Innovations in AI-assisted Healthcare Algorithms
In recent years, the integration of artificial intelligence (AI) into healthcare has revolutionized the way we approach medical treatment and patient care. These innovations in AI-assisted healthcare algorithms are not just technical advancements; they are fundamentally transforming the healthcare landscape, enhancing both the efficiency of medical operations and the quality of patient outcomes. Imagine a world where algorithms can predict diseases before symptoms even arise, or where personalized treatment plans are crafted with the help of comprehensive data analysis. This is not science fiction; it is the reality we are stepping into.
AI algorithms are designed to analyze vast amounts of data, drawing insights that human practitioners might overlook. For instance, through machine learning and deep learning techniques, these algorithms can identify patterns in patient data that help doctors make informed decisions faster than ever before. One of the most exciting aspects of this innovation is the ability to harness predictive analytics. By anticipating patient needs and potential health issues, healthcare providers can proactively manage care, leading to better health outcomes and reduced costs.
Moreover, the role of AI in healthcare extends beyond just diagnostics and treatment. It streamlines operations, reducing administrative burdens and allowing healthcare professionals to focus more on patient care rather than paperwork. This shift not only enhances the patient experience but also improves job satisfaction for healthcare workers. As we delve deeper into the specifics of these innovations, we will uncover how technologies like natural language processing and data mining are being utilized to enhance efficiency and effectiveness in healthcare settings.
However, with these advancements come challenges and ethical considerations that must not be overlooked. Issues surrounding patient privacy, data security, and algorithmic bias are critical discussions that the healthcare community must engage in as we embrace these technologies. It's essential to ensure that as we innovate, we also uphold the principles of fairness and transparency in our healthcare systems. As we explore further, we will examine the development techniques behind these algorithms and the ethical implications they carry.
- What are AI-assisted healthcare algorithms?
These are advanced computational models that analyze healthcare data to improve patient outcomes, streamline operations, and enhance diagnostic accuracy. - How does machine learning contribute to healthcare?
Machine learning algorithms can predict patient outcomes, tailor treatment plans, and analyze medical data to enhance diagnostic processes. - What ethical considerations are associated with AI in healthcare?
Key issues include patient privacy, data security, and the potential for bias in algorithmic decision-making. - What is predictive analytics?
Predictive analytics refers to the use of data, statistical algorithms, and machine learning techniques to identify the likelihood of future outcomes based on historical data.
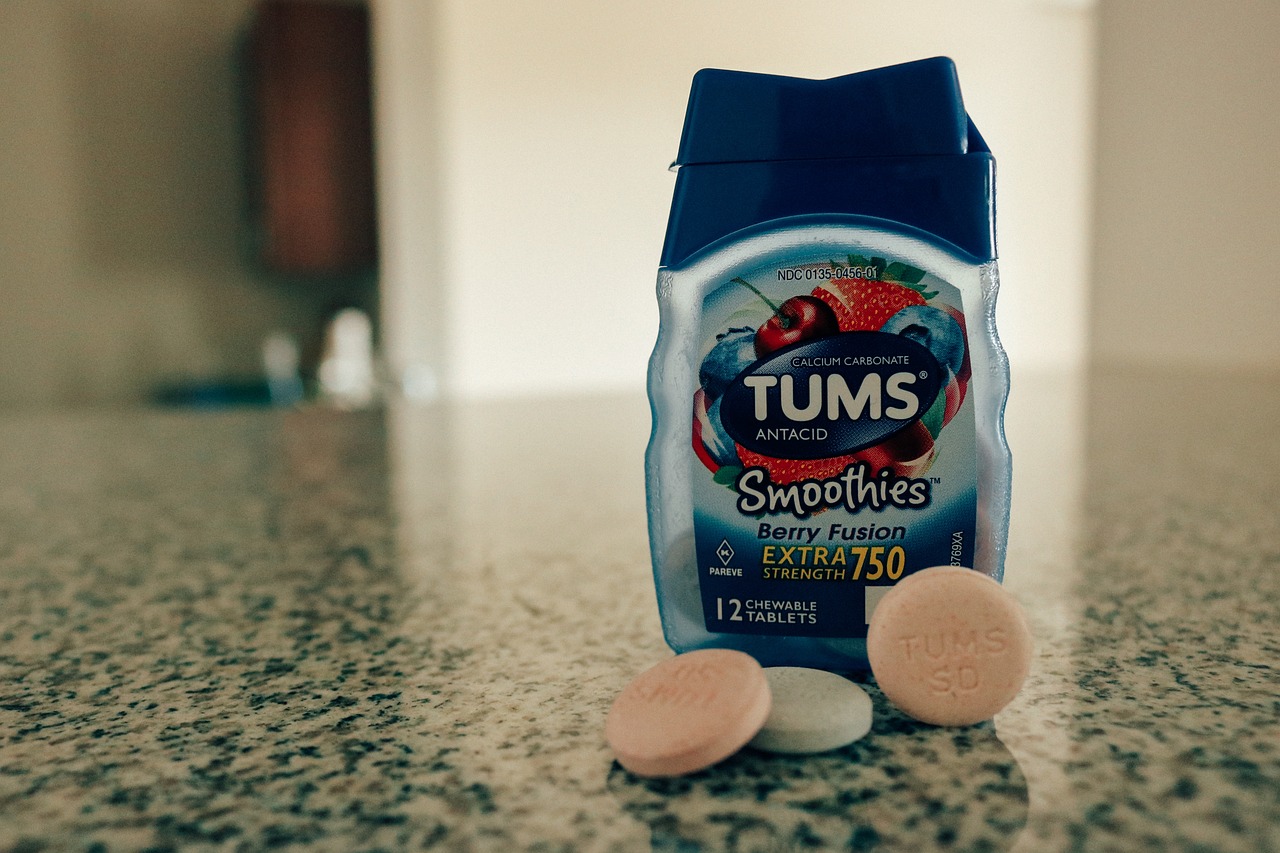
Understanding AI in Healthcare
Artificial Intelligence (AI) is not just a buzzword anymore; it’s a transformative force reshaping the healthcare landscape. Imagine walking into a hospital where algorithms analyze your health data in real-time, predicting potential issues before they arise. This is not science fiction; this is the reality we’re stepping into. AI technologies are being integrated into various aspects of healthcare, enhancing the way providers deliver care and how patients experience it.
At its core, AI in healthcare leverages vast amounts of data to make informed decisions quickly and accurately. From diagnosing diseases to managing patient records, AI systems are designed to assist healthcare professionals in making better choices. For instance, consider the use of AI in radiology. Algorithms can analyze medical images with incredible precision, often spotting anomalies that a human eye might miss. This capability not only speeds up the diagnostic process but also increases the accuracy of results, ultimately leading to better patient outcomes.
Moreover, AI's potential to personalize treatment plans is a game changer. By analyzing data from various sources, including genetic information, lifestyle choices, and historical health records, AI can help in crafting tailored treatment strategies for individuals. This level of customization means that patients receive care that is more aligned with their specific needs, enhancing the effectiveness of treatments and improving overall satisfaction.
But the benefits of AI extend beyond just clinical applications. It also streamlines operations within medical facilities. For example, AI can optimize scheduling, manage inventory, and even predict patient admissions, allowing healthcare providers to allocate resources more efficiently. This not only reduces operational costs but also ensures that patients receive timely care.
As we delve deeper into the world of AI in healthcare, it’s essential to recognize the various applications it encompasses. Here are some key areas where AI is making a significant impact:
- Diagnostics: AI algorithms are being trained to identify diseases from images and lab results, improving diagnostic accuracy.
- Predictive Analytics: By analyzing trends and patterns, AI can forecast patient outcomes and potential health risks.
- Patient Engagement: AI-driven chatbots and virtual assistants are enhancing communication between healthcare providers and patients.
In summary, the integration of AI into healthcare is revolutionizing the industry. It’s empowering providers with tools that enhance decision-making, streamline operations, and personalize patient care. As we continue to explore the potential of AI, it’s clear that its applications will only expand, leading to a future where healthcare is more efficient, effective, and patient-centered.
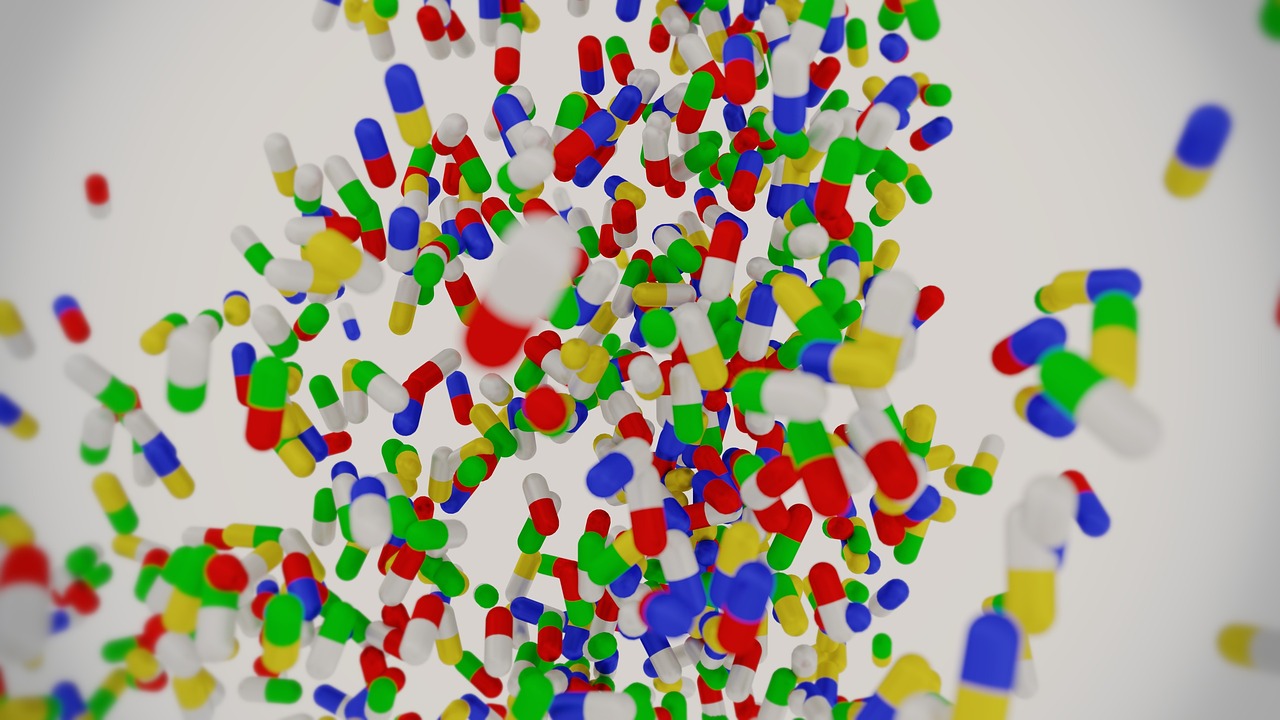
Algorithm Development Techniques
In the rapidly evolving field of healthcare, the development of AI algorithms is at the forefront of innovation. These algorithms are crafted using a variety of sophisticated techniques that enable them to process vast amounts of data, recognize patterns, and make predictions that can significantly enhance patient care. The core methods employed in the development of these algorithms include machine learning, deep learning, and natural language processing. Each of these techniques brings its own unique strengths to the table, contributing to the overall effectiveness of AI in healthcare settings.
Machine learning, for instance, is a subset of AI that focuses on the development of algorithms that can learn from and make predictions based on data. It operates under the premise that systems can automatically improve their performance through experience. This is particularly beneficial in healthcare, where the ability to analyze large datasets can lead to improved patient outcomes. By utilizing machine learning, healthcare providers can harness predictive analytics to anticipate patient needs, optimize treatment plans, and enhance diagnostic accuracy. For example, algorithms can analyze historical patient data to predict which individuals are at higher risk for certain conditions, allowing for early intervention.
Deep learning, on the other hand, is a more advanced form of machine learning that uses neural networks with many layers (hence "deep") to analyze various types of data, including images, text, and sound. In the context of healthcare, deep learning has revolutionized the analysis of medical images. By training algorithms on thousands of images, these systems can learn to identify subtle patterns that may be missed by the human eye. This capability is particularly useful in fields like radiology, where early and accurate detection of conditions such as tumors can make a significant difference in patient outcomes.
Natural language processing (NLP) is another crucial technique that allows AI systems to understand and interpret human language. In healthcare, NLP can be employed to analyze clinical notes, research papers, and patient feedback, transforming unstructured data into actionable insights. For instance, NLP algorithms can sift through thousands of patient records to identify trends or common symptoms, which can inform treatment protocols and improve overall care.
To illustrate the differences and applications of these techniques, consider the following table:
Technique | Description | Applications in Healthcare |
---|---|---|
Machine Learning | Algorithms that learn from data to make predictions. | Predicting patient outcomes, personalizing treatment plans. |
Deep Learning | Advanced neural networks for complex data analysis. | Medical imaging analysis, identifying diseases from scans. |
Natural Language Processing | Understanding and interpreting human language. | Analyzing clinical notes, extracting insights from patient feedback. |
Each of these techniques plays a pivotal role in enhancing the capabilities of AI algorithms in healthcare. By integrating these approaches, developers can create more robust systems that not only improve efficiency but also deliver better patient care. The future of healthcare is undoubtedly intertwined with these technological advancements, and as we continue to refine these algorithms, we can expect even greater improvements in patient outcomes and operational efficiency.
- What is the primary benefit of AI algorithms in healthcare? AI algorithms improve diagnostic accuracy, personalize treatment plans, and enhance patient outcomes.
- How does machine learning differ from deep learning? Machine learning focuses on learning from data, while deep learning uses layered neural networks for more complex data analysis.
- What role does natural language processing play in healthcare? NLP helps analyze and interpret unstructured data, such as clinical notes and patient feedback, to extract valuable insights.
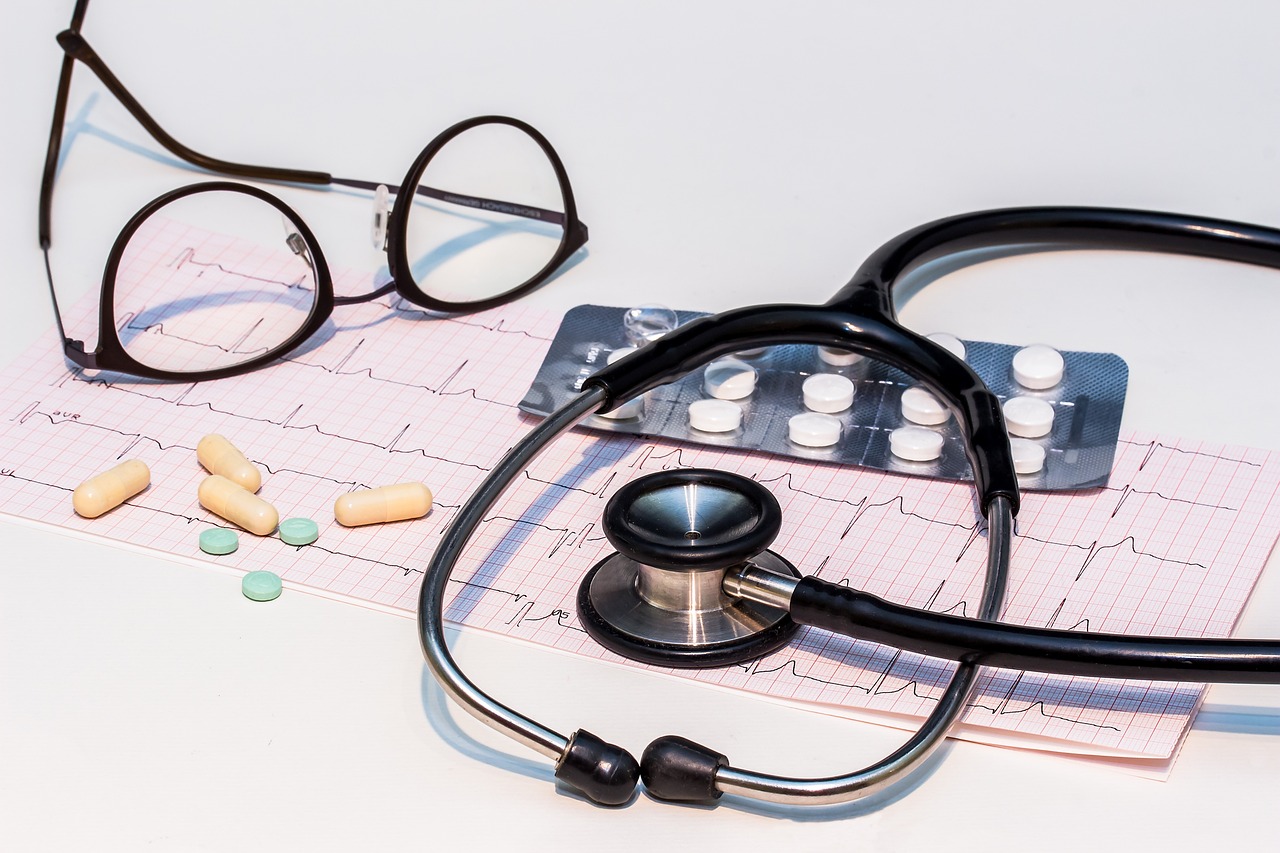
Machine Learning Applications
When we talk about machine learning in healthcare, we're diving into a world where data meets innovation, creating a symbiotic relationship that enhances patient care. Imagine a scenario where a computer can analyze thousands of patient records in the blink of an eye, identifying patterns and predicting outcomes that even the most seasoned doctors might miss. This is the power of machine learning, and it's revolutionizing how we approach healthcare.
One of the most exciting applications of machine learning is in predicting patient outcomes. By analyzing historical data, these algorithms can forecast how a patient might respond to a particular treatment or even predict the likelihood of complications. For instance, if a patient has a certain set of symptoms and medical history, the algorithm can compare this data against a vast database of similar cases, providing insights that help healthcare providers make informed decisions.
Moreover, machine learning is instrumental in personalizing treatment plans. Every patient is unique, and with machine learning, healthcare providers can tailor treatments based on individual characteristics rather than a one-size-fits-all approach. This not only improves patient satisfaction but also enhances the effectiveness of treatments. For example, if a patient is diagnosed with diabetes, machine learning algorithms can analyze their lifestyle, genetic background, and other health data to recommend a customized diet and exercise plan.
Another remarkable application is in the realm of diagnostic accuracy. Machine learning algorithms can sift through mountains of data, including lab results, medical images, and patient histories, to assist doctors in making diagnoses. This is particularly beneficial in fields like radiology, where algorithms can detect anomalies in medical images with a level of precision that rivals human experts. By reducing the chances of misdiagnosis, machine learning not only saves lives but also optimizes treatment pathways.
To give you a clearer picture, consider the following table that highlights some key applications of machine learning in healthcare:
Application | Description | Benefits |
---|---|---|
Predictive Analytics | Forecasting patient outcomes and potential complications. | Proactive decision-making and improved patient management. |
Personalized Treatment | Customizing treatment plans based on individual patient data. | Enhanced treatment effectiveness and patient satisfaction. |
Diagnostic Support | Assisting in diagnosing conditions via data analysis. | Increased diagnostic accuracy and reduced misdiagnosis rates. |
In summary, the applications of machine learning in healthcare are vast and varied, from improving patient outcomes to enhancing operational efficiency. As we continue to harness the power of data, we can look forward to a future where healthcare is not only more effective but also more personalized. The question remains: how far can we go with this technology? The possibilities are endless, and with each advancement, we move closer to a healthcare system that is truly tailored to the needs of every individual.
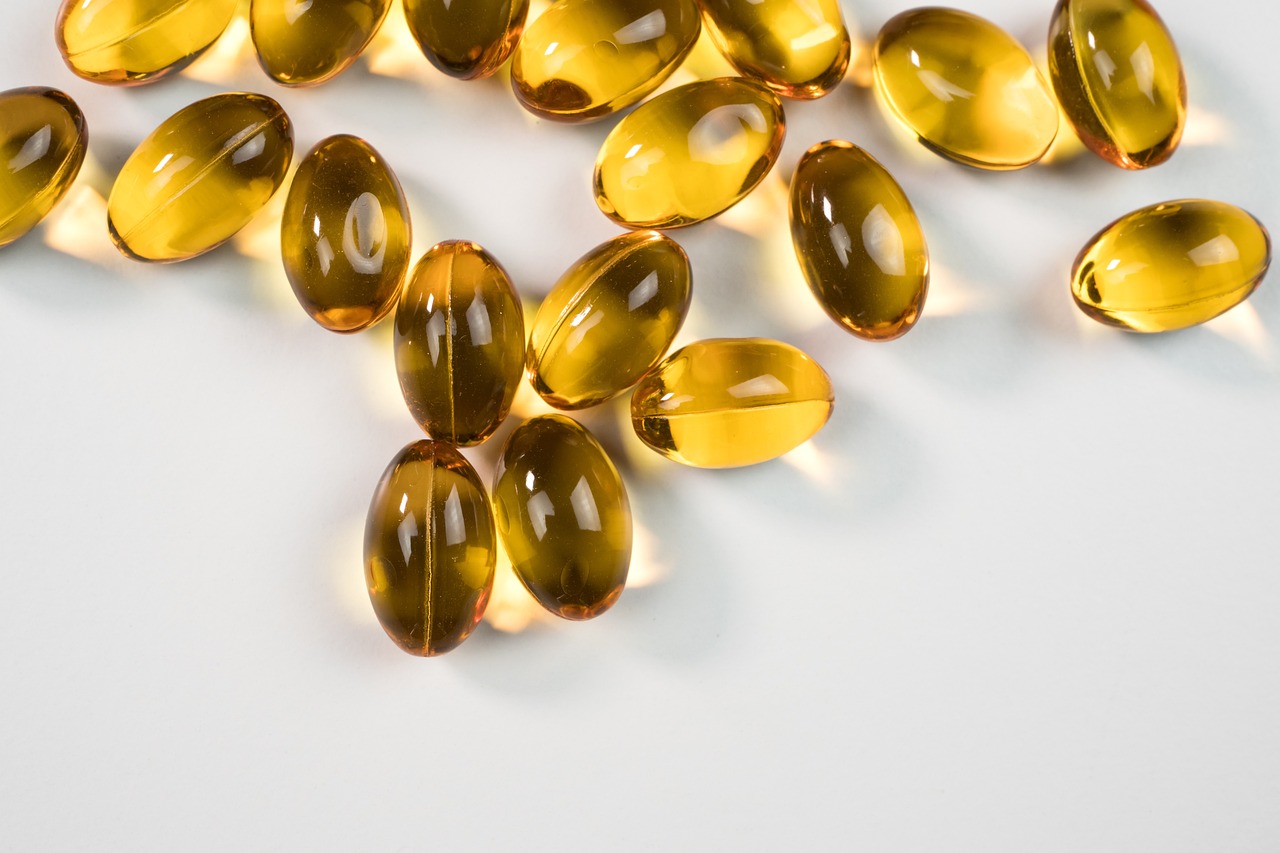
Predictive Analytics
Predictive analytics is rapidly becoming a game-changer in the healthcare industry, enabling providers to harness the power of data to forecast patient needs and improve overall outcomes. By analyzing historical data, predictive models can identify trends and patterns that inform clinical decision-making. Imagine having a crystal ball that not only sees into the future but also helps healthcare professionals make informed choices today. This is the essence of predictive analytics in healthcare.
At its core, predictive analytics utilizes a variety of data sources, including electronic health records (EHRs), patient demographics, and even social determinants of health. By integrating these diverse datasets, healthcare providers can create a comprehensive view of their patients, leading to more accurate predictions. For instance, hospitals can anticipate patient admissions during flu season by analyzing historical admission rates and current health trends, allowing them to allocate resources more efficiently.
Here's how predictive analytics can transform healthcare:
- Forecasting Disease Outbreaks: By analyzing data from various sources, predictive analytics can identify early signs of disease outbreaks, allowing healthcare systems to respond swiftly and effectively.
- Improving Patient Admissions: Predictive models can forecast patient admissions based on historical data, helping hospitals manage staffing and resources effectively.
- Enhancing Treatment Responses: By examining previous treatment outcomes, predictive analytics can help tailor treatment plans to individual patients, increasing the likelihood of successful outcomes.
Moreover, predictive analytics can identify at-risk patients who may require additional support or intervention. For example, algorithms can analyze factors such as medication adherence, lifestyle choices, and previous health issues to flag patients who might be at risk for chronic diseases. This proactive approach not only improves patient care but also reduces healthcare costs by preventing complications before they arise.
However, the implementation of predictive analytics is not without its challenges. Data quality and integration remain significant hurdles. If the data fed into predictive models is incomplete or inaccurate, the predictions can lead to misguided decisions. Therefore, ensuring high-quality, standardized data is crucial for the success of these analytics.
In conclusion, predictive analytics is revolutionizing how healthcare providers approach patient care. By leveraging data to anticipate future needs, healthcare professionals can not only enhance patient outcomes but also streamline operations, ultimately leading to a more efficient healthcare system. The future of healthcare is bright, and predictive analytics is at the forefront of this transformation.
- What is predictive analytics in healthcare? Predictive analytics in healthcare refers to the use of data analysis techniques to predict future patient outcomes and trends based on historical data.
- How can predictive analytics improve patient care? By forecasting potential health issues and treatment responses, predictive analytics allows healthcare providers to tailor interventions and allocate resources more effectively.
- What challenges does predictive analytics face? Key challenges include data quality, integration, and the need for standardized practices to ensure accurate predictions.
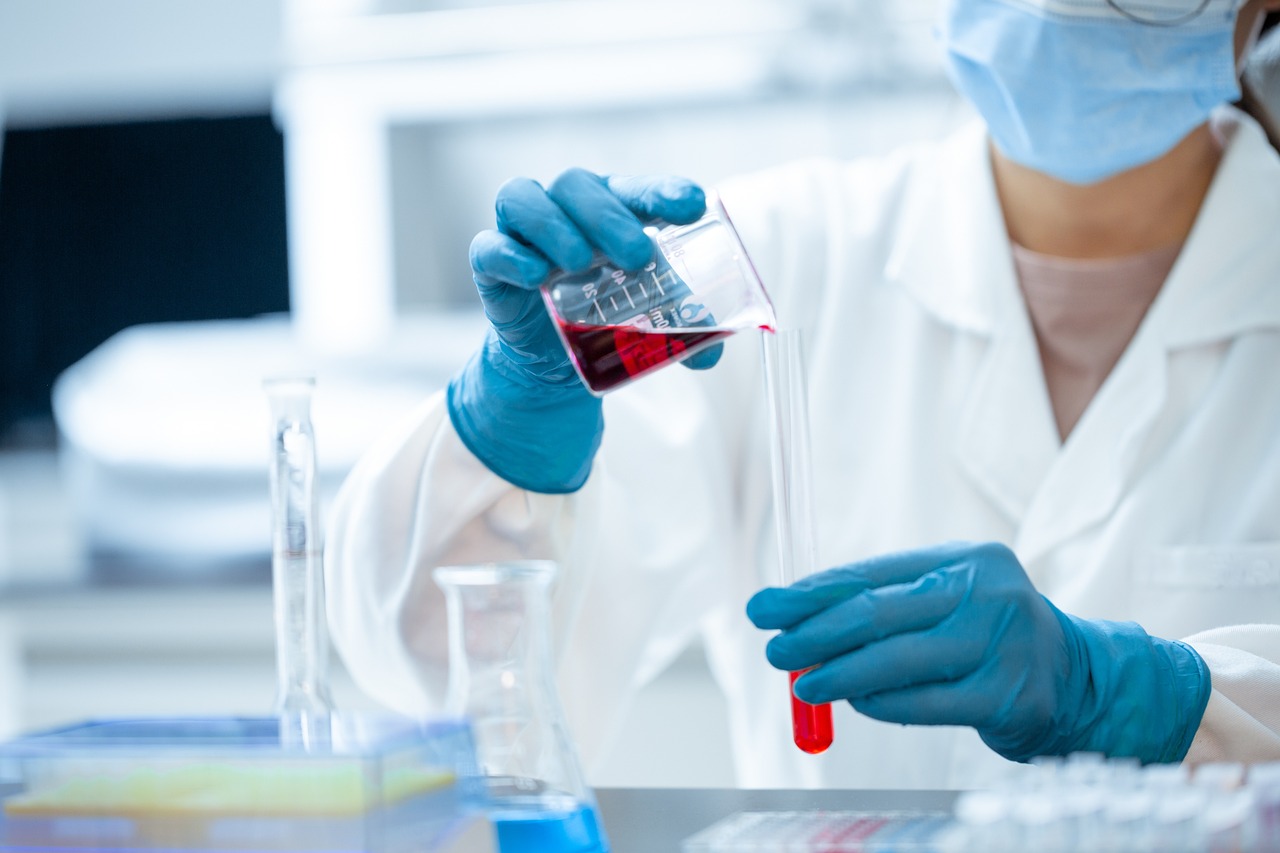
Data Mining Techniques
Data mining techniques have become a cornerstone of modern healthcare, enabling providers to sift through vast amounts of data to uncover actionable insights. Imagine trying to find a needle in a haystack; that's what healthcare professionals face when analyzing patient data without the right tools. By employing advanced data mining methods, they can transform seemingly chaotic information into structured knowledge that enhances patient care and operational efficiency.
One of the most powerful aspects of data mining in healthcare is its ability to identify patterns and trends that may not be immediately obvious. For instance, by analyzing historical patient data, hospitals can predict which patients are at higher risk for certain conditions, allowing for early intervention. This proactive approach not only improves patient outcomes but also reduces overall healthcare costs.
Some of the key data mining techniques utilized in healthcare include:
- Classification: This technique involves categorizing data into predefined classes. In healthcare, this could mean classifying patients based on their likelihood of developing a particular disease.
- Clustering: Clustering groups similar data points together. For example, hospitals might use clustering to identify groups of patients with similar health conditions, enabling tailored treatment plans.
- Regression: Regression analysis helps to predict outcomes based on historical data. This is particularly useful for forecasting patient recovery times or the effectiveness of specific treatments.
- Association Rule Learning: This technique identifies relationships between variables in large datasets. For instance, it can reveal that patients with certain symptoms are more likely to have specific diagnoses.
Moreover, the integration of big data analytics with electronic health records (EHRs) has significantly enhanced the effectiveness of data mining techniques. Healthcare providers can now access comprehensive patient histories, treatment outcomes, and demographic information, allowing for a more holistic view of patient health. This integration not only streamlines operations but also fosters a culture of continuous improvement within healthcare organizations.
To illustrate the impact of data mining techniques, consider the following table that highlights some common applications and their benefits:
Data Mining Technique | Application in Healthcare | Benefits |
---|---|---|
Classification | Predicting disease risk | Early intervention and prevention |
Clustering | Identifying patient groups | Customized treatment plans |
Regression | Forecasting recovery times | Efficient resource allocation |
Association Rule Learning | Understanding symptom-disease relationships | Improved diagnostic accuracy |
In conclusion, data mining techniques are revolutionizing the way healthcare providers approach patient care. By leveraging these methods, they can make informed decisions, enhance treatment efficacy, and ultimately improve patient outcomes. As technology continues to evolve, the potential for data mining in healthcare will only grow, paving the way for a more data-driven future.
Q1: What is data mining in healthcare?
A1: Data mining in healthcare refers to the process of analyzing large datasets to discover patterns, trends, and relationships that can improve patient care and operational efficiency.
Q2: How does data mining improve patient outcomes?
A2: By identifying risk factors and predicting potential health issues, data mining allows healthcare providers to intervene early, leading to better patient outcomes.
Q3: What are some common data mining techniques used in healthcare?
A3: Common techniques include classification, clustering, regression, and association rule learning, each serving different purposes in analyzing patient data.
Q4: Can data mining be applied to electronic health records?
A4: Absolutely! The integration of data mining techniques with electronic health records allows for comprehensive analysis, leading to improved insights and patient care.
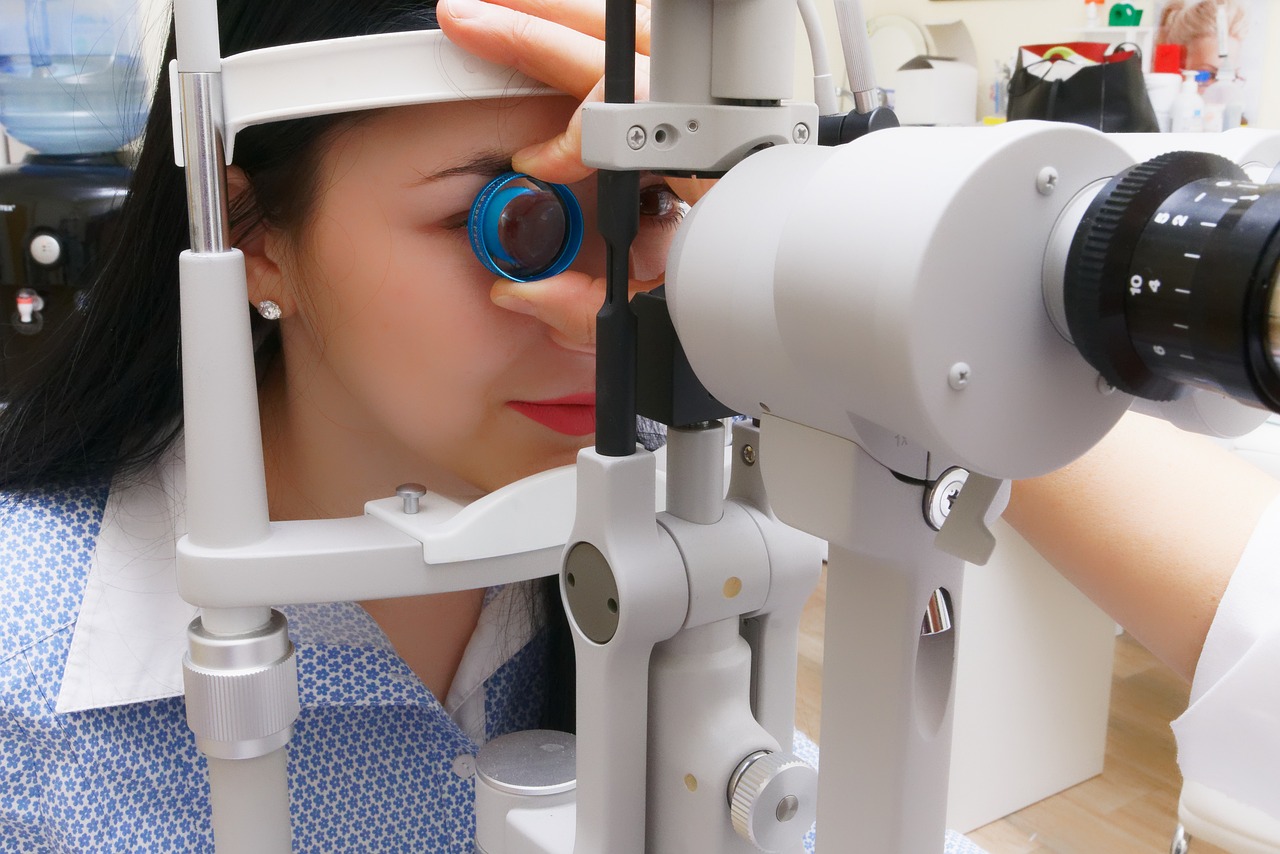
Deep Learning in Medical Imaging
Deep learning has emerged as a revolutionary force in the realm of medical imaging, significantly enhancing the way healthcare professionals interpret and analyze medical images. Imagine the traditional methods of examining X-rays, MRIs, or CT scans, where radiologists would spend hours scrutinizing images for signs of abnormalities. Now, with the advent of deep learning algorithms, this process is not only faster but also more accurate. These algorithms are designed to learn from vast amounts of data, allowing them to identify patterns that may be imperceptible to the human eye.
At the core of deep learning are neural networks, which mimic the way our brains process information. When applied to medical imaging, these networks can be trained on thousands, if not millions, of images to recognize various conditions. For example, a deep learning model can be trained to detect tumors in mammograms with a level of precision that rivals, and sometimes surpasses, that of experienced radiologists. This capability not only improves diagnostic accuracy but also has the potential to reduce the time it takes to arrive at a diagnosis.
One of the most exciting aspects of deep learning in medical imaging is its ability to continuously improve. As more data becomes available, these algorithms can be retrained to enhance their performance, making them increasingly effective over time. This is particularly important in a field like healthcare, where new diseases and conditions emerge regularly. By leveraging deep learning, healthcare providers can stay ahead of the curve, ensuring that they are equipped with the most current and effective diagnostic tools.
Moreover, deep learning is not limited to just one type of imaging modality. It has shown promise across various platforms, including:
- X-rays: Detecting fractures, infections, and tumors.
- CT Scans: Identifying internal bleeding and organ anomalies.
- MRIs: Analyzing soft tissue conditions such as brain tumors and spinal injuries.
- Ultrasounds: Monitoring fetal development and diagnosing abdominal issues.
However, while the potential of deep learning in medical imaging is vast, it does come with its own set of challenges. One significant concern is the interpretability of these algorithms. Healthcare professionals often need to understand how a model arrived at a particular conclusion, especially when it comes to life-altering diagnoses. This calls for ongoing research into making these deep learning models more transparent and explainable.
Additionally, the integration of deep learning technologies into existing healthcare systems requires careful planning and execution. Healthcare providers must ensure that they have the necessary infrastructure to support these advanced algorithms, including robust data storage solutions and high-performance computing capabilities. As such, collaboration between technology developers and healthcare organizations is essential to facilitate a smooth transition.
In conclusion, deep learning in medical imaging is not just a passing trend; it represents a significant leap forward in how we approach diagnosis and treatment in healthcare. By harnessing the power of these advanced algorithms, we can improve patient outcomes, streamline workflows, and ultimately save lives. As we continue to explore the capabilities of deep learning, the future of medical imaging looks incredibly promising.
Q1: How does deep learning improve medical imaging?
A1: Deep learning enhances medical imaging by allowing algorithms to learn from vast datasets, improving diagnostic accuracy and speeding up the analysis process.
Q2: What types of medical images can deep learning analyze?
A2: Deep learning can analyze various types of medical images, including X-rays, CT scans, MRIs, and ultrasounds, making it versatile across different diagnostic modalities.
Q3: Are there any challenges associated with deep learning in medical imaging?
A3: Yes, challenges include the need for interpretability of algorithms and the requirement for robust infrastructure to support these technologies in healthcare settings.
Q4: How can deep learning contribute to better patient outcomes?
A4: By improving diagnostic accuracy and enabling faster decision-making, deep learning can lead to earlier interventions and more personalized treatment plans for patients.
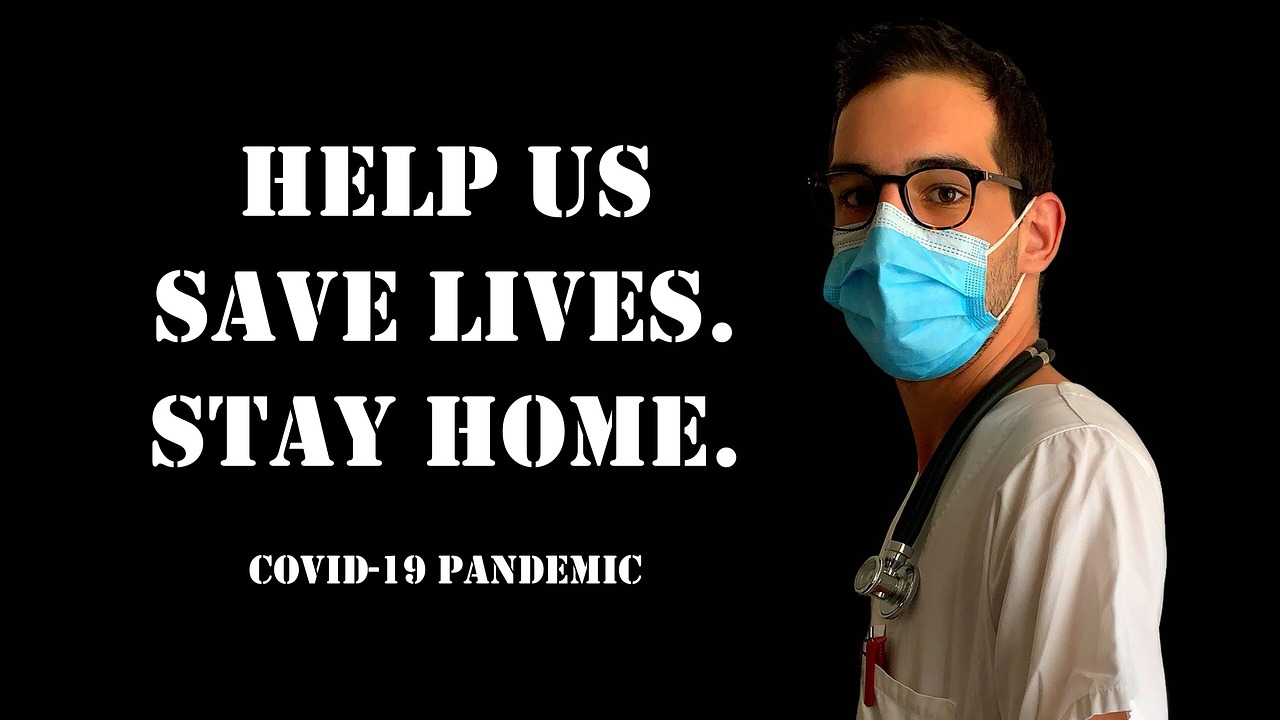
Ethical Considerations
As we dive deeper into the realm of AI-assisted healthcare, it becomes increasingly crucial to address the ethical implications that accompany these technological advancements. While the potential benefits of AI in healthcare are vast, they come with a set of responsibilities that cannot be ignored. We must navigate the complex waters of patient privacy, data security, and algorithmic transparency to ensure that these innovations serve all patients equitably and justly.
One of the primary ethical concerns revolves around patient privacy. With the integration of AI, vast amounts of personal health data are collected and analyzed. This raises questions about who has access to this sensitive information and how it is used. Are patients fully informed about how their data is being utilized? Are they given the option to opt-out? These questions are not just theoretical; they are critical to maintaining trust between healthcare providers and patients. The importance of informed consent cannot be overstated in this context.
Moreover, data security is another pressing concern. As more healthcare systems adopt AI technologies, the risk of data breaches increases. Cybersecurity threats can compromise sensitive patient information, leading to devastating consequences. The healthcare industry must prioritize robust security measures to protect patient data from unauthorized access and potential misuse. This includes implementing advanced encryption techniques and regular security audits to safeguard information.
Transparency in algorithmic decision-making is another ethical pillar that deserves attention. When AI systems make treatment recommendations or diagnostic decisions, patients and healthcare providers alike should understand the basis of these recommendations. If a patient is denied a treatment option, they have the right to know why. This is where the concept of algorithmic accountability becomes essential. Developers and healthcare organizations must strive to create algorithms that are not only effective but also interpretable and explainable.
Additionally, we need to address the issue of bias in algorithms. AI systems learn from data, and if that data is skewed or unrepresentative, the outcomes can be problematic. For instance, if an algorithm is trained primarily on data from one demographic group, it may not perform well for others, leading to unequal treatment. This bias can perpetuate existing disparities in healthcare, making it imperative for developers to use diverse datasets and continuously monitor algorithms for fairness.
Finally, navigating the regulatory landscape is crucial for ensuring ethical AI deployment in healthcare. As the technology evolves, so too must the regulations governing its use. This includes establishing standardized practices to ensure compliance and protect patient rights. Regulatory bodies must work closely with AI developers and healthcare providers to create frameworks that encourage innovation while safeguarding ethical principles.
In conclusion, as we embrace the incredible potential of AI in healthcare, we must remain vigilant about the ethical implications that accompany these advancements. By prioritizing patient privacy, data security, transparency, and fairness, we can harness the power of AI responsibly and equitably, ultimately leading to better health outcomes for all.
- What are the main ethical concerns regarding AI in healthcare? The primary concerns include patient privacy, data security, algorithmic transparency, bias in algorithms, and regulatory challenges.
- How can healthcare providers ensure patient privacy with AI? By implementing strict data protection measures, obtaining informed consent, and being transparent about data usage.
- What is algorithmic bias and why is it a concern? Algorithmic bias occurs when AI systems produce unfair outcomes due to skewed training data, potentially leading to unequal treatment for different patient demographics.
- What role do regulations play in AI healthcare ethics? Regulations help ensure compliance, protect patient rights, and establish standardized practices for the ethical use of AI technologies in healthcare.
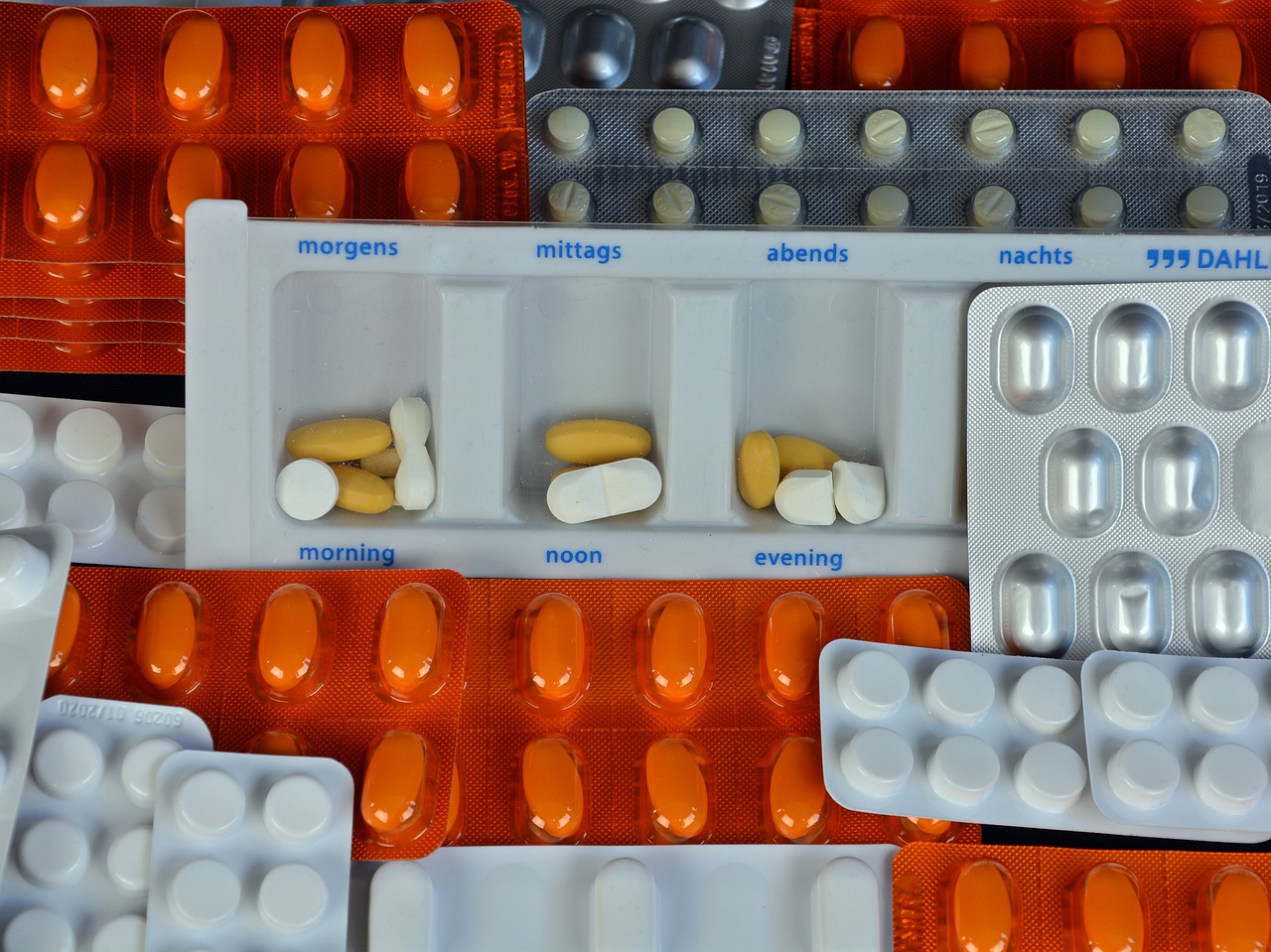
Bias in Algorithms
In the rapidly evolving landscape of AI in healthcare, has emerged as a significant concern that cannot be overlooked. When we think about algorithms, we often envision cold, hard data crunching away to deliver unbiased results. However, the reality is far more complex. Algorithms are only as good as the data they are trained on, and if that data is skewed or incomplete, the outcomes can lead to serious consequences for patient care.
Consider this: if an algorithm is trained primarily on data from a specific demographic, it may perform exceptionally well for that group while simultaneously failing to deliver accurate predictions or recommendations for others. This situation can result in unequal treatment and healthcare disparities, where certain populations might receive subpar care simply because the algorithm wasn't designed with their needs in mind. For instance, a machine learning model developed using data predominantly from young, urban populations might not be effective when applied to older, rural patients.
To illustrate the impact of bias, let’s take a closer look at some of the potential sources of bias in healthcare algorithms:
- Data Collection: If the data collected is not representative of the entire patient population, the algorithm will inevitably reflect those gaps.
- Feature Selection: The criteria used to select features can introduce bias. For example, if socioeconomic status is included as a feature, it may inadvertently disadvantage certain groups.
- Historical Bias: Algorithms trained on historical data may perpetuate existing inequalities in healthcare access and treatment.
Addressing bias in algorithms is not just a technical challenge but also a moral imperative. Developers and healthcare providers must work collaboratively to ensure that the algorithms used in clinical settings are fair, transparent, and inclusive. This means employing diverse datasets that accurately represent the populations they serve and continuously monitoring the algorithms for any signs of bias in their outputs.
Moreover, regulatory bodies are beginning to recognize the importance of addressing bias in AI. They are advocating for guidelines that require transparency in how algorithms are created and tested, as well as regular audits to identify and mitigate biases. The goal is to create a more equitable healthcare system where AI can be a tool for enhancing patient care rather than a source of disparity.
In conclusion, while AI has the potential to revolutionize healthcare, we must remain vigilant about the biases that can creep into algorithms. By continually refining the data we use and ensuring diverse representation, we can harness the power of AI to improve healthcare outcomes for everyone, regardless of their background.
- What is algorithmic bias? Algorithmic bias refers to systematic and unfair discrimination in the outcomes produced by algorithms, often due to biased data or flawed design.
- How can we mitigate bias in healthcare algorithms? Mitigation strategies include using diverse datasets, implementing regular audits, and fostering collaboration between developers and healthcare professionals.
- Why is bias in algorithms a concern for patient care? Bias can lead to unequal treatment and healthcare disparities, negatively affecting patient outcomes and trust in healthcare systems.
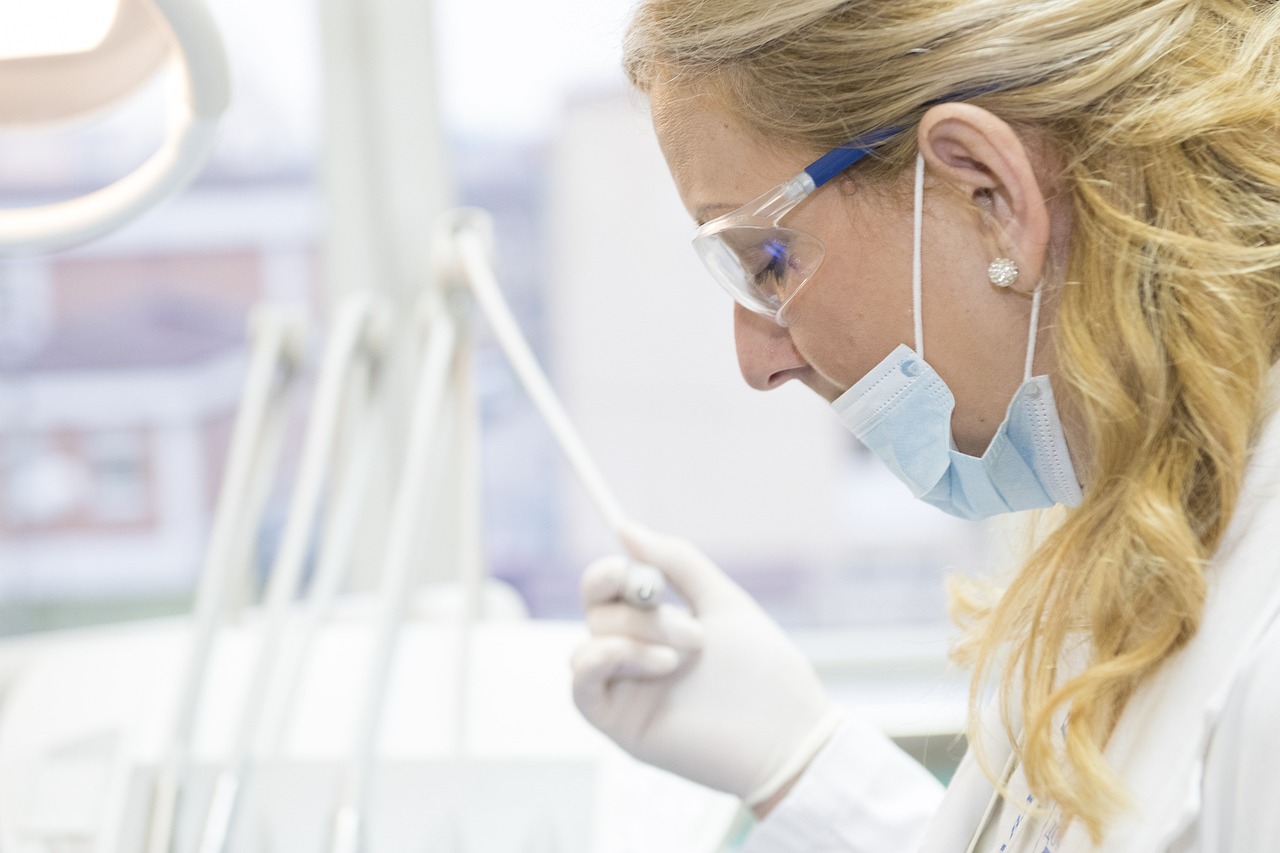
Regulatory Challenges
As the integration of artificial intelligence (AI) into healthcare accelerates, so do the that accompany it. Navigating the complex landscape of regulations is akin to traversing a labyrinth; one wrong turn can lead to significant setbacks, not just for developers but also for healthcare providers and patients. The rapid pace of technological advancement often outstrips the ability of regulatory bodies to create frameworks that ensure safety, efficacy, and fairness in AI applications.
One of the primary challenges is the lack of standardized regulations across different regions and countries. While some jurisdictions have begun to formulate guidelines, others lag behind, leading to a patchwork of laws that can create confusion and inconsistency. This inconsistency can hinder the development and deployment of AI solutions, as companies may be uncertain about compliance requirements. For instance, the FDA in the United States has established some pathways for AI device approval, but these are not universally adopted, leading to disparities in how AI technologies are vetted and implemented globally.
Furthermore, the question of accountability looms large. If an AI system makes a mistake, such as misdiagnosing a condition, who is held responsible? Is it the healthcare provider, the software developer, or the institution that implemented the technology? This ambiguity complicates the regulatory landscape and raises concerns about patient safety. To address these issues, regulatory bodies must work collaboratively with AI developers and healthcare professionals to establish clear guidelines that delineate responsibilities and ensure transparency in AI decision-making processes.
Another significant concern is data privacy and security. AI systems often rely on vast amounts of patient data to function effectively. However, this data must be handled with the utmost care to protect patient privacy. Regulations such as HIPAA in the U.S. set stringent standards for data protection, but the evolving nature of AI technologies means that these regulations must also evolve. There is a pressing need for regulations that can adapt to new technologies while ensuring that patient confidentiality is maintained.
Moreover, the potential for bias in AI algorithms poses another regulatory hurdle. If not properly addressed, biased algorithms can lead to unequal treatment outcomes across different demographics. Regulatory frameworks must include provisions for bias detection and mitigation to ensure that AI systems are fair and equitable. This involves ongoing monitoring and evaluation of AI systems post-deployment, which can be resource-intensive and complex.
In summary, while AI holds immense promise for revolutionizing healthcare, the regulatory challenges it presents cannot be overlooked. It is essential for stakeholders to engage in a continuous dialogue to create a regulatory environment that fosters innovation while protecting patients. Only through collaboration can we hope to navigate these challenges effectively and harness the full potential of AI in healthcare.
- What are the main regulatory challenges associated with AI in healthcare?
The main challenges include a lack of standardized regulations, accountability issues, data privacy concerns, and the potential for algorithmic bias. - How can regulatory bodies ensure the safety of AI technologies?
By establishing clear guidelines for development, implementation, and ongoing monitoring of AI systems, regulatory bodies can enhance safety and efficacy. - Why is bias in AI algorithms a concern?
Bias can lead to unequal treatment outcomes among different patient demographics, which is why it is crucial for regulations to include provisions for bias detection and mitigation.
Frequently Asked Questions
- What is AI-assisted healthcare?
AI-assisted healthcare refers to the integration of artificial intelligence technologies in medical settings to enhance patient care, streamline operations, and improve health outcomes. This includes using algorithms for diagnostics, treatment recommendations, and operational efficiencies.
- How do AI algorithms improve patient outcomes?
AI algorithms analyze vast amounts of data to identify patterns and insights that can lead to better diagnosis and treatment plans. By personalizing care based on individual patient data, these algorithms help healthcare providers make informed decisions that ultimately enhance patient outcomes.
- What are the main techniques used in AI algorithm development?
The primary techniques include machine learning, deep learning, and natural language processing. Each of these techniques plays a crucial role in processing data, making predictions, and improving the accuracy of healthcare applications.
- Can you explain predictive analytics in healthcare?
Predictive analytics involves using historical data to forecast future events, such as disease outbreaks or patient admissions. This proactive approach allows healthcare providers to allocate resources effectively and make timely interventions, ultimately improving patient care.
- What role does deep learning play in medical imaging?
Deep learning enhances the analysis of medical images by enabling algorithms to learn from large datasets of images. This results in more accurate diagnostics, as these algorithms can detect subtle patterns that might be missed by human eyes, leading to better treatment plans.
- What are the ethical concerns surrounding AI in healthcare?
Ethical concerns include patient privacy, data security, and the need for transparency in how algorithms make decisions. It's essential to ensure that AI systems are designed to protect sensitive patient information and that their decision-making processes are understandable to both providers and patients.
- How can bias in AI algorithms affect patient care?
Bias in AI algorithms can lead to unequal treatment outcomes for different patient demographics. If an algorithm is trained on biased data, it may produce skewed results that disadvantage certain groups, highlighting the importance of using diverse datasets in algorithm development.
- What are the regulatory challenges for AI in healthcare?
The regulatory landscape for AI in healthcare is complex, with challenges including ensuring compliance with existing laws and the need for standardized practices. As AI technologies evolve, regulators must adapt to ensure patient safety and ethical use of these tools.