The Power of AI in Data Analysis
In today's rapidly evolving digital landscape, the significance of artificial intelligence (AI) in data analysis cannot be overstated. Imagine a world where data isn't just numbers and figures but a treasure trove of insights waiting to be uncovered. AI is the key that unlocks this treasure, transforming raw data into actionable intelligence that drives decision-making across various industries. This article explores the transformative impact of AI on data analysis, highlighting its capabilities, applications, and future potential.
Data analysis has traditionally been a labor-intensive process, requiring immense human effort to sift through vast amounts of information. However, with the advent of AI, this paradigm is shifting dramatically. AI algorithms can process and analyze data at lightning speed, significantly enhancing productivity and accuracy. It’s like having a supercharged assistant who never tires and can work tirelessly to find patterns and insights that might take a human analyst days or even weeks to uncover.
Moreover, AI's ability to learn and adapt is revolutionizing how businesses approach data analysis. By employing machine learning techniques, AI systems can continuously improve their performance over time, becoming more adept at identifying trends and anomalies. This capability is particularly crucial in dynamic environments where data is constantly changing. For instance, in the finance sector, AI can analyze market trends in real-time, offering traders insights that could be the difference between profit and loss.
But the power of AI in data analysis goes beyond mere speed and efficiency. It also enhances the accuracy of predictions and decisions. Traditional data analysis methods often rely on historical data and human intuition, which can be flawed. In contrast, AI leverages vast datasets to uncover hidden patterns that humans might overlook. This leads to more informed decision-making, reducing the risk of errors and increasing the likelihood of successful outcomes.
As we delve deeper into the various applications of AI in data analysis, it's essential to recognize that this technology is not just a passing trend; it's a fundamental shift in how we interact with data. Industries such as healthcare, finance, retail, and marketing are already reaping the benefits of AI-driven insights, paving the way for a future where data is not just analyzed but understood and utilized in ways we never thought possible.
In conclusion, the power of AI in data analysis is profound and far-reaching. It offers a promising glimpse into a future where data-driven decisions are not only faster but also more accurate and insightful. As we continue to explore the capabilities and applications of AI, one thing is clear: the future of data analysis is bright, and AI is at the forefront of this revolution.
- What is AI in data analysis?
AI in data analysis refers to the use of artificial intelligence technologies to analyze and interpret complex data sets, providing insights and predictions that inform decision-making. - How does machine learning fit into data analysis?
Machine learning, a subset of AI, uses algorithms to identify patterns in data, allowing systems to learn from data and improve their performance over time without explicit programming. - What industries benefit from AI in data analysis?
Industries such as finance, healthcare, retail, and marketing are leveraging AI to enhance their data analysis capabilities, leading to better decision-making and improved outcomes. - Can AI completely replace human analysts?
While AI can significantly enhance data analysis, human analysts bring critical thinking, creativity, and contextual understanding that AI cannot replicate. The best results often come from a collaboration between AI and human expertise.
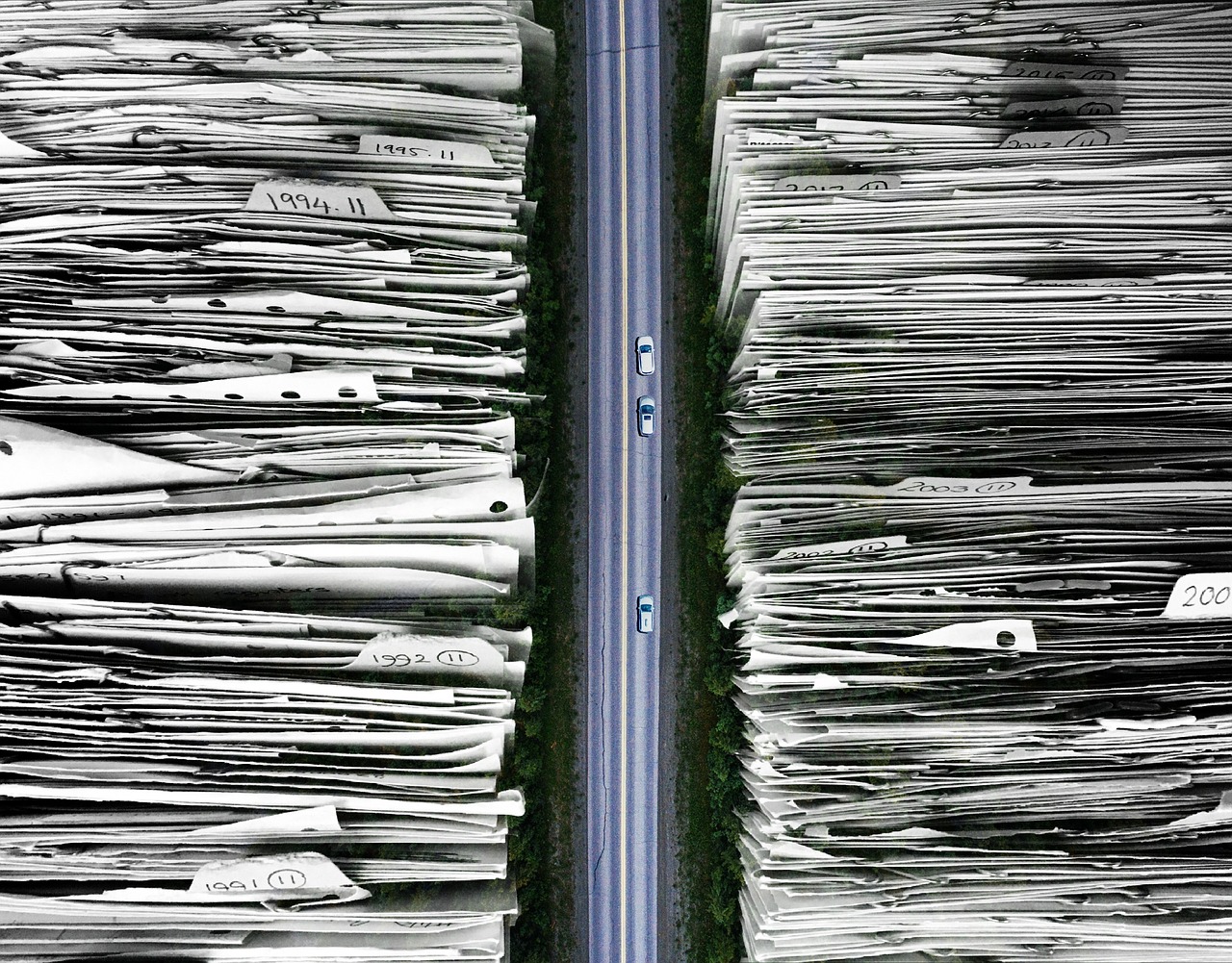
The Role of AI in Data Processing
Artificial Intelligence (AI) has emerged as a game-changer in the realm of data processing. Imagine having a tireless assistant who can sift through mountains of data faster than any human could dream of. That's what AI brings to the table! By automating mundane tasks, AI not only enhances efficiency but also improves accuracy, enabling organizations to make informed decisions based on reliable data.
One of the most significant advantages of AI in data processing is its ability to streamline data management. Traditional methods often involve labor-intensive processes that can lead to human errors and inconsistencies. In contrast, AI algorithms can quickly analyze vast datasets, identify relevant information, and extract insights without the fatigue that comes with manual processing. This automation allows businesses to focus on strategic initiatives rather than getting bogged down by data entry and management tasks.
Furthermore, AI's capability to improve accuracy cannot be overstated. Machine learning algorithms can learn from historical data, continuously refining their predictions and analyses. For instance, in a financial institution, AI can analyze transaction data to detect anomalies and flag potential fraud, significantly reducing the risk of financial loss. This level of precision is crucial in industries where decisions must be based on accurate data interpretations.
Moreover, AI enhances the speed of data analysis. In today’s fast-paced world, the ability to quickly process and analyze data can be the difference between success and failure. AI systems can perform complex calculations and data assessments in a fraction of the time it would take a human analyst. This speed enables companies to respond to market changes swiftly, adapting their strategies based on real-time insights.
To illustrate the transformative impact of AI on data processing, consider the following table that highlights key benefits:
Benefit | Description |
---|---|
Automation | Reduces manual workload, allowing teams to focus on strategic tasks. |
Improved Accuracy | Minimizes human errors, leading to more reliable data interpretations. |
Speed | Processes large datasets rapidly, enabling quick decision-making. |
Scalability | Easily adapts to increasing data volumes without compromising performance. |
As we delve deeper into the capabilities of AI in data processing, it becomes clear that its role is not just about automating tasks but also about enhancing the entire data ecosystem. With the right AI tools, businesses can harness their data's full potential, transforming raw information into actionable insights that drive growth and innovation.
In conclusion, the integration of AI in data processing is revolutionizing how organizations manage and utilize data. By automating processes, improving accuracy, and speeding up analysis, AI is paving the way for smarter decision-making and more efficient operations. As we continue to explore the potential of AI, the future of data analysis looks incredibly promising.
- What is AI in data processing? AI in data processing refers to the use of artificial intelligence technologies to automate and enhance the analysis and management of data.
- How does AI improve data accuracy? AI improves data accuracy by learning from historical data and minimizing human errors through automated processes.
- Can AI help in real-time data analysis? Yes, AI can process and analyze data in real-time, allowing businesses to make quick decisions based on current information.
- What industries benefit from AI in data processing? Industries such as finance, healthcare, marketing, and retail benefit significantly from AI in data processing.
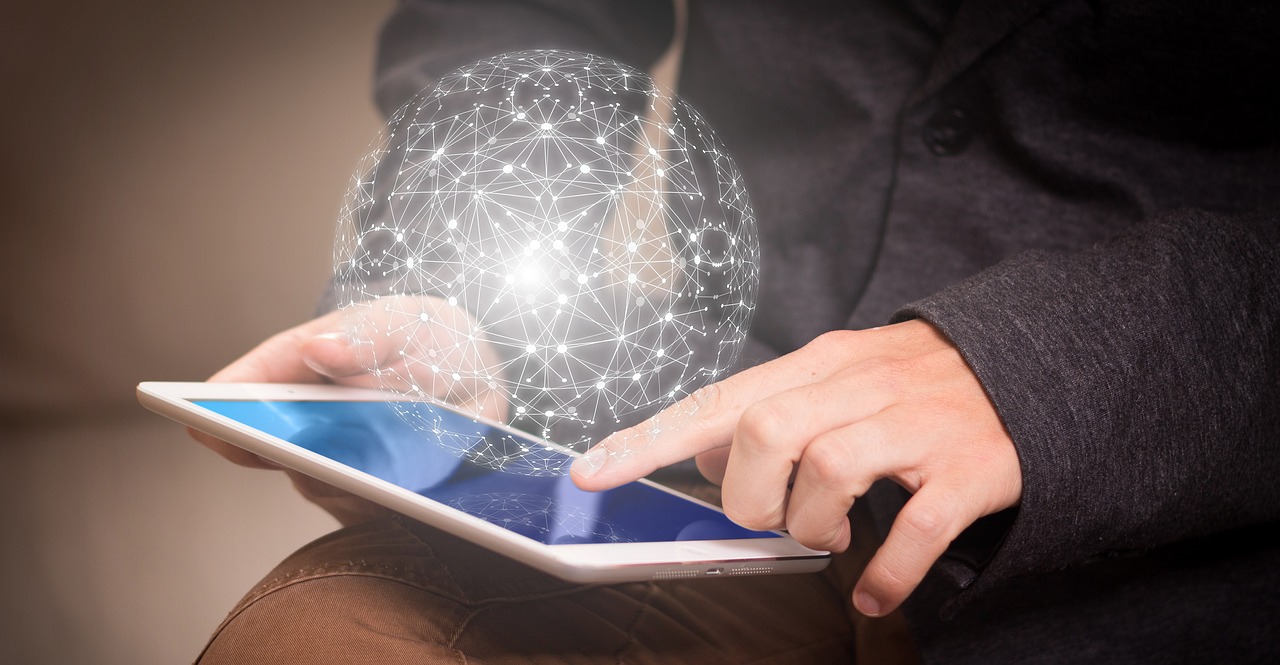
Machine Learning Techniques in Data Analysis
When we talk about the incredible world of data analysis, one cannot overlook the role of machine learning. This fascinating subset of artificial intelligence is like having a super-smart assistant that can sift through mountains of data, uncovering patterns and insights that would take humans ages to find. Imagine having a magnifying glass that not only highlights the details but also predicts what might happen next; that’s machine learning for you! It employs sophisticated algorithms to analyze data, making it a game-changer in various fields, from finance to healthcare.
Machine learning techniques can be broadly categorized into two main types: supervised learning and unsupervised learning. Each of these techniques has its own unique applications and benefits, making them invaluable tools in the data analyst's toolkit. With supervised learning, for instance, we train models on labeled datasets, allowing them to make predictions based on new, unseen data. This is akin to teaching a child to recognize animals by showing them pictures and naming each one. Over time, the child learns to identify dogs, cats, and birds on their own. Similarly, supervised learning algorithms improve their accuracy through exposure to more data.
On the flip side, we have unsupervised learning, which dives into unlabeled data, seeking out hidden structures and patterns without any prior guidance. Think of it as exploring a vast, uncharted territory where you have no map. The thrill comes from discovering new paths and connections that you never knew existed! Techniques such as clustering and association fall under this category, allowing data analysts to segment data into meaningful groups or identify relationships between different variables.
To further illustrate the importance of these techniques, let’s look at some real-world applications:
Technique | Application | Industry |
---|---|---|
Supervised Learning | Credit scoring | Finance |
Unsupervised Learning | Customer segmentation | Retail |
Supervised Learning | Medical diagnosis | Healthcare |
Unsupervised Learning | Market basket analysis | Marketing |
As we can see from the table above, supervised learning is immensely useful in scenarios where prediction and classification are crucial. For instance, in finance, it helps in assessing the creditworthiness of applicants by analyzing historical data. On the other hand, unsupervised learning shines in situations where we want to explore data without pre-defined categories, such as identifying customer segments in retail. This can lead to more personalized marketing strategies, ultimately boosting sales.
However, it's essential to understand that each technique comes with its own set of challenges. Supervised learning, while powerful, can suffer from issues like overfitting, where the model becomes too tailored to the training data and fails to generalize well to new data. Unsupervised learning, too, can be tricky, as the lack of labeled data means we might misinterpret the patterns we uncover. But fear not! With the right strategies and tools, these challenges can be managed effectively.
In conclusion, machine learning techniques are revolutionizing the field of data analysis, providing us with the tools to make informed decisions based on data-driven insights. Whether through supervised or unsupervised learning, these techniques empower businesses to enhance their operations, improve customer experiences, and ultimately drive innovation.
- What is machine learning? - Machine learning is a subset of artificial intelligence that uses algorithms to analyze and learn from data, making predictions or decisions without explicit programming.
- How does supervised learning differ from unsupervised learning? - Supervised learning uses labeled datasets to train models for predictions, while unsupervised learning analyzes unlabeled data to uncover hidden patterns.
- What are some common applications of machine learning? - Common applications include credit scoring in finance, customer segmentation in retail, and medical diagnosis in healthcare.
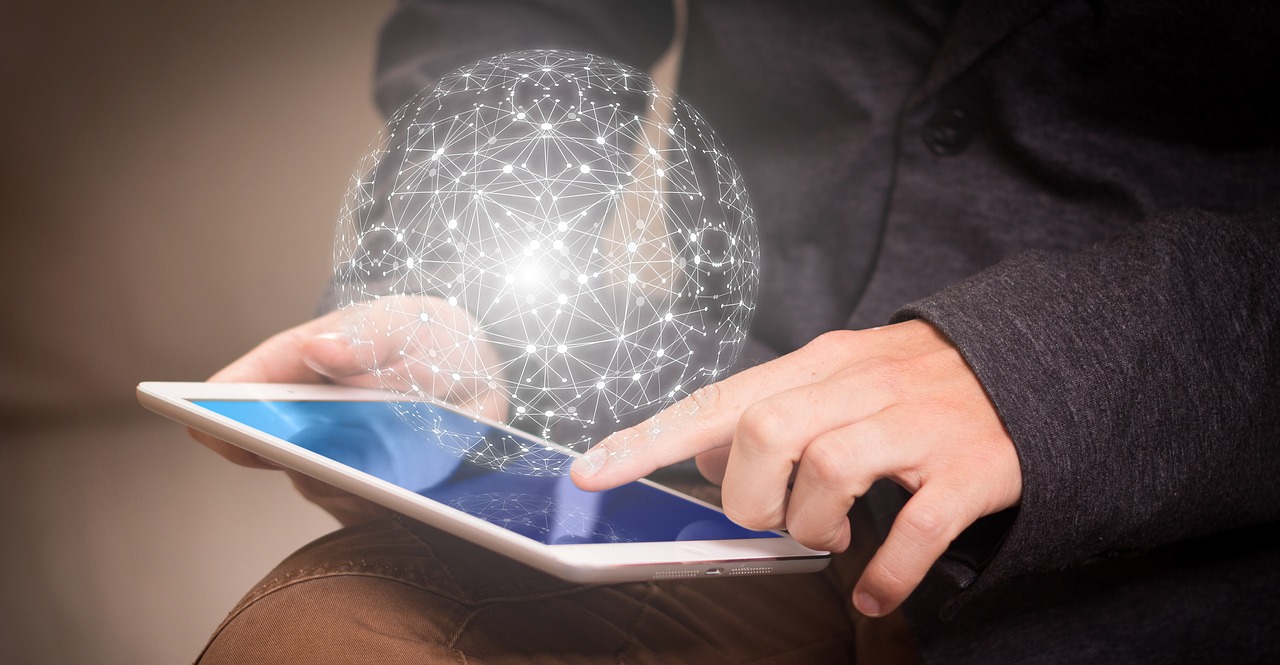
Supervised Learning
Supervised learning is like having a wise mentor guiding you through the complexities of data analysis. In this approach, we train algorithms on labeled datasets, which means that every piece of data comes with the answer key. Imagine teaching a child to recognize different types of fruits by showing them pictures of apples, bananas, and oranges, along with their names. This method allows the algorithm to learn from the examples provided, enabling it to make accurate predictions when faced with new, unseen data.
The importance of supervised learning cannot be overstated. It plays a critical role in various industries, providing the backbone for many predictive models that drive decision-making processes. For instance, in finance, supervised learning algorithms analyze past market data to predict stock prices or assess credit risks. In the healthcare sector, they help in diagnosing diseases by analyzing patient data and identifying patterns that correlate with specific health outcomes. The precision and reliability of these predictions are vital, as they can significantly impact people's lives and business operations.
However, it’s not all sunshine and rainbows in the world of supervised learning. There are challenges that practitioners face, such as overfitting. This occurs when a model learns the training data too well, including its noise and outliers, leading to poor performance on new data. Imagine a student who memorizes answers rather than understanding the material; they might ace the test but struggle with real-world applications. To combat this, techniques such as cross-validation and regularization are employed to ensure that the models generalize well to unseen data.
Data quality is another significant hurdle. If the training data is flawed or biased, the model's predictions will reflect those issues, leading to inaccurate outcomes. Therefore, it is crucial to ensure that the data used for training is clean and representative of the real-world scenarios it aims to predict. In this context, data pre-processing techniques, such as normalization and handling missing values, become essential steps in the supervised learning pipeline.
In summary, supervised learning is a powerful tool in the data analyst's toolkit, providing the means to make informed predictions based on historical data. Its applications span across various fields, making it a versatile and essential technique in the realm of artificial intelligence. As we continue to refine our data collection and model training processes, the potential for supervised learning to transform industries and improve decision-making will only grow.
- What is supervised learning? Supervised learning is a type of machine learning where algorithms are trained on labeled datasets to make predictions.
- How does supervised learning differ from unsupervised learning? Unlike supervised learning, unsupervised learning works with unlabeled data to find hidden patterns without pre-existing labels.
- What are some common applications of supervised learning? Common applications include stock price prediction in finance, disease diagnosis in healthcare, and customer segmentation in marketing.
- What challenges does supervised learning face? Challenges include overfitting, data quality issues, and the need for large amounts of labeled data.
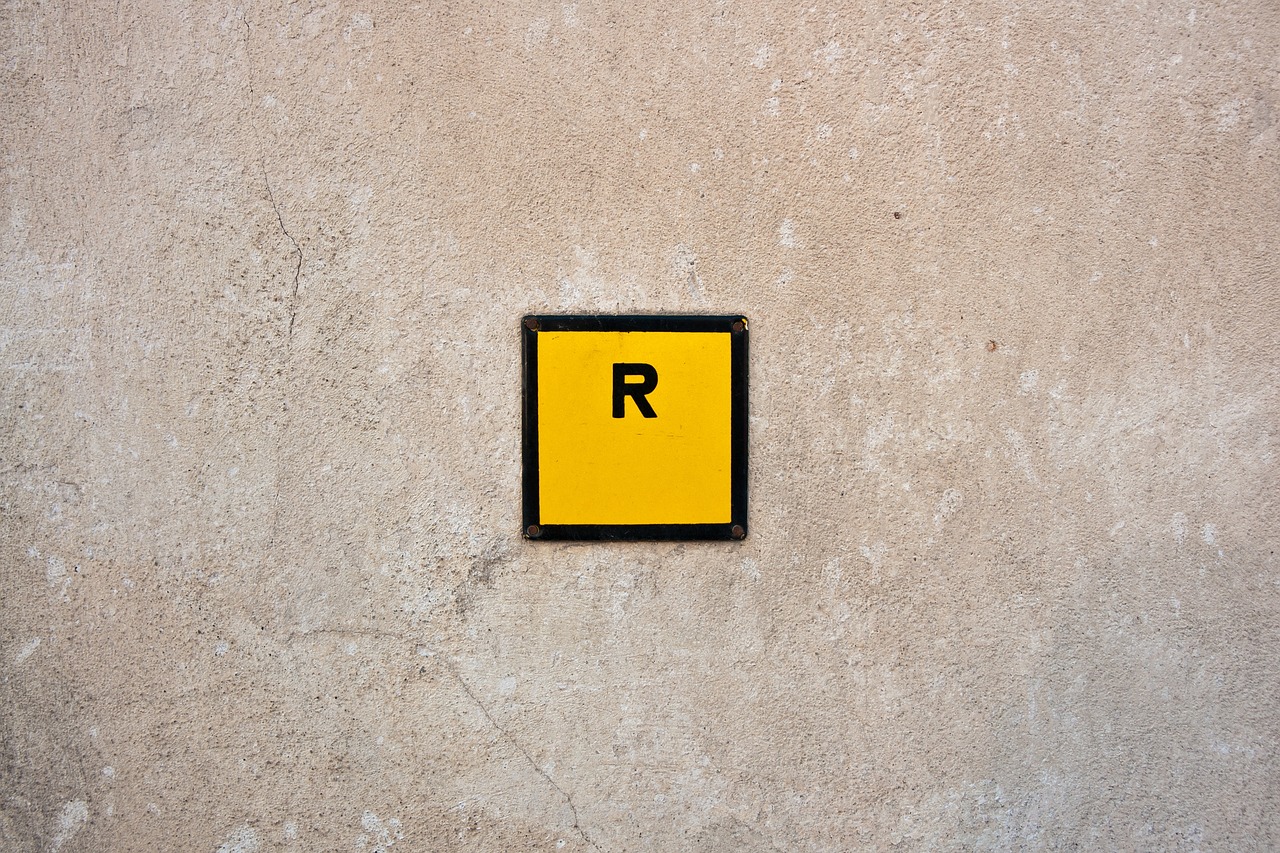
Applications of Supervised Learning
Supervised learning is a powerhouse in the realm of data analysis, offering a plethora of applications across various industries. It operates on the principle of training algorithms using labeled datasets, which allows these models to make predictions or classifications based on new, unseen data. Imagine having a personal assistant who learns your preferences over time and then makes recommendations tailored just for you; that's essentially what supervised learning does for data.
In the finance sector, for example, supervised learning is leveraged to predict stock prices and assess credit risks. By analyzing historical data and identifying patterns, financial institutions can make informed decisions, mitigating risks and maximizing returns. For instance, algorithms can be trained to recognize indicators of potential loan defaults, enabling banks to make more reliable lending decisions.
Similarly, in the healthcare industry, supervised learning plays a critical role in diagnosing diseases. Machine learning models are trained on medical imaging data, such as X-rays and MRIs, to detect anomalies like tumors. This application not only speeds up the diagnostic process but also enhances accuracy, ultimately leading to better patient outcomes. One notable success story is the use of AI in detecting diabetic retinopathy, where models have achieved performance levels comparable to expert ophthalmologists.
Moreover, supervised learning is instrumental in marketing. Companies utilize it to analyze customer behavior and predict future purchasing patterns. By segmenting customers based on their past interactions, businesses can tailor marketing campaigns that resonate with specific audiences. This not only boosts engagement but also enhances conversion rates. For instance, a retailer might use supervised learning to identify which customers are likely to respond to a particular promotion, thereby optimizing their marketing strategy.
However, it's important to note that while supervised learning offers remarkable benefits, it also comes with its own set of challenges. The quality of the labeled data is crucial; if the data is biased or inaccurate, the predictions will be flawed. Additionally, models can suffer from overfitting, where they perform well on training data but fail to generalize to new data. This highlights the need for robust data preparation and validation techniques.
In summary, the applications of supervised learning are vast and varied, spanning finance, healthcare, marketing, and beyond. As industries continue to embrace data-driven decision-making, the role of supervised learning will only become more prominent, driving innovation and efficiency in ways we are just beginning to understand.
- What is supervised learning? Supervised learning is a type of machine learning where algorithms are trained on labeled datasets to make predictions or classifications.
- How is supervised learning used in healthcare? It is used to analyze medical images and predict disease outcomes, improving diagnostic accuracy and patient care.
- What are the challenges of supervised learning? Challenges include data quality issues and the risk of overfitting, which can lead to poor generalization on new data.
- Can supervised learning be applied in marketing? Absolutely! It helps businesses analyze customer behavior and tailor marketing strategies for better engagement.
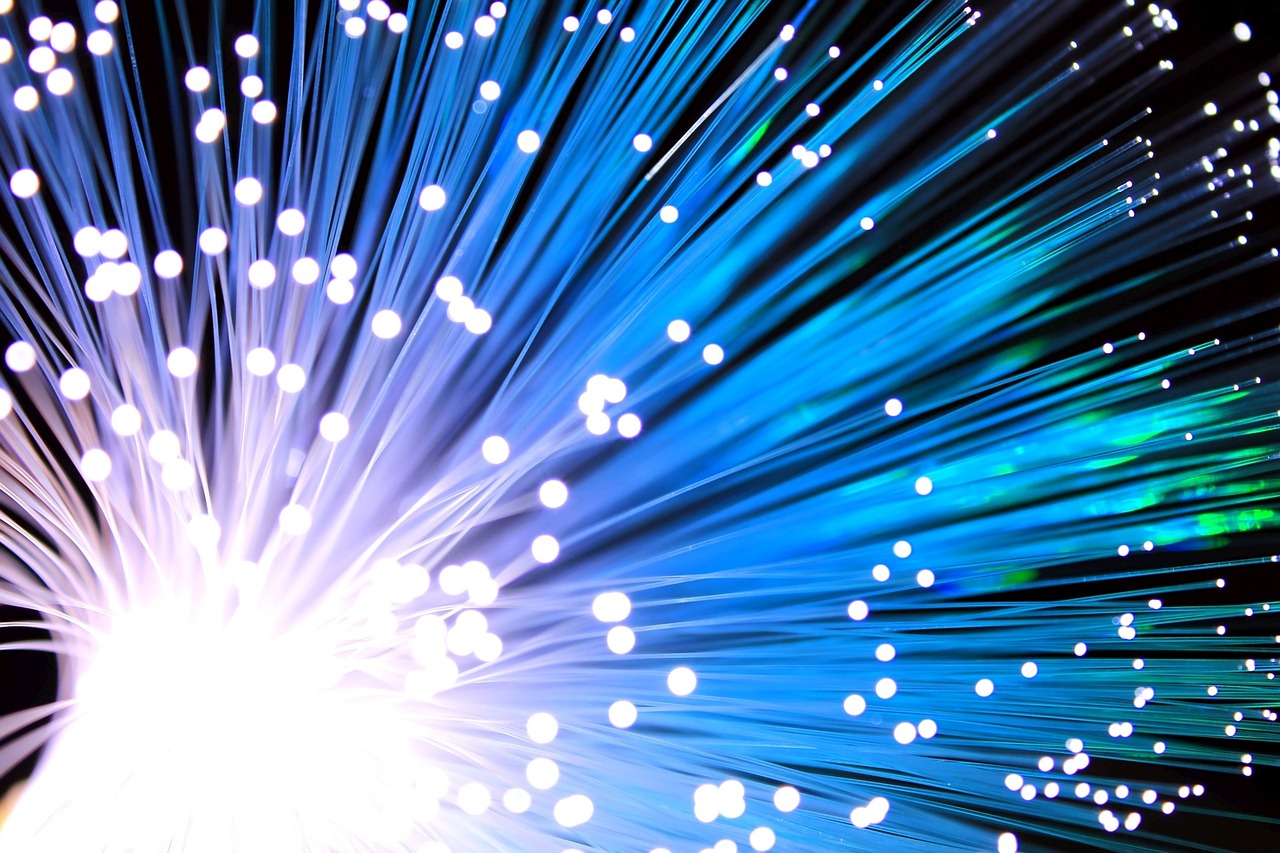
Challenges in Supervised Learning
Even though supervised learning has proven to be a game-changer in the realm of data analysis, it doesn't come without its fair share of challenges. One of the most significant hurdles is overfitting. This occurs when a model learns the training data too well, capturing noise and outliers rather than the underlying pattern. Imagine trying to memorize every detail of a story instead of understanding its main theme — that's what overfitting does to a model. It performs exceptionally well on the training data but falters when faced with new, unseen data.
Another challenge is the quality of the data itself. Supervised learning relies heavily on labeled datasets, and if the data is incomplete or biased, the model's predictions can be skewed. For instance, if a financial model is trained on data that predominantly features one demographic, it may not perform well for others. This issue raises the question: how can we ensure that our training data is both comprehensive and representative?
Moreover, the process of labeling data can be time-consuming and labor-intensive. It often requires domain expertise, which can lead to a bottleneck in the development of machine learning models. To illustrate, consider the medical field: labeling medical images accurately requires trained professionals, and their time is a scarce resource. This scarcity can delay the deployment of potentially life-saving models.
Lastly, there's the challenge of scalability. As datasets grow in size and complexity, the computational resources required to train models can become overwhelming. This can lead to increased costs and longer processing times, which may not be feasible for many organizations. In a world that demands quick insights, this can be a significant disadvantage.
In summary, while supervised learning offers powerful tools for data analysis, practitioners must navigate several challenges, including overfitting, data quality issues, the labor-intensive nature of data labeling, and scalability concerns. Addressing these challenges is essential for harnessing the full potential of supervised learning in various industries.
- What is supervised learning? Supervised learning is a type of machine learning where models are trained on labeled datasets to make predictions on new, unseen data.
- What are the main challenges of supervised learning? The main challenges include overfitting, data quality issues, the labor-intensive nature of labeling data, and scalability concerns.
- How can overfitting be prevented? Overfitting can be prevented by using techniques such as cross-validation, pruning, and regularization.
- Why is data quality important in supervised learning? High-quality data ensures that the model learns accurate patterns, leading to better predictions and insights.
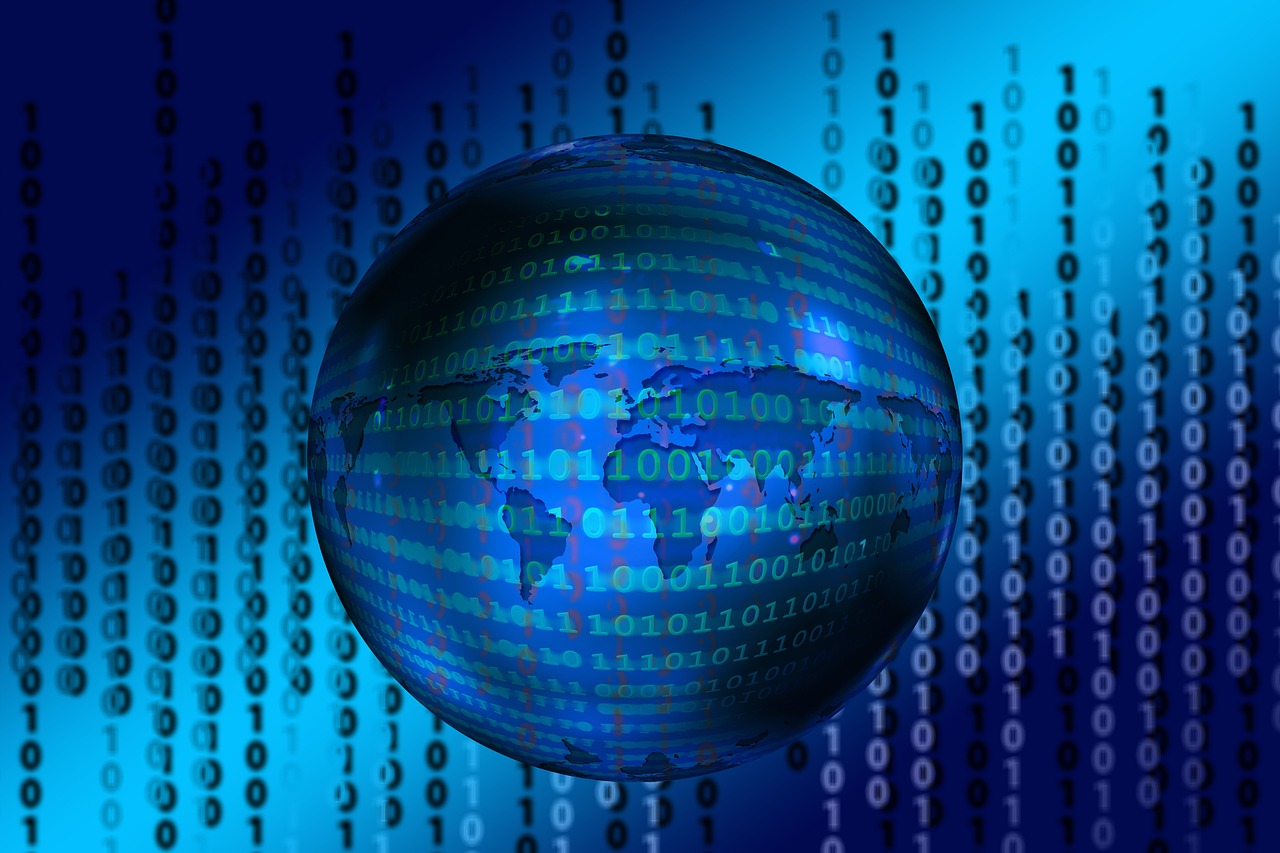
Unsupervised Learning
Unsupervised learning is a fascinating branch of machine learning that focuses on analyzing unlabeled data to uncover hidden patterns, relationships, and structures. Unlike supervised learning, which relies on labeled datasets to train models, unsupervised learning dives into the unknown, making it a powerful tool for data exploration. Imagine wandering through a vast library without any cataloging system; unsupervised learning helps us organize the chaos, revealing insightful connections among the books—much like how it identifies clusters and associations in data.
This technique is particularly valuable in scenarios where data labeling is either impractical or too costly. For instance, consider a company with millions of customer interactions. Manually tagging each interaction would be a monumental task, but unsupervised learning can analyze these interactions to identify customer segments based on behavior, preferences, and purchasing patterns. This allows businesses to tailor their marketing strategies more effectively, ultimately enhancing customer satisfaction and loyalty.
There are several techniques within unsupervised learning, each serving unique purposes:
- Clustering: This technique groups similar data points together. For example, a retail company might use clustering to segment customers into distinct groups based on purchasing behavior, enabling targeted marketing campaigns.
- Dimensionality Reduction: This process simplifies datasets by reducing the number of variables, making it easier to visualize and analyze. Techniques like PCA (Principal Component Analysis) help in compressing data while retaining essential information.
- Anomaly Detection: Unsupervised learning can also identify outliers in data, which is crucial for fraud detection in finance or monitoring equipment health in manufacturing.
One of the most exciting aspects of unsupervised learning is its ability to adapt and evolve. As more data becomes available, these algorithms can refine their understanding of the underlying patterns, leading to more accurate predictions and insights. This adaptability is vital in today’s fast-paced world, where data is constantly being generated.
In summary, unsupervised learning opens the door to a wealth of possibilities in data analysis. By harnessing the power of algorithms to explore uncharted territories within datasets, organizations can uncover valuable insights that drive decision-making and innovation. As we continue to embrace the digital age, the significance of unsupervised learning will only grow, paving the way for smarter, data-driven strategies across various industries.
- What is the main difference between supervised and unsupervised learning?
Supervised learning uses labeled data for training, while unsupervised learning deals with unlabeled data to find hidden patterns. - Can unsupervised learning be used for predictive analytics?
Yes, while unsupervised learning primarily focuses on pattern discovery, the insights gained can inform predictive models by identifying trends and groupings. - What are some common applications of unsupervised learning?
Common applications include customer segmentation, market basket analysis, and anomaly detection in various fields like finance and healthcare.
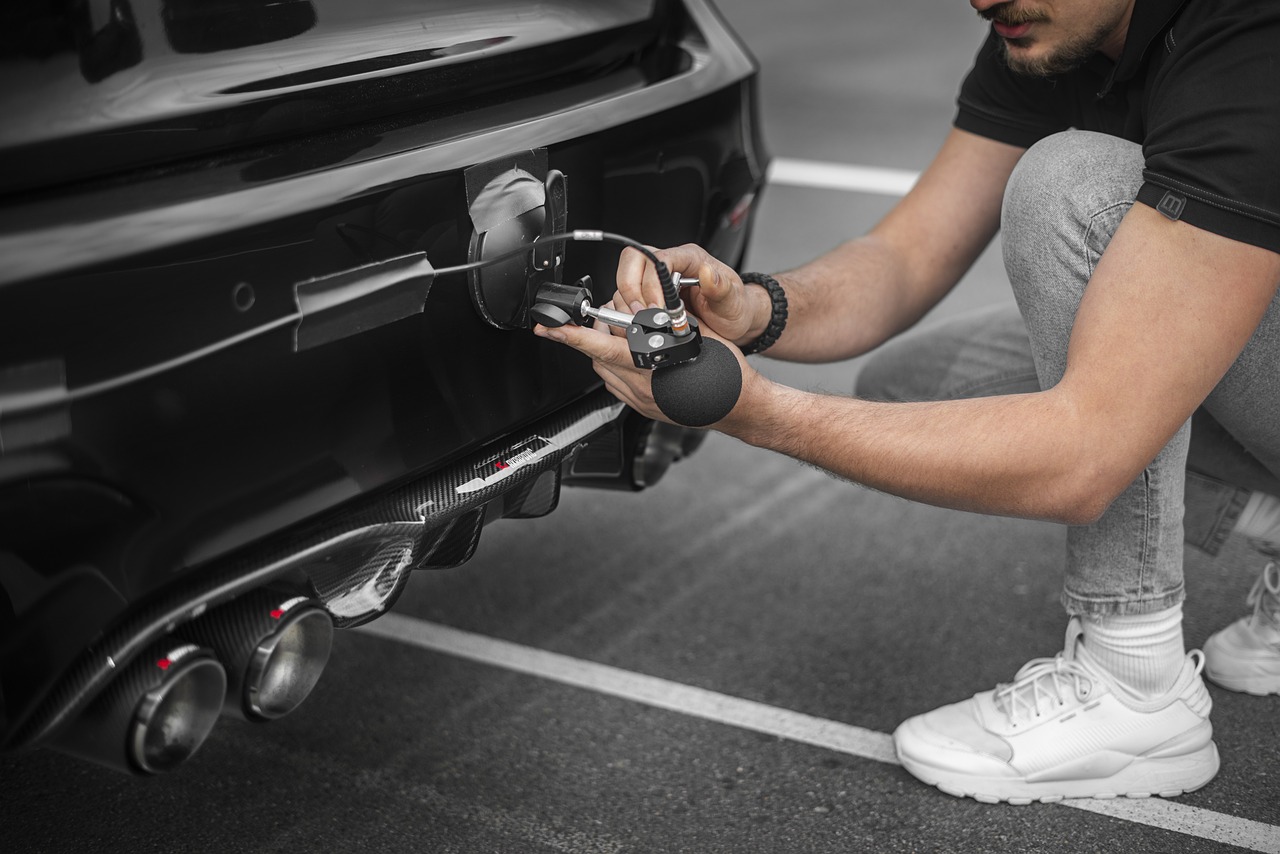
AI Tools and Technologies for Data Analysis
In today's fast-paced world, the sheer volume of data generated daily is staggering. Businesses and organizations are inundated with information, and to make sense of it all, they increasingly rely on AI tools and technologies. These tools not only help in processing vast amounts of data but also empower decision-makers to derive actionable insights swiftly. Imagine trying to find a needle in a haystack; AI technologies act as powerful magnets that pull out the relevant data points, allowing analysts to focus on what truly matters.
One of the key advantages of AI in data analysis is its ability to automate mundane tasks. For instance, data cleaning, which can be a tedious process, is significantly streamlined using AI algorithms. These algorithms can identify and correct errors in datasets, ensuring that the data is accurate and reliable. This not only saves time but also enhances the overall quality of the analysis. In essence, AI tools act as the diligent assistants that every data analyst dreams of, tirelessly working behind the scenes to ensure everything is in order.
Moreover, there are several AI tools specifically designed for different aspects of data analysis. Below are some popular categories:
- Data Visualization Tools: These tools transform complex data sets into visual formats, making it easier for stakeholders to understand trends and patterns. Tools like Tableau and Power BI leverage AI to enhance visualization capabilities, enabling users to create interactive dashboards that tell compelling stories with data.
- Predictive Analytics Platforms: These platforms utilize machine learning algorithms to analyze historical data and predict future outcomes. For example, platforms like IBM Watson and Google Cloud AI can forecast customer behavior, helping businesses tailor their strategies effectively.
- Natural Language Processing (NLP) Tools: NLP tools enable users to analyze and interpret human language data. This is particularly useful in sentiment analysis, where businesses can gauge public opinion about their products or services by analyzing social media data.
As we delve deeper into the realm of AI tools, it's essential to recognize how they integrate into the decision-making processes of various industries. For instance, in the healthcare sector, AI tools can analyze patient data to identify risk factors, leading to proactive healthcare measures. Similarly, in finance, predictive analytics can help in detecting fraudulent transactions before they occur, safeguarding assets and building trust with customers.
Furthermore, the future of AI in data analysis looks promising. With advancements in technology, we can expect more sophisticated tools that will not only enhance data processing but also provide deeper insights through advanced algorithms. Imagine a world where AI can predict market trends with pinpoint accuracy or where healthcare providers can foresee outbreaks before they happen. The possibilities are endless, and the impact of these technologies on data analysis will be profound.
In conclusion, AI tools and technologies are revolutionizing the way we approach data analysis. They not only increase efficiency but also empower organizations to make data-driven decisions with confidence. As we continue to explore these innovations, it’s crucial to stay informed and adapt to the changing landscape of data analysis.
1. What are the main benefits of using AI tools in data analysis?
AI tools enhance efficiency, improve accuracy, and allow for real-time data processing. They automate repetitive tasks, enabling analysts to focus on strategic decision-making.
2. Can AI tools replace human analysts?
While AI tools significantly augment the capabilities of human analysts, they are not a complete replacement. Human intuition and contextual understanding remain vital in interpreting data insights.
3. What industries benefit the most from AI in data analysis?
Industries such as healthcare, finance, marketing, and retail are among those that benefit immensely from AI tools, as they rely heavily on data-driven insights for decision-making.
4. Are there any challenges associated with implementing AI tools?
Yes, challenges include data privacy concerns, the need for quality data, and potential resistance from employees who may fear job displacement.
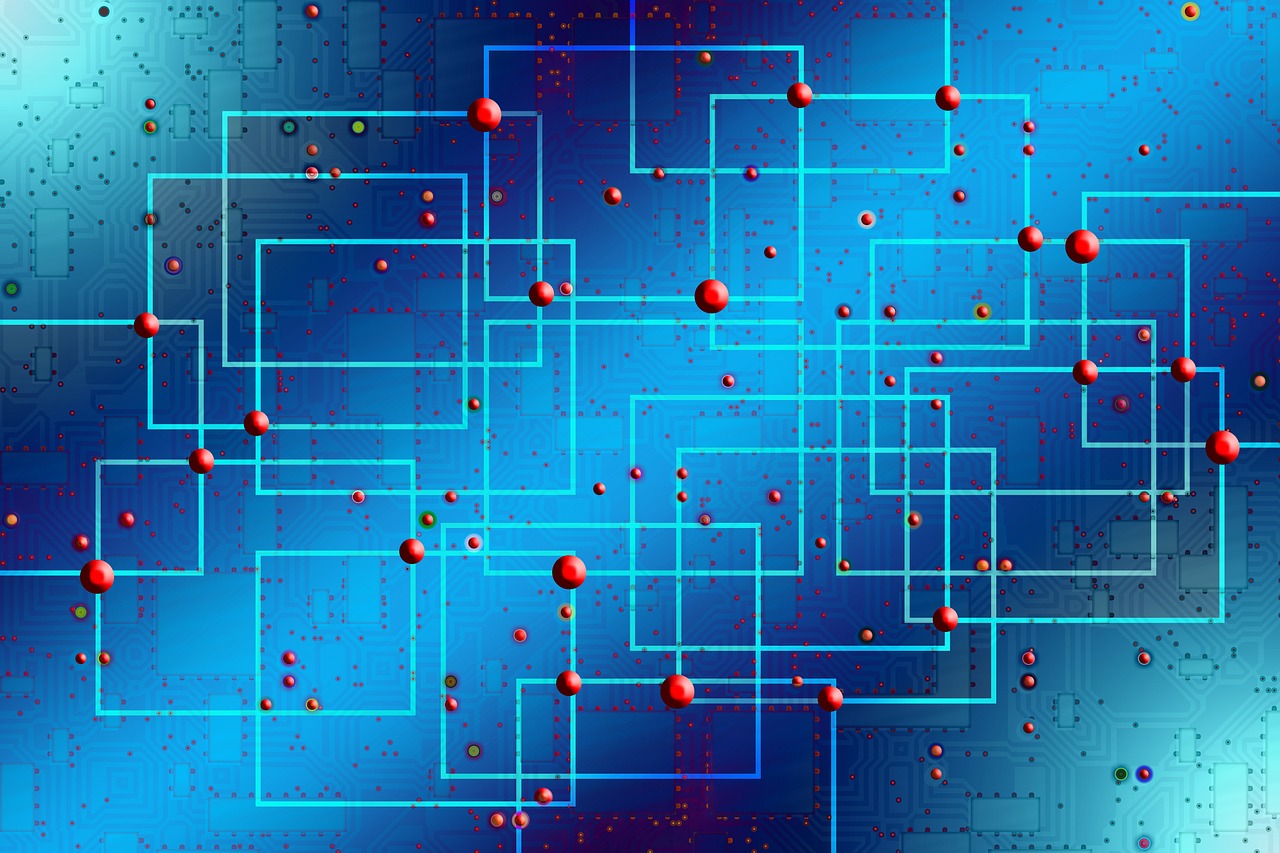
Data Visualization Tools
In today's data-driven world, the ability to effectively communicate insights is more crucial than ever. powered by artificial intelligence (AI) play a pivotal role in transforming complex datasets into easily digestible visuals. Imagine trying to make sense of a mountain of numbers and statistics without a clear way to visualize them—frustrating, right? That’s where these tools come into play, allowing users to see patterns, trends, and anomalies at a glance.
These tools utilize advanced algorithms to create dynamic and interactive visualizations that not only capture attention but also enhance understanding. For instance, rather than presenting data in a dull spreadsheet format, AI-driven visualization tools can generate stunning graphs, heat maps, and dashboards that bring data to life. This transformation is akin to turning a black-and-white photograph into a vibrant, colorful image; it makes the information much more engaging and easier to interpret.
One of the standout features of modern data visualization tools is their ability to incorporate real-time data. This means that businesses can make informed decisions based on the latest information, rather than relying on outdated reports. Consider a retail company monitoring sales data; with real-time visualizations, they can quickly identify which products are flying off the shelves and which are lagging behind. This agility can be the difference between seizing a market opportunity and missing it entirely.
Moreover, many of these tools come equipped with customization options that allow users to tailor their visualizations to specific audiences. Whether it’s for a board meeting, a marketing presentation, or a report to stakeholders, the ability to adjust the complexity and style of the visualizations ensures that the right message is conveyed effectively. Users can choose from various chart types, colors, and layouts to suit their needs.
To give you a clearer picture, here’s a quick comparison of some popular data visualization tools:
Tool Name | Key Features | Best For |
---|---|---|
Tableau | Interactive dashboards, real-time analytics | Businesses needing in-depth analysis |
Power BI | Integration with Microsoft products, user-friendly interface | Organizations using Microsoft ecosystem |
Google Data Studio | Collaboration features, free to use | Small businesses and startups |
QlikView | Associative data model, powerful analytics | Companies needing complex data analysis |
As we move forward, the impact of AI on data visualization is only expected to grow. The integration of machine learning algorithms into these tools means that they can learn from user interactions and improve over time, making them smarter and more effective. This continuous evolution ensures that businesses can stay ahead of the curve, adapting to changes in data and market conditions.
In conclusion, data visualization tools are not just about making data pretty; they are essential for turning raw data into actionable insights. By leveraging the power of AI, these tools empower organizations to make informed decisions, enhance communication, and ultimately drive success. So, the next time you find yourself overwhelmed by numbers, remember that a well-crafted visual can make all the difference.
- What are data visualization tools?
Data visualization tools are software applications that allow users to create visual representations of data, making it easier to understand and analyze.
- Why is data visualization important?
Data visualization is important because it helps to communicate complex data insights clearly and effectively, enabling better decision-making.
- Can I use data visualization tools for free?
Yes, some data visualization tools, like Google Data Studio, offer free versions with limited features, while others may require a subscription for full functionality.
- How can AI improve data visualization?
AI can enhance data visualization by automating the creation of visuals, providing real-time updates, and personalizing visualizations based on user preferences.
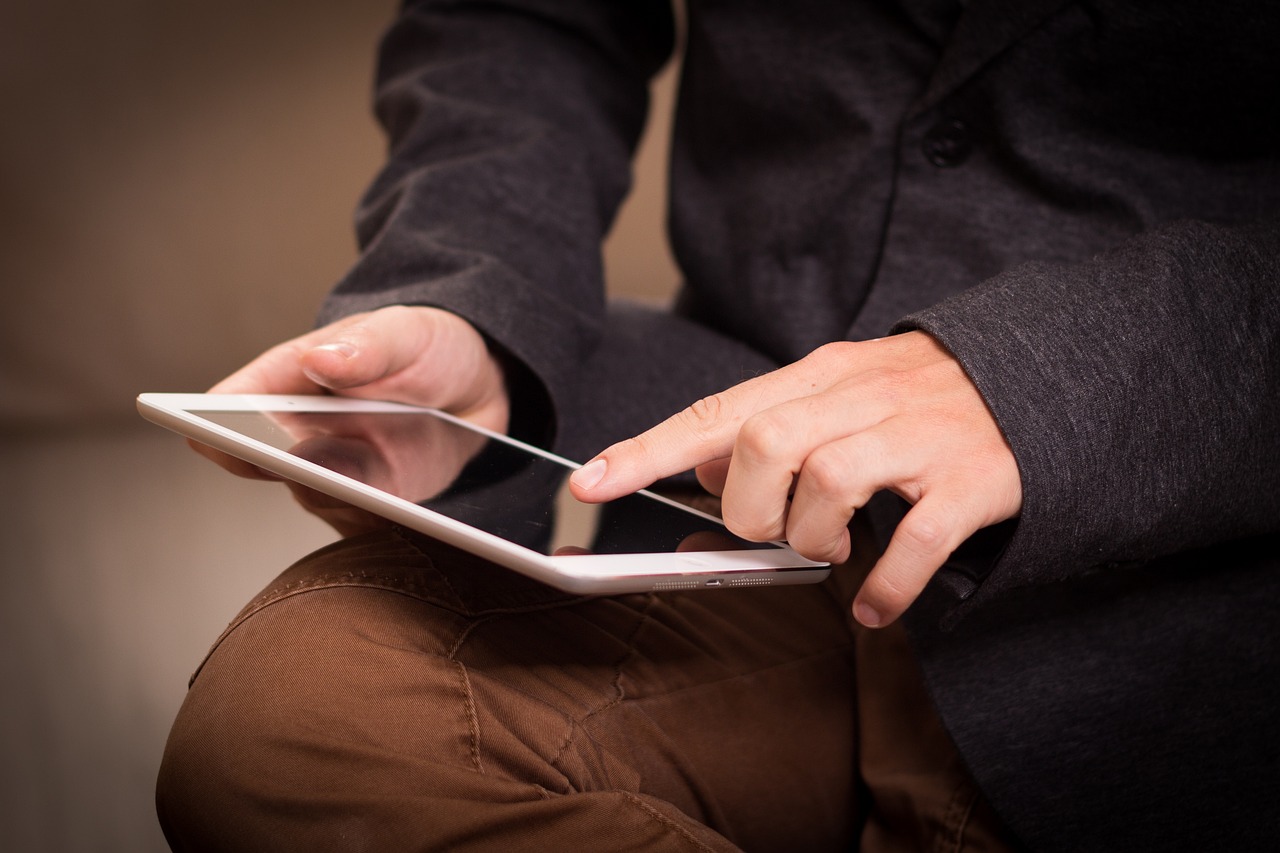
Predictive Analytics Platforms
Predictive analytics platforms have revolutionized the way businesses forecast trends and behaviors. By harnessing the power of artificial intelligence and advanced statistical techniques, these platforms enable organizations to make data-driven decisions with unprecedented accuracy. Imagine being able to predict customer behavior before it even happens—this is the magic of predictive analytics!
At their core, predictive analytics platforms utilize historical data to identify patterns and trends that inform future outcomes. This process is akin to having a crystal ball that not only shows you what might happen but also provides insights into why it’s likely to occur. For instance, a retail company can analyze past sales data to forecast future demand for products, ensuring they stock up on items that are trending. This not only boosts sales but also enhances customer satisfaction by minimizing stockouts.
One of the key functionalities of these platforms is their ability to integrate various data sources. Businesses today collect data from numerous channels, including social media, customer interactions, and sales transactions. Predictive analytics platforms can pull together this diverse data, providing a comprehensive view of the factors influencing business performance. The result? Enhanced accuracy in predictions and more informed decision-making.
Moreover, predictive analytics platforms are not just for large corporations; small and medium-sized enterprises (SMEs) can also leverage these tools to gain a competitive edge. With user-friendly interfaces and scalable solutions, even non-technical users can harness the power of predictive analytics. This democratization of data insights means that businesses of all sizes can benefit from the foresight these platforms provide.
To give you a clearer picture of how predictive analytics platforms function, here’s a brief overview of their key components:
Component | Description |
---|---|
Data Collection | Gathering data from various sources to create a comprehensive dataset. |
Data Processing | Cleaning and transforming data to ensure accuracy and usability. |
Modeling | Applying statistical algorithms to predict future outcomes based on historical data. |
Visualization | Presenting data in an understandable format to facilitate decision-making. |
As industries continue to evolve, the impact of predictive analytics will only grow. From healthcare predicting patient admissions to finance forecasting market trends, the applications are vast and varied. Companies that embrace these platforms will not only stay ahead of the competition but also cultivate a culture of innovation and agility.
In summary, predictive analytics platforms are game-changers in the realm of data analysis. They empower organizations to anticipate changes, optimize operations, and ultimately drive growth. As we look toward the future, the potential of these platforms seems limitless, paving the way for smarter, more informed business strategies.
- What is predictive analytics? Predictive analytics is a branch of advanced analytics that uses historical data, statistical algorithms, and machine learning techniques to identify the likelihood of future outcomes.
- How can predictive analytics benefit my business? By using predictive analytics, businesses can make informed decisions, optimize operations, and improve customer experiences through better forecasting.
- Is predictive analytics only for large companies? No, predictive analytics platforms are scalable and can be beneficial for businesses of all sizes, including small and medium-sized enterprises.
- What types of data do predictive analytics platforms use? These platforms can utilize various data types, including transactional data, social media interactions, and customer feedback.
Frequently Asked Questions
- What is the role of AI in data processing?
AI plays a crucial role in data processing by automating repetitive tasks, which not only saves time but also enhances accuracy. With AI algorithms, businesses can streamline data management and extraction, allowing for quicker insights and more informed decision-making.
- How does supervised learning differ from unsupervised learning?
Supervised learning involves training models on labeled datasets, which means the data is already categorized. This allows the model to make predictions based on known outcomes. In contrast, unsupervised learning analyzes unlabeled data to discover hidden patterns without predefined categories, making it useful for exploratory analysis.
- What are some common applications of machine learning in data analysis?
Machine learning is widely used in various industries. In finance, for instance, it helps predict stock trends, while in healthcare, it can assist in diagnosing diseases based on patient data. These applications demonstrate how machine learning can lead to more accurate and efficient outcomes.
- What challenges do data analysts face when using supervised learning?
Despite its effectiveness, supervised learning can encounter challenges such as overfitting, where models perform well on training data but poorly on new data. Additionally, data quality issues can lead to inaccurate predictions. Addressing these challenges often involves careful data preparation and model validation.
- How do AI tools enhance data visualization?
AI tools enhance data visualization by transforming complex data sets into intuitive visual formats. This makes it easier for stakeholders to grasp insights quickly and effectively communicate findings, leading to better decision-making. With AI, visualizations can also adapt to highlight trends and anomalies automatically.
- What is predictive analytics, and how does it benefit businesses?
Predictive analytics utilizes AI to analyze historical data and forecast future trends and behaviors. This capability is invaluable for businesses as it allows them to anticipate customer needs, optimize operations, and make strategic decisions based on data-driven insights, ultimately leading to competitive advantages.