Vulnerabilities and Ethics in AI Systems
In today's rapidly evolving technological landscape, artificial intelligence (AI) stands at the forefront, promising revolutionary advancements across various sectors. However, with great power comes great responsibility, and the vulnerabilities inherent in AI systems pose significant challenges that we must address. This article delves into the multifaceted vulnerabilities of AI, exploring the ethical implications that arise from their deployment. As we navigate this brave new world, it's crucial to highlight the need for responsible AI development and usage to ensure that these systems benefit society as a whole.
AI systems can be susceptible to a range of vulnerabilities, including data biases, security flaws, and algorithmic errors. These weaknesses can lead to unintended consequences, such as reinforcing stereotypes or making erroneous decisions. For instance, if an AI model is trained on biased data, it may perpetuate those biases in its outputs, leading to unfair treatment of certain groups. Recognizing these vulnerabilities is not just an academic exercise; it's a crucial step in developing robust and reliable AI applications that can be trusted to operate in real-world scenarios.
The deployment of AI raises significant ethical questions that cannot be ignored. Issues of fairness, accountability, and transparency are at the forefront of discussions surrounding AI ethics. As AI systems increasingly influence our lives, addressing these concerns is essential to build trust and ensure responsible AI use in society. For example, when an AI system makes a decision that affects an individual's life—such as in hiring or loan approval—understanding how that decision was made is vital to ensure fairness and accountability.
AI systems often rely on vast amounts of data to function effectively. However, this reliance can lead to serious privacy violations. When individuals' personal data is collected and used without their knowledge or consent, it raises ethical concerns about individual rights and freedoms. Understanding how data is collected and utilized is vital for protecting these rights. For instance, companies must ensure that they are transparent about their data practices and that users have control over their information.
Establishing effective regulations is necessary to safeguard user data and ensure ethical AI practices. Current regulations, such as the General Data Protection Regulation (GDPR) in Europe, set a precedent for data protection. However, there is still much work to be done. Potential improvements could include:
- Stricter penalties for data breaches
- Enhanced user rights regarding data access and deletion
- Clear guidelines for AI system transparency
By enhancing these regulatory frameworks, we can better protect individuals and foster trust in AI technologies.
Informed consent is crucial when it comes to using personal data in AI systems. Users must be made aware of how their data is utilized, and they should have the option to opt-in or opt-out of data collection practices. This part emphasizes the importance of user awareness regarding data usage in AI systems. Without proper consent, the ethical foundation of AI applications crumbles, leading to potential misuse and public distrust.
Bias in AI algorithms can lead to unfair treatment of certain groups, making it imperative to identify and mitigate these biases. For example, facial recognition technology has been criticized for its disproportionately high error rates among people of color. Addressing these biases is fundamental to promoting fairness and equity in AI applications. This requires ongoing scrutiny and the implementation of diverse datasets that accurately reflect the populations affected by AI decisions.
Determining accountability in AI decision-making processes is complex. As AI systems become more autonomous, questions arise about who should be held responsible for AI actions. Is it the developers, the users, or the AI itself? This section explores the implications of accountability for both users and developers, emphasizing that clarity in accountability is essential for ethical AI deployment.
As AI systems operate with increasing autonomy, understanding who is liable for AI-generated outcomes becomes crucial. For instance, if an autonomous vehicle is involved in an accident, should the liability fall on the manufacturer, the software developer, or the vehicle owner? Navigating these legal and ethical challenges requires a comprehensive approach that considers the nuances of AI technology.
Transparency in AI operations fosters trust and understanding among users. The importance of explainability in AI systems cannot be overstated; users should be able to comprehend and challenge AI decisions. When AI systems operate as "black boxes," it becomes difficult for individuals to trust their outputs. By promoting transparency, developers can help users feel more secure and informed about the decisions that affect their lives.
Q: What are the main vulnerabilities of AI systems?
A: AI systems are primarily vulnerable to data biases, security flaws, and algorithmic errors, which can lead to unfair treatment and erroneous outcomes.
Q: How can we ensure ethical AI practices?
A: Establishing robust regulatory frameworks, ensuring informed consent, and promoting transparency and accountability are essential steps in ensuring ethical AI practices.
Q: Why is transparency important in AI?
A: Transparency fosters trust and understanding, allowing users to comprehend and challenge AI decisions, which is crucial for ethical deployment.
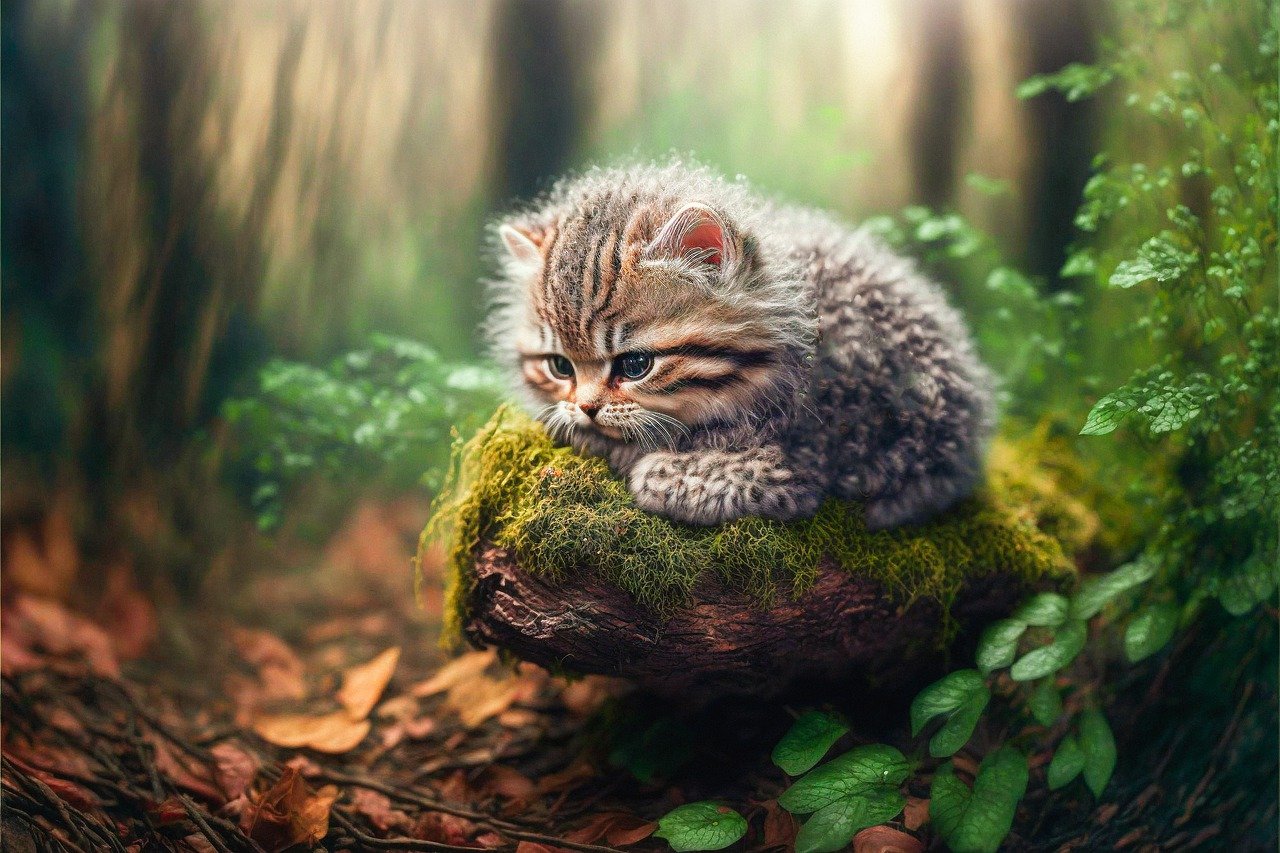
Understanding AI Vulnerabilities
Artificial Intelligence (AI) systems, while revolutionary, are not immune to vulnerabilities that can undermine their effectiveness and reliability. These vulnerabilities can stem from various sources, such as data biases, security flaws, and algorithmic errors. Understanding these weaknesses is crucial for developing AI applications that are not only robust but also trustworthy.
One of the most prominent vulnerabilities is data bias. AI systems learn from historical data, which can often reflect societal biases. For example, if an AI model is trained on data that predominantly features one demographic group, it may inadvertently perpetuate stereotypes or discriminate against underrepresented groups. This can lead to outcomes that are not only unfair but also damaging to those affected. Addressing data bias involves not just improving data collection methods but also actively seeking diverse datasets to ensure a more balanced perspective.
Another significant vulnerability lies in security flaws. AI systems can be targeted by malicious actors who exploit weaknesses in the algorithms or the data they process. For instance, adversarial attacks can manipulate an AI's decision-making process by subtly altering the input data, leading to incorrect outputs. This highlights the importance of implementing robust security measures to protect AI systems from external threats. Developers must continuously test and update their systems to guard against evolving security challenges.
Furthermore, algorithmic errors can occur due to programming mistakes or unforeseen interactions within complex AI systems. These errors can result in unexpected behavior or decisions that may not align with the intended purpose of the AI. For example, an autonomous vehicle might misinterpret a stop sign due to an error in its image recognition algorithm, leading to potentially dangerous situations. This underlines the necessity for thorough testing and validation of AI algorithms before deployment.
In summary, recognizing the vulnerabilities inherent in AI systems is the first step toward developing more reliable and responsible technologies. By focusing on data integrity, enhancing security measures, and ensuring algorithm accuracy, we can mitigate these risks. The journey towards robust AI is ongoing, but understanding these vulnerabilities is essential for fostering trust and promoting ethical AI usage.
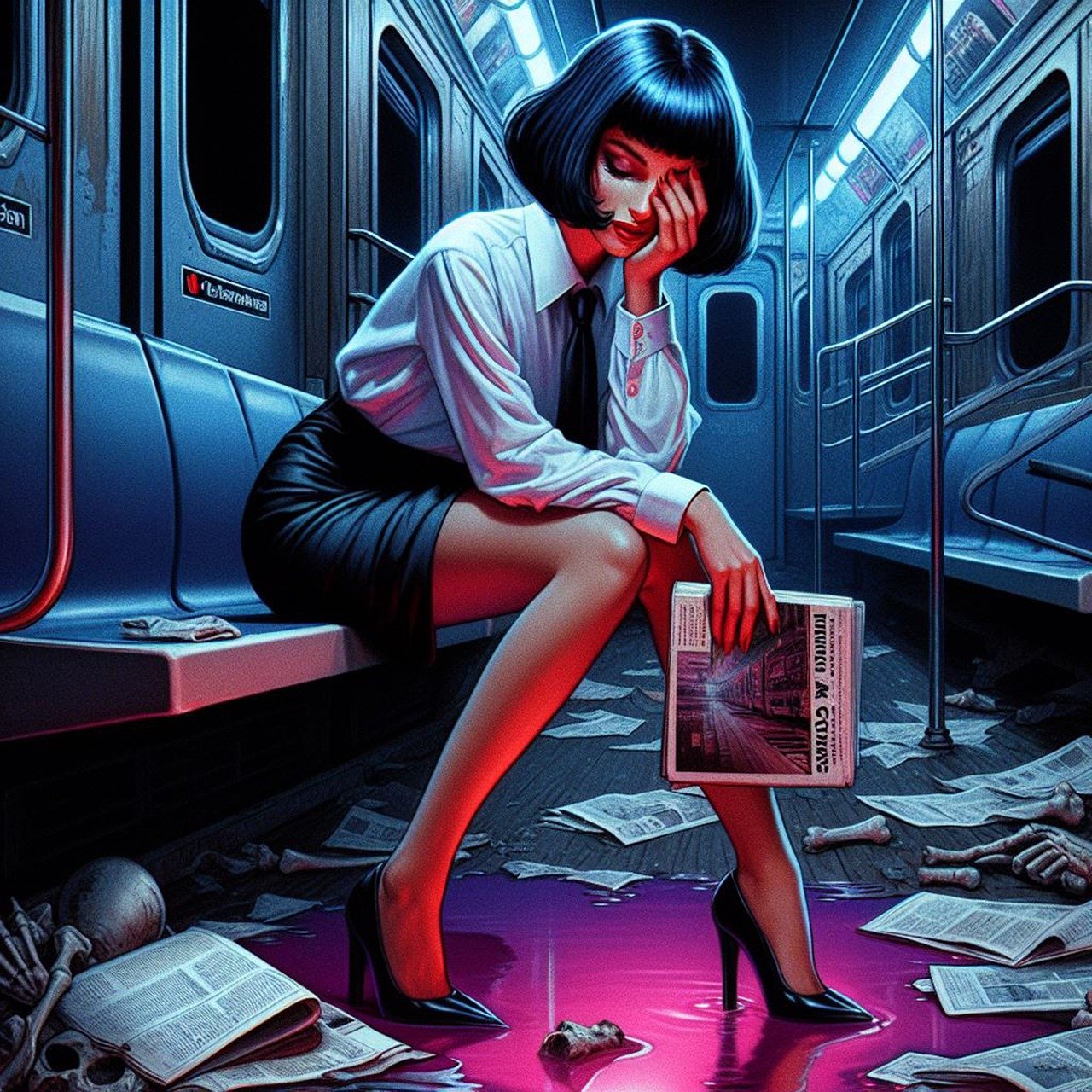
Ethical Implications of AI
The deployment of artificial intelligence (AI) technologies is transforming the way we live, work, and interact with one another. However, with great power comes great responsibility, and the ethical implications of AI are becoming increasingly crucial to consider. As we integrate AI systems into various aspects of our lives, questions about fairness, accountability, and transparency arise. These concerns are not merely theoretical; they have real-world consequences that can impact individuals and communities alike.
One of the most pressing ethical issues is the potential for bias in AI algorithms. When AI systems are trained on data that reflects historical inequalities or prejudices, they can perpetuate and even amplify these biases. For instance, consider a hiring algorithm that favors candidates from certain demographics over others. This not only raises questions about fairness but also has broader societal implications, reinforcing existing disparities. Thus, addressing bias in AI is not just a technical challenge; it’s a moral imperative.
Moreover, accountability in AI systems is a complex issue. When an AI system makes a mistake—say, misidentifying a person in a security system—who is responsible? Is it the developer who created the algorithm, the company that deployed it, or the user who relied on it? The ambiguity surrounding liability can lead to a lack of accountability, which is troubling in a world increasingly reliant on AI for critical decisions. It’s essential for stakeholders to clarify these responsibilities to ensure that users can trust the systems they interact with.
Transparency is another vital ethical consideration. AI systems often operate as "black boxes," making it difficult for users to understand how decisions are made. This lack of clarity can erode trust and prevent users from challenging potentially harmful decisions. For example, if an AI system denies a loan application, the applicant should have the right to know the reasons behind that decision. By fostering transparency and explainability in AI operations, we can empower users and create a more equitable environment.
To address these ethical implications, several strategies can be employed:
- Developing Ethical Guidelines: Organizations should create ethical frameworks that guide AI development and deployment, ensuring that fairness and accountability are prioritized.
- Implementing Bias Mitigation Techniques: Techniques such as diversified training datasets and regular audits can help identify and reduce biases in AI systems.
- Enhancing User Education: Educating users about how AI systems work and their potential implications can foster informed consent and awareness.
In conclusion, the ethical implications of AI are multifaceted and require careful consideration from all stakeholders involved. By prioritizing fairness, accountability, and transparency, we can harness the power of AI while minimizing its risks, ultimately leading to a more just and equitable society.
Q1: What are the main ethical concerns associated with AI?
A1: The primary ethical concerns include bias, accountability, transparency, and data privacy. These issues can lead to unfair treatment of individuals and communities if not addressed properly.
Q2: How can bias in AI be mitigated?
A2: Bias can be mitigated through diverse training datasets, regular audits of AI systems, and implementing ethical guidelines during the development process.
Q3: Why is transparency important in AI systems?
A3: Transparency is crucial because it helps users understand how decisions are made, fostering trust and allowing individuals to challenge potentially harmful outcomes.

Data Privacy Concerns
In today's digital age, where Artificial Intelligence (AI) systems are increasingly integrated into our daily lives, the issue of data privacy has become a hot topic. These systems often rely on vast amounts of data to function effectively, raising significant concerns about how this data is collected, stored, and used. With AI's capability to analyze and process personal information, it’s crucial to understand the implications of such practices on individual privacy. Have you ever wondered what happens to your data once it’s fed into an AI system? This question is more than just curiosity; it’s a fundamental concern that affects everyone.
One of the primary issues surrounding data privacy in AI is the potential for privacy violations. When personal data is collected without explicit consent or adequate protection measures, individuals can find themselves vulnerable to misuse of their information. For instance, consider a scenario where an AI system analyzes user behavior to tailor advertisements. If the data used includes sensitive information—like health records or financial details—what safeguards are in place to protect that data from falling into the wrong hands? This is where the conversation about ethical AI usage becomes paramount.
Moreover, the lack of transparency in how AI systems operate can exacerbate these privacy concerns. Users often have little to no idea about the data collection processes, which can lead to a significant disconnect between the AI systems and the people they serve. To illustrate this point, let’s take a closer look at some common data privacy concerns associated with AI systems:
- Data Breaches: AI systems can be prime targets for cyberattacks, resulting in unauthorized access to personal information.
- Informed Consent: Users may not fully understand what they are consenting to when providing their data, leading to ethical dilemmas.
- Data Retention: How long is personal data stored? Prolonged retention can increase the risk of privacy violations.
To combat these privacy issues, it’s essential to implement robust regulatory frameworks that ensure the ethical use of data in AI systems. This includes establishing clear guidelines on data collection, storage, and usage, which can help protect individual rights and freedoms. Furthermore, promoting user awareness about data privacy can empower individuals to make informed decisions about their personal information. After all, knowledge is power, and in the realm of data privacy, it’s crucial for users to understand how their data is being utilized.
In conclusion, the intersection of AI and data privacy raises profound questions that cannot be overlooked. As we continue to embrace AI technologies, we must prioritize the protection of personal data and ensure that ethical considerations are at the forefront of AI development. Only then can we foster a safe and trustworthy environment for users, where innovation and privacy coexist harmoniously.
- What is data privacy in the context of AI?
Data privacy refers to the proper handling of personal information collected by AI systems, ensuring it is used ethically and protected from unauthorized access. - How can I protect my data when using AI applications?
Always review the privacy policies of applications, opt for services that prioritize data protection, and be cautious about the personal information you share. - What regulations exist to protect data privacy in AI?
Various regulations, such as the General Data Protection Regulation (GDPR) in Europe, aim to safeguard user data and promote ethical AI practices.
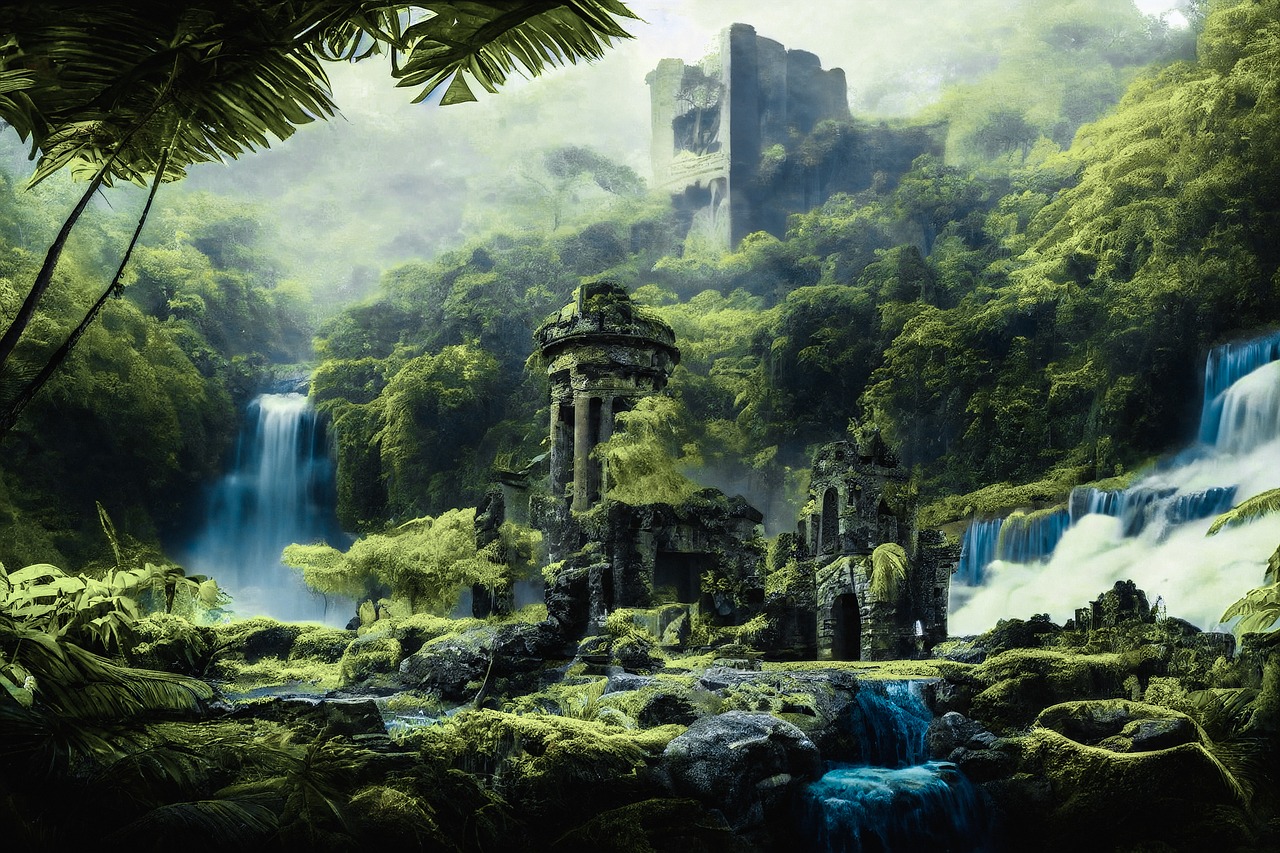
Regulatory Frameworks
In today's digital landscape, the rapid advancement of AI technologies has outpaced the establishment of comprehensive regulatory frameworks. This gap creates a precarious environment where personal data can be mishandled, leading to serious privacy violations and ethical dilemmas. Governments and organizations worldwide are beginning to recognize the urgent need for regulations that not only protect user data but also promote ethical AI practices. The challenge lies in crafting regulations that are flexible enough to adapt to the fast-evolving AI landscape while still providing robust protections for individuals.
One of the most significant developments in this area has been the introduction of the General Data Protection Regulation (GDPR) in the European Union. This regulation sets a high standard for data privacy and protection, mandating that organizations obtain explicit consent from users before collecting their data. It also empowers users with rights such as the ability to access, correct, and delete their personal information. However, while GDPR serves as a model, it is not without its limitations. For instance, its applicability is primarily limited to EU citizens, leaving gaps in protection for individuals outside the region.
Moreover, other countries are now considering similar regulations. For example, California's Consumer Privacy Act (CCPA) has emerged as a significant piece of legislation in the United States, aiming to enhance privacy rights and consumer protection. Such regulations often include provisions that require companies to disclose their data collection practices and allow users to opt-out of data sales. However, the effectiveness of these frameworks depends on rigorous enforcement and compliance from organizations.
To enhance the effectiveness of regulatory frameworks, several key elements should be considered:
- Transparency: Organizations must be required to clearly communicate their data practices to users, ensuring that individuals understand how their data is being used.
- Accountability: Developers and companies should be held accountable for the ethical implications of their AI systems, including the consequences of biased algorithms.
- Adaptability: Regulations should be designed to evolve alongside technological advancements, allowing for timely updates that address emerging challenges in AI.
In conclusion, establishing effective regulatory frameworks is crucial for safeguarding user data and ensuring ethical AI practices. As AI continues to permeate various aspects of our lives, the call for robust regulations will only grow louder. It is imperative for stakeholders, including governments, tech companies, and civil society, to collaborate in crafting regulations that not only protect individual rights but also foster innovation in a responsible manner.
- What are regulatory frameworks? Regulatory frameworks are systems of rules and guidelines that govern how organizations and individuals can collect, use, and protect data.
- Why are regulations important for AI? Regulations are essential for ensuring that AI systems operate ethically, protect user privacy, and promote fairness in decision-making processes.
- How can I protect my data when using AI systems? Always read the privacy policies of AI services, and be aware of your rights regarding data collection and usage.
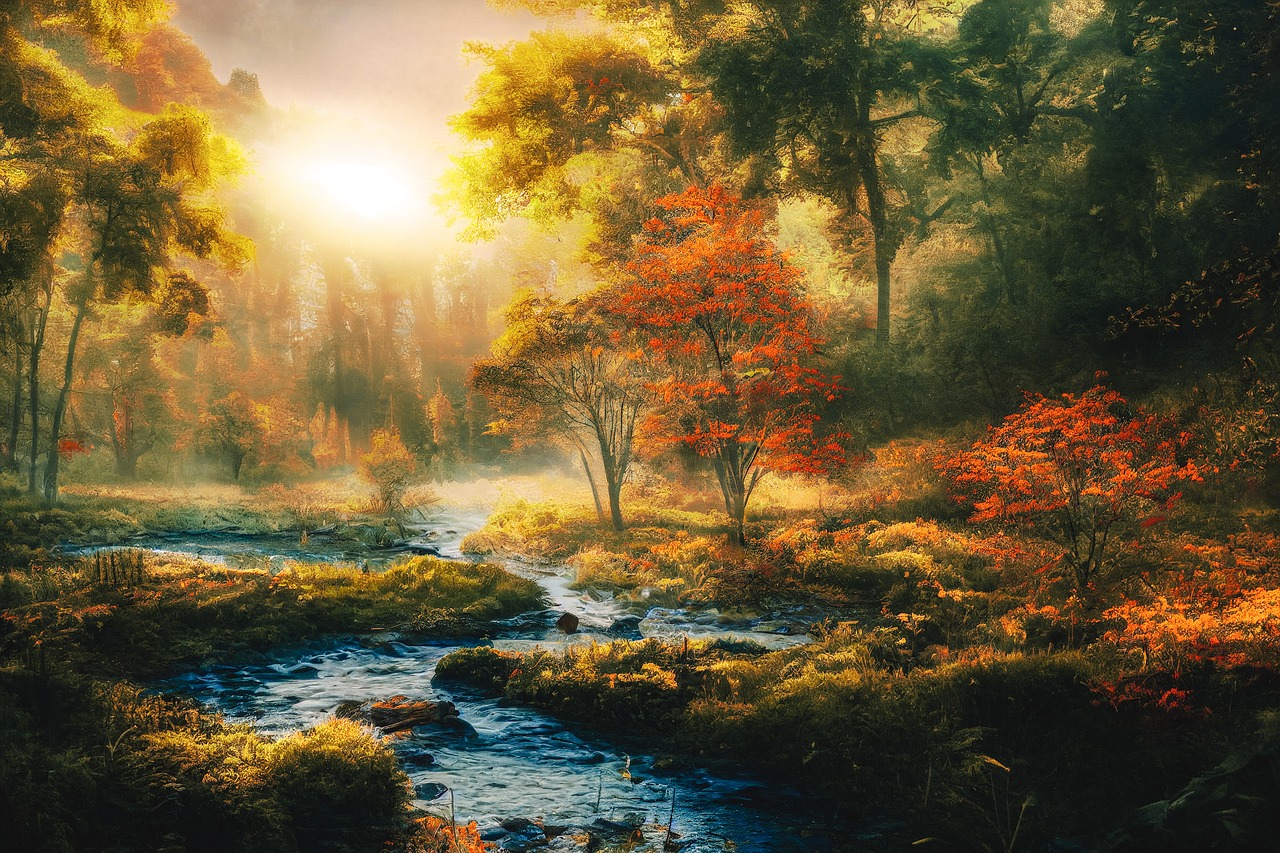
Consent and User Awareness
In today's digital age, where data is the new oil, the concept of informed consent has never been more critical. When it comes to AI systems, users often find themselves in a maze of complex algorithms and data usage policies that can be overwhelming. Many people are unaware of how their personal information is collected, processed, and utilized by AI technologies. This lack of awareness can lead to significant privacy violations and a feeling of disempowerment among users.
To truly empower users, AI developers and companies must prioritize transparency. This means providing clear, concise, and accessible information about data practices. Imagine walking into a restaurant where the menu is written in a language you don't understand. You'd feel lost, right? Similarly, when users are presented with dense legal jargon regarding their data, it creates a barrier to understanding. Therefore, it's essential to break down complex terms into simple language that everyone can grasp.
Furthermore, users should be made aware of their rights regarding their personal data. Here are some key points that should be communicated effectively:
- Right to Access: Users should have the ability to know what data is being collected and how it is used.
- Right to Withdraw Consent: Users should be able to revoke their consent at any time, allowing them to control their data.
- Right to Be Informed: Users need to be informed about any changes in data usage policies.
Moreover, organizations should implement user-friendly interfaces that allow individuals to easily manage their consent preferences. Imagine if you could adjust your privacy settings with a simple toggle switch rather than navigating through a labyrinth of options. This kind of intuitive design can significantly enhance user trust and engagement.
In addition, regular training and awareness programs can help educate users about the implications of their data sharing. By fostering a culture of awareness, users will become more proactive in managing their consent and understanding the potential risks associated with AI technologies. After all, knowledge is power, and when users are informed, they can make better decisions regarding their personal information.
In conclusion, consent and user awareness are not just legal obligations; they are ethical imperatives. As AI systems continue to evolve and permeate our daily lives, it is crucial that we cultivate an environment where users feel informed, empowered, and in control of their data. This not only enhances trust but also paves the way for a more responsible and ethical AI landscape.
Q: What is informed consent in the context of AI?
A: Informed consent refers to the process of ensuring that users are fully aware of how their data will be collected, used, and shared by AI systems before they agree to participate.
Q: How can users manage their consent?
A: Users should look for platforms that provide clear options to manage their consent settings easily. This can include toggling data sharing preferences or opting out of certain data collections.
Q: Why is transparency important in AI?
A: Transparency fosters trust between users and AI systems. When users understand how their data is used, they are more likely to engage with the technology and feel secure in its application.
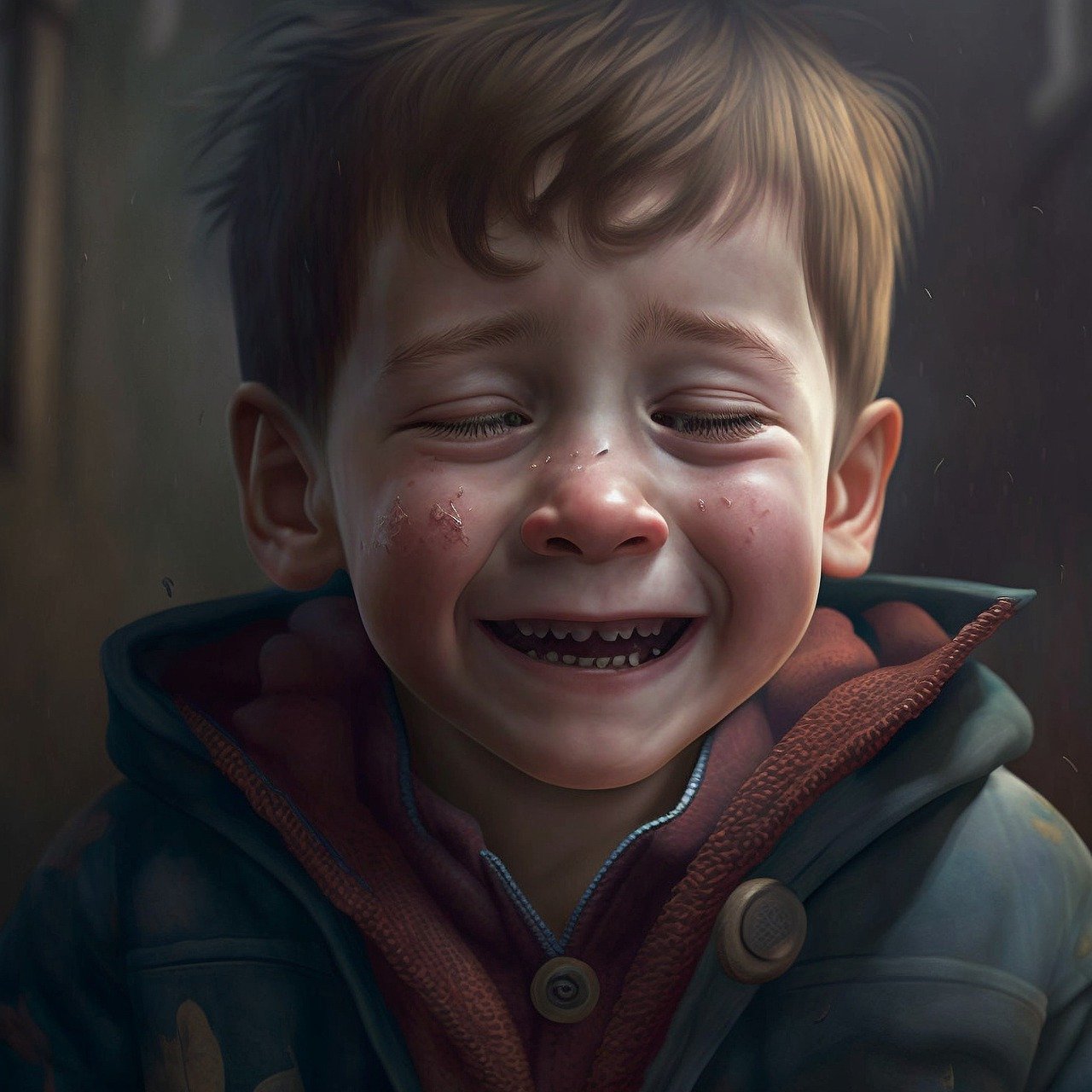
Algorithmic Bias and Fairness
Algorithmic bias is an increasingly pressing issue in the realm of artificial intelligence. It occurs when AI systems produce systematic and unfair outcomes due to prejudiced assumptions in the data or algorithms. Imagine a world where an AI is tasked with hiring candidates, yet it consistently favors one demographic over another. This is not just a hypothetical scenario; it’s a reality that has been observed in various AI applications. The implications of such biases can be profound, leading to significant societal repercussions, including perpetuating stereotypes and widening existing inequalities.
To truly grasp the impact of algorithmic bias, we must first understand its roots. Bias can stem from several sources, such as:
- Data Quality: If the data used to train an AI model is skewed or unrepresentative, the outcomes will reflect those biases.
- Human Bias: AI systems learn from human-generated data, which may contain inherent biases based on societal norms and prejudices.
- Algorithm Design: The way algorithms are structured can inadvertently favor certain groups over others, leading to unfair treatment.
Addressing these biases is not merely a technical challenge; it is a moral imperative. Fairness in AI is about ensuring that all individuals, regardless of their background, have equal opportunities and are treated justly. This raises a critical question: how can we ensure fairness in AI applications? Here are some strategies that can be employed:
- Diverse Data Sets: Utilizing a diverse range of data during the training phase can help mitigate bias.
- Regular Audits: Conducting audits of AI systems can help identify and rectify biases before they lead to negative outcomes.
- Stakeholder Involvement: Engaging diverse stakeholders in the development process can provide broader perspectives and reduce blind spots.
Moreover, fairness is not a one-size-fits-all concept. It requires context and an understanding of the specific domain in which the AI operates. For instance, a healthcare AI system must consider different demographic factors that affect health outcomes, while a loan approval AI should ensure that it does not discriminate against applicants based on race or gender. The challenge lies in defining what fairness means in each context and how to measure it effectively.
In conclusion, tackling algorithmic bias is essential for the ethical deployment of AI technologies. It’s not just about creating advanced algorithms; it’s about creating a fair and just society. By prioritizing fairness and actively working to eliminate biases, we can harness the true potential of AI to benefit everyone, rather than perpetuating existing inequalities. As we move forward, the question remains: are we ready to take responsibility for the algorithms we create?
- What is algorithmic bias? Algorithmic bias refers to systematic and unfair outcomes produced by AI systems due to prejudiced assumptions in the data or algorithms.
- How can algorithmic bias affect society? It can lead to unfair treatment of individuals, perpetuate stereotypes, and widen existing inequalities.
- What are some ways to reduce algorithmic bias? Using diverse data sets, conducting regular audits, and involving diverse stakeholders in the development process can help mitigate bias.
- Why is fairness important in AI? Fairness ensures that all individuals have equal opportunities and are treated justly, which is essential for building trust in AI systems.

Accountability in AI Systems
When we think about accountability in AI systems, it often feels like trying to nail jelly to a wall. The challenge lies in understanding who is responsible when an AI makes a decision that leads to an unintended consequence. Is it the developer who created the algorithm, the company that deployed it, or perhaps even the user who relied on it? These questions are not just academic; they have real-world implications that can affect lives, businesses, and even entire societies.
As AI systems grow more autonomous, the complexity of accountability increases. For instance, if a self-driving car causes an accident, determining liability becomes a challenging puzzle. Is it the manufacturer of the vehicle, the software developer, or the owner of the car? The legal frameworks currently in place are often ill-equipped to handle these scenarios, leading to a gray area where accountability is murky at best.
To illustrate the intricacies of accountability in AI, consider the following table that outlines potential stakeholders and their responsibilities:
Stakeholder | Potential Responsibilities |
---|---|
Developers | Creating algorithms that are fair, unbiased, and transparent. |
Companies | Ensuring ethical deployment and use of AI systems. |
Users | Understanding the limitations and risks associated with AI systems. |
Regulators | Establishing laws and guidelines to govern AI use. |
Moreover, the question of accountability extends beyond just legal implications. It also delves into the realm of ethical responsibilities. If an AI system makes a biased decision, who is accountable for that bias? The answer is not straightforward, but it highlights the importance of designing AI systems that prioritize fairness and equity from the ground up. Developers must actively work to identify and mitigate biases, ensuring that their creations do not perpetuate existing inequalities.
Another crucial aspect of accountability is the need for transparency in AI decision-making processes. When users understand how an AI system arrives at a decision, they are more likely to trust it. This transparency can be achieved through explainable AI, which provides insights into the reasoning behind AI-generated outcomes. For example, if an AI denies a loan application, a transparent system would explain the factors that led to that decision, allowing users to comprehend and, if necessary, contest it.
In conclusion, accountability in AI systems is a multifaceted issue that demands attention from various stakeholders. As AI continues to evolve, so too must our understanding of who is responsible for its actions. By fostering a culture of accountability, we can navigate the complexities of AI and work toward systems that are not only effective but also ethical and just.
- Who is responsible if an AI system makes a mistake?
The responsibility can fall on multiple stakeholders, including developers, companies, and users, depending on the context. - What is explainable AI?
Explainable AI refers to AI systems designed to provide clear and understandable insights into how they make decisions. - Why is transparency important in AI?
Transparency helps build trust and allows users to understand and challenge AI decisions. - How can bias in AI be mitigated?
Bias can be mitigated by implementing diverse datasets, regular auditing, and inclusive development practices.
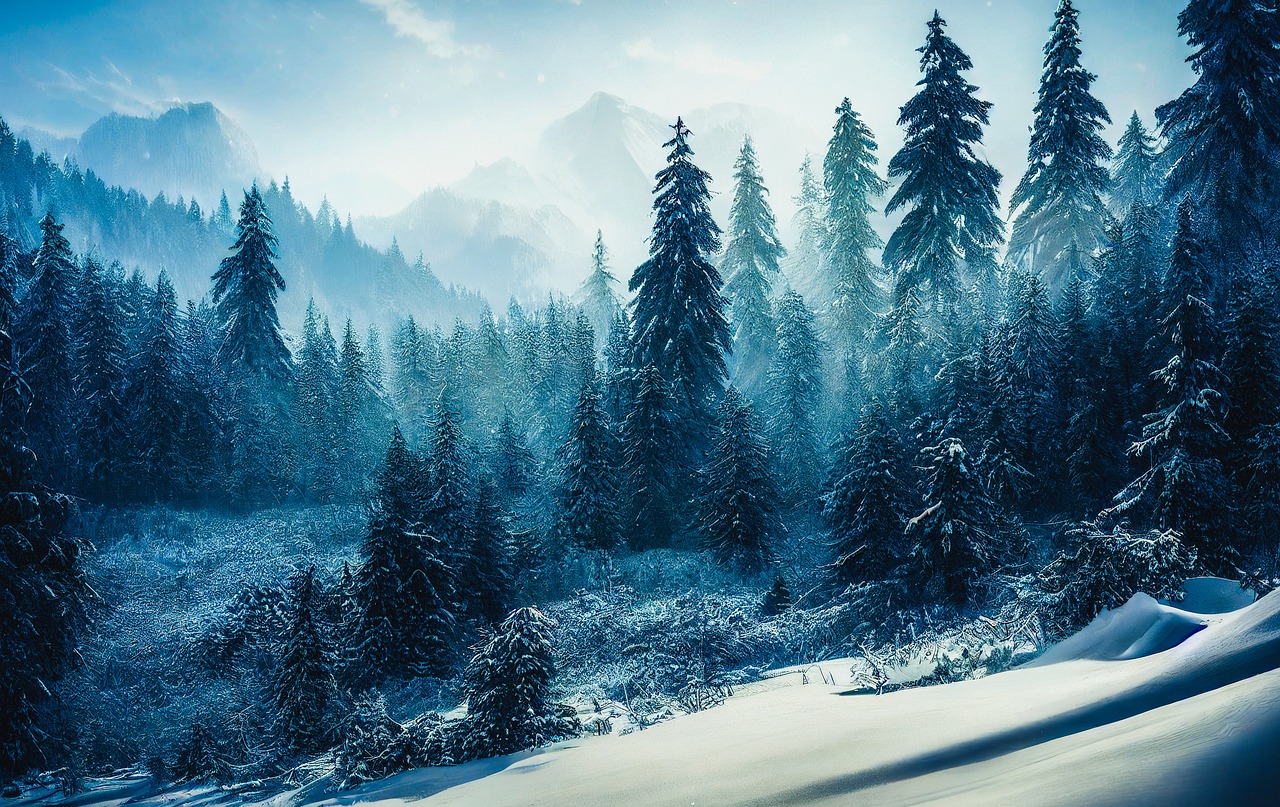
Liability Issues
As we delve deeper into the world of artificial intelligence, one of the most pressing concerns that arises is the question of liability. When an AI system makes a decision that leads to negative outcomes, who is held accountable? Is it the developer, the user, or the AI itself? This complexity stems from the fact that AI systems can operate autonomously, making choices based on algorithms that might not be fully understood even by their creators. This uncertainty can lead to significant legal and ethical dilemmas.
For instance, consider a self-driving car that gets into an accident. The question of liability becomes murky: is it the manufacturer of the vehicle, the software developer, or the owner of the car who should bear the responsibility? In this scenario, there are multiple parties involved, each with varying degrees of control and oversight over the AI system's functioning. This situation can lead to a cascade of legal battles, as each party attempts to deflect blame while victims seek justice.
Moreover, as AI technology continues to evolve, the potential for harm increases. The implications of liability extend beyond physical harm to include financial losses and reputational damage. For example, if an AI system used in financial trading makes erroneous predictions resulting in substantial losses, determining liability becomes crucial. Stakeholders must grapple with questions such as:
- Should the developers be liable for the algorithm's failures?
- What about the users who relied on the AI's advice?
- How do we account for the unpredictable nature of machine learning systems?
To navigate these challenges, some experts advocate for the establishment of clear regulatory frameworks that define liability in AI contexts. These frameworks could help standardize expectations and responsibilities, reducing ambiguity and fostering trust in AI technologies. For instance, regulations could stipulate that developers must ensure a certain level of safety and reliability in their systems, while users must be informed of the risks associated with AI technologies.
Additionally, the concept of insurance is emerging as a potential solution to address liability issues in AI. Just as car insurance protects drivers from financial loss due to accidents, specialized insurance for AI systems could provide coverage for damages caused by AI errors. This approach would not only help mitigate financial risks but also encourage developers to prioritize safety and accountability in their designs.
Ultimately, the conversation around liability in AI is just beginning. As these technologies become more integrated into our daily lives, it is essential for stakeholders—developers, users, and regulators alike—to engage in an ongoing dialogue about responsibility. By addressing these issues head-on, we can work toward a future where AI is not only innovative but also safe and ethical.
- Who is responsible when an AI makes a mistake? Liability can depend on various factors, including the nature of the AI's operation and the relationship between the involved parties.
- Can AI systems be held legally accountable? Currently, AI systems themselves cannot be held accountable; instead, human stakeholders are responsible for their actions.
- What role do regulations play in AI liability? Regulations can help clarify responsibilities and establish standards for safety and accountability in AI systems.
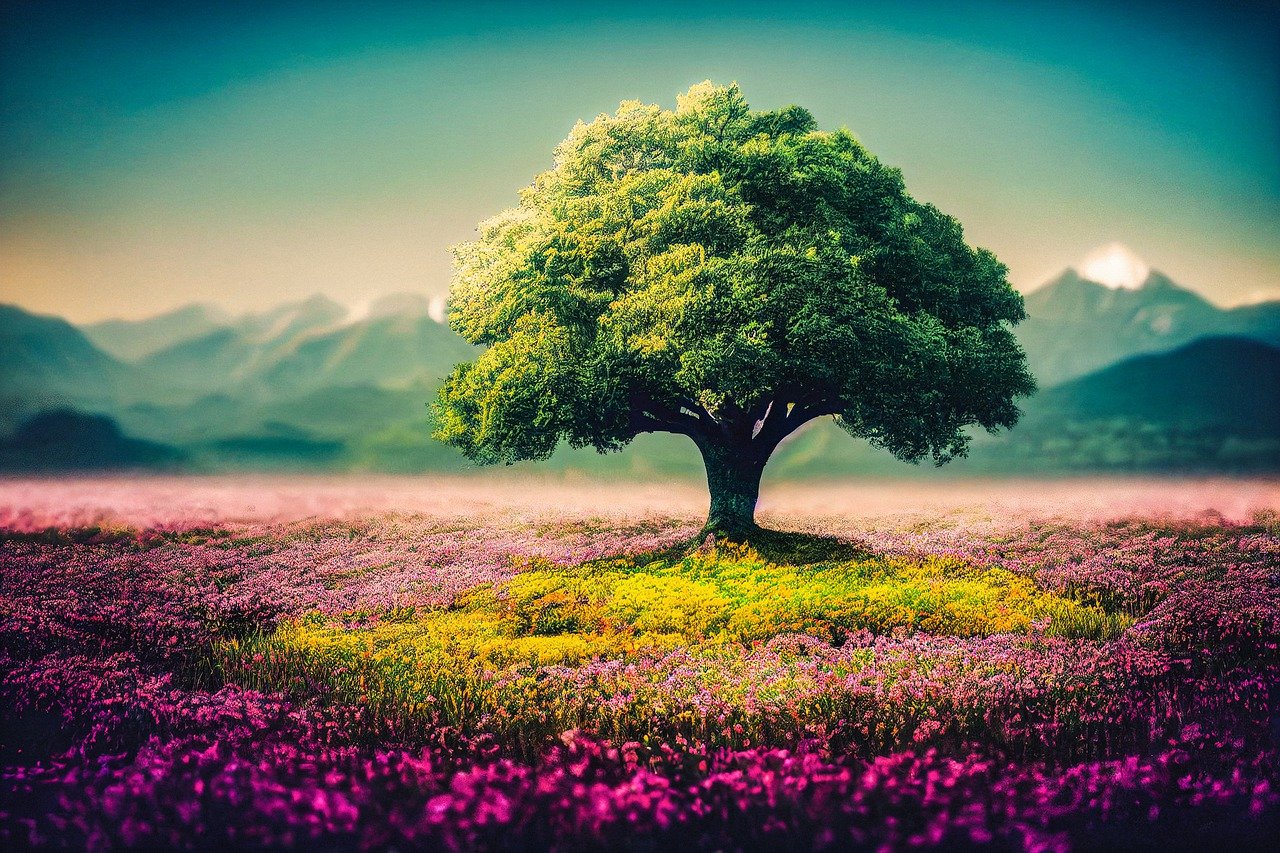
Transparency and Explainability
In the rapidly evolving world of artificial intelligence, transparency and explainability are not just buzzwords; they are essential pillars that support trust and reliability in AI systems. Imagine driving a car with a complex engine that you can't understand—would you feel safe? The same principle applies to AI. When users interact with AI systems, they deserve to know how decisions are made and what factors influence those decisions. This is where transparency comes into play.
Transparency in AI means that the inner workings of the algorithms and the data they use are visible and understandable to users. It answers the fundamental question: How does this AI come to its conclusions? When AI systems are opaque, they can become black boxes, leading to mistrust and skepticism. This lack of clarity can result in severe consequences, especially in critical areas like healthcare, finance, and law enforcement.
To foster trust, AI developers must prioritize explainability. This involves creating models that not only provide outputs but also offer insights into the reasoning behind those outputs. For instance, if an AI model denies a loan application, it should clearly outline the criteria that led to this decision. This could include factors such as credit score, income level, and debt-to-income ratio. By providing this information, users can better understand the process and, if necessary, challenge the decision.
One effective way to enhance transparency is through the use of explainable AI (XAI) techniques. These methods aim to make AI systems more interpretable, allowing users to grasp the rationale behind AI decisions. Some popular XAI techniques include:
- LIME (Local Interpretable Model-agnostic Explanations): This technique helps explain individual predictions by approximating the AI's decision boundary locally.
- SHAP (SHapley Additive exPlanations): SHAP values provide a way to understand the contribution of each feature to a particular prediction.
- Feature Importance: Highlighting which features were most influential in the decision-making process can demystify AI outputs.
Moreover, organizations must consider the ethical implications of their AI systems. By prioritizing transparency and explainability, they not only comply with regulatory requirements but also demonstrate a commitment to ethical practices. This can significantly enhance their reputation and foster customer loyalty.
In conclusion, transparency and explainability are not mere luxuries in AI development; they are necessities. As we continue to integrate AI into our daily lives, understanding how these systems operate will empower users, build trust, and promote responsible AI usage. After all, a well-informed user is a confident user.
Q1: What is the difference between transparency and explainability in AI?
A1: Transparency refers to the clarity of the AI system's processes and data, while explainability focuses on how well the AI can articulate the reasoning behind its decisions.
Q2: Why is explainability important in AI?
A2: Explainability is crucial for building trust, allowing users to understand and challenge AI decisions, which is especially important in high-stakes scenarios.
Q3: How can organizations improve transparency in their AI systems?
A3: Organizations can improve transparency by adopting explainable AI techniques, providing clear documentation, and engaging with users to explain how AI decisions are made.
Frequently Asked Questions
- What are the main vulnerabilities in AI systems?
AI systems can be vulnerable to a range of issues, including data biases, security flaws, and algorithmic errors. These weaknesses can lead to unreliable outputs and unintended consequences, making it crucial to address them during the development process.
- Why is ethical consideration important in AI?
The ethical implications of AI are significant because they directly affect fairness, accountability, and transparency in society. By focusing on these aspects, we can foster trust and ensure that AI technologies are used responsibly and equitably.
- How does data privacy relate to AI?
AI systems often require vast amounts of data, which raises concerns about privacy violations. Understanding how data is collected, stored, and used is essential for protecting individual rights and ensuring that personal information is handled ethically.
- What role do regulatory frameworks play in AI?
Regulatory frameworks are vital for establishing guidelines that safeguard user data and promote ethical AI practices. They help ensure that AI developers adhere to standards that protect users and maintain public trust in AI technologies.
- How can we address algorithmic bias in AI?
Addressing algorithmic bias requires identifying and mitigating biases in AI algorithms. This is fundamental to promoting fairness and equity in AI applications, ensuring that all groups are treated justly and without discrimination.
- Who is accountable for AI decisions?
Determining accountability in AI decision-making is complex. It raises questions about who should be held responsible for the actions of AI systems—be it developers, users, or the AI itself. Understanding these dynamics is crucial for navigating legal and ethical challenges.
- Why is transparency important in AI?
Transparency in AI operations is essential for building trust and understanding among users. When AI systems are explainable, users can better comprehend and challenge AI decisions, leading to more informed interactions with technology.