Predicting the Future of Weather with AI
Welcome to the fascinating world where technology meets nature! In recent years, artificial intelligence (AI) has emerged as a game-changer in the field of meteorology, revolutionizing the way we predict and understand weather patterns. Gone are the days of relying solely on traditional forecasting methods that often left us guessing. Today, AI is paving the way for more accurate predictions, timely alerts, and innovative solutions to combat climate-related challenges. Imagine being able to predict a storm's path with pinpoint accuracy or understanding climate trends that affect our daily lives. This article explores how AI is transforming weather forecasting and what it means for us, the planet, and future generations.
Artificial intelligence is not just a buzzword; it is a powerful tool that is reshaping meteorology. By analyzing vast datasets, AI enhances prediction models and provides real-time weather updates that are crucial for public safety and disaster preparedness. Think of AI as a super-intelligent assistant for meteorologists, tirelessly sifting through mountains of data to find patterns and make sense of the chaos that is our atmosphere. From predicting hurricanes to understanding local weather phenomena, AI technologies are being integrated into weather forecasting systems worldwide. This integration is not just about speed; it's about accuracy and reliability. With AI, we can expect more precise forecasts that can save lives and property.
At the heart of AI-driven weather predictions are machine learning algorithms. These techniques are like the brain of AI, enabling it to learn from data and improve over time. Various methods, such as neural networks and decision trees, are employed to enhance forecasting accuracy and efficiency. Imagine teaching a child to recognize different weather conditions by showing them countless pictures; that’s essentially what machine learning does with weather data. By continuously learning from new information, these algorithms refine their predictions, making them more reliable and insightful.
Neural networks are fascinating because they mimic the way our brain processes information. They excel at recognizing complex patterns in weather data that traditional models might miss. For instance, when predicting severe weather events like tornadoes or thunderstorms, neural networks can analyze historical data, current conditions, and even social media trends to provide timely alerts. This ability to connect the dots in seemingly unrelated data points is what sets neural networks apart. They are the detectives of the weather world, piecing together clues to forecast what’s coming next.
Training neural networks is akin to preparing an athlete for a competition. It involves feeding the network large datasets, allowing it to learn and improve its predictive capabilities. Just as an athlete practices to refine their skills, neural networks undergo rigorous training to optimize their performance. Techniques such as backpropagation and regularization are employed to enhance their learning processes, ensuring they can make accurate predictions even in the face of unpredictable weather. The goal is to create a model that not only predicts well but also adapts to new data effectively.
However, the journey of implementing neural networks is not without its challenges. Issues like overfitting—where a model learns the training data too well and fails to generalize to new data—and data bias can hinder their effectiveness. These challenges highlight the importance of using diverse datasets and employing strategies to mitigate these risks. Just as a chef needs the right ingredients to create a delicious dish, meteorologists must ensure their models are trained on high-quality, representative data to produce accurate forecasts.
Another powerful tool in the AI arsenal is the decision tree. This method provides a visual representation of decision-making processes based on various weather variables. Decision trees simplify complex forecasting models, making them easier to understand and interpret. They work by breaking down the data into branches, leading to different outcomes based on specific conditions. Imagine navigating through a maze where each turn is determined by the weather conditions; decision trees help meteorologists make informed decisions about weather predictions.
AI's effectiveness in weather forecasting heavily relies on diverse data sources. These include satellite imagery, sensor networks, and historical weather data. The quality and availability of this data are crucial for enhancing forecast accuracy. Just like a painter needs a variety of colors to create a masterpiece, meteorologists need rich datasets to paint an accurate picture of the weather. The more data they have, the better equipped they are to make precise predictions.
Satellite imagery plays a pivotal role in weather monitoring and forecasting. AI analyzes this data to detect patterns and predict weather changes. Think of satellites as the eyes in the sky, providing a bird's-eye view of weather systems across the globe. By employing AI to sift through this imagery, meteorologists can identify emerging storm systems, track their movement, and anticipate their impact on different regions.
Ground-based sensors also contribute significantly to real-time weather information. AI integrates this sensor data with other sources to improve local weather predictions and enhance situational awareness. Imagine having a network of weather stations spread across a city, each providing real-time data about temperature, humidity, and wind speed. By combining this information, AI can deliver hyper-local forecasts that are incredibly useful for residents and businesses alike.
The future of AI in weather forecasting is bright, with advancements in predictive analytics, personalized weather services, and climate modeling on the horizon. As technology continues to evolve, we can expect even more sophisticated tools that will enhance our understanding of weather patterns and climate change.
One exciting trend is the rise of personalized weather services. AI allows for tailored forecasts based on individual preferences and locations. Imagine receiving a weather update that tells you exactly when to carry an umbrella or when to plan a picnic, all based on your specific needs. This level of personalization can significantly impact how we plan our daily activities and make informed decisions.
AI can also play a crucial role in addressing climate change challenges. By providing data-driven insights, AI can inform policy decisions and promote sustainable practices in weather management. Just like a compass guides a traveler, AI can help us navigate the complexities of climate change, offering solutions that are both innovative and effective.
- How does AI improve weather forecasting? AI analyzes vast amounts of data to identify patterns and make more accurate predictions.
- What are the main challenges of using AI in meteorology? Challenges include overfitting, data bias, and the need for high-quality datasets.
- Can AI predict extreme weather events? Yes, AI is particularly effective at predicting severe weather events by analyzing historical and real-time data.
- What is the future of AI in weather forecasting? The future includes personalized services, improved predictive analytics, and solutions for climate change.
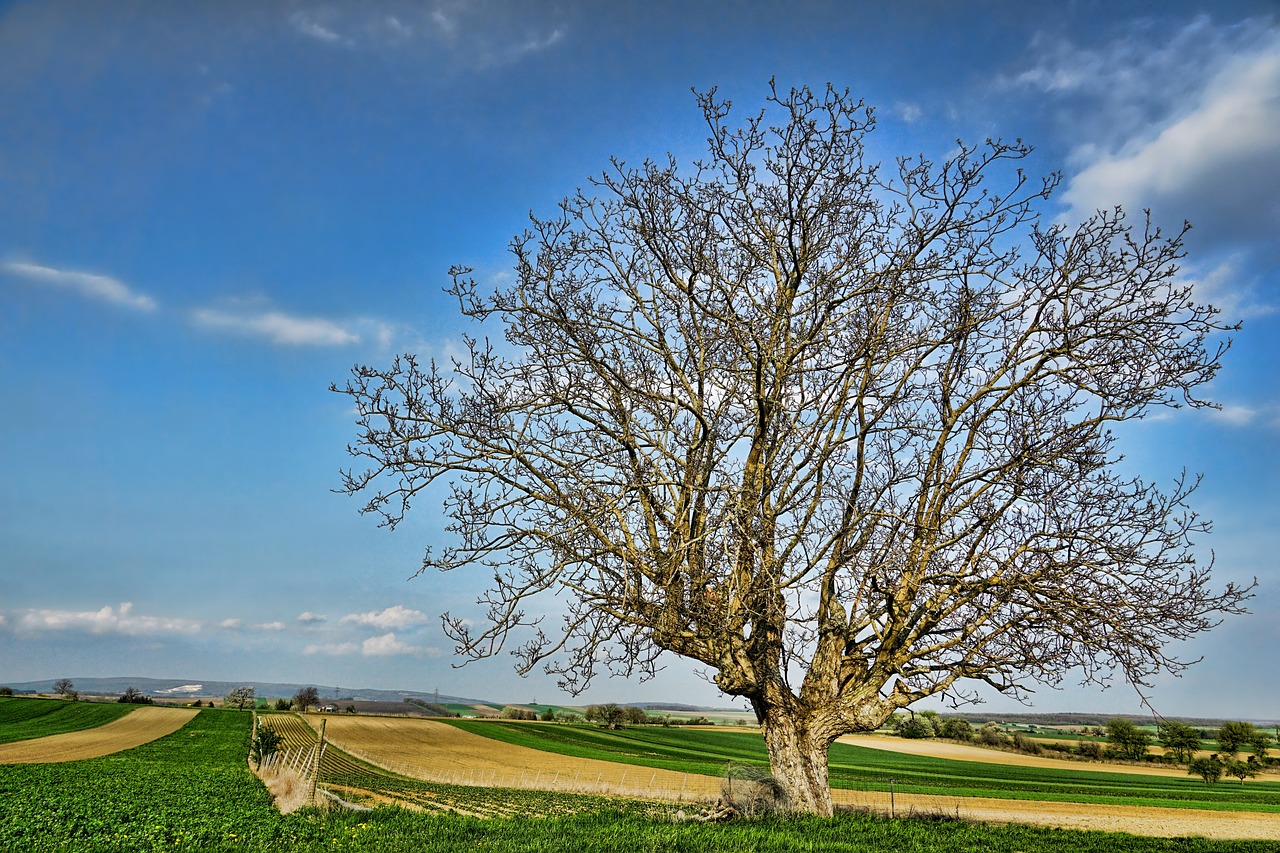
The Role of AI in Meteorology
Artificial intelligence is revolutionizing the field of meteorology in ways that were once thought to be the stuff of science fiction. Imagine a world where weather predictions are not only more accurate but also delivered in real-time, allowing us to prepare for storms, floods, or heatwaves with unprecedented precision. With AI’s ability to analyze vast datasets at lightning speed, meteorologists are now equipped with tools that enhance their forecasting capabilities significantly.
At the core of this transformation is the integration of advanced algorithms that can process and interpret complex weather patterns. Traditional forecasting methods often relied on historical data and simple models, but AI takes this a step further. By utilizing machine learning and deep learning techniques, meteorologists can now identify intricate relationships within the data that were previously undetectable. For instance, AI can analyze satellite images, radar data, and atmospheric readings in real-time, providing insights that help predict severe weather events with remarkable accuracy.
Moreover, the role of AI extends beyond just prediction. It also plays a crucial part in data assimilation, which is the process of integrating data from various sources to create a more comprehensive picture of current weather conditions. This is particularly important in rapidly changing environments where conditions can shift dramatically within minutes. AI systems can continuously learn and adapt, improving their forecasting models over time, which means that the more data they process, the better they become at predicting future weather scenarios.
One of the most exciting aspects of AI in meteorology is its potential for real-time updates. Imagine receiving alerts on your smartphone about sudden weather changes in your area, allowing you to adjust your plans accordingly. This capability is not just a convenience; it can save lives and property by providing timely warnings about dangerous weather conditions. For example, AI-driven systems can analyze patterns that precede severe thunderstorms or tornadoes, giving communities precious extra minutes to prepare.
Furthermore, the implications of AI in meteorology stretch far beyond individual forecasts. It can also inform large-scale climate models, helping scientists understand long-term trends and the impacts of climate change. By analyzing historical weather patterns alongside current data, AI can help predict how climate change will affect weather systems, allowing for better preparation and response strategies. This is particularly vital in our current climate crisis, where understanding and mitigating the effects of climate change is more important than ever.
In summary, the role of AI in meteorology is multifaceted and transformative. From enhancing predictive accuracy to providing real-time updates and informing climate models, AI is set to redefine how we understand and interact with our weather systems. As technology continues to evolve, the potential for AI in this field is not only exciting but also essential for navigating the challenges of our changing climate.
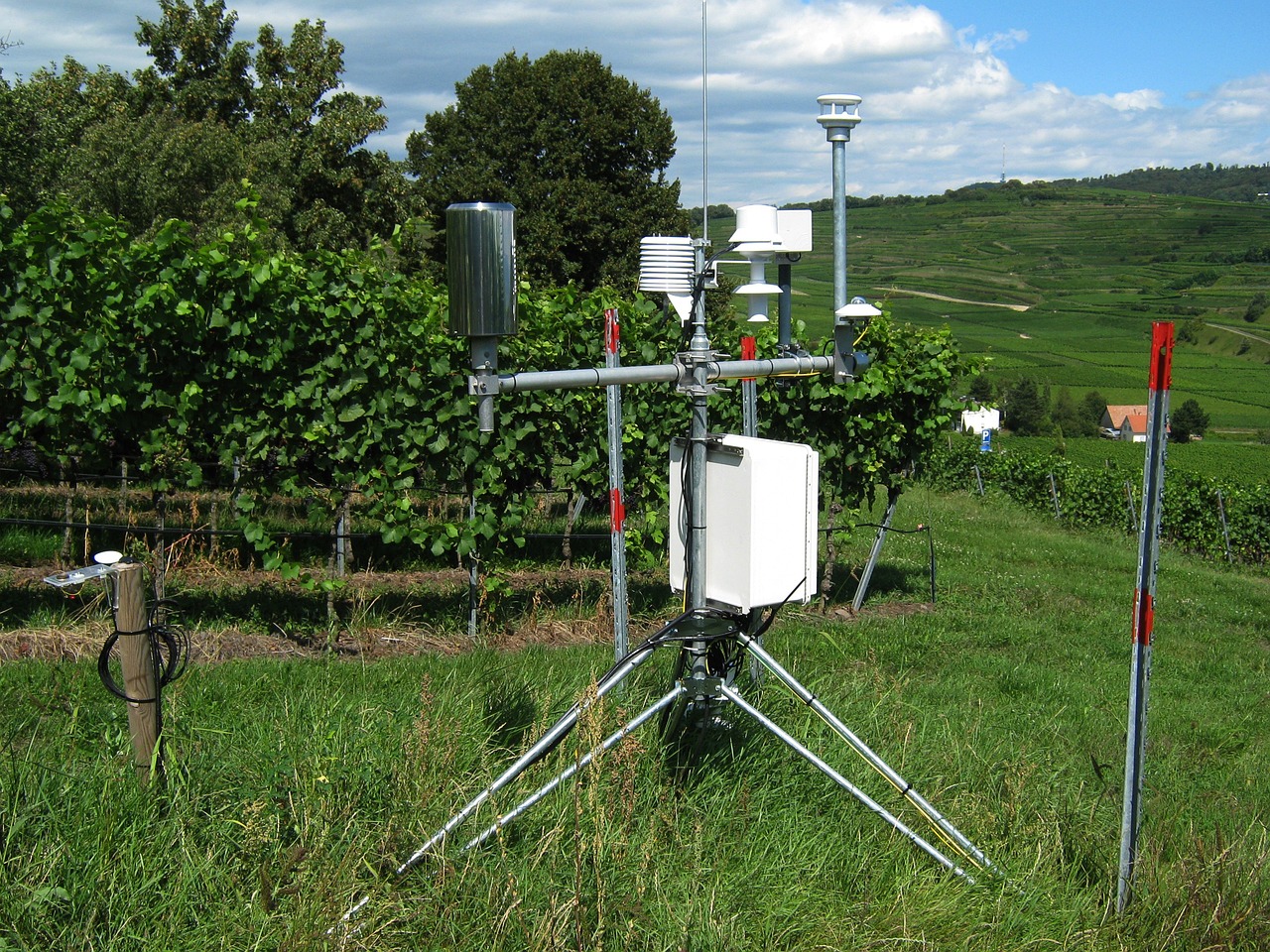
Machine Learning Techniques
When it comes to revolutionizing weather forecasting, machine learning techniques are the unsung heroes behind the scenes. These advanced algorithms analyze enormous datasets and identify patterns that would be nearly impossible for humans to detect. By leveraging techniques such as neural networks and decision trees, meteorologists can enhance the accuracy and efficiency of weather predictions, making them more reliable than ever before.
Imagine trying to predict the weather as if you were piecing together a jigsaw puzzle. Each piece represents a different variable, such as temperature, humidity, and wind speed. Traditional methods can be like trying to fit the pieces together without knowing what the final picture looks like. However, machine learning acts like a seasoned puzzle master, quickly identifying where each piece belongs based on previous experiences and vast amounts of data.
One of the standout features of machine learning in meteorology is its ability to learn from historical data. This means that as new weather patterns emerge, machine learning models can adapt and refine their predictions. For instance, if a specific weather pattern leads to a series of storms, machine learning algorithms can analyze that data and adjust future predictions accordingly. This adaptability is crucial, especially in a world where climate change is causing weather patterns to shift unpredictably.
Let's take a closer look at two prominent machine learning techniques:
- Neural Networks: These algorithms are designed to mimic the way the human brain processes information. They excel in recognizing complex patterns in data, making them particularly effective for predicting severe weather events like hurricanes or tornadoes. By training on vast datasets, neural networks can identify subtle signals that indicate an impending storm.
- Decision Trees: This technique provides a visual representation of decision-making processes based on various weather variables. Decision trees simplify complex forecasting models by breaking down predictions into a series of if-then scenarios, making it easier for meteorologists to understand and communicate potential weather outcomes.
While these techniques are powerful, they are not without their challenges. For instance, neural networks can sometimes suffer from overfitting, where they become too tailored to the training data and lose their ability to generalize to new data. Additionally, biases in the data can lead to skewed predictions, which is why it's essential to continually refine and optimize these models.
In summary, machine learning techniques are transforming the landscape of weather forecasting, enabling us to make predictions with a level of accuracy that was once thought impossible. As these technologies continue to evolve, we can expect even more innovative solutions to emerge, helping us better understand and respond to the ever-changing climate.
- What is machine learning in weather forecasting? Machine learning in weather forecasting refers to the use of algorithms that analyze vast datasets to identify patterns and improve prediction accuracy.
- How do neural networks work? Neural networks mimic the human brain's functioning by recognizing complex patterns in data, making them effective for predicting severe weather events.
- What are decision trees? Decision trees are a type of machine learning algorithm that provides a visual representation of decision-making processes based on various weather variables.
- What challenges do machine learning models face? Common challenges include overfitting, data bias, and the need for continual refinement to improve accuracy.
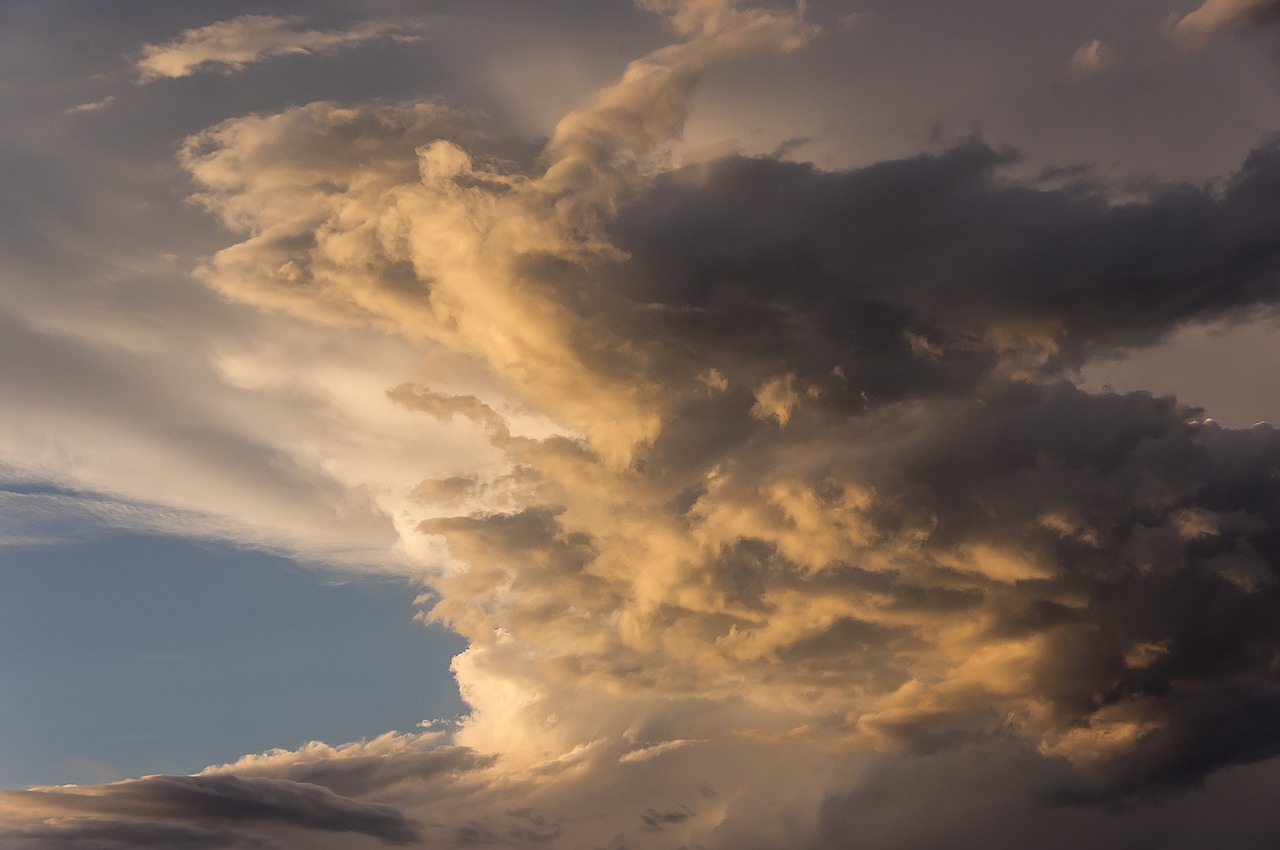
Neural Networks in Weather Prediction
Neural networks are like the brain of a weather forecasting system, capable of recognizing complex patterns in vast amounts of data. Imagine trying to find a needle in a haystack; neural networks excel at sifting through the hay to find that needle, which in this case, are the subtle indicators of impending weather changes. By mimicking the way our brains process information, these networks can analyze historical weather data and current atmospheric conditions to make accurate predictions about future weather events.
One of the most exciting aspects of neural networks is their ability to learn from the data they process. This means that the more data they are exposed to, the better they become at predicting weather patterns. For instance, when trained on historical data of hurricanes, a neural network can identify patterns that precede such events, allowing meteorologists to issue warnings much earlier than traditional methods. This capability is crucial in saving lives and minimizing property damage during severe weather events.
Neural networks operate through layers of interconnected nodes, similar to neurons in the human brain. Each node processes a small piece of information and passes it on to the next layer. This structure allows for the analysis of various weather parameters, such as temperature, humidity, wind speed, and atmospheric pressure, all at once. The result is a more nuanced understanding of how these factors interact, leading to improved accuracy in weather forecasts.
However, training these networks is not without challenges. For instance, the quality of data plays a significant role in the effectiveness of the neural networks. If the data is biased or incomplete, the predictions can be skewed. Moreover, overfitting is a common issue where the model performs exceptionally well on training data but fails to generalize to new, unseen data. To combat this, meteorologists often employ techniques such as cross-validation and regularization to ensure that the neural network remains robust.
In summary, neural networks are revolutionizing weather prediction by enhancing our ability to forecast severe weather events and climate trends. Their capacity for learning and pattern recognition makes them a powerful tool in meteorology. As technology continues to advance, the integration of neural networks into weather forecasting systems will likely lead to even greater accuracy and efficiency, ultimately benefiting society as a whole.
- What are neural networks? Neural networks are computational models inspired by the human brain, used to recognize patterns and make predictions based on data.
- How do neural networks improve weather forecasting? They analyze vast datasets to identify patterns in weather phenomena, leading to more accurate predictions.
- What challenges do neural networks face in weather prediction? Common challenges include data quality issues, overfitting, and the need for large datasets for effective training.
- Can neural networks predict severe weather events? Yes, they can analyze historical data to identify indicators of severe weather, allowing for earlier warnings.
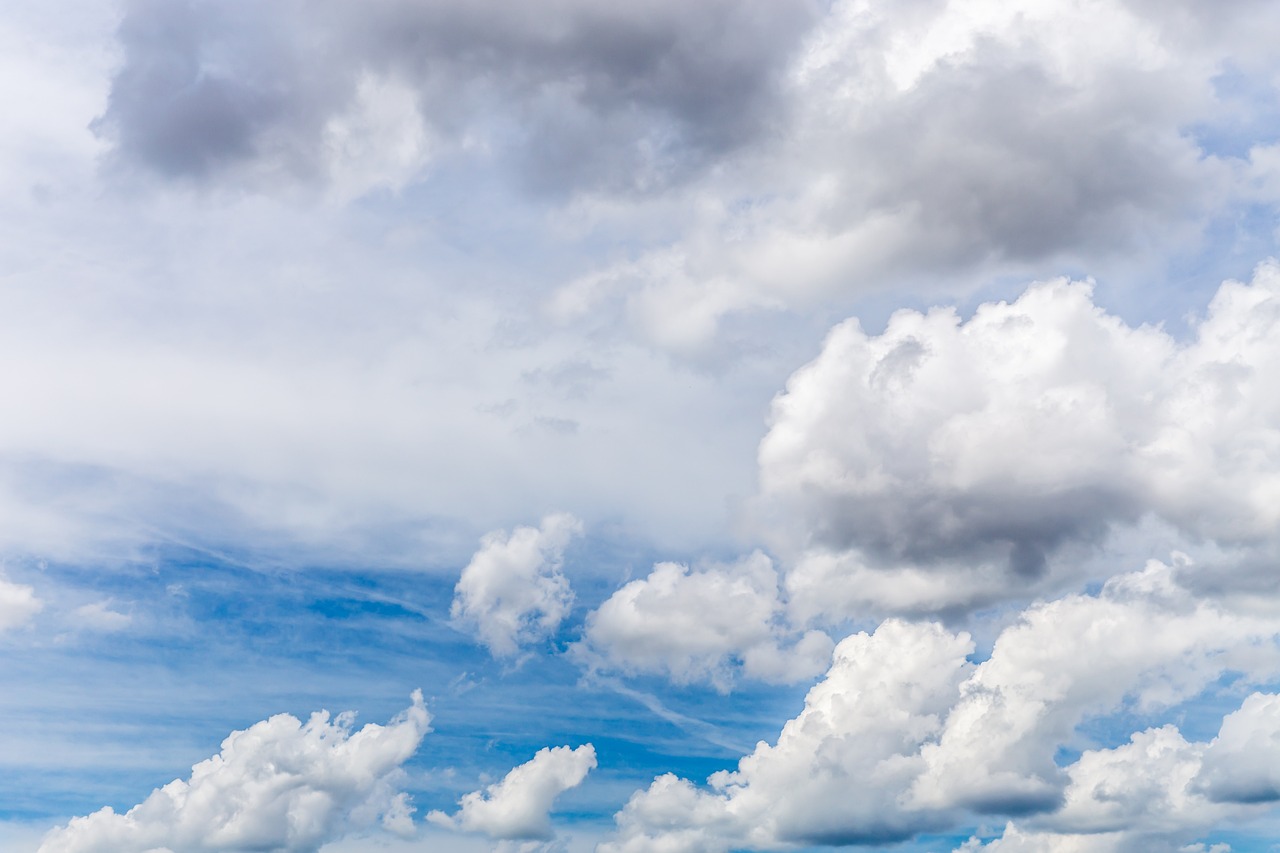
Training Neural Networks
Training neural networks is a fascinating yet complex process that requires a deep understanding of both the underlying algorithms and the data being utilized. At its core, training involves feeding the network large volumes of data so it can learn to recognize patterns and make accurate predictions. Think of it like teaching a child to recognize animals; the more examples you show them, the better they become at identifying different species. In the context of weather forecasting, neural networks are trained using historical weather data, satellite imagery, and various atmospheric parameters.
One of the key components in training neural networks is the backpropagation algorithm. This method allows the network to adjust its internal parameters based on the errors it makes during predictions. Essentially, when the network predicts a weather event incorrectly, backpropagation helps it learn from that mistake by adjusting its weights. This iterative process continues until the model reaches an acceptable level of accuracy. However, achieving this can be challenging, as it requires careful tuning of hyperparameters, such as learning rate, batch size, and the number of layers in the network.
Moreover, the quality of the training data plays a crucial role in the performance of neural networks. If the data is noisy or unrepresentative, the model may struggle to generalize its predictions. For instance, if a neural network is trained predominantly on data from a specific region, it may not perform well when predicting weather for a different area. Therefore, it’s essential to ensure that the training dataset is diverse and comprehensive, capturing a wide range of weather conditions and phenomena.
To optimize the training process, various techniques can be employed:
- Data Augmentation: This involves creating modified versions of the training data to increase its size and diversity, which helps improve the model's robustness.
- Regularization Techniques: Methods like dropout and L2 regularization help prevent overfitting, ensuring that the model performs well on unseen data.
- Early Stopping: This technique monitors the model's performance on a validation set and stops training when performance begins to degrade, thus preserving the best model.
In summary, training neural networks for weather prediction is a multifaceted endeavor that requires not only a strong foundation in machine learning principles but also a keen understanding of meteorological data. As technology advances, the methods and tools available for training these models will continue to evolve, promising even more accurate and reliable weather forecasts in the future.
- What is the primary goal of training neural networks in weather forecasting?
The primary goal is to enable the network to accurately predict weather events by recognizing complex patterns in historical and real-time data.
- How long does it take to train a neural network for weather predictions?
The training time can vary widely depending on the complexity of the model, the size of the dataset, and the computational resources available. It can range from hours to days.
- What are the common challenges faced during the training process?
Common challenges include overfitting, insufficient data quality, and the need for extensive tuning of hyperparameters.
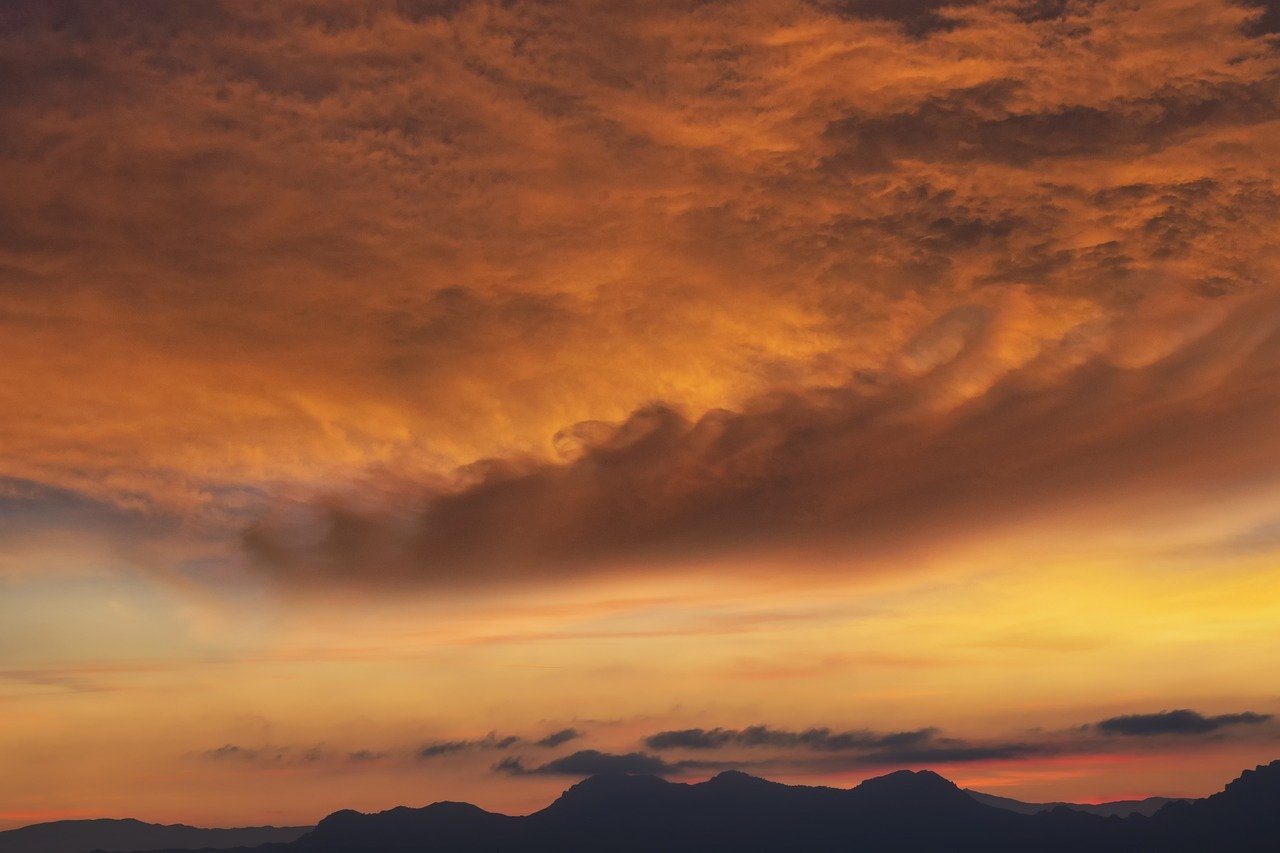
Challenges in Neural Network Implementation
When it comes to implementing neural networks for weather forecasting, the journey is not without its bumps. While these models hold immense potential for improving prediction accuracy, they also face several significant challenges that can hinder their effectiveness. One major issue is overfitting, where the model learns the training data too well, including its noise and outliers, making it less effective on new, unseen data. Imagine a student who memorizes every detail of a textbook but struggles to apply that knowledge in a real-world scenario; that's what overfitting does to neural networks.
Another challenge is data bias. If the data used to train these models is not representative of the broader environment, the predictions can be skewed. For instance, if a neural network is trained primarily on data from urban areas, it may fail to accurately predict weather patterns in rural regions. This can lead to significant discrepancies in forecasts, which can be detrimental in situations where accurate weather information is crucial, such as during severe weather events.
Moreover, the computational cost associated with training neural networks can be quite high. These models require substantial processing power and time to analyze vast amounts of data, which can be a barrier for smaller organizations or those with limited resources. Think of it like trying to bake a complex cake; if you don't have the right tools or ingredients, the outcome may not be what you hoped for.
To address these challenges, researchers and developers are continuously exploring various strategies. For instance, techniques such as cross-validation can help mitigate overfitting by ensuring that the model is tested on different subsets of data. Additionally, employing data augmentation methods can enhance the diversity of training datasets, making them more representative and reducing bias. Finally, leveraging cloud computing resources can help alleviate the computational burden, allowing for more efficient training processes.
In summary, while the implementation of neural networks in weather forecasting is promising, it is essential to navigate the challenges of overfitting, data bias, and computational costs. By addressing these issues, we can unlock the full potential of AI in meteorology and pave the way for more accurate and reliable weather predictions.
- What is overfitting in neural networks?
Overfitting occurs when a model learns the details and noise in the training data to the extent that it negatively impacts its performance on new data. - How can data bias affect weather predictions?
If the training data is not representative of all weather conditions or regions, the model may produce inaccurate forecasts for underrepresented areas. - What are some strategies to combat overfitting?
Techniques such as cross-validation, regularization, and using simpler models can help mitigate the risk of overfitting. - Why is computational cost a concern for neural networks?
Training complex neural networks can require significant computational resources, which may be a barrier for organizations with limited budgets.
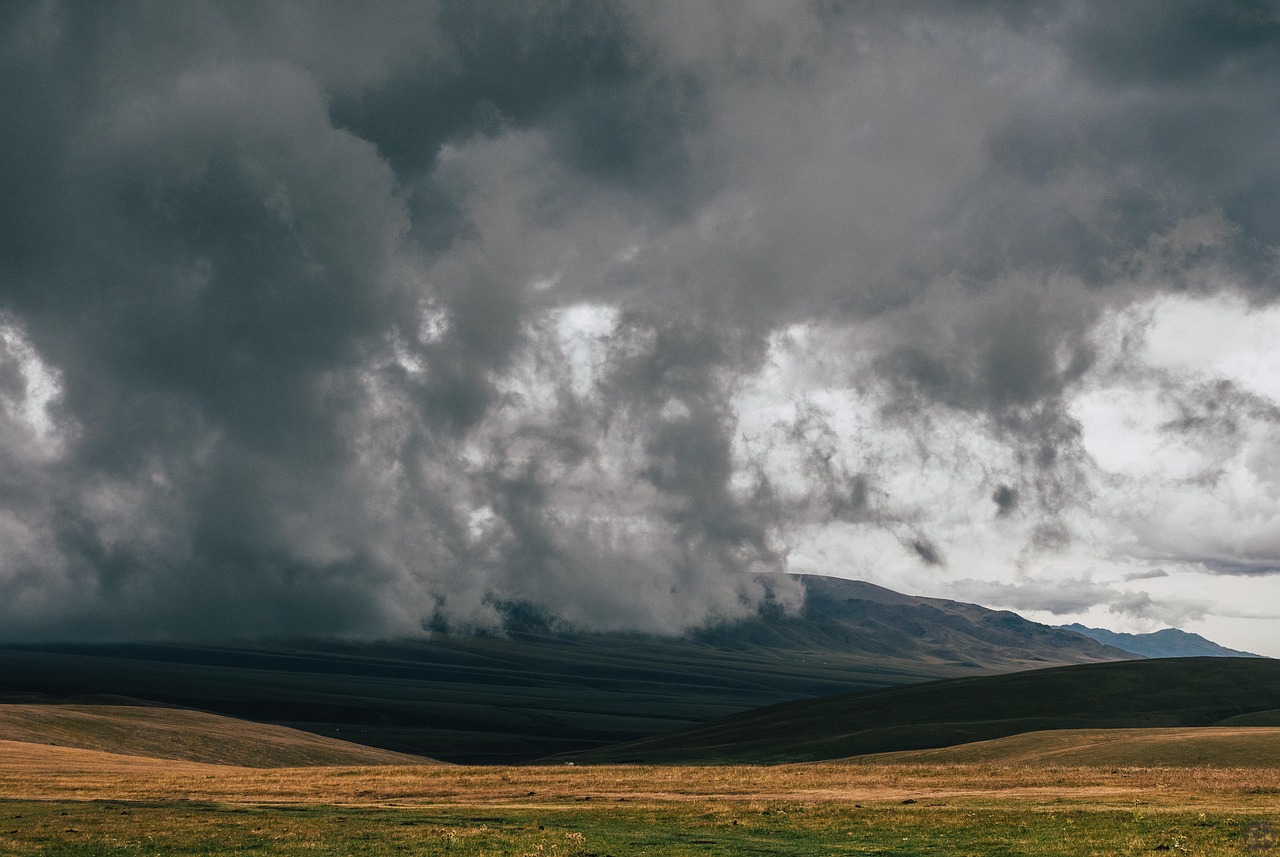
Decision Trees and Their Applications
Decision trees are a powerful tool in the realm of artificial intelligence, particularly in weather forecasting. They serve as a visual representation of decision-making processes based on various weather variables. Imagine a tree branching out, where each split represents a decision point based on specific data inputs like temperature, humidity, and wind speed. This structure makes it easier for meteorologists to understand complex relationships in weather data, thereby enhancing the overall forecasting process.
One of the primary advantages of decision trees is their interpretability. Unlike some other machine learning models that operate like a black box, decision trees provide clear insights into how decisions are made. This transparency is crucial for meteorologists who need to explain their predictions to the public and policymakers. For instance, if a decision tree indicates that a high humidity level and low pressure lead to storm conditions, it allows meteorologists to communicate these factors effectively to the community, promoting better preparedness.
Moreover, decision trees can handle both categorical and numerical data, making them versatile in various forecasting scenarios. They can be used for predicting severe weather events, such as thunderstorms or hurricanes, by analyzing historical data patterns. In this context, decision trees help identify critical thresholds that trigger these extreme weather conditions.
To illustrate their effectiveness, consider the following table that summarizes some common applications of decision trees in weather forecasting:
Application | Description |
---|---|
Severe Weather Prediction | Identifying conditions that lead to events like tornadoes or hurricanes. |
Climate Trend Analysis | Assessing long-term climate changes based on historical weather data. |
Local Weather Forecasting | Providing accurate short-term forecasts for specific regions. |
Risk Assessment | Evaluating the potential impact of weather events on communities. |
In addition to their practical applications, decision trees are also beneficial for modeling interactions between different weather variables. For instance, a decision tree can show how temperature and humidity interact to influence precipitation levels. This ability to model interactions allows meteorologists to create more nuanced and accurate forecasts, which can be particularly valuable in regions prone to rapid weather changes.
However, it's essential to recognize that decision trees also have limitations. They can be prone to overfitting, especially when dealing with complex datasets. This means that while they may perform exceptionally well on training data, their predictive accuracy may drop when applied to new, unseen data. To counter this challenge, meteorologists often use techniques like pruning, which simplifies the tree by removing branches that have little significance. This process enhances the model's generalization capabilities, ensuring that forecasts remain reliable across various conditions.
In conclusion, decision trees are an invaluable asset in the field of weather forecasting. Their ability to provide clear, interpretable results while handling various types of data makes them a preferred choice among meteorologists. As AI continues to evolve, the integration of decision trees with other machine learning techniques promises to enhance forecasting accuracy even further, paving the way for more informed decision-making in weather-related scenarios.

Data Sources for AI Weather Forecasting
When it comes to predicting the unpredictable, the role of data sources in AI weather forecasting can't be overstated. Artificial intelligence thrives on data, and the more diverse and rich the data, the better the predictions. Imagine trying to solve a complex puzzle with only a few pieces; it’s nearly impossible! Similarly, AI requires a comprehensive set of data to accurately forecast weather patterns and phenomena.
One of the primary data sources is satellite imagery. Satellites orbiting the Earth collect a massive amount of visual and infrared data, allowing meteorologists to monitor cloud formations, storm systems, and more. The beauty of AI lies in its ability to process this data at lightning speed, identifying patterns that human eyes might miss. By analyzing satellite images, AI can detect early signs of severe weather events, such as hurricanes or thunderstorms, giving us precious time to prepare.
Another critical source of data comes from ground-based sensor networks. These sensors are scattered across various geographical locations, collecting real-time weather information such as temperature, humidity, wind speed, and atmospheric pressure. This data is invaluable for local weather predictions. When AI integrates sensor data with satellite imagery and historical weather data, it creates a more robust and accurate forecasting model. Think of it as combining the insights from various experts to get a well-rounded view of the situation.
Furthermore, historical weather data plays a pivotal role in training AI models. By feeding AI systems with vast amounts of past weather data, they learn to recognize patterns and trends. This historical perspective allows AI to make educated predictions about future weather conditions. For instance, if a certain weather pattern typically precedes a snowstorm in a specific region, the AI can flag that pattern and alert meteorologists to the potential for snow.
It's also essential to consider the quality and availability of these data sources. Poor-quality data can lead to inaccurate predictions, which can have serious consequences, especially in extreme weather situations. As such, data cleansing and validation processes are crucial to ensure that the AI systems are working with the best information possible.
In summary, the integration of diverse data sources—satellite imagery, ground-based sensors, and historical weather data—forms the backbone of AI weather forecasting. By leveraging these rich datasets, AI can enhance the accuracy of weather predictions and provide timely insights that can save lives and property. The future of weather forecasting is undoubtedly bright, with AI leading the charge in transforming how we understand and respond to our ever-changing climate.
- What types of data are used in AI weather forecasting? AI weather forecasting utilizes satellite imagery, ground-based sensor data, and historical weather data to create accurate predictions.
- How does AI improve the accuracy of weather forecasts? AI analyzes vast datasets quickly, identifying complex patterns that can lead to more precise predictions.
- Can AI predict severe weather events? Yes, by analyzing satellite data and other sources, AI can detect early signs of severe weather, providing valuable warnings.
- Why is data quality important in AI weather forecasting? Poor-quality data can lead to inaccurate predictions, which can have significant consequences in extreme weather situations.
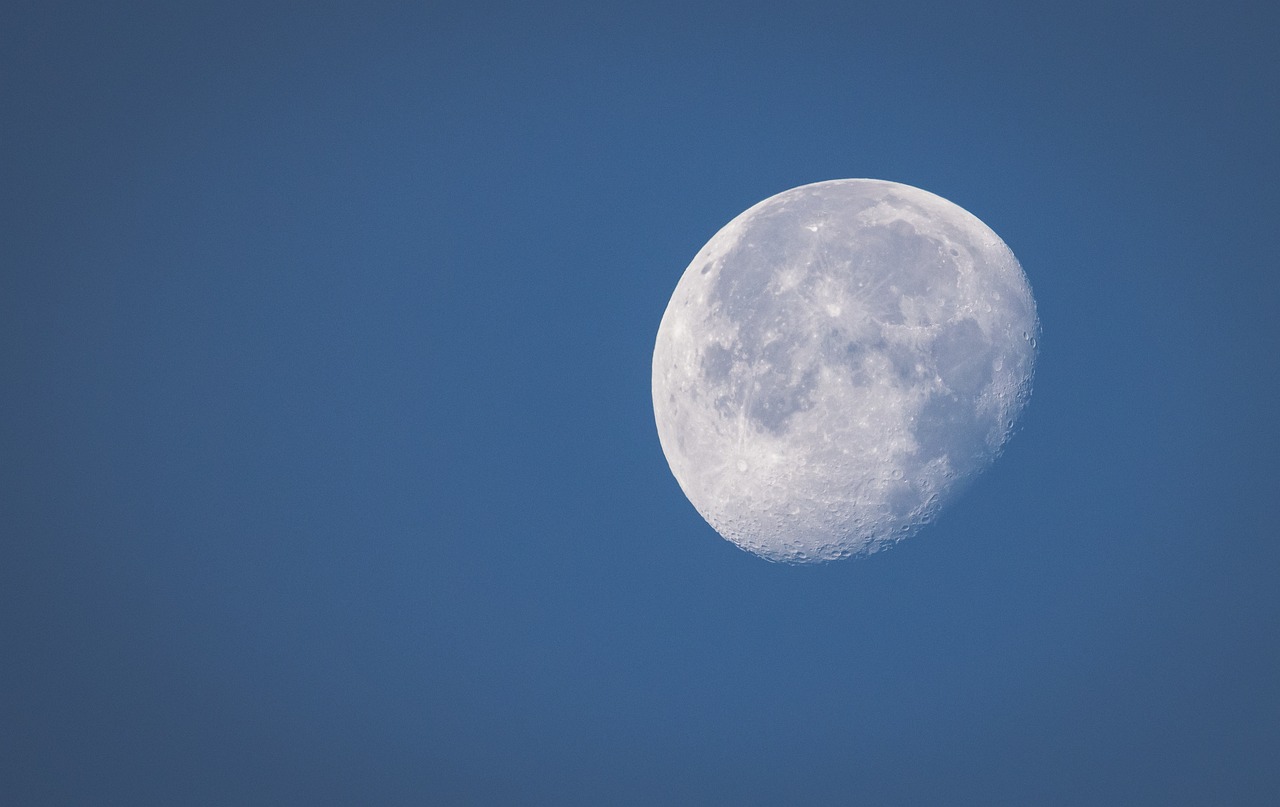
Satellite Imagery Analysis
Satellite imagery has become an indispensable tool in the field of meteorology, revolutionizing the way we monitor and predict weather patterns. With the ability to capture vast amounts of data from space, satellites provide a comprehensive view of atmospheric conditions that ground-based observations simply cannot match. This technology allows meteorologists to analyze cloud formations, track storm systems, and even identify temperature variations across different regions. But how does artificial intelligence enhance the capabilities of satellite imagery analysis? Let's dive deeper.
By employing machine learning algorithms, AI can sift through the enormous datasets generated by satellites, extracting meaningful patterns and trends that would be nearly impossible for humans to identify alone. For instance, neural networks can process images to detect subtle changes in cloud cover or moisture levels, which can be critical indicators of impending weather events. The integration of AI in satellite imagery analysis not only boosts the speed of data processing but also significantly improves the accuracy of weather predictions.
One of the most exciting advancements in this field is the ability of AI to perform real-time analysis. Traditional methods often involve delayed reporting, which can lead to missed opportunities for timely warnings. However, with AI-driven systems, meteorologists can receive instant updates on weather conditions, allowing them to respond more effectively to severe weather threats. This capability is especially crucial during hurricane season when every second counts.
Moreover, the use of AI in satellite imagery analysis facilitates the identification of climate trends over time. By comparing historical satellite data with current observations, AI can help researchers understand how climate change is affecting weather patterns. This insight is invaluable for developing strategies to mitigate the impacts of climate change on communities and ecosystems.
To illustrate the power of AI-enhanced satellite imagery analysis, consider the following table that outlines some key applications:
Application | Description | Benefits |
---|---|---|
Storm Tracking | Real-time monitoring of storm systems using satellite data. | Improved accuracy in predicting storm paths and intensity. |
Temperature Mapping | Analyzing surface temperature variations across regions. | Enhanced understanding of heatwaves and cold fronts. |
Cloud Pattern Recognition | Identifying cloud formations that indicate severe weather. | Early warnings for thunderstorms and tornadoes. |
In summary, satellite imagery analysis powered by artificial intelligence is a game changer for meteorology. It not only enhances our ability to predict weather with greater accuracy but also equips us with the tools necessary to understand and adapt to our changing climate. As we continue to innovate in this space, the potential for AI to transform weather forecasting remains boundless.
- What is satellite imagery? Satellite imagery refers to images of the Earth taken from satellites orbiting the planet, providing a bird's-eye view of weather patterns and environmental changes.
- How does AI improve weather forecasting? AI analyzes large datasets quickly and accurately, identifying patterns that enhance the precision of weather predictions.
- Can AI predict severe weather events? Yes, AI can analyze data in real-time to predict severe weather events, allowing for timely warnings and better preparedness.
- What role do satellites play in climate change research? Satellites provide critical data for monitoring climate changes over time, helping scientists understand trends and develop strategies to combat climate issues.
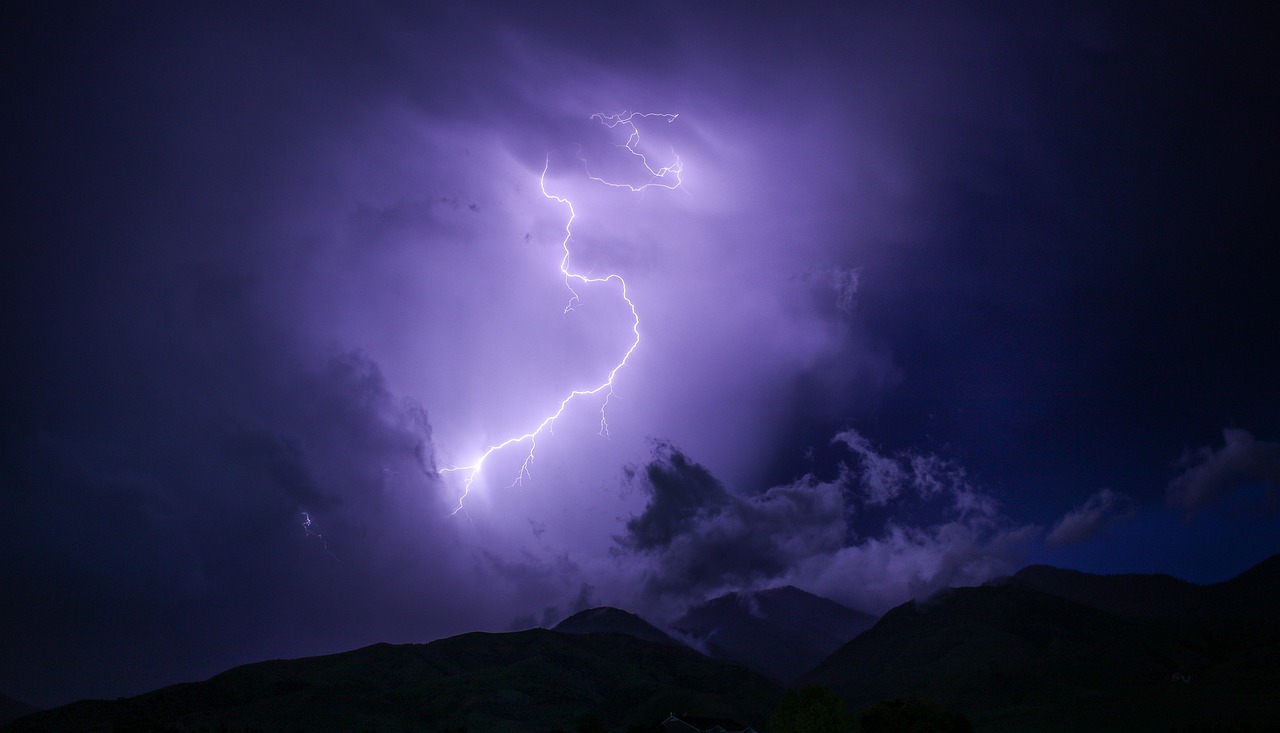
Integrating Sensor Data
When it comes to weather forecasting, the integration of sensor data is like adding a secret ingredient to a recipe that takes it from good to absolutely phenomenal. Ground-based sensors are scattered across various locations, capturing real-time weather information such as temperature, humidity, wind speed, and atmospheric pressure. This data is vital for creating accurate and localized weather forecasts. Imagine trying to predict the weather for a picnic without knowing if it's raining just a few blocks away—this is where sensor data shines!
AI excels at processing and analyzing this vast amount of sensor data, allowing meteorologists to gain insights that were previously unattainable. For instance, by combining sensor data with satellite imagery and historical weather patterns, AI can create a comprehensive picture of current and future weather conditions. This integration not only enhances the accuracy of forecasts but also improves situational awareness during severe weather events.
Moreover, the real-time nature of sensor data enables quick responses to changing weather conditions. For example, if a sudden storm is detected by ground sensors, alerts can be issued immediately, giving people the time they need to prepare. This capability is particularly crucial in areas prone to severe weather, where every minute counts. The integration process involves several steps:
- Data Collection: Sensors collect data continuously, feeding it into centralized systems.
- Data Processing: AI algorithms process this data, filtering out noise and identifying significant weather patterns.
- Forecast Generation: The processed data is then used to update forecasts in real-time, ensuring they reflect the latest conditions.
This synergy between AI and sensor data is not just about improving forecasts; it's also about enhancing public safety and preparedness. By utilizing sensor networks effectively, communities can develop better emergency response strategies and resource allocation plans during adverse weather conditions. It's a win-win situation where technology and nature work hand in hand to protect lives and property.
As we look to the future, the potential for integrating sensor data with AI technology is vast. Innovations such as IoT (Internet of Things) devices will further enhance data collection capabilities, leading to even more precise and localized weather predictions. The more data we gather, the better we can understand and anticipate the whims of Mother Nature. So, the next time you check the weather, remember that behind those forecasts is a complex network of sensors and AI working tirelessly to keep you informed and safe.
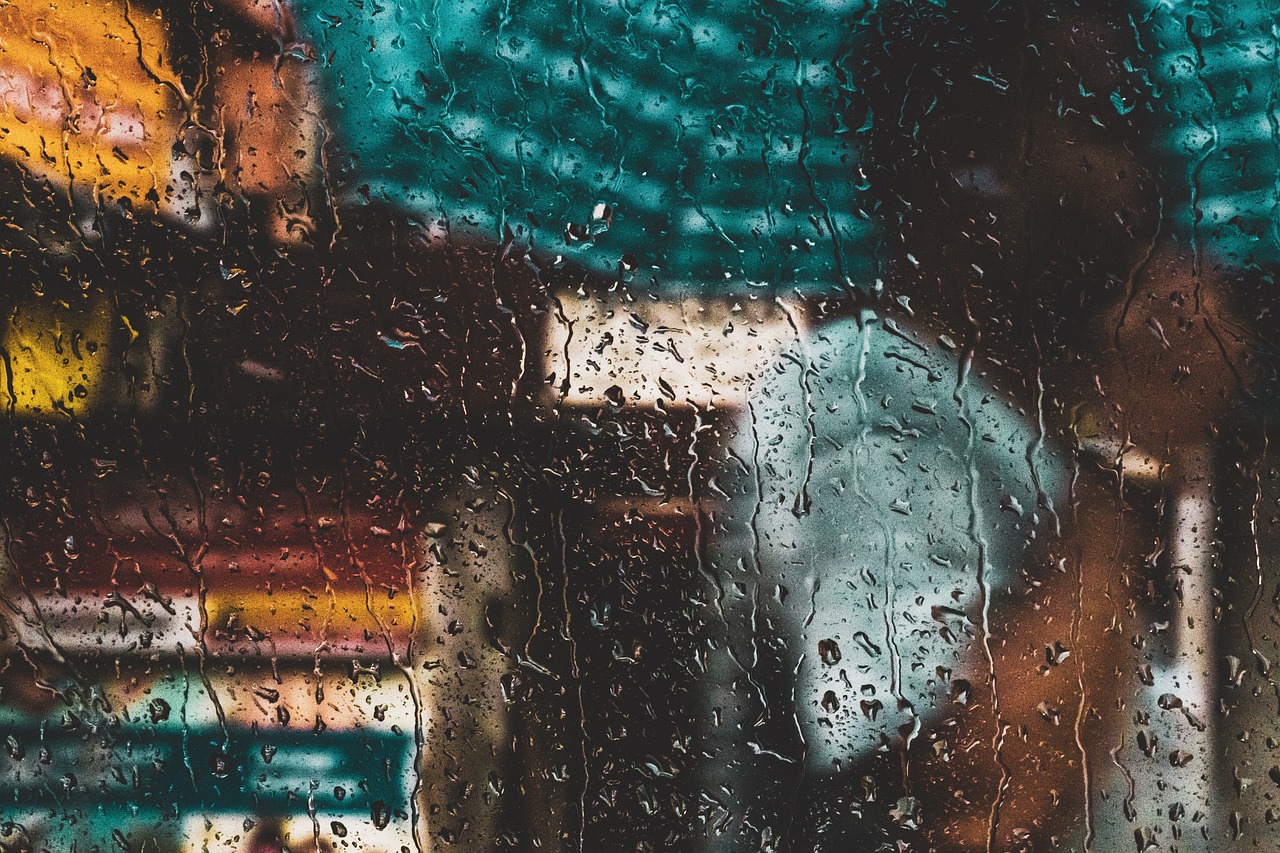
Future Trends in AI Weather Forecasting
The future of AI in weather forecasting is not just bright; it's practically glowing with possibilities! As technology continues to advance, we can expect a wave of innovations that will fundamentally change how we understand and predict weather patterns. Imagine waking up to a personalized weather report that not only tells you if it will rain but also suggests the best time for your morning jog based on real-time atmospheric data. This is just a glimpse of what’s to come!
One of the most exciting trends is the rise of predictive analytics. By harnessing the power of AI, meteorologists can analyze historical weather data alongside current patterns to forecast future conditions with unprecedented accuracy. This means fewer surprises, more accurate alerts for severe weather events, and ultimately, lives saved. With AI, we’re not just predicting the weather; we’re understanding it on a deeper level.
Moreover, the concept of personalized weather services is gaining traction. With AI's ability to process vast amounts of data from various sources, weather forecasts can be tailored to individual preferences. For instance, if you’re planning a picnic, AI can deliver hyper-local forecasts that consider not just the temperature but also wind speed, humidity, and even pollen counts. This level of personalization could reshape how businesses operate, enabling them to make more informed decisions based on localized weather predictions.
In addition to personalization, AI is set to play a crucial role in addressing the challenges posed by climate change. As we face increasingly unpredictable weather patterns, AI can provide valuable insights that inform policy decisions and promote sustainable practices. By analyzing data on temperature changes, precipitation patterns, and extreme weather events, AI can help governments and organizations develop strategies to mitigate the effects of climate change. For example, AI can assist in optimizing water resource management during droughts or floods, ensuring that communities are better prepared for the challenges ahead.
To illustrate the potential of AI in weather forecasting, consider the following table showcasing key future trends:
Trend | Description | Impact |
---|---|---|
Predictive Analytics | Utilizing historical and real-time data for accurate forecasts | Improved accuracy and timely alerts for severe weather |
Personalized Weather Services | Tailored forecasts based on user preferences and locations | Enhanced user experience and informed decision-making |
Climate Change Solutions | AI-driven insights for sustainable policy-making | Better preparedness for climate-related challenges |
In summary, the future of AI in weather forecasting is poised to revolutionize our understanding of the atmosphere. With advancements in predictive analytics, personalized services, and climate change solutions, we are entering an era where weather forecasts are not just about what to expect tomorrow; they are about understanding our environment and making informed choices for a sustainable future. The question is, are we ready to embrace these changes?
- How does AI improve weather forecasting?
AI enhances weather forecasting by analyzing vast datasets, improving prediction models, and providing real-time updates, leading to more accurate forecasts. - What are personalized weather services?
Personalized weather services use AI to deliver tailored forecasts based on individual preferences and local conditions, making the information more relevant. - Can AI help with climate change?
Yes, AI can analyze climate data and provide insights that help inform policy decisions and promote sustainable practices.
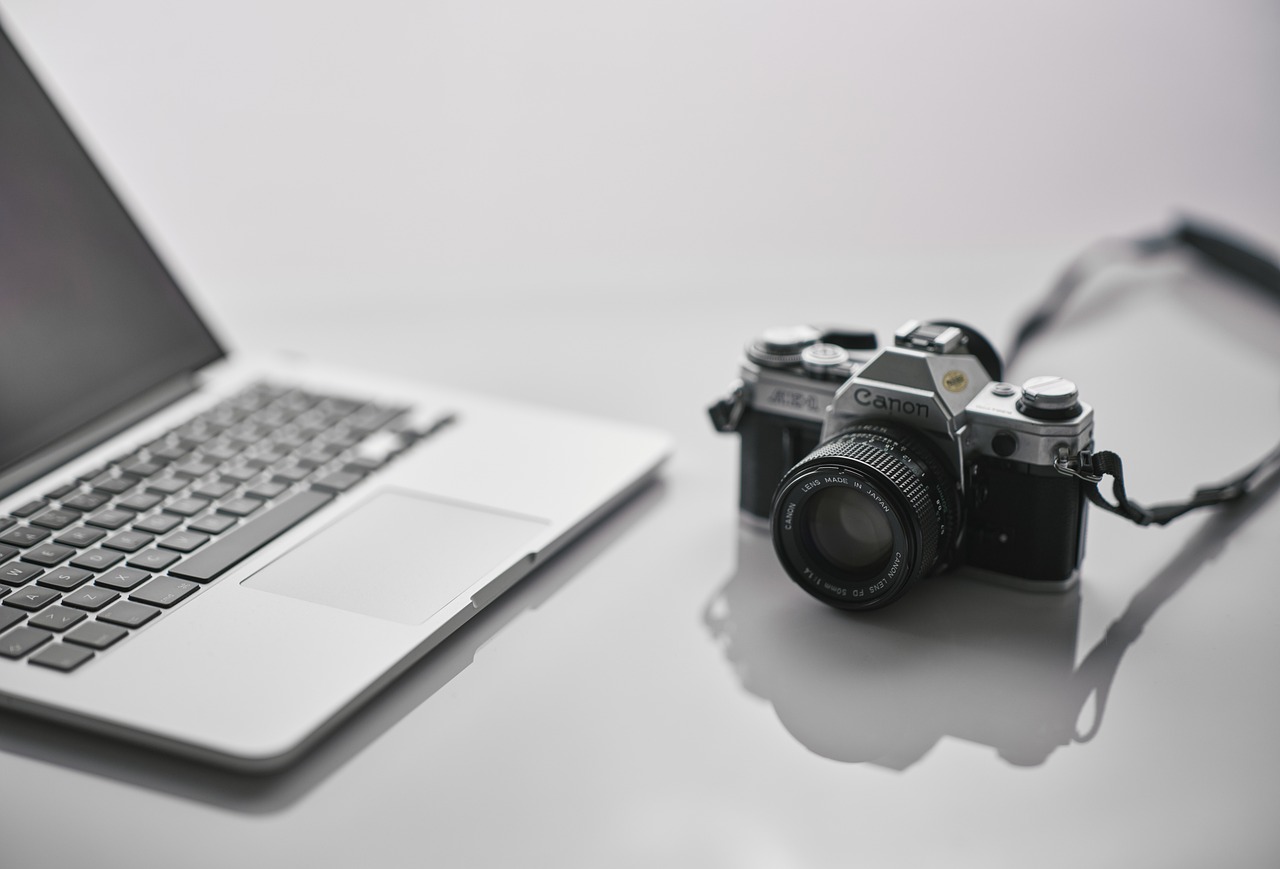
Personalized Weather Services
Imagine waking up in the morning and receiving a weather update that not only tells you about the day's forecast but also tailors its advice to your specific plans. This is the magic of , a groundbreaking application of artificial intelligence that is reshaping how we interact with weather data. Gone are the days of generic forecasts that apply to large regions; now, AI can provide hyper-localized predictions that consider your unique location, preferences, and activities.
At the heart of these personalized services is the ability of AI to analyze vast amounts of data quickly and efficiently. By integrating information from various sources—such as satellite imagery, sensor networks, and even social media trends—AI can create a comprehensive picture of weather patterns that is specific to you. For instance, if you’re planning a picnic in the park, AI can alert you to any sudden changes in weather, such as unexpected rain or temperature drops, ensuring you’re always prepared.
Moreover, the implications of personalized weather services extend beyond individual users. Businesses, particularly those in sectors like agriculture, logistics, and tourism, can leverage these tailored forecasts to make informed decisions. For example, farmers can receive alerts about optimal planting conditions or potential frost, while delivery companies can optimize routes based on real-time weather conditions. This not only enhances operational efficiency but also contributes to cost savings and improved customer satisfaction.
To illustrate how personalized weather services work, consider the following table that outlines the key features and benefits:
Feature | Benefit |
---|---|
Hyper-local forecasts | Accurate predictions tailored to your specific location. |
Real-time updates | Instant alerts for sudden weather changes, helping you stay informed. |
Activity-based recommendations | Customized advice based on your planned activities, ensuring you’re always prepared. |
Integration with smart devices | Seamless connectivity with home automation systems for proactive weather management. |
As we move forward, the potential for personalized weather services continues to expand. With advancements in AI and machine learning, we can expect even more sophisticated algorithms that not only predict weather patterns but also learn from user feedback. This means that the more you interact with your weather service, the better it will understand your preferences and needs. Imagine a system that knows you prefer sunny days for outdoor activities and automatically notifies you of upcoming sunny spells while advising you to stay indoors during storms.
In conclusion, personalized weather services represent a significant leap forward in how we experience weather forecasting. By harnessing the power of AI, we can enjoy a more tailored approach to weather information that enhances our daily lives and supports critical decision-making for businesses. As technology continues to evolve, the future of weather forecasting looks not only brighter but also more personalized than ever!
- What are personalized weather services? Personalized weather services use AI to provide tailored weather forecasts based on individual preferences and specific locations.
- How does AI improve weather forecasting? AI analyzes vast datasets, learns from patterns, and generates real-time updates, significantly enhancing forecasting accuracy.
- Can businesses benefit from personalized weather services? Yes, businesses can use tailored forecasts to optimize operations, reduce costs, and improve customer satisfaction by making informed decisions based on accurate weather predictions.
- What data sources are used for personalized weather services? These services typically use satellite imagery, ground-based sensor data, historical weather data, and user-generated data to create a comprehensive forecast.
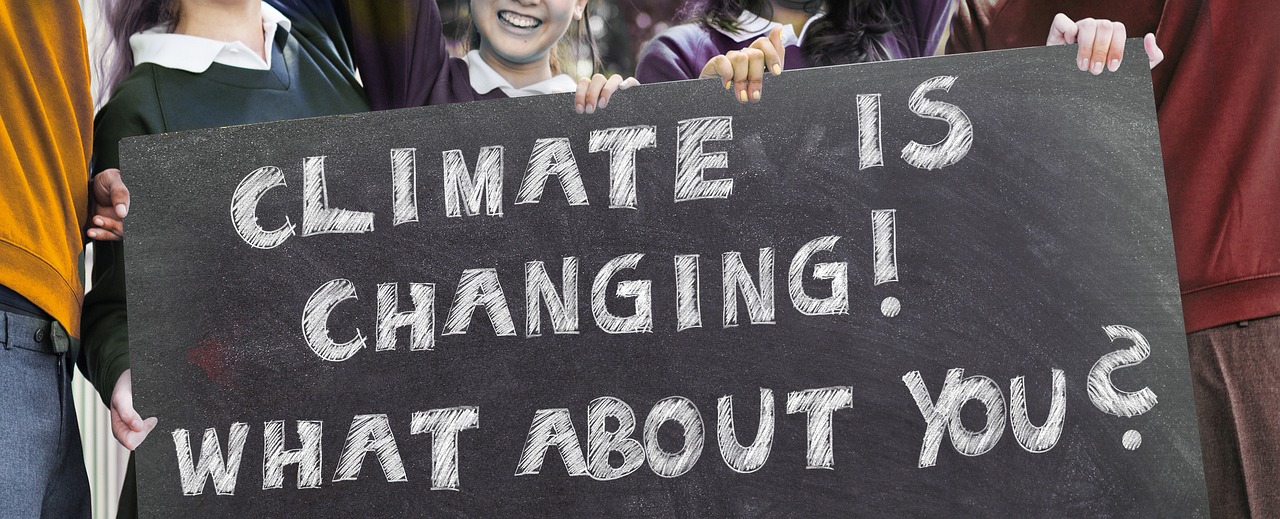
Climate Change and AI Solutions
As the world grapples with the daunting challenges posed by climate change, artificial intelligence emerges as a beacon of hope, offering innovative solutions that can significantly mitigate its impacts. The ability of AI to process vast amounts of data and identify patterns is transforming how we approach climate-related issues. Imagine AI as a powerful magnifying glass, allowing us to see the intricate details of climate trends that were previously hidden in the noise of data.
One of the most compelling applications of AI in combating climate change is its role in predictive analytics. By analyzing historical weather patterns, satellite imagery, and real-time sensor data, AI can forecast climate-related events with remarkable accuracy. This predictive capability enables governments and organizations to prepare for extreme weather events, such as hurricanes and floods, thereby saving lives and reducing economic losses. For instance, AI models can predict the likelihood of a flood in a specific area, allowing for timely evacuations and resource allocation.
Moreover, AI can optimize energy consumption, a critical factor in reducing carbon emissions. Through smart grids and energy management systems, AI analyzes consumption patterns and adjusts energy distribution in real-time. This not only enhances efficiency but also supports the integration of renewable energy sources, such as solar and wind. By predicting energy demand and supply fluctuations, AI helps utilities manage resources more effectively, reducing reliance on fossil fuels.
Another fascinating area where AI is making strides is in climate modeling. Traditional climate models often struggle with the complexity of interactions between various environmental factors. However, AI can simulate these interactions with greater precision, providing insights into future climate scenarios. This is crucial for policymakers who need to understand potential outcomes of their decisions. For example, AI-driven models can help predict how different carbon reduction strategies will impact global temperatures over the next few decades.
Furthermore, AI is instrumental in promoting sustainable practices across various sectors. In agriculture, for instance, AI systems analyze soil health, weather conditions, and crop patterns to recommend optimal planting times and resource usage. This not only maximizes yield but also minimizes environmental impact. By using data-driven approaches, farmers can reduce water consumption and chemical fertilizers, contributing to a more sustainable food system.
In summary, the intersection of climate change and AI solutions is a promising frontier. As we harness the power of AI, we can develop more effective strategies for mitigating the impacts of climate change, improving our resilience, and fostering sustainability. The journey is not without its challenges, but the potential benefits are too significant to ignore. As we continue to innovate and integrate AI into our climate strategies, we move closer to a more sustainable future.
- How does AI contribute to climate change solutions? AI enhances predictive analytics, optimizes energy consumption, and improves climate modeling, enabling better decision-making for climate action.
- Can AI help in disaster management related to climate change? Yes, AI can predict extreme weather events, allowing for timely evacuations and resource allocation to mitigate impacts.
- What role does AI play in sustainable agriculture? AI analyzes environmental data to recommend optimal planting times and resource usage, promoting sustainable farming practices.
- Are there any challenges in implementing AI for climate solutions? Yes, challenges include data quality, ethical considerations, and the need for interdisciplinary collaboration to maximize AI's potential.
Frequently Asked Questions
- How is AI improving weather forecasting?
AI enhances weather forecasting by analyzing vast amounts of data quickly and accurately. It uses machine learning algorithms to identify patterns in historical weather data, which leads to better predictions and real-time updates.
- What are some common machine learning techniques used in weather predictions?
Common techniques include neural networks and decision trees. Neural networks help in recognizing complex patterns, while decision trees simplify the decision-making process by visually representing weather variables.
- What role do neural networks play in predicting severe weather events?
Neural networks mimic human brain functions, allowing them to analyze complex datasets to predict severe weather events like storms and hurricanes. They improve accuracy by learning from past data and adapting to new information.
- What challenges do neural networks face in weather forecasting?
Neural networks can struggle with issues like overfitting, where they perform well on training data but poorly on new data. Data bias is another challenge that can skew predictions, but techniques exist to mitigate these issues.
- How does AI utilize satellite imagery for weather forecasting?
AI analyzes satellite imagery to detect weather patterns and changes. This analysis helps meteorologists understand large-scale weather phenomena and improve forecasting accuracy.
- Why is data quality important in AI weather forecasting?
High-quality data is essential for accurate predictions. Poor data can lead to incorrect forecasts, which can have serious consequences, especially in severe weather situations.
- What are personalized weather services, and how do they work?
Personalized weather services use AI to provide tailored forecasts based on individual preferences and locations. This means you can receive updates that are relevant to your specific needs, enhancing user experience and satisfaction.
- Can AI help in addressing climate change?
Yes, AI can play a significant role in tackling climate change by providing insights that inform policy decisions and promote sustainable practices. It can analyze trends and predict future climate scenarios, aiding in effective climate management.