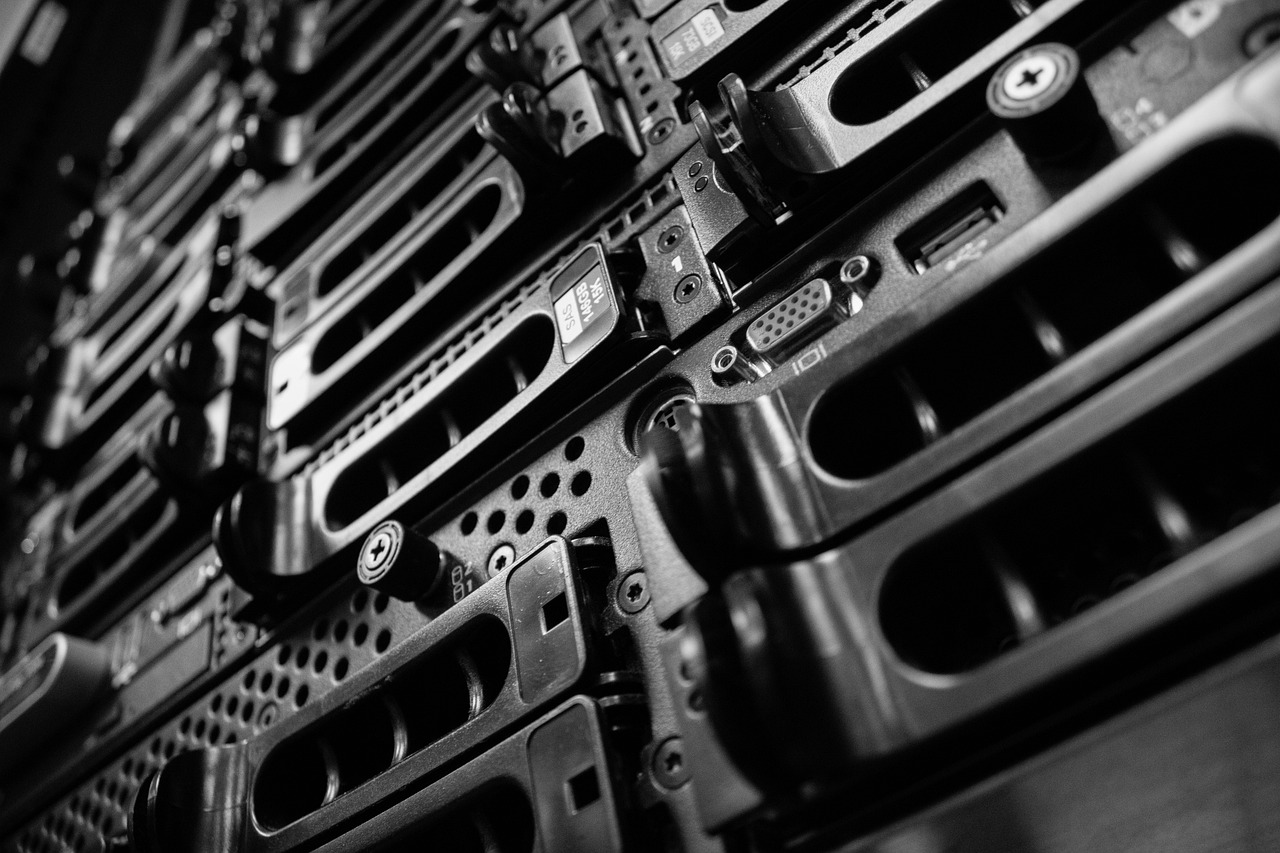
Data Visualization Enhanced by AI
In the age of information overload, where data streams in from every conceivable source, data visualization has emerged as a beacon of clarity. But what happens when you sprinkle a little artificial intelligence into the mix? The result is a powerful synergy that transforms complex data sets into visually compelling stories that are easy to digest. Imagine trying to read a dense novel versus flipping through a beautifully illustrated picture book; the latter captures your attention and communicates its message effortlessly.
AI-driven data visualization tools are revolutionizing how businesses interpret their data. These tools can analyze vast amounts of information and present it in a format that is not only visually appealing but also highly informative. By leveraging advanced algorithms, AI can identify patterns and trends that might be completely invisible to the naked eye. For instance, a retailer might use AI to visualize sales data across different regions, allowing them to quickly pinpoint areas of underperformance and adjust their strategies accordingly.
One of the most significant advantages of AI-enhanced data visualization is its ability to provide real-time insights. Organizations can monitor key performance indicators (KPIs) as they happen, enabling them to react to emerging trends and issues instantly. This capability is crucial in industries where timing is everything, such as finance, healthcare, and e-commerce. With AI, businesses can create dynamic dashboards that update in real time, offering a continuous stream of relevant information.
Industry | AI Visualization Benefits | Example Use Case |
---|---|---|
Finance | Real-time risk assessment | Monitoring stock market fluctuations |
Healthcare | Patient data analysis | Tracking patient recovery rates |
E-commerce | Sales trend analysis | Identifying peak shopping times |
Moreover, the interactivity of these visualizations allows users to dive deeper into the data. Imagine a dashboard where you can click on various elements to filter data by time, region, or demographic. This level of interaction empowers users to explore data tailored to their specific needs, leading to more informed decision-making. The ability to customize visualizations not only enhances user engagement but also fosters a culture of data-driven thinking within organizations.
In conclusion, data visualization enhanced by AI is not just a trend; it’s a necessary evolution in how we understand and utilize data. By turning raw numbers into engaging visuals, AI helps stakeholders at all levels grasp insights quickly and make decisions that can propel their organizations forward. As we continue to generate and collect more data, the importance of effective visualization will only grow, making AI an indispensable tool in the modern data landscape.
- What is AI-driven data visualization? AI-driven data visualization refers to the use of artificial intelligence technologies to create visual representations of data that are more insightful and easier to understand.
- How does AI improve data visualization? AI improves data visualization by identifying patterns, trends, and anomalies in data, and presenting them in an interactive and engaging format.
- Why is real-time data analysis important? Real-time data analysis allows organizations to respond to emerging trends and make timely decisions, which is crucial for maintaining a competitive edge.

Real-Time Data Analysis
In today's fast-paced digital landscape, has become a game changer for businesses looking to maintain a competitive edge. Imagine being able to sift through mountains of data as it streams in, identifying trends and anomalies almost instantaneously. This capability allows organizations to make decisions based on the most current information available, rather than relying on outdated reports. With the integration of artificial intelligence (AI), this process is not only faster but also more accurate, enabling companies to respond to emerging situations with remarkable agility.
Consider this: in industries like finance, healthcare, and e-commerce, the ability to analyze data in real time can mean the difference between capitalizing on a fleeting opportunity or missing out completely. For instance, a retail company can monitor customer behavior on its website and adjust its marketing strategies on the fly, ensuring that the right products are highlighted to the right customers at the right time. This is where AI’s predictive capabilities come into play, analyzing patterns and forecasting future behaviors based on current data.
Moreover, the use of AI in real-time data analysis extends beyond just monitoring trends; it also enhances operational efficiency. By automating data processing, organizations can free up valuable human resources to focus on strategic tasks rather than getting bogged down in data entry and analysis. This shift not only improves productivity but also fosters a culture of innovation, as teams can devote their time to creative problem-solving and strategic planning.
To illustrate the impact of real-time data analysis powered by AI, let’s take a look at a few key benefits:
- Immediate Insights: Organizations can make informed decisions quickly, based on the latest data.
- Proactive Decision-Making: Businesses can anticipate issues before they escalate, allowing for timely interventions.
- Enhanced Customer Experience: Companies can tailor their offerings to meet customer needs in real time, improving satisfaction and loyalty.
- Cost Efficiency: Streamlining data processes reduces operational costs and minimizes waste.
Furthermore, AI-driven tools are now equipped with sophisticated algorithms that can analyze vast datasets in seconds, providing real-time dashboards that visualize data trends and performance metrics. These interactive dashboards allow users to drill down into specific datasets, uncovering insights that are tailored to their unique business needs. As we move forward, the combination of AI and real-time data analysis will undoubtedly shape the future of decision-making across industries, empowering organizations to not only keep up with the pace of change but to thrive in it.
- What is real-time data analysis?
Real-time data analysis refers to the process of continuously inputting and analyzing data as it is generated, allowing for immediate insights and decision-making.
- How does AI enhance real-time data analysis?
AI enhances real-time data analysis by automating data processing, identifying patterns, and forecasting trends, which allows organizations to react swiftly to changes.
- What industries benefit from real-time data analysis?
Industries such as finance, healthcare, e-commerce, and logistics benefit significantly from real-time data analysis due to the need for immediate insights and quick decision-making.
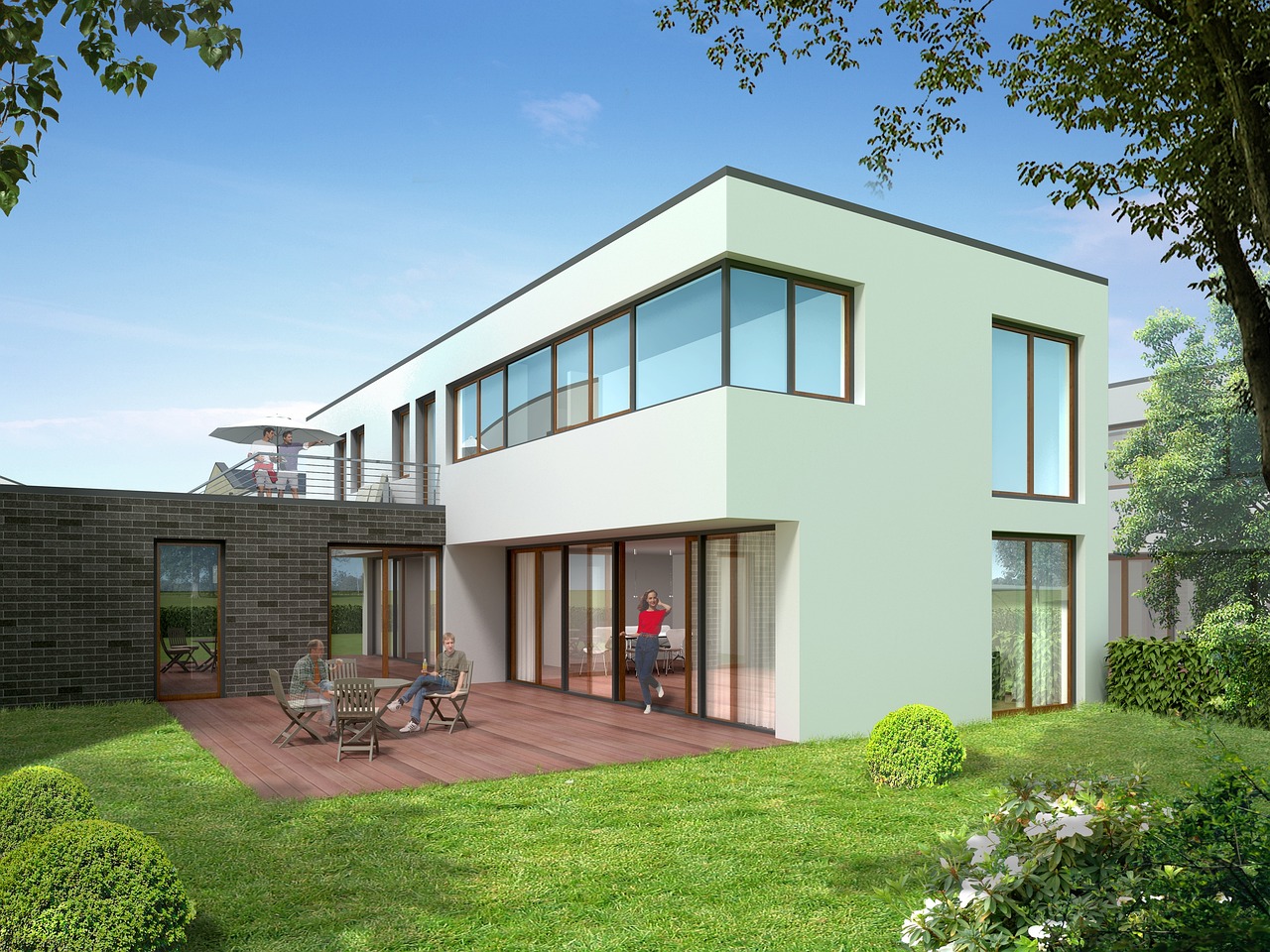
Interactive Dashboards
In today’s fast-paced business environment, making decisions based on data can feel like trying to catch smoke with your bare hands. This is where come into play, providing a tangible way to visualize and interact with complex datasets. Imagine having a command center at your fingertips, where you can manipulate data and gain insights with just a few clicks. These dashboards are not just pretty pictures; they are powerful tools that empower users to dive deep into their data.
One of the most exciting features of interactive dashboards is their ability to offer dynamic visualizations. Unlike static reports that can quickly become outdated, interactive dashboards allow users to filter, drill down, and explore data in real time. For instance, a sales manager can view overall sales performance at a glance but can also click through to see performance by region, product line, or even individual sales representatives. This level of interactivity transforms the way decisions are made, turning data from a passive collection of numbers into an engaging narrative that drives action.
Furthermore, the integration of AI technologies enhances these dashboards significantly. AI can analyze patterns and trends within the data, providing predictive insights that help businesses anticipate future outcomes. For example, an interactive dashboard could alert a marketing team about potential shifts in customer preferences based on real-time data analysis, allowing them to adjust their strategies proactively. The ability to visualize these insights means that stakeholders can grasp complex information quickly, facilitating faster and more informed decision-making.
Another key advantage of interactive dashboards is their user-friendly design. They often feature drag-and-drop functionalities, allowing users to customize their views without needing extensive technical knowledge. This democratization of data means that not just data analysts, but also marketing teams, executives, and even customer service representatives can engage with data effectively. Everyone in the organization can become a data-driven decision-maker, fostering a culture of transparency and collaboration.
To illustrate the impact of interactive dashboards, consider the following table that outlines their benefits:
Benefit | Description |
---|---|
Real-Time Insights | Access to live data enables quick responses to emerging trends. |
User Engagement | Interactive features enhance user interaction and understanding of data. |
Customization | Users can tailor dashboards to meet their specific needs and preferences. |
Collaboration | Facilitates sharing of insights across departments, promoting teamwork. |
In conclusion, interactive dashboards are revolutionizing the way businesses approach data analysis. They transform static data into engaging, actionable insights that can drive strategic decisions. As AI continues to evolve, the capabilities of these dashboards will only expand, making them an essential tool for any organization looking to thrive in the data-driven age.
- What is an interactive dashboard?
An interactive dashboard is a visual representation of data that allows users to engage with and manipulate the data in real-time for better insights. - How does AI enhance interactive dashboards?
AI analyzes data patterns and trends, providing predictive insights that help users make informed decisions quickly. - Who can use interactive dashboards?
Anyone in an organization, from data analysts to executives, can use interactive dashboards to gain insights and drive decisions. - Are interactive dashboards easy to use?
Yes, they are designed to be user-friendly, often featuring drag-and-drop functionalities for easy customization.
Frequently Asked Questions
- What is the role of AI in big data analysis?
AI plays a crucial role in big data analysis by streamlining data processing, enhancing decision-making, and uncovering patterns that might go unnoticed. It allows organizations to handle vast amounts of data efficiently, providing timely insights that drive innovation.
- How does AI improve predictive analytics?
By integrating AI with predictive analytics, organizations can forecast trends and behaviors more accurately. This combination enables data-driven decision-making, giving businesses a competitive edge by allowing them to anticipate market changes and customer needs.
- What are machine learning algorithms and why are they important?
Machine learning algorithms are essential for analyzing big data as they identify patterns and make automated predictions. They enhance the accuracy of data analysis, which is vital for organizations looking to leverage data for strategic planning.
- What is the difference between supervised and unsupervised learning?
Supervised learning involves training a model on labeled data, meaning the outcome is known, while unsupervised learning deals with unlabeled data, where the model tries to find patterns on its own. Understanding these differences helps organizations choose the right approach for their specific analysis needs.
- How does deep learning differ from traditional machine learning?
Deep learning is a subset of machine learning that uses neural networks to analyze data, particularly unstructured data like images and text. It allows for more complex pattern recognition and provides richer insights compared to traditional methods.
- What is Natural Language Processing (NLP) and its significance in big data?
NLP enables AI to understand and analyze human language, making it possible for businesses to extract valuable insights from customer feedback, social media, and other textual data. This capability is vital for improving customer experience and making informed decisions.
- How does AI enhance data visualization?
AI-driven data visualization tools transform complex datasets into easily digestible formats, allowing stakeholders to quickly grasp insights. This enhances understanding and facilitates informed decision-making based on visual data representations.
- What are interactive dashboards and how do they benefit organizations?
Interactive dashboards powered by AI provide dynamic visualizations that allow users to explore data in depth. This tailored exploration helps uncover specific insights relevant to individual needs, improving overall data analysis and decision-making processes.
- Can AI perform real-time data analysis?
Yes, AI is capable of real-time data analysis, allowing organizations to react promptly to emerging trends and issues. This responsiveness enhances operational efficiency and helps businesses stay ahead in a fast-paced market.