Machine Learning Vs AI: Clearing the Confusion
In today's tech-driven world, the terms Artificial Intelligence (AI) and Machine Learning (ML) are often thrown around interchangeably, leading to a whirlwind of confusion. But let’s take a moment to clear the air. Imagine AI as the grand umbrella under which various technologies reside, and ML as one of those exciting, innovative tools that help make AI smarter. So, what exactly does this mean? Let’s dive deeper into the fascinating realms of AI and ML, exploring their definitions, applications, and how they intertwine to shape our modern lives.
Artificial Intelligence is like the brain of a robot, designed to mimic human intelligence. It encompasses a range of technologies that allow machines to perform tasks that typically require human cognitive functions, such as learning, reasoning, and problem-solving. Imagine having a personal assistant who can understand your requests, learn from your habits, and make decisions on your behalf—that’s AI in action! From voice recognition systems like Siri and Alexa to sophisticated algorithms that power self-driving cars, AI is transforming industries at an unprecedented rate.
Now, let’s zoom in on Machine Learning. Think of it as the nervous system of AI, where algorithms are trained to learn from and make predictions based on data. Unlike traditional programming, where rules are hard-coded, ML allows systems to improve over time through experience. For instance, consider how Netflix recommends shows based on what you’ve watched. It uses ML algorithms to analyze your viewing habits and suggest content you might love. This ability to learn from data is what makes ML a game-changer in the tech landscape.
So, how do AI and ML relate to each other? Picture AI as the vast ocean and ML as a river flowing through it. ML is a subset of AI that enhances its capabilities by enabling machines to learn from data rather than relying solely on pre-programmed rules. Without ML, AI would lack the ability to adapt and evolve. This relationship is crucial: ML algorithms provide the tools that allow AI systems to become more intelligent and efficient, leading to better outcomes in various applications.
Machine Learning can be categorized into three main types, each with its unique characteristics:
- Supervised Learning: This type involves training a model on a labeled dataset, where the input data is paired with the correct output. It’s like teaching a child with flashcards—showing them the answer helps them learn!
- Unsupervised Learning: Here, the model works with unlabeled data, trying to find patterns and relationships on its own. Think of it as exploring a new city without a map—you discover hidden gems along the way!
- Reinforcement Learning: This type involves training algorithms to make decisions through trial and error, receiving feedback from their actions. It’s akin to teaching a dog new tricks—rewarding them when they get it right encourages them to keep trying!
AI technology is not just a distant concept; it’s woven into the fabric of our daily lives. From virtual assistants that help manage our schedules to recommendation systems that suggest products based on our preferences, AI is everywhere! Here are a few examples that illustrate its impact:
- Smart Home Devices: Thermostats that learn your habits to optimize energy use.
- Healthcare: AI algorithms that assist in diagnosing diseases by analyzing medical images.
- Finance: Fraud detection systems that learn to identify suspicious transactions.
Despite their incredible advancements, both AI and ML face significant challenges. Issues such as ethical concerns and data privacy are at the forefront of discussions among developers and policymakers. As we integrate these technologies into our lives, it’s crucial to address these challenges to ensure responsible and fair development. For instance, how do we ensure that AI systems are not biased? What measures can we take to protect sensitive data? These questions are vital for the future of AI and ML.
Looking ahead, the future of AI and ML is brimming with possibilities. As technology continues to evolve, we can expect significant advancements in areas such as natural language processing and computer vision. These developments will not only enhance the capabilities of AI but also open new avenues for innovation across various sectors.
However, with great power comes great responsibility. The rise of AI and ML raises important questions about job displacement and the creation of new roles. While some fear that machines will take over jobs, others argue that these technologies will create new opportunities that we can’t yet imagine. Striking a balance between technological advancement and workforce adaptation is crucial for a sustainable future.
As AI and ML evolve, ethical considerations become increasingly important. Developers and organizations must prioritize responsibility and transparency in their AI initiatives. This means ensuring that AI systems are designed to be fair, accountable, and aligned with societal values. After all, the future of AI is not just about technology; it’s about how we choose to use it to enhance our lives.
Question | Answer |
---|---|
What is the main difference between AI and ML? | AI is the broader concept of machines mimicking human intelligence, while ML is a subset of AI focused on learning from data. |
How does Machine Learning work? | ML algorithms learn from data by identifying patterns and making predictions without being explicitly programmed for each task. |
What are some real-world applications of AI? | AI is used in virtual assistants, recommendation systems, healthcare diagnostics, and fraud detection, among others. |

Understanding Artificial Intelligence
Artificial Intelligence (AI) is not just a buzzword; it's a revolutionary concept that mimics human intelligence through machines. Imagine a world where computers can think, learn, and make decisions—this is the essence of AI. At its core, AI encompasses a variety of technologies and methodologies that allow machines to perform tasks that typically require human intelligence. These tasks can range from simple data analysis to complex problem-solving scenarios, and the implications are vast. AI is transforming industries at an unprecedented rate, reshaping how we interact with technology and each other.
To better grasp the intricacies of AI, it's essential to understand its core components. Generally, AI can be categorized into two main types: Narrow AI and General AI. Narrow AI, also known as weak AI, is designed to perform a specific task, such as facial recognition or language translation. On the other hand, General AI, or strong AI, refers to a theoretical form of AI that possesses the ability to understand, learn, and apply intelligence across a wide range of tasks, much like a human. While we have made significant strides in Narrow AI, achieving General AI remains a challenge that researchers are actively exploring.
AI applications are everywhere in our daily lives, often in ways we may not even realize. For instance, when you receive personalized recommendations on streaming services or when virtual assistants like Siri or Alexa respond to your queries, you are interacting with AI. These systems analyze vast amounts of data to recognize patterns and make informed decisions, enhancing user experience and efficiency.
Moreover, AI's impact extends beyond consumer technology. In healthcare, AI algorithms analyze medical data to assist in diagnosing diseases, while in finance, they detect fraudulent transactions in real-time. The automotive industry is also witnessing a revolution with the advent of self-driving cars, which rely heavily on AI to navigate and make decisions on the road. The possibilities are endless, and as AI continues to evolve, its applications will only expand.
However, with great power comes great responsibility. As AI technologies become more integrated into our lives, ethical considerations emerge. Questions about data privacy, bias in algorithms, and the potential for misuse must be addressed to ensure that AI serves humanity positively. As we delve deeper into the world of AI, it's crucial to foster a dialogue around these issues, ensuring that the technology develops responsibly and ethically.
In summary, understanding AI is essential to grasping its potential and implications in our society. As we continue to innovate and push the boundaries of what machines can do, we must remain vigilant about the ethical considerations and strive for a future where AI enhances our lives without compromising our values.
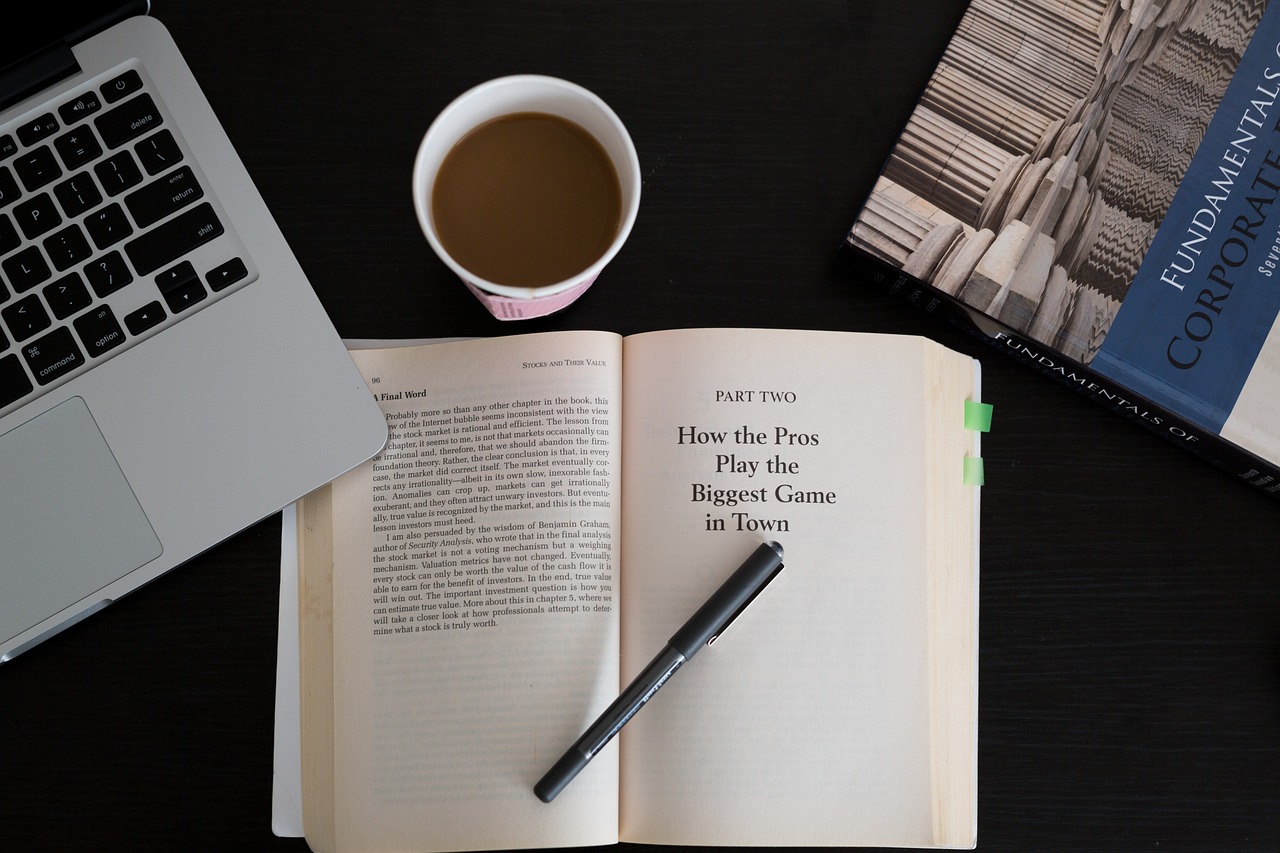
Defining Machine Learning
Machine Learning (ML) is often hailed as the backbone of modern Artificial Intelligence (AI). But what exactly does it mean? At its core, Machine Learning is a subset of AI that focuses on the development of algorithms and statistical models that enable computers to perform specific tasks without explicit instructions. Instead of being programmed with a fixed set of rules, ML systems learn from data, identifying patterns and making decisions based on the information they process. This is akin to teaching a child how to recognize objects by showing them numerous examples, rather than merely telling them what those objects are.
In the realm of ML, there are several key principles that guide its functionality. One of the most important is the concept of training data. This is the dataset used to train the ML model, allowing it to learn and make predictions. The quality and quantity of this data can significantly impact the model's performance. Just like a student who studies well will likely perform better on an exam, a machine that learns from diverse and high-quality data will yield more accurate results.
Another essential aspect of ML is the distinction between supervised and unsupervised learning. In supervised learning, the model is trained on labeled data, meaning the input data is paired with the correct output. For example, if you wanted to train a model to recognize cats in images, you would provide it with thousands of images labeled as either 'cat' or 'not cat'. On the other hand, unsupervised learning allows the model to find patterns and relationships in data without any labeled responses. Imagine a group of friends trying to sort themselves into groups based on shared interests without any prior knowledge of each other's preferences.
In addition to supervised and unsupervised learning, there is also reinforcement learning, where an agent learns to make decisions by receiving rewards or penalties based on its actions. This method is often likened to training a pet; when the pet performs a desired behavior, it receives a treat, reinforcing that action for the future. This technique has gained traction in various applications, from robotics to gaming, where agents learn to navigate complex environments.
Real-world applications of machine learning are vast and varied. From healthcare—where ML algorithms assist in diagnosing diseases by analyzing medical images—to finance, where they help detect fraudulent transactions, the potential of ML is enormous. In the realm of e-commerce, personalized recommendations based on user behavior have transformed the way consumers shop, making the experience more tailored and engaging.
To summarize, defining Machine Learning involves understanding its role as a powerful tool within the broader AI landscape. It revolves around algorithms that learn from data, enabling machines to perform tasks that traditionally required human intelligence. By harnessing the principles of supervised, unsupervised, and reinforcement learning, ML continues to revolutionize industries and reshape our daily lives.
- What is the difference between AI and Machine Learning? AI is the broader concept of machines being able to carry out tasks in a way that we would consider "smart", while ML is a specific subset of AI that focuses on learning from data.
- Can Machine Learning operate without human intervention? Yes, once a machine learning model is trained, it can make predictions or decisions without human input, although it often requires ongoing maintenance and updates.
- What are some common tools used in Machine Learning? Popular tools include TensorFlow, PyTorch, and Scikit-learn, which provide frameworks for developing ML models.
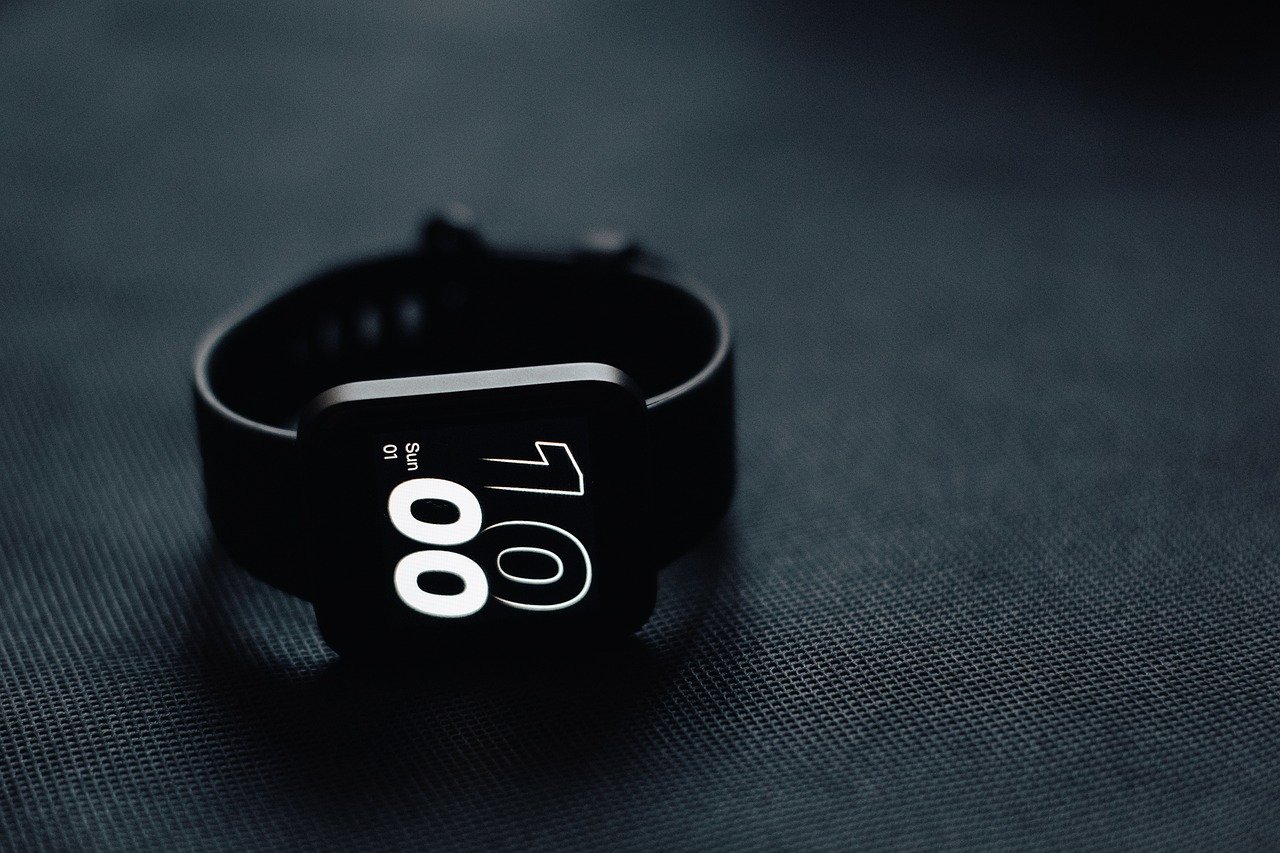
The Relationship Between AI and ML
When diving into the world of technology, you might find yourself tangled in the web of terms like Artificial Intelligence (AI) and Machine Learning (ML). While they often get tossed around interchangeably, understanding the relationship between them is crucial. Think of AI as the umbrella term that encompasses various technologies aimed at mimicking human intelligence, while ML is one of the sparkling jewels hanging from that umbrella. In other words, all ML is AI, but not all AI is ML.
To put it simply, AI is the broader concept of machines being able to carry out tasks in a way that we would consider “smart.” This includes everything from natural language processing to robotics. On the other hand, ML is a specific approach to achieving AI, using data and algorithms to allow machines to learn from experiences. Picture AI as a vast ocean, with ML being one of the many streams that flow into it, each contributing to the overall ecosystem of intelligent behavior.
As we delve deeper into this relationship, we see that ML enhances AI capabilities significantly. For instance, AI systems that incorporate ML can analyze vast amounts of data, identify patterns, and make predictions with a level of accuracy that traditional programming simply can't achieve. This capability is what makes applications like self-driving cars and personalized recommendations possible.
To illustrate the relationship further, let’s look at a few examples of how ML acts as a catalyst for AI advancements:
- Natural Language Processing: ML algorithms help AI understand and generate human language, making applications like chatbots and virtual assistants more effective.
- Image Recognition: AI systems powered by ML can identify objects, faces, and even emotions in images, revolutionizing fields like healthcare and security.
- Predictive Analytics: Businesses use ML to analyze customer behavior and predict future trends, enhancing decision-making processes.
The synergy between AI and ML is not just about enhancing existing technologies; it’s also about paving the way for future innovations. As ML techniques evolve, they enable AI systems to become more adaptable and efficient, creating a feedback loop that fosters continuous improvement. This interdependence is why many experts believe that the future of technology lies in the harmonious integration of AI and ML.
However, while the relationship between AI and ML is exciting, it also comes with its own set of challenges. As ML algorithms become more complex, the need for transparency and interpretability in AI systems grows. Understanding how these systems arrive at their conclusions is essential, especially in sensitive areas like healthcare and criminal justice. The dialogue around this relationship is ongoing, and as both fields evolve, so too will our understanding of their interplay.
In conclusion, the relationship between AI and ML is a dynamic one, characterized by mutual enhancement. As we continue to explore this fascinating realm, it’s clear that ML will remain a pivotal player in the development of AI technologies, shaping the way we interact with machines and redefining the boundaries of what’s possible.
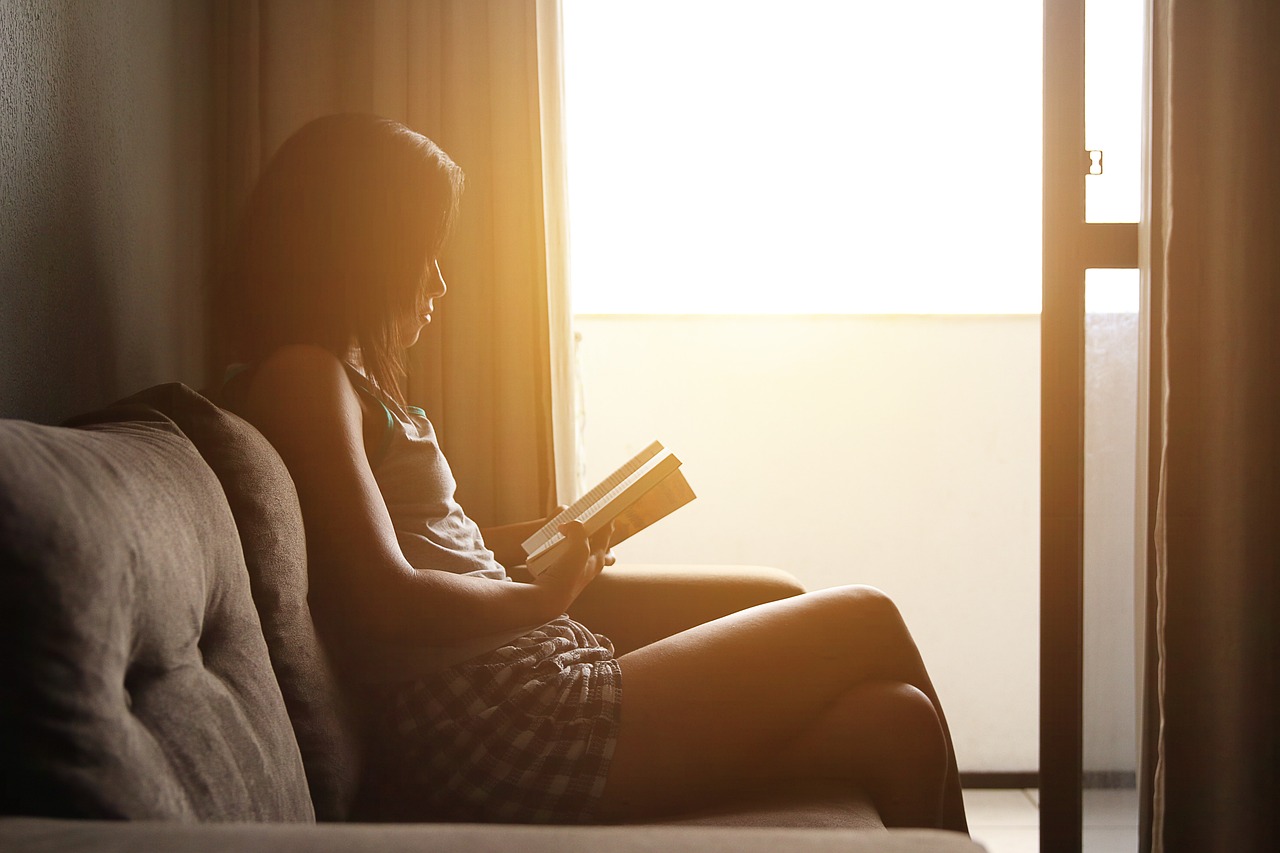
Types of Machine Learning
When diving into the fascinating world of Machine Learning (ML), it’s essential to understand that it’s not a one-size-fits-all concept. Instead, ML can be categorized into three primary types: supervised learning, unsupervised learning, and reinforcement learning. Each of these categories has its unique characteristics and applications, which we'll explore in detail.
Supervised learning is like having a personal tutor guiding you through your studies. In this method, the algorithm is trained on a labeled dataset, which means that each training example is paired with an output label. Imagine teaching a child to recognize animals by showing them pictures of cats and dogs, along with the corresponding names. This approach allows the model to learn from the input-output pairs and make predictions on new, unseen data. Common algorithms used in supervised learning include linear regression, decision trees, and support vector machines.
On the other hand, unsupervised learning is akin to exploring a new city without a map. Here, the algorithm is given data without any labels or predefined categories. The goal is to uncover hidden patterns or structures within the data. For example, consider a scenario where a retailer analyzes customer purchasing behavior without prior knowledge of their preferences. Using unsupervised learning techniques like clustering or dimensionality reduction, the retailer can identify distinct customer segments and tailor marketing strategies accordingly. This type of learning is particularly useful for tasks like anomaly detection and market basket analysis.
Lastly, we have reinforcement learning, which can be compared to training a pet with rewards and punishments. In this model, an agent learns to make decisions by interacting with its environment and receiving feedback in the form of rewards or penalties. For instance, think of a robot navigating a maze: it receives positive reinforcement for reaching the end and negative feedback for hitting walls. Over time, the robot learns the best path to take. Reinforcement learning has gained traction in various fields, including robotics, game playing, and autonomous vehicles.
To summarize, here’s a quick comparison of the three types of Machine Learning:
Type | Description | Example Applications |
---|---|---|
Supervised Learning | Learning from labeled data to predict outcomes. | Spam detection, image classification |
Unsupervised Learning | Finding hidden patterns in unlabeled data. | Customer segmentation, anomaly detection |
Reinforcement Learning | Learning through trial and error to maximize rewards. | Game AI, robotic control |
In conclusion, understanding the different types of Machine Learning is crucial for anyone looking to harness its power effectively. Whether you’re developing predictive models, uncovering insights from data, or training intelligent agents, knowing which approach to use can make all the difference in achieving your goals.
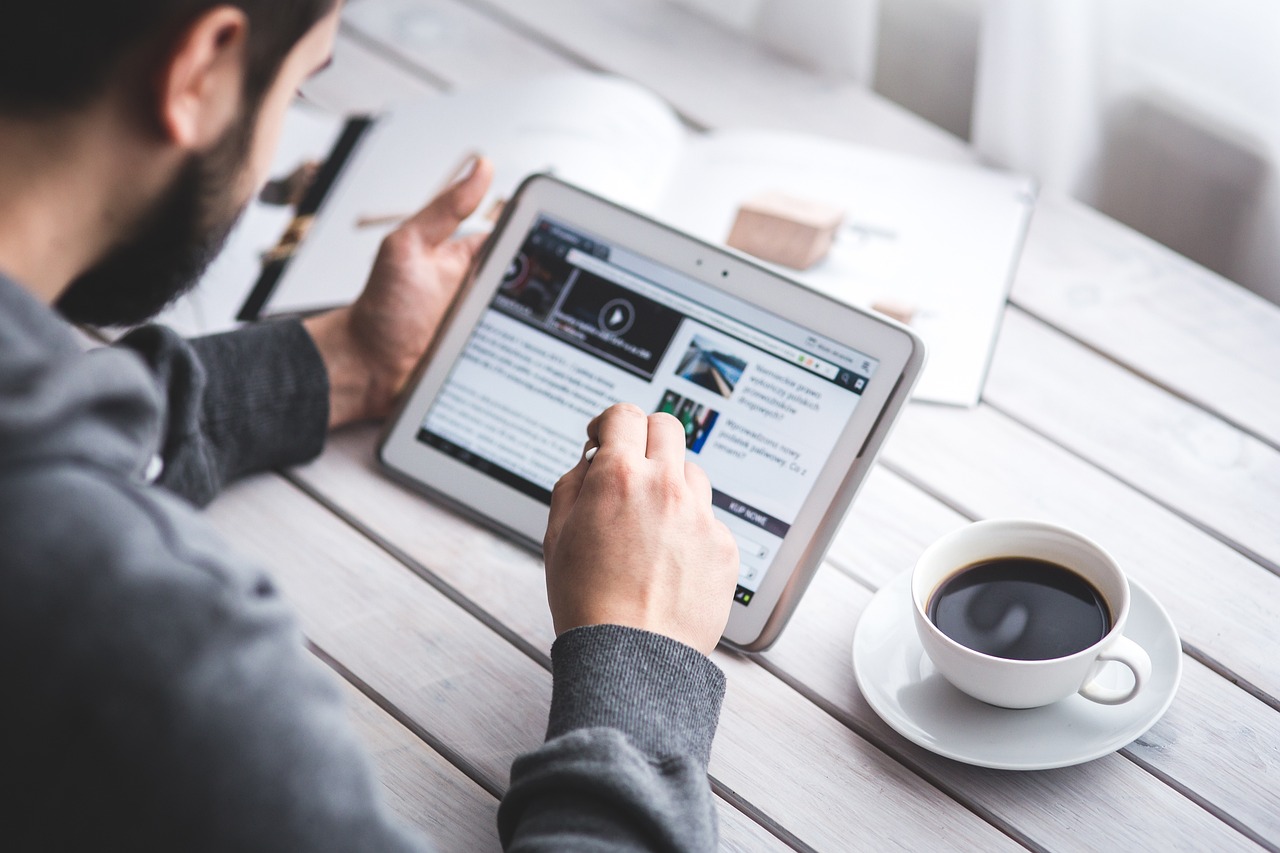
Applications of AI in Daily Life
Artificial Intelligence has seamlessly woven itself into the fabric of our daily lives, often without us even realizing it. From the moment we wake up to the sound of a smart alarm clock to the time we wind down with our favorite streaming service, AI is there, enhancing our experiences and making our lives easier. It’s like having a personal assistant who knows your preferences and habits, always ready to help you navigate through your day.
One of the most visible applications of AI is in virtual assistants like Siri, Alexa, and Google Assistant. These AI-powered tools respond to our voice commands, helping us with everything from setting reminders to controlling smart home devices. Imagine asking your assistant to play your favorite playlist or to dim the lights without lifting a finger. It's almost like magic, but it's all thanks to AI!
Another significant area where AI shines is in recommendation systems. Whether you’re scrolling through Netflix, browsing Amazon, or checking out Spotify, AI algorithms analyze your past behavior to suggest content that you might enjoy. This personalized touch not only enhances user experience but also keeps us engaged, leading to longer viewing times and increased customer satisfaction. It’s as if the platform knows you better than you know yourself!
Moreover, AI plays a crucial role in the realm of healthcare. From predictive analytics that help doctors diagnose diseases to AI-powered chatbots that provide medical advice, the impact of AI in healthcare is profound. For instance, AI algorithms can analyze medical images with incredible accuracy, assisting radiologists in detecting anomalies that might be missed by the human eye. This not only improves patient outcomes but also streamlines the workflow in medical facilities.
In the world of finance, AI is transforming how we manage our money. Algorithms are employed to detect fraudulent transactions in real-time, ensuring our financial security. Additionally, robo-advisors use AI to provide personalized investment advice based on individual risk tolerance and financial goals. It’s like having a financial advisor available 24/7, guiding you through the complexities of investment strategies.
Furthermore, AI is revolutionizing the transportation industry. From self-driving cars to traffic management systems, AI is enhancing safety and efficiency on the roads. Companies like Tesla are at the forefront of this movement, utilizing AI to create vehicles that can navigate and make decisions just like a human driver. As we move towards a future with autonomous vehicles, the way we commute will drastically change, making travel safer and more convenient.
Lastly, let’s not forget about AI's influence on customer service. Chatbots and virtual customer assistants are becoming increasingly common on websites, providing instant support to users. They can handle a multitude of inquiries, from tracking orders to answering frequently asked questions, all while improving response times and customer satisfaction. This level of service is something that was unimaginable just a few years ago.
In summary, AI is not just a futuristic concept; it is actively shaping our present and enhancing our daily experiences. Its applications span across various sectors, making tasks easier and more efficient. As we continue to integrate AI into our lives, it’s essential to stay informed about its benefits and challenges, ensuring that we harness its potential responsibly.
- What is the difference between AI and Machine Learning?
AI is the broader concept of machines being able to carry out tasks in a way that we would consider “smart,” while Machine Learning is a specific subset of AI that focuses on the idea that systems can learn from data, identify patterns, and make decisions with minimal human intervention.
- How does AI impact job markets?
AI can lead to job displacement in some sectors, but it also creates new job opportunities in AI development, maintenance, and oversight. The key is for the workforce to adapt and acquire new skills relevant to the evolving job landscape.
- Are there ethical concerns surrounding AI?
Yes, ethical concerns include data privacy, algorithmic bias, and the potential for misuse of AI technologies. It is crucial for developers and organizations to prioritize ethical standards in AI development.
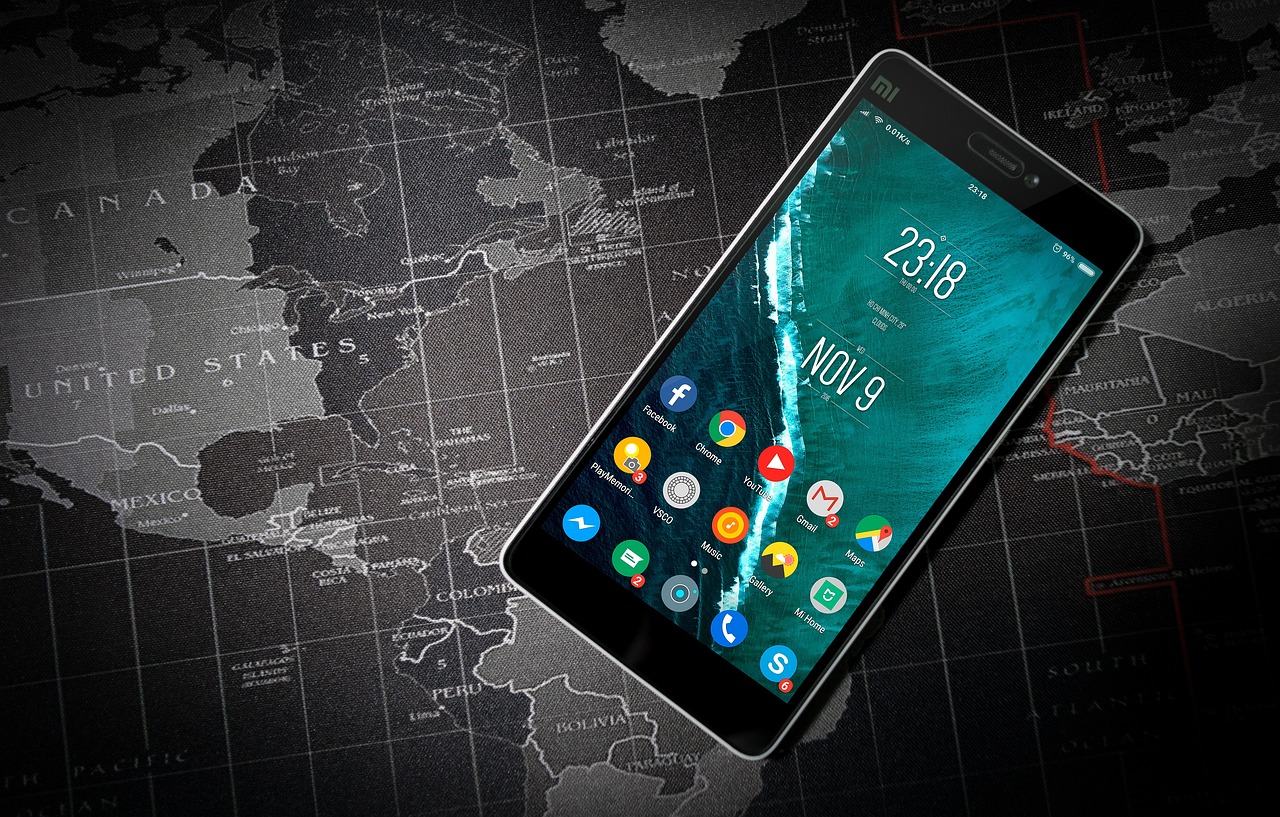
Challenges in AI and ML
As we dive deeper into the realms of Artificial Intelligence (AI) and Machine Learning (ML), it's essential to acknowledge that the journey is not without its hurdles. While these technologies promise a future filled with innovation and efficiency, they also bring forth a plethora of challenges that need to be addressed. One of the most pressing issues is the ethical implications surrounding AI and ML. With machines making decisions that can significantly impact human lives, questions arise: Who is responsible for these decisions? What happens if an AI system makes a mistake? The answers to these questions are still being explored, and the lack of clear guidelines can lead to a slippery slope of accountability.
Another significant challenge lies in data privacy. As ML algorithms rely heavily on vast amounts of data to learn and improve, there is a growing concern about how this data is collected, stored, and used. For instance, consider the personal information that is often fed into these systems—social media interactions, online purchases, and even health records. If mishandled, this data could lead to severe privacy violations. Companies must tread carefully to ensure that they respect user privacy while still leveraging data to enhance their AI capabilities.
Moreover, the issue of bias in AI algorithms cannot be overlooked. If the data used to train these models is biased, the outcomes will inevitably reflect that bias, leading to unfair treatment of certain groups. For example, facial recognition technology has been shown to misidentify individuals from minority backgrounds at a disproportionately higher rate than others. This not only raises ethical concerns but also undermines the trust that users place in these technologies.
In addition to ethical and privacy concerns, there is also the challenge of technical limitations. While ML has made significant strides, it still struggles with understanding context and nuance in human communication. For instance, natural language processing (NLP) systems can misinterpret sarcasm or idiomatic expressions, leading to misunderstandings. As a result, the effectiveness of AI in areas such as customer service or content moderation can be compromised, which can frustrate users.
Lastly, the skills gap in the workforce presents another challenge. As AI and ML technologies continue to evolve, there's a growing demand for professionals who are well-versed in these fields. However, educational institutions and training programs are often lagging behind the rapid pace of technological advancement. This disparity can lead to a shortage of qualified individuals capable of developing and managing these systems, ultimately hindering progress.
In summary, while the potential of AI and ML is vast, it is imperative to address these challenges head-on. By fostering discussions around ethics, improving data privacy measures, mitigating bias, enhancing technical capabilities, and closing the skills gap, we can pave the way for a future where AI and ML are not only powerful but also responsible and equitable.
- What are the main ethical concerns associated with AI and ML?
Ethical concerns primarily revolve around accountability for decisions made by AI, the potential for bias in algorithms, and the implications of data privacy. - How does bias affect AI systems?
Bias in AI systems can lead to unfair treatment of individuals or groups, particularly if the training data reflects societal prejudices. - What is the skills gap in AI and ML?
The skills gap refers to the shortage of qualified professionals who can develop and manage AI and ML technologies, which can impede progress in these fields. - How can companies ensure data privacy when using AI?
Companies can ensure data privacy by implementing robust data protection policies, obtaining user consent, and being transparent about data usage.
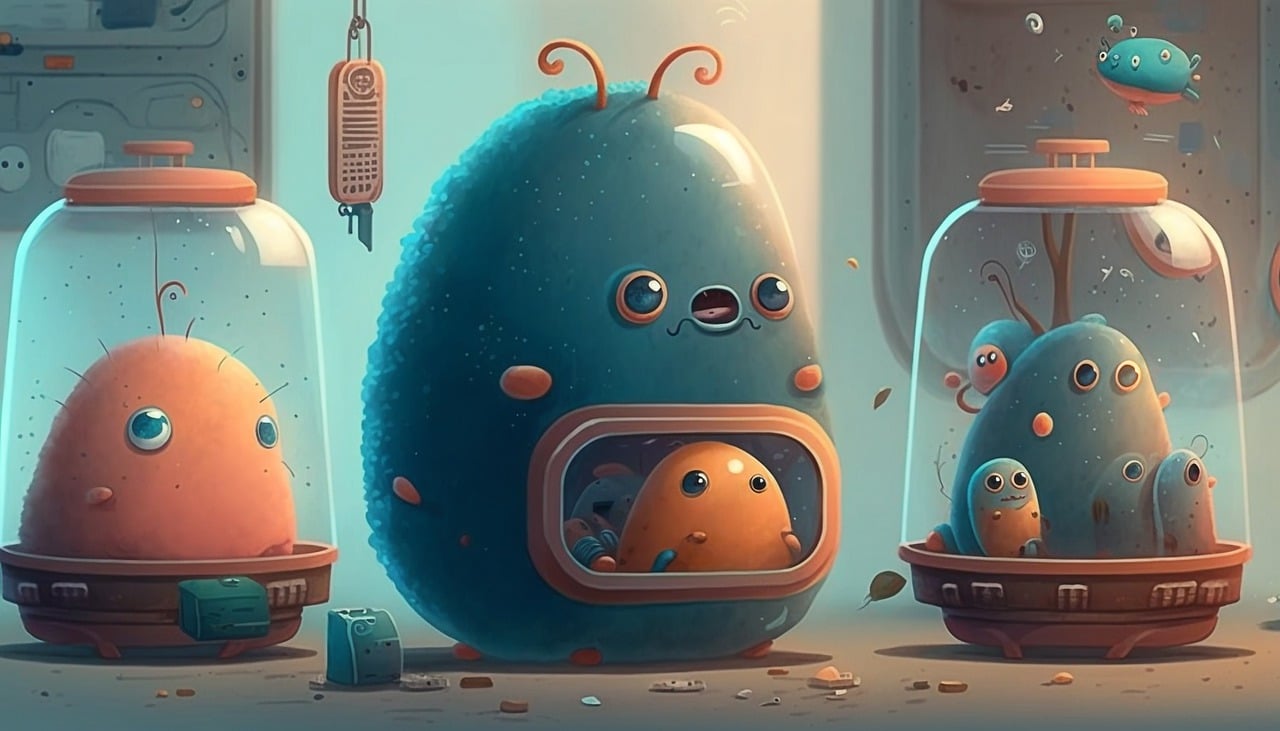
The Future of AI and Machine Learning
As we look to the future, the realms of Artificial Intelligence (AI) and Machine Learning (ML) are poised for remarkable transformations. The pace of technological advancement is accelerating, and the implications of these changes are profound. Imagine a world where machines not only assist us but anticipate our needs, learning from our behaviors and preferences in real-time. This is not just a distant dream; it’s becoming a reality faster than we might expect.
One of the most exciting aspects of AI and ML is their ability to transform industries. From healthcare to finance, the integration of these technologies is reshaping how businesses operate. For instance, in healthcare, AI algorithms can analyze vast amounts of medical data to identify patterns that humans might overlook. This leads to faster diagnoses and personalized treatment plans, ultimately improving patient outcomes. Similarly, in finance, ML algorithms are employed for fraud detection, risk assessment, and even algorithmic trading, showcasing the versatility of these technologies.
Moreover, we can anticipate advancements in natural language processing (NLP), which will enable machines to understand and interact with human language more effectively. This could revolutionize customer service, making virtual assistants more intuitive and responsive. Imagine chatting with a bot that understands your emotions and provides empathetic responses! The potential for enhancing user experience is limitless.
However, with great power comes great responsibility. As AI and ML continue to evolve, ethical considerations will play a crucial role in their development. Questions regarding data privacy, algorithmic bias, and the transparency of AI systems will need to be addressed. Developers and organizations must prioritize ethical guidelines to ensure that these technologies are used responsibly. After all, the goal should be to create systems that enhance human capabilities rather than replace them.
Looking ahead, we might also see an increase in the collaboration between humans and machines. This hybrid approach can lead to innovative solutions that leverage the strengths of both parties. For example, in creative fields such as art and music, AI can serve as a collaborator, offering new ideas while allowing human creators to maintain their unique touch. This synergy could lead to unprecedented creativity and innovation.
Additionally, the future of AI and ML is likely to include more accessible technology. As these tools become more user-friendly, we can expect a democratization of AI, allowing smaller businesses and individuals to harness its power without needing extensive technical knowledge. This could spur a wave of innovation from unexpected sources, driving further advancements in the field.
In conclusion, the future of AI and Machine Learning is not just about technological advancements; it’s about how these technologies will shape our society. As we embrace the possibilities, it’s essential to remain vigilant about the ethical implications and strive for a future where AI and ML work harmoniously with humanity, enhancing our lives rather than complicating them.
- What is the difference between AI and ML? AI is the broader concept of machines being able to carry out tasks in a way that we would consider "smart," while ML is a subset of AI that focuses specifically on the idea that machines can learn from data.
- How will AI impact jobs in the future? AI will likely automate certain tasks, leading to job displacement in some areas, but it will also create new jobs that require human oversight and creativity.
- What ethical concerns are associated with AI? Ethical concerns include data privacy, algorithmic bias, and the need for transparency in AI decision-making processes.
- Can AI and humans work together? Absolutely! The future holds great potential for collaboration between AI and humans, combining the strengths of both to achieve innovative solutions.
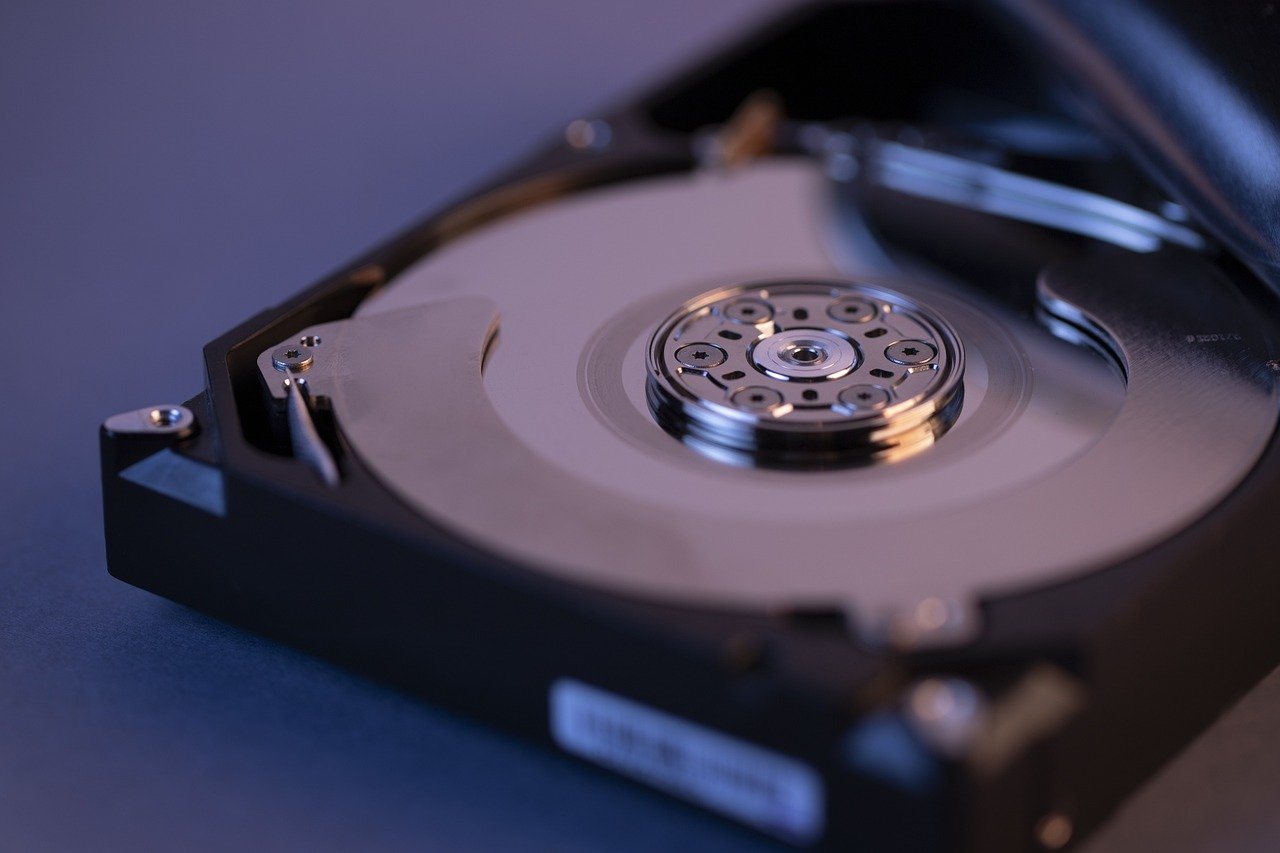
Impact on Employment
The rise of Artificial Intelligence (AI) and Machine Learning (ML) is reshaping the employment landscape in profound ways. As we embrace these technologies, the conversation around job displacement and creation becomes increasingly relevant. Many people worry that robots and algorithms will take over their jobs, leading to widespread unemployment. But is that the whole story? Not quite!
While it's true that certain roles, particularly those involving repetitive tasks, are at risk, AI and ML also pave the way for new job opportunities that we can't even imagine yet. Think of it this way: when the internet emerged, many traditional jobs vanished, but countless new ones were born in its wake. The same principle applies here. As machines take over mundane tasks, humans can focus on more complex and creative endeavors.
Moreover, the integration of AI and ML into various sectors is creating demand for skilled professionals who can develop, manage, and maintain these technologies. For instance, roles such as data scientists, AI ethicists, and machine learning engineers are becoming increasingly sought after. Companies are looking for individuals who can bridge the gap between technology and human needs, ensuring that AI systems are not only efficient but also ethical and responsible.
To illustrate the impact on employment, consider the following table that outlines potential job displacement and creation across different industries:
Industry | Jobs at Risk of Displacement | New Jobs Created |
---|---|---|
Manufacturing | Assembly line workers, quality control inspectors | Automation specialists, robotics engineers |
Healthcare | Radiologists, data entry clerks | AI health consultants, telemedicine coordinators |
Finance | Bank tellers, loan officers | Financial analysts, compliance officers |
As you can see, while some jobs may disappear, others are emerging in their place. This transition requires a shift in mindset, where both employers and employees must adapt to the evolving job market. Upskilling and reskilling will become essential for the workforce to thrive in this new era. It's not just about keeping up; it's about staying ahead!
Furthermore, organizations must play a pivotal role in this transition. They need to invest in training programs that equip employees with the necessary skills to work alongside AI and ML technologies. This collaborative approach will not only enhance productivity but also foster a culture of innovation and creativity.
In conclusion, while the impact of AI and ML on employment raises valid concerns, it also opens up a world of possibilities. By embracing change and preparing for the future, we can navigate this technological revolution together, ensuring that human ingenuity and machine efficiency coexist harmoniously.
- Will AI take away all jobs? - Not all jobs will be taken away; many will evolve, and new roles will emerge.
- What skills should I develop to stay relevant? - Focus on skills like data analysis, programming, and emotional intelligence.
- How can companies support their employees during this transition? - By providing training and reskilling opportunities to help employees adapt to new technologies.
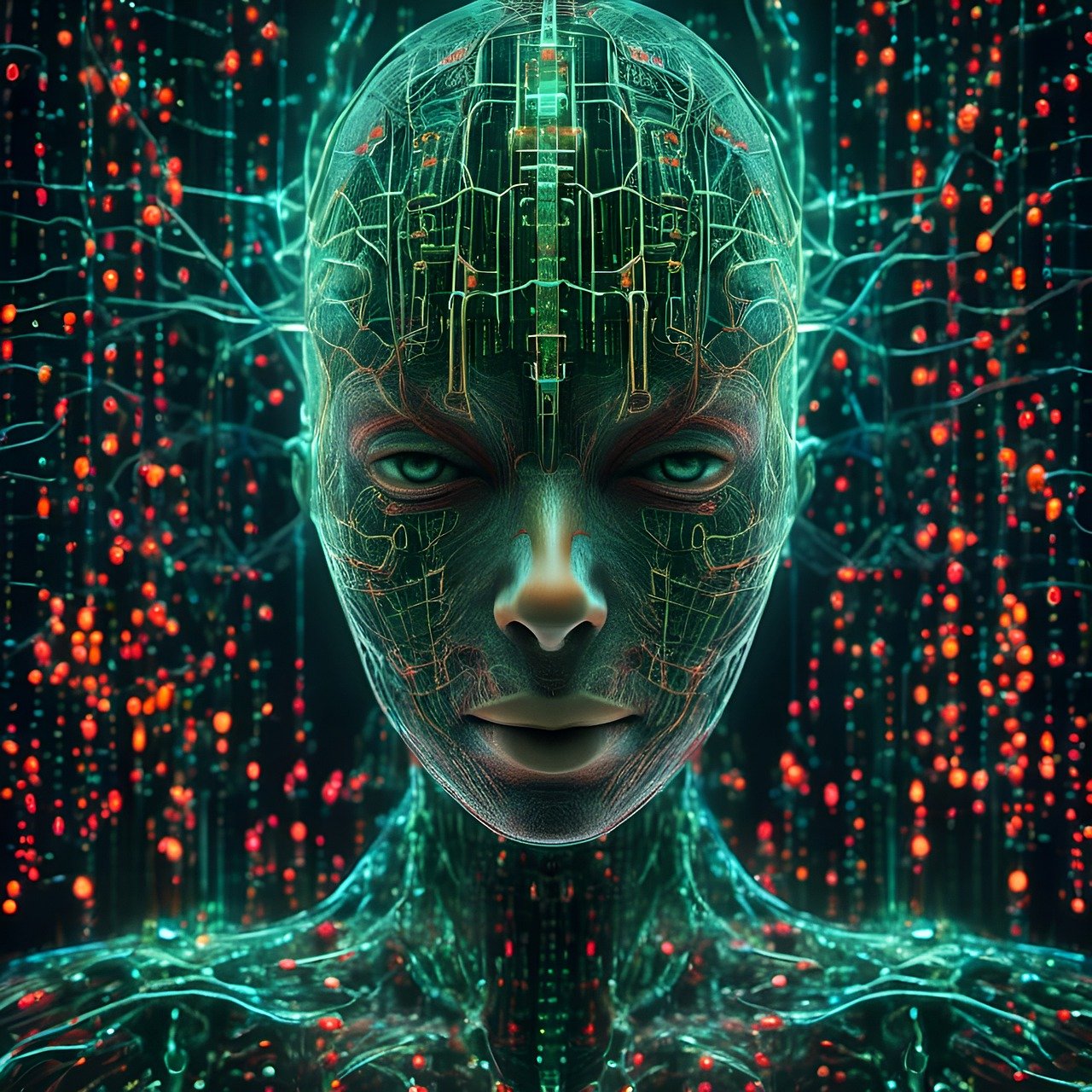
Ethical Considerations
As we plunge deeper into the realms of Artificial Intelligence (AI) and Machine Learning (ML), the conversation around ethics becomes increasingly important. These technologies hold immense potential to revolutionize our lives, but they also come with a set of ethical dilemmas that we cannot afford to overlook. One of the primary concerns is bias in algorithms. If the data fed into an AI system is biased, the outcomes will inevitably reflect that bias, leading to unfair treatment of individuals or groups. For example, facial recognition technology has been shown to misidentify people of color at a higher rate than white individuals, raising serious questions about its deployment in law enforcement and security.
Moreover, there’s the issue of data privacy. With AI systems collecting vast amounts of personal data, how do we ensure that this information is used responsibly? The potential for misuse or unauthorized access is a significant concern. It's essential for organizations to implement stringent data protection measures and be transparent about how they collect and use data. This transparency fosters trust and allows users to make informed decisions about their data.
Another critical aspect of ethical considerations in AI and ML is the question of accountability. When an AI system makes a decision that negatively impacts someone’s life, who is responsible? Is it the developer, the organization, or the AI itself? Establishing clear lines of accountability is vital to ensure that there are repercussions for harmful actions taken by AI systems. This is particularly relevant in sectors such as healthcare, where AI-driven diagnostic tools can significantly affect patient outcomes.
Additionally, we must consider the impact on employment. As AI and ML technologies continue to advance, many fear that they will lead to widespread job displacement. While some jobs may become obsolete, new opportunities will also emerge. Balancing the benefits of automation with the potential for job loss is a complex ethical challenge that requires careful consideration and proactive measures to retrain and support affected workers.
In light of these concerns, it's clear that the development of AI and ML must be guided by a robust ethical framework. Organizations need to prioritize ethical considerations by:
- Conducting regular audits of their AI systems to identify and mitigate bias.
- Implementing data privacy policies that comply with regulations like GDPR.
- Creating clear accountability structures for AI decision-making.
- Investing in workforce retraining programs to prepare for the future job market.
Ultimately, the goal should be to harness the power of AI and ML in a way that promotes fairness, transparency, and accountability. By doing so, we can ensure that these technologies serve humanity positively and ethically, paving the way for a future where AI complements our lives rather than complicates them.
Q1: What are the main ethical concerns regarding AI and ML?
A1: The main ethical concerns include bias in algorithms, data privacy issues, accountability for AI decisions, and the impact on employment.
Q2: How can organizations address bias in AI systems?
A2: Organizations can address bias by regularly auditing their AI systems, using diverse datasets for training, and involving diverse teams in the development process.
Q3: Why is data privacy important in AI?
A3: Data privacy is crucial because AI systems often handle sensitive personal information, and improper use or breaches can lead to significant harm to individuals.
Q4: What should be done to support workers displaced by AI?
A4: Companies and governments should invest in retraining programs and provide support to help workers transition into new roles that emerge as technology evolves.
Frequently Asked Questions
-
What is the difference between Artificial Intelligence and Machine Learning?
Artificial Intelligence (AI) is the broader concept of machines being able to carry out tasks in a way that we would consider "smart." In contrast, Machine Learning (ML) is a subset of AI that focuses specifically on the idea that systems can learn from data, improve their performance over time, and make decisions without being explicitly programmed for each task.
-
How is Machine Learning used in everyday applications?
Machine Learning is everywhere! From personalized recommendations on streaming services like Netflix to voice recognition in virtual assistants like Siri and Alexa, ML algorithms analyze data patterns to improve user experiences. It’s like having a personal assistant that knows what you like and helps you find it!
-
Can AI and ML replace human jobs?
While AI and ML can automate certain tasks, they also create new job opportunities. Think of it as a double-edged sword; some jobs may be lost, but new roles in tech development, data analysis, and AI ethics are emerging. The key is for the workforce to adapt and learn new skills that align with these technological advancements.
-
What are the ethical concerns surrounding AI and ML?
Ethical considerations in AI and ML include issues like data privacy, algorithmic bias, and the potential for misuse of technology. It’s crucial for developers and organizations to prioritize responsible AI practices, ensuring that these technologies are used for the greater good and do not harm individuals or society.
-
What does the future hold for AI and Machine Learning?
The future of AI and ML is bright and full of possibilities! We can expect advancements in areas like natural language processing, autonomous vehicles, and personalized healthcare. However, it’s essential to navigate these advancements responsibly, considering the implications they have on our daily lives and the world at large.