Decoding Future of Financial Market with AI
The financial market is undergoing a seismic shift, and at the heart of this transformation lies artificial intelligence (AI). As we plunge deeper into the 21st century, AI is not just a buzzword; it's a game-changer that is reshaping how we think about investing, trading, and managing financial risks. Imagine a world where machines can analyze market trends faster than any human could ever dream of, making decisions based on data that is constantly being updated in real time. That world is not far off—it’s already here!
AI's impact on finance can be likened to the introduction of the internet in the 1990s. Just as the internet revolutionized communication and commerce, AI is set to redefine the financial landscape. With its ability to process vast amounts of data quickly and efficiently, AI is enhancing decision-making processes, improving risk assessments, and streamlining operations. But what does this mean for investors and financial institutions? Are they ready to embrace this technological revolution, or will they be left behind in the dust?
As we explore the potential of AI in finance, it’s essential to understand the driving forces behind its adoption. Factors such as the increasing volume of data, the demand for faster decision-making, and the need for improved accuracy are pushing financial institutions to integrate AI into their operations. The stakes are high, and those who harness the power of AI stand to gain a significant competitive edge. However, with great power comes great responsibility, and the challenges that accompany AI integration cannot be overlooked.
From algorithmic trading to risk management innovations, the applications of AI in finance are as diverse as they are impactful. For instance, algorithmic trading is revolutionizing how trades are executed, allowing for optimal pricing and minimal human error. High-frequency trading takes this a step further, leveraging AI to make lightning-fast trades based on minute market fluctuations. But while these technologies offer incredible advantages, they also introduce new risks that must be carefully navigated.
As we stand on the cusp of this new era, it’s crucial for investors and institutions alike to stay informed and adaptable. The future of finance is not merely about adopting new technologies; it’s about understanding the implications of those technologies and preparing for a landscape that is constantly evolving. So, are you ready to decode the future of the financial market with AI? The journey has just begun!
- What is AI's role in the financial market? AI plays a critical role in improving decision-making, risk assessment, and operational efficiency in finance.
- How does algorithmic trading work? Algorithmic trading uses AI to execute trades at optimal prices, minimizing human error.
- What are the risks associated with AI in finance? Risks include data privacy concerns, regulatory compliance challenges, and ethical considerations.
- What does the future hold for AI in finance? The future is likely to see more advanced applications of AI, reshaping the financial landscape further.
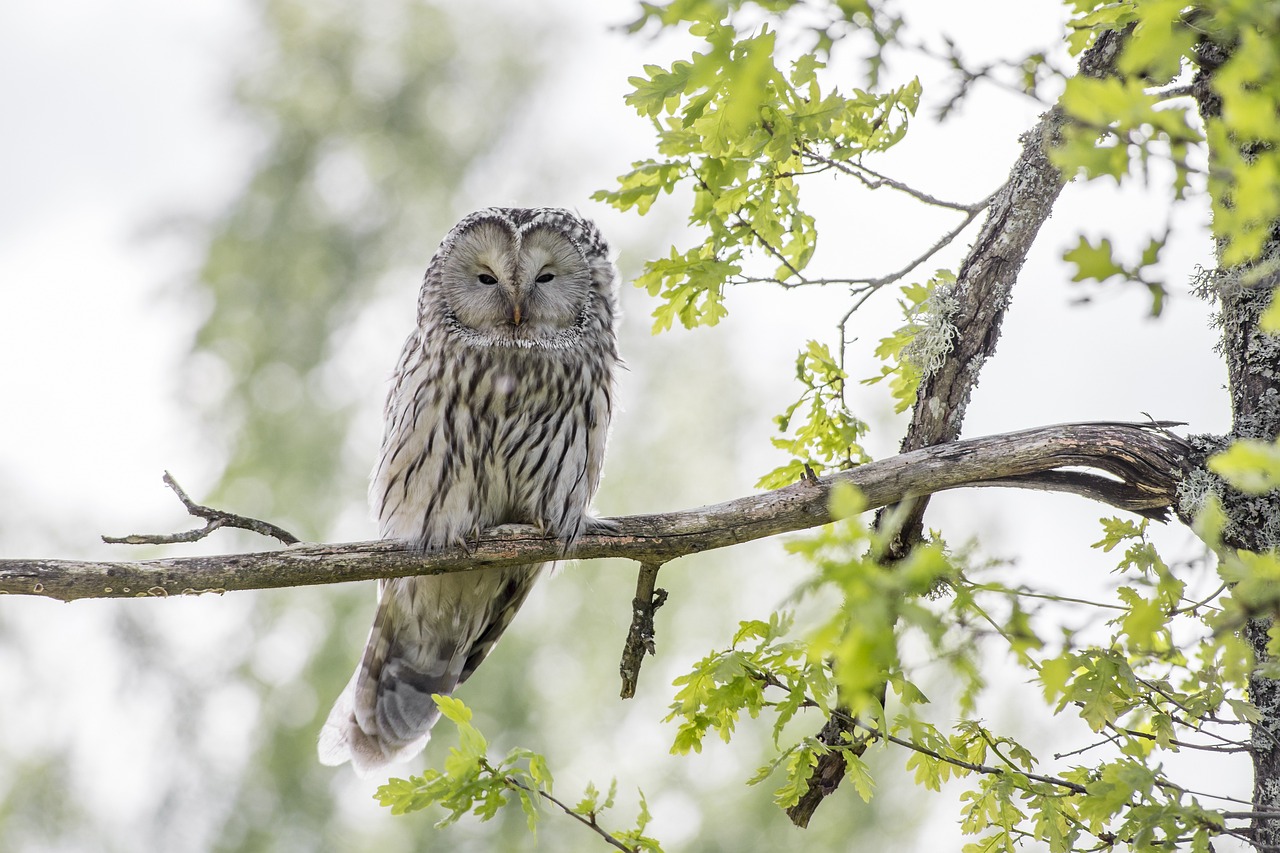
The Rise of AI in Finance
Artificial intelligence is not just a buzzword anymore; it's a game-changer in the financial sector. Over the last few years, we have witnessed a dramatic shift in how financial institutions operate, thanks to the integration of AI technologies. Imagine a world where decisions are made faster than a blink of an eye, where risks are assessed with pinpoint accuracy, and where operational efficiency is at an all-time high. This is the reality that AI is creating in the finance industry.
So, what’s driving this revolution? Several factors are at play here. First and foremost, the sheer volume of data generated in the financial sector is staggering. Every second, countless transactions occur, producing a wealth of information that traditional methods struggle to analyze effectively. AI, with its ability to process and analyze vast datasets, is stepping in to fill this gap. It allows financial analysts to make informed decisions based on real-time data rather than relying on outdated reports or gut feelings.
Another key driver is the increasing demand for personalized financial services. Customers today expect tailored solutions that fit their unique needs. AI enables financial institutions to create personalized investment strategies and risk profiles, enhancing customer satisfaction. By analyzing individual behaviors and preferences, AI can suggest products and services that are most likely to resonate with clients, making the financial experience more engaging and relevant.
Moreover, the competitive landscape is changing. With fintech startups emerging and challenging traditional banks, established institutions are feeling the pressure to innovate. AI not only helps in enhancing customer experience but also improves operational efficiency. For instance, AI-powered chatbots are now common in customer service, providing instant responses and freeing up human agents to handle more complex queries.
Additionally, the regulatory environment is becoming more favorable towards AI adoption. Governments and regulatory bodies are recognizing the potential of AI in improving compliance and risk management. This shift is encouraging more financial institutions to invest in AI technologies, further accelerating its rise in finance.
In summary, the rise of AI in finance is driven by the need for data analysis, personalized services, competitive pressures, and a supportive regulatory environment. As we delve deeper into this transformative journey, it's essential to understand the specific applications of AI in trading, risk management, and beyond. The future is bright, but it comes with its own set of challenges that we must navigate carefully.
- What is AI's role in finance? AI enhances decision-making, improves risk assessment, and increases operational efficiency.
- How does AI improve customer service in finance? Through chatbots and personalized services that cater to individual client needs.
- What challenges does AI face in the financial sector? Data privacy concerns, regulatory compliance, and ethical considerations are significant challenges.
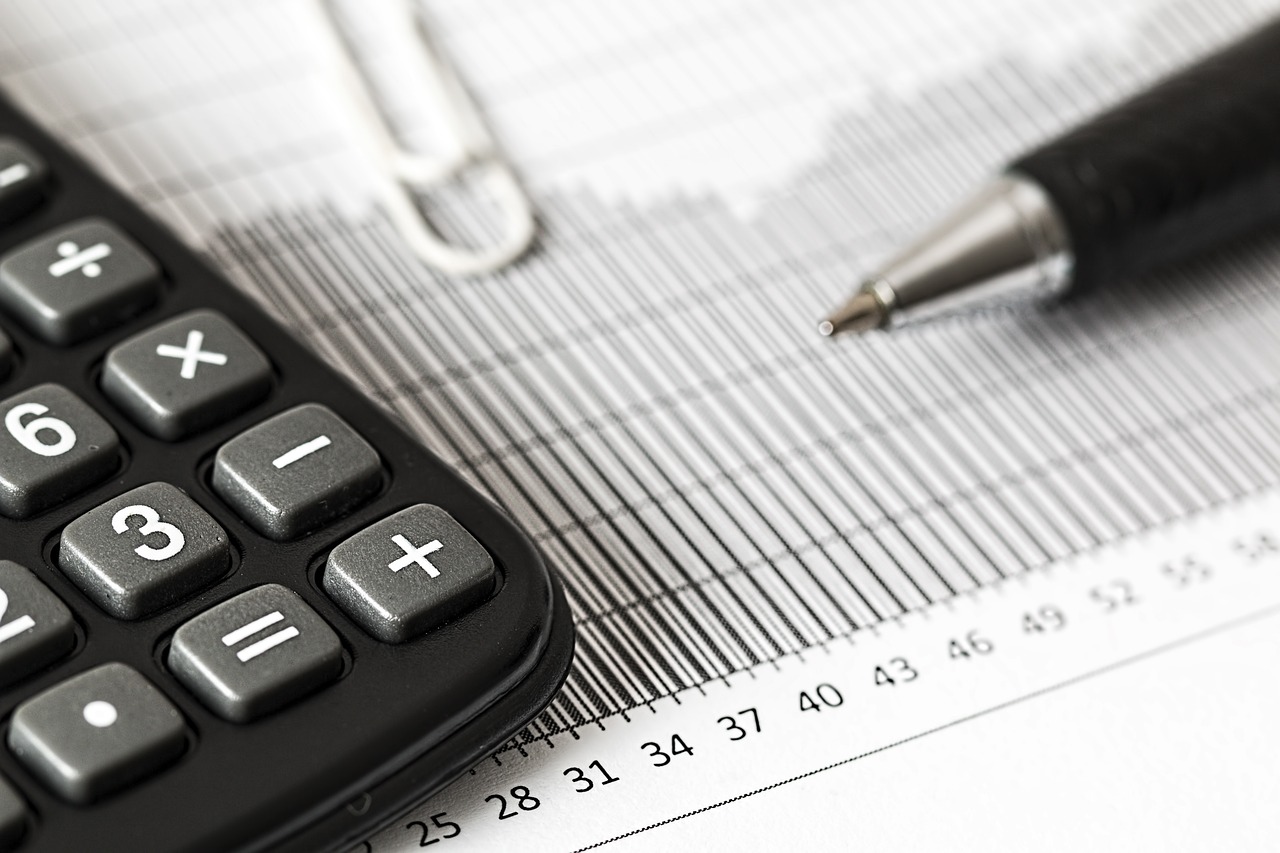
AI Applications in Trading
Artificial Intelligence (AI) is not just a buzzword; it's a game-changer in the world of trading. The financial markets are notoriously volatile, and the stakes are high. With AI technologies, traders can now analyze vast datasets at lightning speed, identify patterns, and predict market trends with unprecedented accuracy. Imagine having a super-smart assistant that can sift through mountains of data, pinpointing opportunities that a human might miss. This is the power of AI in trading, and it’s reshaping the landscape for both institutional investors and retail traders alike.
One of the most significant ways AI is making waves in trading is through algorithmic trading. This method employs complex algorithms to execute trades at optimal prices, all while minimizing human error. Think of it as a finely tuned machine that operates on logic and data rather than emotions. Such strategies can analyze market conditions in real-time, allowing for decisions that are not just reactive but proactive. The benefits of algorithm-driven trading are manifold, including increased efficiency, reduced costs, and the ability to capitalize on fleeting market opportunities.
Algorithmic trading has transformed the way trades are executed. Traditional trading often relies on human intuition and experience, which can be clouded by emotions. In contrast, algorithmic trading uses mathematical models and formulas to determine the best times to buy or sell assets. This method can process vast amounts of information, including historical data, market conditions, and even news events, to make informed decisions. The result? A trading strategy that is not only faster but also more reliable.
Within the realm of algorithmic trading lies high-frequency trading (HFT). This approach leverages AI to make rapid trades based on market fluctuations, often executing thousands of orders in a matter of seconds. HFT firms utilize advanced algorithms to analyze multiple markets and execute orders at lightning speed, capitalizing on minute price discrepancies. However, while the advantages of HFT are clear—such as increased liquidity and tighter spreads—there are also potential risks. These include market manipulation and the possibility of exacerbating market volatility during times of stress.
Another exciting application of AI in trading is sentiment analysis. This technique involves using AI to gauge market sentiment by analyzing news articles, social media posts, and other public opinions. By understanding how the market feels about a particular asset or economic event, traders can make more informed decisions. For instance, if AI detects a surge in negative sentiment around a stock due to a scandal, traders can act quickly to mitigate losses. This ability to read the market's mood adds a layer of depth to trading strategies that was previously unattainable.
To sum it up, AI applications in trading are revolutionizing how decisions are made in the financial markets. With tools like algorithmic trading, high-frequency trading, and sentiment analysis, traders can better navigate the complexities of the market. The future of trading is undoubtedly intertwined with the advancements in AI, making it an exciting time for investors and institutions alike.
- What is algorithmic trading? Algorithmic trading uses computer algorithms to execute trades based on predefined criteria, enhancing speed and accuracy.
- How does AI improve trading strategies? AI analyzes large datasets and identifies trends, enabling traders to make data-driven decisions quickly.
- What are the risks associated with high-frequency trading? Risks include market manipulation and increased volatility, particularly in turbulent market conditions.
- How does sentiment analysis work? Sentiment analysis uses AI to evaluate public sentiment from various sources, helping traders gauge market emotions.
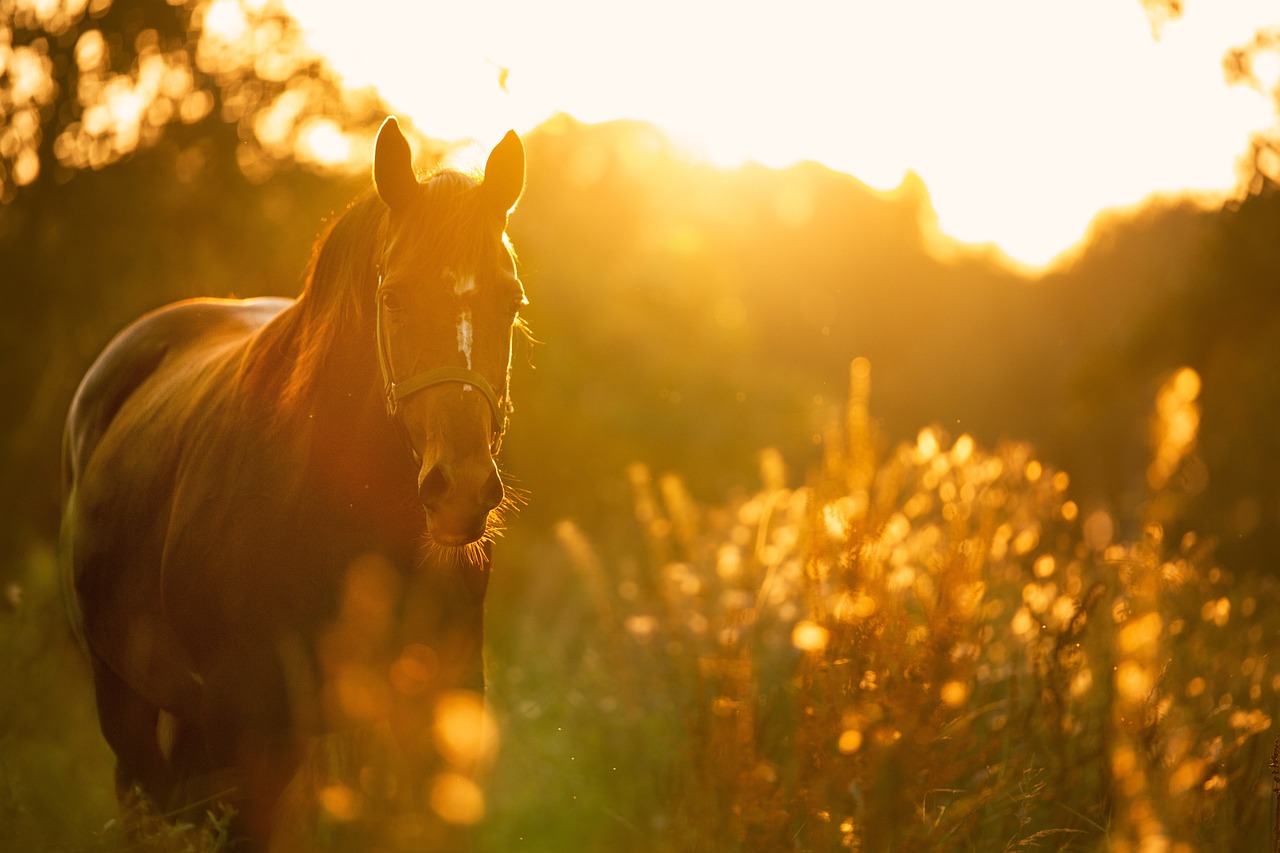
Algorithmic Trading
Algorithmic trading is like having a personal assistant who never sleeps, tirelessly analyzing market data to execute trades at lightning speed. By leveraging the power of artificial intelligence and sophisticated algorithms, traders can make decisions based on a multitude of factors that would be impossible for a human to process in real-time. Imagine trying to read thousands of financial reports, news articles, and social media posts all at once; that’s the kind of heavy lifting algorithmic trading is designed to handle.
At its core, algorithmic trading involves using computer programs to follow a defined set of instructions for placing trades. These instructions can be based on timing, price, quantity, or any mathematical model that traders believe will yield a profitable outcome. The beauty of this approach is that it minimizes human error and emotional decision-making, which are often the culprits behind poor trading outcomes. But how does it really work? Let’s break it down:
- Data Analysis: Algorithms analyze vast amounts of historical data to identify patterns and trends. This analysis can include everything from stock prices to economic indicators, ensuring that traders have a comprehensive view of the market.
- Execution: Once a trading opportunity is identified, algorithms execute trades at optimal prices, often in milliseconds. This speed can make a significant difference in the competitive world of trading.
- Backtesting: Before deploying an algorithm in the real market, traders can backtest it against historical data to see how it would have performed. This helps to refine the strategy and reduce risk.
The benefits of algorithmic trading are numerous. For one, it allows for high-frequency trading (HFT), where thousands of trades can be executed in a fraction of a second. This strategy is particularly popular among institutional investors who can capitalize on small price movements that occur in the market. Moreover, algorithmic trading can enhance market liquidity, making it easier for buyers and sellers to execute trades without significantly impacting prices.
However, it’s not all sunshine and rainbows. Algorithmic trading also comes with its own set of challenges. For instance, the reliance on technology means that any glitch or failure in the system can lead to catastrophic losses. Just think of the infamous "Flash Crash" of 2010, where the Dow Jones Industrial Average plummeted by over 1,000 points in minutes due to algorithmic trading errors. Furthermore, the competitive nature of algorithmic trading can lead to a race to the bottom, where firms invest heavily in technology to gain an edge, driving up costs and potentially leading to market instability.
In conclusion, algorithmic trading represents a remarkable intersection of technology and finance, offering unprecedented opportunities for efficiency and profitability. As we continue to embrace AI in trading, it’s essential for traders and institutions to remain vigilant about the associated risks while harnessing the potential of these powerful tools. After all, in the world of finance, it’s not just about having the best tools; it’s about knowing how to use them wisely.
- What is algorithmic trading? Algorithmic trading uses computer algorithms to automate trading decisions based on predefined criteria.
- How does algorithmic trading reduce human error? By relying on data-driven algorithms, it minimizes emotional decision-making and human mistakes.
- What are the risks associated with algorithmic trading? Risks include technical failures, market volatility, and potential regulatory scrutiny.
- Can individual investors use algorithmic trading? Yes, many platforms offer algorithmic trading tools for individual investors, though they may not have the same capabilities as institutional systems.
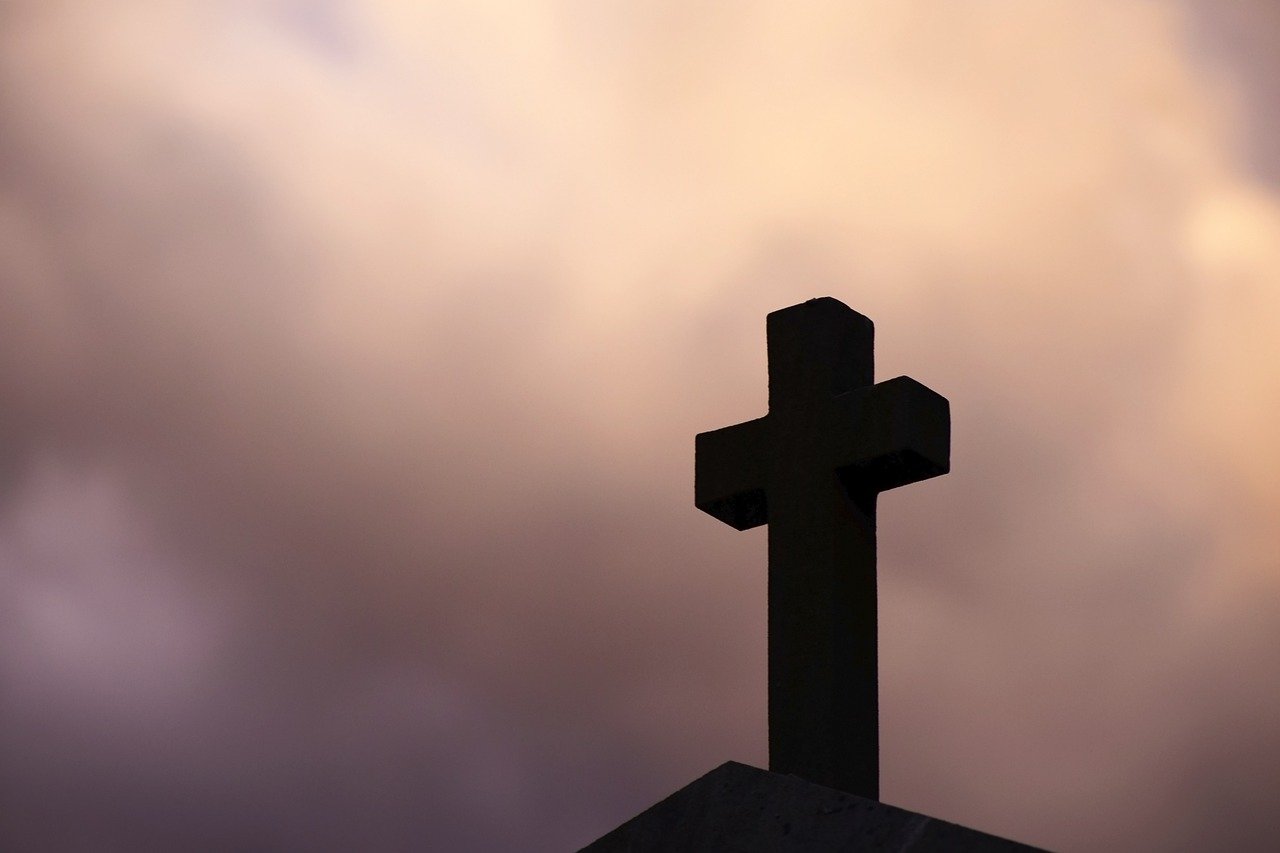
High-Frequency Trading
High-frequency trading (HFT) is like the Olympic sprint of the financial world, where milliseconds can mean the difference between profit and loss. This cutting-edge trading strategy leverages advanced algorithms and powerful computing to make a multitude of trades at incredibly high speeds. Imagine a sprinter who can not only run faster than anyone else but also has the ability to predict the finish line's position based on the slightest changes in the air. That's essentially what HFT does in the stock market; it capitalizes on tiny price discrepancies that exist for only a fleeting moment.
One of the key advantages of high-frequency trading is its ability to process vast amounts of data in real-time. Traders use algorithms that can analyze market conditions, execute trades, and even adjust strategies within fractions of a second. This level of speed and efficiency allows HFT firms to take advantage of market inefficiencies that traditional traders might miss. For instance, if a stock's price is slightly lower on one exchange compared to another, an HFT algorithm can quickly buy the stock on the cheaper exchange and sell it on the more expensive one, pocketing the difference before the opportunity disappears.
However, while HFT can be incredibly lucrative, it also comes with its own set of risks. The very speed that makes it so appealing can also lead to significant losses if the algorithms misinterpret data or if market conditions change unexpectedly. Just like a sprinter can trip and fall, an HFT strategy can falter if not carefully monitored and adjusted. Moreover, the reliance on technology means that any technical glitch can result in catastrophic consequences, as seen in the infamous Flash Crash of 2010, where the market plunged dramatically in a matter of minutes due to automated trading errors.
Additionally, the competitive nature of HFT has led to a sort of arms race among firms. Each company is constantly trying to outpace the others by developing faster algorithms and investing in better technology. This race can lead to increased operational costs, which may eventually squeeze out smaller players who can't keep up. As a result, the landscape of high-frequency trading is not just about speed; it's also about who has the resources to innovate and adapt.
To further illustrate the impact of high-frequency trading, consider the following table that outlines the key characteristics and implications:
Characteristic | Implication |
---|---|
Speed of Execution | Allows for rapid trades and exploitation of market inefficiencies. |
Algorithmic Strategies | Increases the complexity of trading strategies and data analysis. |
Market Impact | Can lead to increased volatility and sudden market movements. |
Regulatory Scrutiny | Heightened focus from regulators due to potential market manipulation risks. |
In summary, high-frequency trading represents a fascinating intersection of technology and finance. While it offers incredible opportunities for profit, it also comes with challenges that require constant vigilance and innovation. As the financial landscape continues to evolve, so too will the strategies employed by HFT firms, making it an area worth watching for both investors and regulators alike.
- What is high-frequency trading? High-frequency trading (HFT) is a trading strategy that uses powerful algorithms to execute a large number of trades at extremely high speeds.
- What are the advantages of HFT? The primary advantages include the ability to capitalize on small price discrepancies and execute trades faster than traditional methods.
- What are the risks associated with HFT? Risks include technical glitches, market volatility, and the potential for significant financial losses if algorithms misinterpret data.
- How does HFT impact the stock market? HFT can increase market liquidity but may also contribute to volatility and sudden market changes.
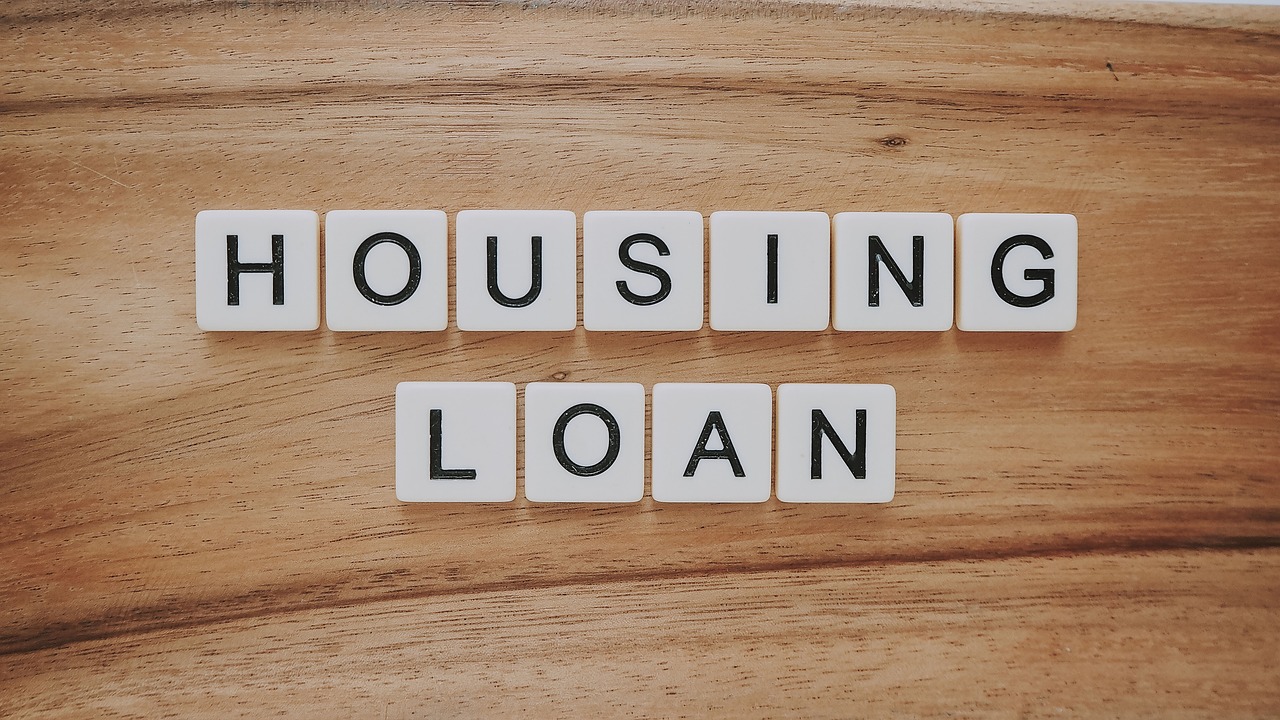
Sentiment Analysis
In the dynamic world of finance, understanding market sentiment is akin to having a sixth sense. employs the power of artificial intelligence to gauge the collective mood of investors by analyzing vast amounts of data from various sources, including news articles, social media posts, and even financial reports. This innovative approach allows traders to make informed decisions based on the prevailing attitudes and emotions in the market, rather than relying solely on traditional metrics.
Imagine walking into a bustling market where everyone is talking about the latest trends. Some are excited about a new product, while others express skepticism. In much the same way, sentiment analysis captures these varied perspectives and translates them into actionable insights. By utilizing natural language processing (NLP) and machine learning algorithms, AI can sift through thousands of online conversations and extract valuable sentiment indicators. This not only helps in predicting short-term price movements but also assists investors in understanding the underlying factors that drive market dynamics.
One of the key benefits of sentiment analysis is its ability to provide real-time insights. Traders can monitor sentiment shifts as they happen, allowing them to react swiftly to changing market conditions. For instance, if a major news outlet publishes a negative report about a company, sentiment analysis tools can quickly assess the impact of that news on social media and trading platforms. This rapid feedback loop enables traders to adjust their strategies proactively, potentially mitigating losses or capitalizing on emerging opportunities.
However, while sentiment analysis offers powerful advantages, it is not without its challenges. The accuracy of sentiment analysis can be influenced by various factors, such as the quality of data sources and the nuances of human language. Sarcasm, irony, and context can easily skew sentiment readings, leading to misinterpretations. Therefore, it is crucial for financial institutions to employ robust algorithms and continuously refine their models to enhance accuracy.
To illustrate the impact of sentiment analysis on trading strategies, consider the following table that outlines the correlation between sentiment scores and stock price movements:
Sentiment Score | Stock Price Movement |
---|---|
Positive | ↑ 5% within 24 hours |
Neutral | Stable |
Negative | ↓ 3% within 24 hours |
As seen in the table, a positive sentiment score often correlates with upward stock price movements, while negative sentiments can lead to declines. This correlation underscores the importance of sentiment analysis in making timely and informed trading decisions.
In conclusion, sentiment analysis represents a game-changing tool in the arsenal of modern traders. By harnessing the power of AI to interpret market sentiment, investors can gain a competitive edge, navigate the complexities of financial markets, and ultimately enhance their investment strategies. As technology continues to evolve, the role of sentiment analysis in finance is likely to expand, paving the way for even more sophisticated trading techniques.
- What is sentiment analysis? - Sentiment analysis is a technique used to determine the emotional tone behind a series of words, helping to understand the attitudes and opinions of investors.
- How does AI improve sentiment analysis? - AI enhances sentiment analysis by processing large volumes of data quickly and accurately, using natural language processing to understand context and nuances in language.
- Can sentiment analysis predict market trends? - While not foolproof, sentiment analysis can provide valuable insights into market trends by identifying shifts in investor sentiment that may precede price movements.
- What are the limitations of sentiment analysis? - Limitations include challenges in accurately interpreting sarcasm, irony, and context, which can lead to misinterpretations of sentiment.
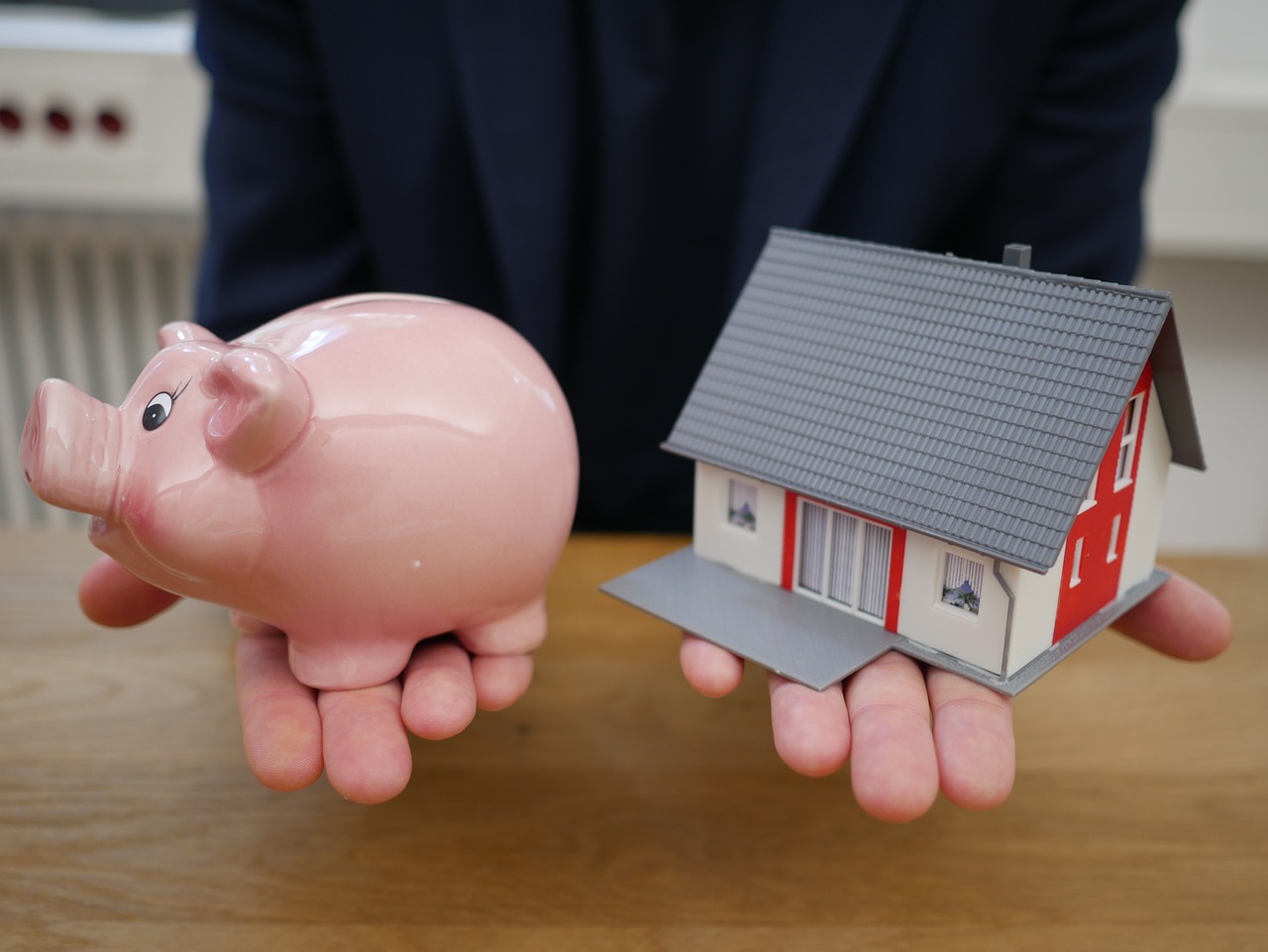
Risk Management Innovations
In today's fast-paced financial landscape, the integration of artificial intelligence (AI) into risk management is nothing short of revolutionary. Financial institutions are increasingly turning to AI to enhance their risk assessment processes, providing a level of precision and efficiency that traditional methods simply cannot match. Imagine trying to navigate a stormy sea without a compass; that's how it feels to manage risks without the aid of AI. With AI, firms can now utilize predictive analytics to foresee potential pitfalls and make informed decisions that safeguard their assets.
One of the most significant advantages of AI in risk management is its ability to analyze vast amounts of data in real-time. This capability allows financial institutions to identify patterns and anomalies that might indicate a potential risk. For example, AI can sift through historical data and current market trends to predict the likelihood of default on loans or investments, enabling firms to adjust their strategies proactively. In essence, AI acts like a seasoned navigator, guiding institutions through the choppy waters of financial uncertainty.
Moreover, AI-driven tools can enhance real-time monitoring of risk factors. By continuously analyzing market conditions, these tools provide alerts when certain thresholds are met, allowing companies to react swiftly. For instance, if a sudden market downturn is detected, AI systems can trigger automated responses, such as liquidating assets or adjusting investment portfolios to minimize losses. This level of responsiveness is crucial in a world where time is often the difference between profit and loss.
To illustrate the impact of AI in risk management, consider the following table that highlights key innovations:
Innovation | Description | Benefits |
---|---|---|
Predictive Analytics | Using historical data to forecast future risks. | Improved accuracy in risk assessment. |
Real-Time Monitoring | Constant analysis of market conditions. | Immediate alerts for risk mitigation. |
Automated Decision-Making | AI systems making real-time trading decisions. | Increased efficiency and reduced human error. |
However, it's essential to recognize that while AI offers tremendous potential, it is not without its challenges. Financial institutions must ensure that their AI systems are robust and secure, as the reliance on technology increases the risk of data breaches and cyberattacks. Therefore, implementing stringent security measures is crucial to protect sensitive financial information and maintain customer trust.
In conclusion, the innovations brought about by AI in risk management are transforming how financial institutions operate. By leveraging predictive analytics and real-time monitoring, firms can navigate the complexities of the market with greater confidence. Yet, as we embrace these advancements, we must also remain vigilant about the associated risks. The journey towards a more secure financial future is just beginning, and with AI at the helm, the possibilities are limitless.
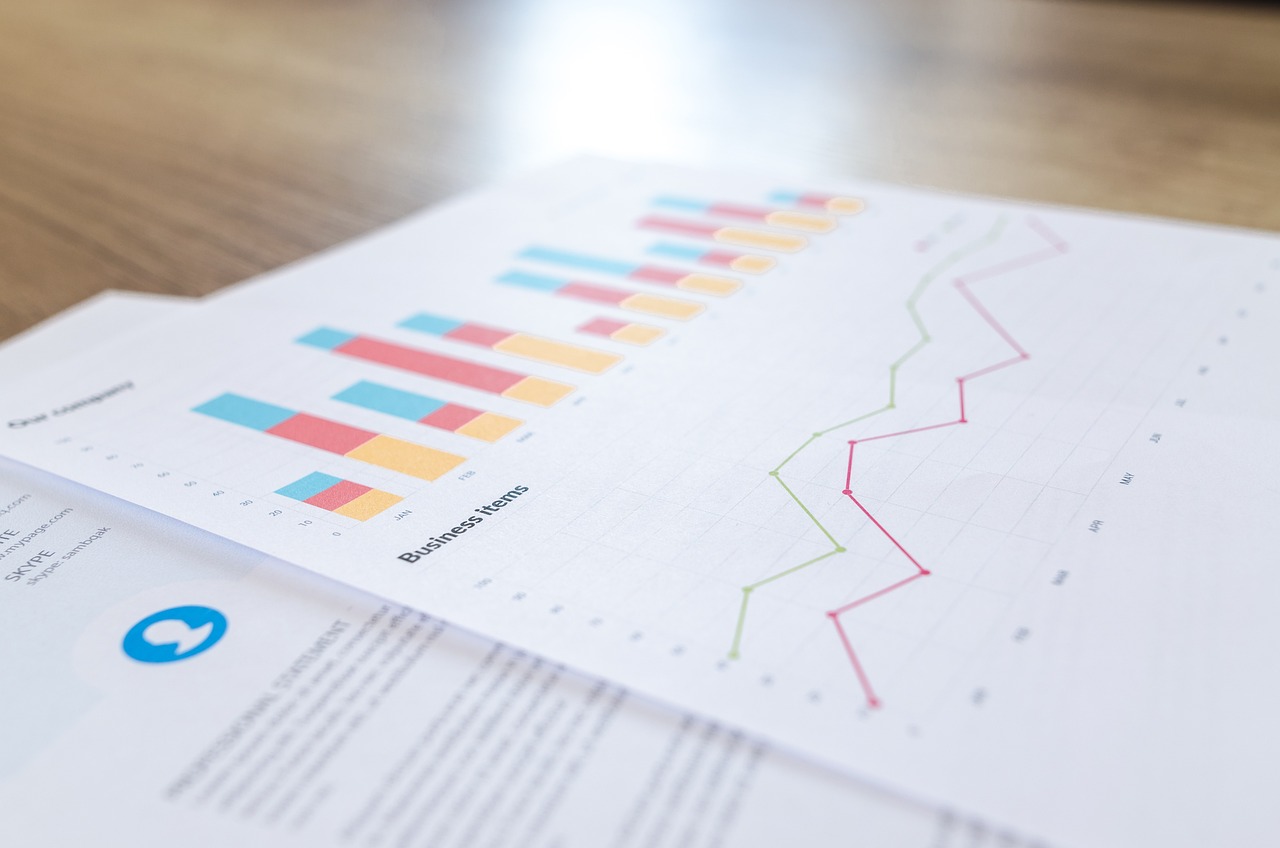
Challenges of AI Integration
As the financial sector embraces the wave of artificial intelligence, it also faces a myriad of challenges that can’t be overlooked. While the benefits of AI are tantalizing—think enhanced efficiency, improved decision-making, and lower operational costs—the road to successful integration is paved with obstacles. One of the most pressing issues is data privacy. In a world where data is the new oil, financial institutions are tasked with handling sensitive information responsibly. The implications of mishandling this data can be catastrophic, not just for the institutions but for their clients as well.
Moreover, as AI systems become more sophisticated, the need for robust security measures becomes paramount. Institutions must invest heavily in cybersecurity to protect against breaches that could expose confidential financial data. This brings us to another challenge: regulatory compliance. The financial landscape is already heavily regulated, and integrating AI technologies adds layers of complexity. Financial regulations must evolve to keep pace with AI advancements, which can sometimes feel like trying to hit a moving target. Institutions are often left grappling with how to ensure compliance while still fostering innovation.
Furthermore, ethical considerations cannot be ignored. AI algorithms can inadvertently perpetuate biases present in the data they are trained on, leading to unfair outcomes. Imagine a loan approval process that unfairly disadvantages certain demographics due to biased data inputs. This not only poses a risk to the institution's reputation but also raises significant ethical questions about fairness and equality in financial services. Therefore, it’s crucial for institutions to establish clear guidelines and frameworks to mitigate these risks.
Here's a quick summary of the main challenges faced by financial institutions when integrating AI:
Challenge | Description |
---|---|
Data Privacy | Handling sensitive information responsibly and protecting against breaches. |
Regulatory Compliance | Adapting to evolving regulations while fostering innovation. |
Ethical Considerations | Avoiding biases in AI algorithms that can lead to unfair outcomes. |
In conclusion, while the integration of AI into the financial sector promises a future filled with opportunities, it’s essential to navigate these challenges carefully. Institutions must prioritize data security, stay ahead of regulatory changes, and ensure ethical practices are at the forefront of their AI strategies. The road may be rocky, but with careful planning and execution, the rewards can be monumental.
- What are the main challenges of integrating AI in finance? Data privacy, regulatory compliance, and ethical considerations are the primary challenges.
- How can financial institutions ensure data privacy? By implementing robust cybersecurity measures and adhering to strict data handling protocols.
- Why is regulatory compliance a challenge for AI integration? Financial regulations are complex and must evolve to keep pace with rapidly advancing AI technologies.
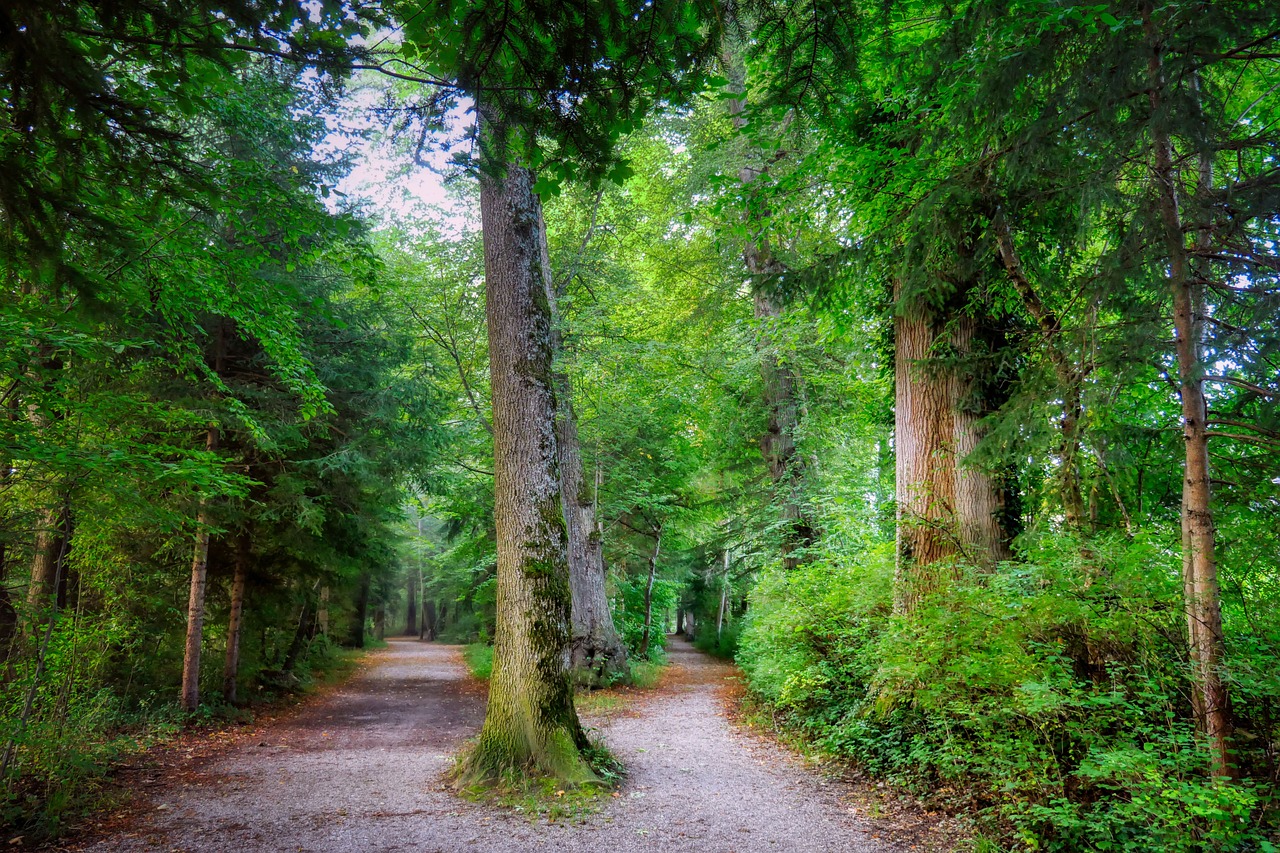
Data Privacy Concerns
As we venture deeper into the era of artificial intelligence in finance, one cannot ignore the looming shadow of . The financial sector is a treasure trove of sensitive information, from personal identification details to intricate transaction histories. When AI systems process this data to enhance decision-making and risk assessment, the question arises: how secure is this information? The reality is that every time a financial institution integrates AI, it opens the door to potential vulnerabilities.
Imagine your financial data as a precious jewel, locked away in a vault. Now, consider that vault being accessed by a sophisticated AI system. While this AI can analyze and draw insights from your data, it also poses a risk if the vault's security isn’t robust. A breach could lead to catastrophic consequences, not just for individuals but for entire institutions. Therefore, ensuring data privacy is paramount.
Moreover, the regulatory landscape surrounding data privacy is evolving. With regulations such as the General Data Protection Regulation (GDPR) in Europe and various state-level laws in the United States, financial institutions must navigate a complex web of compliance requirements. These regulations mandate strict protocols for data handling, storage, and sharing, adding another layer of challenge for firms looking to innovate with AI.
To address these concerns, financial institutions are increasingly investing in robust cybersecurity measures. This includes advanced encryption techniques, regular security audits, and employee training programs to ensure that everyone understands the importance of data privacy. Here’s a brief overview of some key strategies being employed:
Data Privacy Strategy | Description |
---|---|
Encryption | Data is transformed into a secure format that can only be read by authorized parties. |
Access Controls | Limiting data access to only those who need it for their roles. |
Regular Audits | Conducting periodic checks to ensure compliance with data privacy regulations. |
Employee Training | Educating staff on best practices for handling sensitive information. |
Despite these measures, the question of trust remains. Can consumers truly feel secure knowing that their financial data is being processed by AI? Building trust is crucial for the widespread adoption of AI in finance. Institutions must be transparent about how they use data, what measures they have in place to protect it, and the potential risks involved. This transparency can foster a more trusting relationship between consumers and financial institutions, ultimately leading to a more successful integration of AI technologies.
In conclusion, while the integration of AI into the financial sector holds great promise, it is accompanied by significant data privacy concerns that cannot be overlooked. As we advance, striking a balance between innovation and privacy will be essential. Financial institutions must prioritize data protection to ensure that the benefits of AI do not come at the cost of consumer trust.
- What are the main data privacy concerns in AI? The main concerns include the potential for data breaches, unauthorized access to sensitive information, and compliance with evolving regulations.
- How can financial institutions protect sensitive data? Institutions can implement encryption, access controls, regular audits, and employee training to safeguard sensitive information.
- Why is consumer trust important in AI adoption? Consumer trust is vital for the successful integration of AI technologies, as it encourages individuals to share their data for improved services.
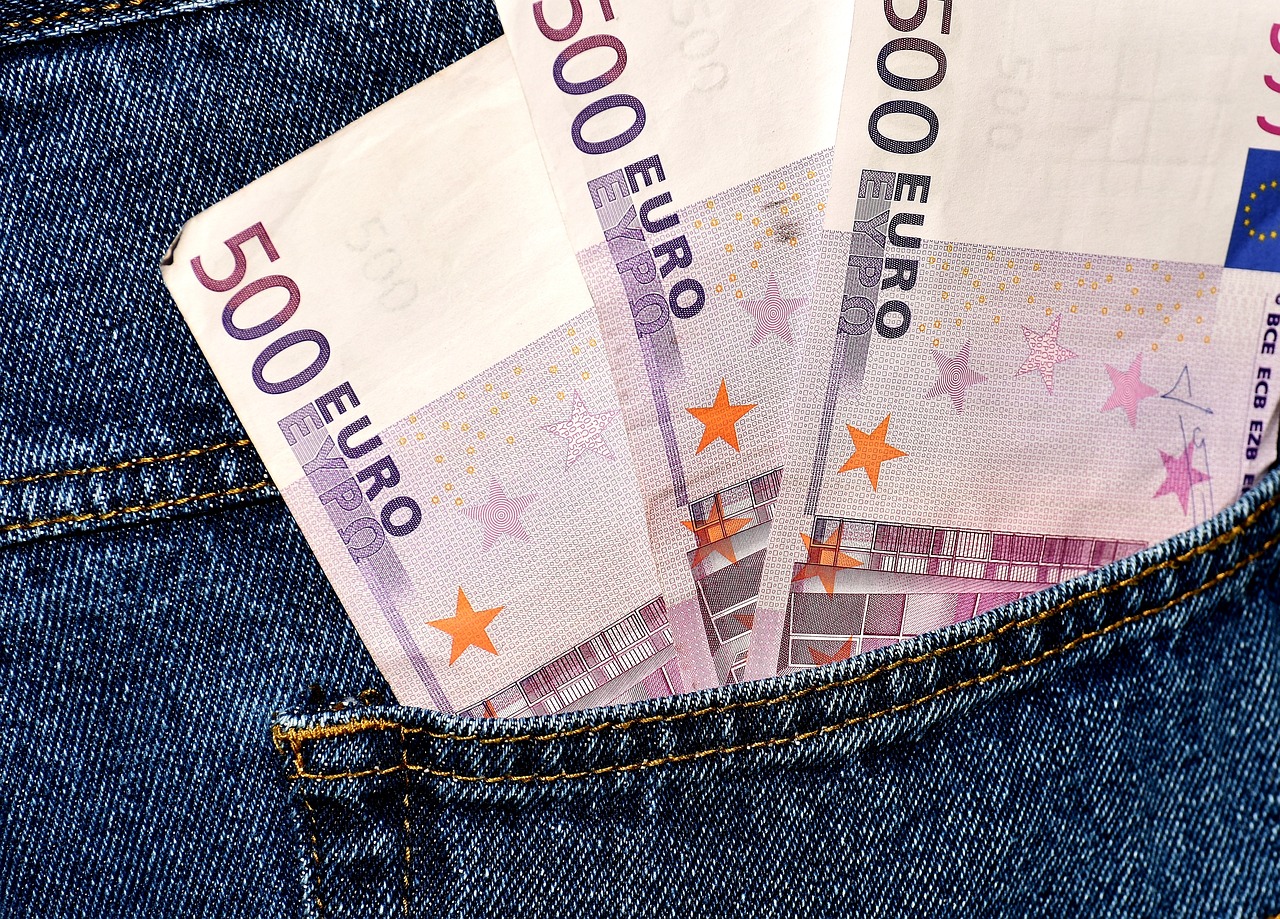
Regulatory Compliance
As the financial landscape evolves with the integration of artificial intelligence (AI), the need for becomes increasingly critical. Financial institutions are now navigating a labyrinth of regulations that must adapt to the rapid pace of technological advancements. This is no small feat, as compliance not only involves adhering to existing laws but also anticipating future regulations that may emerge as AI technologies continue to develop.
One of the primary challenges in ensuring regulatory compliance is the dynamic nature of AI itself. Financial regulations are often slow to catch up with technological innovations, leading to a gap that can expose institutions to risks. For instance, while AI can enhance efficiency and decision-making, it also introduces complexities in accountability and transparency. Regulators must grapple with questions such as: Who is responsible for decisions made by an AI system? How can we ensure that AI algorithms are not biased? These questions highlight the urgent need for a robust framework that governs the use of AI in finance.
Moreover, the global nature of finance means that institutions operate across different jurisdictions, each with its own set of regulations. This creates a patchwork of compliance requirements that can be daunting. Financial institutions must not only understand local regulations but also be aware of international standards and practices. The following table illustrates some of the key regulatory bodies and their respective focuses:
Regulatory Body | Focus Area |
---|---|
Financial Stability Board (FSB) | Global financial stability |
European Securities and Markets Authority (ESMA) | European financial markets |
U.S. Securities and Exchange Commission (SEC) | U.S. securities markets |
Office of the Comptroller of the Currency (OCC) | National banks and federal savings associations |
To navigate these complexities, many financial institutions are investing in compliance technologies, often referred to as RegTech. These tools leverage AI to automate compliance processes, monitor transactions in real-time, and ensure adherence to regulations. By utilizing AI-driven solutions, institutions can significantly reduce the risk of non-compliance and enhance their ability to respond to regulatory changes swiftly.
In conclusion, while the integration of AI in finance presents remarkable opportunities, it also necessitates a proactive approach to regulatory compliance. Financial institutions must engage with regulators, invest in compliance technologies, and foster a culture of transparency and accountability. Only by doing so can they harness the full potential of AI while mitigating risks associated with regulatory non-compliance.
- What is regulatory compliance in finance?
Regulatory compliance refers to the process of ensuring that financial institutions adhere to laws, regulations, and guidelines set by governing bodies. - How does AI impact regulatory compliance?
AI can enhance regulatory compliance by automating processes, monitoring transactions, and providing insights that help institutions stay compliant with evolving regulations. - What are some challenges of regulatory compliance with AI?
Challenges include the rapid pace of technological change, the need for transparency in AI systems, and the complexities of operating across multiple jurisdictions.
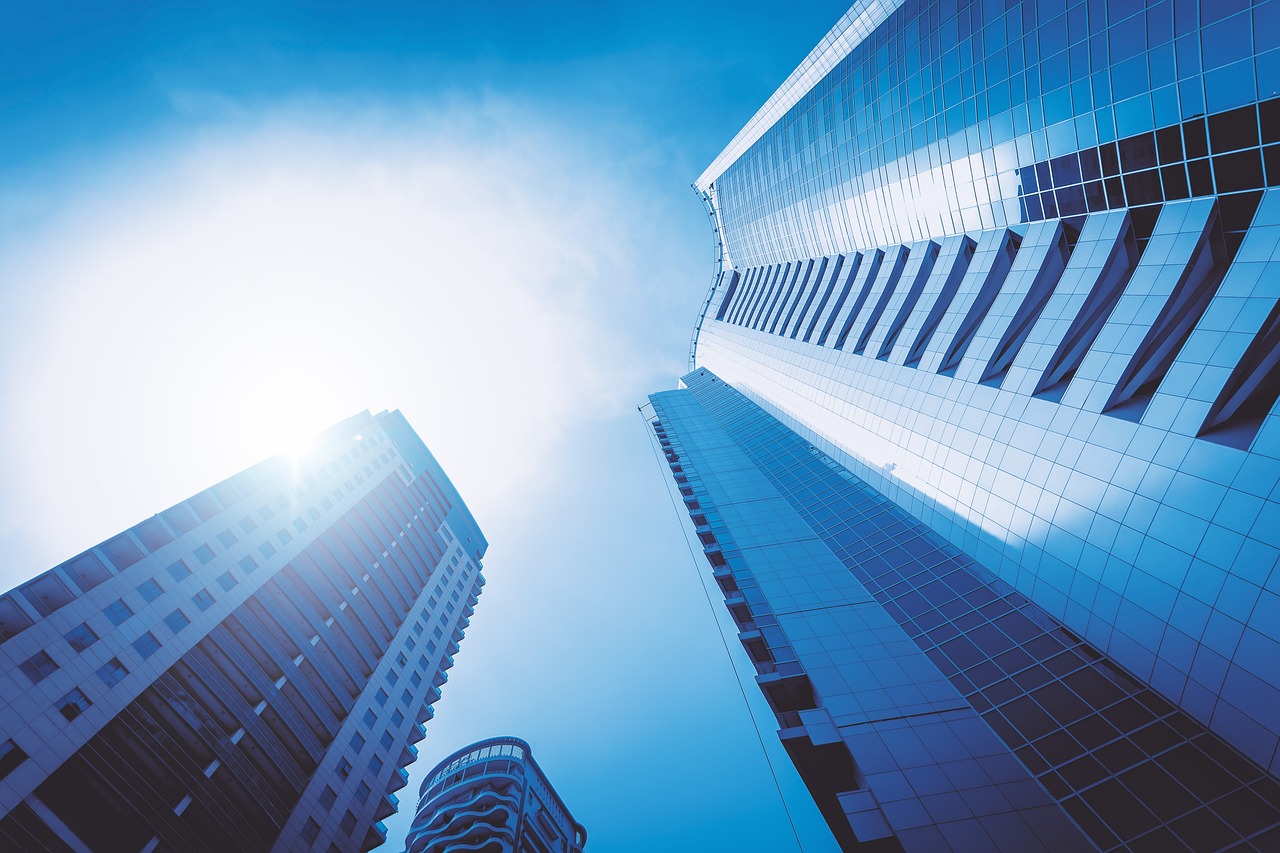
The Future of AI in Finance
As we stand on the brink of a new era in finance, the impact of artificial intelligence is poised to be nothing short of revolutionary. The future of AI in finance is not just about automating tasks or improving efficiencies; it's about fundamentally transforming how we approach investing, risk management, and customer interaction. Imagine a world where financial decisions are not only data-driven but also enhanced by real-time analysis and predictive modeling. This is the exciting frontier that awaits us.
One of the most significant trends we can anticipate is the rise of personalized financial services. AI algorithms will enable institutions to analyze individual customer data, leading to tailored investment strategies that align with personal goals and risk appetites. This level of customization can create a more engaging experience for clients, resulting in higher satisfaction and loyalty. Just as you wouldn’t wear a one-size-fits-all suit, why should your financial strategy be generic?
Moreover, we can expect AI to play a crucial role in enhancing regulatory compliance. As the financial landscape becomes increasingly complex, AI can help institutions navigate the labyrinth of regulations by providing real-time compliance monitoring and reporting. This not only reduces the risk of hefty fines but also allows firms to focus on innovation rather than getting bogged down in paperwork. Imagine a compliance officer who never sleeps, tirelessly ensuring that every transaction adheres to the latest regulations!
Another exciting development will be the integration of blockchain technology with AI. The combination of these two technologies has the potential to create a more transparent and secure financial environment. For instance, smart contracts powered by AI could automate and enforce contractual agreements without the need for intermediaries. This could lead to faster transactions, reduced costs, and minimized fraud. The synergy between AI and blockchain could very well be the backbone of the next-generation financial ecosystem.
However, the future is not without its challenges. As we embrace AI, we must also be vigilant about the ethical implications and potential biases embedded in algorithms. Financial institutions will need to prioritize ethical AI practices to ensure that their systems are fair and transparent. This means regularly auditing AI models, ensuring diverse data representation, and maintaining accountability for decisions made by AI systems. After all, just because a machine can make a decision doesn’t mean it should be free from scrutiny.
In conclusion, the future of AI in finance is bright and filled with possibilities. As we harness the power of AI, we can expect to see a more efficient, personalized, and secure financial landscape. However, it will require a concerted effort from all stakeholders to navigate the challenges and ensure that this technology serves the greater good. The financial market is on the cusp of a transformation, and those who adapt to these changes will not only survive but thrive.
- What role will AI play in investment strategies? AI will enable more personalized and data-driven investment strategies, allowing for better alignment with individual goals.
- How can AI improve regulatory compliance? AI can provide real-time monitoring and reporting, helping institutions navigate complex regulations more efficiently.
- What are the ethical considerations of using AI in finance? Institutions must ensure fairness, transparency, and accountability in AI systems to mitigate potential biases and ethical concerns.
Frequently Asked Questions
- What is AI's role in the financial market?
AI is transforming the financial market by enhancing decision-making, improving risk assessment, and increasing operational efficiency. It allows financial institutions to analyze vast amounts of data quickly, leading to more informed investment strategies and better management of resources.
- How does algorithmic trading work?
Algorithmic trading uses AI algorithms to execute trades at optimal prices, minimizing human error. These algorithms analyze market conditions and execute trades based on pre-defined criteria, allowing for faster and more efficient trading compared to traditional methods.
- What are the benefits of high-frequency trading?
High-frequency trading leverages AI to make rapid trades based on minute market fluctuations. It offers advantages like increased liquidity and the ability to capitalize on small price changes. However, it also comes with risks, such as market volatility and the potential for significant losses.
- What is sentiment analysis in finance?
Sentiment analysis uses AI to evaluate market sentiment by analyzing news articles and social media posts. This information helps traders gauge public perception and make informed trading decisions, as market sentiment can significantly influence price movements.
- How does AI improve risk management?
AI enhances risk management by providing predictive analytics and real-time monitoring. Financial institutions can use AI tools to better assess potential risks and implement strategies to mitigate them, leading to more robust financial practices.
- What are the data privacy concerns associated with AI in finance?
AI in finance raises significant data privacy issues, especially regarding the handling of sensitive financial information. Ensuring robust security measures and compliance with data protection regulations is crucial for maintaining customer trust and safeguarding personal data.
- How do financial regulations adapt to AI technologies?
Financial regulations must evolve to address the challenges posed by AI technologies. This includes ensuring compliance while fostering innovation. Regulatory bodies are working on frameworks that balance the need for oversight with the benefits of AI advancements.
- What does the future hold for AI in finance?
The future of AI in finance is promising, with continuous advancements expected to reshape the industry. Emerging trends may include more sophisticated predictive analytics, enhanced customer service through AI chatbots, and further automation of trading processes.