The Prospective Future of AI: Quantum Computing
As we stand on the brink of a technological revolution, the convergence of artificial intelligence (AI) and quantum computing is set to redefine the landscape of innovation. Imagine a world where machines not only learn but also think at lightning speeds, solving problems that are currently beyond our reach. This article explores the intersection of these two groundbreaking technologies, discussing potential advancements, challenges, and the transformative impact they may have on various industries in the coming years.
To grasp the potential of quantum computing, we first need to understand its fundamental principles. Unlike classical computers that use bits as the smallest unit of data, quantum computers utilize quantum bits or qubits. These qubits can exist in multiple states simultaneously, thanks to a phenomenon known as superposition. This allows quantum computers to perform complex calculations at unprecedented speeds, making them exceptionally powerful for specific tasks. Additionally, qubits can be entangled, meaning the state of one qubit can depend on the state of another, no matter the distance between them. This unique property enables quantum computers to process vast amounts of data in parallel, offering a glimpse into a future where computational limits are vastly expanded.
The combination of AI and quantum computing holds immense potential, creating a synergy that could lead to unprecedented breakthroughs. Imagine AI systems that can analyze vast datasets in real-time, uncovering patterns and insights that were previously hidden. This section examines how these technologies can enhance each other, leading to advancements in data analysis, machine learning, and problem-solving capabilities.
One of the most exciting prospects of integrating quantum computing with AI is the significant speedup in data processing tasks. Traditional AI applications often struggle with large datasets, leading to delays in decision-making. However, quantum algorithms can outperform classical methods in specific scenarios. For instance, consider the following:
- Optimization Problems: Quantum algorithms can solve complex optimization problems much faster than classical algorithms, making them ideal for logistics, finance, and resource management.
- Machine Learning: Quantum machine learning algorithms can analyze data more efficiently, potentially leading to better predictive models and insights.
- Simulation of Quantum Systems: Quantum computing can simulate molecular interactions at an atomic level, which is a game-changer for industries like pharmaceuticals.
These advancements will not only provide faster insights but also significantly improve the accuracy of AI models, making them more reliable and effective.
Several quantum algorithms, such as Grover's and Shor's, can be tailored for AI applications. Grover's algorithm, for instance, can search unsorted databases quadratically faster than any classical algorithm, while Shor's algorithm can factor large numbers exponentially faster. By leveraging these algorithms, AI systems can optimize their performance and efficiency, leading to breakthroughs in various fields.
In the real world, the integration of AI and quantum computing is already showing promise in several areas:
- Drug Discovery: Researchers are using quantum computing to simulate molecular structures, speeding up the drug discovery process.
- Financial Modeling: Quantum algorithms can analyze market trends and risks more effectively than classical methods, providing better investment strategies.
- Complex System Simulations: Industries such as climate science and logistics benefit from enhanced simulations that can predict outcomes with greater accuracy.
Despite the immense potential, several challenges hinder the widespread adoption of quantum computing in AI. Technical limitations, such as qubit coherence times and error rates, pose significant hurdles. Additionally, there is a pressing need for skilled professionals who can bridge the gap between quantum physics and AI development. Addressing these challenges will be crucial for unlocking the full potential of these technologies.
As AI and quantum computing evolve, ethical concerns arise that cannot be overlooked. The implications of these technologies on privacy, security, and decision-making processes in society are profound. We must navigate these waters carefully to ensure that advancements benefit humanity as a whole.
One of the most pressing concerns is data privacy. Quantum computing poses risks to current encryption methods, potentially compromising sensitive data. As quantum computers become more powerful, they could break traditional encryption standards, leading to significant vulnerabilities. This scenario underscores the urgent need for new security measures that can withstand the capabilities of quantum computing.
Moreover, AI systems can perpetuate biases, and the integration of quantum computing may exacerbate these issues. As we develop more complex algorithms, it is essential to address bias and ensure fairness in AI systems. This means implementing rigorous testing and validation processes to mitigate bias and promote equitable outcomes.
Q1: What is quantum computing?
Quantum computing is a revolutionary approach to processing information that utilizes quantum bits (qubits) to perform calculations at unprecedented speeds.
Q2: How does AI benefit from quantum computing?
Quantum computing enhances AI by enabling faster data processing, improving machine learning algorithms, and optimizing complex problem-solving tasks.
Q3: What are the challenges of integrating AI and quantum computing?
Challenges include technical limitations, the need for skilled professionals, and addressing ethical concerns such as data privacy and bias.
Q4: What are some real-world applications of AI and quantum computing?
Real-world applications include drug discovery, financial modeling, and complex system simulations, showcasing the transformative potential of these technologies.
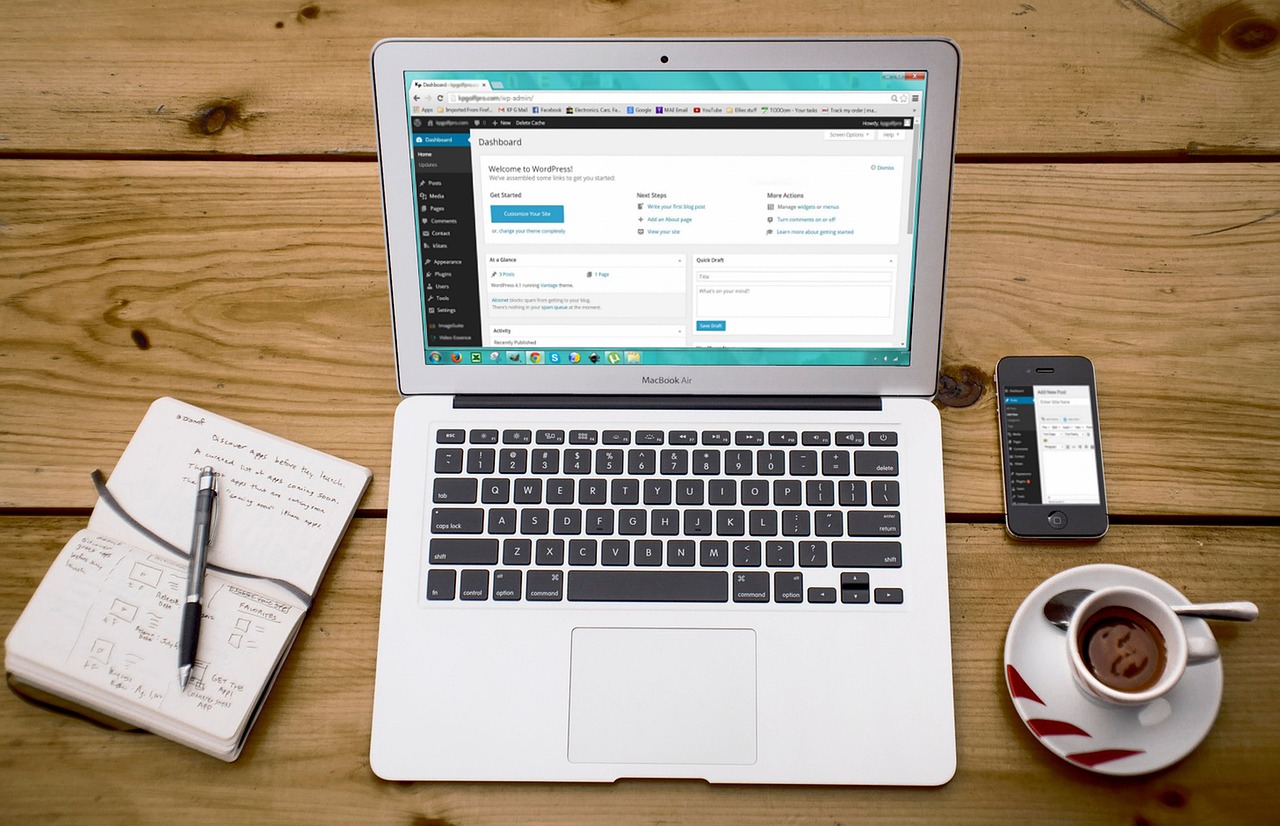
Understanding Quantum Computing
Quantum computing represents a revolutionary leap in the field of information processing. Unlike classical computers, which use bits as the smallest unit of data (0s and 1s), quantum computers utilize quantum bits or qubits. These qubits can exist in multiple states simultaneously, thanks to a phenomenon known as superposition. Imagine a spinning coin; while it’s spinning, it’s not just heads or tails but can be considered both until it lands. This unique capability allows quantum computers to perform complex calculations at speeds that were once thought to be impossible.
But wait, there's more! Quantum computers also leverage another principle called entanglement. When qubits become entangled, the state of one qubit can depend on the state of another, no matter how far apart they are. This interconnectedness can lead to exponential increases in processing power. For example, while a classical computer might take years to solve certain problems, a quantum computer could potentially solve them in mere seconds. This is not just a theoretical concept; it’s a tangible shift that could redefine how we approach computing.
To better understand how quantum computing differs from classical computing, consider the following table:
Aspect | Classical Computing | Quantum Computing |
---|---|---|
Data Unit | Bit (0 or 1) | Qubit (0, 1, or both) |
Processing Speed | Linear scaling | Exponential scaling |
Problem Solving | Sequential | Simultaneous |
Principles Used | Boolean algebra | Quantum mechanics |
As we dive deeper into the world of quantum computing, it’s essential to grasp its potential applications. From cryptography to drug discovery, the possibilities seem endless. However, this technology is still in its infancy, and researchers are working tirelessly to overcome various challenges. The landscape of quantum computing is rapidly evolving, and as we continue to explore its intricacies, we can only imagine the transformative impact it will have on our lives and industries.
In conclusion, understanding quantum computing isn't just about grasping complex theories and principles; it’s about recognizing a new paradigm that could redefine our relationship with technology. As we stand on the brink of this new era, the question remains: are we ready to embrace the future of computing?
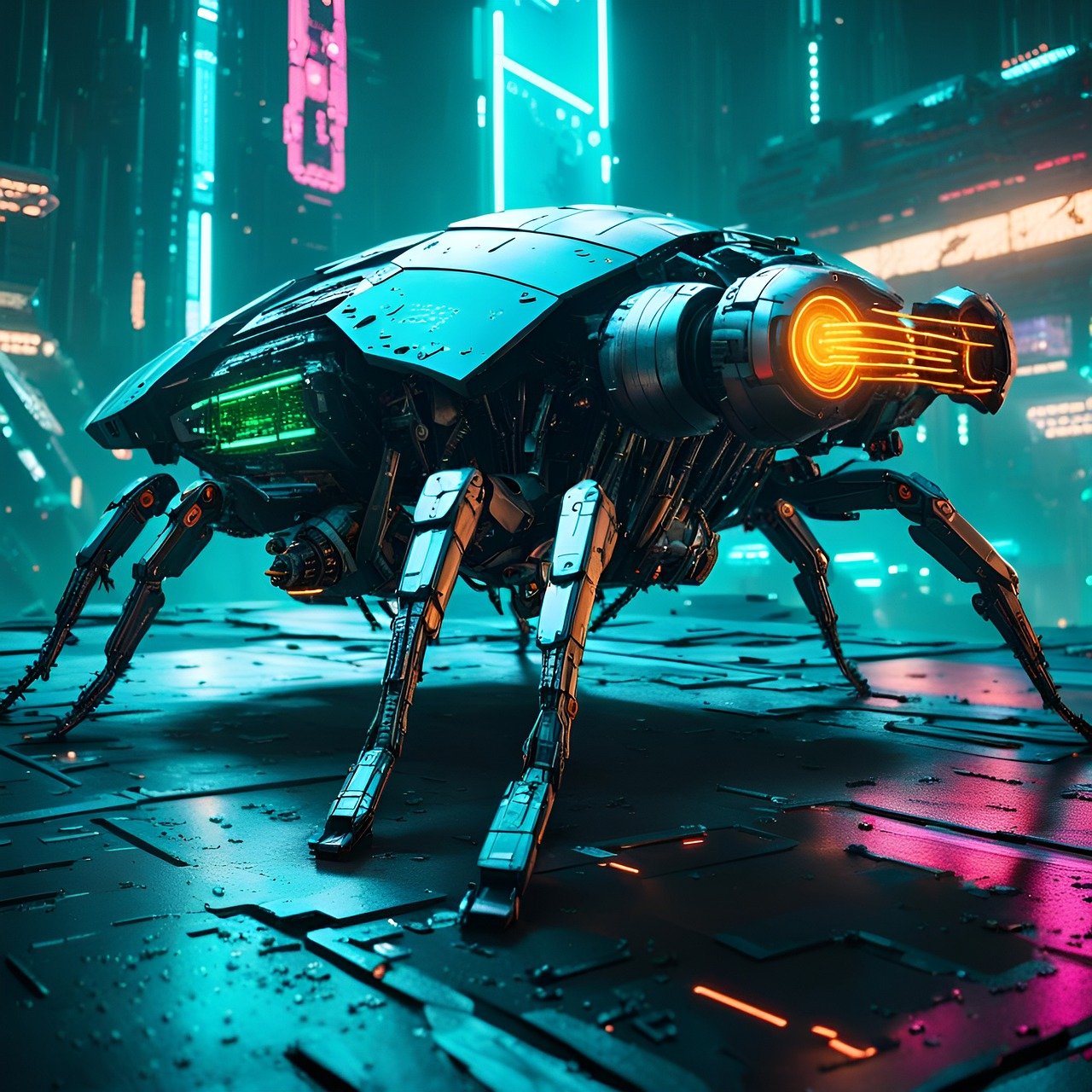
AI and Quantum Synergy
The fusion of artificial intelligence (AI) and quantum computing is like mixing oil and water, but in the most exciting way possible. Imagine the immense power of quantum bits, or qubits, working hand-in-hand with AI algorithms. This collaboration could unlock doors we didn't even know existed in the realms of data analysis, machine learning, and problem-solving. The synergy between these two groundbreaking technologies is not just a theoretical concept; it’s a tangible force that promises to revolutionize how we tackle complex challenges.
To illustrate this synergy, consider the way traditional computers process information. They operate on binary bits, which can be either 0 or 1. Quantum computers, however, leverage the unique properties of quantum mechanics, allowing qubits to exist in multiple states simultaneously. This capability enables quantum computers to perform certain calculations much faster than classical computers. When we apply this speed to AI, the results can be astounding. For instance, think about how quickly AI systems could analyze vast datasets or simulate complex scenarios if they were powered by quantum computing. It’s like comparing a bicycle to a rocket ship!
One of the most exciting prospects of AI and quantum synergy is the development of quantum algorithms specifically designed for AI applications. These algorithms can enhance machine learning processes, enabling AI systems to learn from data more efficiently and effectively. For example, consider Grover's algorithm, which can search unsorted databases quadratically faster than any classical algorithm. This speed boost could allow AI systems to sift through massive datasets in a fraction of the time, leading to quicker insights and more informed decision-making.
Moreover, the combination of AI and quantum computing can significantly enhance predictive analytics. By harnessing quantum computing's ability to process large amounts of data at lightning speed, AI can generate predictions that are not only faster but also more accurate. This could have profound implications across various industries, from healthcare to finance. Imagine AI systems that can predict disease outbreaks or market trends with unprecedented precision, allowing organizations to respond proactively rather than reactively.
However, the journey towards realizing this synergy is not without its hurdles. While the theoretical benefits are immense, the practical implementation of quantum computing in AI applications faces several challenges. The technology is still in its infancy, and many technical limitations need to be addressed before we can fully harness its potential. Additionally, there is a pressing need for skilled professionals who understand both AI and quantum computing to bridge the gap between these fields.
In conclusion, the intersection of AI and quantum computing is a thrilling frontier filled with potential. As we continue to explore this synergy, we may uncover groundbreaking solutions that can transform entire industries. The road ahead may be fraught with challenges, but the rewards of this technological marriage could be nothing short of revolutionary. Are you ready to embrace the future of AI powered by quantum computing?
- What is quantum computing? Quantum computing is a revolutionary computing technology that uses quantum bits (qubits) to perform calculations at speeds unattainable by classical computers.
- How does AI benefit from quantum computing? AI can leverage quantum computing to process data more efficiently, enhance machine learning algorithms, and improve predictive analytics.
- What are some real-world applications of AI and quantum computing synergy? Applications include drug discovery, financial modeling, and complex system simulations, where rapid data processing can lead to significant breakthroughs.
- What challenges do AI and quantum computing face? Challenges include technical limitations, the need for skilled professionals, and the integration of these technologies into existing systems.
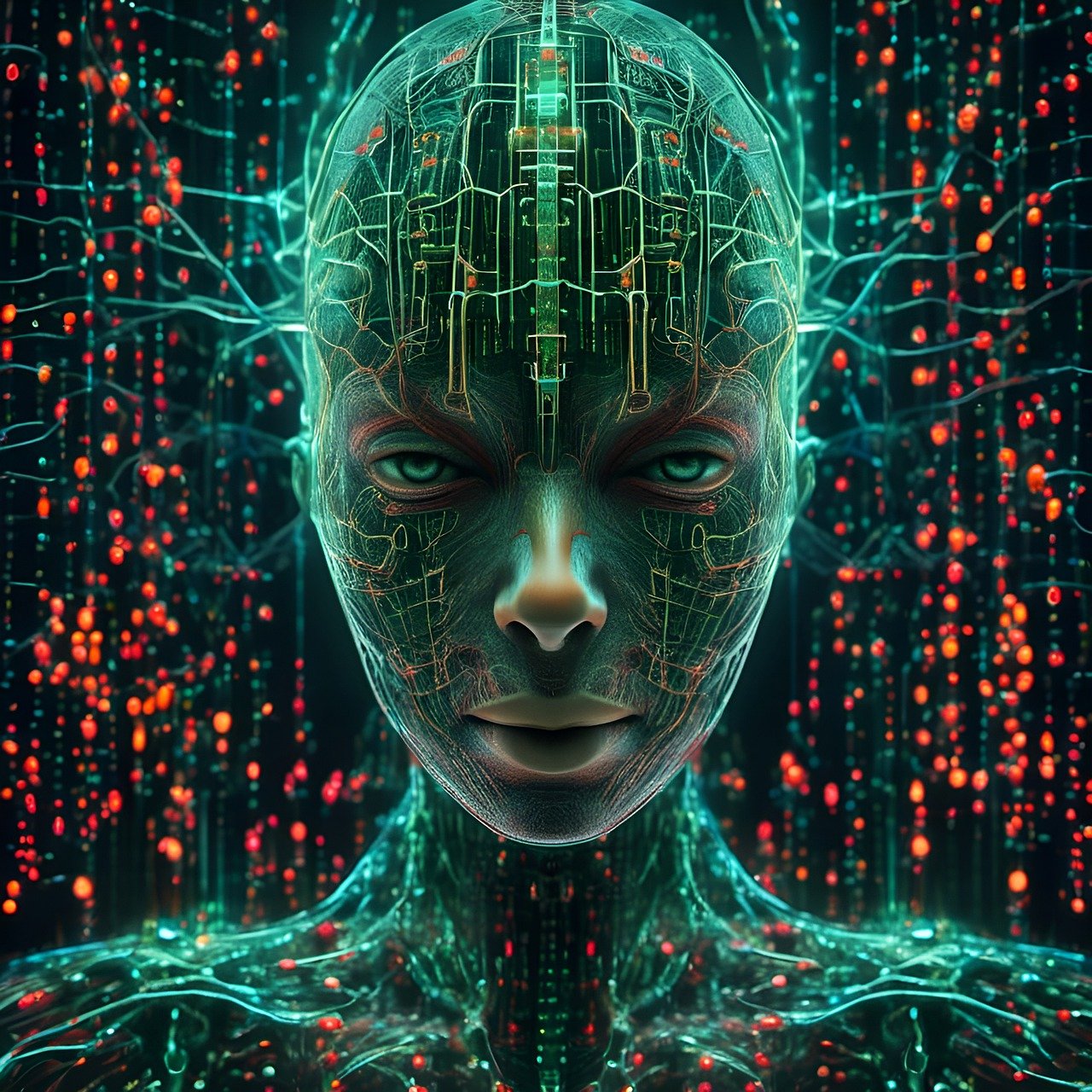
Enhanced Data Processing
In the rapidly evolving landscape of technology, the intersection of artificial intelligence (AI) and quantum computing is poised to redefine how we process data. Imagine a world where complex calculations that currently take days or even weeks can be completed in mere seconds. This is not just a dream; it’s becoming a reality with quantum computing. By leveraging the unique properties of quantum bits, or qubits, we can achieve a level of data processing that classical computers simply cannot match.
One of the most compelling aspects of quantum computing is its ability to handle vast datasets with remarkable efficiency. Traditional algorithms struggle with the sheer volume of data generated today, leading to bottlenecks in analysis and decision-making. Quantum algorithms, on the other hand, can optimize these processes, allowing for faster insights and solutions. For instance, consider the following scenarios where quantum algorithms could outperform classical methods:
- Drug Discovery: Quantum computing can analyze molecular structures and interactions at an unprecedented speed, drastically reducing the time needed for drug development.
- Financial Modeling: In finance, quantum algorithms can simulate market conditions and assess risk more effectively, providing better strategies for investment.
- Complex System Simulations: Whether it's climate modeling or urban planning, quantum computing can manage and analyze multi-variable systems more efficiently.
To illustrate the potential of quantum computing in enhancing data processing for AI, let’s take a closer look at some specific quantum algorithms that are making waves in this field. For example, Grover's algorithm offers a quadratic speedup for unstructured search problems, meaning that tasks that would typically require N steps can be completed in roughly √N steps. This is particularly useful for AI applications that rely on searching through large datasets to identify patterns or anomalies.
Similarly, Shor's algorithm revolutionizes factoring large numbers, which is fundamental for encryption and security. By harnessing the power of quantum mechanics, it could potentially break encryption methods that protect sensitive data today. This not only highlights the incredible speed of quantum computing but also raises questions about security and data privacy in the future.
As we explore these advancements, it’s essential to recognize that the integration of AI and quantum computing is still in its infancy. Challenges remain, including the need for robust quantum hardware and the development of new algorithms specifically designed for AI tasks. However, the potential benefits of enhanced data processing are immense, paving the way for breakthroughs that could change industries forever.
1. What is quantum computing?
Quantum computing is a type of computation that uses quantum mechanics to process information. Unlike classical computers that use bits, quantum computers use qubits, which can represent both 0 and 1 simultaneously, allowing for much faster calculations.
2. How does quantum computing enhance AI?
Quantum computing enhances AI by enabling faster data processing, improving algorithm efficiency, and allowing for the analysis of larger datasets, which can lead to more accurate predictions and insights.
3. What are some real-world applications of AI and quantum computing?
Real-world applications include drug discovery, financial modeling, and complex system simulations, where the combination of AI and quantum computing can significantly improve outcomes.
4. What challenges does quantum computing face?
Challenges include technical limitations, the need for skilled professionals, and the development of new algorithms tailored for quantum computing applications.
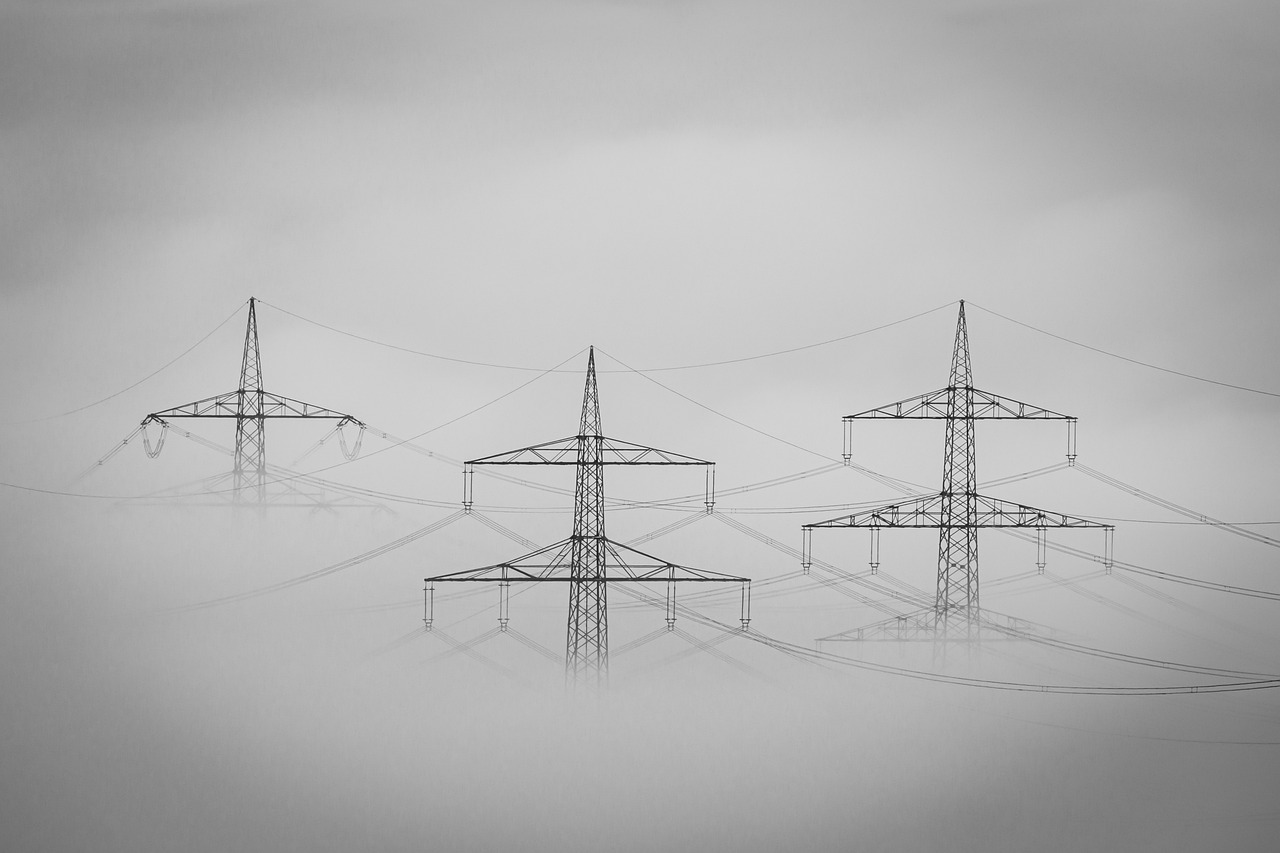
Quantum Algorithms for AI
Quantum algorithms are the backbone of the synergy between artificial intelligence (AI) and quantum computing. These algorithms leverage the unique properties of quantum mechanics, such as superposition and entanglement, to perform tasks that would be infeasible for classical computers. One of the most notable quantum algorithms is Grover's algorithm, which provides a quadratic speedup for unstructured search problems. Imagine trying to find a needle in a haystack; while a classical computer would sift through each strand of hay one by one, Grover's algorithm allows a quantum computer to explore multiple strands simultaneously, drastically reducing the time it takes to find the needle.
Another significant quantum algorithm is Shor's algorithm, which is particularly famous for its ability to factor large integers efficiently. This capability has profound implications for cryptography, as many encryption techniques rely on the difficulty of factoring large numbers. By integrating Shor's algorithm into AI systems, we can enhance the security of machine learning models, ensuring that sensitive data remains protected even in a quantum computing era.
Moreover, quantum algorithms can be tailored specifically for various AI applications, leading to optimized performance and efficiency. For instance, in the realm of machine learning, quantum versions of classical algorithms, such as quantum support vector machines or quantum neural networks, are being developed. These quantum-enhanced models can process vast amounts of data more quickly than their classical counterparts, enabling faster training times and improved predictive accuracy.
To illustrate the potential of quantum algorithms in AI, consider the following table that compares classical and quantum algorithm capabilities:
Algorithm Type | Classical Algorithms | Quantum Algorithms |
---|---|---|
Search Problems | O(N) | O(√N) |
Integer Factorization | O(exp(N^(1/3))) | O((log N)²) |
Training Time for Machine Learning | Linear or Polynomial Time | Potentially Exponential Speedup |
The integration of these quantum algorithms into AI frameworks is not just theoretical; researchers are already exploring practical implementations that can transform industries. For example, in drug discovery, quantum algorithms can analyze molecular structures and interactions at unprecedented speeds, accelerating the identification of potential drug candidates. Similarly, in finance, quantum algorithms can optimize portfolios and assess risk factors faster than traditional methods, providing a competitive edge in market analysis.
In conclusion, the development of quantum algorithms tailored for AI applications is paving the way for a future where intelligent systems can operate at speeds and efficiencies previously thought impossible. As we continue to explore this exciting intersection, the potential for groundbreaking advancements in technology and society is limitless.

Real-World Applications
As we stand on the brink of a technological revolution, the integration of artificial intelligence (AI) and quantum computing is beginning to bear fruit in several industries. Imagine a world where complex problems are solved in mere seconds, where the potential for innovation is limitless. This is not just a dream; it’s becoming a reality through real-world applications that showcase the synergy between these groundbreaking technologies.
One of the most promising fields is drug discovery. Traditional methods of discovering new medications can take years, if not decades, and often involve a lot of trial and error. However, with the power of quantum computing, researchers can analyze vast datasets and simulate molecular interactions at unprecedented speeds. For instance, quantum algorithms can predict how different compounds will interact with biological targets, significantly shortening the time it takes to bring new drugs to market.
In the realm of financial modeling, quantum computing is revolutionizing how financial institutions assess risk and optimize portfolios. By utilizing quantum algorithms, banks can perform complex calculations that would take classical computers an impractical amount of time. This capability allows them to simulate various market scenarios and make more informed decisions, ultimately leading to better financial products for consumers. Imagine being able to predict market shifts before they happen—quantum computing makes this possible.
Another exciting application can be found in complex system simulations. Industries such as logistics and supply chain management are leveraging AI combined with quantum computing to optimize their operations. For example, companies can simulate entire supply chains to identify bottlenecks and inefficiencies, allowing them to make data-driven decisions that enhance productivity. The ability to forecast outcomes based on real-time data can drastically improve operational efficiency.
Moreover, the field of climate modeling stands to benefit immensely from this technological convergence. Quantum computing can process vast amounts of environmental data, enabling scientists to create more accurate models of climate change. By understanding the intricate interactions within our climate systems, we can better prepare for future challenges and implement effective strategies for sustainability.
In summary, the real-world applications of AI and quantum computing are not just theoretical; they are actively transforming industries. As these technologies continue to evolve, we can expect to see even more innovative solutions that will drive progress and change the way we live and work. The future is indeed bright, and the possibilities are endless.
- What industries will benefit most from AI and quantum computing? Industries such as healthcare, finance, logistics, and climate science are poised to gain significantly from these technologies.
- How does quantum computing enhance AI capabilities? Quantum computing can process data at speeds unattainable by classical computers, allowing AI systems to analyze and learn from vast datasets more efficiently.
- Are there any risks associated with the integration of AI and quantum computing? Yes, there are challenges, including data privacy issues and the potential for bias in AI algorithms that must be addressed as these technologies evolve.
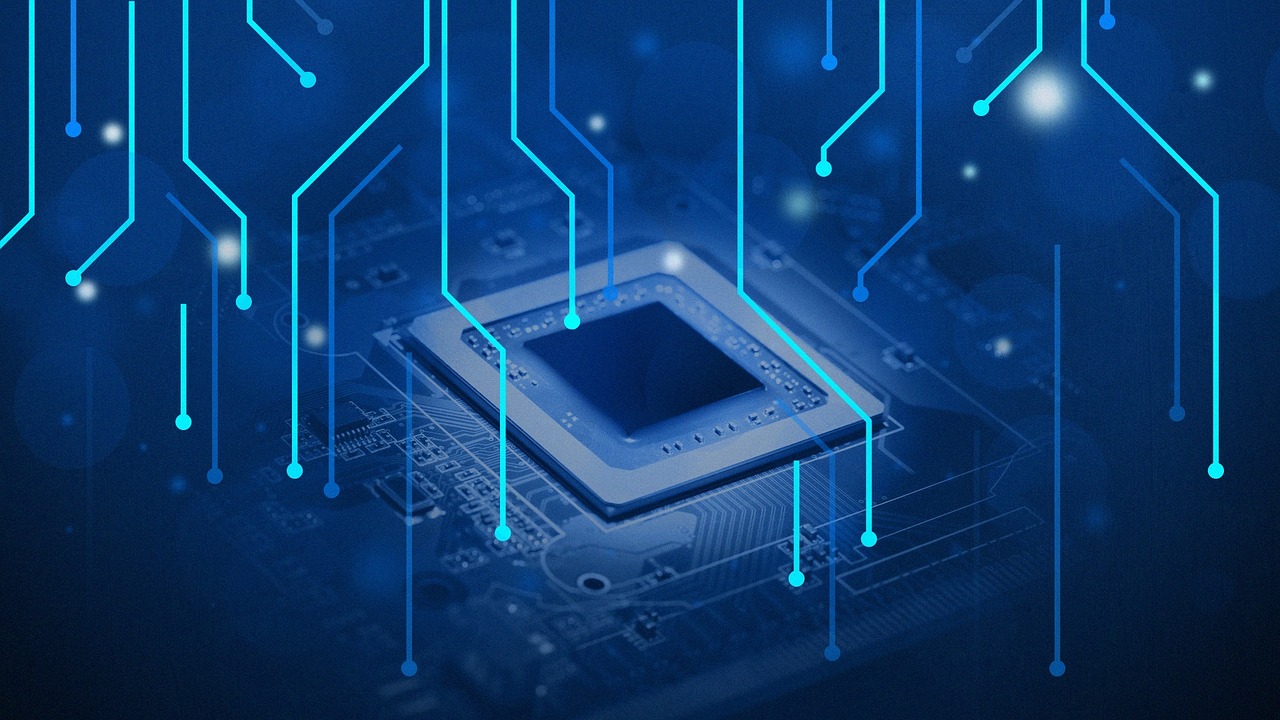
Challenges Ahead
The journey toward integrating quantum computing with artificial intelligence is not without its hurdles. As we stand on the brink of a technological revolution, several challenges loom large, threatening to slow down progress. These challenges range from technical limitations to the pressing need for skilled professionals in the field. Imagine trying to cross a bridge that hasn’t been fully built yet; that’s how it feels navigating the current landscape of quantum AI.
One of the most significant obstacles is the technical limitations of quantum computers themselves. While they promise unprecedented processing power, the reality is that most quantum systems are still in their infancy. They are prone to errors and require extremely low temperatures to function, which complicates their integration into existing infrastructures. This is akin to trying to use a high-performance sports car on a bumpy dirt road—it just doesn’t work well without the right conditions.
Furthermore, the shortage of skilled professionals in both AI and quantum computing fields poses a substantial barrier. The complexity of quantum mechanics combined with advanced AI techniques means that only a handful of experts truly understand how to harness this synergy effectively. Educational institutions are scrambling to catch up, but the demand far exceeds the supply. Companies looking to innovate may find themselves in a talent drought, unable to secure the expertise needed to drive their quantum AI initiatives forward.
In addition to these challenges, there are also financial constraints. Developing quantum technologies and training personnel requires significant investment. Many organizations, especially startups, may find it difficult to allocate the necessary resources. It’s like trying to fund a space mission when you can barely afford a ticket to the local amusement park. The financial burden can deter innovation and slow down the pace of development.
Moreover, the integration of quantum computing into existing AI frameworks can lead to compatibility issues. Current AI systems are designed to run on classical computing architectures, and adapting them to leverage quantum capabilities may not be straightforward. This is similar to trying to fit a square peg into a round hole; without the right adjustments, the systems may not function optimally.
Lastly, we must not overlook the regulatory challenges that accompany the rise of these technologies. As quantum computing becomes more prevalent, governments and regulatory bodies will need to establish guidelines to ensure safe and ethical use. This could lead to a complex web of regulations that may hinder innovation if not managed properly.
In summary, while the potential of combining AI with quantum computing is enormous, we must navigate a series of challenges to realize this vision. From technical limitations and skills shortages to financial constraints and regulatory hurdles, the path forward is fraught with difficulties. However, overcoming these challenges could pave the way for groundbreaking advancements that could transform industries and improve lives.
- What is quantum computing? Quantum computing is a revolutionary approach to processing information using quantum bits (qubits) to perform complex calculations at much higher speeds than classical computers.
- How can AI benefit from quantum computing? AI can significantly benefit from quantum computing through enhanced data processing, optimization of algorithms, and improved machine learning capabilities.
- What are the main challenges in integrating AI with quantum computing? The main challenges include technical limitations, a shortage of skilled professionals, financial constraints, compatibility issues, and regulatory challenges.
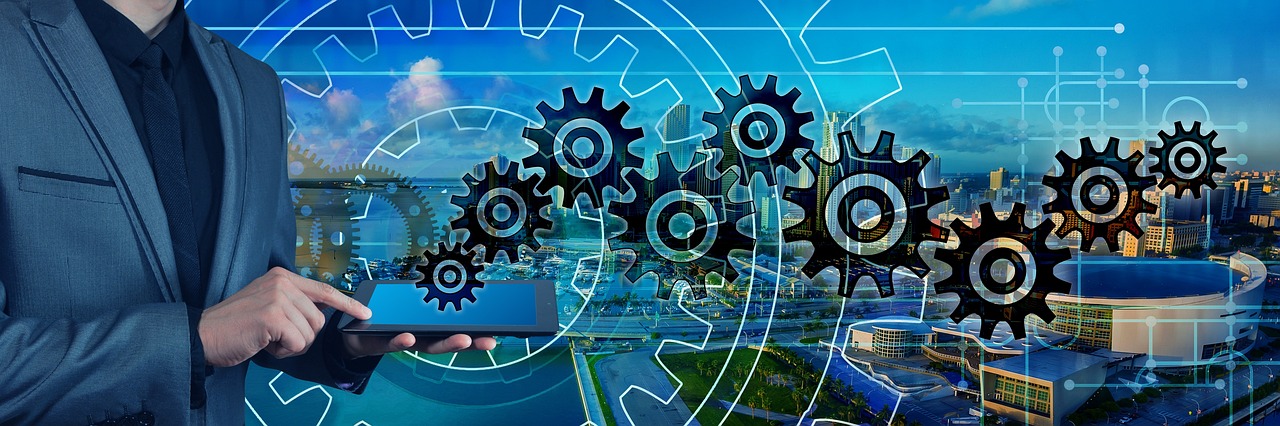
Ethical Considerations
As we stand on the brink of a technological revolution with the convergence of artificial intelligence and quantum computing, it's crucial to pause and reflect on the ethical implications of these advancements. The rapid evolution of these technologies brings about profound changes in our society, but with great power comes great responsibility. One of the most pressing concerns revolves around data privacy. With quantum computing's ability to potentially break current encryption methods, sensitive information could be at risk. Imagine a world where your private conversations, financial details, and personal data are no longer secure. This possibility raises urgent questions about how we protect our information in a quantum future.
Moreover, the integration of AI with quantum computing could amplify existing biases within AI systems. If these systems learn from historical data that contains biases, they may perpetuate and even exacerbate these issues, leading to unfair outcomes in critical areas such as hiring, law enforcement, and lending. It's essential to ensure that as we develop these powerful tools, we also prioritize fairness and equity. Addressing bias in AI algorithms is not just a technical challenge; it requires a commitment to ethical standards and practices that prioritize human rights and dignity.
In addition to these concerns, we must also consider the implications for decision-making processes. As AI systems become more sophisticated, they will increasingly influence our choices, from what news we read to how we manage our health. This raises questions about accountability and transparency. Who is responsible when an AI system makes a mistake? How can we ensure that these systems act in the best interests of individuals and society as a whole?
To navigate these ethical challenges, a multidisciplinary approach is essential. Engaging ethicists, technologists, policymakers, and the public in discussions about the future of AI and quantum computing can help us establish a framework for responsible innovation. This collaboration can pave the way for creating guidelines and regulations that not only foster innovation but also protect individuals and communities from potential harms.
In summary, while the potential for AI and quantum computing to transform our world is immense, we must tread carefully. By addressing ethical considerations upfront, we can harness these technologies to create a future that benefits everyone, rather than a select few. It's not just about what these technologies can do; it's about what they should do for humanity.
- What are the main ethical concerns associated with AI and quantum computing?
The primary concerns include data privacy, bias and fairness in AI algorithms, and the implications for decision-making processes. - How can we ensure data privacy in a quantum computing world?
Developing new encryption methods and security measures will be crucial to protect sensitive information from quantum threats. - What steps can be taken to address bias in AI systems?
Regular audits of AI systems, diverse training datasets, and inclusive development teams can help mitigate bias. - Who is responsible for the decisions made by AI systems?
Accountability in AI decision-making is a complex issue that requires clear guidelines and regulations to determine responsibility.

Data Privacy Issues
The advent of quantum computing brings with it a wave of excitement, but it also raises significant data privacy concerns that cannot be ignored. As quantum technologies advance, they threaten to undermine the very foundations of our current encryption systems. Traditional encryption methods, such as RSA and AES, rely on the complexity of certain mathematical problems, which quantum computers can solve with astonishing speed. This means that sensitive information, from personal data to corporate secrets, could be at risk of exposure.
Imagine a world where your private communications, financial transactions, and health records are no longer secure. It’s a daunting thought, isn’t it? The potential for quantum computers to crack encryption could lead to widespread data breaches and identity theft. As we increasingly rely on digital platforms for everything from banking to social networking, the implications of compromised data privacy become more severe. In fact, a recent study indicated that over 60% of organizations are concerned about the impact of quantum computing on their data security strategies.
To address these challenges, researchers and cybersecurity experts are racing against time to develop quantum-resistant encryption methods. These new cryptographic techniques are designed to withstand the computational power of quantum machines, ensuring that our data remains safe even in a post-quantum world. However, the transition to these new systems is not straightforward. It involves not only technological advancements but also significant changes in policy and regulation.
Moreover, the conversation around data privacy in the context of quantum computing is not just about technology; it’s also about ethics. As we navigate these uncharted waters, we must consider the implications of our choices. For instance, how do we ensure that the deployment of quantum technologies does not disproportionately affect vulnerable populations? The need for transparency and fairness in the development of these technologies is paramount. As we move forward, we must ask ourselves: Are we prepared to safeguard our privacy in the face of such rapid technological change?
In summary, the intersection of quantum computing and data privacy presents a complex landscape that requires careful consideration. As we embrace the potential of quantum technologies, we must also prioritize the protection of our personal information. The future of data privacy in a quantum world hinges on our ability to innovate responsibly and ethically.
- What is quantum computing? Quantum computing is a revolutionary technology that uses quantum bits (qubits) to perform complex calculations much faster than traditional computers.
- How does quantum computing affect data privacy? Quantum computing poses a threat to current encryption methods, potentially allowing unauthorized access to sensitive data.
- What are quantum-resistant encryption methods? These are advanced cryptographic techniques designed to secure data against the capabilities of quantum computers.
- Why is ethical consideration important in quantum computing? Ethical considerations ensure that the development and deployment of quantum technologies do not harm individuals or society, particularly vulnerable populations.

Bias and Fairness
As we delve deeper into the realms of artificial intelligence (AI) and quantum computing, one critical aspect that demands our attention is the issue of . AI systems are increasingly being integrated into decision-making processes across various sectors, from hiring practices to criminal justice. However, if these systems are trained on biased data, they can inadvertently perpetuate and amplify existing inequalities. Imagine a scenario where a hiring algorithm favors candidates based on flawed historical data, leading to a less diverse workforce. This is not just a theoretical concern; it’s a reality that many organizations face today.
The integration of quantum computing into AI could potentially exacerbate these biases if not handled correctly. Quantum algorithms can process vast amounts of data at speeds previously unimaginable, but if the data fed into these systems is biased, the outcomes will reflect those biases. For instance, if a quantum AI model is trained on data that disproportionately represents one demographic, it might make decisions that unfairly disadvantage others. Therefore, it is crucial to ensure that the data used is representative and fair.
Moreover, the complexity of quantum computing adds another layer of challenge. Unlike classical computing, where algorithms are more straightforward to interpret, quantum algorithms can be intricate and opaque. This opacity can make it difficult to identify and correct biases. To illustrate, consider the following table that outlines the potential sources of bias in AI systems:
Source of Bias | Description |
---|---|
Training Data | Data that is unbalanced or not representative of the population can lead to biased outcomes. |
Algorithm Design | Design choices made by developers can introduce bias if not carefully considered. |
Feedback Loops | Systems that learn from their own predictions can reinforce existing biases over time. |
Addressing bias in AI and quantum computing requires a concerted effort from developers, researchers, and policymakers. Here are some strategies that can be employed to promote fairness:
- Diverse Data Collection: Actively seek out diverse datasets to train AI models, ensuring that all demographics are represented.
- Algorithm Transparency: Develop models that allow for greater interpretability, making it easier to identify and rectify biases.
- Regular Audits: Implement regular audits of AI systems to assess their performance across different demographic groups.
In conclusion, as AI and quantum computing continue to evolve, the importance of addressing bias and ensuring fairness cannot be overstated. The potential for these technologies to transform industries is immense, but we must tread carefully to avoid exacerbating existing inequalities. By prioritizing fairness in AI development, we can harness the power of quantum computing responsibly and ethically, paving the way for a more equitable future.
- What is bias in AI? Bias in AI refers to the systematic favoritism or discrimination against certain groups based on the data used to train the algorithms.
- How can quantum computing help reduce bias? Quantum computing can process large datasets more efficiently, allowing for more nuanced analysis and potentially identifying biases that classical computing might miss.
- What are some examples of bias in AI systems? Examples include biased hiring algorithms that favor certain demographics or predictive policing systems that disproportionately target specific communities.
Frequently Asked Questions
- What is quantum computing?
Quantum computing is a groundbreaking technology that leverages the principles of quantum mechanics to process information. Unlike classical computers that use bits (0s and 1s), quantum computers utilize quantum bits or qubits, which can exist in multiple states simultaneously. This unique capability allows quantum computers to perform complex calculations at speeds that were previously unimaginable.
- How does AI benefit from quantum computing?
The synergy between AI and quantum computing can lead to remarkable advancements in various fields. Quantum computing can drastically enhance data processing speeds for AI applications, enabling faster insights and more efficient problem-solving. For example, quantum algorithms can optimize machine learning tasks, leading to superior performance compared to traditional methods.
- What are some real-world applications of AI and quantum computing?
AI and quantum computing are already making waves in several industries. Some notable applications include drug discovery, where quantum simulations can predict molecular interactions; financial modeling, which benefits from rapid calculations of complex scenarios; and complex system simulations that require immense computational power. These applications showcase the transformative potential of combining these two technologies.
- What challenges does quantum computing face in AI implementation?
Despite its potential, quantum computing faces significant hurdles in practical implementation. Technical limitations, such as error rates and qubit coherence times, pose challenges for reliable computations. Additionally, there is a pressing need for skilled professionals who understand both AI and quantum computing, which is currently a rare combination.
- What ethical concerns arise from the integration of AI and quantum computing?
As AI and quantum computing evolve, ethical considerations become increasingly important. Issues like data privacy are critical, as quantum computers could potentially break current encryption methods, putting sensitive information at risk. Furthermore, the potential for bias in AI systems may be exacerbated by the integration of quantum computing, making it essential to address fairness in algorithm design.
- How does quantum computing impact data privacy?
Quantum computing poses significant risks to data privacy because it can render many existing encryption techniques ineffective. This means that sensitive data, such as personal information and financial records, could be exposed to unauthorized access. As a result, there is a growing need for new security measures that can withstand the power of quantum computing.
- What measures can be taken to ensure fairness in AI algorithms?
To ensure fairness in AI algorithms, it is crucial to implement rigorous testing and validation processes that identify and mitigate biases. This includes diversifying training data, employing fairness-aware algorithms, and continuously monitoring AI systems for biased outcomes. By addressing these issues proactively, we can work towards creating more equitable AI solutions.