The Future of Artificial Intelligence in Disaster Response
As we stand on the brink of a technological revolution, the integration of artificial intelligence (AI) into disaster response is not just a possibility; it’s an impending reality that promises to reshape our approach to crisis management. Imagine a world where our responses to natural disasters are not only faster but also more accurate and efficient. This is the potential that AI brings to the table. From predicting catastrophic events to facilitating recovery efforts, AI is set to become a game-changer in how we prepare for and respond to disasters.
In recent years, we’ve witnessed the devastating impacts of natural disasters, whether it’s hurricanes sweeping through coastal cities or earthquakes rattling communities. The unpredictability of these events can leave us feeling helpless. However, with AI's ability to analyze vast amounts of data in real-time, we can enhance our preparedness and response strategies. Think of AI as a modern-day oracle, sifting through data to provide insights that can save lives and resources.
As we delve deeper into this transformative technology, it’s essential to recognize its multifaceted applications. AI is not just about crunching numbers; it’s about understanding patterns, predicting outcomes, and ultimately making informed decisions that can mitigate the impacts of disasters. By harnessing the power of AI, we can create a future where disaster response is proactive rather than reactive.
However, the journey towards fully integrating AI into disaster response is not without its challenges. Issues such as data privacy, technology access, and the need for skilled personnel must be addressed to maximize AI’s potential. Moreover, ethical considerations surrounding AI decision-making in life-threatening situations cannot be overlooked. As we navigate this complex landscape, establishing clear guidelines and frameworks will be crucial in ensuring that AI serves humanity’s best interests.
In conclusion, the future of artificial intelligence in disaster response is bright, filled with possibilities that can lead to safer, more resilient communities. As we continue to explore and innovate, it’s imperative that we remain vigilant and proactive in addressing the challenges that come with this powerful technology. The road ahead may be complex, but with collaboration and commitment, we can harness AI to create a safer world for all.
- What is the role of AI in disaster prediction?
AI plays a crucial role in analyzing large datasets to forecast disasters like hurricanes and earthquakes, thereby improving preparedness and response strategies. - How does AI enhance real-time data analysis during crises?
AI algorithms can process and analyze information from various sources, providing timely insights that inform decision-making in disaster situations. - What are the ethical considerations of using AI in disaster response?
Ethical concerns include the implications of AI decision-making in life-threatening scenarios and the need for guidelines to govern its use. - What challenges does AI face in disaster response?
Challenges include data privacy issues, access to technology, and the necessity for trained personnel to implement AI solutions effectively.
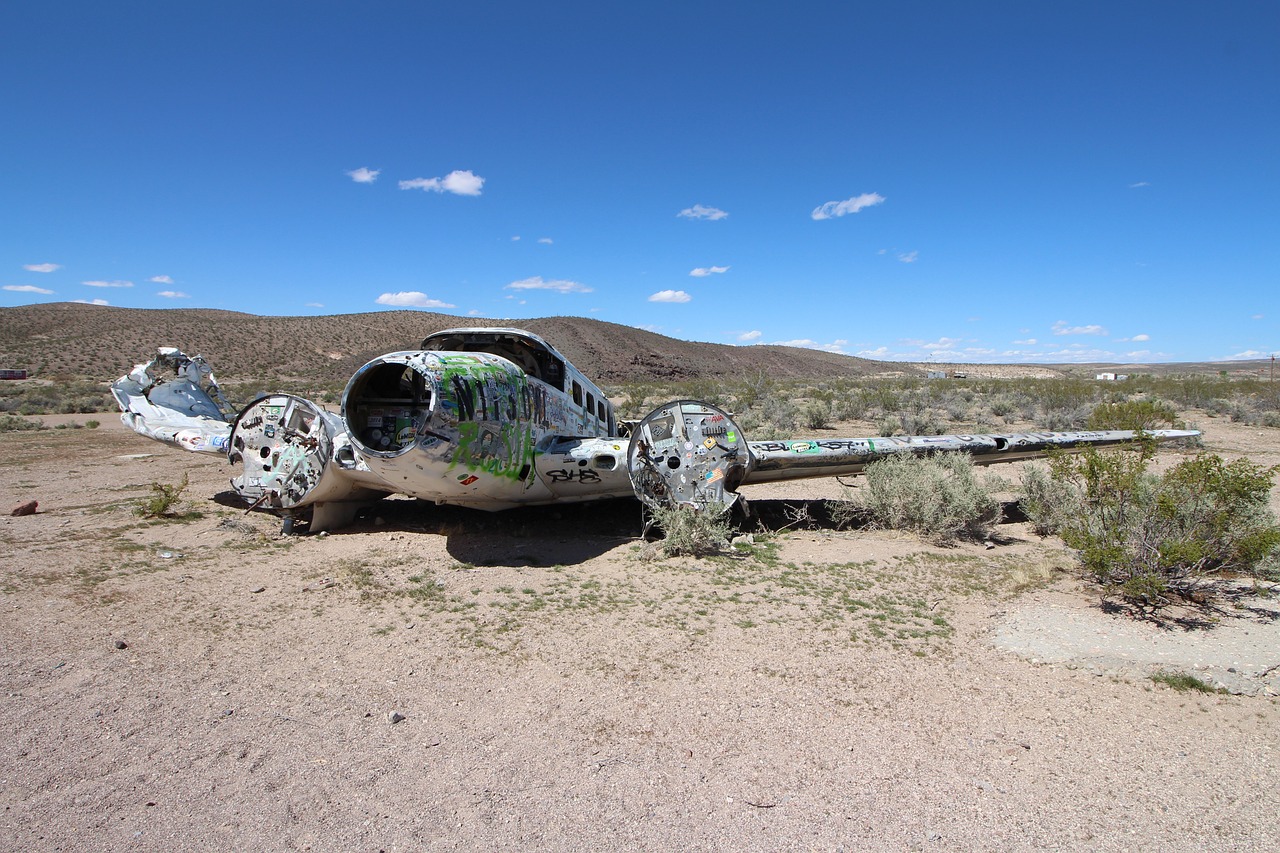
AI Applications in Disaster Prediction
Artificial Intelligence (AI) is not just a buzzword; it's a game-changer in how we approach disaster prediction. Imagine having the ability to foresee a hurricane days in advance or predict an earthquake's potential impact before it strikes. This is no longer a distant dream but a reality being shaped by AI technologies. By analyzing vast datasets—ranging from weather patterns to geological information—AI systems can identify trends and anomalies that human analysts might overlook. This leads to improved preparedness and response strategies that can save lives and minimize damage.
One of the most significant advantages of AI in disaster prediction is its capacity to process and analyze data at lightning speed. Traditional methods of prediction often rely on historical data and human intuition, which can be slow and prone to error. In contrast, AI algorithms can sift through terabytes of information in real-time, allowing for timely and informed decision-making. For instance, when a storm is brewing, AI can analyze satellite images, weather reports, and even social media posts to provide a comprehensive overview of the situation. This allows emergency services to act swiftly, mobilizing resources where they are needed most.
Furthermore, AI applications in disaster prediction are not limited to just natural disasters. They extend to various scenarios, including pandemics, industrial accidents, and even terrorist threats. For example, machine learning models can analyze patterns in health data to predict outbreaks of diseases, enabling healthcare systems to respond proactively. The versatility of AI in this domain is astounding, making it an invaluable tool for governments and organizations worldwide.
To illustrate the impact of AI in disaster prediction, consider the following table that outlines some key AI applications:
Application | Description | Impact |
---|---|---|
Hurricane Forecasting | Utilizes satellite data and weather models to predict storm paths. | Improved evacuation plans and resource allocation. |
Earthquake Prediction | Analyzes seismic data to identify patterns that precede quakes. | Enhanced building codes and preparedness measures. |
Flood Risk Assessment | Combines rainfall data and topographical information to assess flood risks. | Timely alerts and flood management strategies. |
Public Health Monitoring | Tracks disease outbreaks using health data and social media trends. | Proactive healthcare responses and resource allocation. |
In conclusion, the transformative role of AI in disaster prediction cannot be overstated. By harnessing the power of data and advanced algorithms, we can foresee potential disasters and take proactive measures to mitigate their impacts. As we continue to refine these technologies, the future looks promising for enhanced disaster preparedness and response.
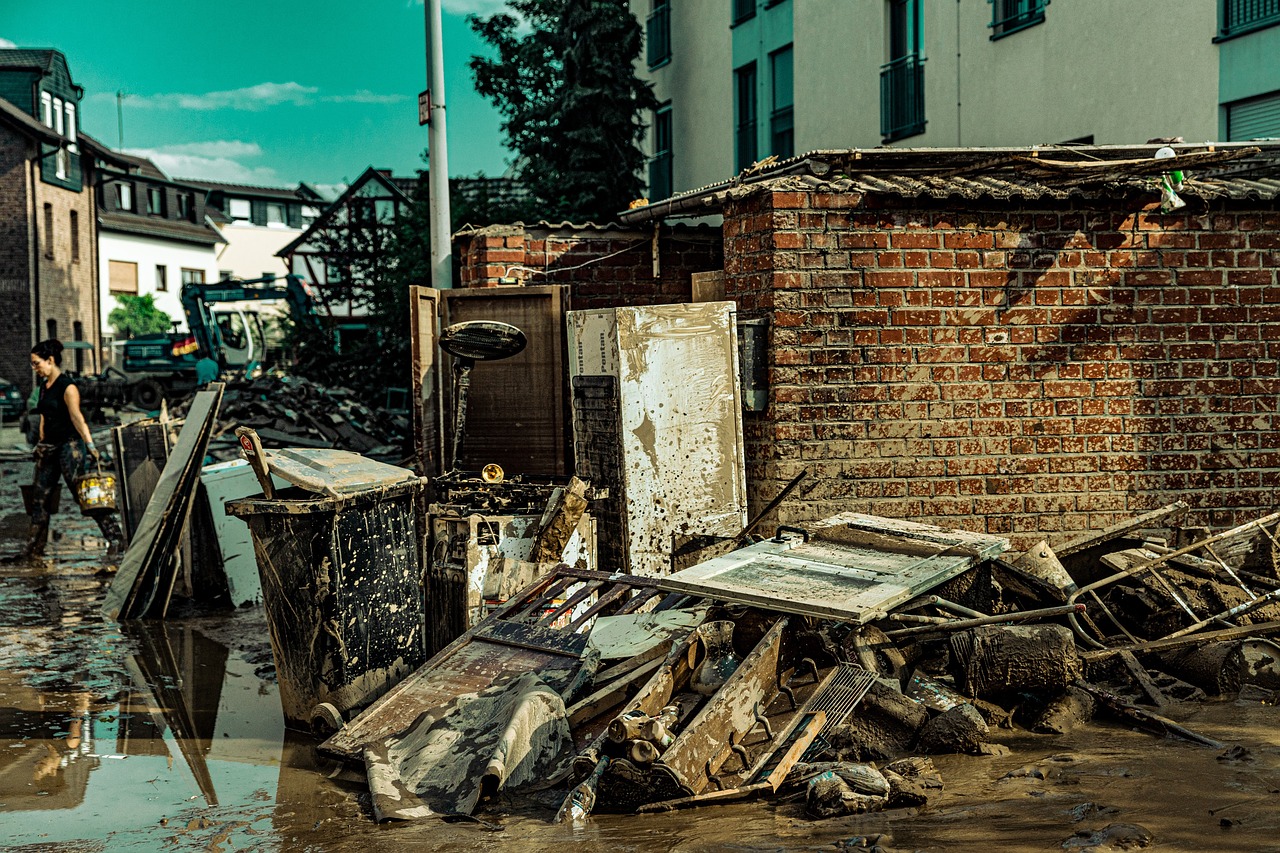
Real-Time Data Analysis
In today's fast-paced world, the ability to process information in real-time has become a game-changer, especially in the realm of disaster response. Imagine being able to predict the path of a hurricane or assess the damage from an earthquake almost instantly. This is where artificial intelligence (AI) steps in, acting as a powerful ally for emergency responders. By leveraging advanced algorithms and vast amounts of data, AI can analyze information from various sources—social media, satellite imagery, weather reports, and more—to provide timely insights that can save lives.
During a disaster, the clock is ticking, and every second counts. AI's real-time data analysis capabilities allow for rapid decision-making, which is crucial for effective response efforts. For example, algorithms can sift through social media posts to identify areas most affected by a disaster, helping authorities prioritize their resources. This capability not only enhances situational awareness but also enables responders to allocate aid more effectively. The result? A more coordinated and efficient response that can significantly reduce the impact of disasters on communities.
One of the most fascinating aspects of AI's role in real-time data analysis is its ability to integrate multiple data streams. By pulling together information from various sources, AI can create a comprehensive picture of the situation at hand. For instance, consider the following data sources:
- Weather Data: Real-time updates on storm patterns, temperatures, and precipitation levels.
- Geospatial Data: Satellite imagery that shows the extent of flooding or damage.
- Social Media Feeds: Public sentiment and reports from individuals on the ground.
- Emergency Services Reports: Updates from first responders on the ground.
By analyzing these diverse data sets, AI can identify trends and anomalies that might otherwise go unnoticed. For instance, if a sudden spike in social media posts about flooding is detected, AI can quickly correlate this information with weather data to determine if immediate action is needed. This kind of proactive approach is invaluable in disaster management.
Moreover, the use of AI in real-time data analysis is not just about speed; it's also about accuracy. Traditional methods of assessing disaster impact often rely on human interpretation, which can be slow and prone to errors. In contrast, AI algorithms can process large volumes of data with remarkable precision, ensuring that decision-makers have access to the most reliable information available. This accuracy is critical when lives are on the line and resources are limited.
As we look towards the future of disaster response, the integration of AI technologies will undoubtedly become more sophisticated. With advancements in machine learning and data analytics, we can expect even greater improvements in how real-time data is utilized during emergencies. The potential to harness AI for predictive analytics will further enhance our preparedness and response capabilities, allowing us to not only react to disasters but also anticipate and mitigate their impacts before they occur.
In summary, real-time data analysis powered by AI is revolutionizing disaster response efforts. By providing timely insights, enhancing situational awareness, and improving the accuracy of information, AI is helping to create a safer and more resilient world. As we continue to innovate and refine these technologies, the possibilities for improving disaster management are truly exciting.
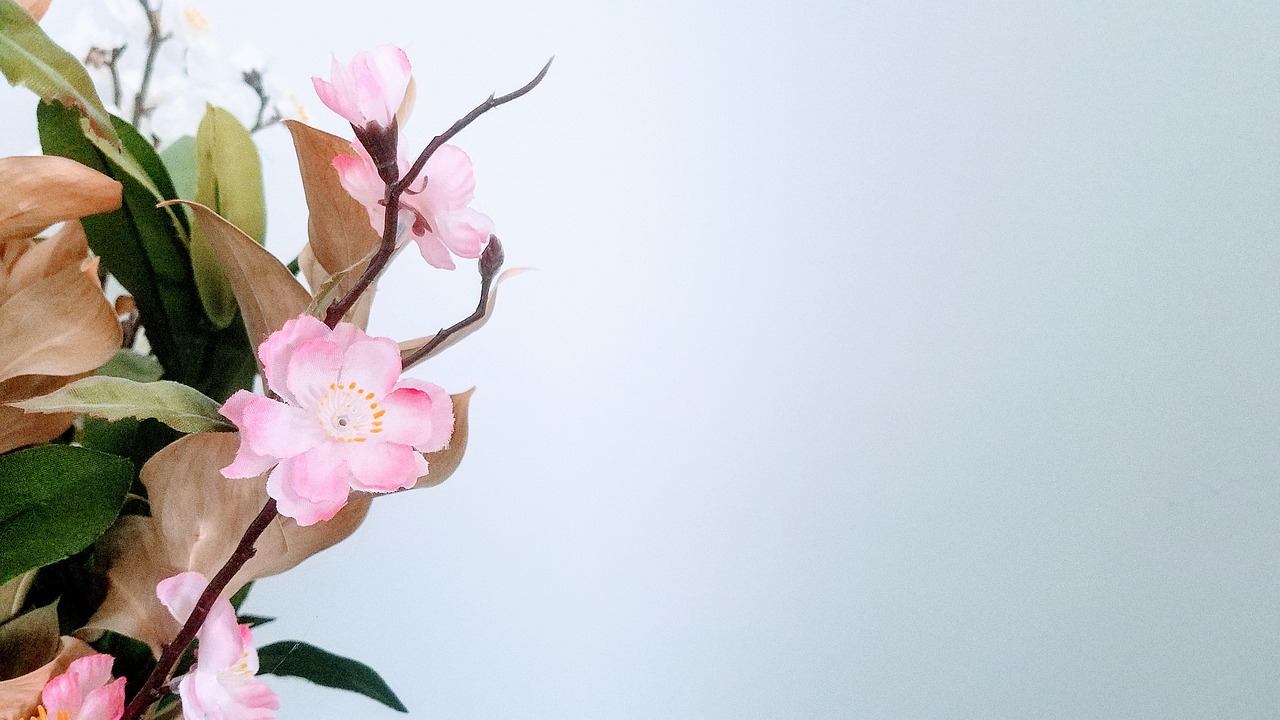
Machine Learning Techniques
Machine learning is at the forefront of revolutionizing disaster management, acting like a crystal ball that helps us foresee potential calamities. By leveraging vast amounts of data, machine learning algorithms can identify patterns and correlations that human analysts might overlook. Imagine sifting through mountains of information and extracting actionable insights in the blink of an eye—that's the power of AI in disaster response!
One of the most effective machine learning techniques used in this field is supervised learning. In supervised learning, algorithms are trained on labeled datasets, where the outcomes are already known. This technique can be particularly useful for predicting the likelihood of specific disasters based on historical data. For instance, if we have past records of hurricanes, a supervised learning model can analyze factors such as wind speed, humidity, and temperature to predict future occurrences.
Another exciting technique is unsupervised learning, which helps in discovering hidden patterns within unlabeled data. This approach is akin to exploring uncharted territory—data without predefined categories can reveal surprising insights. In disaster management, unsupervised learning can be used to cluster geographic areas based on vulnerability levels, helping authorities prioritize resources effectively.
Additionally, reinforcement learning is gaining traction in disaster response. This method involves training algorithms through trial and error, akin to teaching a dog new tricks. By simulating various disaster scenarios, reinforcement learning can optimize emergency response strategies, ensuring that resources are allocated in the most efficient manner. For example, it can help determine the best routes for evacuation or the optimal placement of emergency supplies.
To summarize the key machine learning techniques in disaster response, here's a quick table:
Technique | Description | Application in Disaster Management |
---|---|---|
Supervised Learning | Trains on labeled datasets to predict outcomes. | Forecasting disasters based on historical data. |
Unsupervised Learning | Discovers hidden patterns in unlabeled data. | Clustering areas by vulnerability levels. |
Reinforcement Learning | Optimizes strategies through trial and error. | Improving resource allocation during emergencies. |
These machine learning techniques are not just theoretical; they are being applied in real-world scenarios to save lives and enhance disaster response efforts. As we continue to refine these algorithms, the potential for more accurate predictions and faster responses grows exponentially. The future of disaster management is not just about reacting to crises but anticipating them, thanks to the transformative power of machine learning.
- What is machine learning?
Machine learning is a subset of artificial intelligence that focuses on the development of algorithms that allow computers to learn from and make predictions based on data. - How does machine learning help in disaster response?
Machine learning helps in predicting disasters, analyzing real-time data, and optimizing resource allocation during emergencies. - What are the main types of machine learning?
The main types include supervised learning, unsupervised learning, and reinforcement learning, each serving different purposes in data analysis and prediction. - Are there any ethical concerns with using AI in disaster management?
Yes, ethical considerations include data privacy, decision-making in life-threatening situations, and ensuring equitable access to technology.
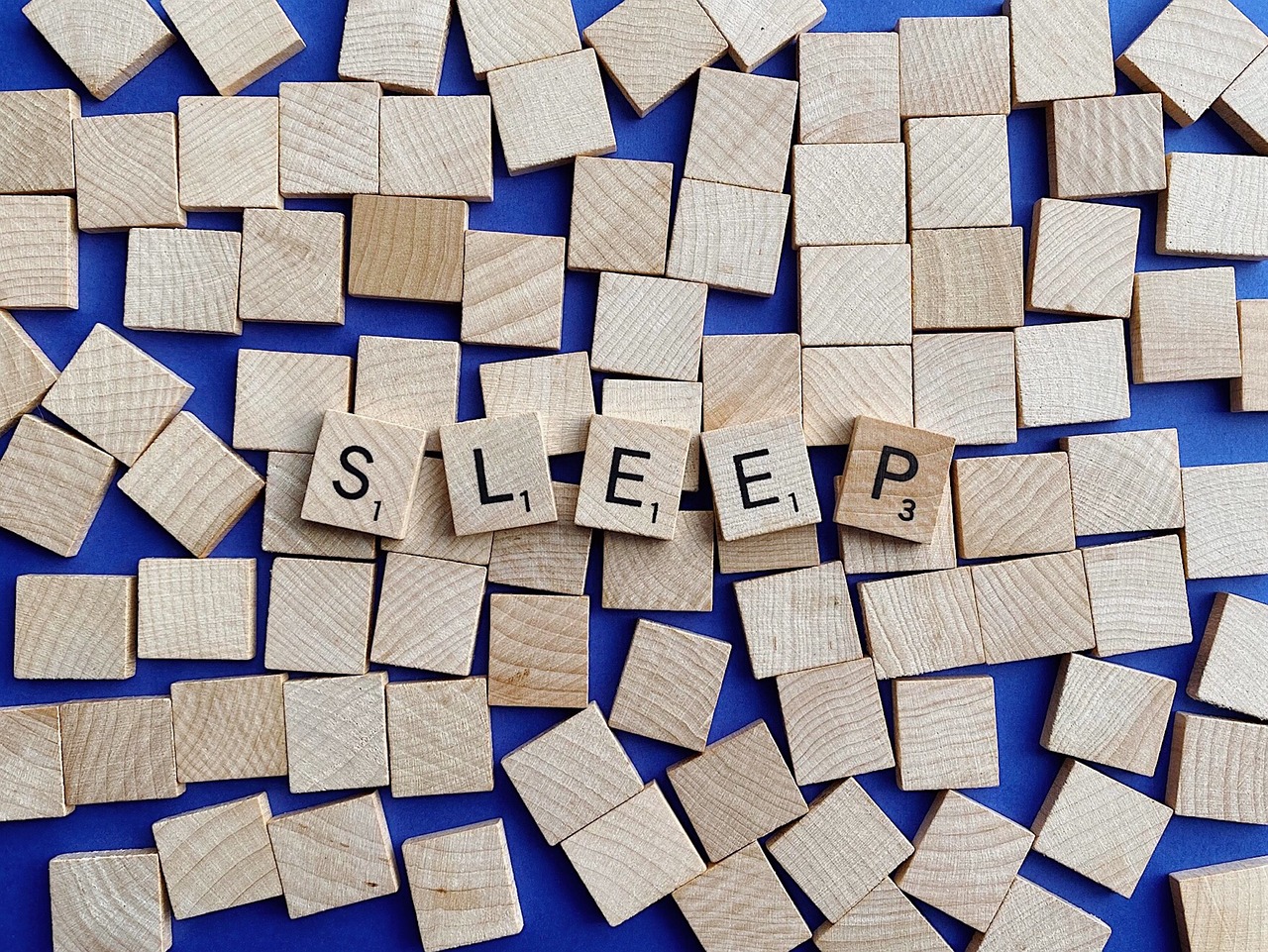
Predictive Modeling
Predictive modeling stands as a cornerstone in the realm of disaster management, serving as a powerful tool that allows emergency services to anticipate and prepare for potential calamities. Imagine being able to foresee a storm before it hits, or predicting the aftermath of an earthquake with a high degree of accuracy. This is precisely what predictive modeling enables through the use of sophisticated algorithms and vast data sets. By simulating various disaster scenarios, AI-driven models can provide invaluable insights that help authorities make informed decisions.
At its core, predictive modeling involves the analysis of historical data and the identification of patterns that can indicate future events. For instance, by examining past hurricane data, machine learning algorithms can predict the likelihood of future storms based on factors such as ocean temperatures, wind patterns, and atmospheric conditions. This proactive approach not only enhances preparedness but also significantly reduces the risks associated with disasters.
To illustrate the impact of predictive modeling, consider the following table that summarizes key components and benefits of this approach:
Component | Description | Benefit |
---|---|---|
Data Collection | Gathering historical and real-time data from various sources. | Provides a comprehensive view of potential disaster scenarios. |
Algorithm Development | Creating models that can analyze data patterns effectively. | Enhances accuracy in predicting disaster occurrences. |
Scenario Simulation | Running simulations to evaluate different disaster impacts. | Helps in planning and resource allocation during emergencies. |
Decision Support | Providing actionable insights to emergency responders. | Facilitates timely and effective response strategies. |
Moreover, predictive modeling is not just about anticipating disasters; it also plays a crucial role in understanding the potential impacts on communities and infrastructure. For example, by analyzing socio-economic data along with environmental factors, predictive models can estimate how many people might be affected and what resources will be needed for recovery. This holistic view allows for a more strategic approach to disaster response, ensuring that aid reaches those who need it most.
As we look to the future, the integration of predictive modeling with other AI technologies promises to enhance our disaster preparedness even further. The combination of real-time data analysis, machine learning, and predictive modeling creates a robust framework for anticipating and responding to disasters, ultimately saving lives and minimizing damage. In this ever-evolving landscape, it’s crucial for stakeholders to embrace these innovations and work together to build resilient communities.
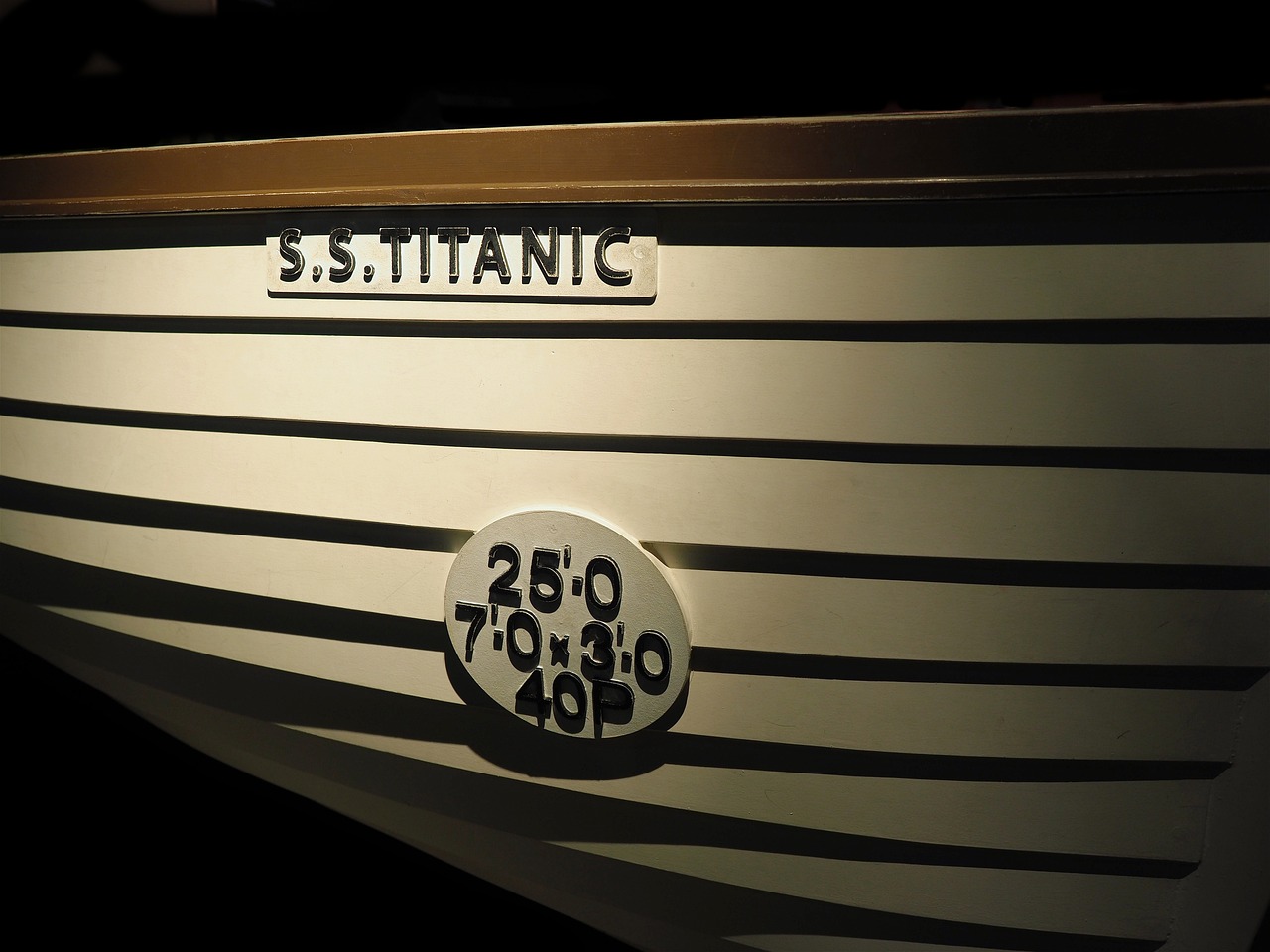
Sentiment Analysis
In the chaotic aftermath of a disaster, understanding how the public feels can be just as crucial as knowing the extent of the damage. This is where comes into play. By leveraging AI tools, authorities can sift through mountains of social media posts, news articles, and online comments to gauge public sentiment during a crisis. Imagine trying to read the room in a packed auditorium—it's overwhelming! But with sentiment analysis, AI acts like a skilled interpreter, translating the noise into clear insights.
During disasters, people often turn to platforms like Twitter and Facebook to express their fears, frustrations, and needs. AI algorithms can analyze this data to identify trends and emotions, providing emergency services with real-time feedback on how the community is coping. For instance, if a significant number of posts express anger about slow response times, this can prompt authorities to adjust their strategies on the fly. The ability to capture and interpret these sentiments not only aids in immediate response but also shapes future communication strategies.
Moreover, sentiment analysis can be categorized into various emotional tones, such as:
- Positive: Expressions of gratitude towards responders.
- Negative: Frustration regarding resource shortages.
- Neutral: Informational updates about the situation.
By analyzing these tones, authorities can tailor their messaging. For example, if the sentiment is predominantly negative, officials might prioritize transparency and reassurance in their communications. This proactive approach not only helps in managing public expectations but also fosters trust between communities and their leaders.
However, it's important to note that sentiment analysis isn't foolproof. Misinterpretations can occur, especially if sarcasm or cultural nuances are involved. Therefore, while AI provides a powerful tool for understanding public sentiment, human oversight remains essential to ensure that the insights drawn are accurate and actionable. In this way, sentiment analysis stands as a bridge, connecting the raw data of human emotion with the structured response efforts of disaster management.
- What is sentiment analysis? Sentiment analysis is the process of using AI to determine the emotional tone behind a series of words, often used to gauge public opinion during crises.
- How does sentiment analysis help in disaster response? It helps authorities understand public feelings and needs, allowing for more effective communication and response strategies.
- Are there limitations to sentiment analysis? Yes, sentiment analysis can misinterpret sarcasm and cultural nuances, so human oversight is necessary.
- Which platforms are analyzed for sentiment? Common platforms include social media sites like Twitter, Facebook, and news articles.
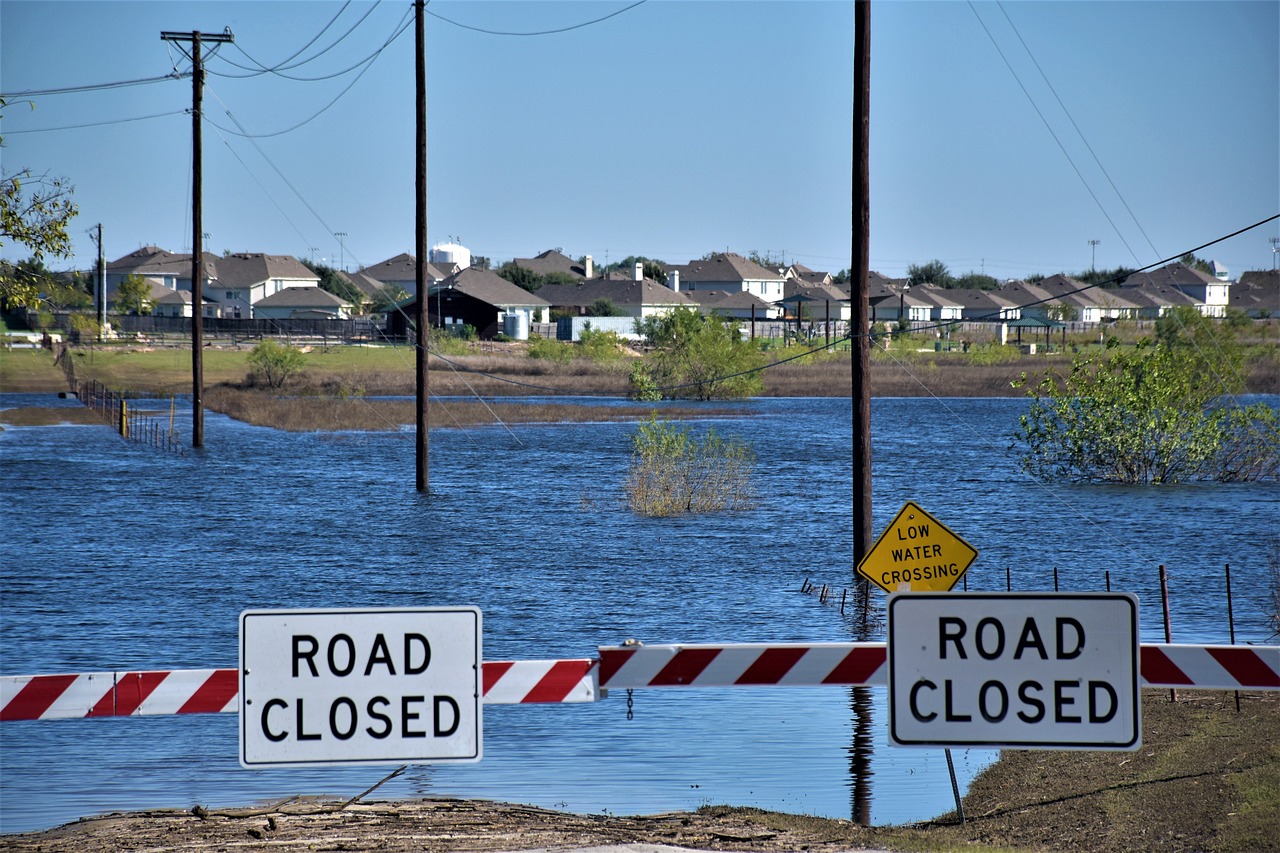
AI in Recovery Efforts
After a disaster strikes, the path to recovery can often feel like navigating a maze. Communities face the daunting task of rebuilding lives, infrastructure, and economies. This is where artificial intelligence (AI) steps in, acting as a guiding light through the chaos. By harnessing the power of AI, recovery efforts can be significantly enhanced, making the process not only faster but also more efficient. Imagine having a tool that can predict the most effective ways to allocate resources, assess damage with pinpoint accuracy, and even help communities rebuild in a smarter, more sustainable way. Sounds incredible, right?
One of the most crucial roles AI plays in recovery is in resource allocation. After a disaster, resources such as food, medical supplies, and shelter become vital. AI algorithms can analyze real-time data to determine where these resources are most needed. For instance, if a hurricane has devastated a coastal town, AI can quickly evaluate the extent of the damage and the immediate needs of the affected population. This ensures that aid is delivered promptly to those who need it most, rather than getting lost in bureaucratic red tape.
Additionally, AI is instrumental in damage assessment. Traditional methods of assessing damage can be slow and labor-intensive. However, with AI-powered drones and satellite imagery, it’s possible to gather and analyze data much more rapidly. These technologies can identify affected areas, estimate the extent of the damage, and provide valuable insights that help emergency services prioritize their efforts. For example, a recent study showed that using AI for damage assessment reduced the time taken to evaluate a disaster area by over 50% compared to conventional methods.
Moreover, AI doesn't just stop at immediate recovery; it also plays a pivotal role in rebuilding efforts. By utilizing predictive modeling, AI can simulate various rebuilding scenarios, helping planners make informed decisions. This not only includes physical infrastructure but also social aspects like community resilience. AI can analyze past recovery efforts to identify what worked and what didn't, ensuring that future rebuilding projects are designed to be more resilient against future disasters. Think of it as having a crystal ball that helps you learn from the past to build a better future.
To illustrate the impact of AI in recovery efforts, consider the following table that summarizes its applications:
Application | Description | Benefits |
---|---|---|
Resource Allocation | AI analyzes data to determine where resources are needed most. | Faster aid delivery, reduced waste of resources. |
Damage Assessment | Utilizes drones and satellite imagery for rapid damage evaluation. | Quick identification of affected areas, prioritization of response. |
Rebuilding Planning | Predictive modeling simulates rebuilding scenarios. | Informed decision-making, enhanced community resilience. |
In conclusion, the integration of AI into recovery efforts is transforming how communities respond to disasters. By leveraging advanced technologies, we can ensure that recovery is not just a process but a pathway to resilience. The future holds exciting possibilities, and as we continue to innovate, we must embrace these changes to create safer, more prepared communities.
- How does AI improve disaster recovery?
AI enhances disaster recovery by optimizing resource allocation, speeding up damage assessments, and aiding in strategic rebuilding efforts. - What technologies are used in AI for disaster response?
Technologies such as machine learning, predictive modeling, and satellite imagery are commonly used in AI applications for disaster response. - Are there any risks associated with using AI in disaster recovery?
Yes, risks include data privacy concerns, reliance on technology, and the potential for algorithmic bias, which must be managed carefully. - How can communities prepare for future disasters using AI?
Communities can invest in AI technologies, collaborate with tech companies, and implement training programs to enhance their disaster preparedness.
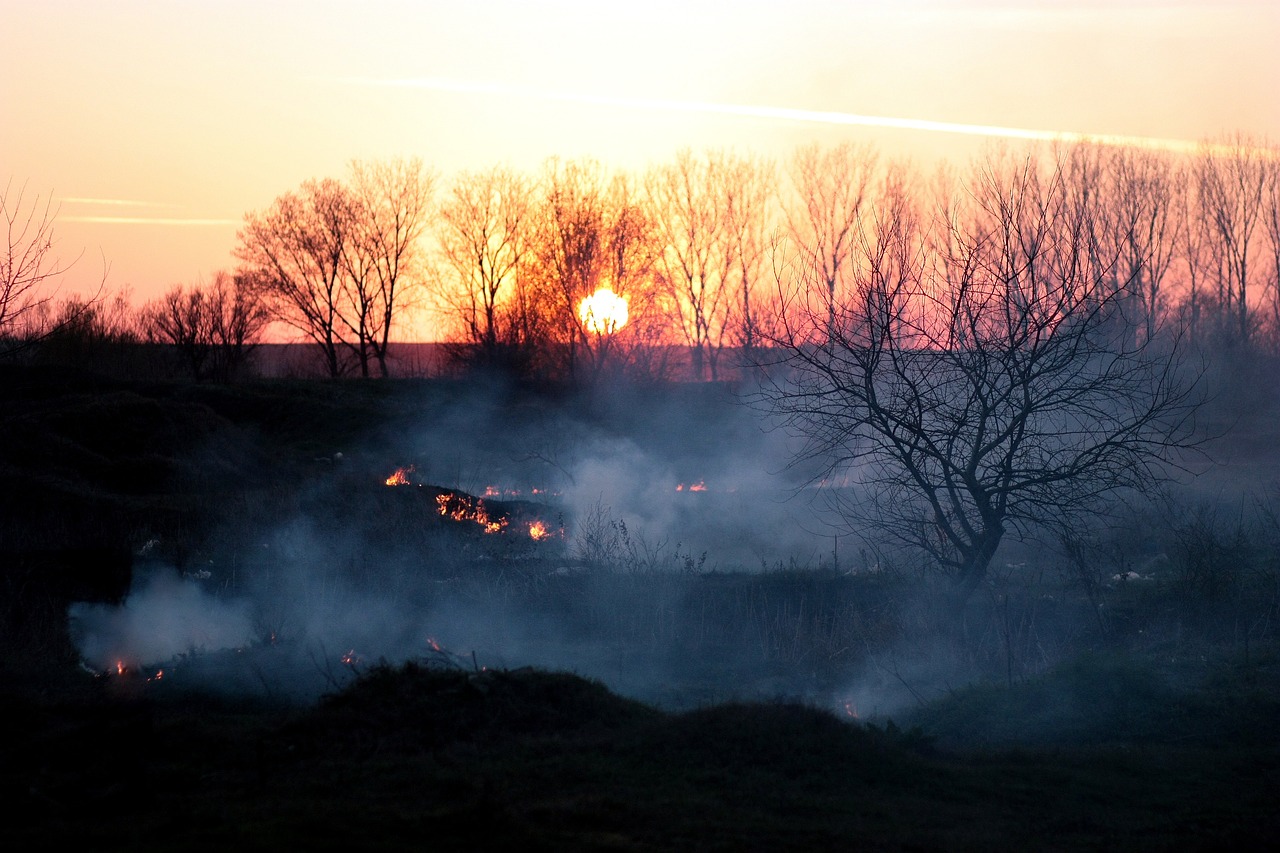
Challenges in Implementing AI
Despite the promising potential of artificial intelligence (AI) in disaster response, several significant challenges hinder its full implementation. One of the primary obstacles is data privacy. In a world increasingly concerned about personal information security, the collection and analysis of vast amounts of data can raise serious ethical issues. For instance, during natural disasters, individuals may share sensitive information on social media or through emergency services, which could be misused if not handled correctly. This concern necessitates robust data protection measures to ensure that individuals' rights are respected while still allowing for effective AI-driven disaster management.
Another challenge is technology access. In many regions, particularly in developing countries, the infrastructure required to support advanced AI technologies may not be available. This lack of access can create a digital divide, where only certain communities benefit from AI advancements while others are left vulnerable. Additionally, the implementation of AI systems often requires substantial financial investment, which may not be feasible for all disaster response organizations. As a result, many areas may struggle to adopt these innovative solutions, even when they are available.
Moreover, the need for skilled personnel cannot be overstated. AI technologies are complex and require a workforce that is not only familiar with the technology but also possesses the ability to interpret the data generated effectively. Unfortunately, there is currently a shortage of professionals trained in AI and data analysis, particularly in the context of disaster response. This skills gap can lead to underutilization of AI tools, preventing organizations from maximizing their potential benefits.
In addition to these challenges, the integration of AI with existing disaster response systems poses a significant hurdle. Traditional methods have been in place for years, and transitioning to AI-driven processes can be met with resistance from personnel accustomed to conventional practices. Ensuring seamless collaboration between AI technologies and existing infrastructures requires careful planning and training. Organizations must focus on creating a culture of innovation that embraces change, allowing for a smoother integration process.
To summarize, while AI holds immense promise for enhancing disaster response efforts, it is essential to address these challenges head-on. Organizations must prioritize data privacy, bridge the technology access gap, invest in training skilled personnel, and facilitate the integration of AI with existing systems. By doing so, they can unlock the full potential of AI in saving lives and improving the resilience of communities during disasters.
- What are the main challenges of implementing AI in disaster response?
The main challenges include data privacy concerns, technology access issues, a shortage of skilled personnel, and integration difficulties with existing systems. - How can organizations address data privacy issues?
Organizations can implement robust data protection measures and establish clear ethical guidelines to ensure sensitive information is handled appropriately. - Why is technology access important for AI in disaster response?
Technology access is crucial because it ensures all communities, particularly those in developing regions, can benefit from AI advancements and are not left vulnerable during disasters. - What role does skilled personnel play in AI implementation?
Skilled personnel are essential for interpreting AI-generated data and ensuring that AI tools are utilized effectively in disaster response efforts.
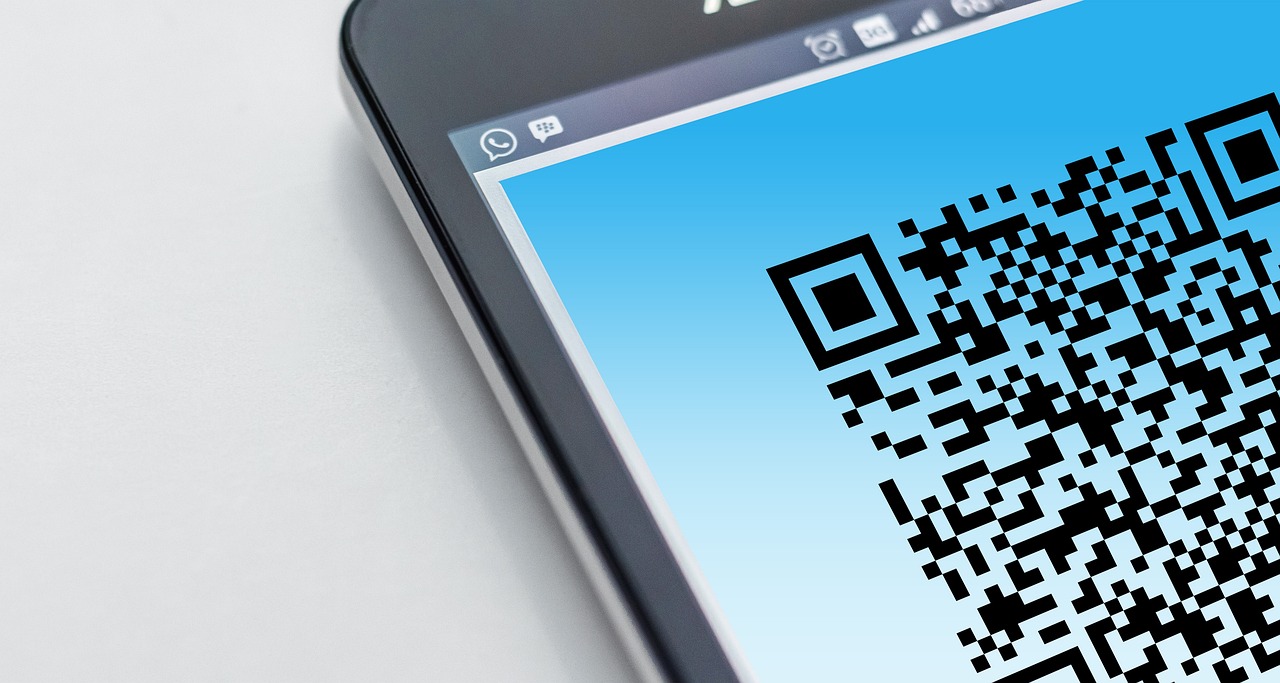
Ethical Considerations
As we dive deeper into the integration of artificial intelligence in disaster response, we must pause and reflect on the that accompany this powerful technology. The stakes are incredibly high; decisions made by AI can directly impact lives, especially in critical situations like natural disasters. Imagine a scenario where an AI system decides which areas receive immediate aid based on data analysis. What happens if the data is flawed or biased? Such dilemmas highlight the urgent need for ethical guidelines in AI applications.
One of the primary concerns is accountability. When an AI system makes a decision that leads to adverse outcomes, who is responsible? Is it the developers, the organizations that deploy the technology, or the AI itself? Establishing clear lines of accountability is crucial to ensure that all parties involved are held to a standard of responsibility. Furthermore, transparency in AI algorithms is essential. Stakeholders must understand how decisions are made to trust these systems. If an AI model is a black box, how can we ensure its decisions are fair and just?
Another ethical aspect to consider is the potential for bias in AI systems. Training data can often reflect societal prejudices, leading to skewed predictions and decisions. For instance, if an AI model is trained predominantly on data from urban areas, it may not perform well in predicting disasters in rural settings. This can exacerbate existing inequalities in disaster response. Therefore, it is vital to ensure diverse and representative data sets are utilized in training AI systems.
Moreover, the issue of privacy cannot be overlooked. During disasters, vast amounts of personal data are collected, often from social media and other platforms. While this data can provide valuable insights, it also raises concerns about how this information is used and who has access to it. Striking a balance between leveraging data for effective disaster response and respecting individuals' privacy rights is a significant ethical challenge.
To address these concerns, organizations must establish comprehensive ethical frameworks that guide the development and deployment of AI in disaster response. These frameworks should include:
- Clear accountability structures to determine responsibility for AI decisions.
- Transparency measures that allow stakeholders to understand AI decision-making processes.
- Bias mitigation strategies to ensure equitable treatment across diverse populations.
- Privacy protection protocols to safeguard personal data collected during disasters.
By prioritizing these ethical considerations, we can harness the full potential of AI in disaster response while ensuring that the technology serves humanity in a fair and just manner. As we move forward, ongoing dialogue among technologists, ethicists, and disaster response professionals will be essential to navigate the complex landscape of AI ethics.
Q: What ethical concerns are associated with AI in disaster response?
A: Key concerns include accountability for decisions made by AI, potential biases in data, transparency in algorithms, and privacy issues regarding personal data collection.
Q: How can bias in AI systems be mitigated?
A: Utilizing diverse and representative data sets during the training phase can help reduce bias in AI systems, ensuring equitable treatment across different demographics.
Q: Why is transparency important in AI decision-making?
A: Transparency allows stakeholders to understand how AI systems make decisions, fostering trust and accountability in critical situations.
Q: What role do ethical frameworks play in AI development?
A: Ethical frameworks provide guidelines that help ensure AI technologies are developed and deployed responsibly, prioritizing fairness, accountability, and privacy.
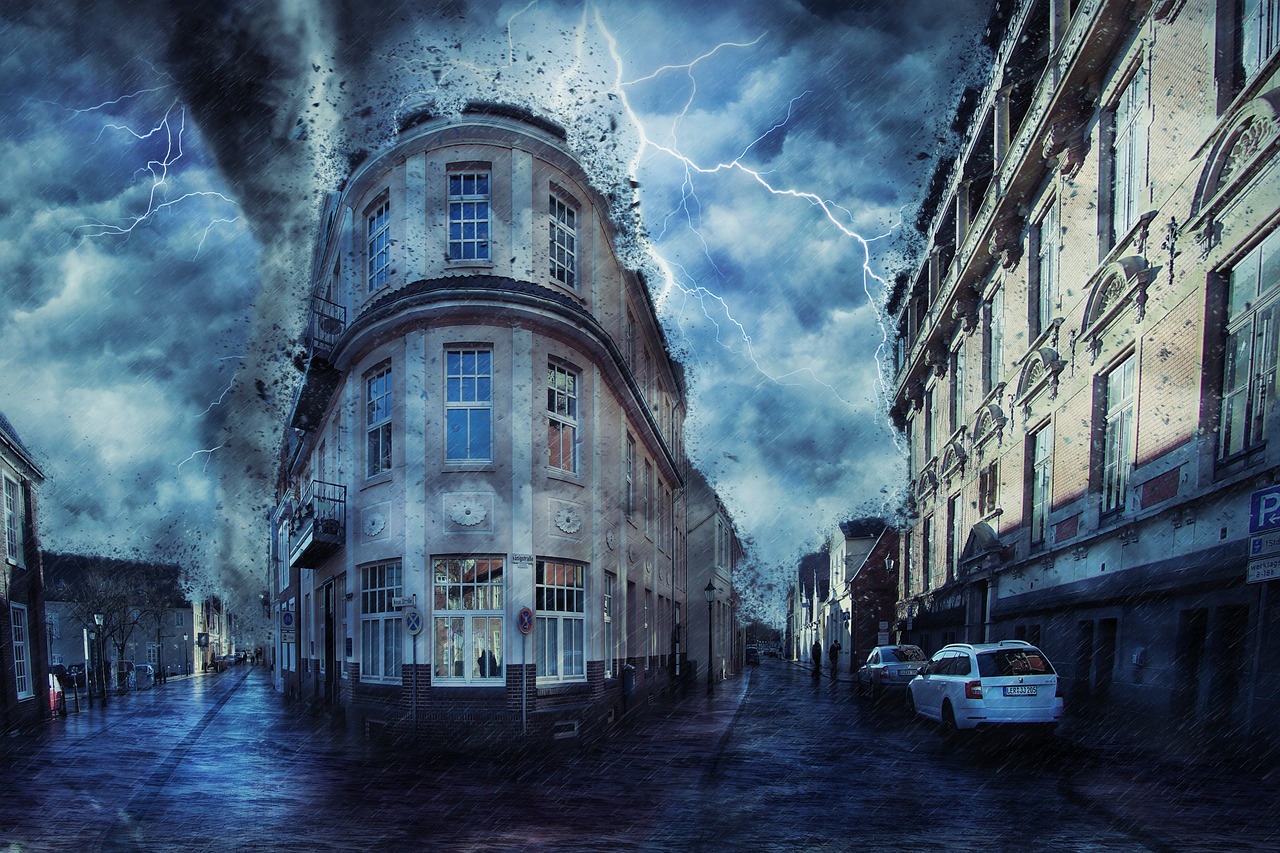
Integration with Existing Systems
Integrating artificial intelligence with existing disaster response systems is not just a technical challenge; it's a complex puzzle that involves various stakeholders, technologies, and processes. Imagine trying to fit a new piece into a jigsaw puzzle where the pieces have been in place for years. The existing systems have their own protocols, workflows, and data formats, which can make the integration of AI technologies feel like trying to fit a square peg into a round hole. This complexity is compounded by the need for real-time data sharing and communication between AI tools and traditional systems.
One of the primary hurdles is the data interoperability issue. Many disaster response organizations rely on legacy systems that may not support the latest data formats or technologies. This can lead to significant delays in response times, as teams struggle to merge AI-generated insights with existing operational data. To overcome this, organizations must invest in creating standardized data formats and protocols that facilitate seamless communication between AI systems and traditional software.
Moreover, training personnel to effectively use these integrated systems is crucial. Emergency responders and decision-makers need to understand how to interpret AI-driven insights and incorporate them into their decision-making processes. This requires comprehensive training programs that not only focus on the technical aspects of AI but also emphasize the importance of human judgment in crisis situations. The goal is to create a synergy where AI enhances human capabilities rather than replacing them.
Another challenge lies in the cultural shift that integration demands. Many organizations have a long-standing culture of relying on established methods and may be resistant to adopting new technologies. To foster acceptance, it's essential to demonstrate the tangible benefits of AI in disaster response, such as improved efficiency, faster response times, and better resource allocation. Engaging stakeholders in the development and implementation process can help ease this transition, making them feel like a vital part of the change rather than passive recipients of new technology.
To illustrate the potential of successful integration, consider a hypothetical scenario where an AI system analyzes social media data during a natural disaster. It detects patterns indicating rising public concern about flooding in a specific area. The AI then alerts local emergency services, who can quickly verify the situation using their existing systems. This timely information allows responders to allocate resources effectively, ensuring that help reaches those in need without unnecessary delays. Such scenarios highlight the importance of a well-integrated system that leverages both AI capabilities and traditional response frameworks.
In conclusion, while the integration of AI into existing disaster response systems presents challenges, the potential benefits far outweigh the hurdles. By focusing on data interoperability, training, and cultural acceptance, organizations can create a robust framework that enhances their disaster response capabilities. The future of disaster management will undoubtedly rely on these integrated systems, where AI and human expertise work hand in hand to save lives and protect communities.
- What are the main challenges of integrating AI into disaster response?
The main challenges include data interoperability, the need for training personnel, and overcoming cultural resistance within organizations.
- How can organizations ensure effective use of AI in disaster management?
Organizations can ensure effective use by investing in training programs, creating standardized data formats, and engaging stakeholders throughout the process.
- What role does human judgment play in AI-driven disaster response?
Human judgment is crucial, as it helps interpret AI-generated insights and make informed decisions during crises.
Frequently Asked Questions
- What role does AI play in disaster prediction?
AI plays a transformative role in disaster prediction by analyzing vast datasets to forecast events such as hurricanes, earthquakes, and floods. This technology enhances preparedness and response strategies, allowing authorities to take proactive measures to mitigate impacts.
- How does AI improve real-time data analysis during disasters?
AI enhances real-time data analysis by processing information from multiple sources, such as weather reports, social media, and sensor data. This capability helps emergency responders make informed decisions quickly, ensuring timely and effective action during crises.
- What are machine learning techniques used in disaster management?
Machine learning techniques, such as predictive modeling and pattern recognition, are utilized in disaster management to identify trends and anticipate potential disasters. These methods enable quicker responses and more accurate predictions, ultimately saving lives and resources.
- How does predictive modeling work in disaster response?
Predictive modeling uses AI-driven algorithms to simulate various disaster scenarios based on historical data and current conditions. By anticipating potential impacts, emergency services can better plan their resources and strategies to effectively respond to disasters.
- What is sentiment analysis, and how is it used in disasters?
Sentiment analysis involves evaluating public reactions during disasters by analyzing social media posts and other online content. This information helps authorities understand public sentiment and tailor their communication strategies to effectively convey critical information and updates.
- How does AI assist in recovery efforts after a disaster?
AI plays a vital role in post-disaster recovery by optimizing resource allocation, assessing damage, and facilitating rebuilding efforts. By analyzing data on affected areas, AI can help ensure that recovery processes are efficient and targeted to the needs of the community.
- What challenges exist in implementing AI for disaster response?
Implementing AI in disaster response faces challenges such as data privacy concerns, unequal access to technology, and the need for skilled personnel to operate AI systems. Addressing these issues is crucial for maximizing the benefits of AI in emergency situations.
- What ethical considerations are involved with AI in disaster scenarios?
The use of AI in disaster response raises ethical questions, particularly regarding decision-making in life-threatening situations. Establishing clear ethical guidelines is essential to ensure that AI applications prioritize human safety and welfare in crisis management.
- How can AI be integrated with existing disaster response systems?
Integrating AI with traditional disaster response systems can be complex, but it is achievable through strategic planning and collaboration. Ensuring seamless communication and data sharing between AI technologies and existing infrastructures is key to enhancing overall disaster response effectiveness.