Deep Learning Innovations: Shaping the Future of AI
Deep learning is not just a buzzword; it's a revolutionary force that's reshaping the landscape of artificial intelligence (AI). As we dive into the intricacies of this technology, we uncover layers of innovation that are not only fascinating but also vital for the future of various industries. Imagine a world where machines can learn, adapt, and make decisions almost like humans. That's the promise of deep learning. From healthcare to autonomous vehicles, the impact of these innovations is profound, and the potential is virtually limitless.
At its core, deep learning mimics the human brain's neural networks, allowing computers to process vast amounts of data and learn from it. This capability has led to breakthroughs that were once thought to be the realm of science fiction. For instance, consider how voice assistants like Siri and Alexa have become integral to our daily lives. They rely on deep learning algorithms to understand and respond to our commands accurately. This technology is not just about efficiency; it's about enhancing our interaction with machines in a way that feels natural and intuitive.
As we explore the latest advancements in deep learning, it's essential to recognize that we are in the midst of a technological revolution. The algorithms driving this progress are becoming increasingly sophisticated, enabling machines to perform tasks that require human-like understanding and reasoning. For example, convolutional neural networks (CNNs) have transformed image recognition, allowing applications to identify objects in photographs with remarkable accuracy. Similarly, recurrent neural networks (RNNs) have opened new frontiers in natural language processing, enabling machines to understand context and nuance in human language.
The implications of these advancements are staggering. In healthcare, deep learning is being harnessed to analyze medical images, providing doctors with powerful tools to diagnose conditions more accurately and swiftly. Meanwhile, in the automotive industry, CNNs are at the heart of self-driving technology, helping vehicles navigate complex environments by interpreting visual data in real-time. This level of innovation not only enhances safety but also promises to revolutionize transportation as we know it.
However, with great power comes great responsibility. As we embrace the potential of deep learning, we must also confront the challenges it presents. Issues such as data scarcity, interpretability of models, and the high computational costs associated with training deep learning networks require our attention. Addressing these challenges is crucial for ensuring that deep learning can achieve its full potential while maintaining trust and transparency in its applications.
Looking ahead, the future trends in deep learning are exciting. We are witnessing advancements in unsupervised learning and transfer learning that could further democratize AI, making it accessible to a broader range of applications and industries. The possibilities are endless, and as we continue to innovate, we must remain vigilant in addressing the ethical implications of this technology. After all, the goal of deep learning is not just to create smarter machines but to enhance the human experience in a meaningful way.
- What is deep learning? - Deep learning is a subset of machine learning that uses neural networks to analyze and learn from vast amounts of data.
- How does deep learning differ from traditional machine learning? - Unlike traditional machine learning, which often requires manual feature extraction, deep learning automatically discovers patterns and features from raw data.
- What are the main applications of deep learning? - Deep learning is used in various fields, including healthcare, autonomous vehicles, finance, and natural language processing.
- What challenges does deep learning face? - Key challenges include the need for large datasets, model interpretability, and high computational costs.
- What does the future hold for deep learning? - The future of deep learning includes advancements in unsupervised learning, transfer learning, and greater integration into everyday applications.
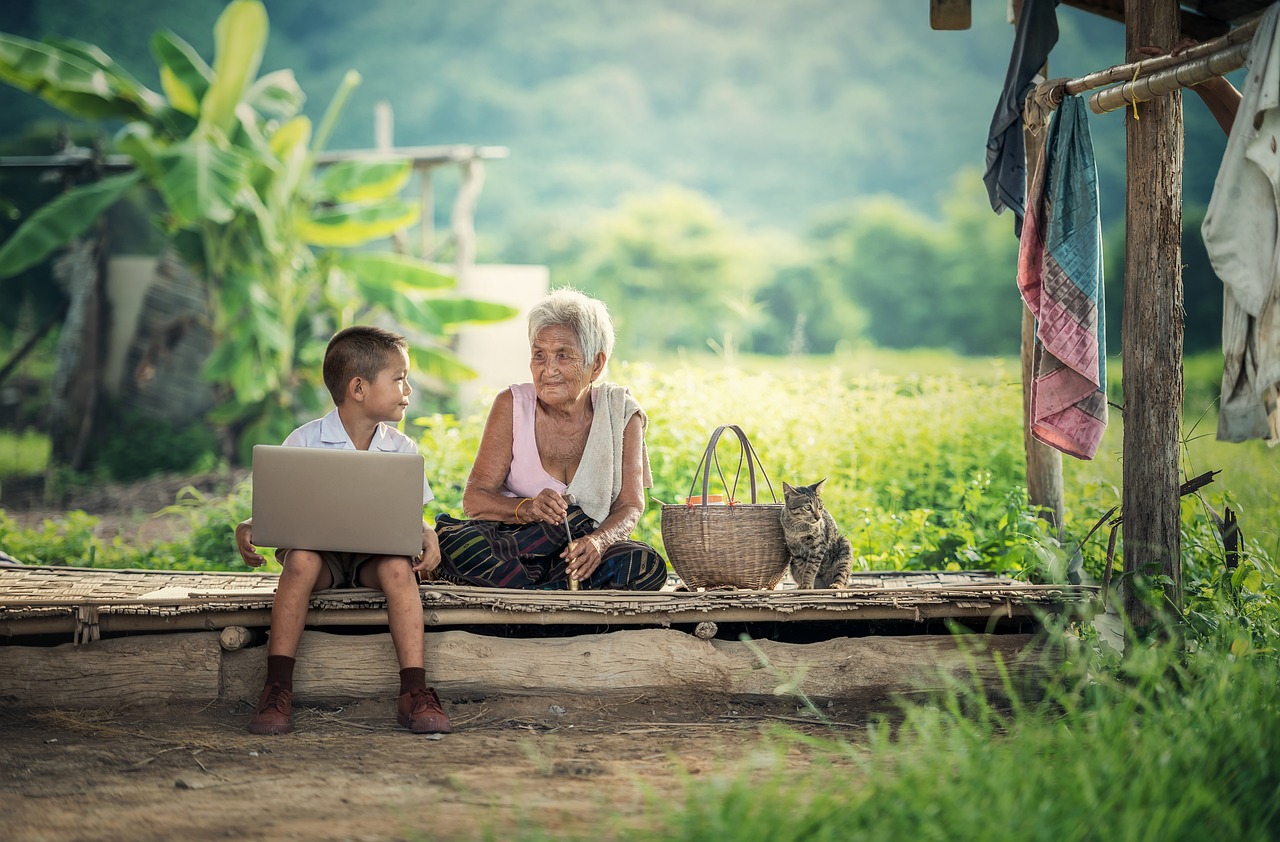
The Evolution of Deep Learning
Deep learning, a subset of artificial intelligence, has come a long way since its inception. To truly appreciate its current capabilities, we need to journey back to its roots. The concept of neural networks dates back to the 1950s, when pioneers like Frank Rosenblatt introduced the Perceptron, a simple model that mimicked the way human brains process information. However, it wasn't until the 1980s that the field began to gain traction with the introduction of backpropagation, a method that allowed networks to learn from their mistakes, refining their outputs through multiple iterations.
Fast forward to the 21st century, and deep learning has evolved into a sophisticated and powerful tool. The increase in computational power, along with the availability of vast amounts of data, has propelled deep learning into the spotlight. Today, we see complex architectures such as Convolutional Neural Networks (CNNs) and Recurrent Neural Networks (RNNs), which have transformed how we approach problems in fields ranging from image recognition to natural language processing.
One of the key turning points in this evolution was the advent of Deep Belief Networks (DBNs) in the late 2000s. These networks allowed for unsupervised learning, enabling systems to learn from unlabelled data, which was a game-changer in deep learning applications. The success of DBNs laid the groundwork for further advancements, leading to the development of more intricate architectures and techniques.
To illustrate this evolution, consider the following timeline:
Year | Event |
---|---|
1958 | Introduction of the Perceptron by Frank Rosenblatt |
1986 | Backpropagation algorithm popularized by Geoffrey Hinton |
2006 | Introduction of Deep Belief Networks by Geoffrey Hinton |
2012 | AlexNet wins ImageNet competition, showcasing CNNs |
2014 | Generative Adversarial Networks (GANs) introduced by Ian Goodfellow |
This timeline captures just a few milestones in the rich history of deep learning. Each breakthrough has contributed to the growing complexity and capability of neural networks. As we continue to innovate and refine these technologies, the potential applications seem limitless. Imagine a world where machines can not only recognize faces but also understand emotions, or where they can predict the stock market with remarkable accuracy. The possibilities are endless, and it all stems from the foundational work laid down by early researchers.
In essence, the evolution of deep learning is a fascinating narrative of persistence, innovation, and scientific inquiry. As we look to the future, we can only anticipate the next wave of breakthroughs that will further shape the landscape of artificial intelligence. With every advancement, we get closer to creating systems that can think, learn, and adapt in ways that were once the realm of science fiction.
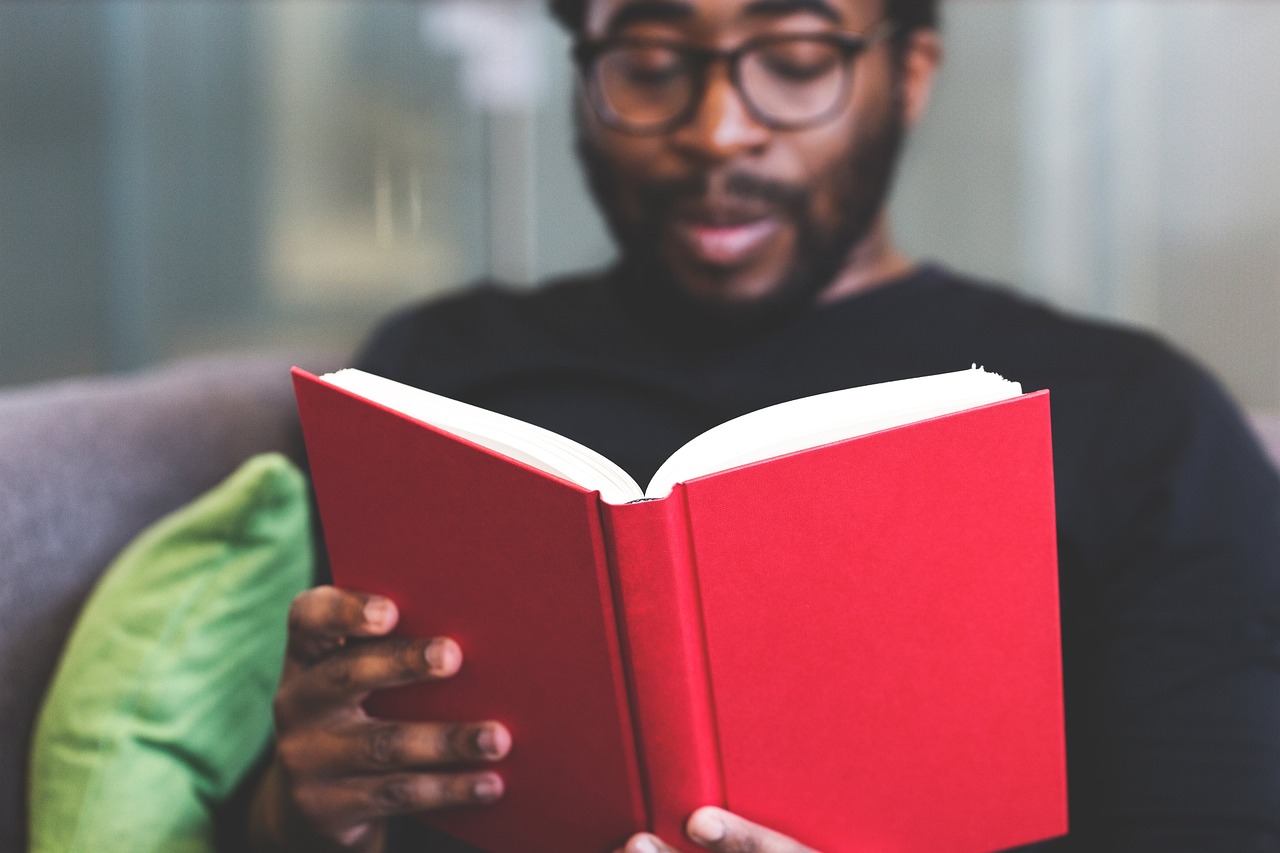
Key Algorithms Driving Progress
In the ever-evolving world of artificial intelligence, deep learning stands out as a groundbreaking force, primarily due to its core algorithms that drive remarkable advancements. These algorithms serve as the backbone of various AI applications, enabling machines to learn from vast amounts of data, recognize patterns, and make predictions with astonishing accuracy. Among the most influential algorithms are Convolutional Neural Networks (CNNs) and Recurrent Neural Networks (RNNs), each tailored to excel in different domains.
Let's start by diving into Convolutional Neural Networks (CNNs). These algorithms have transformed the field of image processing and computer vision. By mimicking the way humans perceive visual information, CNNs utilize a unique architecture that includes layers of convolutional filters. This allows them to effectively capture spatial hierarchies in images, making them incredibly adept at tasks like image classification and object detection. For instance, when you upload a photo to social media, CNNs are often at work, identifying faces, objects, and even the context of the image. The power of CNNs lies in their ability to learn from large datasets, improving their accuracy over time.
But how do CNNs actually work? At their core, they consist of several key components:
- Convolutional Layers: These layers apply filters to the input data, helping the model to identify features such as edges, textures, and shapes.
- Pooling Layers: These layers reduce the dimensionality of the data, allowing the model to focus on the most important features while discarding noise.
- Fully Connected Layers: At the end of the network, these layers make the final classifications based on the features extracted from the previous layers.
Now, let's explore the applications of CNNs in various fields. One of the most significant areas is healthcare. CNNs are revolutionizing medical imaging by enhancing diagnostics and improving patient outcomes. For example, in radiology, CNNs can analyze X-rays and MRIs with a level of precision that rivals human experts. This not only speeds up the diagnostic process but also reduces the chances of human error, ultimately leading to better treatment plans for patients.
Moreover, CNNs are making strides in the realm of autonomous vehicles. Imagine a self-driving car navigating through a busy city. How does it know when to stop, turn, or avoid obstacles? The answer lies in CNNs. They help vehicles interpret complex environments by recognizing traffic signs, pedestrians, and other vehicles on the road. This capability is crucial for ensuring safety and efficiency in autonomous driving systems.
Shifting gears, let's talk about Recurrent Neural Networks (RNNs). Unlike CNNs, which excel at processing spatial data, RNNs are designed for sequential data. Think of them as the storytellers of the AI world, capable of understanding context and maintaining a memory of previous inputs. This makes them indispensable for tasks such as natural language processing and time series forecasting.
RNNs function by passing information from one step of the sequence to the next, allowing them to capture dependencies over time. This is particularly useful in applications like language translation, where the meaning of a word can change based on the words that come before it. For instance, when translating a sentence, an RNN can remember the context of previous words, enabling it to produce more accurate translations.
In summary, the key algorithms driving progress in deep learning—CNNs and RNNs—are not just technical constructs; they are the engines powering a new era of AI. From enhancing medical diagnostics to enabling self-driving cars and improving language translation, these algorithms are reshaping our world in ways we are just beginning to understand. As we continue to innovate and refine these technologies, the possibilities seem endless.
- What are the main differences between CNNs and RNNs? CNNs are primarily used for image data, while RNNs are designed for sequential data like text and time series.
- How do CNNs improve medical imaging? They enhance diagnostic accuracy by analyzing images for patterns and anomalies that might be missed by human eyes.
- Can RNNs be used for real-time applications? Yes, RNNs can process data in real-time, making them suitable for applications like speech recognition and live language translation.
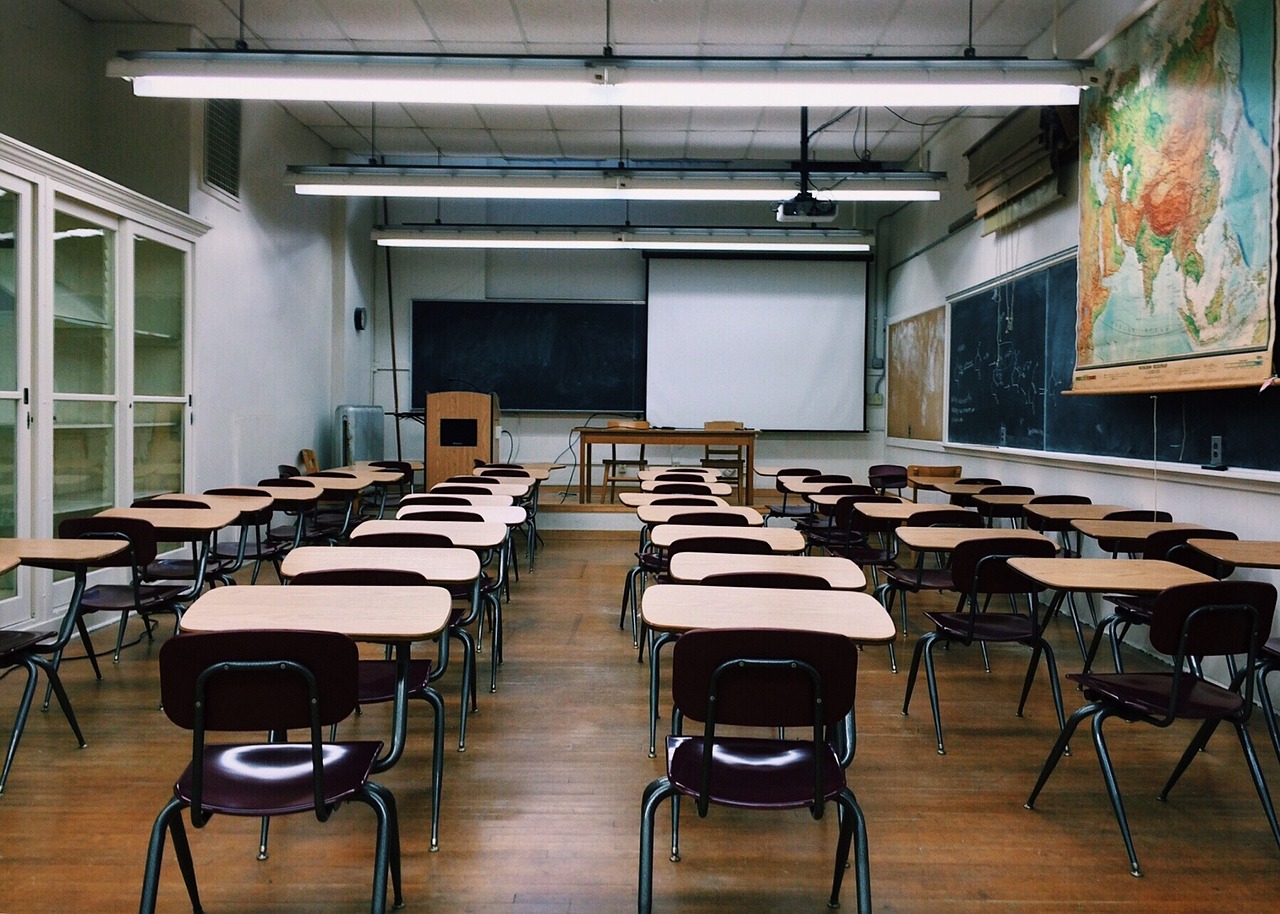
Convolutional Neural Networks (CNNs)
Convolutional Neural Networks, or CNNs, have truly revolutionized the field of image processing and computer vision. Imagine trying to find a needle in a haystack; that's what it used to feel like for computers trying to analyze images. But with the advent of CNNs, they can now sift through vast amounts of visual data with remarkable efficiency. At their core, CNNs are designed to automatically and adaptively learn spatial hierarchies of features from images, making them incredibly effective for tasks such as image classification, object detection, and even facial recognition.
The architecture of a CNN is quite fascinating. Typically, it consists of several layers that serve different purposes. These include:
- Convolutional Layers: These layers apply filters to the input image to create feature maps that help identify patterns.
- Pooling Layers: These layers reduce the dimensionality of the feature maps, retaining the most important information while discarding the less significant data.
- Fully Connected Layers: At the end of the network, these layers make the final classifications based on the features extracted by the previous layers.
One of the most exciting aspects of CNNs is their versatility. They are not just limited to static images; they can also be applied to video data, enabling real-time analysis in various applications. For instance, in the realm of healthcare, CNNs are making waves in medical imaging. They enhance diagnostics by analyzing X-rays, MRIs, and CT scans with a level of precision that was previously unattainable. This advanced image analysis helps doctors make more accurate diagnoses, ultimately leading to improved patient outcomes.
Furthermore, CNNs are playing a crucial role in the development of autonomous vehicles. These self-driving cars rely heavily on CNNs to interpret their surroundings. By processing images from multiple cameras and sensors, CNNs allow vehicles to recognize objects, lane markings, and pedestrians, enabling them to navigate complex environments safely. Imagine a car that can "see" the world as you do; that's the power of CNNs at work.
In summary, CNNs are a game-changer in the field of artificial intelligence. Their ability to learn from data, adapt to new challenges, and perform complex tasks with ease is paving the way for innovations across various industries. As we continue to explore the potential of CNNs, we can only expect to see even more groundbreaking applications emerge in the near future.
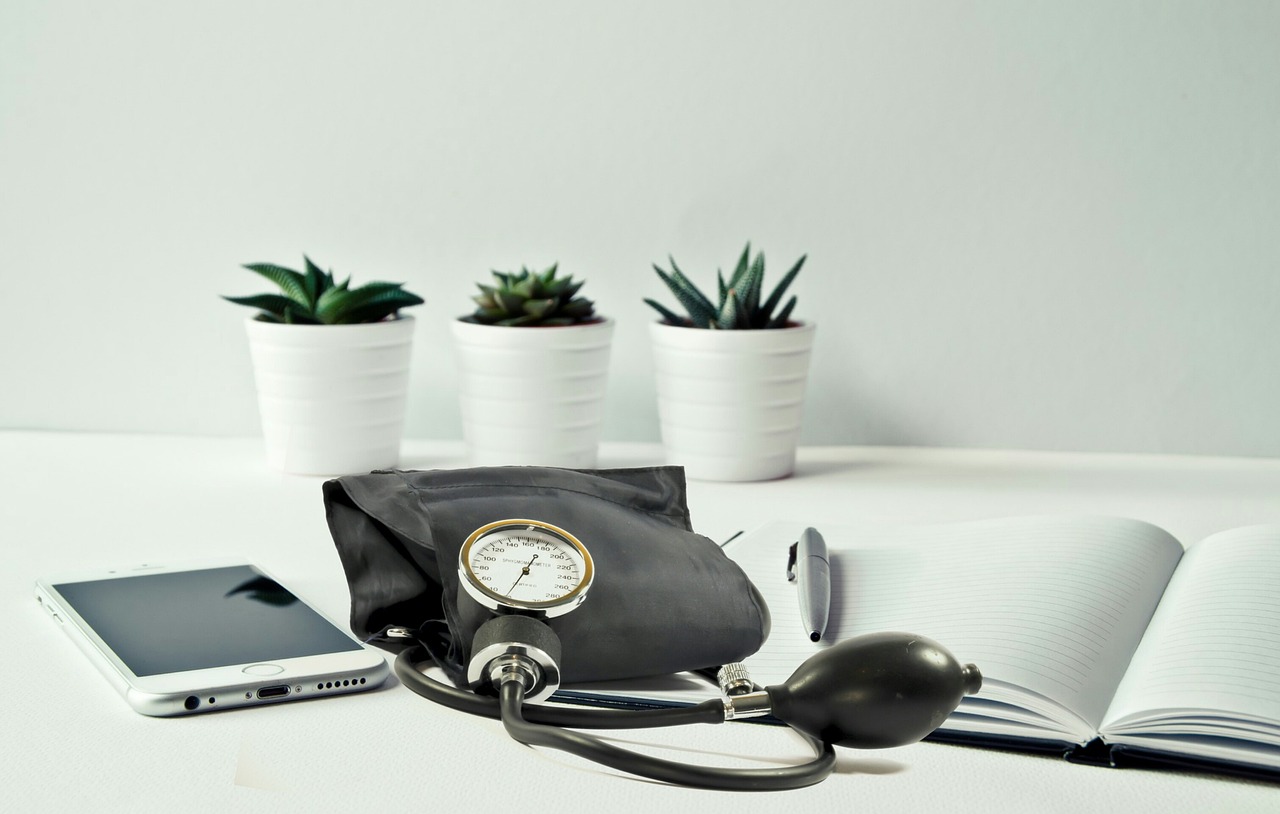
Applications in Healthcare
Deep learning has carved out a remarkable niche in the healthcare sector, transforming the way medical professionals diagnose and treat illnesses. Imagine a world where doctors can detect diseases at their earliest stages, often before symptoms even appear. This is not just a dream; it's becoming a reality thanks to the power of convolutional neural networks (CNNs) and other deep learning technologies. These advanced algorithms analyze vast amounts of medical imaging data, enabling healthcare providers to make more informed decisions.
One of the most significant applications of deep learning in healthcare is in the realm of medical imaging. For instance, CNNs are adept at interpreting images from MRIs, CT scans, and X-rays. They can identify patterns and anomalies that may be missed by the human eye, leading to earlier and more accurate diagnoses. In fact, studies have shown that deep learning models can outperform radiologists in certain diagnostic tasks, such as detecting tumors or fractures. This capability not only enhances the accuracy of diagnoses but also significantly reduces the time needed to analyze images, allowing healthcare professionals to focus more on patient care.
Furthermore, deep learning is being utilized in pathology, where it assists in analyzing tissue samples. By training models on thousands of labeled images, these systems can recognize cancerous cells with remarkable precision. This not only streamlines the diagnostic process but also minimizes the chances of human error, which can be critical in life-and-death situations.
In addition to diagnostics, deep learning is making strides in personalized medicine. By analyzing a patient’s genetic information alongside their medical history, deep learning algorithms can predict how they will respond to specific treatments. This level of customization is revolutionary, as it allows for tailored treatment plans that maximize efficacy while minimizing side effects. Imagine a scenario where your treatment is designed just for you, taking into account your unique biological makeup—this is the future that deep learning is paving the way for.
Moreover, deep learning applications extend to drug discovery, where it accelerates the identification of potential drug candidates. Traditional methods can take years and involve extensive trial-and-error. However, by leveraging vast datasets and predictive modeling, deep learning can significantly shorten this timeline, leading to faster development of new therapies and treatments. In essence, it acts like a supercharged research assistant, sifting through data at lightning speed to identify patterns that could lead to breakthroughs.
To summarize, the applications of deep learning in healthcare are vast and varied, encompassing:
- Enhanced diagnostics through medical imaging analysis.
- Improved accuracy in pathology for cancer detection.
- Personalized treatment plans based on genetic and medical history.
- Accelerated drug discovery processes.
The implications of these advancements are profound. As deep learning continues to evolve, we can expect even greater improvements in patient care, leading to better health outcomes and more efficient healthcare systems. The integration of these technologies not only empowers healthcare professionals but also provides patients with the hope of receiving more accurate and timely medical interventions.
Q1: How does deep learning improve medical imaging?
A1: Deep learning algorithms, particularly convolutional neural networks (CNNs), analyze medical images to identify patterns and anomalies that may be missed by human radiologists, leading to earlier and more accurate diagnoses.
Q2: Can deep learning personalize treatment plans?
A2: Yes, by analyzing a patient’s genetic information and medical history, deep learning can help predict how they will respond to specific treatments, allowing for tailored medical care.
Q3: What role does deep learning play in drug discovery?
A3: Deep learning accelerates the identification of potential drug candidates by analyzing vast datasets, significantly shortening the timeline for developing new therapies.
Q4: Are there risks associated with using deep learning in healthcare?
A4: While deep learning offers many benefits, challenges such as data privacy, model interpretability, and the need for high-quality datasets remain important considerations that the healthcare industry must address.
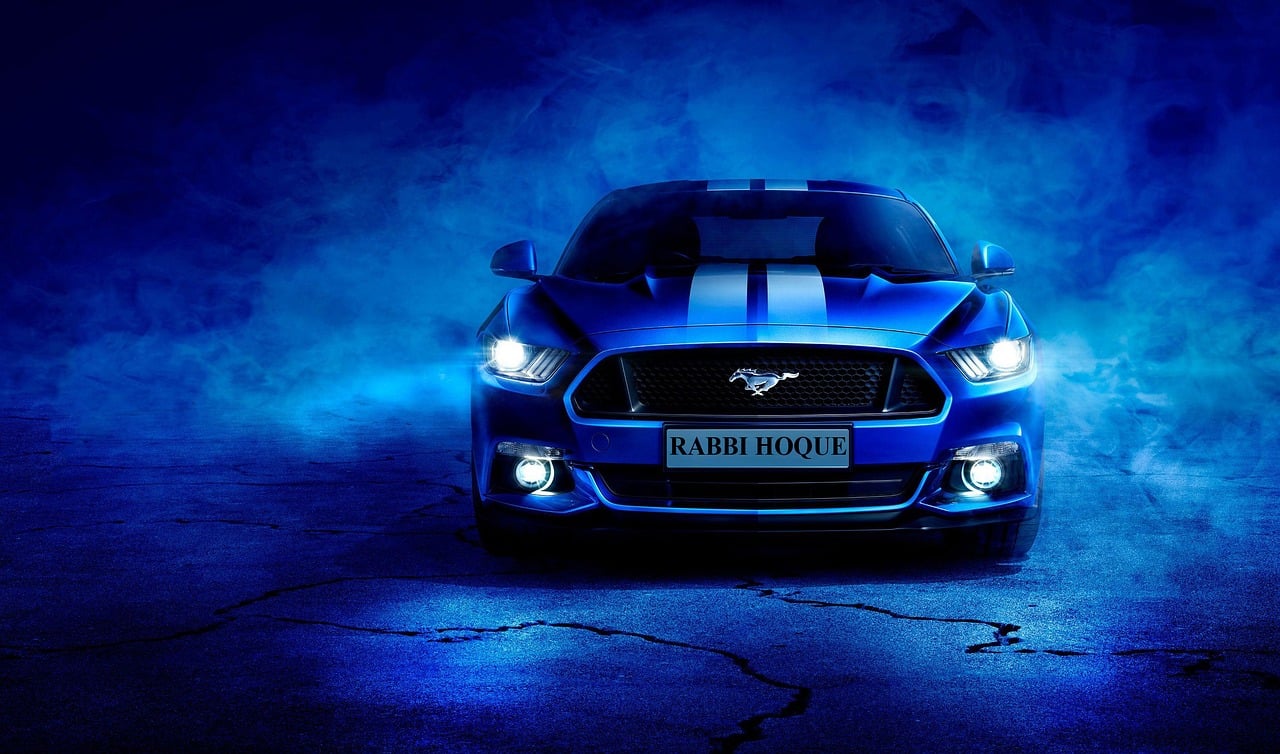
Impact on Autonomous Vehicles
When we think about the future of transportation, autonomous vehicles often come to mind as a groundbreaking innovation. The role of Convolutional Neural Networks (CNNs) in this realm cannot be overstated. These sophisticated algorithms are the backbone of the perception systems that allow self-driving cars to interpret their surroundings. Imagine a car that can 'see' the world just like a human does, identifying pedestrians, traffic signs, and obstacles with remarkable accuracy. This is not science fiction; it’s the reality that CNNs are helping to create.
At the heart of this technology lies the ability of CNNs to process and analyze vast amounts of visual data in real-time. For instance, when a self-driving car is navigating through a busy city, it relies on cameras and sensors to capture images of its environment. The CNN processes these images, extracting features such as edges, shapes, and textures. This information is crucial for making split-second decisions, such as when to stop, accelerate, or change lanes. The efficiency of CNNs in image classification tasks allows these vehicles to operate safely and effectively, even in complex scenarios.
Furthermore, the impact of CNNs extends beyond just recognizing objects. They also play a pivotal role in understanding the context of a scene. For example, a CNN can differentiate between a child playing on the sidewalk and an adult walking by, allowing the vehicle to prioritize its responses accordingly. This level of understanding is vital for ensuring the safety of all road users. The technology is designed to minimize the risk of accidents, making roads safer for everyone.
However, the integration of CNNs into autonomous vehicles is not without challenges. The need for high-quality data is paramount. Training these networks requires thousands, if not millions, of labeled images to ensure they can recognize and respond to various scenarios accurately. Additionally, the computational power needed to process this data in real-time is significant, necessitating advanced hardware solutions.
As we look to the future, the potential for CNNs in autonomous vehicles remains immense. With ongoing research and development, we can expect even more sophisticated algorithms that enhance the capabilities of self-driving technology. This could lead to vehicles that not only drive themselves but also learn from their experiences, continually improving their performance and safety features.
To sum it up, CNNs are revolutionizing the way autonomous vehicles operate. They enable cars to 'see' and understand their environment, making informed decisions that enhance safety and efficiency. As this technology evolves, we can anticipate a future where self-driving cars become a common sight, transforming our daily commutes and reshaping urban landscapes.
- What are Convolutional Neural Networks (CNNs)?
CNNs are a class of deep learning algorithms that excel in processing visual data, allowing machines to recognize patterns and objects in images. - How do CNNs improve autonomous vehicle safety?
By accurately detecting and classifying objects in real-time, CNNs help self-driving cars make informed decisions, reducing the likelihood of accidents. - What challenges do CNNs face in autonomous vehicles?
Challenges include the need for large datasets, high computational power, and ensuring the models can interpret complex driving environments accurately.
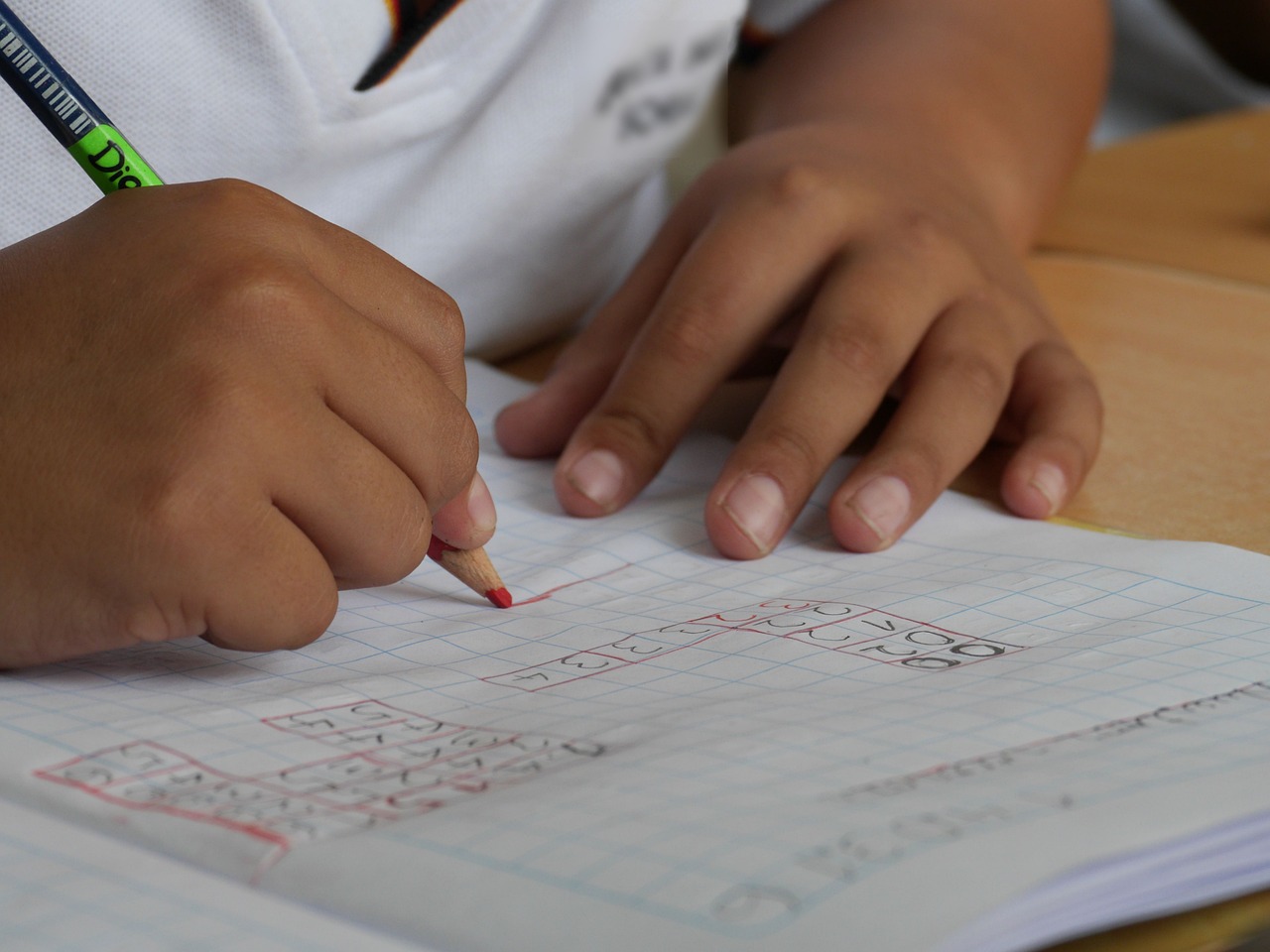
Recurrent Neural Networks (RNNs)
Recurrent Neural Networks, or RNNs, are a fascinating innovation in the field of deep learning, primarily designed to handle sequential data. Imagine trying to understand a sentence; you don’t just look at each word in isolation, but rather, you grasp the context formed by the entire sequence. RNNs mimic this human-like ability by maintaining a memory of previous inputs, allowing them to make predictions based on the entire sequence rather than just the current input. This unique architecture enables RNNs to excel in tasks such as natural language processing, speech recognition, and even music generation.
At the core of an RNN's functionality is its ability to loop back on itself. This means that the output from one step can be fed back into the network as input for the next step. Think of it like a storyteller who remembers the plot so far while weaving in new elements. This memory aspect is crucial when dealing with data that has a temporal aspect, such as time series data or any form of sequential input.
However, RNNs are not without their challenges. One significant issue is the vanishing gradient problem, which can hinder the training of the network. When gradients are backpropagated through many layers, they can become exceedingly small, making it difficult for the network to learn from earlier inputs. To combat this, variations of RNNs, such as Long Short-Term Memory (LSTM) networks and Gated Recurrent Units (GRUs), were developed. These architectures include mechanisms that allow them to maintain information over longer periods, effectively addressing the shortcomings of traditional RNNs.
The applications of RNNs are as diverse as they are impactful. For instance, in natural language processing, RNNs are used for tasks like language translation and sentiment analysis, where understanding the context of words is essential. In the realm of speech recognition, RNNs help convert spoken language into text by processing audio signals sequentially. Furthermore, they are also employed in time series forecasting, where predicting future values based on past observations is crucial for fields like finance and meteorology.
As we look to the future, the potential for RNNs continues to expand. With ongoing research aimed at improving their efficiency and effectiveness, we can expect to see even more innovative applications emerge. The integration of RNNs with other technologies, such as reinforcement learning and attention mechanisms, is likely to enhance their capabilities further, making them an essential tool in the arsenal of AI developers.
- What are RNNs used for? RNNs are primarily used for tasks involving sequential data, including natural language processing, speech recognition, and time series forecasting.
- What is the vanishing gradient problem? The vanishing gradient problem occurs when gradients become too small during backpropagation, making it difficult for RNNs to learn from earlier inputs.
- How do LSTMs differ from traditional RNNs? LSTMs incorporate mechanisms to maintain information over longer periods, effectively addressing the limitations of standard RNNs.
- Can RNNs be used for image processing? While RNNs are not typically used for image processing, they can be combined with convolutional neural networks (CNNs) to analyze video data.
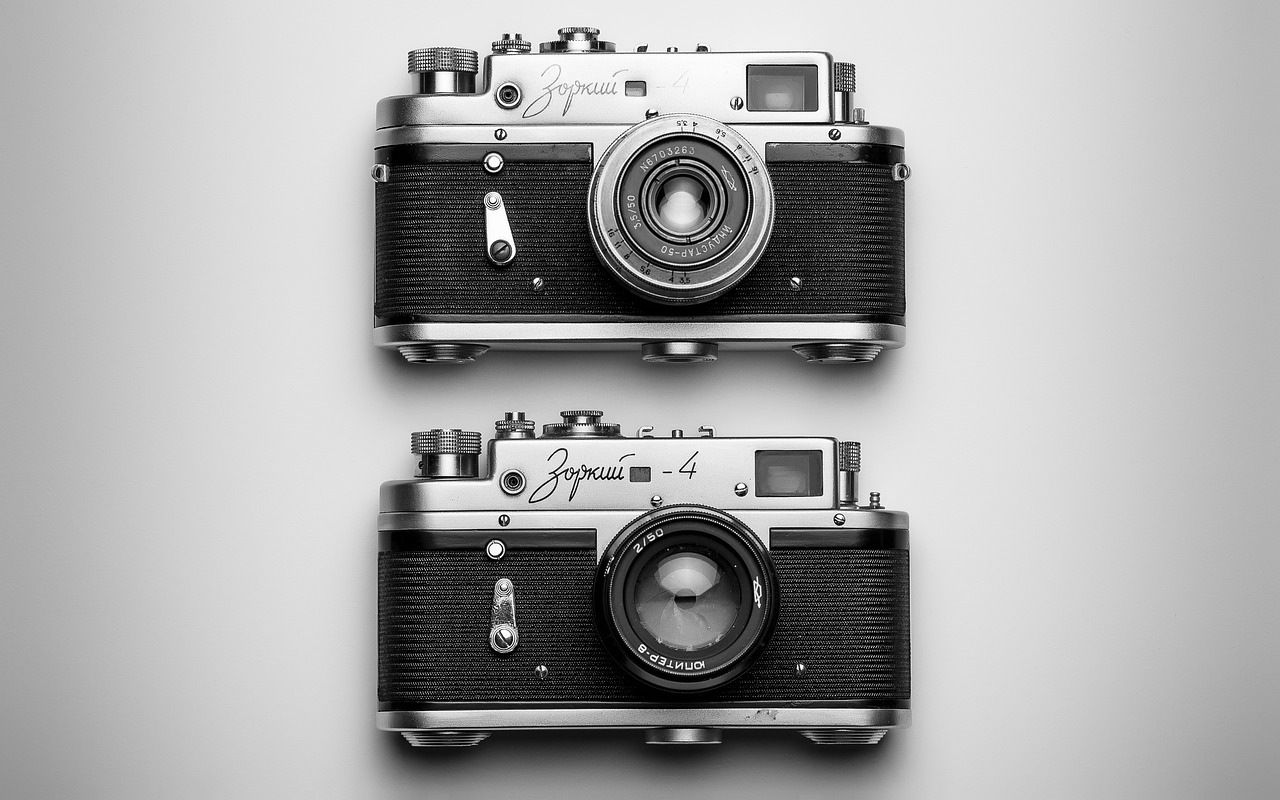
Challenges and Limitations
Despite the remarkable advancements and transformative potential of deep learning, it is not without its challenges and limitations. As we dive deeper into the complexities of this technology, we uncover several hurdles that researchers and practitioners must navigate to fully harness the power of deep learning. One of the most pressing issues is the requirement for vast amounts of data. Training deep learning models effectively requires large, high-quality datasets, which can be a significant barrier, especially in niche applications where data is scarce. Imagine trying to teach a child a new language using only a handful of words; similarly, deep learning models struggle to generalize from limited data.
Moreover, the quality of data is equally crucial. Poor quality data can lead to biased models that produce unreliable outcomes. For instance, if a facial recognition system is trained primarily on images of individuals from a specific demographic, it may perform poorly when encountering individuals from other backgrounds. This bias not only affects the performance of the models but also raises ethical concerns about fairness and accountability in AI applications.
Another significant challenge is the interpretability of deep learning models. These models often operate as "black boxes," making it difficult for users to understand how decisions are made. This lack of transparency can be problematic, especially in high-stakes fields like healthcare or finance, where understanding the rationale behind a model's prediction is crucial. For example, if a deep learning model suggests a treatment plan for a patient, doctors need to trust and understand the reasoning behind that suggestion. Efforts to improve model interpretability are ongoing, but it remains a complex issue that needs addressing.
Additionally, the computational costs associated with training deep learning models can be prohibitive. The resources required—both in terms of hardware and energy consumption—are substantial. As deep learning models grow in size and complexity, so do the demands on computational power. This can limit accessibility for smaller organizations or researchers with fewer resources, creating a disparity in who can leverage these powerful tools.
In summary, while deep learning is reshaping the landscape of artificial intelligence, it is essential to acknowledge and address these challenges. By tackling issues related to data scarcity, model interpretability, and computational costs, we can pave the way for more robust and equitable applications of deep learning technology.
- What is the biggest challenge in deep learning today? The biggest challenge is often the need for large, high-quality datasets, which can be difficult to obtain in many fields.
- How does data quality affect deep learning models? Poor quality data can lead to biased and unreliable models, resulting in inaccurate predictions and outcomes.
- Why is interpretability important in deep learning? Interpretability is crucial for trust and transparency, especially in critical applications like healthcare and finance, where understanding model decisions is necessary.
- Are there alternatives to deep learning? Yes, traditional machine learning methods can sometimes be more effective, especially in scenarios with limited data or where interpretability is a priority.
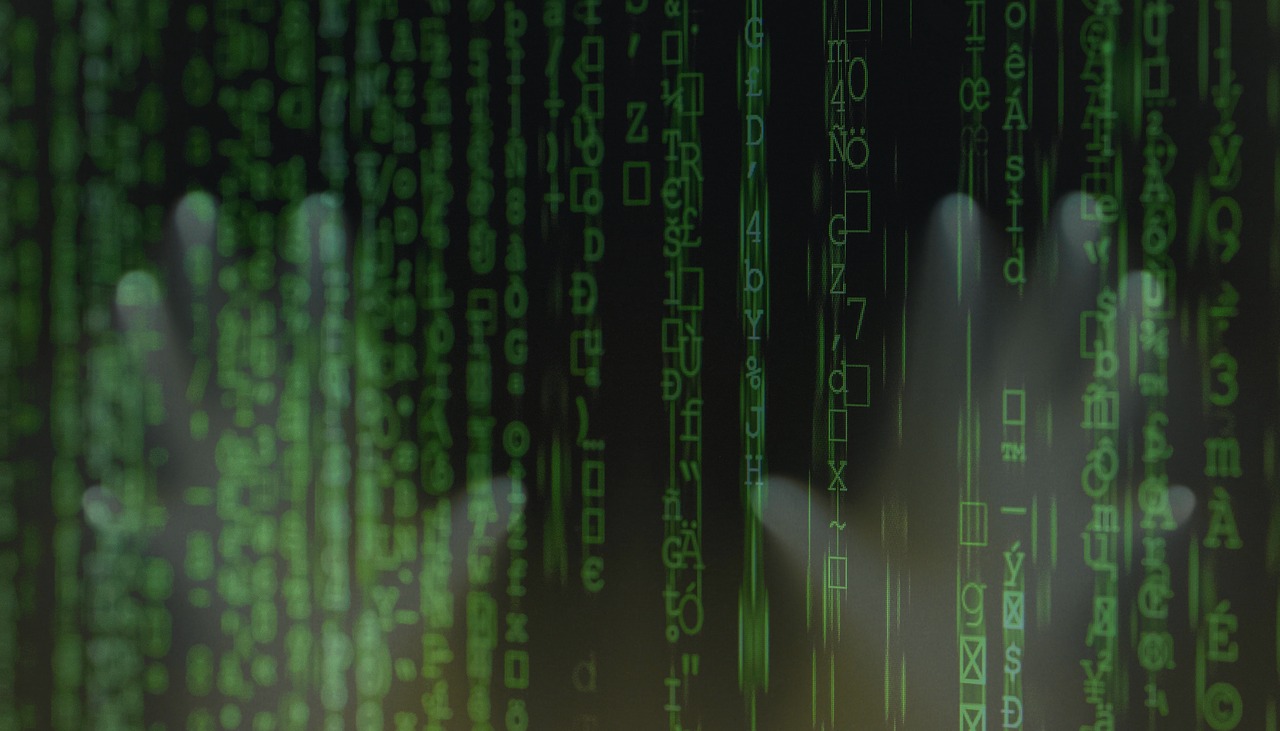
Data Scarcity and Quality
In the world of deep learning, data is often viewed as the lifeblood of any project. However, the reality is that many organizations face significant challenges related to data scarcity and quality. Imagine trying to build a skyscraper without a solid foundation; that’s how critical high-quality data is for the success of deep learning models. The need for large, diverse datasets is not just a minor hurdle; it's a significant barrier that can hinder the effectiveness of even the most sophisticated algorithms.
When we talk about data scarcity, we're referring to the lack of sufficient data to train deep learning models effectively. This issue is particularly pronounced in specialized fields, such as healthcare or rare event prediction, where collecting data can be both challenging and time-consuming. For instance, consider a deep learning model designed to detect a rare disease. If there are only a handful of cases available for training, the model will struggle to learn the necessary patterns, leading to poor performance in real-world applications.
On the other hand, data quality is just as important. High-quality data is characterized by its accuracy, completeness, and relevance. Poor-quality data can introduce noise into the model, resulting in inaccurate predictions and unreliable outcomes. For example, if a dataset contains mislabeled images or incomplete records, the model's ability to generalize to new data diminishes significantly. This is akin to trying to solve a puzzle with missing pieces; the end result will never be complete or coherent.
To tackle these challenges, researchers and practitioners are exploring several strategies:
- Data Augmentation: This technique involves artificially increasing the size of the training dataset by creating modified versions of existing data. For example, in image processing, techniques like rotation, flipping, and cropping can help generate new images from the original dataset.
- Transfer Learning: By leveraging pre-trained models that have been trained on large datasets, practitioners can fine-tune these models on smaller, domain-specific datasets. This approach can significantly reduce the amount of data needed for effective training.
- Synthetic Data Generation: In some cases, generating synthetic data using simulations or generative models can fill gaps in the dataset, providing additional training examples without the need for real-world data collection.
Addressing data scarcity and quality is not just an academic exercise; it has real-world implications. Organizations that successfully navigate these challenges can unlock the full potential of deep learning, leading to innovations that can transform industries. As we continue to explore the depths of artificial intelligence, it becomes increasingly clear that the foundation of any successful deep learning initiative lies in the quality and quantity of the data it is built upon.
- What is data scarcity in deep learning? Data scarcity refers to the insufficient amount of data available for training deep learning models, which can lead to poor performance.
- How does data quality affect deep learning models? High-quality data is crucial for accurate predictions. Poor-quality data can introduce noise and lead to unreliable outcomes.
- What are some strategies to overcome data scarcity? Strategies include data augmentation, transfer learning, and synthetic data generation.
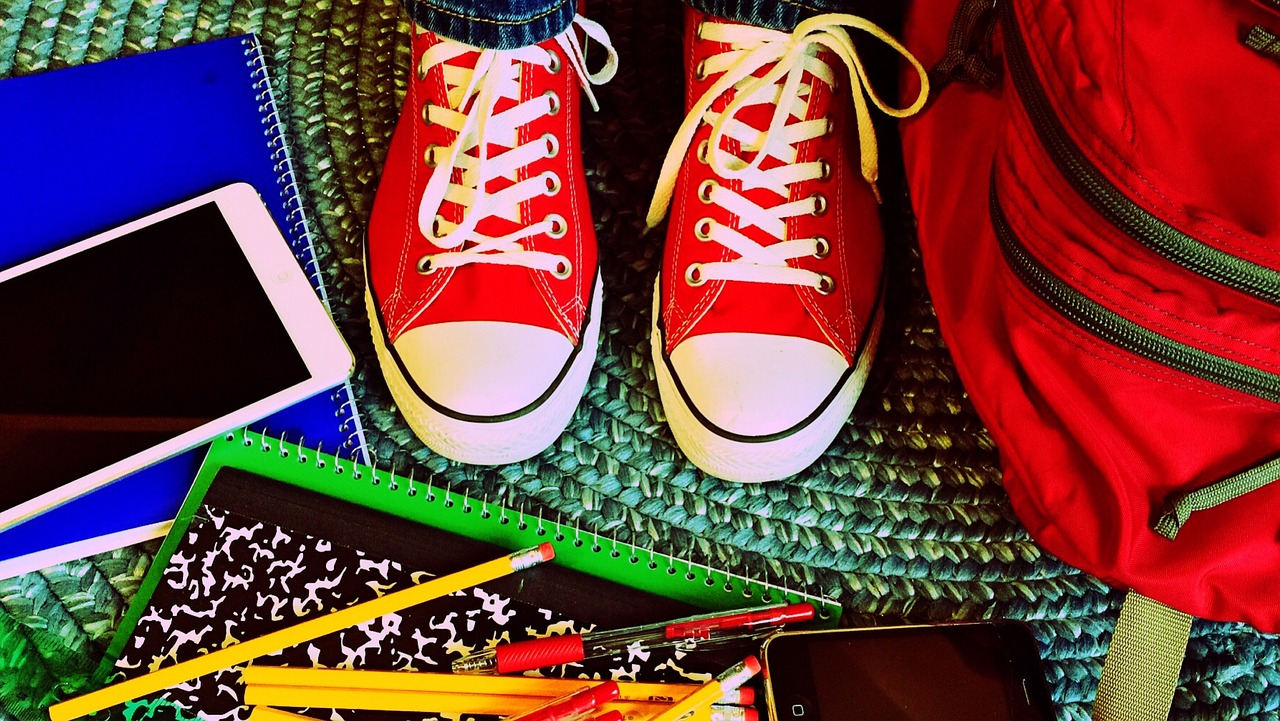
Interpreting Deep Learning Models
When we talk about deep learning, we're diving into a realm that often feels like black magic. Models can achieve astonishing feats, but understanding how they arrive at their conclusions is a different ballgame altogether. This lack of transparency can be a major roadblock, especially in fields where trust is paramount, like healthcare or finance. So, how do we make sense of these complex models? Well, it starts with a few key techniques designed to shed light on the inner workings of deep learning.
One of the most popular methods for interpreting deep learning models is called Layer-wise Relevance Propagation (LRP). This technique essentially breaks down the model's predictions and assigns relevance scores to each input feature. Imagine peeling back the layers of an onion; LRP helps us see which features contributed most to the final decision. For instance, in medical imaging, this could mean highlighting specific areas in an X-ray that led a model to diagnose a condition.
Another useful approach is SHAP (SHapley Additive exPlanations), which uses game theory to determine the contribution of each feature to the model's output. Think of it like a team sport where every player has a role to play. SHAP quantifies how much each feature contributes to a particular prediction, allowing us to understand the 'why' behind the model's decisions. This is particularly beneficial in scenarios where stakeholders need to justify or explain the model’s output.
Moreover, Grad-CAM (Gradient-weighted Class Activation Mapping) is a fantastic tool for visualizing the areas of an image that most influenced the model's prediction. It generates heatmaps that highlight regions of interest, making it easier for practitioners to interpret the model's focus. This is especially useful in applications like autonomous driving, where understanding what the model sees can be a matter of safety.
Despite these advancements, interpreting deep learning models is still a work in progress. The challenge lies not just in developing these tools but also in integrating them into existing workflows. As we strive for more interpretability, it’s crucial to balance transparency with performance. After all, the goal is not just to understand our models better but to ensure they are reliable and trustworthy.
In summary, while deep learning models can be complex and opaque, several techniques are emerging to help us decode their decisions. By employing methods like LRP, SHAP, and Grad-CAM, we can begin to bridge the gap between model performance and interpretability. As we continue to innovate in this space, we’ll likely see even more tools developed that prioritize transparency, allowing us to harness the full potential of deep learning while maintaining trust in its applications.
- What is the importance of interpreting deep learning models?
Interpreting deep learning models is crucial for building trust, ensuring accountability, and enhancing model performance across various applications. - How does SHAP work?
SHAP utilizes concepts from game theory to measure the contribution of each feature to the model's predictions, providing a clear understanding of the decision-making process. - Can interpretability tools be applied to all deep learning models?
While many interpretability tools are versatile, their effectiveness can vary depending on the model architecture and the specific application.
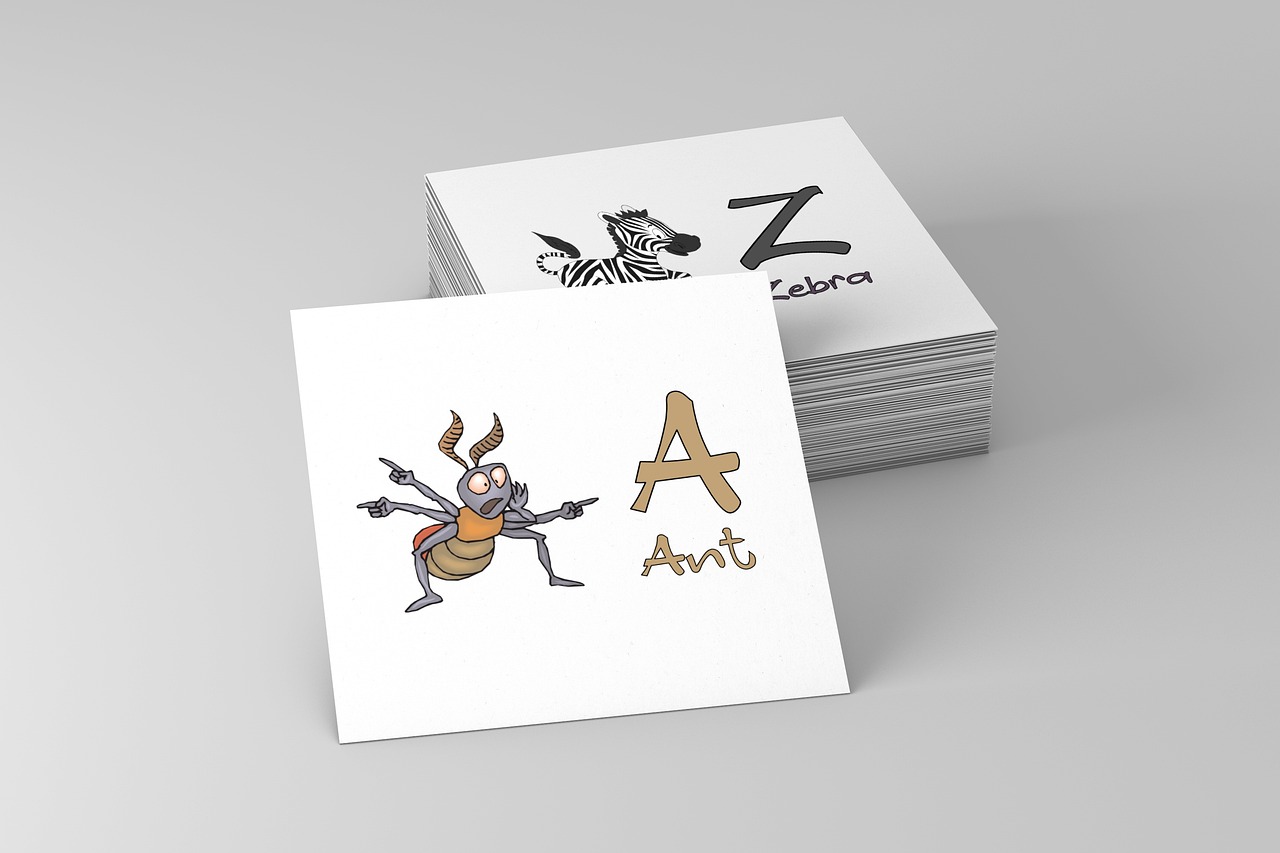
Future Trends in Deep Learning
As we look to the horizon of artificial intelligence, the future of deep learning is not just bright; it's practically glowing with potential! Innovations are sprouting like wildflowers in spring, and it's fascinating to consider how these advancements will reshape industries and our everyday lives. One of the most exciting trends is the rise of unsupervised learning. Unlike traditional methods that require labeled data, unsupervised learning allows algorithms to explore and learn from unstructured data. Imagine teaching a child to recognize objects without telling them what each one is called! This approach could lead to breakthroughs in fields such as natural language processing and image analysis.
Another trend on the rise is transfer learning. This technique allows models trained on one task to be repurposed for another, significantly reducing the amount of data and time needed for training. For example, a model trained to recognize cats can be fine-tuned to identify dogs with minimal additional data. The implications of this are enormous, especially for startups and smaller companies that may lack the resources to train models from scratch. Imagine the possibilities when businesses can leverage existing models to jumpstart their AI initiatives!
Moreover, the integration of deep learning with edge computing is set to revolutionize how we process data. By moving computations closer to the data source, we can reduce latency and improve response times for applications like autonomous vehicles and smart devices. Picture a self-driving car making split-second decisions based on real-time data without having to communicate with a distant server—it’s a game-changer!
In addition to these trends, we are also witnessing a surge in the development of explainable AI (XAI). As deep learning models grow more complex, understanding their decision-making processes becomes crucial. XAI aims to make these black-box models more transparent, allowing users to grasp how and why decisions are made. This is essential for building trust, especially in sensitive areas like healthcare and finance. Imagine receiving a medical diagnosis from an AI system that not only tells you what’s wrong but also explains the reasoning behind its conclusion!
Finally, as we embrace the future of deep learning, we can’t ignore the ethical considerations that come with it. As AI systems become more powerful, ensuring they are used responsibly will be paramount. This includes addressing biases in datasets, ensuring data privacy, and promoting fairness in AI applications. The conversation around ethical AI is gaining momentum, and it’s crucial for developers, businesses, and policymakers to collaborate and establish guidelines that promote the responsible use of technology.
- What is unsupervised learning? - It is a type of machine learning where algorithms learn from unstructured data without labeled outputs.
- How does transfer learning work? - Transfer learning allows a pre-trained model to be adapted for a different but related task, saving time and resources.
- What is explainable AI? - Explainable AI refers to methods and techniques that make the outputs of AI models understandable to humans.
- Why is ethical AI important? - Ethical AI ensures that AI systems are used responsibly, addressing issues like bias, privacy, and fairness.
Frequently Asked Questions
-
What is deep learning?
Deep learning is a subset of artificial intelligence (AI) that focuses on using neural networks with many layers (hence "deep") to analyze various types of data. It mimics the way humans learn from experience, making it particularly effective for tasks like image and speech recognition.
-
How does deep learning differ from traditional machine learning?
While traditional machine learning relies on feature extraction and often requires manual intervention to identify patterns, deep learning automates this process through its layered architecture. This allows deep learning models to learn complex patterns directly from raw data without extensive preprocessing.
-
What are Convolutional Neural Networks (CNNs)?
CNNs are a class of deep learning algorithms specifically designed for processing structured grid data, such as images. They use convolutional layers to automatically detect features and patterns, making them highly effective for tasks like image classification and object detection.
-
How are CNNs used in healthcare?
CNNs are transforming healthcare by enhancing medical imaging diagnostics. They can analyze X-rays, MRIs, and other images to identify anomalies, assisting doctors in making quicker and more accurate diagnoses, ultimately improving patient care.
-
What role do Recurrent Neural Networks (RNNs) play?
RNNs are designed to handle sequential data, making them ideal for applications like natural language processing and time series forecasting. They remember previous inputs due to their internal memory, allowing them to make predictions based on sequences.
-
What are the challenges faced by deep learning?
Deep learning faces several challenges, including the need for large datasets, high computational costs, and difficulties in model interpretability. These can hinder the deployment of deep learning solutions in real-world scenarios.
-
How can data scarcity be addressed in deep learning?
To tackle data scarcity, researchers are exploring techniques such as data augmentation, transfer learning, and synthetic data generation. These methods help improve model performance even when high-quality datasets are limited.
-
Why is model interpretability important?
Model interpretability is crucial for building trust in AI systems. Understanding how a deep learning model makes decisions can help users and stakeholders ensure the model is functioning correctly and ethically, especially in sensitive areas like healthcare and finance.
-
What future trends can we expect in deep learning?
Future trends in deep learning include advancements in unsupervised learning and transfer learning, which will enable models to learn more efficiently from fewer examples. These developments could significantly enhance AI's capabilities across various industries.