Transforming E-commerce with Big Data Analytics
In today's fast-paced digital marketplace, the integration of big data analytics is nothing short of a game changer for e-commerce businesses. Imagine a world where every click, every scroll, and every purchase is meticulously analyzed to unlock profound insights about consumer behavior. This is the reality that big data brings to the table, and it's revolutionizing how businesses operate, engage with customers, and ultimately drive sales. By leveraging vast amounts of data, companies can not only enhance customer experiences but also optimize their operations in ways that were previously unimaginable.
At the heart of this transformation is the ability to gather and process data from multiple sources—social media interactions, website traffic, customer reviews, and purchase histories. This data is then analyzed to uncover patterns and trends that inform strategic decisions. For instance, companies can identify which products are trending, understand seasonal buying behaviors, and even predict future sales. The power of big data lies in its ability to turn raw information into actionable insights, allowing e-commerce businesses to stay one step ahead of the competition.
Moreover, big data analytics facilitates a deeper understanding of customer preferences and behaviors. This insight is crucial for creating personalized shopping experiences that resonate with individual customers. Think about it: when you receive tailored recommendations that align with your interests, it feels less like marketing and more like a conversation with a friend who truly understands your needs. This level of personalization not only boosts customer engagement but also significantly enhances conversion rates, driving sales and fostering brand loyalty.
As we delve deeper into the various aspects of big data analytics in e-commerce, we'll explore how businesses can harness these insights to improve customer segmentation, enhance supply chain efficiency, and even prevent fraud. The potential is limitless, and the companies that embrace this data-driven approach are poised to lead the industry into the future.
Big data plays a crucial role in e-commerce by providing insights into customer behavior, preferences, and trends, enabling businesses to make informed decisions that enhance their strategies and offerings.
Data analytics allows e-commerce businesses to personalize shopping experiences, tailoring recommendations and marketing messages to individual customers, which significantly boosts engagement and conversion rates.
Effective customer segmentation techniques leverage big data to categorize consumers based on their behaviors and preferences, allowing for targeted marketing strategies that resonate with specific audience segments.
Behavioral segmentation focuses on analyzing customer interactions and purchasing patterns, enabling businesses to tailor their offerings and marketing efforts based on observed behaviors and preferences.
Demographic segmentation utilizes data such as age, gender, and income to create targeted marketing campaigns that appeal to specific groups, enhancing relevance and increasing the likelihood of conversions.
Predictive analytics uses historical data to forecast future trends and customer behaviors, helping e-commerce businesses make proactive decisions regarding inventory management, marketing strategies, and customer engagement.
Big data analytics optimizes supply chain operations by providing real-time insights into inventory levels, demand forecasting, and supplier performance, leading to improved efficiency and reduced costs.
Advanced analytics tools help businesses manage inventory levels more effectively, reducing excess stock and minimizing stockouts, ultimately resulting in better cash flow and customer satisfaction.
Analyzing supplier performance data enables e-commerce companies to identify reliable suppliers, negotiate better terms, and ensure timely deliveries, enhancing overall supply chain efficiency.
Big data analytics enhances fraud detection capabilities in e-commerce by identifying unusual patterns and anomalies in transactions, allowing businesses to take preventive measures and protect their assets.
Real-time monitoring of transactions enables businesses to quickly identify and respond to potential fraudulent activities, minimizing losses and maintaining customer trust.
Machine learning algorithms analyze vast amounts of transaction data to detect fraud patterns, continuously improving their accuracy over time and reducing false positives in fraud detection efforts.
- What is big data analytics? Big data analytics refers to the process of examining large and varied data sets to uncover hidden patterns, correlations, and other insights.
- How does big data impact e-commerce? It allows e-commerce businesses to understand customer behavior, optimize operations, and enhance personalization, leading to increased sales and customer satisfaction.
- What are some examples of big data in e-commerce? Examples include personalized product recommendations, targeted marketing campaigns, and real-time inventory management.
- Can small businesses benefit from big data analytics? Absolutely! Small businesses can leverage big data analytics to make informed decisions, optimize marketing strategies, and improve customer engagement.
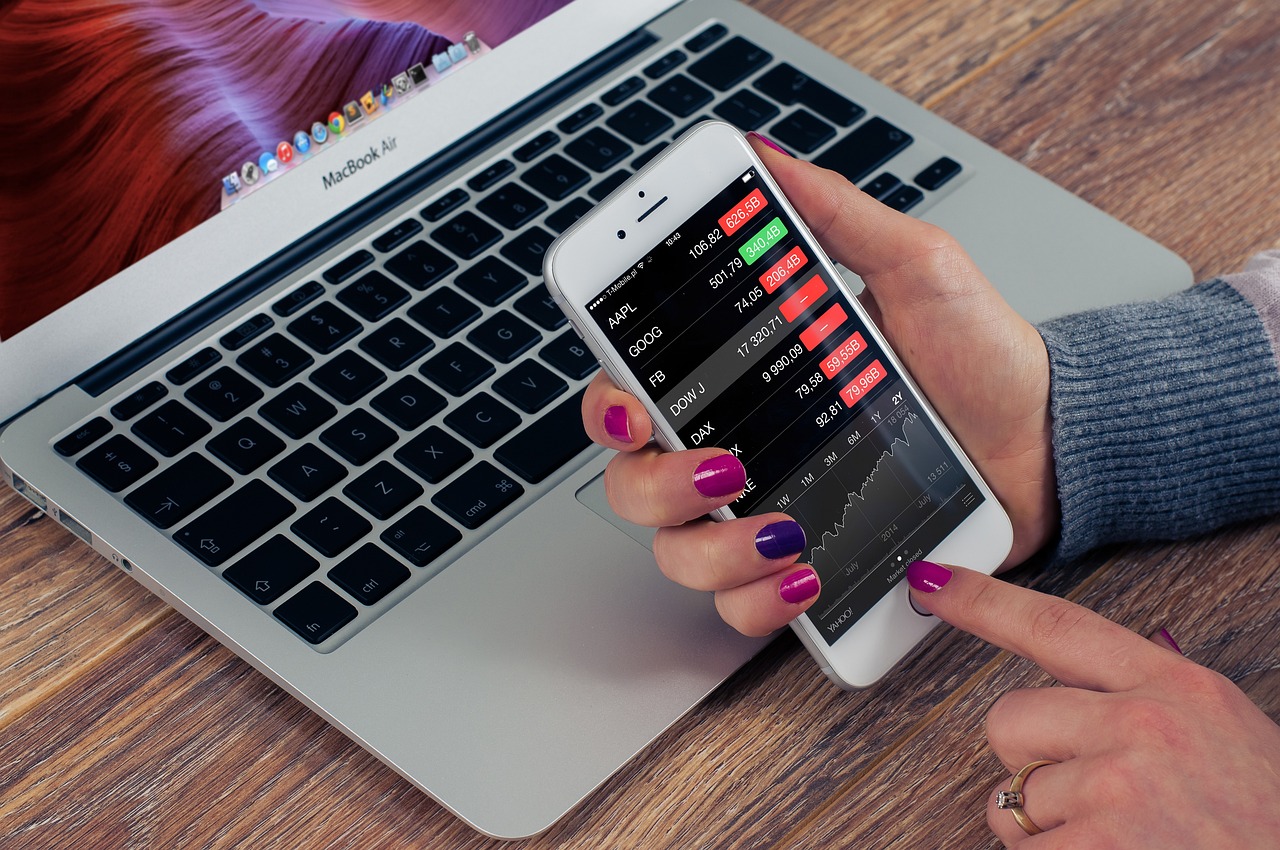
The Role of Big Data in E-commerce
Big data is not just a buzzword; it’s the lifeblood of modern e-commerce. Imagine walking into a store where every product is tailored to your tastes, every recommendation feels like it was handpicked just for you. That’s the power of big data analytics in the e-commerce landscape. By harnessing vast amounts of data, businesses can gain profound insights into customer behavior, preferences, and emerging market trends. This allows them to make informed decisions that not only enhance their strategies but also refine their offerings.
Consider this: every click, every purchase, and every abandoned cart generates data. This data, when analyzed, reveals patterns that can help businesses understand what customers want and need. For instance, if a customer frequently browses athletic wear but rarely purchases it, this information can prompt targeted marketing campaigns or personalized recommendations. By tapping into these insights, e-commerce companies can create a shopping experience that feels intuitive and engaging.
Moreover, big data drives operational efficiency. It helps businesses optimize their inventory by predicting which products will be in demand based on historical data and current trends. For example, if data shows a spike in demand for eco-friendly products, a company can adjust its stock levels accordingly. This not only improves customer satisfaction by ensuring that popular items are available but also minimizes excess inventory, thus reducing costs.
In addition, big data analytics allows e-commerce businesses to monitor customer feedback in real-time. By analyzing reviews and social media mentions, companies can quickly identify areas for improvement and respond to customer concerns. This proactive approach not only enhances the customer experience but also builds brand loyalty.
To sum it up, the role of big data in e-commerce is multifaceted. It empowers businesses to:
- Understand customer preferences and behaviors
- Optimize inventory management
- Enhance marketing strategies through targeted campaigns
- Monitor customer feedback and improve service

Personalization through Data Analytics
In today's fast-paced digital world, personalization has become the name of the game in e-commerce. Imagine walking into a store where the sales associate knows your name, remembers your past purchases, and can recommend products tailored just for you. That’s the kind of experience data analytics can provide online. By harnessing the power of big data, e-commerce businesses can create highly personalized shopping experiences that resonate with individual customers, leading to increased engagement and conversion rates.
Data analytics allows businesses to sift through mountains of customer data to uncover valuable insights. This means understanding what products customers are interested in, their shopping habits, and even their preferred communication channels. With this information, companies can tailor their recommendations and marketing messages to meet specific needs. For instance, if a customer frequently buys athletic gear, they might receive personalized emails featuring the latest running shoes or exclusive discounts on fitness apparel. This level of customization not only enhances the shopping experience but also builds customer loyalty.
To achieve effective personalization, e-commerce businesses often employ various customer segmentation techniques. These techniques categorize consumers based on their behaviors, preferences, and demographics, allowing for targeted marketing strategies that resonate with specific audience segments. Here are a couple of key methods:
- Behavioral Segmentation: This approach analyzes customer interactions and purchasing patterns. By observing how customers engage with the website—such as what products they view, how long they stay on certain pages, and their purchase history—businesses can tailor their offerings and marketing efforts. For example, if a customer frequently browses kitchen appliances, they might receive targeted ads for the latest gadgets, leading to higher chances of conversion.
- Demographic Segmentation: Utilizing data such as age, gender, and income, this technique helps create marketing campaigns that appeal to specific groups. For instance, a luxury brand might target high-income individuals with exclusive offers, while a budget-friendly retailer may focus on promotional deals for younger audiences. This enhances relevance and increases the likelihood of conversions.
Moreover, the integration of predictive analytics takes personalization to another level. By analyzing historical data, businesses can forecast future trends and customer behaviors. This means anticipating what products will be in demand and adjusting marketing strategies accordingly. Imagine receiving a personalized recommendation for a winter coat in the fall because the algorithm predicts that you’re likely to be shopping for cold-weather gear based on your past purchases. This proactive approach not only enhances customer satisfaction but also drives sales.
In conclusion, personalization through data analytics is not just a trend; it's a necessity in the competitive e-commerce landscape. By leveraging big data to understand customer preferences and behaviors, businesses can create tailored experiences that engage customers on a deeper level. This ultimately leads to increased sales and a loyal customer base, proving that in the world of e-commerce, the more personalized the experience, the better the results.
- What is the importance of personalization in e-commerce?
Personalization enhances customer experience, increases engagement, and boosts conversion rates by tailoring recommendations and marketing messages to individual preferences. - How does data analytics improve customer segmentation?
Data analytics enables businesses to categorize customers based on their behaviors and preferences, allowing for targeted marketing strategies that resonate with specific audience segments. - What role does predictive analytics play in personalization?
Predictive analytics forecasts future trends and customer behaviors, allowing businesses to proactively tailor their marketing strategies and product offerings.
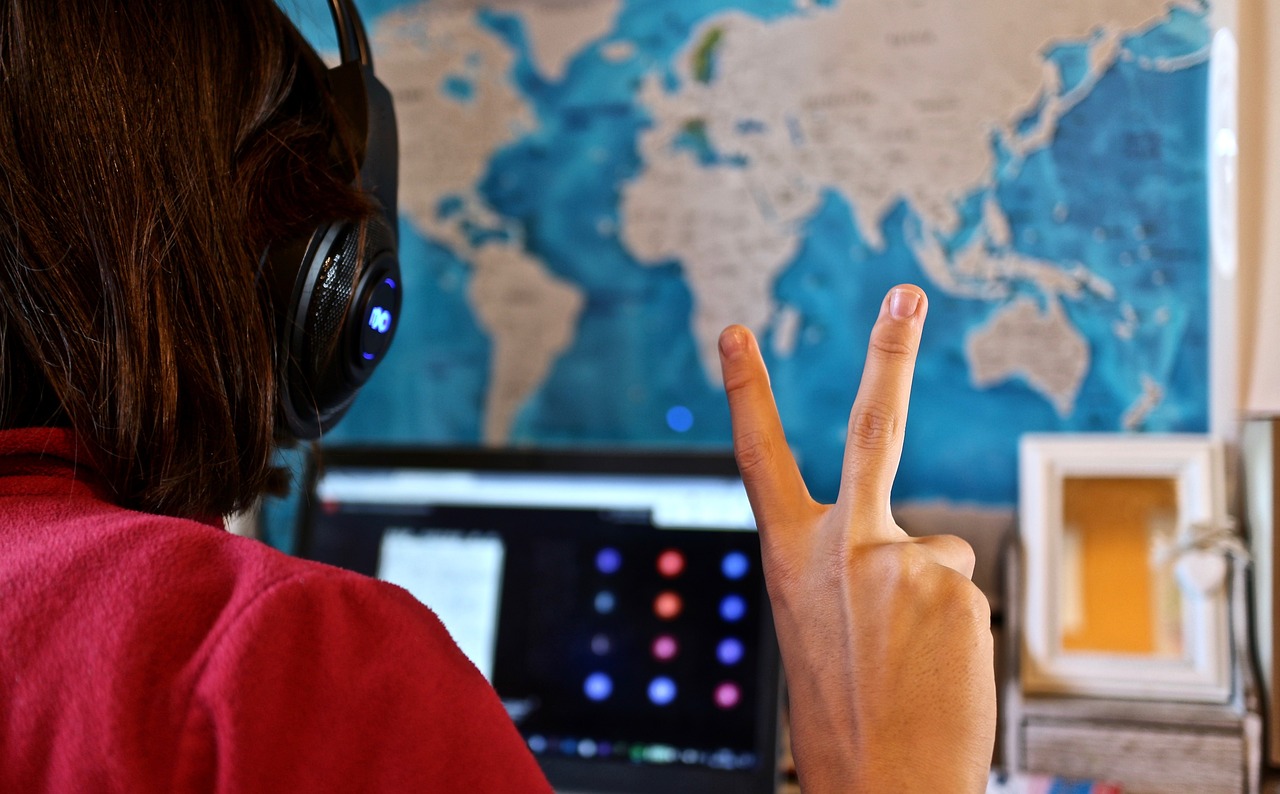
Customer Segmentation Techniques
In the ever-evolving landscape of e-commerce, understanding your customers is akin to holding a treasure map. The right can unlock valuable insights, allowing businesses to cater to specific needs and preferences. By leveraging big data analytics, companies can categorize consumers into distinct groups based on various criteria, enabling them to craft targeted marketing strategies that resonate deeply with their audience.
One of the most effective methods of segmentation is behavioral segmentation. This technique goes beyond basic demographics, analyzing how customers interact with your brand. For instance, consider how a shopper navigates your website: Do they frequently abandon their shopping cart? Are they drawn to specific product categories? By understanding these behaviors, businesses can tailor their offerings. Imagine sending personalized emails to customers who frequently browse but don’t purchase, enticing them with special discounts or reminders about items they’ve shown interest in.
On the other hand, demographic segmentation utilizes fundamental data points such as age, gender, and income. This method allows businesses to create marketing campaigns that are not only relevant but also appealing to specific groups. For example, a fashion retailer might target young adults with trendy styles while promoting classic pieces to an older demographic. This tailored approach enhances the relevance of marketing messages, increasing the likelihood of conversions.
Moreover, combining these techniques can yield even greater results. For instance, a business could employ both behavioral and demographic segmentation to create a comprehensive profile of their customers. By analyzing data from various sources, businesses can identify patterns and preferences, leading to a more nuanced understanding of their audience. This multifaceted approach allows for hyper-targeted marketing campaigns, ensuring that messages are not just sent, but are actually received and appreciated by the right people.
To illustrate, let’s consider a hypothetical e-commerce company that sells fitness gear. By using behavioral segmentation, they notice that a segment of their customers frequently purchases running shoes but rarely buys workout apparel. Through demographic analysis, they discover that these customers are primarily young professionals aged 25-35. By combining these insights, the company can create targeted marketing campaigns that promote complementary products, such as fitness apparel or accessories, specifically to this demographic. This strategy not only enhances customer experience but also drives increased sales.
In summary, employing effective customer segmentation techniques is essential for e-commerce businesses aiming to thrive in a competitive market. By utilizing behavioral and demographic data, companies can create personalized marketing strategies that resonate with their audience, ultimately leading to enhanced engagement and higher conversion rates.
- What is customer segmentation?
Customer segmentation is the process of dividing a customer base into distinct groups based on shared characteristics, behaviors, or preferences. This allows businesses to tailor their marketing efforts to meet the specific needs of each group.
- Why is customer segmentation important in e-commerce?
It helps e-commerce businesses understand their customers better, allowing for personalized marketing strategies that enhance customer engagement and increase conversion rates.
- What are the common techniques for customer segmentation?
Common techniques include behavioral segmentation, demographic segmentation, psychographic segmentation, and geographic segmentation.
- How can data analytics improve customer segmentation?
Data analytics provides insights into customer behaviors and preferences, enabling businesses to categorize their customers more accurately and develop targeted marketing strategies.

Behavioral Segmentation
Behavioral segmentation is a powerful tool that allows e-commerce businesses to dive deep into the psyche of their customers. By analyzing customer interactions and purchasing patterns, companies can tailor their offerings and marketing efforts to meet the specific needs and preferences of different consumer groups. Imagine walking into a store where every item is curated just for you based on your past shopping habits—this is precisely what behavioral segmentation aims to achieve in the digital realm.
At its core, behavioral segmentation categorizes customers based on their actions, such as purchasing frequency, brand loyalty, and product usage. This approach enables businesses to identify patterns and trends that might otherwise go unnoticed. For instance, a customer who frequently buys fitness gear may be targeted with exclusive offers on new workout products, while another who browses but rarely purchases might receive personalized discounts to encourage a sale.
Here are some key aspects of behavioral segmentation that highlight its significance:
- Purchase History: Understanding what customers have bought in the past allows businesses to predict what they might be interested in next. This data is invaluable for crafting targeted marketing campaigns.
- Engagement Level: By analyzing how often customers interact with the brand—whether through emails, social media, or website visits—companies can tailor their communication strategies to enhance customer engagement.
- Customer Journey Mapping: Tracking the steps customers take from awareness to purchase helps businesses identify pain points in the buying process, allowing them to optimize the customer experience.
Moreover, behavioral segmentation can lead to a deeper understanding of customer loyalty. For example, identifying loyal customers who frequently purchase can prompt businesses to reward them with loyalty programs or exclusive offers, fostering a sense of belonging and encouraging repeat purchases.
In essence, behavioral segmentation is not just about categorizing customers; it's about creating a personalized shopping experience that resonates with their unique preferences and behaviors. By leveraging this data-driven approach, e-commerce businesses can not only enhance their marketing strategies but also build lasting relationships with their customers, ultimately leading to increased sales and brand loyalty.
1. What is behavioral segmentation?
Behavioral segmentation is the process of dividing customers into groups based on their behaviors, such as purchasing habits, brand interactions, and product usage. This helps businesses tailor their marketing strategies to meet the specific needs of each group.
2. Why is behavioral segmentation important for e-commerce?
It allows e-commerce businesses to create personalized experiences for customers, which can lead to higher engagement, increased conversion rates, and improved customer loyalty.
3. How can businesses implement behavioral segmentation?
Businesses can implement behavioral segmentation by analyzing customer data from various sources, such as purchase history, website analytics, and social media interactions, to identify distinct customer behaviors and preferences.
4. Can behavioral segmentation change over time?
Yes, customer behaviors can evolve due to various factors such as market trends, personal circumstances, or changes in brand offerings. Therefore, businesses should continuously monitor and adjust their segmentation strategies.

Demographic Segmentation
Demographic segmentation is a powerful tool in the e-commerce arsenal, allowing businesses to tailor their marketing strategies based on specific characteristics of their target audience. By analyzing data such as age, gender, income, and education level, companies can create highly relevant campaigns that resonate with different consumer segments. Imagine walking into a store where every product is tailored just for you; that's the magic of demographic segmentation in the digital world!
For instance, a clothing retailer may analyze its customer base and discover that a significant portion of its audience consists of young adults aged 18-24. By tailoring their marketing messages to appeal to this demographic, the retailer can highlight trendy styles and affordable prices, ultimately driving higher engagement and sales. Similarly, a luxury brand targeting affluent customers can focus on exclusivity and premium quality in its campaigns, ensuring that the messaging aligns perfectly with the expectations of its audience.
Moreover, demographic segmentation allows for precise targeting, leading to better return on investment (ROI) for marketing efforts. Here’s how it typically works:
Demographic Factor | Marketing Strategy |
---|---|
Age | Targeted ads featuring age-appropriate products |
Gender | Personalized campaigns highlighting gender-specific items |
Income | Promotions for products that fit within the income bracket |
Location | Localized marketing campaigns based on regional preferences |
In addition to enhancing marketing effectiveness, demographic segmentation also improves customer satisfaction. When consumers receive messages and offers that are directly relevant to their lives, they feel valued and understood. This emotional connection can lead to increased brand loyalty, as customers are more likely to return to a brand that recognizes their unique needs. In a world where consumers are bombarded with generic advertisements, personalized approaches stand out like a beacon in the night.
However, it’s important to note that while demographic segmentation is beneficial, it should not be the only strategy employed. Combining demographic data with other segmentation methods, such as behavioral or psychographic segmentation, can lead to even more insightful marketing strategies. This holistic approach allows businesses to understand not just who their customers are, but also how they think and behave, creating a comprehensive picture that drives effective marketing decisions.
- What is demographic segmentation? Demographic segmentation is the process of dividing a target market into smaller groups based on demographic factors such as age, gender, income, and education level.
- Why is demographic segmentation important in e-commerce? It allows businesses to tailor their marketing strategies to specific audience segments, improving engagement, conversion rates, and customer satisfaction.
- How can I implement demographic segmentation? Start by collecting customer data through surveys, purchase history, and analytics tools. Then, analyze this data to identify key demographic characteristics and adjust your marketing strategies accordingly.
- Can demographic segmentation be combined with other types of segmentation? Absolutely! Combining demographic segmentation with behavioral and psychographic segmentation provides a more comprehensive understanding of your audience, leading to more effective marketing strategies.
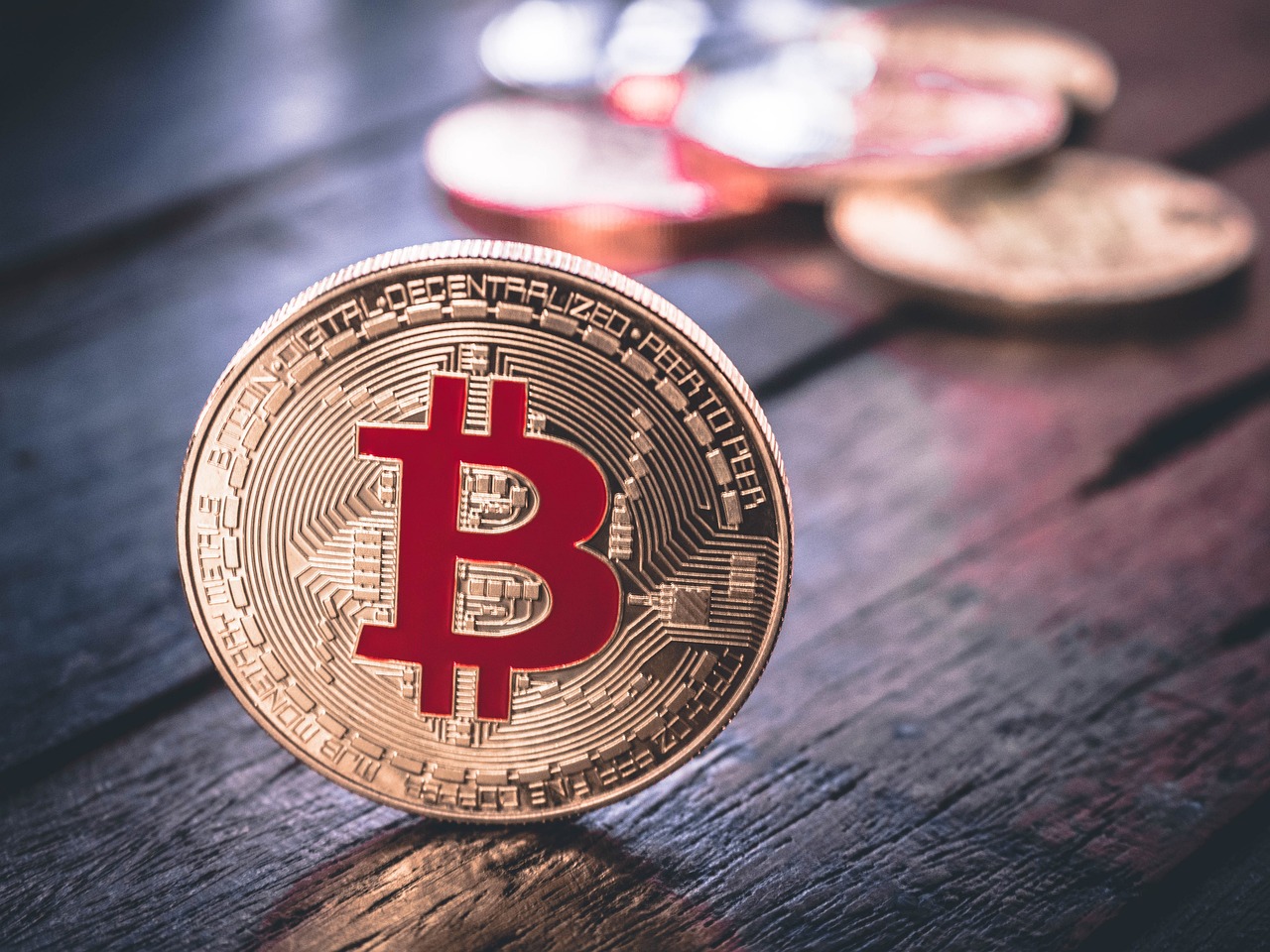
Predictive Analytics in E-commerce
In the fast-paced world of e-commerce, predictive analytics is like having a crystal ball that shows businesses what their customers are likely to do next. By analyzing historical data, e-commerce platforms can forecast future trends and customer behaviors, allowing them to stay one step ahead of the competition. Imagine being able to predict what your customers will want before they even search for it! This not only enhances the shopping experience but also drives sales and increases customer loyalty.
One of the key benefits of predictive analytics is its ability to inform inventory management. For instance, if a retailer notices a trend in summer apparel purchases during a particular month, they can adjust their inventory levels accordingly. This proactive approach minimizes the risk of overstocking or running out of popular items, which can be a nightmare for both businesses and customers. The result? Happier customers who find what they need and a healthier bottom line for the retailer.
Moreover, predictive analytics can significantly enhance marketing strategies. By understanding customer preferences and purchasing patterns, businesses can create targeted marketing campaigns that resonate with specific segments of their audience. For example, if data shows that a particular demographic is more likely to purchase eco-friendly products, a retailer can tailor their promotions to highlight sustainable options. This not only increases the chances of conversion but also fosters a deeper connection with the brand.
To illustrate the power of predictive analytics in e-commerce, consider the following table that showcases how businesses can leverage different types of data:
Data Type | Application | Benefit |
---|---|---|
Customer Purchase History | Forecast future purchases | Increased sales through targeted promotions |
Website Analytics | Identify popular products | Optimized inventory and marketing strategies |
Social Media Trends | Gauge customer sentiment | Improved product development and customer engagement |
In addition to improving marketing and inventory management, predictive analytics also aids in enhancing customer engagement. By anticipating customer needs, businesses can send personalized recommendations and timely reminders, making customers feel valued and understood. For instance, if a customer frequently buys athletic gear, sending them a notification about a new line of running shoes can lead to increased engagement and higher conversion rates.
As predictive analytics continues to evolve, its integration with machine learning is set to take e-commerce to new heights. Machine learning algorithms can analyze vast amounts of data at lightning speed, uncovering insights that would be impossible for humans to detect. This synergy allows businesses not only to predict trends but also to adapt their strategies in real-time, ensuring they remain relevant in a constantly changing market.
In conclusion, predictive analytics is a game-changer in the e-commerce landscape. By leveraging data effectively, businesses can enhance customer experiences, optimize their operations, and ultimately drive sales. It’s like having a roadmap that guides e-commerce companies toward success, helping them navigate the complexities of consumer behavior and market dynamics.
- What is predictive analytics? Predictive analytics involves using historical data to forecast future outcomes, helping businesses make informed decisions.
- How does predictive analytics benefit e-commerce? It helps in inventory management, marketing strategies, and enhancing customer engagement by anticipating customer needs.
- Can predictive analytics improve customer satisfaction? Yes, by providing personalized experiences and ensuring products are available when customers want them.
- Is machine learning part of predictive analytics? Absolutely! Machine learning enhances predictive analytics by analyzing large datasets quickly and accurately.
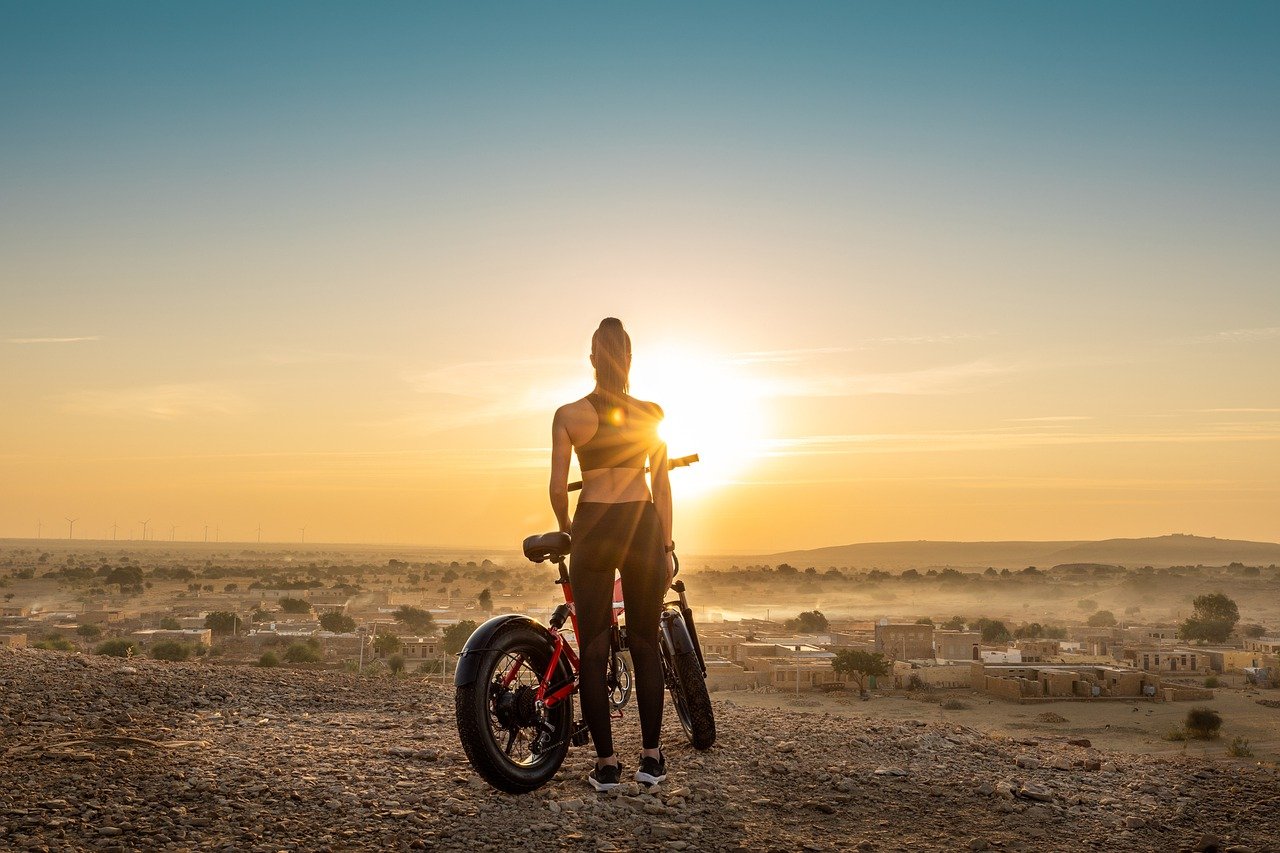
Enhancing Supply Chain Efficiency
This article explores how big data analytics is revolutionizing the e-commerce industry, enhancing customer experiences, optimizing operations, and driving sales through data-driven insights and strategies.
Big data plays a crucial role in e-commerce by providing insights into customer behavior, preferences, and trends, enabling businesses to make informed decisions that enhance their strategies and offerings.
Data analytics allows e-commerce businesses to personalize shopping experiences, tailoring recommendations and marketing messages to individual customers, which significantly boosts engagement and conversion rates.
Effective customer segmentation techniques leverage big data to categorize consumers based on their behaviors and preferences, allowing for targeted marketing strategies that resonate with specific audience segments.
Behavioral segmentation focuses on analyzing customer interactions and purchasing patterns, enabling businesses to tailor their offerings and marketing efforts based on observed behaviors and preferences.
Demographic segmentation utilizes data such as age, gender, and income to create targeted marketing campaigns that appeal to specific groups, enhancing relevance and increasing the likelihood of conversions.
Predictive analytics uses historical data to forecast future trends and customer behaviors, helping e-commerce businesses make proactive decisions regarding inventory management, marketing strategies, and customer engagement.
Big data analytics optimizes supply chain operations by providing real-time insights into inventory levels, demand forecasting, and supplier performance, leading to improved efficiency and reduced costs. Imagine trying to navigate a bustling city without a GPS; you'd likely get lost or take longer routes. This is how traditional supply chain management operates without big data—inefficient and often misguided. With big data, businesses can pinpoint exactly where they need to focus their resources, ensuring that every step in the supply chain is as smooth as possible.
One of the most significant benefits of big data in supply chain management is its ability to enhance inventory management. Advanced analytics tools allow businesses to monitor inventory levels in real-time, helping them to reduce excess stock while minimizing stockouts. This not only improves cash flow but also boosts customer satisfaction. For instance, if a popular product is running low, businesses can quickly adjust their orders to meet demand, ensuring that customers find what they want when they want it.
Moreover, big data analytics enables companies to evaluate supplier performance. By analyzing data related to delivery times, quality of goods, and pricing, businesses can identify reliable suppliers and negotiate better terms. This process is akin to shopping for the best deal; you wouldn't settle for the first option if you know there are better ones out there. In the same way, e-commerce companies can leverage data to make informed decisions about their suppliers, ultimately enhancing overall supply chain efficiency.
Aspect | Traditional Approach | Big Data Analytics |
---|---|---|
Inventory Management | Static monitoring | Real-time insights |
Supplier Evaluation | Ad-hoc assessments | Data-driven analysis |
Demand Forecasting | Historical trends | Predictive modeling |
In conclusion, big data analytics not only streamlines supply chain processes but also empowers businesses to make proactive decisions that can lead to significant cost savings and enhanced customer satisfaction. By embracing data-driven strategies, e-commerce companies can stay ahead of the competition, ensuring that they are always one step closer to meeting their customers' needs.
- What is big data analytics in e-commerce? Big data analytics involves analyzing vast amounts of data to gain insights into customer behavior, optimize operations, and improve decision-making in the e-commerce sector.
- How does big data improve supply chain efficiency? It provides real-time insights into inventory levels, demand forecasting, and supplier performance, leading to better management and reduced costs.
- Can big data help with fraud detection? Yes, big data analytics enhances fraud detection capabilities by identifying unusual patterns and anomalies in transactions.
- What role does predictive analytics play in e-commerce? Predictive analytics forecasts future trends and customer behaviors, helping businesses make proactive decisions regarding inventory and marketing strategies.

Inventory Management Solutions
In the fast-paced world of e-commerce, effective inventory management is not just a luxury; it's a necessity. With the sheer volume of products and the speed at which consumer preferences can change, businesses need to stay ahead of the curve. This is where advanced analytics tools come into play. By leveraging big data, e-commerce companies can gain real-time insights into their inventory levels, allowing them to make informed decisions that enhance their operational efficiency.
Imagine walking into a store where every shelf is perfectly stocked, and every product is exactly what the customer is looking for. This is the goal of effective inventory management—ensuring that the right products are available at the right time. By utilizing data analytics, businesses can monitor inventory trends, predict demand, and streamline their supply chain processes. For instance, if analytics reveal that certain products are flying off the shelves during specific seasons, businesses can adjust their stock levels accordingly, avoiding the pitfalls of overstocking or stockouts.
Furthermore, advanced analytics tools can provide insights into customer purchasing patterns. By analyzing historical sales data, e-commerce businesses can identify which products are frequently bought together, allowing them to create bundled offers or targeted promotions. This not only enhances the customer shopping experience but also boosts sales and improves inventory turnover rates.
To illustrate the impact of effective inventory management solutions, consider the following table:
Benefit | Description |
---|---|
Reduced Costs | Minimizing excess stock reduces holding costs and frees up cash flow. |
Improved Customer Satisfaction | Ensuring products are in stock means customers are more likely to find what they want. |
Enhanced Decision Making | Real-time data allows businesses to make proactive decisions about stock levels. |
In addition to these benefits, businesses can also utilize automated inventory management systems that integrate with their e-commerce platforms. These systems can automatically update stock levels, notify managers of low inventory, and even reorder products when they reach a certain threshold. This level of automation not only saves time but also minimizes the risk of human error, leading to a more streamlined operation.
Overall, embracing advanced inventory management solutions powered by big data analytics is a game-changer for e-commerce businesses. It allows them to operate more efficiently, respond to market demands swiftly, and ultimately, enhance customer satisfaction. In a world where consumer preferences can shift overnight, having the right tools to manage inventory effectively is essential for staying competitive.
- What is the importance of inventory management in e-commerce?
Inventory management is crucial as it helps businesses maintain optimal stock levels, reduces costs, and ensures customer satisfaction by having products available when needed. - How can big data analytics improve inventory management?
Big data analytics provides insights into customer behavior and purchasing trends, allowing businesses to forecast demand accurately and manage their inventory accordingly. - What are some common inventory management solutions?
Common solutions include automated inventory management systems, real-time tracking software, and demand forecasting tools.

Supplier Performance Analytics
In the fast-paced world of e-commerce, understanding and optimizing supplier performance is crucial for maintaining a competitive edge. involves the systematic evaluation of suppliers based on various metrics, helping businesses make informed decisions that directly impact their bottom line. By analyzing data related to delivery times, quality of goods, and pricing structures, e-commerce companies can identify which suppliers consistently meet or exceed expectations and which ones may be lagging behind.
One of the key benefits of utilizing supplier performance analytics is the ability to foster stronger relationships with reliable suppliers. When businesses can pinpoint their top-performing suppliers, they can negotiate better terms, such as discounts for bulk purchases or improved delivery schedules. This not only enhances operational efficiency but also contributes to a more collaborative partnership. For instance, if a supplier consistently delivers on time, a business might choose to prioritize orders with that supplier, knowing that they can trust them to meet customer demands.
Moreover, data visualization tools play a significant role in supplier performance analytics. By transforming raw data into easy-to-understand visual formats, businesses can quickly spot trends and anomalies. For example, a dashboard displaying key performance indicators (KPIs) such as on-time delivery rates, defect rates, and cost fluctuations can provide a comprehensive overview of supplier performance at a glance. This visual representation not only aids in decision-making but also helps in identifying potential risks before they escalate. Below is an example of how a simple supplier performance table might look:
Supplier Name | On-Time Delivery Rate | Quality Rating | Pricing Competitiveness |
---|---|---|---|
Supplier A | 95% | 4.8/5 | Competitive |
Supplier B | 85% | 4.2/5 | Above Average |
Supplier C | 90% | 4.5/5 | Competitive |
Additionally, incorporating feedback loops into the supplier performance analytics process can further enhance the relationship between e-commerce businesses and their suppliers. By regularly sharing performance data with suppliers, businesses can encourage improvements and foster a culture of accountability. This not only helps suppliers understand their performance metrics but also opens the door for discussions on how to enhance service levels and product quality.
In conclusion, leveraging supplier performance analytics is not just about crunching numbers; it's about creating a strategic advantage in a highly competitive landscape. By focusing on data-driven insights, e-commerce businesses can optimize their supply chains, reduce costs, and ultimately deliver a better experience to their customers. As the e-commerce industry continues to evolve, those who embrace the power of analytics will undoubtedly lead the charge toward greater efficiency and profitability.
- What is supplier performance analytics? Supplier performance analytics involves evaluating suppliers based on various metrics such as delivery times, quality of goods, and pricing to optimize supply chain efficiency.
- How can data visualization tools help in supplier performance analytics? Data visualization tools transform complex data into easy-to-understand visuals, allowing businesses to quickly identify trends and make informed decisions.
- Why is it important to have a feedback loop with suppliers? A feedback loop fosters communication and accountability, encouraging suppliers to improve their performance based on shared data.

Fraud Detection and Prevention
In the fast-paced world of e-commerce, have become critical components for businesses aiming to safeguard their assets and maintain customer trust. As online shopping continues to grow, so does the sophistication of fraudulent activities. This is where the power of big data analytics comes into play, offering a robust framework to identify and mitigate risks associated with fraud.
One of the most effective strategies in combating e-commerce fraud is through real-time transaction monitoring. By continuously analyzing transactions as they occur, businesses can quickly spot any unusual patterns or anomalies that may indicate fraudulent behavior. For instance, if a customer suddenly makes multiple high-value purchases from different locations within a short period, this could trigger an alert for further investigation. This proactive approach not only minimizes potential losses but also reinforces customer confidence in the security of their transactions.
Moreover, leveraging machine learning algorithms enhances fraud detection capabilities significantly. These algorithms are designed to analyze vast amounts of transaction data, detecting subtle patterns that might go unnoticed by human analysts. As they process more data, these systems continuously improve their accuracy, adapting to new fraud tactics and reducing the number of false positives. This is crucial because a high rate of false positives can lead to legitimate customers being flagged as potential fraudsters, damaging the customer experience.
To illustrate how these technologies work together, consider the following table showcasing the key features of real-time monitoring and machine learning algorithms:
Feature | Real-time Monitoring | Machine Learning Algorithms |
---|---|---|
Speed of Detection | Immediate | Continuous Learning |
Data Analysis | Transaction Patterns | Historical and Behavioral Data |
False Positives | Potentially High | Minimized Over Time |
Customer Impact | Quick Response | Improved Accuracy |
As e-commerce businesses adopt these advanced techniques, they not only protect themselves from fraud but also enhance the overall shopping experience for their customers. By ensuring that transactions are secure, companies can foster a sense of trust and reliability, which is essential in today's digital marketplace.
In conclusion, the integration of big data analytics into fraud detection and prevention strategies is no longer optional; it is a necessity. Businesses that embrace these technologies will not only safeguard their operations but also build stronger relationships with their customers. After all, in the world of e-commerce, trust is the currency that drives sales.
- What is big data analytics in e-commerce? Big data analytics involves analyzing large sets of data to uncover patterns, trends, and insights that can inform business strategies, enhance customer experiences, and improve operational efficiency.
- How does machine learning help in fraud detection? Machine learning algorithms analyze historical transaction data to identify patterns of fraudulent behavior, continuously improving their accuracy to reduce false positives and enhance detection capabilities.
- What are the benefits of real-time transaction monitoring? Real-time monitoring allows businesses to quickly identify and respond to suspicious activities, minimizing potential losses and maintaining customer trust in the security of their transactions.
- Can big data analytics improve customer experience? Yes, by personalizing shopping experiences and ensuring secure transactions, big data analytics can significantly enhance customer satisfaction and loyalty.
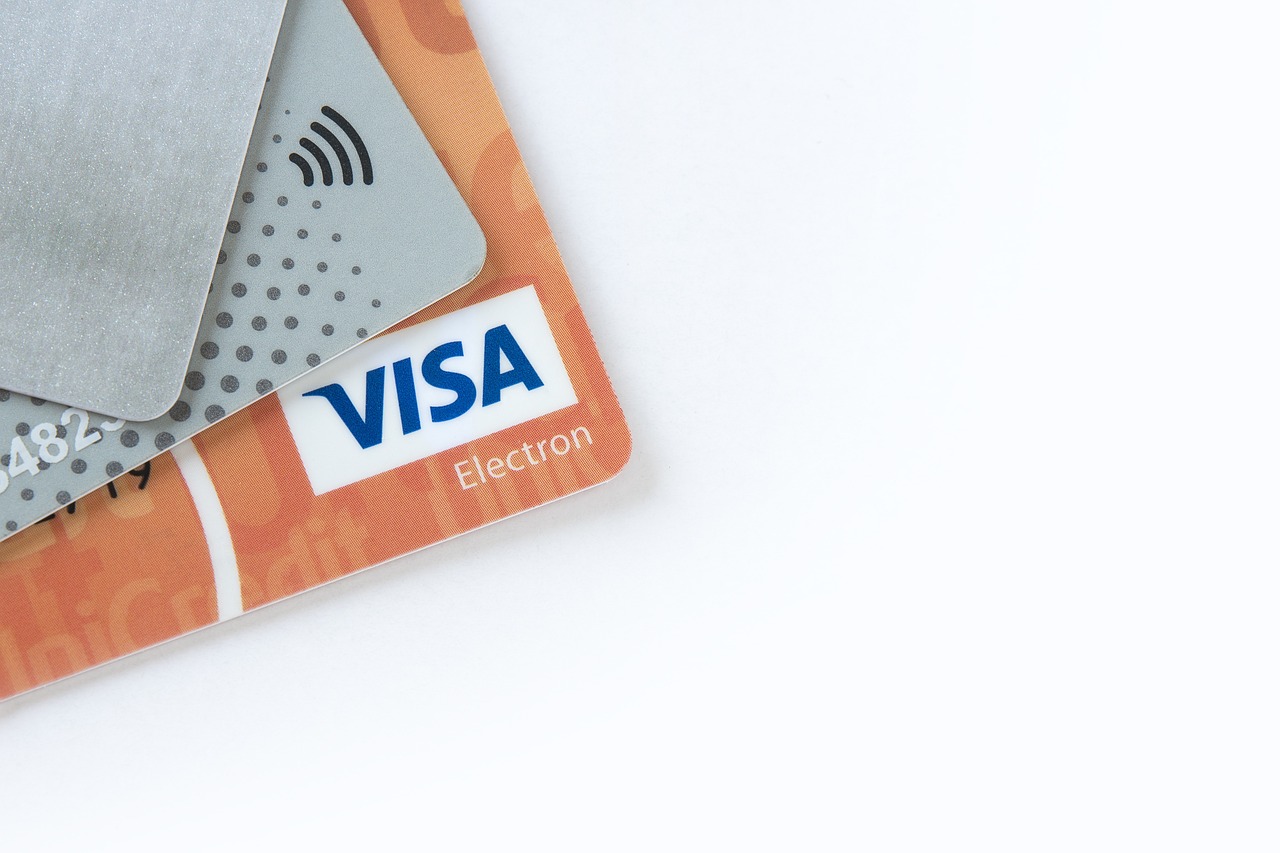
Real-time Transaction Monitoring
In the fast-paced world of e-commerce, has emerged as a game-changer for businesses aiming to safeguard their operations and customer trust. Imagine being able to spot a potential fraudster before they even complete a transaction! This is the power of real-time monitoring, where every transaction is scrutinized as it happens, allowing businesses to act swiftly and decisively. By integrating advanced analytics tools, e-commerce platforms can analyze transaction data in real-time, identifying unusual patterns or anomalies that could indicate fraudulent activity.
One of the key benefits of real-time transaction monitoring is its ability to enhance customer trust. When customers know that their transactions are being monitored for fraud, they feel more secure making purchases. This sense of security can lead to higher conversion rates, as customers are more likely to complete their purchases without the worry of fraud. Moreover, businesses can implement alerts that trigger immediate responses to suspicious activities, such as freezing accounts or requiring additional verification steps, which further protects both the customer and the business.
Moreover, the integration of machine learning algorithms with real-time monitoring systems allows for continuous improvement in fraud detection capabilities. These algorithms learn from historical data, adapting to new fraud patterns and minimizing false positives over time. For instance, if a customer typically makes purchases from a specific geographical area but suddenly tries to buy from a different country, the system can flag this transaction for further review. This proactive approach not only helps in preventing losses but also in maintaining a seamless shopping experience for genuine customers.
To illustrate how real-time transaction monitoring works, consider the following example:
Transaction ID | Customer Location | Transaction Amount | Status |
---|---|---|---|
TX123456 | New York, USA | $150.00 | Approved |
TX123457 | Tokyo, Japan | $300.00 | Flagged for Review |
TX123458 | Berlin, Germany | $75.00 | Approved |
In this table, you can see how transactions are monitored in real-time. The second transaction, which originated from an unexpected location for the customer, was flagged for review. This immediate action can prevent potential fraud before it escalates, ensuring that the business and its customers remain protected.
In summary, is not just a tool; it's a vital strategy in the e-commerce landscape. By leveraging advanced analytics and machine learning, businesses can enhance their fraud detection capabilities, protect their assets, and foster a secure shopping environment. As e-commerce continues to evolve, adopting such proactive measures will be essential for staying ahead of fraudsters and maintaining customer loyalty.
- What is real-time transaction monitoring?
Real-time transaction monitoring refers to the continuous analysis of transactions as they occur to identify suspicious activities and prevent fraud. - How does real-time monitoring enhance customer trust?
By actively monitoring transactions for fraud, businesses can reassure customers that their purchases are secure, leading to higher conversion rates. - Can real-time monitoring prevent all types of fraud?
While it significantly reduces the risk of fraud, no system is foolproof. Continuous improvement and adaptation are necessary to stay ahead of evolving fraud tactics. - How do machine learning algorithms contribute to fraud detection?
Machine learning algorithms analyze historical transaction data to identify patterns and improve the accuracy of fraud detection over time.

Machine Learning Algorithms
In the rapidly evolving world of e-commerce, have emerged as a game-changer, transforming how businesses detect and prevent fraud. These algorithms analyze vast amounts of transaction data in real-time, identifying patterns that may indicate fraudulent activity. Imagine having a vigilant security guard that never sleeps; that’s what machine learning does for e-commerce transactions. It continuously scans through data, learning from each interaction, and improving its ability to recognize suspicious behavior.
One of the key advantages of using machine learning in fraud detection is its ability to adapt over time. Traditional fraud detection methods often rely on static rules that can be easily bypassed by sophisticated fraudsters. In contrast, machine learning algorithms utilize dynamic models that evolve based on new data. This means they can identify emerging fraud patterns that might not have been previously recognized. For instance, if a new type of fraudulent transaction begins to surface, a well-trained machine learning model can quickly learn to flag such transactions for further investigation.
Moreover, machine learning algorithms significantly reduce the number of false positives—legitimate transactions that are incorrectly flagged as fraudulent. This is crucial for maintaining customer trust. Customers expect a seamless shopping experience, and frequent false alarms can lead to frustration and lost sales. By using advanced algorithms that analyze the context of each transaction, e-commerce businesses can minimize disruptions and provide a smoother checkout process.
To illustrate how machine learning algorithms function in fraud detection, consider the following table that outlines the typical workflow:
Step | Description |
---|---|
Data Collection | Gather transaction data from various sources, including past transactions, user behavior, and device information. |
Feature Engineering | Identify relevant features that may indicate fraud, such as transaction amount, frequency, and geographic location. |
Model Training | Use historical data to train the machine learning model, allowing it to learn the characteristics of both fraudulent and legitimate transactions. |
Real-time Analysis | Deploy the model to analyze transactions in real-time, flagging any that exhibit suspicious behavior for further review. |
Feedback Loop | Continuously update the model with new data and feedback from fraud investigations to improve its accuracy over time. |
In summary, machine learning algorithms are essential for modern e-commerce platforms aiming to combat fraud effectively. They not only enhance security but also ensure a better shopping experience for customers. As these algorithms continue to evolve, they will play an even more critical role in safeguarding online transactions and fostering customer confidence.
- What is machine learning? Machine learning is a subset of artificial intelligence that enables systems to learn from data and improve their performance over time without being explicitly programmed.
- How do machine learning algorithms detect fraud? They analyze transaction patterns and behaviors, learning from historical data to identify anomalies that may indicate fraudulent activities.
- Can machine learning reduce false positives? Yes, machine learning algorithms can significantly reduce false positives by considering the context of transactions and continuously improving their detection models.
- Is machine learning only used for fraud detection? No, machine learning has numerous applications in e-commerce, including personalized marketing, customer segmentation, and inventory management.
Frequently Asked Questions
- What is big data analytics in e-commerce?
Big data analytics in e-commerce refers to the process of collecting, analyzing, and interpreting vast amounts of data generated from consumer interactions and transactions. This data provides insights into customer behavior, preferences, and market trends, enabling businesses to make informed decisions that enhance their strategies and offerings.
- How does big data improve customer personalization?
Big data enhances customer personalization by allowing e-commerce businesses to tailor shopping experiences based on individual preferences and behaviors. By analyzing data, companies can provide personalized product recommendations, targeted marketing messages, and customized promotions that significantly boost customer engagement and conversion rates.
- What are customer segmentation techniques?
Customer segmentation techniques involve categorizing consumers into specific groups based on various criteria such as demographics, behaviors, and purchasing patterns. This targeted approach allows businesses to develop marketing strategies that resonate with particular audience segments, ultimately driving better results.
- What is predictive analytics and why is it important?
Predictive analytics uses historical data to forecast future trends and customer behaviors. It's crucial for e-commerce businesses as it helps them make proactive decisions regarding inventory management, marketing strategies, and customer engagement, ensuring they stay ahead of market demands.
- How does big data enhance supply chain efficiency?
Big data enhances supply chain efficiency by providing real-time insights into inventory levels, demand forecasting, and supplier performance. This leads to improved operations, reduced costs, and better overall performance, allowing businesses to meet customer demands more effectively.
- What role does big data play in fraud detection?
Big data plays a significant role in fraud detection by identifying unusual patterns and anomalies in transaction data. This allows businesses to quickly respond to potential fraudulent activities, minimizing losses and maintaining customer trust through effective preventive measures.
- How do machine learning algorithms help in fraud detection?
Machine learning algorithms analyze vast amounts of transaction data to detect fraud patterns. They continuously improve their accuracy over time, which helps reduce false positives and enhances the overall effectiveness of fraud detection efforts in e-commerce.