AI & Ethics: A Framework for Action
The rapid evolution of artificial intelligence (AI) has sparked a crucial conversation about its ethical implications. As we integrate AI into various facets of our lives—from healthcare to finance, and even entertainment—understanding the ethical landscape becomes paramount. This article aims to provide a structured framework for navigating the complex intersection of AI and ethics, ensuring that we consider the responsibilities that come with deploying these powerful technologies.
At its core, AI ethics revolves around the question of how we can harness the potential of AI while safeguarding human values and societal norms. With great power comes great responsibility, and as we stand on the brink of an AI-driven future, it is essential to reflect on the challenges and implications of our technological advancements. The decisions we make today will shape the world of tomorrow, and a solid ethical foundation will guide us in making choices that benefit everyone.
As we dive deeper into the intricacies of AI ethics, we will explore various principles that serve as guiding lights in this uncharted territory. These principles—fairness, accountability, transparency, and privacy—are not just buzzwords; they are essential pillars that help mitigate the risks associated with AI technologies. By understanding these ethical principles, we can better navigate the murky waters of AI deployment and governance.
So, what does this all mean for individuals and society at large? It means that as users, developers, and policymakers, we must engage in a thoughtful dialogue about the implications of AI. We need to ask ourselves critical questions: How do we ensure that AI systems are fair and just? Who is accountable for the decisions made by AI? How can we maintain transparency in AI operations? By addressing these questions, we can create a more ethical framework for AI that prioritizes human dignity and societal welfare.
In the sections that follow, we'll delve into the foundational principles of AI ethics, examining their relevance and application in real-world scenarios. It’s not just about understanding these concepts; it’s about putting them into action. Together, we can pave the way for a future where AI serves humanity positively and ethically.
- What is AI ethics? AI ethics refers to the moral implications and responsibilities associated with the development and deployment of artificial intelligence technologies.
- Why is fairness important in AI? Fairness in AI ensures that systems do not perpetuate biases or discrimination, promoting equitable outcomes for all users.
- How can accountability be enforced in AI systems? Accountability can be established through clear guidelines, regulations, and frameworks that define responsibility for AI decisions.
- What role does transparency play in AI ethics? Transparency fosters trust by ensuring that AI systems are understandable and their decision-making processes are clear to users.
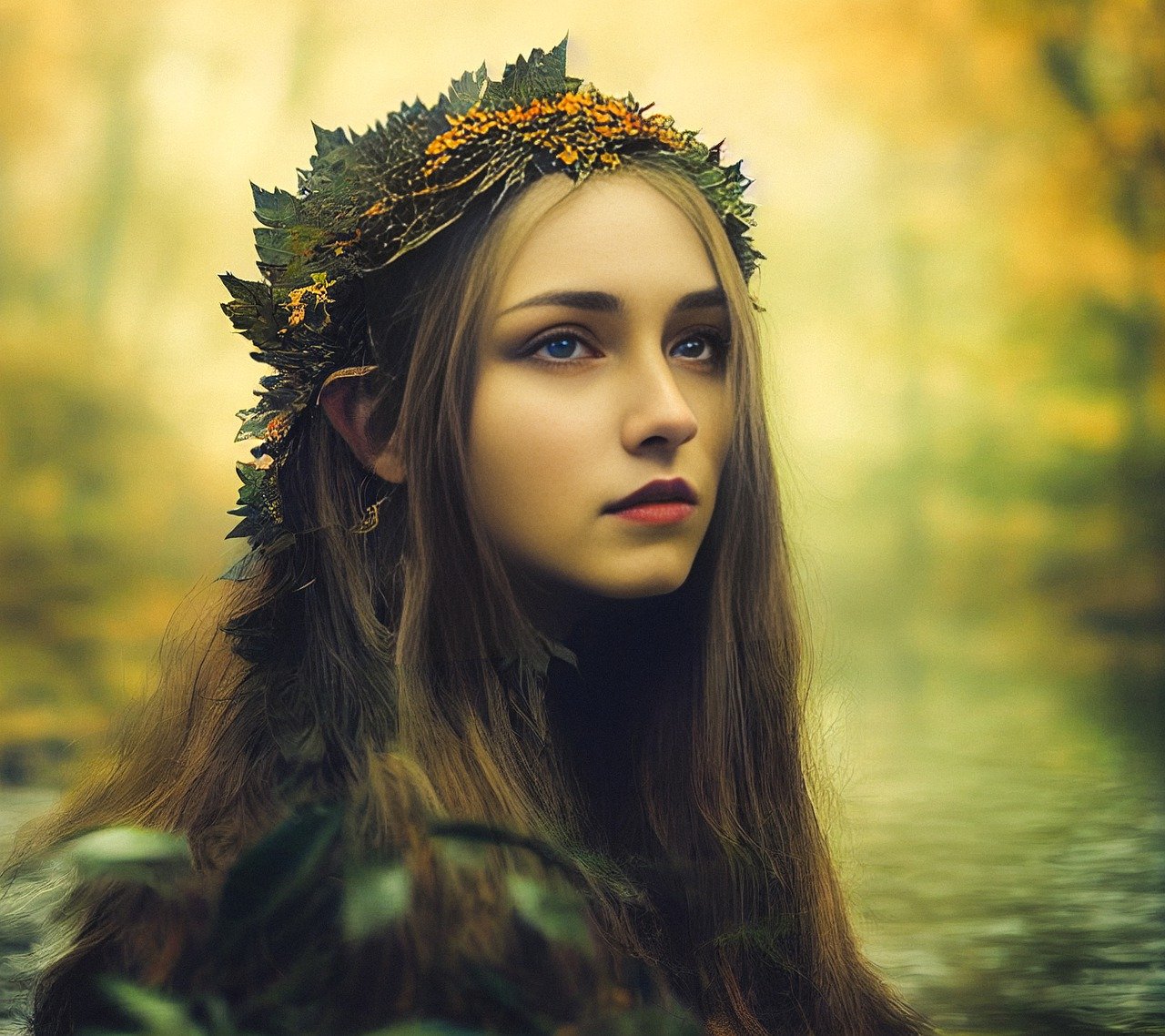
Understanding AI Ethics
In today's rapidly evolving technological landscape, the importance of artificial intelligence (AI) cannot be overstated. It permeates our daily lives, influencing everything from the music we listen to, to the recommendations we receive on social media, and even the decisions made in healthcare. However, with great power comes great responsibility. This is where AI ethics steps in, serving as a crucial framework for understanding not just the capabilities of AI, but also its implications and challenges.
At its core, AI ethics is about ensuring that the development and deployment of AI technologies are guided by principles that promote fairness, accountability, and transparency. The ethical considerations surrounding AI are not merely academic; they have real-world implications that can affect individuals and communities in profound ways. Imagine a world where AI systems make decisions that impact your life—be it in hiring, lending, or even law enforcement. Wouldn’t you want to know that these systems operate on principles that are just and equitable?
The intersection of AI and ethics is not just a theoretical concept; it’s a pressing concern that demands our attention. As AI continues to evolve, we must ask ourselves critical questions: How do we ensure that AI systems do not perpetuate existing biases? Who is responsible when an AI system makes a mistake? What frameworks can we put in place to hold AI accountable? These questions are not just for tech experts; they are for all of us, as we navigate a future increasingly shaped by AI.
To truly understand AI ethics, we must explore its foundational principles. These principles serve as a compass, guiding developers, policymakers, and users alike. They are essential in creating AI that not only performs well but also aligns with our societal values. Below are some of the key ethical principles that are gaining traction in discussions about AI:
- Fairness: Ensuring that AI systems do not discriminate against any group.
- Accountability: Establishing clear lines of responsibility for AI decisions.
- Transparency: Making AI processes understandable to users and stakeholders.
- Privacy: Safeguarding personal data against misuse.
As we delve deeper into these principles, it's vital to recognize that ethical AI is not merely a checkbox to tick off during development. It requires ongoing dialogue, collaboration, and a commitment to continuous improvement. The challenges are significant, but the opportunities to create a more equitable and just society through responsible AI deployment are even greater.
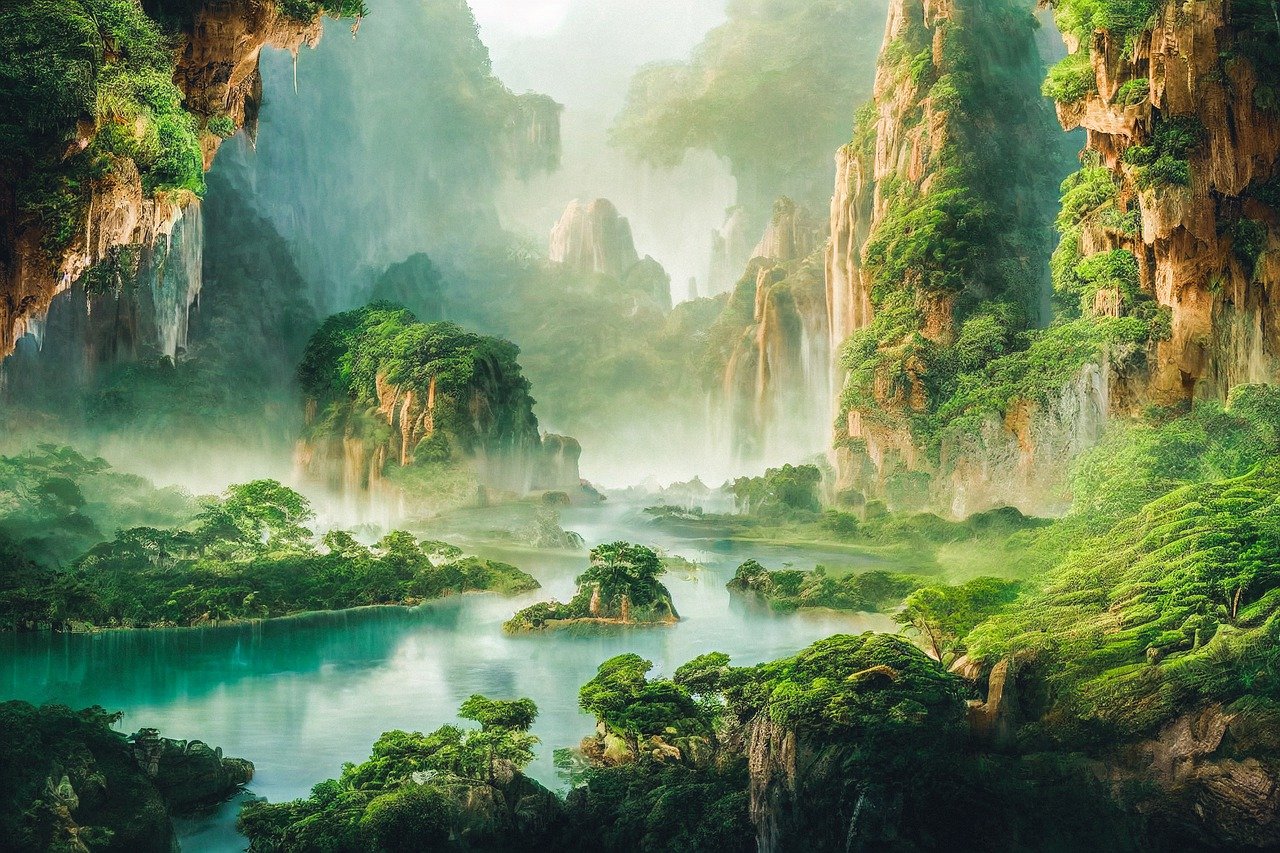
Key Ethical Principles
As we navigate the rapidly evolving landscape of artificial intelligence, it's crucial to anchor our understanding in a set of . These principles not only guide the development of AI technologies but also ensure that they serve humanity in a responsible manner. The four cornerstones of AI ethics include fairness, accountability, transparency, and privacy. Each of these principles plays a pivotal role in mitigating the risks associated with AI, fostering trust, and promoting equitable outcomes across diverse populations.
Let’s take a closer look at these principles. Fairness in AI is about ensuring that algorithms do not perpetuate existing biases or create new forms of discrimination. This means that developers must actively seek to identify and eliminate biases in their datasets and algorithms. It’s not just about avoiding harm; it’s about actively promoting equitable outcomes for all users, regardless of their background. This requires a commitment to inclusivity in data collection and model training, which is essential for building systems that reflect the diversity of our society.
Next, we have accountability. As AI systems make decisions that can significantly impact lives, it's vital to establish clear lines of responsibility. Who is accountable when an AI system makes a mistake? Is it the developer, the organization deploying the AI, or perhaps even the AI itself? This question of accountability is not just a legal concern; it’s also a matter of public trust. When users understand who is responsible for AI decisions, they are more likely to trust the technology and its applications.
Then, we delve into transparency. In a world where AI systems are often seen as black boxes, it’s essential to promote clear communication about how these systems function and make decisions. Transparency fosters trust and understanding among users. Imagine if you could see the inner workings of an AI system, much like peeking behind the curtain in a magic show. This understanding can demystify AI and empower users to engage with it more confidently.
Finally, we must consider privacy. As AI systems often rely on vast amounts of personal data, maintaining user privacy is paramount. It’s not just about compliance with regulations; it’s about respecting individuals’ rights and fostering a sense of security. Developers must implement robust data protection measures and ensure that users have control over their data. This is akin to having a safe where you can store your valuables, knowing that only you have the key.
In summary, the ethical principles of fairness, accountability, transparency, and privacy are not merely theoretical concepts; they are practical guidelines that can help shape the future of AI. By embedding these principles into the design and deployment of AI systems, we can work towards a future where technology serves as a force for good, enhancing our lives while respecting our rights and dignity.
- What is the importance of fairness in AI?
Fairness ensures that AI systems do not discriminate against any group, promoting equitable outcomes across society. - How can accountability be established in AI systems?
Clear lines of responsibility must be defined, so users know who to turn to when AI systems make erroneous decisions. - Why is transparency crucial for AI?
Transparency helps users understand how AI systems work, fostering trust and encouraging responsible use. - What measures can protect user privacy in AI?
Implementing strong data protection protocols and allowing users control over their data are essential for safeguarding privacy.
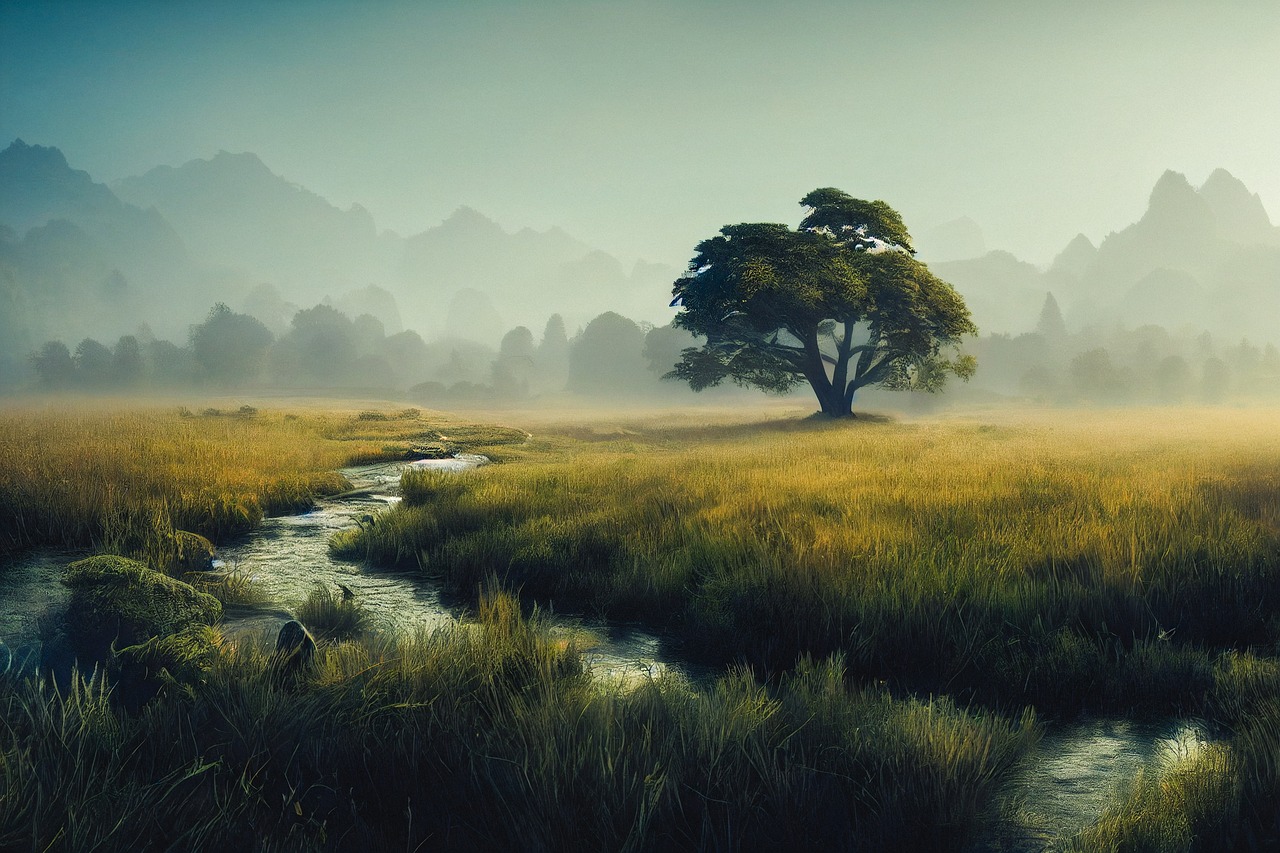
Fairness in AI
When we talk about , we’re diving into a realm that’s not just technical but deeply human. Imagine a world where decisions that affect our lives—like job applications, loan approvals, or even legal judgments—are made by algorithms that might not treat everyone equally. It’s a bit like playing a game where the rules are rigged, and some players have an unfair advantage. This is why understanding fairness in AI is crucial; it’s about ensuring that technology serves everyone, not just a select few.
At the heart of this discussion lies the concept of bias. AI systems learn from data, and if that data reflects existing societal biases, the AI will likely perpetuate and even amplify those biases. For instance, if an AI system is trained on historical hiring data that favors one demographic over others, it might continue to favor that demographic in its recommendations. This creates a cycle of inequality that can be hard to break. Therefore, addressing bias is not just a technical challenge; it’s a moral imperative.
So, how do we tackle these biases and strive for equitable outcomes? First, we must recognize the sources of bias in AI systems. This can stem from various factors, such as:
- Data Selection: If the data used to train AI is not representative of the entire population, the outcomes will skew towards the majority.
- Algorithm Design: The way algorithms are structured can inadvertently favor certain groups over others.
- Human Oversight: Bias can also be introduced through the subjective choices made by developers and data scientists.
To combat these issues, several strategies can be implemented. One of the most effective approaches is to ensure diversity in the data sets used for training AI. This means actively seeking out data that includes voices and experiences from all segments of society. Additionally, employing inclusive practices during the development phase can help identify potential biases early on. For instance, involving diverse teams in the design process can lead to more comprehensive solutions that take different perspectives into account.
Moreover, there are frameworks and tools available that can help promote fairness in AI applications. These include:
- Fairness Audits: Regularly assessing AI systems for biased outcomes can help identify and rectify inequalities.
- Algorithmic Transparency: Developing algorithms that can be easily understood and scrutinized by stakeholders fosters accountability.
- Regulatory Guidelines: Implementing policies that mandate fairness checks in AI systems can ensure ethical practices are followed.
In summary, fairness in AI is not just about avoiding discrimination; it’s about actively promoting equity. By recognizing biases, implementing diverse data practices, and utilizing fairness frameworks, we can create AI systems that truly reflect our values of inclusivity and justice. After all, the goal of AI should be to enhance human life, not complicate it with inequalities. As we continue to innovate, let’s ensure that fairness remains at the forefront of our AI endeavors.
- What is fairness in AI? Fairness in AI refers to the principle that AI systems should treat all individuals equally and not perpetuate existing biases.
- How can bias be identified in AI systems? Bias can be identified through fairness audits, which assess the outcomes of AI decisions across different demographic groups.
- What strategies can promote fairness in AI? Strategies include using diverse data sets, conducting regular audits, and ensuring inclusive practices throughout the development process.
- Why is transparency important in AI? Transparency helps build trust between AI systems and users, allowing stakeholders to understand how decisions are made.
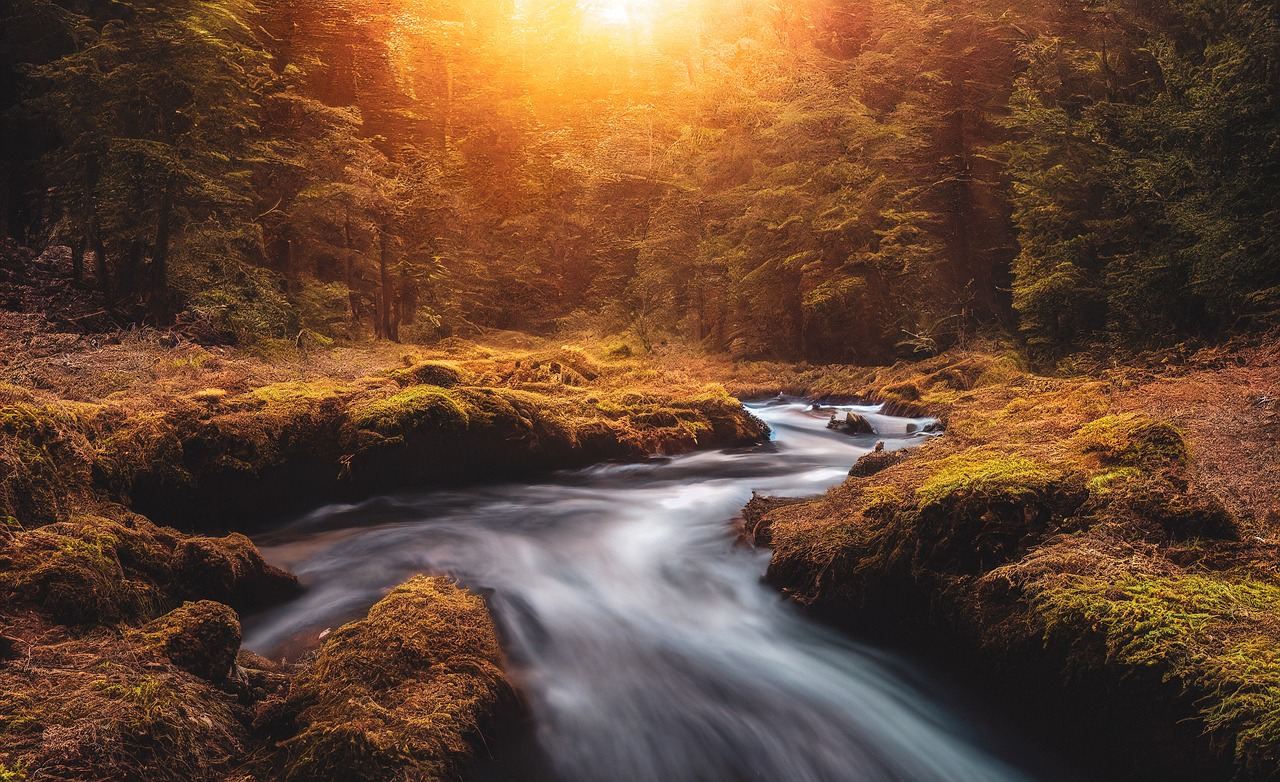
Addressing Bias
In the rapidly evolving landscape of artificial intelligence, bias has emerged as a significant concern that can skew results and perpetuate inequality. Bias in AI systems can stem from various sources, such as the data used to train these algorithms, the design choices made by developers, and even the cultural contexts in which these systems operate. It’s crucial to recognize that bias is not merely a technical flaw; it can have real-world implications that affect people's lives, especially in critical areas like hiring, law enforcement, and healthcare.
To effectively address bias, we must first identify its presence within AI algorithms. This involves rigorous testing and analysis of the data sets employed. For instance, if an AI system is trained on data that predominantly represents one demographic, it may not perform well for underrepresented groups. Therefore, creating diverse data sets is essential. This means not only including a variety of demographic groups but also ensuring that the data reflects a wide range of experiences and perspectives.
Moreover, implementing inclusive practices during the development phase can significantly mitigate bias. This could involve assembling diverse teams of developers and stakeholders who can provide different viewpoints and challenge assumptions. By fostering an environment where multiple voices are heard, we can enhance the design process and create more equitable AI systems.
Another effective strategy is the use of bias detection tools. These tools can help developers identify and rectify biases in their algorithms before deployment. For example, techniques like adversarial testing can simulate various scenarios to see how the AI behaves across different demographic groups. This proactive approach can prevent biases from becoming embedded in the system, ensuring that outcomes are fair and just.
Ultimately, the goal is to achieve equitable outcomes across all AI applications. This means that AI systems should not only be designed to be efficient but also to be just. To this end, developers can utilize frameworks such as the Fairness Toolkit that provide guidelines and metrics for measuring fairness in AI outputs. By prioritizing fairness, we can help ensure that AI serves as a tool for empowerment rather than a mechanism for discrimination.
In conclusion, addressing bias in AI is not just a technical challenge; it’s a moral imperative. By being vigilant about the data we use, the teams we assemble, and the tools we employ, we can create AI systems that truly reflect the diverse world we live in. The journey towards unbiased AI is ongoing, but with concerted effort and commitment, we can pave the way for a future where technology uplifts everyone.
- What is bias in AI? Bias in AI refers to systematic errors that lead to unfair outcomes, often due to skewed training data or flawed algorithms.
- How can bias be detected in AI systems? Bias can be detected through rigorous testing, analysis of data sets, and the use of bias detection tools.
- Why is diversity important in AI development? Diversity helps ensure that multiple perspectives are considered, which can lead to more equitable AI systems.
- What are some tools for addressing bias? Tools like the Fairness Toolkit and adversarial testing can help developers identify and rectify biases in AI algorithms.
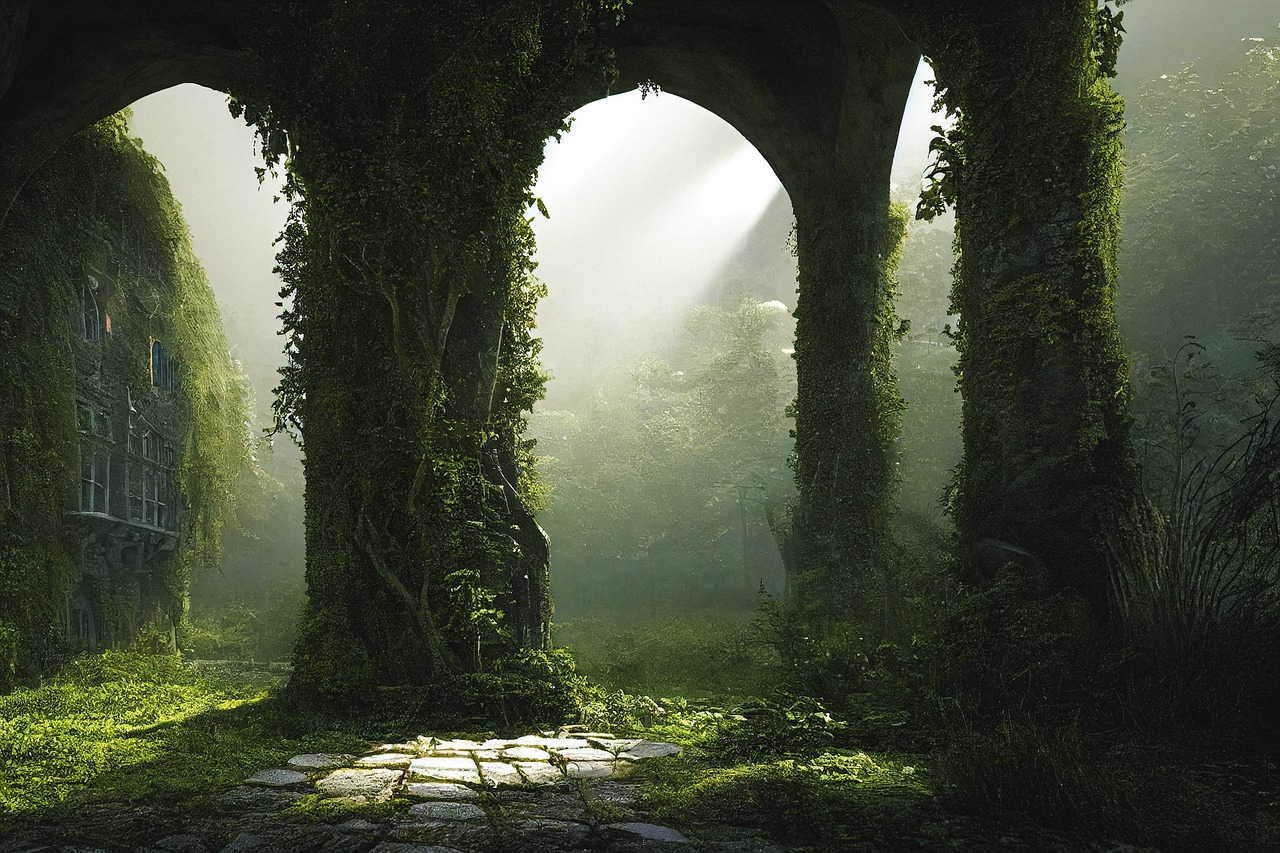
Equitable Outcomes
When we talk about in the realm of artificial intelligence, we're diving deep into the heart of fairness and justice. Imagine a world where AI systems not only perform tasks efficiently but also do so without perpetuating existing biases or inequalities. This is not just a lofty ideal; it's a necessity for building a future where technology serves everyone equally. Achieving equitable outcomes involves creating frameworks and tools that ensure AI applications benefit all segments of society, especially those historically marginalized.
To truly understand the importance of equitable outcomes, we must first recognize that AI systems are only as good as the data they are trained on. If the data reflects societal biases, the AI will inevitably learn and replicate these biases. Therefore, one of the primary strategies for promoting fairness is to ensure that the data sets used in AI training are diverse and representative. This means including voices and experiences from various demographics, ensuring that no group is left behind. After all, if we want AI to reflect our society accurately, it must be trained on data that reflects the rich tapestry of human experience.
Moreover, organizations can implement specific frameworks to promote equitable outcomes. For instance, employing impact assessments before deploying AI systems can help identify potential biases and their implications on different groups. These assessments act as a checkpoint, allowing developers to consider the broader societal impact of their AI solutions. By asking critical questions, such as, "Who might be negatively affected by this technology?" or "Does this system favor one group over another?" organizations can take proactive steps to mitigate risks.
Another essential aspect of achieving equitable outcomes is the continuous monitoring of AI systems post-deployment. This is where feedback loops come into play. By gathering data on how AI systems perform across different populations, organizations can make necessary adjustments to algorithms, ensuring they remain fair and effective over time. This iterative process not only improves the technology but also builds trust with users who see their concerns being addressed.
In summary, striving for equitable outcomes in AI is not just about compliance; it's about creating a future where technology uplifts everyone. By focusing on diverse data sets, conducting thorough impact assessments, and establishing robust feedback mechanisms, we can pave the way for AI systems that truly embody fairness and justice. This commitment to equity not only enhances the effectiveness of AI applications but also fosters a sense of community and shared responsibility among developers, users, and society at large.
- What are equitable outcomes in AI? Equitable outcomes refer to AI systems that deliver fair and just results across diverse populations, avoiding biases and inequalities.
- How can biases in AI be mitigated? By using diverse and representative data sets, conducting impact assessments, and implementing continuous monitoring of AI systems.
- Why is transparency important in AI? Transparency fosters trust and understanding by providing clear communication about how AI systems function and make decisions.
- What role do regulations play in AI ethics? Regulations help establish guidelines and standards for ethical AI practices, ensuring that technology aligns with societal values.
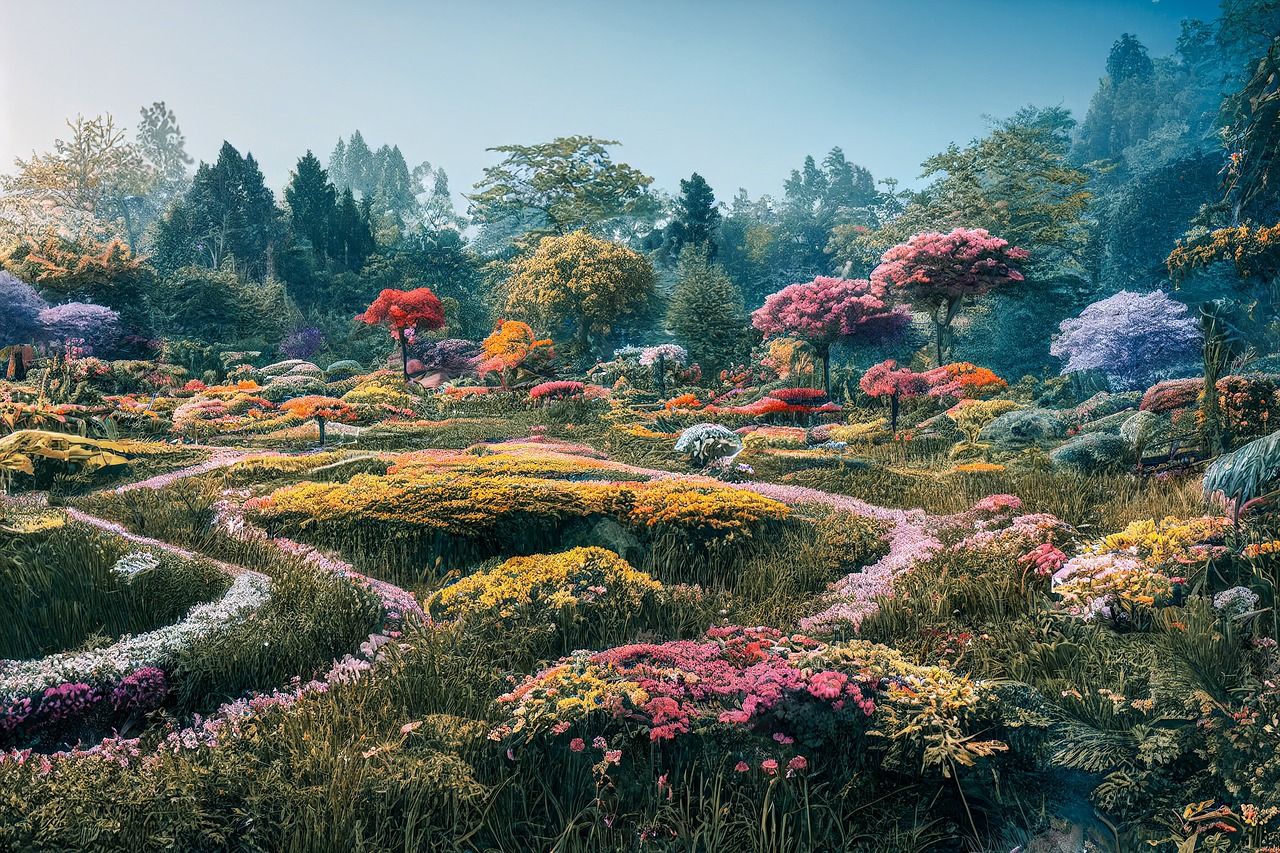
Accountability in AI
Accountability in artificial intelligence (AI) is not just a buzzword; it’s a fundamental principle that underpins trust and governance in the digital age. When we think about AI systems making decisions that can significantly affect our lives—whether it's approving a loan, diagnosing a medical condition, or even recommending content on social media—we must ask ourselves, who is responsible for these decisions? This question is crucial because the implications of accountability stretch far beyond mere technicalities; they touch on ethics, legality, and societal values.
In a world increasingly driven by AI, the stakes are high. Imagine a scenario where an AI system denies someone a job based on biased data. Who do you hold accountable for that decision? The developers? The organization using the AI? Or the AI itself? This complexity is where accountability becomes essential. Clear lines of responsibility need to be established to ensure that AI systems are not only effective but also just and fair. Without accountability, we risk eroding public trust in AI technologies, leading to widespread skepticism and resistance to their adoption.
To navigate these challenges, organizations must implement robust accountability frameworks. These frameworks should outline the roles and responsibilities of various stakeholders involved in the AI lifecycle, from development to deployment. Here are some key elements that can enhance accountability in AI:
- Clear Ownership: Establish who is responsible for the AI system's outcomes. This could be a specific team or an individual, ensuring that there is a point of contact for any issues that arise.
- Documentation: Maintain comprehensive records of the AI development process, including data sources, algorithms used, and decision-making criteria. This transparency is vital for understanding how decisions are made.
- Regular Audits: Conduct frequent audits of AI systems to assess their performance and identify any biases or errors. This proactive approach can help mitigate risks before they escalate.
- User Feedback Mechanisms: Create channels for users to report issues or concerns regarding AI decisions. This feedback can be invaluable for continuous improvement and accountability.
Moreover, the implications of accountability extend to legal frameworks as well. As regulations around AI evolve, organizations must align their practices with laws that govern data protection, discrimination, and ethical standards. For instance, the General Data Protection Regulation (GDPR) in Europe emphasizes the right to explanation, meaning that individuals should be able to understand the reasoning behind automated decisions that affect them. This legal requirement not only promotes accountability but also empowers users, giving them a sense of agency in an increasingly automated world.
In conclusion, accountability in AI is not merely an operational necessity; it is a moral imperative. As we continue to integrate AI into various sectors, establishing clear lines of accountability will be crucial to fostering trust and ensuring that these powerful technologies serve the greater good. By embracing accountability, we can harness the full potential of AI while safeguarding ethical standards and societal values.
- What does accountability in AI mean? Accountability in AI refers to the responsibility of individuals or organizations to ensure that AI systems operate ethically and transparently, with clear lines of responsibility for their outcomes.
- Why is accountability important in AI? It is important because it helps build trust in AI systems, ensures ethical decision-making, and provides a framework for addressing any potential harms caused by these technologies.
- How can organizations ensure accountability in their AI systems? Organizations can ensure accountability by establishing clear ownership, maintaining thorough documentation, conducting regular audits, and implementing user feedback mechanisms.
- What role do regulations play in AI accountability? Regulations provide legal frameworks that mandate ethical practices, such as the right to explanation under GDPR, thereby reinforcing accountability in AI systems.
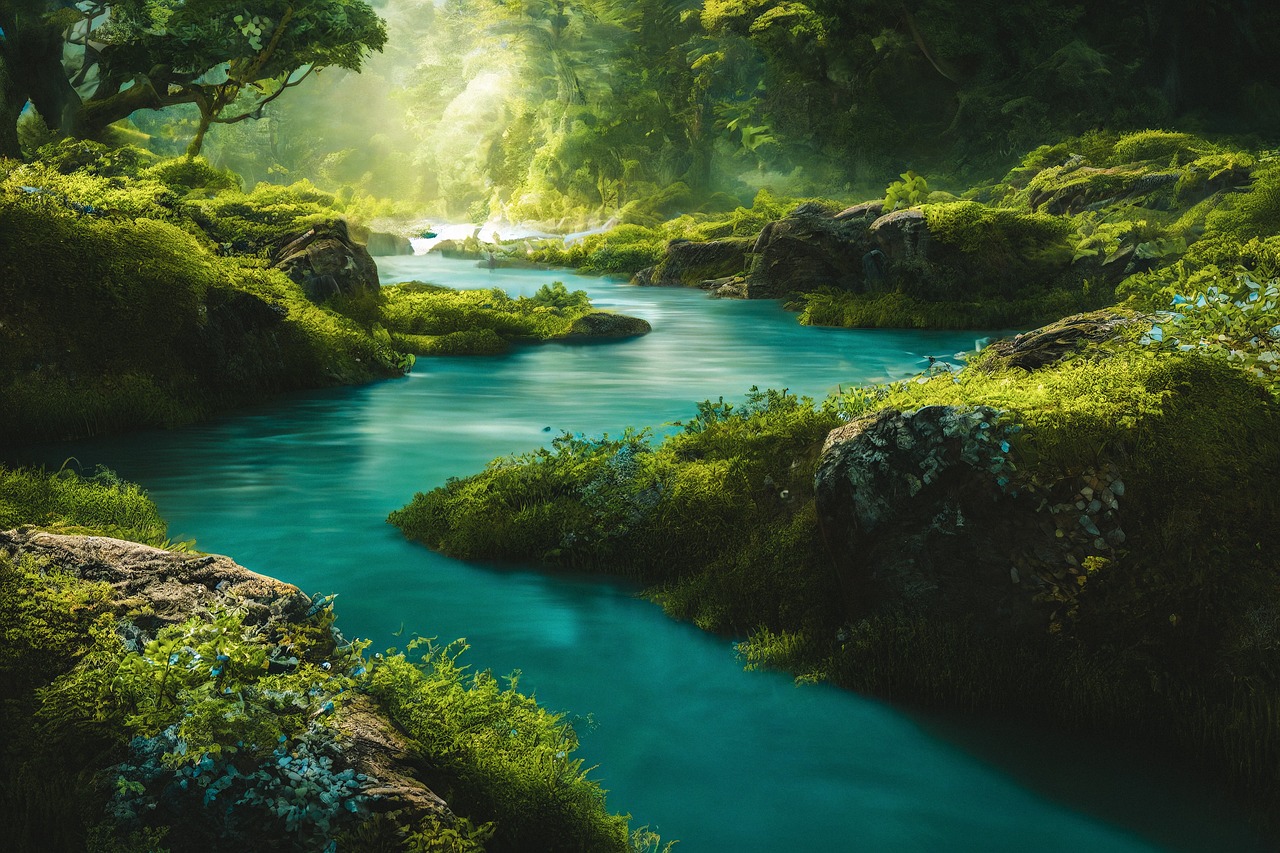
Transparency in AI
In the rapidly evolving world of artificial intelligence, transparency has emerged as a cornerstone principle that cannot be overlooked. Imagine trying to navigate a dense fog without a map or a compass; that’s what it feels like for many when they interact with AI systems that operate behind a veil of secrecy. Transparency in AI is not just about making systems understandable; it’s about fostering a culture of trust and accountability between the technology and its users. As AI becomes more integrated into everyday life, the demand for clear communication regarding how these systems function and make decisions has never been more critical.
One of the primary reasons transparency is vital is that it helps demystify the decision-making processes of AI systems. Users deserve to know how their data is being utilized and how decisions that affect their lives are made. This clarity not only enhances user trust but also empowers individuals to make informed choices. When users understand the algorithms at play, they are more likely to engage with these technologies positively. Furthermore, transparency can serve as a safeguard against the misuse of AI, ensuring that ethical standards are upheld and that systems are not operating in a black box.
To illustrate the importance of transparency, consider the following aspects:
- Public Trust: Transparent AI systems are more likely to gain public trust. When users feel informed about how decisions are made, they are less likely to harbor fears or misconceptions about AI.
- Accountability: Transparency facilitates accountability. If an AI system makes a mistake, understanding the decision-making process allows for proper attribution of responsibility and the opportunity to rectify errors.
- Compliance: Many industries are governed by strict regulations regarding data use and privacy. Transparent AI systems can help organizations comply with these regulations by providing clear insights into how data is processed.
Moreover, the concept of Explainable AI (XAI) is a significant component of transparency. XAI refers to methods and techniques in AI that make the outputs of AI systems understandable to humans. This approach is crucial, especially in high-stakes scenarios like healthcare or criminal justice, where understanding the rationale behind AI decisions can have profound implications. For instance, if an AI system denies a loan application, the applicant has the right to know why. This not only promotes fairness but also allows for the opportunity to challenge or appeal decisions made by AI.
As we look toward the future, it is essential to consider how regulatory frameworks can support transparency in AI. Governments and organizations need to establish guidelines that require AI developers to disclose information about their systems' functionalities and decision-making processes. Such regulations could include:
Regulatory Aspect | Description |
---|---|
Mandatory Disclosure | Requiring AI developers to provide clear documentation of how their systems work. |
Impact Assessments | Conducting assessments to evaluate the societal impact of AI systems before deployment. |
User Education | Implementing programs to educate users on AI functionalities and limitations. |
In conclusion, transparency in AI is not merely a regulatory checkbox; it is a fundamental requirement for building trust and ensuring ethical practices in technology. As we navigate the complexities of AI, prioritizing transparency will lead to more responsible and beneficial outcomes for society. By embracing transparency, we can create a future where AI serves as a powerful ally rather than a mysterious adversary.
- What is transparency in AI? Transparency in AI refers to the clear communication of how AI systems function, including their decision-making processes and data usage.
- Why is transparency important? It fosters trust, accountability, and compliance with regulations, empowering users to make informed decisions.
- What is Explainable AI (XAI)? XAI is an approach that aims to make AI outputs understandable to humans, crucial in high-stakes scenarios.
- How can regulations support transparency? Regulations can mandate disclosure, conduct impact assessments, and promote user education about AI systems.
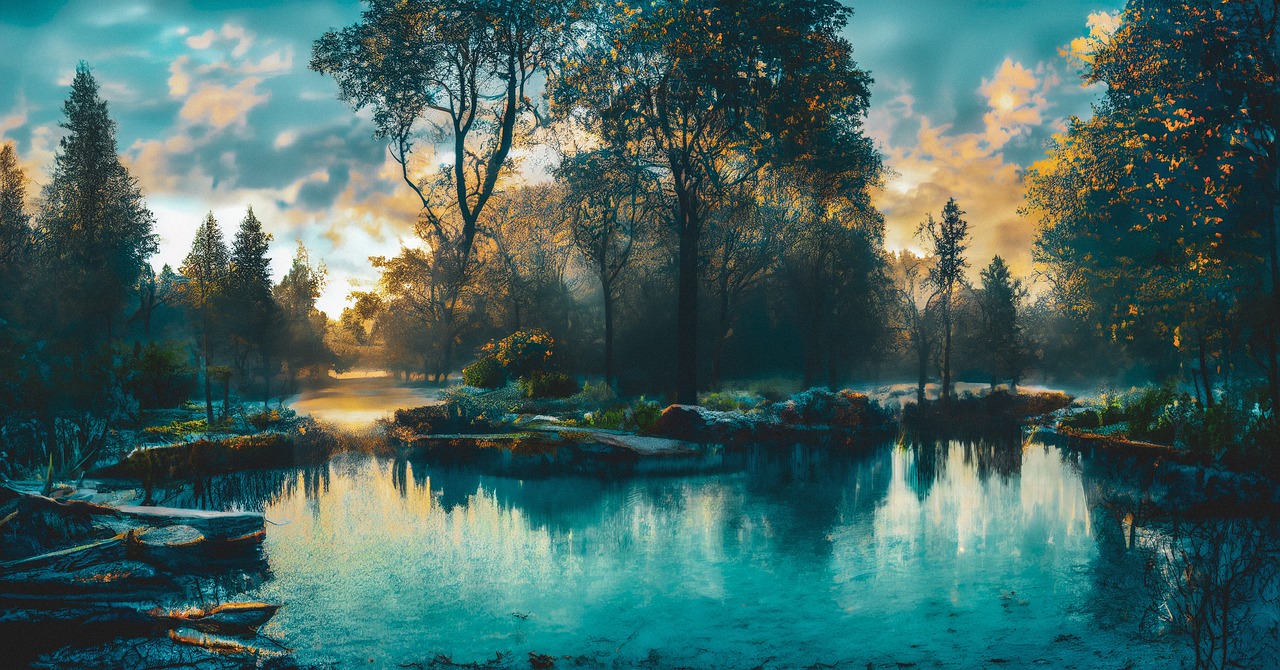
Explainable AI
When we talk about , we’re diving into a realm that’s absolutely crucial for the future of technology and society. Imagine you’re driving a car, and suddenly, the navigation system tells you to take a turn without explaining why. You’d probably feel uneasy, right? This analogy perfectly encapsulates the essence of explainability in AI systems. If users can't understand how decisions are made, trust erodes, and the technology becomes a black box, shrouded in mystery.
The goal of explainable AI is to make the decision-making processes of AI systems transparent and understandable to humans. This is especially vital in sectors like healthcare, finance, and law, where AI decisions can significantly impact lives. Think about it: if an AI system denies a loan or diagnoses a medical condition, the individuals affected deserve to know the reasoning behind those decisions. Without this clarity, the potential for harm—intentional or otherwise—grows exponentially.
There are several approaches to achieving explainability in AI. For instance, model-agnostic methods can be applied to any AI system, providing insights into how decisions are made regardless of the underlying technology. On the other hand, model-specific methods focus on the intricacies of particular algorithms, like decision trees or neural networks, to offer tailored explanations. Here’s a simple breakdown of the two:
Method Type | Description |
---|---|
Model-Agnostic | Applicable to any AI model, providing general insights. |
Model-Specific | Focuses on specific algorithms, offering detailed explanations. |
Moreover, the importance of explainable AI extends beyond just understanding. It plays a pivotal role in ensuring compliance with regulations and ethical standards. For instance, in the European Union, the General Data Protection Regulation (GDPR) emphasizes the right to explanation, mandating that individuals can seek clarity on automated decisions affecting them. This legal framework not only promotes transparency but also encourages organizations to adopt practices that prioritize explainability.
However, achieving explainability is not without its challenges. Many advanced AI models, particularly deep learning systems, operate on complex layers of data processing that can be difficult to decipher, even for experts. This complexity raises the question: how do we balance performance with interpretability? Striking this balance is essential, as overly simplistic models may fail to capture the nuances of data, while overly complex models may become too opaque.
To tackle these challenges, researchers and practitioners are exploring various strategies, including:
- Developing visualization tools that help users understand model decisions.
- Creating simplified surrogate models that approximate the behavior of complex models.
- Enhancing user interfaces to present explanations in intuitive ways.
As we continue to integrate AI into our daily lives, the demand for explainable AI will only grow. Users need to feel confident in the decisions made by these systems. After all, trust is the bedrock of any relationship, and it’s no different when it comes to technology. By prioritizing explainable AI, we’re not just enhancing user experience; we’re fostering a culture of accountability and responsibility in the tech landscape.
- What is Explainable AI? Explainable AI refers to methods and techniques in AI that make the decision-making processes of AI systems transparent and understandable to humans.
- Why is Explainable AI important? It builds trust, ensures compliance with regulations, and allows users to understand the reasoning behind AI decisions, which is crucial in sensitive areas like healthcare and finance.
- What are the challenges of implementing Explainable AI? The main challenges include the complexity of advanced AI models, the trade-off between performance and interpretability, and the need for effective communication of explanations.
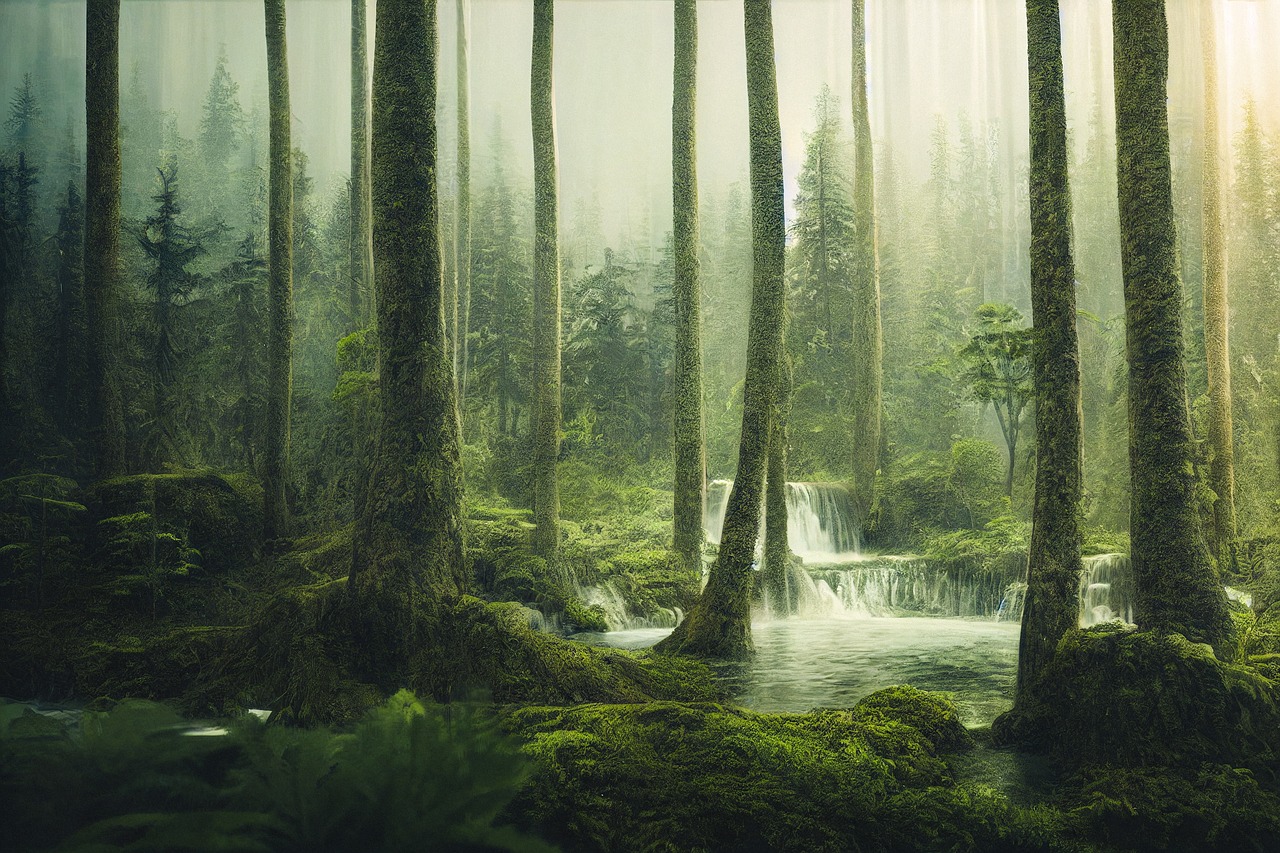
Regulatory Frameworks
In the ever-evolving landscape of artificial intelligence, establishing robust is critical to ensure that AI technologies are developed and deployed responsibly. As AI systems become increasingly integrated into our daily lives, the need for guidelines that align with ethical considerations and societal values has never been more pressing. Regulatory frameworks serve as a roadmap, guiding organizations and governments in the ethical use of AI while protecting the rights and interests of individuals and communities.
Across the globe, various governments and organizations are beginning to recognize the importance of implementing regulations that address the unique challenges posed by AI. These frameworks typically encompass a variety of components, including:
- Standards and Guidelines: Establishing clear standards for AI development ensures that ethical considerations are integrated from the outset, minimizing risks associated with bias, privacy violations, and accountability failures.
- Compliance Mechanisms: Regulatory bodies need to enforce compliance with established guidelines to ensure that organizations adhere to ethical practices in AI deployment.
- Public Engagement: Involving stakeholders, including the public, in the regulatory process helps to foster transparency and trust, as diverse perspectives can inform better decision-making.
For instance, the European Union has taken significant steps towards creating a comprehensive regulatory framework for AI with its proposed AI Act. This legislation aims to categorize AI applications based on risk levels, imposing stricter requirements on high-risk systems while allowing for more flexibility with lower-risk applications. Such proactive measures demonstrate a commitment to responsible AI governance, ensuring that innovation does not come at the cost of ethical considerations.
Additionally, organizations like the OECD and the IEEE have developed principles and guidelines that encourage ethical AI practices. These principles often emphasize the importance of fairness, accountability, and transparency, providing a foundation for organizations to build upon as they navigate the complexities of AI ethics.
However, the development of regulatory frameworks is not without its challenges. Striking the right balance between fostering innovation and ensuring ethical compliance can be difficult. Overly restrictive regulations may stifle creativity and slow down technological advancements, while lax regulations could lead to significant ethical breaches and societal harm. Therefore, it is essential for regulators to engage in continuous dialogue with AI developers, ethicists, and the public to create adaptable frameworks that can evolve alongside technological advancements.
As we look to the future, the establishment of effective regulatory frameworks will be paramount in shaping the trajectory of AI technologies. By prioritizing ethical considerations and aligning regulations with societal values, we can foster an environment where AI thrives responsibly, ultimately benefiting everyone.
1. What are regulatory frameworks in AI?
Regulatory frameworks in AI are structured guidelines and standards established by governments and organizations to ensure the ethical development and deployment of artificial intelligence technologies.
2. Why are regulatory frameworks necessary for AI?
They are necessary to mitigate risks associated with AI, such as bias, lack of accountability, and privacy concerns, while promoting public trust and ethical practices in AI deployment.
3. How do different countries approach AI regulation?
Countries vary in their approaches; for example, the European Union is developing comprehensive regulations, while other nations may adopt more flexible guidelines to encourage innovation.
4. Can regulatory frameworks adapt to technological advancements?
Yes, effective regulatory frameworks should be designed to evolve alongside technological advancements, allowing for continuous dialogue and updates based on new developments in AI.
Frequently Asked Questions
- What is AI ethics?
AI ethics refers to the moral principles and guidelines that govern the development and implementation of artificial intelligence technologies. It encompasses considerations such as fairness, accountability, transparency, and privacy, ensuring that AI systems are designed and used responsibly, with respect for human rights and societal values.
- Why is fairness important in AI?
Fairness in AI is crucial because biased algorithms can lead to discriminatory outcomes, affecting marginalized groups disproportionately. By prioritizing fairness, developers can create AI systems that promote equitable treatment and opportunities for all individuals, regardless of their background.
- How can biases in AI be addressed?
Addressing biases in AI involves several strategies, including using diverse data sets, implementing inclusive practices during development, and regularly auditing algorithms for bias. It's essential to continuously monitor AI systems to identify and rectify any unintended biases that may arise.
- What does accountability mean in the context of AI?
Accountability in AI refers to the responsibility of developers, organizations, and users for the decisions made by AI systems. It emphasizes the need for clear lines of responsibility and the importance of establishing trust between AI technologies and their users.
- Why is transparency essential in AI?
Transparency is vital in AI because it fosters trust and understanding among users and stakeholders. When AI systems clearly communicate how they function and make decisions, it allows users to make informed choices and enhances compliance with ethical standards.
- What is explainable AI?
Explainable AI (XAI) refers to AI systems designed to provide understandable justifications for their outputs. This is important for user trust, as it allows individuals to comprehend how decisions are made, thereby increasing accountability and ensuring that AI aligns with ethical principles.
- Are there existing regulations for AI ethics?
Yes, various governments and organizations have begun implementing regulatory frameworks to ensure ethical AI practices. These guidelines aim to align AI development with societal values and address concerns related to privacy, security, and fairness in AI applications.
- How can organizations implement ethical AI practices?
Organizations can implement ethical AI practices by adopting a framework that prioritizes fairness, accountability, and transparency in their AI systems. This includes conducting regular audits, engaging diverse stakeholders, and providing training on ethical considerations in AI development.