The Future of Cybersecurity: Role of AI and Machine Learning
The digital landscape is evolving at an unprecedented pace, and with it, the threats that lurk in the shadows of cyberspace are becoming increasingly sophisticated. As we dive deeper into the **future of cybersecurity**, it's essential to understand how artificial intelligence (AI) and machine learning (ML) are not just buzzwords, but pivotal players in the battle against cybercrime. Imagine a world where your data is protected by intelligent systems that learn and adapt just like a human would, but at lightning speed. This is not science fiction; it's happening right now, and it's transforming the way we approach cybersecurity.
At the heart of this transformation is the ability of AI and ML to process vast amounts of data in real-time. Cybersecurity professionals are often overwhelmed with information, and the sheer volume of data can make it challenging to detect threats before they escalate. However, with AI-powered tools, organizations can analyze patterns and anomalies with remarkable efficiency. Think of it as having a supercharged detective on your team, one that can sift through mountains of data to find the needle in the haystack before it becomes a crisis.
As we look ahead, it's crucial to recognize the emerging threats that are shaping the cybersecurity landscape. Cybercriminals are constantly adapting their strategies, employing techniques that can bypass traditional security measures. This is where AI and ML come into play, offering a proactive approach to threat detection and response. By leveraging these technologies, organizations can stay one step ahead of attackers, ensuring that they are not just reacting to threats but anticipating them.
As technology advances, so do the techniques employed by cybercriminals. Understanding these emerging threats is crucial for developing effective cybersecurity strategies that leverage AI and machine learning for enhanced protection. From ransomware attacks that encrypt your data to sophisticated phishing scams designed to steal sensitive information, the landscape is fraught with dangers.
One of the most alarming trends is the rise of **automated attacks**. Cybercriminals are using AI to automate their attacks, making them faster and more efficient. This means that organizations must be equipped with equally powerful tools to defend against these threats. The integration of AI and ML into cybersecurity not only enhances detection capabilities but also streamlines response strategies, allowing for quicker remediation of incidents.
Artificial intelligence significantly improves threat detection capabilities by analyzing vast amounts of data in real-time, identifying patterns, and recognizing anomalies that might indicate a security breach. This capability enables quicker responses, which is critical in minimizing the impact of cyber incidents. Imagine a security system that learns from every interaction, becoming smarter and more effective over time. That's the power of AI in cybersecurity.
AI systems can monitor user behavior to establish baselines, allowing them to detect deviations that may suggest malicious activity. This proactive identification of threats can prevent potential breaches before they escalate. For instance, if an employee suddenly accesses sensitive data outside of their usual scope of work, AI can flag this behavior for further investigation.
Insider threats pose a significant risk to organizations. AI-driven behavioral analysis can help identify potential insider threats by flagging unusual actions taken by employees, thereby mitigating risks before they cause damage. This is akin to having a vigilant guard who knows the routines of every employee and can spot when something is amiss.
Machine learning algorithms can be trained to recognize phishing attempts by analyzing email patterns and content. This helps organizations filter out malicious communications and protect sensitive information. With the rise of sophisticated phishing techniques, having an AI system that can learn and adapt to new threats is invaluable.
AI enhances incident response by automating repetitive tasks, allowing security teams to focus on strategic decision-making. This leads to faster containment of threats and reduced impact on organizational operations. Imagine a fire alarm system that not only alerts you to danger but also calls the fire department and starts extinguishing the fire. That's the level of efficiency AI brings to incident response.
Machine learning algorithms can analyze historical vulnerability data to predict potential weaknesses in systems. This enables organizations to prioritize patching and mitigation efforts effectively, thereby strengthening overall security. By understanding where vulnerabilities are most likely to occur, businesses can allocate resources more strategically.
By employing predictive analytics, organizations can foresee potential cyber threats and vulnerabilities. This allows for proactive measures to be taken before an attack occurs, enhancing their defensive posture. It’s like having a crystal ball that reveals the potential pitfalls before they can be exploited by attackers.
Machine learning systems continuously improve by learning from new data, ensuring that cybersecurity measures remain effective against evolving threats. This adaptability is crucial in a landscape where cyber threats are constantly changing. It’s like training for a marathon; the more you learn and adapt your strategy, the better prepared you are to face the challenges ahead.
While AI and machine learning offer significant advantages in cybersecurity, there are challenges such as data privacy concerns, the need for skilled personnel, and the potential for algorithmic bias that organizations must navigate. As with any powerful tool, it's essential to use AI responsibly and ensure that it complements human intelligence rather than replacing it.
- What is the role of AI in cybersecurity? AI helps in detecting and responding to threats faster and more efficiently by analyzing large data sets and identifying patterns.
- Can AI prevent all cyber attacks? While AI enhances security measures, it cannot guarantee complete protection. Continuous monitoring and human oversight are still essential.
- What are the main challenges in using AI for cybersecurity? Challenges include data privacy concerns, the need for skilled personnel, and potential biases in algorithms.
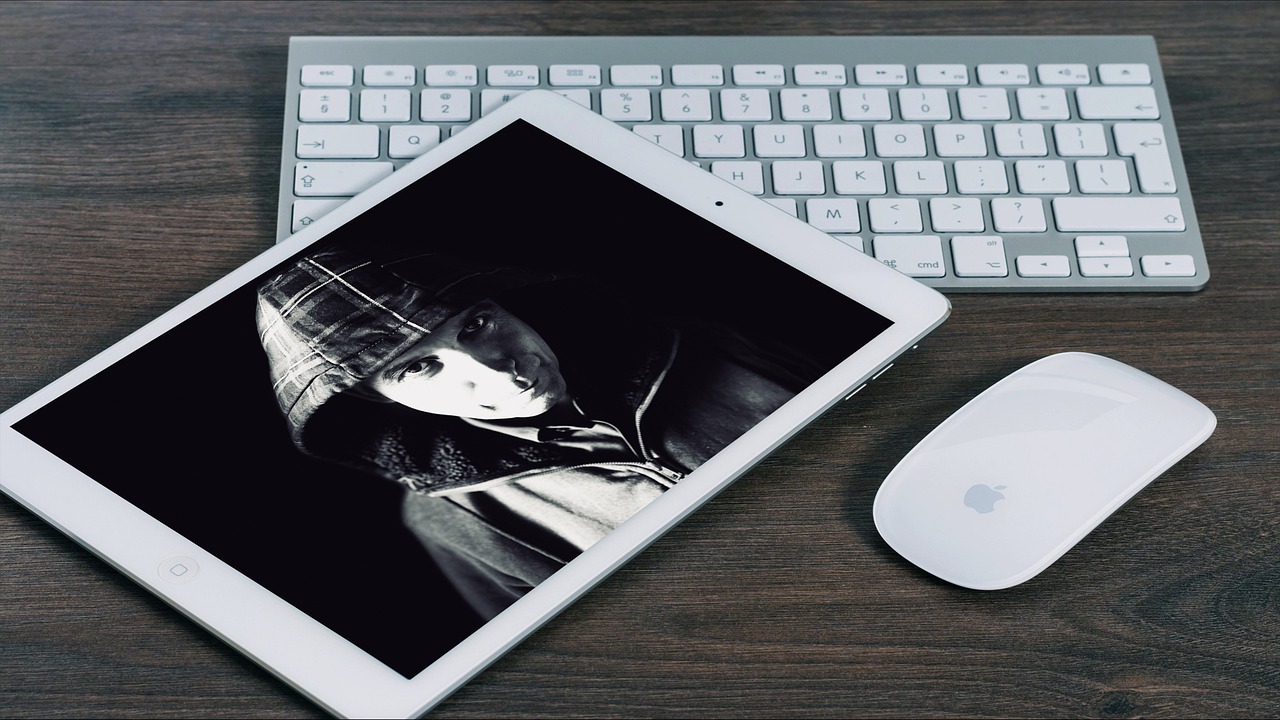
Emerging Threats in Cybersecurity
As we propel further into the digital age, the landscape of cybersecurity is rapidly evolving. New technologies and innovations bring about an array of opportunities, but they also create a playground for cybercriminals. It's like a game of cat and mouse where the stakes are higher than ever. The question is, how can organizations stay one step ahead? Understanding these emerging threats is crucial for developing effective cybersecurity strategies that leverage the power of AI and machine learning.
One of the most alarming trends is the rise of ransomware attacks. These malicious attacks encrypt an organization's data, holding it hostage until a ransom is paid. The sophistication of these attacks is increasing, with cybercriminals employing advanced tactics to bypass traditional security measures. In 2023 alone, there was a significant uptick in ransomware incidents, leading to millions in losses for businesses worldwide.
Another growing concern is the threat posed by Internet of Things (IoT) devices. As more devices connect to the internet, each one becomes a potential entry point for attackers. Imagine a smart thermostat in your home; if not properly secured, it could be hacked, giving cybercriminals access to your entire network. The sheer volume of data generated by these devices makes it challenging to monitor and secure them effectively.
Moreover, we cannot overlook the rise of social engineering attacks, where attackers manipulate individuals into divulging confidential information. These attacks often target human psychology, making them particularly dangerous. For instance, a simple phishing email can lead to devastating consequences if an employee unknowingly clicks on a malicious link. The key takeaway here is that as technology advances, so do the techniques employed by cybercriminals.
To combat these threats, organizations must adopt a proactive approach to cybersecurity. This involves not just implementing robust security measures but also fostering a culture of security awareness among employees. Training staff to recognize the signs of phishing attempts and suspicious activities can significantly reduce the risk of falling victim to these emerging threats.
In summary, the cybersecurity landscape is fraught with challenges, but by understanding the nature of these emerging threats, organizations can better prepare themselves to defend against them. It's essential to stay informed and adaptable, as the world of cyber threats is constantly changing. The battle may seem daunting, but with the right strategies and technologies in place, organizations can fortify their defenses and protect their assets.
- What are the most common emerging threats in cybersecurity? Ransomware, IoT vulnerabilities, and social engineering attacks are among the most prevalent.
- How can organizations protect themselves from these threats? By implementing robust security measures, training employees, and adopting proactive cybersecurity strategies.
- Is AI effective in combating emerging cyber threats? Yes, AI can enhance threat detection and response capabilities, making it a valuable tool in cybersecurity.
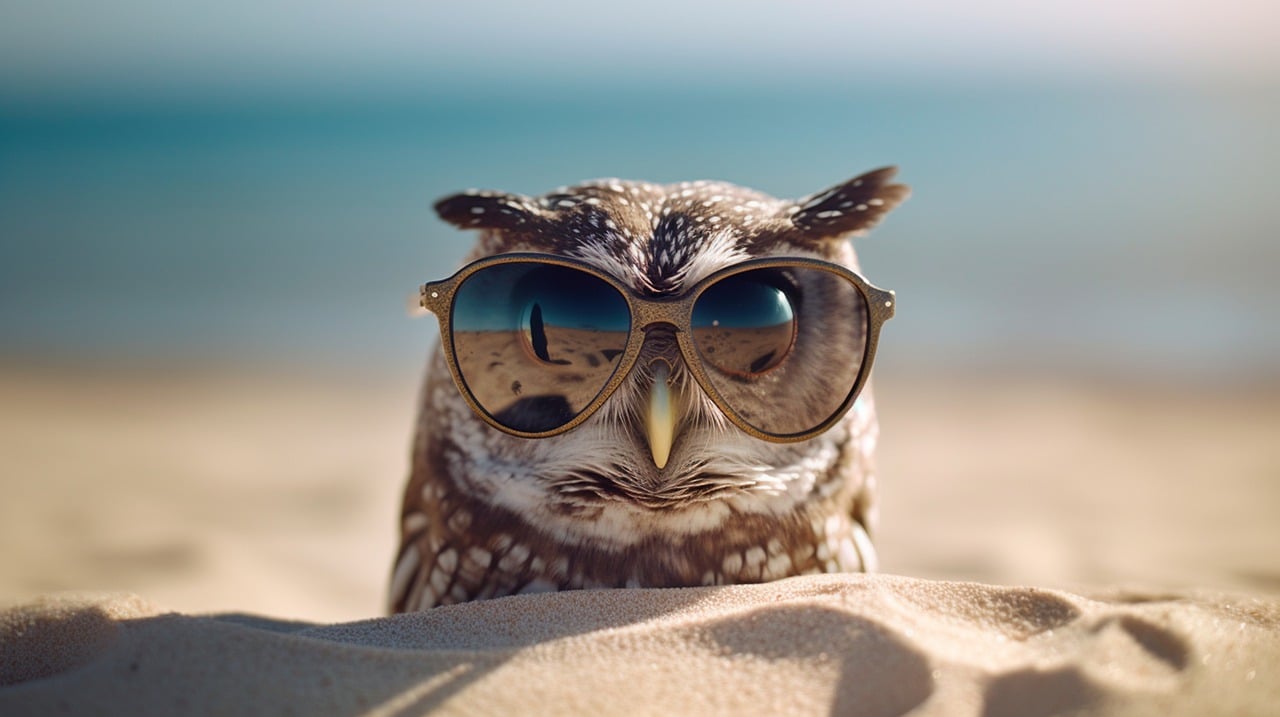
AI-Powered Threat Detection
In today's digital landscape, where data flows like a raging river and cyber threats lurk around every corner, artificial intelligence (AI) has emerged as a game-changer in the field of cybersecurity. Imagine having a vigilant security guard who never sleeps, tirelessly analyzing every piece of data that passes through your systems. That's precisely what AI-powered threat detection does! By leveraging advanced algorithms, AI can sift through vast amounts of data in real time, identifying patterns and anomalies that human analysts might miss. This capability not only enhances the speed of threat detection but also significantly improves the accuracy of identifying potential breaches.
One of the standout features of AI in threat detection is its ability to learn and adapt. Traditional security systems often rely on static rules and signatures to identify threats, which can be easily bypassed by sophisticated attacks. In contrast, AI systems utilize machine learning to continuously evolve their understanding of what constitutes normal behavior within a network. This means that as cybercriminals develop new tactics, AI systems can quickly adjust their detection mechanisms, ensuring that organizations remain one step ahead of potential threats.
Moreover, AI's prowess in behavioral analysis plays a crucial role in enhancing security measures. By establishing baselines of normal user behavior, AI can detect deviations that may indicate malicious activity. For instance, if an employee who typically accesses files during business hours suddenly logs in at midnight and downloads sensitive data, the AI system can flag this unusual behavior for further investigation. This proactive approach allows organizations to address threats before they escalate into full-blown incidents.
Another significant area where AI shines is in combating insider threats. These threats can be particularly insidious, as they often come from within the organization itself. AI-driven behavioral analysis can help identify potential insider threats by monitoring employee actions and flagging any unusual patterns. For example, if an employee who has never accessed certain confidential files suddenly starts downloading them, the system can trigger an alert, allowing security teams to investigate the situation before any damage is done.
Phishing attacks, a common tactic employed by cybercriminals, also fall victim to AI's advanced capabilities. Machine learning algorithms can be trained to recognize phishing attempts by analyzing email patterns and content. This means that organizations can filter out malicious communications, protecting sensitive information from falling into the wrong hands. With AI's ability to adapt and learn from new phishing techniques, organizations can stay ahead of the curve, significantly reducing the risk of successful phishing attacks.
In summary, AI-powered threat detection is revolutionizing the way organizations approach cybersecurity. By harnessing the power of machine learning and behavioral analysis, businesses can enhance their threat detection capabilities, respond to incidents more swiftly, and ultimately build a more resilient security posture. As technology continues to evolve, the integration of AI in cybersecurity will undoubtedly become increasingly vital in the ongoing battle against cyber threats.
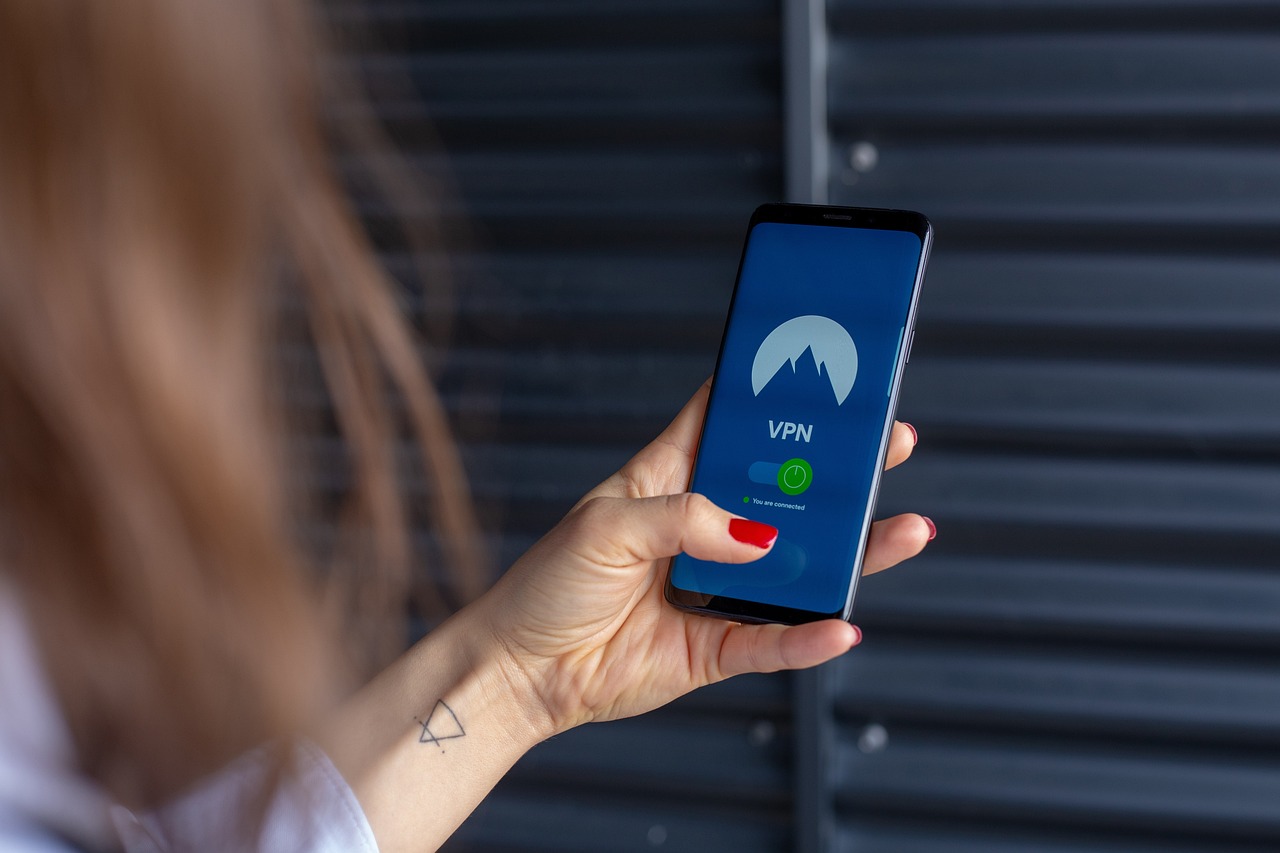
Behavioral Analysis
In the vast and ever-evolving world of cybersecurity, stands out as a powerful tool that leverages the capabilities of artificial intelligence (AI) to enhance security measures. Imagine a security system that not only reacts to threats but also anticipates them by understanding how users typically behave. This is where behavioral analysis comes into play. By establishing a baseline of normal user behavior, AI systems can detect deviations that might indicate malicious activity. For instance, if an employee who usually logs in from the office suddenly accesses sensitive data from a different country, the system can flag this as a potential security breach.
The beauty of behavioral analysis lies in its proactive approach. Instead of waiting for a breach to occur, organizations can identify potential threats before they escalate into serious incidents. This is especially crucial in today's digital landscape, where cyber threats are becoming increasingly sophisticated. By monitoring user interactions with systems, AI can create a comprehensive profile of normal behavior patterns, allowing it to spot unusual activities that could signify an attack.
Moreover, behavioral analysis is not just about detecting external threats; it also plays a vital role in addressing insider threats. These threats can be particularly challenging to manage, as they often involve trusted employees who may inadvertently or intentionally compromise security. AI-driven behavioral analysis can help organizations identify these risks by flagging unusual actions taken by employees. For example, if an employee suddenly downloads an unusually large amount of sensitive data or accesses files that are not relevant to their job role, the system can alert security teams to investigate further. This proactive identification is crucial for mitigating risks before they lead to significant damage.
To illustrate the effectiveness of behavioral analysis, consider the following table that outlines how AI systems can identify different types of anomalies in user behavior:
Type of Anomaly | Example | Potential Risk |
---|---|---|
Unusual Login Locations | Login from a foreign country | Account compromise |
Data Access Patterns | Accessing sensitive files not related to job | Insider threat |
Sudden Increase in Data Downloads | Employee downloads large volumes of data | Data exfiltration |
By employing such advanced techniques, organizations can significantly enhance their security posture. However, it's essential to note that while behavioral analysis is a powerful tool, it is not foolproof. False positives can occur, leading to unnecessary investigations and potential disruptions in workflow. Therefore, organizations must strike a balance between security and operational efficiency, ensuring that their systems are both effective and user-friendly.
In conclusion, the role of behavioral analysis in cybersecurity cannot be overstated. It allows organizations to stay one step ahead of cybercriminals by proactively identifying potential threats and addressing them before they can cause harm. As technology continues to advance, integrating behavioral analysis into cybersecurity strategies will be crucial for maintaining robust security measures in an increasingly complex digital world.
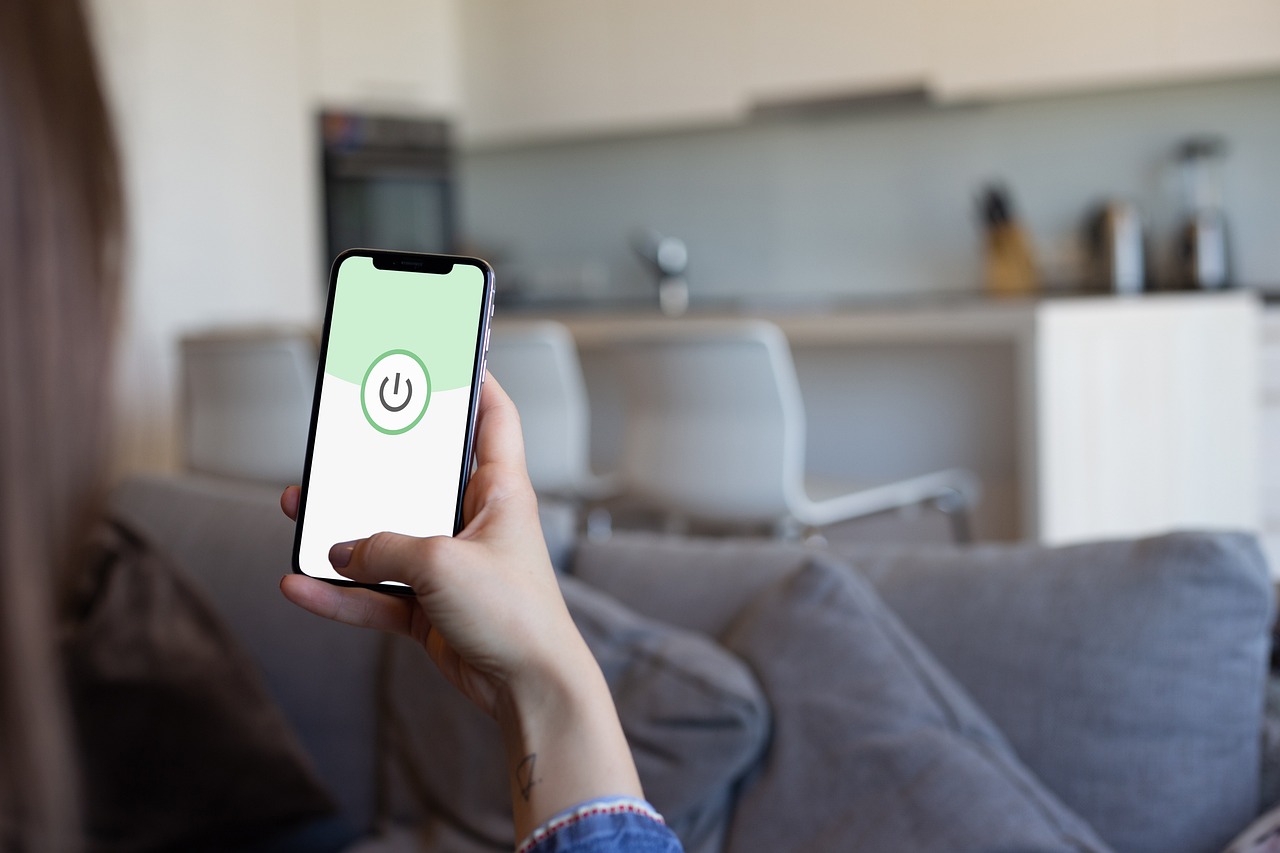
Insider Threats
Insider threats are often considered one of the most challenging aspects of cybersecurity. Unlike external attacks that come from outside an organization, insider threats originate from within. These threats can stem from employees, contractors, or any individual with access to sensitive information. The motivations behind these threats can vary widely, ranging from malicious intent to negligence. For instance, an employee might intentionally leak confidential data for personal gain, or they might inadvertently expose sensitive information through careless actions.
AI-driven behavioral analysis plays a pivotal role in identifying and mitigating these insider threats. By monitoring user activity, AI systems can establish a baseline of normal behavior for each employee. This means that if an employee suddenly accesses files they typically don’t interact with or attempts to download large amounts of data, the system can flag this as suspicious activity. This proactive approach not only helps in early detection but also reduces the potential damage caused by insider threats.
To illustrate the significance of addressing insider threats, consider the following statistics:
Type of Insider Threat | Percentage of Incidents |
---|---|
Malicious Insider | 30% |
Negligent Insider | 50% |
Compromised Insider | 20% |
This table highlights that a significant portion of insider threats are due to negligence, which underscores the importance of not only monitoring behavior but also educating employees about cybersecurity best practices. Organizations must create a culture of security awareness, where employees understand the implications of their actions and are equipped to recognize potential threats.
Furthermore, AI systems can enhance the detection of insider threats by utilizing machine learning algorithms that adapt over time. These systems learn from new data, continuously refining their ability to identify unusual patterns of behavior. For example, if an employee who typically works 9 to 5 suddenly logs in at odd hours and accesses sensitive files, the system can automatically alert security teams for further investigation.
In conclusion, addressing insider threats is crucial for maintaining robust cybersecurity. By leveraging AI and machine learning, organizations can not only detect potential threats before they escalate but also foster a proactive security culture among employees. This dual approach not only protects sensitive information but also ensures that organizations remain resilient in the face of evolving cyber threats.
- What are insider threats? Insider threats refer to security risks that originate from within an organization, typically involving employees or contractors who have access to sensitive information.
- How can AI help in detecting insider threats? AI can analyze user behavior to establish baselines and detect anomalies that may indicate malicious activity, allowing for early intervention.
- What percentage of insider threats are due to negligence? Approximately 50% of insider threats are attributed to negligent actions by employees, highlighting the need for proper training and awareness.
- Can machine learning systems adapt to new threats? Yes, machine learning systems continuously learn from new data, ensuring they remain effective against evolving insider threats.
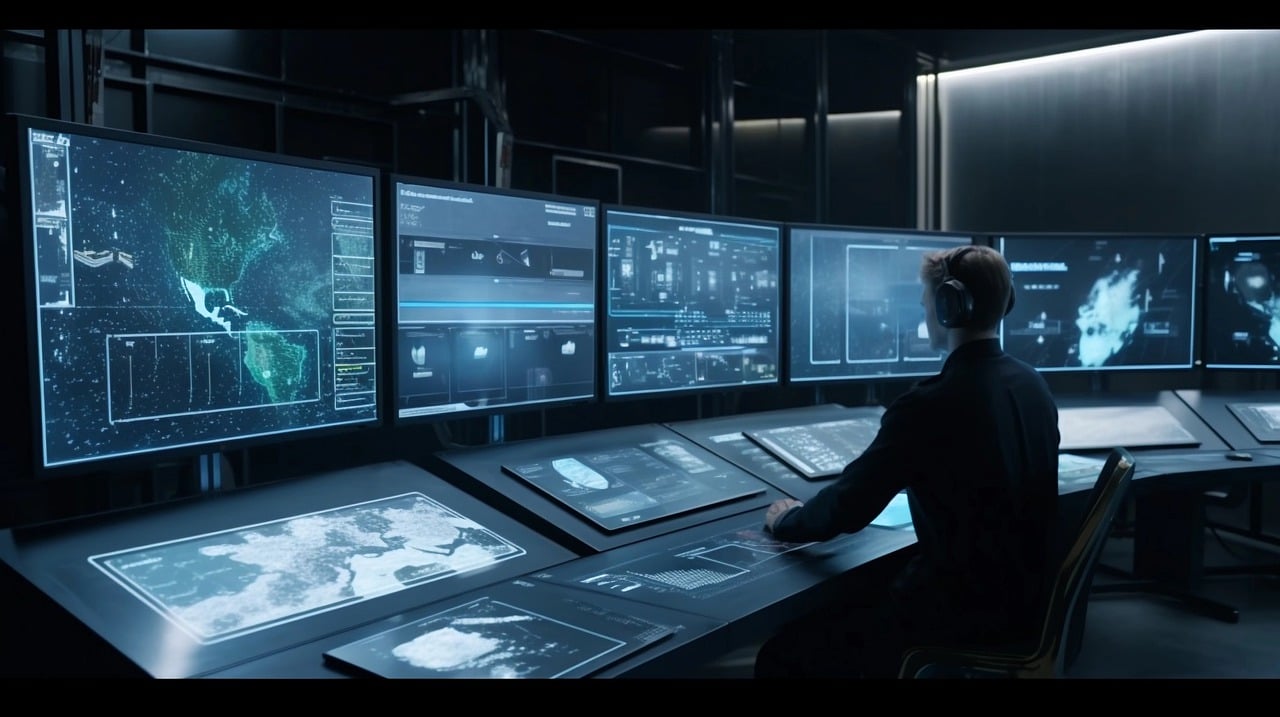
Phishing Detection
Phishing is one of the most prevalent cyber threats today, and its deceptive tactics can ensnare even the most vigilant users. Imagine receiving an email that looks like it’s from your bank, urging you to verify your account details. This is the essence of phishing—malicious actors masquerading as trustworthy entities to steal sensitive information. Thankfully, machine learning algorithms have emerged as powerful allies in the fight against these deceptive practices. By analyzing email patterns and content, these algorithms can effectively identify potential phishing attempts before they reach the unsuspecting user.
Machine learning models are trained on vast datasets that include both legitimate and phishing emails, enabling them to recognize subtle differences that may go unnoticed by the human eye. For instance, they can detect unusual sender addresses, suspicious links, and even the tone of the message. This capability not only enhances the accuracy of phishing detection but also reduces the rate of false positives, ensuring that genuine communications are not mistakenly flagged as threats.
Moreover, organizations can implement these machine learning systems to continuously improve their phishing detection mechanisms. As new phishing strategies evolve, the algorithms adapt by learning from new data inputs. This continuous learning is crucial, as it helps in keeping pace with the ever-changing landscape of cyber threats. In fact, the effectiveness of these systems can be illustrated in the following table:
Phishing Detection Method | Effectiveness | Adaptability |
---|---|---|
Rule-based filters | Moderate | Low |
Machine learning algorithms | High | High |
By employing machine learning for phishing detection, organizations can significantly enhance their security posture. However, it’s not just about having the technology; it’s also about educating employees. Users should be trained to recognize the signs of phishing attempts and understand the importance of reporting suspicious emails. This combination of technology and human vigilance creates a robust defense against phishing attacks.
In conclusion, as phishing tactics become increasingly sophisticated, the role of machine learning in detecting these threats cannot be overstated. By analyzing data patterns and learning from new threats, organizations can stay one step ahead of cybercriminals, ensuring that their sensitive information remains secure. So, the next time you receive an email that seems too good to be true, remember that AI is working tirelessly in the background to protect you from falling victim to these cunning scams.
- What is phishing? Phishing is a cyber attack that involves tricking individuals into providing sensitive information by pretending to be a trustworthy source.
- How does machine learning help in phishing detection? Machine learning analyzes email patterns and content to identify potential phishing attempts, improving detection accuracy and reducing false positives.
- Can phishing attacks evolve? Yes, phishing tactics are constantly changing, which is why continuous learning systems are essential for effective detection.
- What should I do if I receive a suspicious email? Do not click on any links or provide information. Report it to your organization's IT department or email provider.
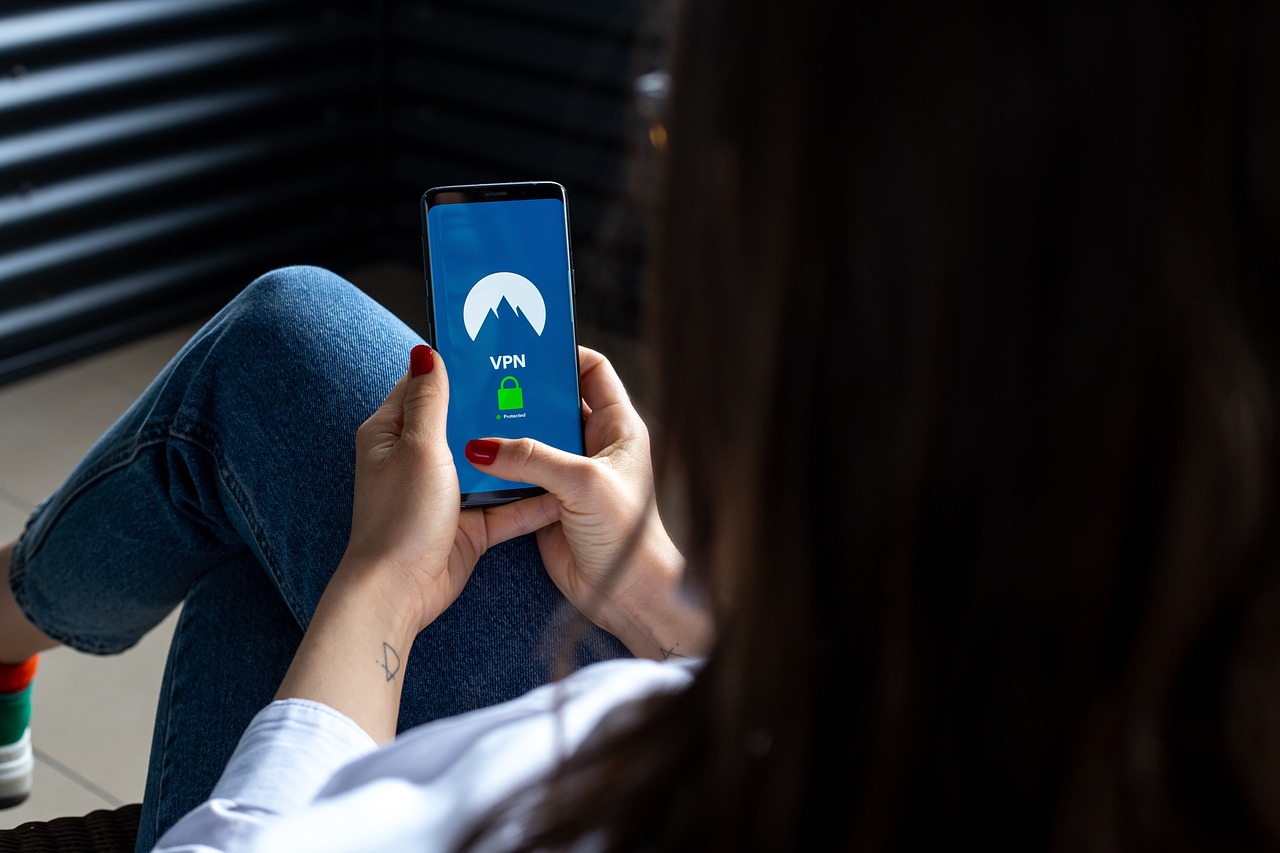
Automated Incident Response
In today's fast-paced digital landscape, the ability to respond to cyber incidents swiftly is not just an advantage; it's a necessity. That's where comes into play. Imagine a scenario where your cybersecurity team is bombarded with alerts from various systems, and each second counts. AI-driven automation swoops in like a superhero, taking charge of repetitive tasks and allowing human experts to focus on what they do best—strategic decision-making.
Automated incident response systems work by leveraging advanced algorithms that can analyze incoming data, identify threats, and initiate predefined responses without human intervention. This means that when a potential security breach is detected, the system can automatically isolate affected systems, block malicious traffic, and even initiate communication with relevant stakeholders. It's like having a digital fire extinguisher that activates the moment it senses flames.
One of the significant benefits of this technology is its ability to reduce the mean time to respond (MTTR). For organizations, this translates to less downtime and minimized damage during a cyber incident. According to a recent study, companies that implemented automated incident response saw their MTTR decrease by up to 50%, allowing them to recover from attacks more swiftly and efficiently.
Moreover, automated incident response systems can be tailored to fit the unique needs of an organization. For example, consider the following components that can be integrated into these systems:
Component | Description |
---|---|
Threat Intelligence | Integrates real-time data from various sources to identify emerging threats. |
Playbooks | Predefined actions that the system can take in response to specific threats. |
Alert Prioritization | Ranks alerts based on severity, ensuring critical issues are addressed first. |
Of course, while automated incident response offers tremendous benefits, it is not without its challenges. Organizations must ensure that their systems are configured correctly to avoid false positives, which can lead to unnecessary alarms and wasted resources. Additionally, it's essential to maintain a balance between automation and human oversight. After all, while machines can handle repetitive tasks efficiently, human intuition and creativity are irreplaceable when it comes to complex decision-making.
In conclusion, automated incident response represents a crucial evolution in the realm of cybersecurity. By streamlining processes and enhancing efficiency, organizations can not only protect their assets more effectively but also cultivate a culture of proactive security. As we continue to navigate the complexities of the digital world, embracing automation will undoubtedly be a game-changer in our fight against cyber threats.
- What is automated incident response? Automated incident response refers to the use of AI and machine learning technologies to detect, analyze, and respond to cybersecurity incidents without human intervention.
- How does automated incident response improve security? It enhances security by reducing response times, minimizing human error, and allowing security teams to focus on more strategic tasks.
- Can automated systems handle all types of cyber threats? While automated systems can manage many threats effectively, they should be complemented by human oversight to address more complex situations.
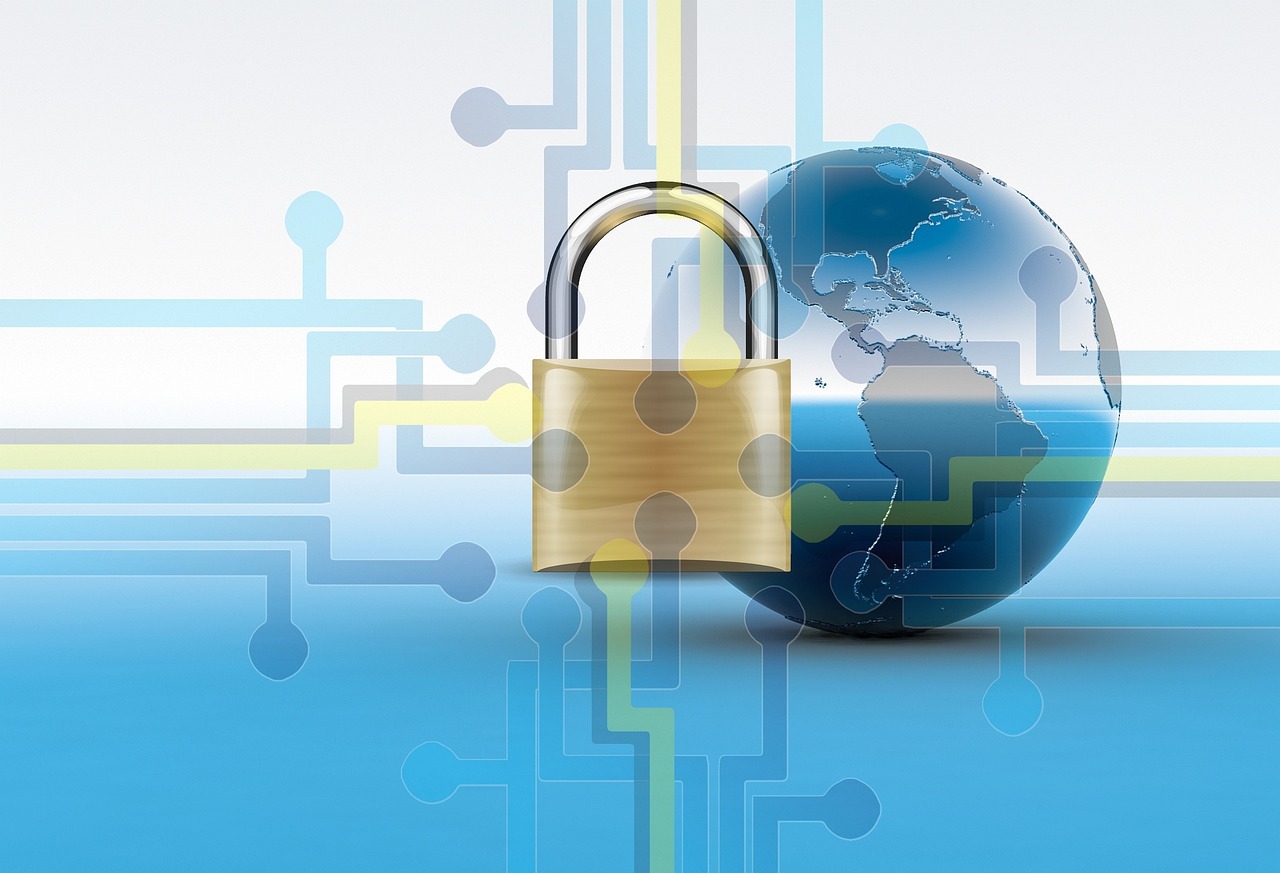
Machine Learning in Vulnerability Management
In today's fast-paced digital landscape, the concept of vulnerability management has evolved significantly, thanks in large part to the advent of machine learning technologies. Traditional methods of identifying and managing vulnerabilities often rely on manual processes that can be both time-consuming and prone to human error. However, with machine learning algorithms stepping into the spotlight, organizations can now harness the power of data to enhance their vulnerability management strategies. These algorithms are designed to analyze historical vulnerability data, which allows them to predict potential weaknesses in systems with remarkable accuracy.
Imagine trying to find a needle in a haystack; that’s what vulnerability management used to feel like. But with machine learning, it’s as if you have a magnet that not only finds the needle but also predicts where more needles might appear in the future. By analyzing patterns and trends in data, machine learning can help organizations prioritize their patching and mitigation efforts effectively. This predictive capability is essential because it allows security teams to focus their resources on the most critical vulnerabilities, thereby strengthening the overall security posture of the organization.
Furthermore, the integration of machine learning into vulnerability management isn't just about prediction; it's also about continuous improvement. Machine learning systems are designed to learn and adapt over time. As they process new data, they refine their algorithms, ensuring that the cybersecurity measures in place remain effective against evolving threats. This continuous learning cycle means that organizations can stay one step ahead of cybercriminals, who are always looking for new ways to exploit weaknesses.
To illustrate the impact of machine learning in vulnerability management, consider the following table that highlights some key benefits:
Benefit | Description |
---|---|
Proactive Threat Detection | Machine learning algorithms can predict potential vulnerabilities before they are exploited, allowing for timely intervention. |
Resource Optimization | By prioritizing vulnerabilities based on risk, organizations can allocate their resources more efficiently. |
Adaptability | Machine learning systems continuously evolve, ensuring that they remain effective against new and emerging threats. |
However, it’s important to note that while machine learning offers significant advantages, it is not a silver bullet. Organizations must still invest in foundational cybersecurity practices and maintain a skilled workforce to interpret the insights generated by these advanced technologies. The interplay between human expertise and machine learning is what ultimately leads to a robust vulnerability management strategy.
In summary, machine learning is revolutionizing the way organizations approach vulnerability management. By leveraging predictive analytics and continuous learning capabilities, businesses can not only identify and prioritize vulnerabilities more effectively but also enhance their overall security posture. As we look to the future, it's clear that the fusion of machine learning and cybersecurity will play a pivotal role in safeguarding our digital environments.
- What is vulnerability management? Vulnerability management is the process of identifying, evaluating, treating, and reporting on security vulnerabilities in systems and software.
- How does machine learning improve vulnerability management? Machine learning analyzes vast amounts of historical data to predict potential vulnerabilities, allowing organizations to prioritize their responses effectively.
- Are there any challenges in implementing machine learning for vulnerability management? Yes, challenges include data privacy concerns, the need for skilled personnel, and the possibility of algorithmic bias.
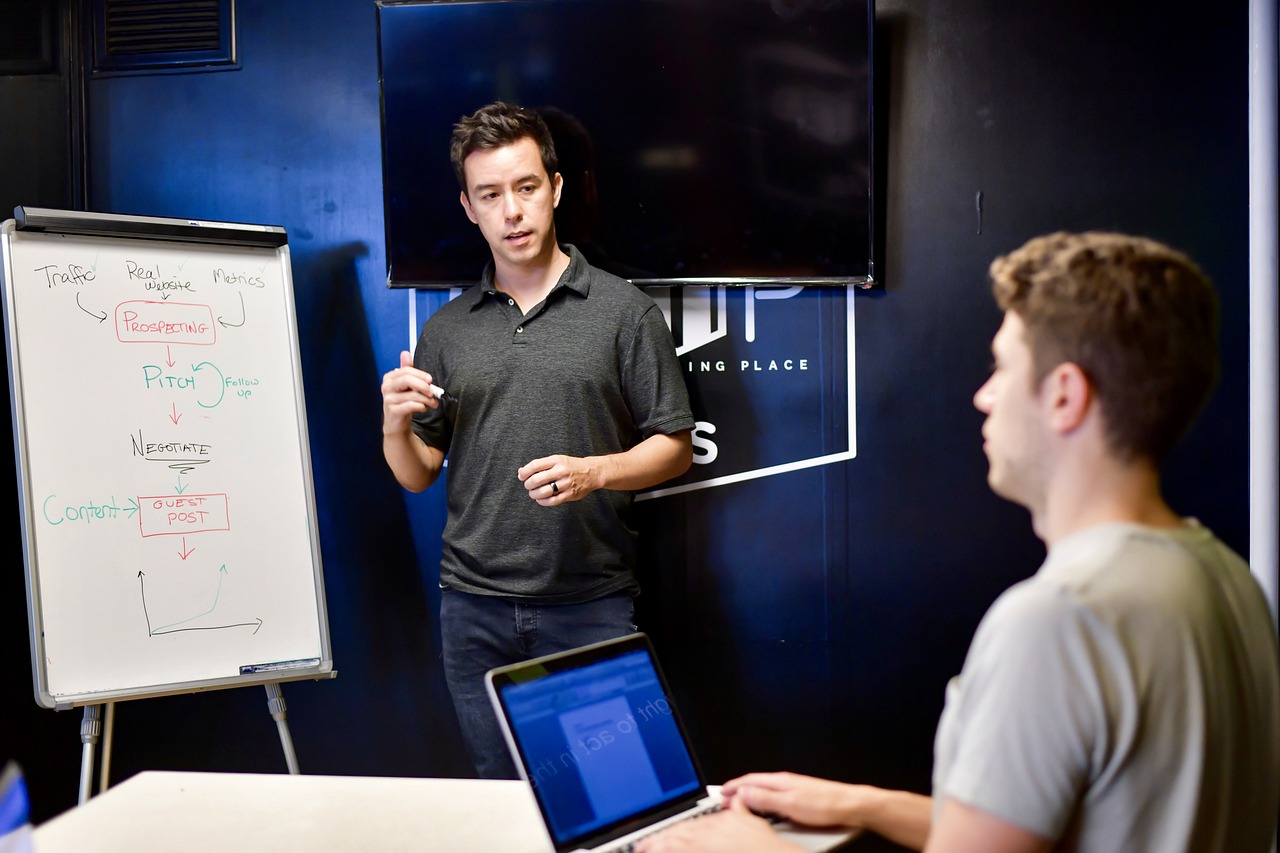
Predictive Analytics
In the ever-evolving landscape of cybersecurity, has emerged as a game-changer. Imagine having a crystal ball that allows organizations to foresee potential cyber threats and vulnerabilities before they manifest. This isn't just wishful thinking; it's the power of predictive analytics at work. By leveraging historical data and advanced algorithms, businesses can identify patterns and trends that indicate where the next attack might originate. For instance, if a particular type of malware has been prevalent in a specific sector, predictive analytics can help organizations in that sector bolster their defenses.
One of the most significant advantages of predictive analytics is its ability to enable proactive measures. Instead of waiting for an attack to occur, organizations can take steps to strengthen their defenses. This proactive approach is akin to a football team studying their opponent's plays before the game, allowing them to anticipate moves and counteract effectively. By analyzing data from past incidents, organizations can pinpoint vulnerabilities that are likely to be exploited in future attacks.
Consider the following key components of predictive analytics in cybersecurity:
- Data Collection: Gathering vast amounts of data from various sources, including network traffic, user behavior, and threat intelligence feeds.
- Pattern Recognition: Using machine learning algorithms to identify patterns that may indicate potential threats.
- Risk Assessment: Evaluating the likelihood and potential impact of identified threats on the organization.
- Actionable Insights: Providing recommendations for security measures based on predictive findings.
Moreover, organizations can enhance their security posture by integrating predictive analytics with their existing security frameworks. This integration allows for real-time monitoring and alerts, ensuring that any unusual activity is addressed immediately. For example, if predictive analytics identifies a spike in login attempts from an unusual location, security teams can investigate further before any breach occurs.
However, it's essential to recognize that predictive analytics is not a silver bullet. While it significantly enhances an organization's ability to foresee and mitigate risks, it must be part of a comprehensive cybersecurity strategy. Organizations should combine predictive analytics with other security measures, such as incident response plans, employee training, and robust firewalls, to create a layered defense against cyber threats.
In conclusion, the role of predictive analytics in cybersecurity cannot be overstated. By anticipating potential threats and vulnerabilities, organizations can stay one step ahead of cybercriminals, ensuring a more secure digital environment. As technology continues to advance, embracing predictive analytics will be crucial for organizations aiming to protect their assets and maintain trust with their customers.
- What is predictive analytics in cybersecurity?
Predictive analytics involves using historical data and algorithms to forecast potential cyber threats and vulnerabilities, allowing organizations to take proactive measures to enhance their security. - How does predictive analytics enhance cybersecurity?
By identifying patterns and trends in data, predictive analytics enables organizations to anticipate attacks, assess risks, and implement preventive measures before a breach occurs. - Can predictive analytics replace traditional cybersecurity measures?
No, predictive analytics should complement traditional cybersecurity measures. It is most effective when integrated into a comprehensive security strategy. - What types of data are used in predictive analytics?
Data sources can include network traffic logs, user behavior analytics, threat intelligence feeds, and historical incident reports.
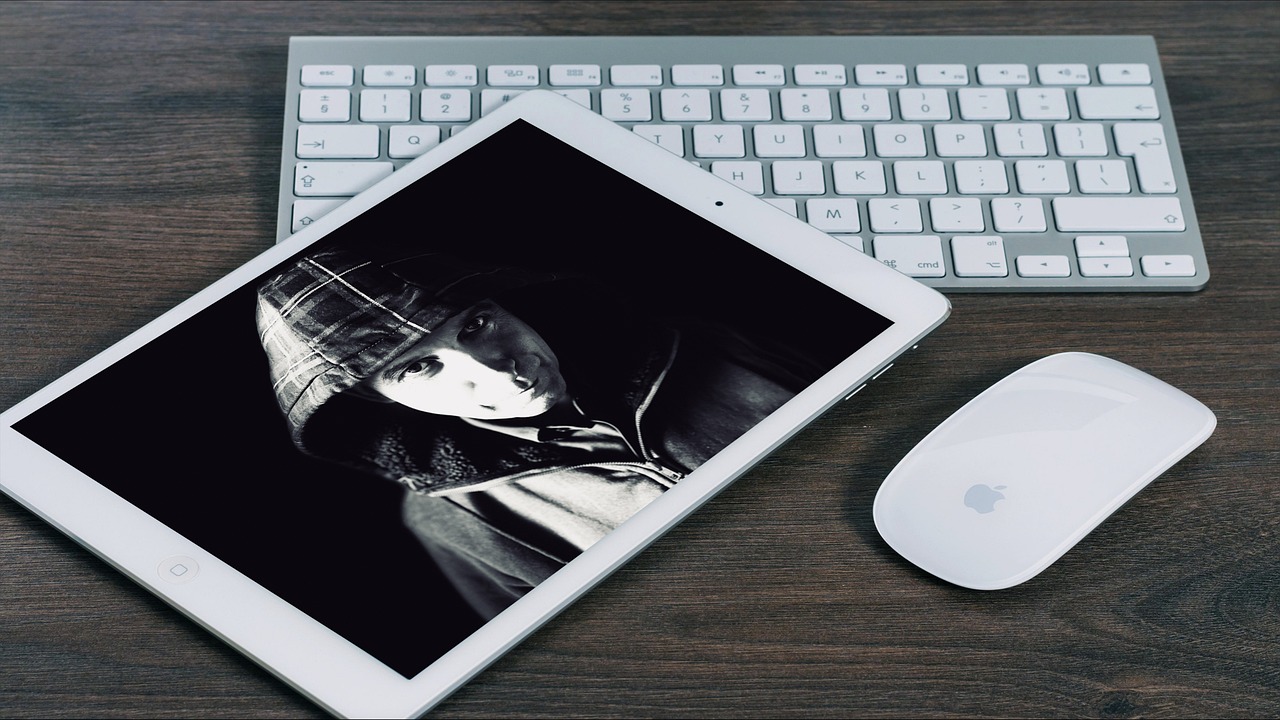
Continuous Learning Systems
This article explores how artificial intelligence and machine learning technologies are shaping the future of cybersecurity, enhancing threat detection, response strategies, and overall system resilience against evolving cyber threats.
As technology advances, so do the techniques employed by cybercriminals. Understanding these emerging threats is crucial for developing effective cybersecurity strategies that leverage AI and machine learning for enhanced protection.
Artificial intelligence significantly improves threat detection capabilities by analyzing vast amounts of data in real-time, identifying patterns, and recognizing anomalies that might indicate a security breach, thus enabling quicker responses.
AI systems can monitor user behavior to establish baselines, allowing them to detect deviations that may suggest malicious activity, enhancing security measures through proactive identification of threats before they escalate.
Insider threats pose a significant risk to organizations. AI-driven behavioral analysis can help identify potential insider threats by flagging unusual actions taken by employees, thereby mitigating risks before they cause damage.
Machine learning algorithms can be trained to recognize phishing attempts by analyzing email patterns and content, helping organizations to filter out malicious communications and protect sensitive information.
AI enhances incident response by automating repetitive tasks, allowing security teams to focus on strategic decision-making. This leads to faster containment of threats and reduced impact on organizational operations.
Machine learning algorithms can analyze historical vulnerability data to predict potential weaknesses in systems, enabling organizations to prioritize patching and mitigation efforts effectively, thereby strengthening overall security.
By employing predictive analytics, organizations can foresee potential cyber threats and vulnerabilities, allowing for proactive measures to be taken before an attack occurs, enhancing their defensive posture.
In the ever-evolving landscape of cybersecurity, represent a game-changing approach. These systems leverage the power of machine learning to constantly adapt and improve based on new data and emerging threats. Imagine a cybersecurity system that learns from every interaction, every attempted breach, and every piece of malware. It’s like having a digital immune system that doesn’t just react but evolves to defend against new viruses. This capability is essential because cyber threats are not static; they change and adapt, often faster than traditional security measures can respond.
Continuous learning systems utilize algorithms that can analyze vast amounts of data, identifying patterns and anomalies over time. This process enables them to predict potential threats before they become critical issues. For instance, if a new type of malware is detected in a specific region, a continuous learning system can quickly analyze its behavior and share this information across all systems, enhancing overall security. This proactive approach is vital because it allows organizations to stay one step ahead of cybercriminals, adapting their defenses in real-time.
Moreover, these systems are designed to learn from their mistakes. If a particular response to a threat fails, the system analyzes what went wrong and adjusts its algorithms accordingly. This feedback loop not only strengthens the system but also fosters a culture of continuous improvement within the organization. In essence, it’s about creating a dynamic cybersecurity environment where learning and adaptation are part of the core strategy.
To illustrate the effectiveness of continuous learning systems, consider the following table, which highlights the key benefits:
Benefit | Description |
---|---|
Real-time Adaptation | Systems can adjust their defenses as new threats emerge, ensuring ongoing protection. |
Enhanced Accuracy | Continuous learning minimizes false positives by refining threat detection algorithms. |
Proactive Defense | Organizations can anticipate and mitigate risks before they escalate into serious threats. |
Resource Efficiency | Automated learning reduces the need for constant human intervention, freeing up security teams. |
In conclusion, continuous learning systems are not just a trend; they are a necessity in the fight against cyber threats. By embracing these technologies, organizations can create a more resilient cybersecurity posture, better equipped to handle the challenges of tomorrow.
While AI and machine learning offer significant advantages in cybersecurity, there are challenges such as data privacy concerns, the need for skilled personnel, and the potential for algorithmic bias that organizations must navigate.
- What is the role of AI in cybersecurity? AI plays a crucial role in enhancing threat detection, automating responses, and continuously learning from new threats to improve security measures.
- How does machine learning improve threat detection? Machine learning analyzes vast amounts of data to identify patterns and anomalies, enabling quicker detection of potential threats.
- What are continuous learning systems? These systems adapt and improve over time by learning from new data and past experiences, making them essential for effective cybersecurity.
- What challenges do organizations face when implementing AI? Organizations may encounter data privacy issues, a shortage of skilled professionals, and the risk of algorithmic bias.
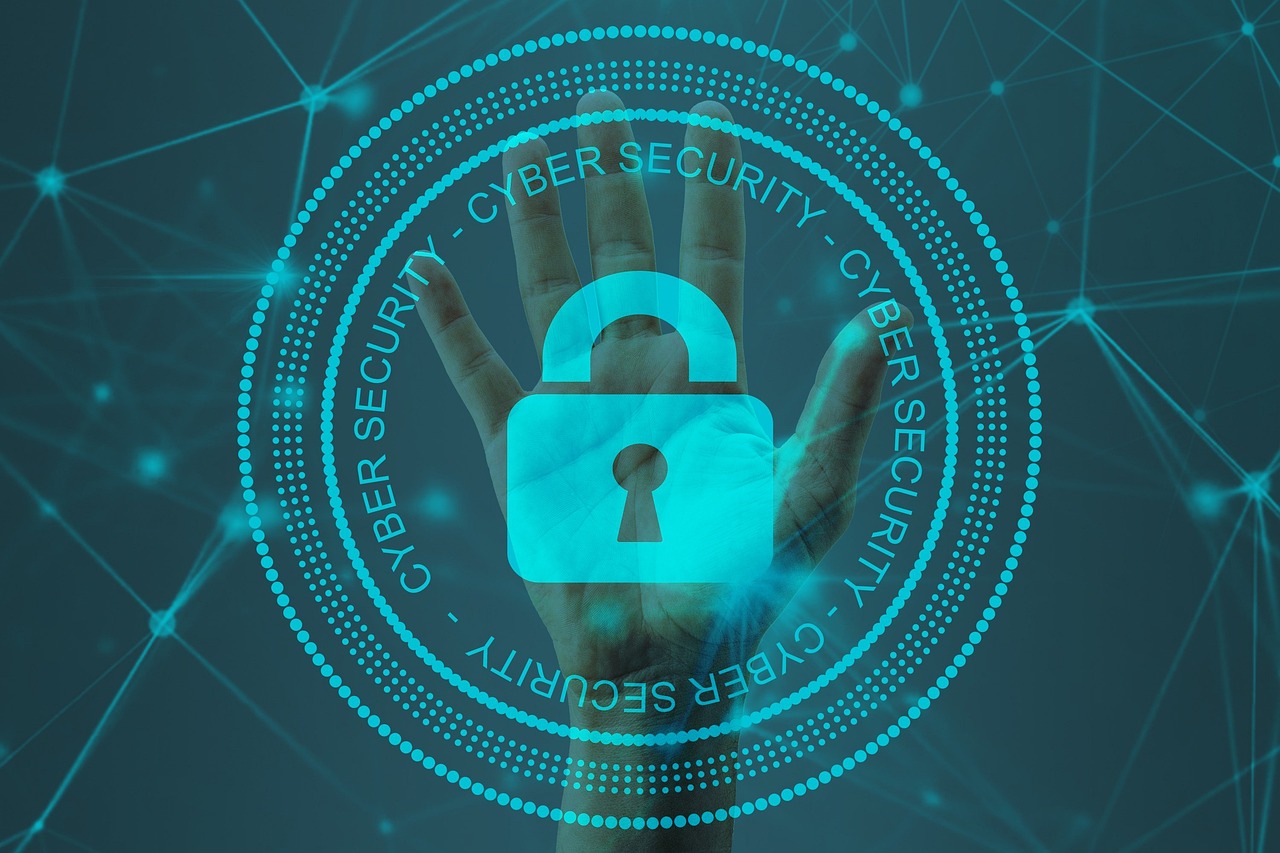
Challenges in Implementing AI
While the integration of artificial intelligence (AI) and machine learning into cybersecurity presents exciting opportunities, it also comes with its fair share of challenges. One of the most pressing issues organizations face is data privacy concerns. As AI systems require access to vast amounts of data to learn and make decisions, sensitive information can be inadvertently exposed or misused. This raises significant ethical questions and legal implications, especially with regulations like GDPR in place. Organizations must navigate these waters carefully to ensure compliance while reaping the benefits of AI.
Another challenge is the need for skilled personnel. The demand for cybersecurity professionals who are well-versed in AI and machine learning technologies is skyrocketing. However, there is a notable shortage of talent in this field. Organizations often struggle to find individuals who possess both cybersecurity expertise and the technical know-how to implement AI solutions effectively. This gap can lead to delays in deployment and ultimately hinder the effectiveness of AI-driven security measures.
Additionally, there is the potential for algorithmic bias. AI systems learn from historical data, which means that if the data used to train these systems is biased, the resulting AI can perpetuate or even amplify these biases. For instance, if an AI system is trained on data that underrepresents certain groups, it may fail to detect threats effectively within those demographics. This not only poses a risk to the security of those individuals but can also lead to reputational damage for organizations that rely on such biased systems.
Moreover, the complexity of AI systems can create challenges in implementation. Integrating AI into existing cybersecurity frameworks requires significant changes to infrastructure and processes. Organizations may need to invest heavily in new technologies and training to ensure that their staff can effectively work with these advanced systems. This can be a daunting task, especially for smaller businesses with limited resources.
Finally, the cost of implementation can be a significant barrier. While AI can ultimately save organizations money through improved efficiency and threat mitigation, the initial investment in technology, training, and ongoing maintenance can be substantial. Companies must weigh these costs against the potential benefits and determine whether they are ready to make such an investment.
In summary, while AI and machine learning hold the promise of revolutionizing cybersecurity, organizations must be prepared to face several challenges. From data privacy and the need for skilled personnel to algorithmic bias and implementation costs, navigating these hurdles will be crucial for successfully leveraging AI in the fight against cyber threats.
- What are the main challenges in implementing AI in cybersecurity?
Data privacy concerns, the need for skilled personnel, algorithmic bias, complexity of systems, and implementation costs are the primary challenges. - How can organizations address data privacy concerns?
Organizations should implement strict data governance policies and ensure compliance with regulations like GDPR to protect sensitive information. - Why is there a shortage of skilled personnel in AI and cybersecurity?
The rapid growth of technology has outpaced the availability of trained professionals, leading to a talent gap in the industry. - What is algorithmic bias, and why is it a concern?
Algorithmic bias occurs when AI systems reflect the biases present in their training data, potentially leading to unfair or ineffective outcomes.
Frequently Asked Questions
- What is the role of AI in cybersecurity?
AI plays a crucial role in cybersecurity by enhancing threat detection and response strategies. It analyzes large volumes of data in real-time, identifying patterns and anomalies that could indicate a security breach, allowing organizations to respond more quickly to potential threats.
- How does machine learning improve vulnerability management?
Machine learning enhances vulnerability management by analyzing historical data to predict potential weaknesses in systems. This allows organizations to prioritize their patching efforts and strengthen their security posture effectively.
- What are insider threats, and how can AI help detect them?
Insider threats arise from individuals within an organization who may misuse their access to cause harm. AI can help detect these threats by monitoring user behavior and flagging any unusual actions that deviate from established baselines, allowing for proactive risk mitigation.
- Can AI help in detecting phishing attempts?
Absolutely! Machine learning algorithms can be trained to recognize phishing attempts by analyzing email patterns and content. This capability helps organizations filter out malicious communications, protecting sensitive information from cybercriminals.
- What challenges do organizations face when implementing AI in cybersecurity?
Organizations may encounter several challenges when implementing AI in cybersecurity, including data privacy concerns, the need for skilled personnel to manage AI systems, and the potential for algorithmic bias that could lead to ineffective security measures.
- How does continuous learning in machine learning systems benefit cybersecurity?
Continuous learning allows machine learning systems to adapt and improve over time by learning from new data. This ensures that cybersecurity measures remain effective against evolving threats and can respond to new attack vectors in real-time.
- What is predictive analytics in the context of cybersecurity?
Predictive analytics involves using historical data to foresee potential cyber threats and vulnerabilities. By employing this approach, organizations can take proactive measures to enhance their defenses before an attack occurs, significantly improving their security posture.