Artificial Intelligence in Supply Chains: Future Scenario
As we stand on the brink of a technological revolution, artificial intelligence (AI) is poised to transform the landscape of supply chains in ways we could only dream of a few years ago. Imagine a world where logistics are streamlined, costs are slashed, and customer satisfaction reaches new heights—all thanks to the power of AI. This article delves into the multifaceted impact of AI on supply chains, exploring future scenarios that promise to reshape logistics and distribution. From predictive analytics to real-time inventory management, the potential of AI is vast and exciting.
The integration of AI into supply chains is not just about automation; it’s about enhancing human capabilities. Think of AI as a co-pilot that helps businesses navigate the complexities of supply chain management. With its ability to analyze vast amounts of data, AI provides invaluable insights that drive better decision-making. The future of supply chains will be characterized by increased efficiency, reduced operational costs, and improved service levels. As we dive deeper into this topic, we’ll uncover the benefits, challenges, and innovations that will define the next era of supply chains.
One of the most significant advantages of AI in supply chains is its ability to enhance operational efficiency. By automating routine tasks and optimizing processes, businesses can focus on strategic initiatives that drive growth. Moreover, AI-powered tools can predict demand fluctuations, allowing companies to adjust their operations proactively. This not only improves inventory management but also ensures that customers receive their products on time, every time.
However, the journey toward AI-driven supply chains is not without its hurdles. Companies must grapple with data quality issues, the need for skilled personnel, and ethical considerations. As we move forward, it’s essential to address these challenges head-on to unlock the full potential of AI in supply chains. The future is bright, but it requires careful navigation to ensure that innovation does not come at the cost of ethical standards and workforce stability.
- What are the main benefits of AI in supply chains? AI enhances efficiency, reduces costs, and improves decision-making through data analysis.
- How does AI improve demand forecasting? AI uses predictive analytics to anticipate demand fluctuations, optimizing inventory levels and ensuring product availability.
- What challenges do companies face when implementing AI? Companies often encounter data quality issues, a shortage of skilled personnel, and ethical considerations.
- Will AI lead to job displacement? Yes, but it also creates opportunities for reskilling employees for new roles in an evolving supply chain landscape.
- What is the future of AI in supply chains? The future promises continued innovation, including advancements in machine learning, blockchain integration, and enhanced collaboration.
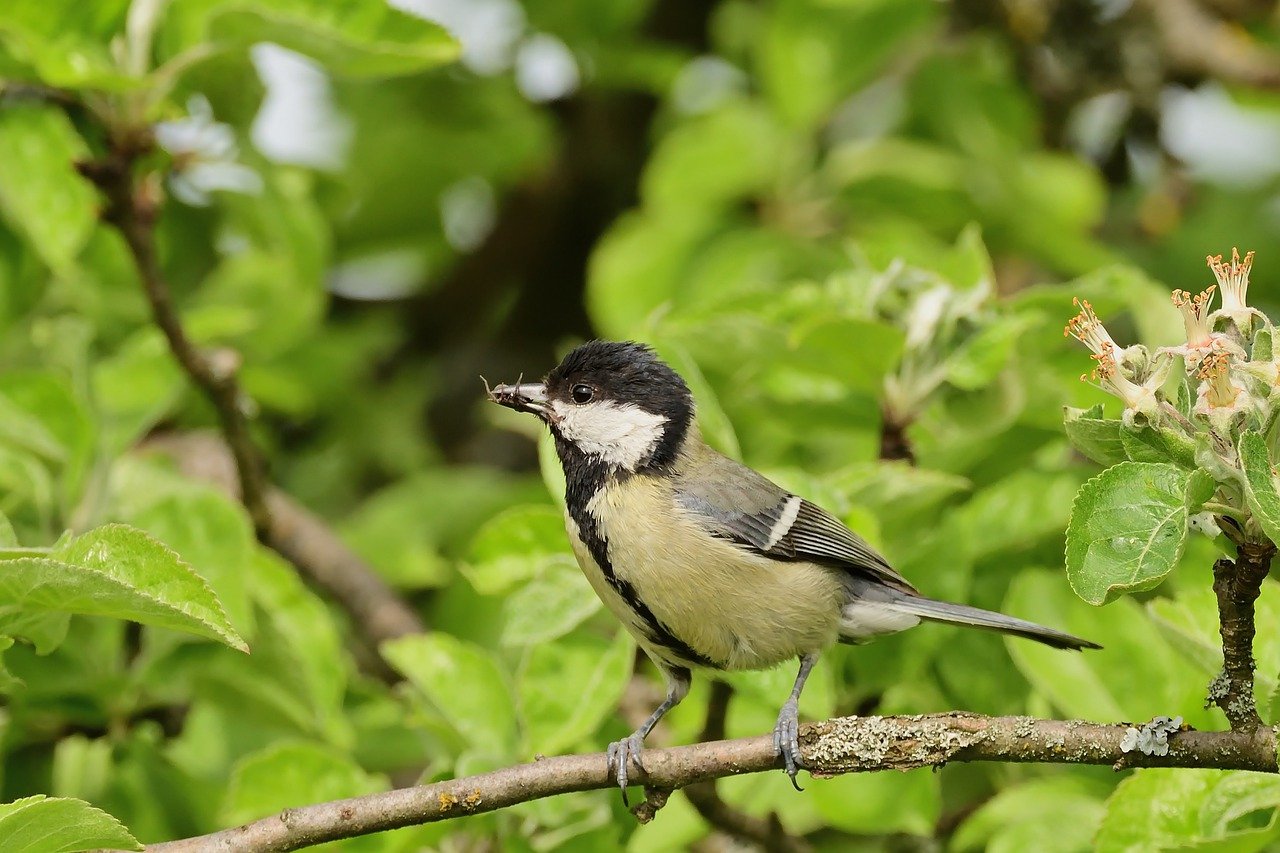
The Role of AI in Supply Chain Optimization
Artificial Intelligence (AI) is not just a buzzword; it is a game changer in the realm of supply chain management. Imagine a world where logistics run like a well-oiled machine, where every decision is backed by data, and inefficiencies are a thing of the past. That's the promise of AI in supply chain optimization. By harnessing the power of AI technologies, businesses can enhance operational efficiency, reduce costs, and make smarter decisions that propel them ahead of the competition.
At its core, AI optimizes supply chains by analyzing vast amounts of data to uncover insights that were previously hidden. This involves using advanced algorithms that can process historical data, current trends, and even external factors like weather patterns or economic shifts. By doing so, companies can not only streamline their operations but also anticipate challenges before they arise. For instance, if a retailer notices a spike in demand for a particular product during a specific season, AI can help them adjust their inventory levels accordingly, ensuring that shelves are stocked just right. This level of foresight is invaluable in today's fast-paced market.
Moreover, AI enhances decision-making processes through predictive modeling. This means that businesses can simulate various scenarios based on different variables, allowing them to choose the best course of action. For example, if a supplier suddenly faces a delay, AI can quickly analyze alternative suppliers, transportation routes, and delivery times to minimize disruption. This agility is crucial in maintaining customer satisfaction and operational continuity.
Another significant advantage of AI in supply chain optimization is its ability to facilitate communication and collaboration across the supply chain ecosystem. By integrating AI tools, stakeholders—from manufacturers to distributors—can share real-time data and insights. This transparency fosters a collaborative environment where everyone is on the same page, reducing the risk of miscommunication and errors. Imagine a scenario where a manufacturer can instantly inform a retailer about production delays or changes in delivery schedules. This proactive approach can save time, reduce costs, and enhance overall efficiency.
To illustrate the impact of AI on supply chain optimization, consider the following table that summarizes key benefits:
Benefit | Description |
---|---|
Enhanced Efficiency | AI streamlines processes, reducing time and resources spent on manual tasks. |
Cost Reduction | By optimizing inventory and logistics, companies can significantly lower operational costs. |
Improved Decision-Making | Data-driven insights allow businesses to make informed decisions quickly. |
Greater Agility | AI enables businesses to respond swiftly to market changes and disruptions. |
Collaboration | Real-time data sharing fosters better communication among supply chain partners. |
In conclusion, the role of AI in supply chain optimization is transformative. As businesses continue to embrace these technologies, the logistics landscape will evolve, becoming more efficient, agile, and responsive to consumer needs. The future of supply chains is bright, and AI is at the forefront of this revolution.
- What is AI in supply chain management? AI in supply chain management refers to the use of artificial intelligence technologies to enhance various aspects of supply chain operations, from inventory management to logistics.
- How does AI improve decision-making in supply chains? AI improves decision-making by providing data-driven insights and predictive analytics, allowing businesses to anticipate challenges and make informed choices.
- What are some challenges of implementing AI in supply chains? Challenges include data quality issues, the need for skilled personnel, and potential resistance to change from employees.
- Can AI help reduce costs in supply chains? Yes, by optimizing inventory levels and streamlining operations, AI can significantly lower operational costs.
- How does AI facilitate collaboration in supply chains? AI facilitates collaboration by enabling real-time data sharing among supply chain partners, fostering better communication and coordination.
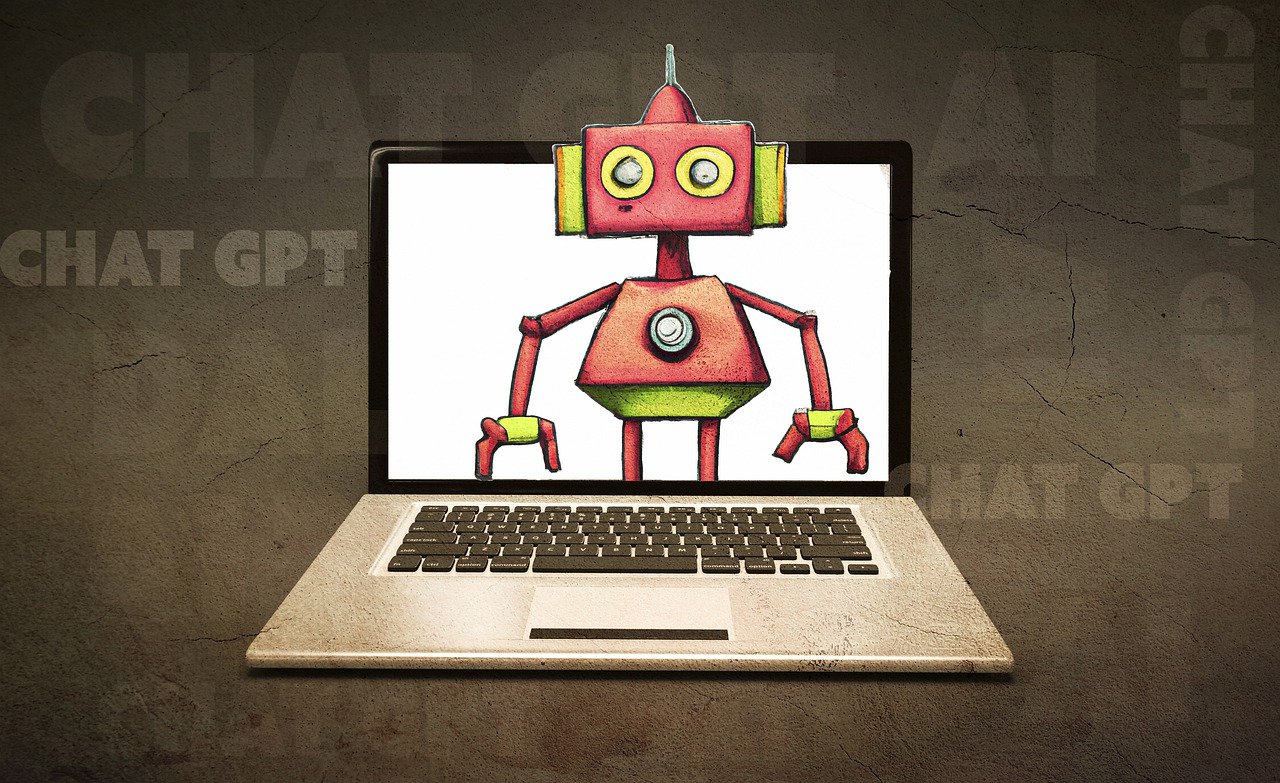
Predictive Analytics and Demand Forecasting
Predictive analytics is like having a crystal ball for businesses, especially in the realm of supply chains. Imagine being able to foresee demand fluctuations before they happen—sounds like magic, right? But with the power of artificial intelligence (AI), this magic is becoming a reality. By leveraging advanced algorithms and vast amounts of data, companies can not only anticipate what products customers will want but also when they will want them. This foresight allows businesses to optimize their inventory levels, ensuring they have the right products available at the right time, which leads to enhanced customer satisfaction.
At the heart of predictive analytics are machine learning algorithms. These algorithms sift through historical data to identify patterns and trends, making informed predictions about future demand. For instance, if a company notices a spike in demand for a specific product during the holiday season based on previous years' data, they can adjust their supply chain strategy accordingly. This proactive approach not only helps in maintaining adequate stock levels but also significantly reduces the risk of overstocking or stockouts, which can be costly.
To make accurate predictions, businesses must tap into a variety of data sources. These can include:
- Market trends
- Consumer behavior analytics
- Seasonal sales data
- Competitor analysis
By combining these diverse data points, companies can enhance the accuracy of their demand forecasts. For example, integrating social media trends with historical sales data can provide insights into emerging consumer preferences, allowing businesses to pivot their strategies swiftly. This level of agility is essential in today’s fast-paced market environment.
However, it's not all smooth sailing. Implementing predictive analytics comes with its own set of challenges. One of the most significant hurdles is the quality of data. If the data being analyzed is flawed or incomplete, the predictions will be off, leading to poor decision-making. Additionally, companies need skilled personnel who can interpret the data effectively and implement the necessary changes in strategy. This requirement for expertise can be a barrier for smaller businesses that may lack the resources to invest in both technology and talent.
In conclusion, predictive analytics and demand forecasting powered by AI are revolutionizing the way businesses operate within their supply chains. By anticipating demand, optimizing inventory, and utilizing diverse data sources, companies are not only improving efficiency but also significantly enhancing customer satisfaction. As we continue to embrace these technologies, the potential for growth and innovation in supply chains will only expand, paving the way for a more responsive and agile marketplace.
Q: What is predictive analytics?
A: Predictive analytics involves using historical data and algorithms to forecast future trends and behaviors, helping businesses make informed decisions.
Q: How can AI improve demand forecasting?
A: AI enhances demand forecasting by analyzing large datasets to identify patterns, which allows businesses to predict customer demand more accurately.
Q: What are some challenges in implementing predictive analytics?
A: Challenges include data quality issues, the need for skilled personnel, and the costs associated with technology investment.
Q: Why is accurate demand forecasting important?
A: Accurate demand forecasting helps businesses maintain optimal inventory levels, reduce costs, and improve customer satisfaction by ensuring product availability.
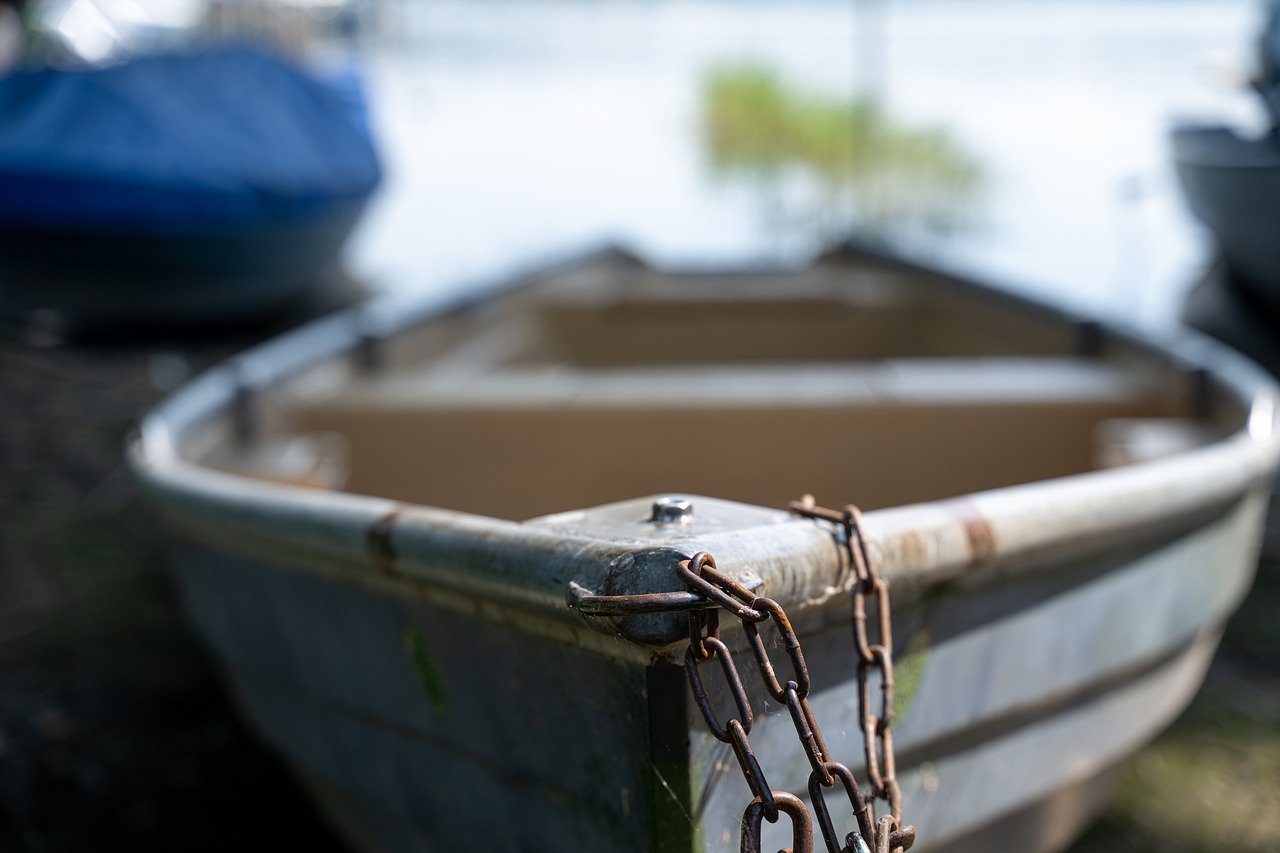
Machine Learning Algorithms
Machine learning algorithms are the backbone of modern predictive analytics in supply chains. They act like astute detectives, sifting through mountains of historical data to uncover hidden patterns that might otherwise go unnoticed. Imagine having a crystal ball that not only shows you what has happened in the past but also predicts what is likely to happen in the future. This is precisely what machine learning does for businesses operating within complex supply chains. By leveraging these algorithms, companies can make informed decisions that enhance their operational efficiency and responsiveness to market demands.
At the heart of machine learning is the ability to learn from data without being explicitly programmed. This means that as more data becomes available, the algorithms can refine their predictions, much like a fine wine maturing over time. They analyze various factors that influence supply chain dynamics, such as seasonality, consumer buying behavior, and even economic indicators. The result? Companies can forecast demand with remarkable accuracy, ensuring they have the right products available at the right time.
To illustrate the power of machine learning algorithms in supply chain management, consider the following table that showcases different types of algorithms and their applications:
Algorithm Type | Application |
---|---|
Regression Analysis | Predicting sales trends based on historical data |
Classification Algorithms | Segmenting customers for targeted marketing |
Clustering Algorithms | Identifying similar products for inventory management |
Time Series Analysis | Forecasting future demand based on past sales |
These algorithms are not just theoretical; they are actively used by companies to optimize their supply chains. For example, a retail company might use regression analysis to predict which products will sell best during the holiday season, allowing them to stock accordingly. Similarly, time series analysis can help in anticipating demand surges during special events or promotions, ensuring that stock levels are sufficient to meet customer needs.
However, it’s essential to recognize that while machine learning algorithms are powerful, they are not without challenges. The effectiveness of these algorithms heavily relies on the quality and quantity of the data fed into them. If the data is flawed or incomplete, the predictions can be misleading. Additionally, businesses must invest in skilled personnel who can manage these sophisticated systems and interpret the results accurately. This brings us to a critical point: the need for organizations to foster a culture of continuous learning and adaptation, ensuring that they can keep pace with the rapidly evolving landscape of artificial intelligence in supply chains.
In conclusion, machine learning algorithms serve as a vital tool in the arsenal of supply chain management. They enable businesses to not only react to changes in demand but also to anticipate them, transforming reactive strategies into proactive ones. As companies continue to harness the power of these algorithms, the potential for innovation and efficiency within supply chains is boundless. The future is bright for those who embrace this technology, as it holds the key to unlocking unprecedented levels of operational excellence.
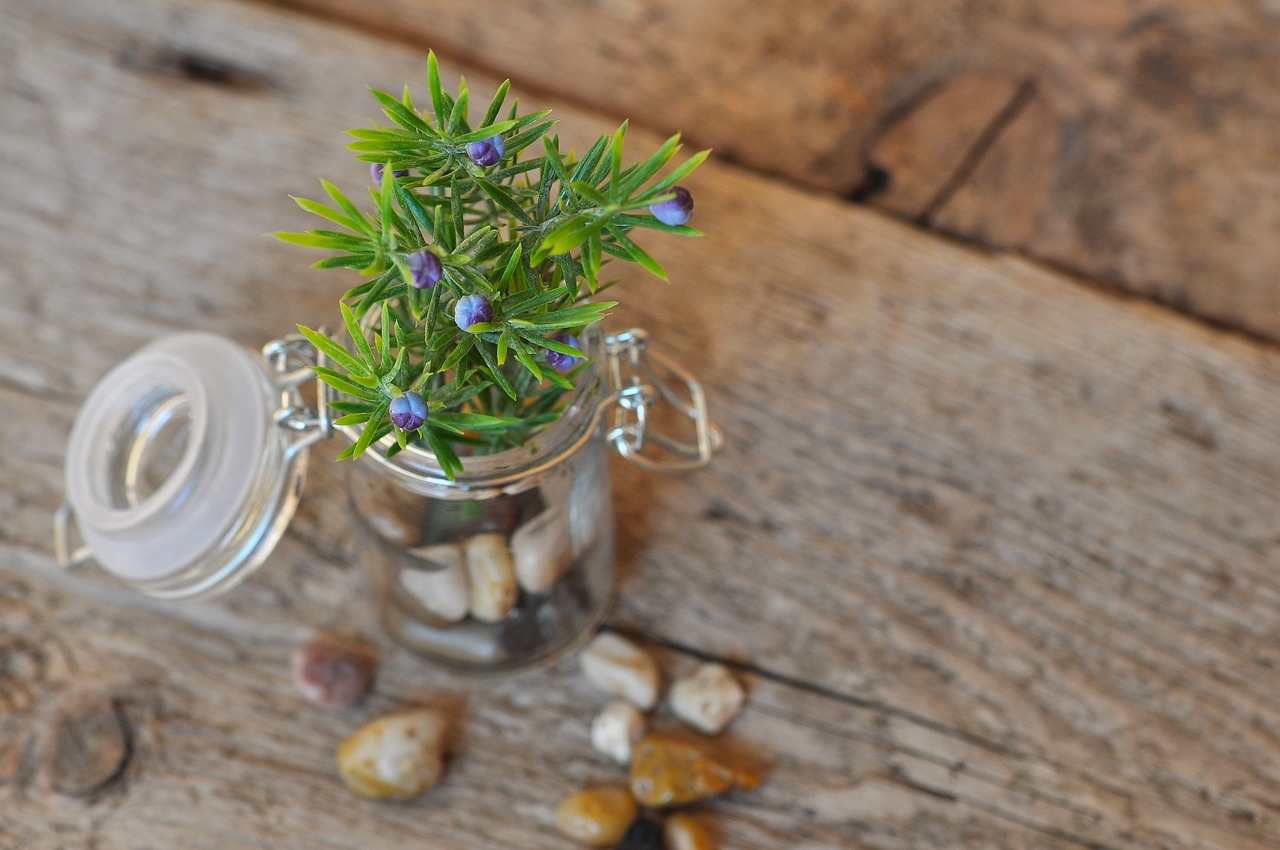
Data Sources for Accurate Forecasting
In the rapidly evolving world of supply chain management, the accuracy of demand forecasting can make or break a business. Data sources play a crucial role in this process, as they provide the necessary insights to predict future trends and consumer behavior. By leveraging a multitude of data points, companies can enhance their forecasting models and ensure they meet customer demands effectively.
One of the primary sources of data for accurate forecasting is historical sales data. Analyzing past sales figures allows businesses to identify patterns and trends over time. For instance, seasonal fluctuations in demand can be spotted, enabling companies to prepare in advance. However, relying solely on historical data can lead to missed opportunities, especially if market conditions change unexpectedly.
Additionally, market trends are vital for forecasting accuracy. Keeping an eye on industry reports, news articles, and economic indicators can provide insights into emerging trends that may affect consumer behavior. For example, if a new technology is gaining popularity, businesses can adjust their inventory accordingly to capitalize on the trend.
Another essential data source is consumer behavior analytics. With the rise of e-commerce, companies have access to a wealth of information regarding customer preferences and purchasing habits. By analyzing data from websites, social media, and customer feedback, businesses can better understand what their customers want and when they want it. This information can be invaluable for fine-tuning inventory levels and ensuring product availability.
Furthermore, integrating external data sources, such as weather forecasts and economic indicators, can significantly enhance forecasting accuracy. For example, a sudden change in weather can affect the demand for certain products, such as winter clothing or air conditioning units. By incorporating this external data into their forecasting models, companies can make more informed decisions.
To illustrate the importance of these data sources, consider the following table that summarizes various data sources and their impacts on forecasting:
Data Source | Impact on Forecasting |
---|---|
Historical Sales Data | Identifies past trends and patterns. |
Market Trends | Highlights emerging consumer interests and shifts. |
Consumer Behavior Analytics | Provides insights into preferences and purchasing habits. |
External Data (Weather, Economic Indicators) | Enhances accuracy by considering external factors. |
In conclusion, the integration of diverse data sources is essential for achieving accurate demand forecasting in supply chains. By harnessing the power of historical data, market trends, consumer behavior analytics, and external factors, businesses can not only improve their forecasting capabilities but also enhance overall operational efficiency. As the landscape of supply chain management continues to evolve, companies that adapt and utilize these data sources effectively will undoubtedly gain a competitive edge.
- What are the key benefits of using data for forecasting? Utilizing data for forecasting helps businesses anticipate demand, optimize inventory, and enhance customer satisfaction.
- How can external factors affect demand forecasting? External factors such as weather changes or economic shifts can significantly influence consumer behavior and purchasing patterns.
- Why is historical data important for forecasting? Historical data allows businesses to identify trends and patterns over time, helping them make informed predictions about future demand.
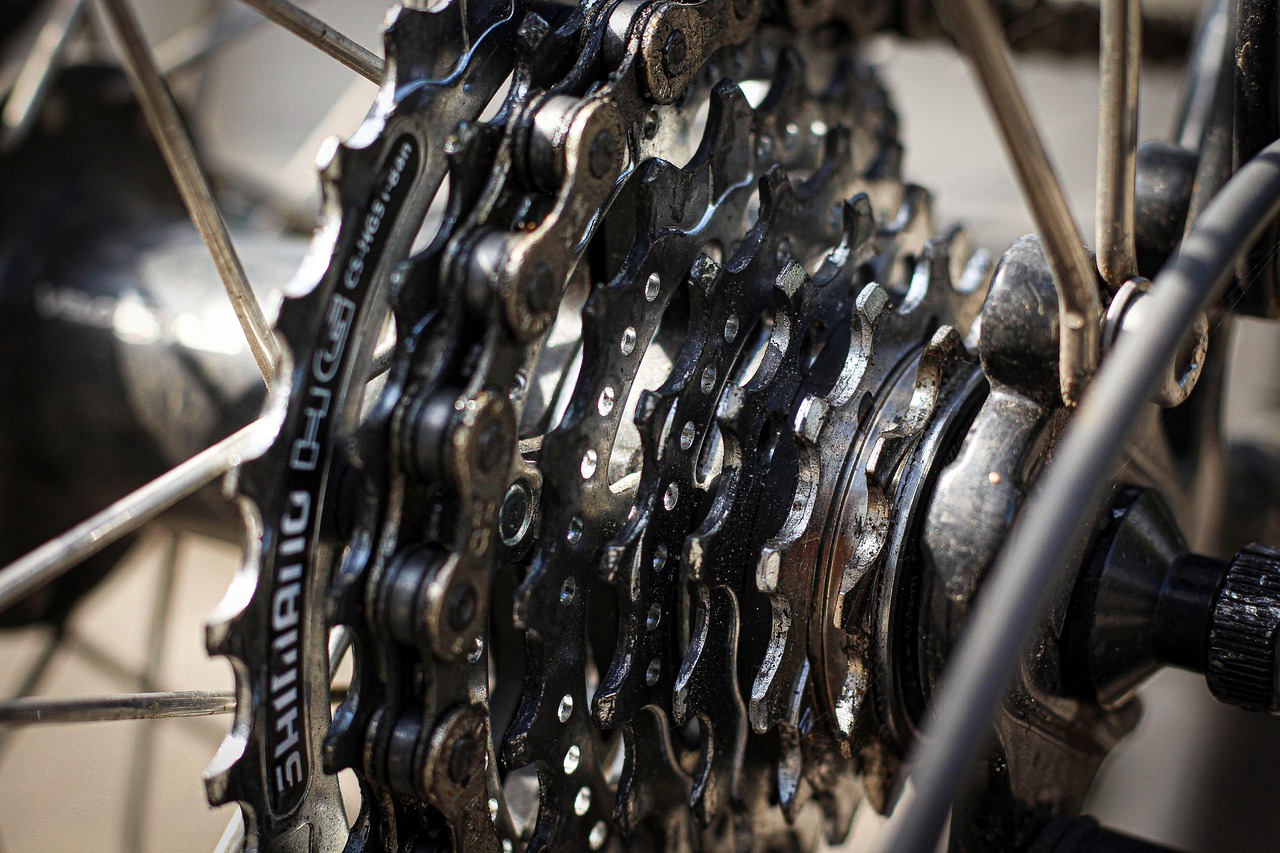
Challenges in Implementation
Implementing predictive analytics in supply chains is not without its hurdles. One of the most significant challenges companies face is ensuring the quality of data. Inaccurate, incomplete, or outdated data can lead to flawed predictions, which in turn can disrupt inventory management and customer satisfaction. Imagine trying to navigate a ship without a reliable map; that’s what businesses experience when they rely on poor data. To combat this, organizations must invest in robust data management systems that ensure the information feeding into their AI models is both accurate and timely.
Another challenge arises from the need for skilled personnel. The implementation of AI technologies requires a workforce that understands both the technology itself and the nuances of supply chain dynamics. This skill gap can be daunting. Companies may find themselves in a race against time to train existing employees or recruit new talent who are proficient in data analytics and machine learning. It’s akin to trying to build a high-tech car without having the right mechanics on hand; you can have all the fancy parts, but without skilled hands, it won’t run smoothly.
Moreover, the integration of AI into existing systems can be a complex process. Many organizations operate on legacy systems that are not easily compatible with modern AI tools. This can lead to significant operational disruptions during the transition phase. Companies must therefore plan meticulously, often requiring a phased approach to implementation that allows for testing and adjustments along the way.
Lastly, there’s the issue of change management. Employees may be resistant to adopting new technologies, fearing job loss or feeling overwhelmed by new processes. To counteract this, it’s essential for leadership to foster a culture of innovation and adaptability, emphasizing the benefits of AI and providing training to ease the transition. It’s not just about implementing new technology; it’s about bringing the team along for the ride and ensuring that everyone understands their role in this new landscape.
In summary, while the potential of AI in supply chains is immense, the challenges of implementation must be addressed with careful planning and a strategic approach. By focusing on data quality, investing in talent, ensuring compatibility with existing systems, and managing change effectively, organizations can navigate these hurdles and unlock the full benefits of AI.
- What are the primary challenges in implementing AI in supply chains? The main challenges include data quality issues, the need for skilled personnel, integration with legacy systems, and resistance to change among employees.
- How can companies ensure data quality for AI applications? Companies can invest in robust data management systems and regularly audit their data sources to maintain accuracy and relevance.
- What skills are necessary for employees to adapt to AI technologies? Employees should have a strong understanding of data analytics, machine learning, and the specific dynamics of the supply chain.
- How can organizations manage employee resistance to AI? By fostering a culture of innovation and providing comprehensive training, organizations can help employees understand the benefits of AI and their role in the new system.

Real-Time Inventory Management
In today's fast-paced business environment, has emerged as a crucial component of effective supply chain operations. Imagine a world where businesses can instantly see their stock levels, track shipments, and manage replenishment processes with the click of a button. This is not just a dream; it's a reality made possible by the integration of artificial intelligence (AI) into inventory management systems. By leveraging AI technologies, companies can achieve unprecedented visibility and control over their inventory, leading to enhanced efficiency and reduced operational costs.
One of the key advantages of real-time inventory management is the ability to minimize waste. Traditional inventory methods often rely on periodic audits and estimates, which can lead to overstocking or stockouts. AI-powered systems, on the other hand, provide continuous tracking of stock levels, allowing businesses to maintain optimal inventory levels. This is particularly important for industries with perishable goods, where timely management can prevent significant losses. As a result, companies can not only improve their bottom line but also contribute to sustainability by reducing waste.
Moreover, the integration of AI in inventory management facilitates better decision-making. By analyzing real-time data, businesses can identify trends and patterns that inform strategic planning. For instance, if an AI system detects a sudden spike in demand for a particular product, it can automatically alert managers to adjust their inventory levels accordingly. This proactive approach not only enhances customer satisfaction by ensuring product availability but also strengthens the overall supply chain resilience.
However, implementing real-time inventory management systems is not without its challenges. Companies must invest in advanced technology and ensure that their staff is adequately trained to use these systems effectively. Additionally, the accuracy of the data being fed into the AI algorithms is paramount. Poor data quality can lead to incorrect forecasts and decisions, which can ultimately harm the business. Therefore, organizations must prioritize data integrity and invest in robust data management practices.
To illustrate the impact of real-time inventory management, consider the following table that compares traditional inventory management practices with AI-driven approaches:
Aspect | Traditional Inventory Management | AI-Driven Inventory Management |
---|---|---|
Data Visibility | Periodic audits and estimates | Continuous real-time tracking |
Responsiveness | Reactive adjustments | Proactive decision-making |
Waste Reduction | High risk of overstocking/stockouts | Optimized inventory levels |
Customer Satisfaction | Inconsistent product availability | Enhanced availability and service |
As we move forward, the importance of real-time inventory management will only grow. Businesses that adopt AI-driven solutions will not only improve their operational efficiency but also gain a competitive edge in the marketplace. In an era where customer expectations are at an all-time high, being able to deliver products swiftly and accurately is essential for success.
- What is real-time inventory management?
Real-time inventory management refers to the continuous tracking and management of stock levels using advanced technologies like AI, allowing businesses to make informed decisions quickly. - How does AI improve inventory management?
AI enhances inventory management by providing real-time data analysis, optimizing stock levels, and predicting demand fluctuations, which leads to improved efficiency and customer satisfaction. - What challenges are associated with implementing AI in inventory management?
Challenges include the need for high-quality data, investment in technology, and training staff to effectively utilize AI systems. - Can real-time inventory management reduce waste?
Yes, by maintaining optimal inventory levels and preventing overstocking or stockouts, businesses can significantly reduce waste, especially in industries dealing with perishable goods.
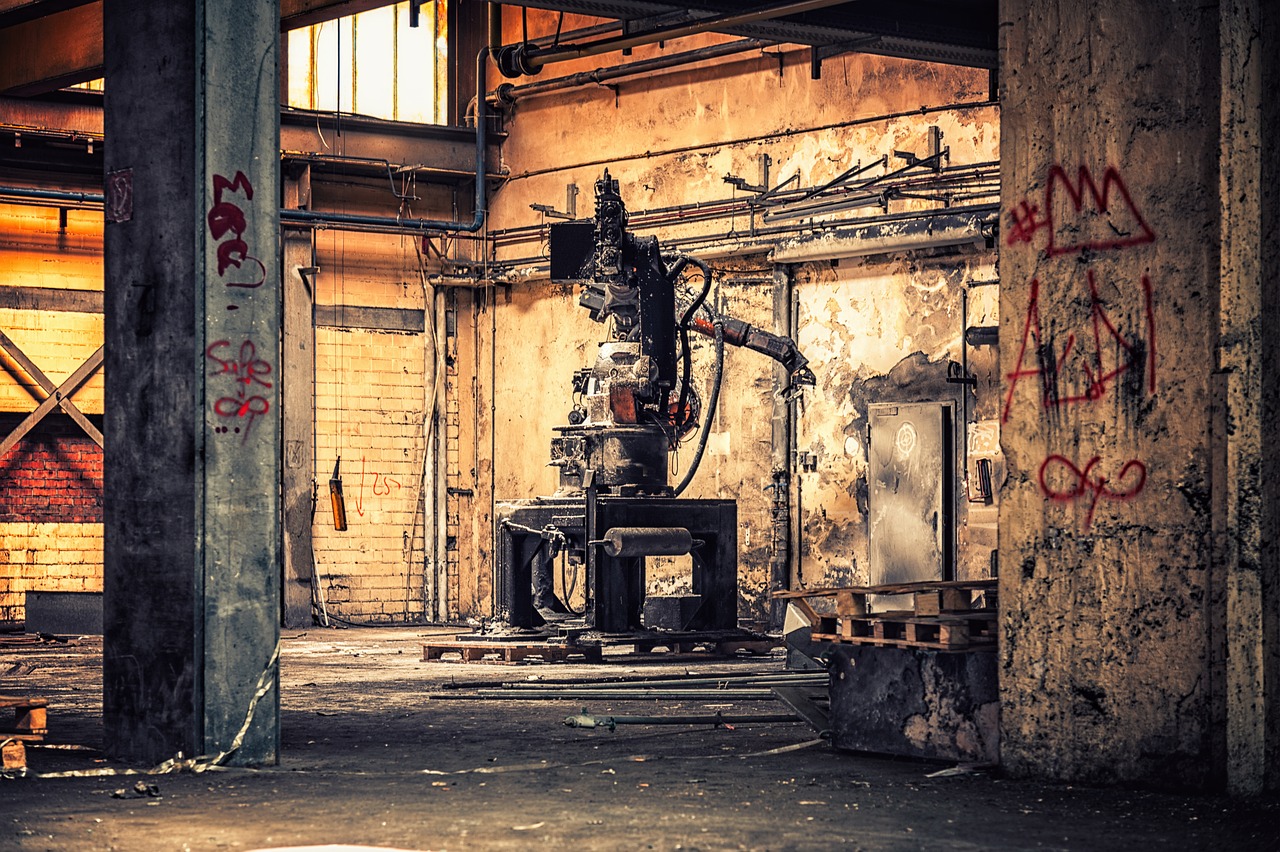
AI-Driven Robotics and Automation
In today's fast-paced world, are not just buzzwords; they're the backbone of modern supply chains. Imagine a bustling warehouse where robots whiz by, efficiently managing inventory while humans focus on strategic tasks. This isn't a scene from a sci-fi movie; it's happening right now! The integration of artificial intelligence into robotics is transforming supply chain operations, making them more efficient, cost-effective, and less prone to human error.
One of the most significant advantages of AI in robotics is the enhancement of operational efficiency. By automating repetitive tasks, businesses can reduce labor costs and minimize errors that often occur in manual processes. For instance, consider the traditional method of order picking, where employees manually sift through aisles to find products. With AI-powered robots, this process becomes a breeze. These robots can swiftly navigate through the warehouse, locate items, and even deliver them to packing stations, all without breaking a sweat!
Moreover, AI-driven robotics are not limited to just warehouses. They are also making waves in the last-mile delivery segment. Imagine ordering a package and having it delivered by a drone or an autonomous vehicle that uses AI to optimize its route. This innovation is revolutionizing how goods reach consumers, enhancing delivery speed and reliability. The ability of these machines to analyze traffic patterns and weather conditions in real-time allows for smarter, faster deliveries, ensuring customers receive their products when they want them.
However, the transition to AI-driven robotics isn't without its challenges. Companies must invest in the right technology and ensure their workforce is prepared for this shift. Training employees to work alongside robots and understanding how to leverage these technologies effectively is crucial. It's not just about replacing human labor; it's about creating a collaborative environment where humans and machines can thrive together.
To give you a clearer picture of how AI-driven robotics are reshaping the landscape, let’s take a look at some key areas where they are making a significant impact:
Area of Impact | Description |
---|---|
Warehouse Operations | Automation of inventory management and order fulfillment processes. |
Last-Mile Delivery | Use of drones and autonomous vehicles to enhance delivery efficiency. |
Cost Reduction | Lower labor costs and reduced errors lead to significant savings. |
Enhanced Accuracy | AI algorithms improve the precision of inventory tracking and order fulfillment. |
As we look towards the future, it's clear that AI-driven robotics and automation will continue to evolve. Companies that embrace these technologies will not only streamline their operations but also gain a competitive edge in the ever-changing marketplace. The question remains: are you ready to embrace the robotic revolution?
- What are the primary benefits of AI-driven robotics in supply chains? AI-driven robotics enhance efficiency, reduce costs, and improve accuracy in operations.
- How do AI robots impact last-mile delivery? They optimize delivery routes and increase speed and reliability through autonomous technology.
- What challenges do companies face when implementing AI robotics? Companies must invest in technology, train employees, and manage the transition effectively.
- Will AI-driven robotics replace human jobs? While some jobs may be automated, new roles will emerge that focus on managing and collaborating with these technologies.
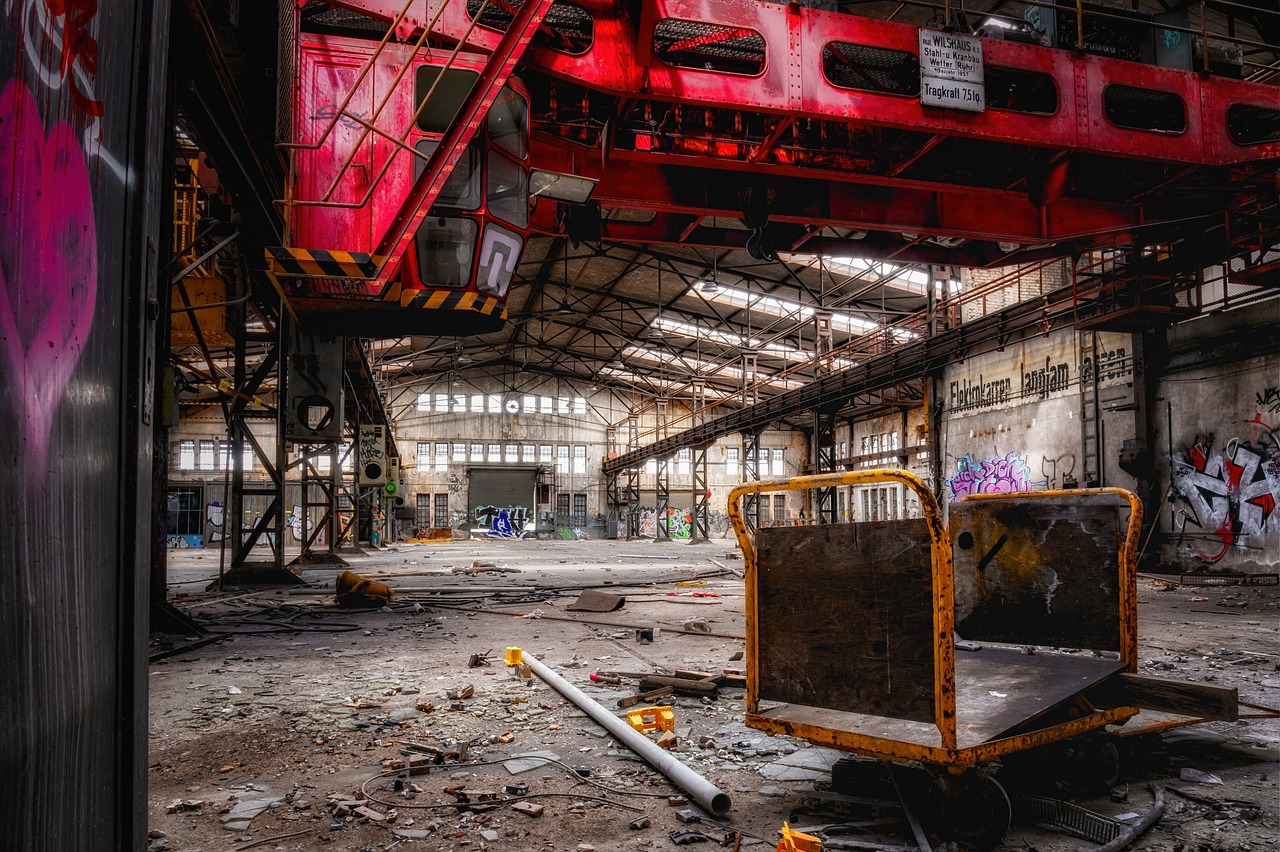
Warehouse Automation
Warehouse automation is revolutionizing the logistics industry, transforming the way goods are stored, picked, and shipped. By integrating advanced technologies such as robotics, artificial intelligence (AI), and the Internet of Things (IoT), businesses are able to streamline their operations and significantly enhance efficiency. Imagine a bustling warehouse where robots glide effortlessly along aisles, picking items with precision, while human workers focus on more strategic tasks. This is not just a futuristic dream; it’s happening right now!
One of the primary benefits of warehouse automation is the increased speed of order fulfillment. Automated systems can process orders much faster than human workers, reducing the time it takes to pick, pack, and ship products. For example, a fully automated warehouse can fulfill orders in mere minutes, compared to the hours it might take in a traditional setup. This speed not only enhances customer satisfaction but also gives companies a competitive edge in the market.
Moreover, the accuracy of order fulfillment is vastly improved through automation. With advanced picking technologies, such as robotic arms and automated guided vehicles (AGVs), the risk of human error is minimized. This means fewer mistakes in order picking, leading to lower return rates and happier customers. In fact, studies have shown that automated systems can achieve accuracy rates of over 99%, a feat that is difficult to match with manual operations.
Another critical aspect of warehouse automation is its ability to optimize space utilization. Automated storage and retrieval systems (AS/RS) can maximize vertical space and organize inventory in a way that human workers might find challenging. By utilizing high-density storage solutions, companies can store more products in less space, ultimately reducing overhead costs. This is especially important in urban areas where real estate is at a premium.
However, transitioning to an automated warehouse is not without its challenges. The initial investment can be substantial, and companies must carefully evaluate their return on investment (ROI). Additionally, integrating new technologies with existing systems can be complex, requiring skilled personnel who understand both the technology and the logistics involved. As with any major change, it’s crucial for businesses to approach automation with a well-thought-out strategy.
In conclusion, warehouse automation is a game-changer in the supply chain landscape. By enhancing speed, accuracy, and space utilization, businesses can not only meet the growing demands of consumers but also prepare for future challenges in the logistics sector. As technology continues to evolve, the possibilities for warehouse automation are limitless, paving the way for smarter, more efficient supply chains.
- What is warehouse automation? Warehouse automation refers to the use of technology and systems to automate processes within a warehouse, including storage, retrieval, and order fulfillment.
- What are the benefits of warehouse automation? The benefits include increased speed and accuracy of order fulfillment, optimized space utilization, and reduced labor costs.
- What technologies are used in warehouse automation? Common technologies include robotics, automated guided vehicles (AGVs), and automated storage and retrieval systems (AS/RS).
- Are there challenges in implementing warehouse automation? Yes, challenges can include high initial costs, the complexity of integration with existing systems, and the need for skilled personnel.
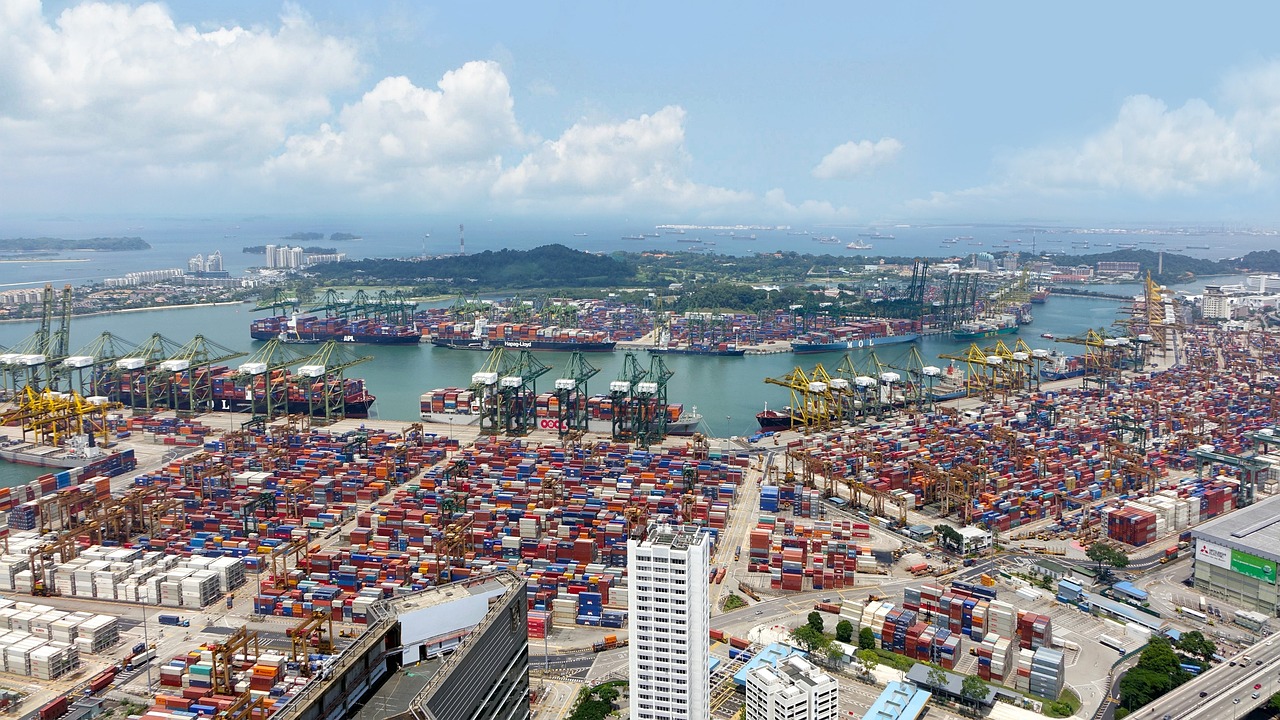
Last-Mile Delivery Innovations
In the ever-evolving world of supply chains, last-mile delivery has become a critical focus for businesses aiming to enhance customer satisfaction and operational efficiency. This last leg of the delivery journey is where products transition from distribution centers to the end consumer, and it often represents the most expensive and complex segment of the supply chain. As consumer expectations rise, fueled by the convenience of e-commerce, companies are turning to innovative solutions powered by artificial intelligence to streamline this process.
One of the most exciting advancements in last-mile delivery is the use of autonomous vehicles and drones. These technologies are not just futuristic concepts; they are becoming a reality in urban and suburban areas. Imagine a scenario where a drone swiftly navigates through the skies, delivering packages directly to your doorstep. This not only speeds up delivery times but also reduces the carbon footprint associated with traditional delivery trucks. Companies like Amazon and Google are already testing these innovations, pushing the boundaries of what we thought was possible.
Moreover, AI algorithms are optimizing delivery routes in real-time. By analyzing traffic patterns, weather conditions, and even local events, these systems can determine the most efficient paths for delivery vehicles. This means that packages can arrive faster and with less fuel consumption. For instance, a delivery driver might receive an update mid-route, suggesting an alternate path that avoids a traffic jam, thus ensuring timely delivery. The integration of machine learning in route optimization is a game-changer, transforming how businesses operate.
Another significant innovation in last-mile delivery is the concept of smart lockers. These secure, self-service kiosks allow customers to pick up their packages at their convenience. Instead of waiting at home for a delivery that may arrive late, customers can retrieve their items from a locker located in a nearby grocery store or shopping center. This approach not only enhances convenience but also reduces the number of failed delivery attempts, which can be costly for businesses. Smart lockers are becoming increasingly popular, especially in urban areas where delivery challenges are more pronounced.
However, while these innovations hold great promise, they also come with challenges. The implementation of autonomous vehicles and drones raises questions about regulatory compliance and safety. Companies must navigate a complex landscape of regulations that vary by region, ensuring that their delivery methods meet legal standards while also addressing public safety concerns. Additionally, the reliance on technology can lead to vulnerabilities, such as potential system failures or cybersecurity threats.
In conclusion, the future of last-mile delivery looks bright, with AI-driven innovations paving the way for enhanced efficiency and customer satisfaction. As these technologies continue to evolve, we can expect to see even more creative solutions that address the challenges of this critical supply chain segment. The question remains: Are we ready to embrace these changes and adapt our logistics strategies accordingly?
- What is last-mile delivery? Last-mile delivery refers to the final step of the delivery process where goods are transported from a distribution center to the end consumer.
- How is AI used in last-mile delivery? AI is utilized in last-mile delivery through route optimization, autonomous vehicles, and delivery drones, enhancing efficiency and reducing costs.
- What are smart lockers? Smart lockers are secure kiosks that allow customers to pick up their packages at their convenience, reducing failed delivery attempts.
- What challenges do businesses face in last-mile delivery? Businesses face challenges such as regulatory compliance, safety concerns, and potential cybersecurity threats while implementing new delivery technologies.
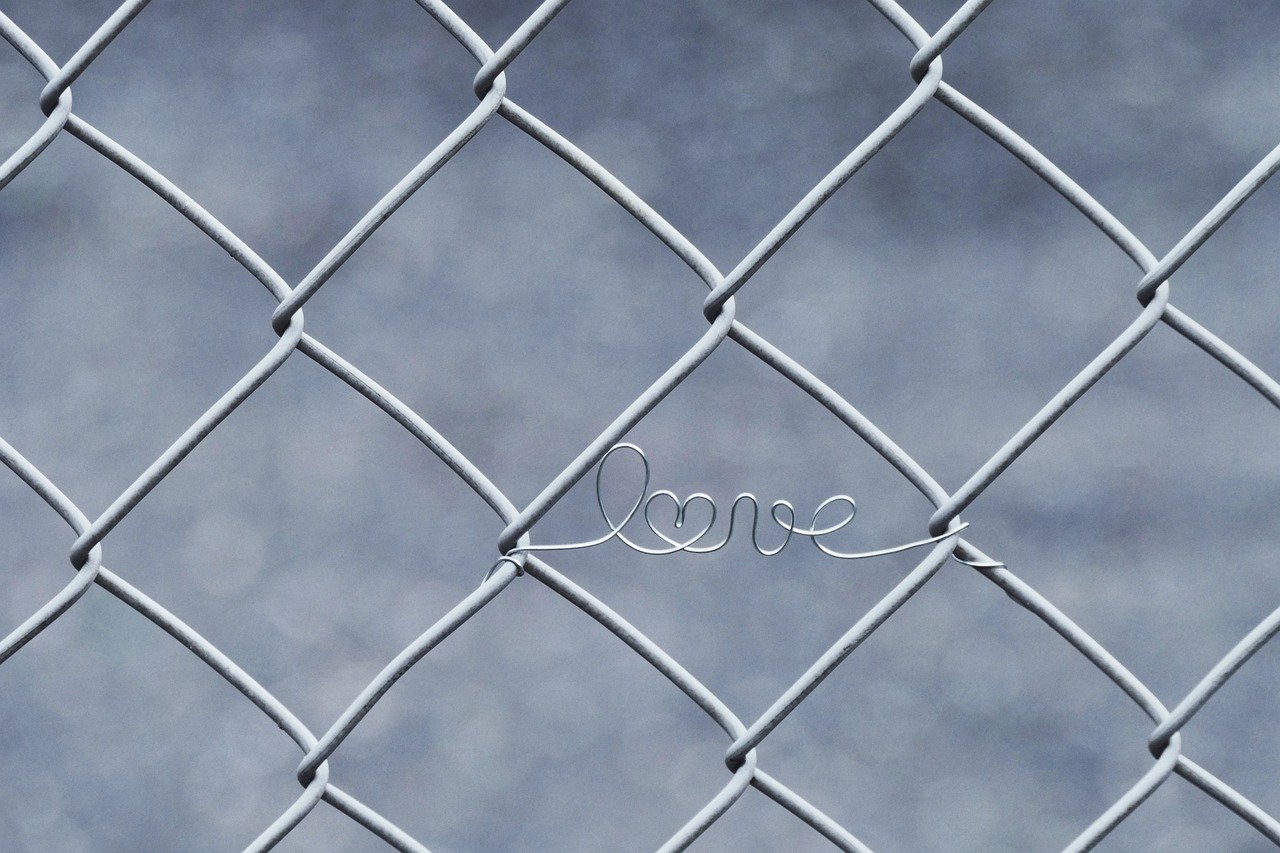
Challenges and Ethical Considerations
While the integration of artificial intelligence (AI) into supply chains offers a plethora of advantages, it is crucial to address the that come with it. As we embrace this technological revolution, we must be aware of the potential pitfalls that could disrupt the very systems we aim to improve. One of the most pressing concerns is data privacy. With the increasing digitization of supply chain processes, vast amounts of sensitive data are being collected and analyzed. This raises significant questions about how this data is stored, who has access to it, and how it is protected from breaches. Companies must ensure they comply with regulations such as GDPR and CCPA, which emphasize the importance of safeguarding personal information.
Another challenge that cannot be overlooked is the potential for job displacement. As AI technologies automate various tasks, there is a legitimate fear that many employees may find themselves out of work. This reality necessitates a proactive approach to workforce management, where businesses must invest in reskilling programs to help employees transition into new roles that AI cannot easily replicate. It’s like teaching an old dog new tricks; the goal is to adapt and thrive in a changing environment rather than be left behind.
Moreover, the ethical implications of automated decision-making present a complex dilemma. When AI systems make decisions based on algorithms, there is a risk of bias creeping into these processes. For instance, if the training data used to develop these algorithms contains biases, the outcomes could disproportionately affect certain groups. This raises the question: how do we ensure that AI operates fairly and transparently? Establishing clear guidelines and ethical standards for AI deployment in supply chains is essential to mitigate these risks.
To summarize, while AI holds the key to a more efficient and optimized supply chain, it also brings forth challenges that require careful consideration. Companies must navigate the murky waters of data privacy, workforce displacement, and ethical decision-making to harness AI's full potential responsibly. The future of supply chains will not only be defined by technological advancements but also by how we address these critical issues.
- What are the main challenges of implementing AI in supply chains?
The primary challenges include data privacy concerns, potential job displacement, and the ethical implications of automated decision-making. - How can companies protect sensitive data in supply chains?
Companies can protect data by implementing robust cybersecurity measures, ensuring compliance with data protection regulations, and conducting regular audits. - What strategies can help mitigate job displacement due to AI?
Strategies include investing in reskilling programs, offering training for new technologies, and creating new job opportunities within the organization. - How can bias in AI algorithms be addressed?
Addressing bias involves using diverse training data, regularly testing algorithms for fairness, and establishing ethical guidelines for AI development.
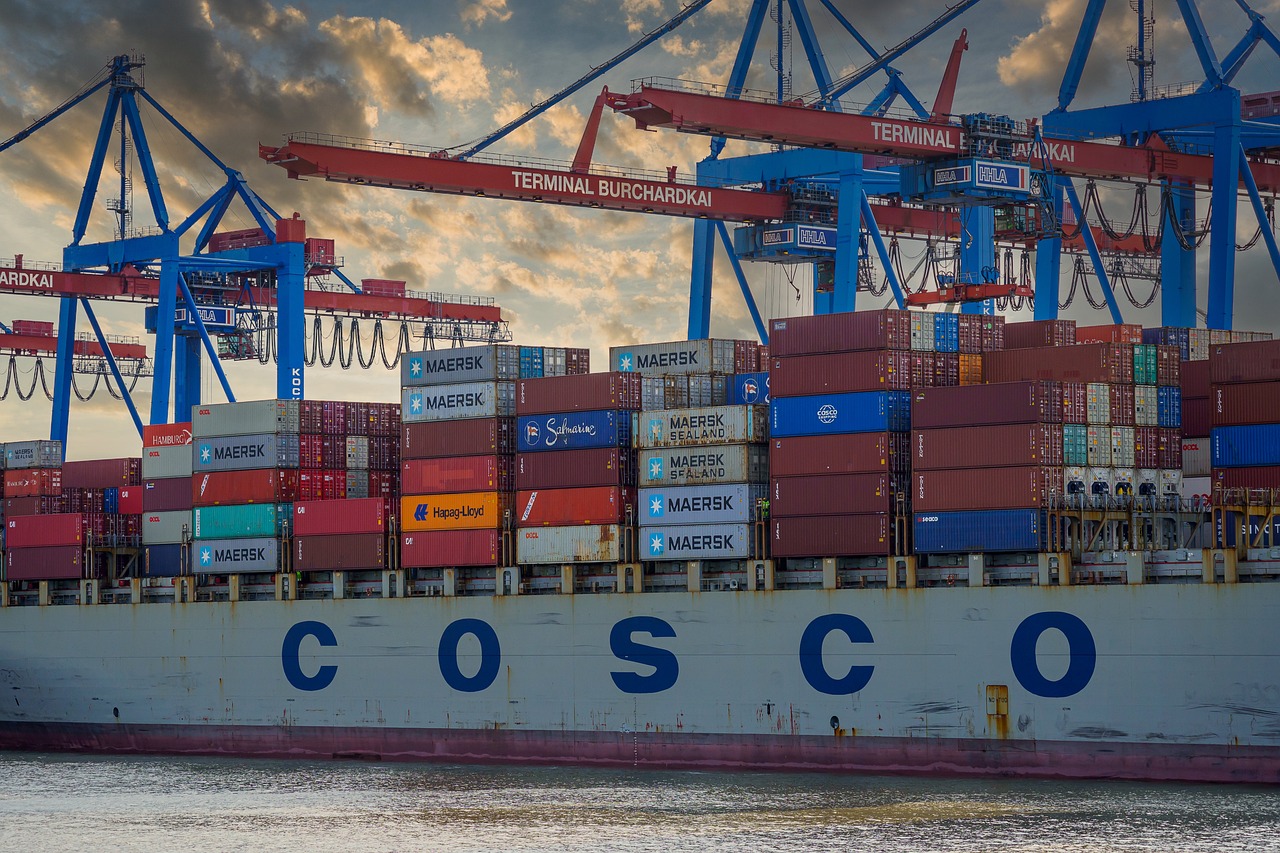
Data Privacy and Security Risks
As supply chains embrace the digital age, the integration of artificial intelligence brings forth a myriad of benefits, but it also raises significant concerns regarding data privacy and security. With the ever-increasing reliance on data-driven decision-making, sensitive information about customers, suppliers, and operational processes is constantly being collected, stored, and analyzed. This creates a treasure trove of data that, if not adequately protected, could become a target for cybercriminals.
Imagine your personal information being stolen and used for malicious purposes; that’s the reality many businesses face today. The stakes are high, and the consequences of a data breach can be devastating. Companies can suffer not only financial losses due to theft but also reputational damage that can take years to recover from. In fact, according to a recent study, over 60% of small businesses close within six months of a cyber attack. This statistic highlights the urgency for organizations to prioritize data security.
To mitigate these risks, organizations must adopt a multi-layered approach to data security. This includes implementing stringent access controls, encryption for sensitive data, and regular security audits. Additionally, educating employees about data privacy best practices is crucial, as human error is often the weakest link in cybersecurity. Here are some key strategies businesses can implement:
- Data Encryption: Protecting sensitive information by converting it into a secure format that can only be read by authorized parties.
- Access Controls: Restricting data access to only those employees who need it for their roles, reducing the risk of insider threats.
- Regular Audits: Conducting frequent assessments of data security measures to identify vulnerabilities and ensure compliance with regulations.
- Employee Training: Providing ongoing training for employees on data privacy and security protocols to minimize the risk of accidental breaches.
Moreover, compliance with data protection regulations such as the General Data Protection Regulation (GDPR) and the California Consumer Privacy Act (CCPA) is essential. These regulations impose strict guidelines on how organizations handle personal data, and non-compliance can result in hefty fines. Therefore, it’s not just about protecting data; it’s about adhering to legal standards that safeguard individual privacy rights.
In conclusion, while the adoption of AI in supply chains presents exciting opportunities for efficiency and innovation, it also necessitates a robust framework for data privacy and security. Companies must be proactive in addressing these risks, implementing comprehensive security measures, and fostering a culture of awareness around data protection. After all, in the world of supply chains, trust is the currency that drives successful relationships with customers and partners alike.
- What are the main data privacy risks associated with AI in supply chains?
Data privacy risks include unauthorized access to sensitive information, potential data breaches, and non-compliance with regulations. - How can companies protect their data?
By implementing encryption, access controls, regular audits, and employee training on data security best practices. - What regulations should companies be aware of?
Companies should be aware of GDPR, CCPA, and other local data protection laws that govern the handling of personal data.
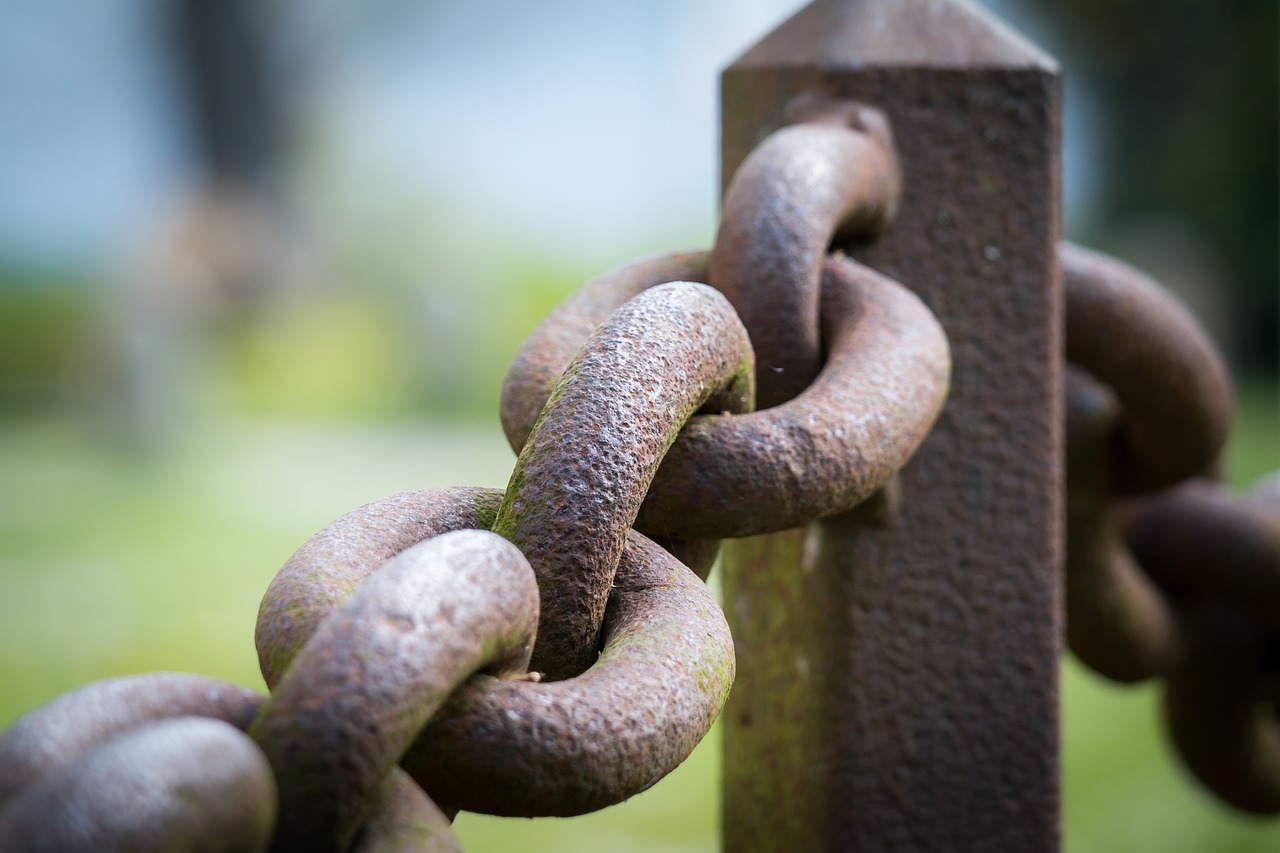
Addressing Workforce Displacement
The rise of artificial intelligence (AI) in supply chains is a double-edged sword. On one hand, it brings forth unprecedented efficiency and innovation; on the other, it raises a significant concern regarding workforce displacement. As machines and algorithms take over tasks traditionally performed by humans, many workers find themselves questioning their job security. This shift is akin to a tidal wave, sweeping through industries and necessitating a robust response to mitigate its impact on the workforce.
To address these challenges, companies must adopt a proactive approach. First and foremost, reskilling and upskilling programs are essential. Workers need to be equipped with new skills that align with the evolving landscape of supply chain operations. For instance, training programs can focus on teaching employees how to work alongside AI technologies, emphasizing the importance of human oversight in automated processes. By fostering a culture of continuous learning, organizations can help their workforce adapt to the changing demands of the industry.
Moreover, organizations should consider implementing career transition initiatives. These initiatives can assist displaced workers in finding new roles within the company or even in different sectors. By offering resources such as career counseling, job placement services, and mentorship programs, businesses can smooth the transition for employees affected by automation. This not only helps individuals but also strengthens the company’s reputation as a socially responsible employer.
It’s also vital to engage in open dialogues with employees about the future of work. Transparency can alleviate fears and foster a sense of security among workers. Companies could hold regular town hall meetings or workshops to discuss the implications of AI, share success stories of reskilled employees, and outline the organization’s commitment to supporting its workforce. This engagement can create a more cohesive environment where employees feel valued and informed.
Lastly, collaboration with educational institutions can play a pivotal role in preparing the future workforce. By partnering with universities and training centers, companies can help shape curricula that focus on the skills needed in an AI-driven supply chain. This collaboration ensures that new entrants into the workforce are better equipped to meet the demands of the industry, ultimately leading to a more skilled labor pool.
As we navigate this transformative era, it’s crucial to remember that technology should augment human capabilities rather than replace them. By taking proactive steps to address workforce displacement, organizations can not only enhance their operational efficiency but also foster a resilient workforce ready to tackle the challenges of the future.
- What is workforce displacement? Workforce displacement refers to the loss of jobs due to automation and technological advancements, where machines or software take over tasks previously performed by humans.
- How can companies help displaced workers? Companies can assist displaced workers by offering reskilling programs, career transition initiatives, and engaging in open communication about the changes in the workplace.
- Is AI replacing all jobs in the supply chain? While AI is automating certain tasks, it is also creating new roles that require human oversight and interaction, thus shifting the nature of work rather than eliminating it entirely.
- What skills are important for the future workforce? Skills such as data analysis, critical thinking, and the ability to work with AI technologies will be crucial for workers in the future supply chain landscape.
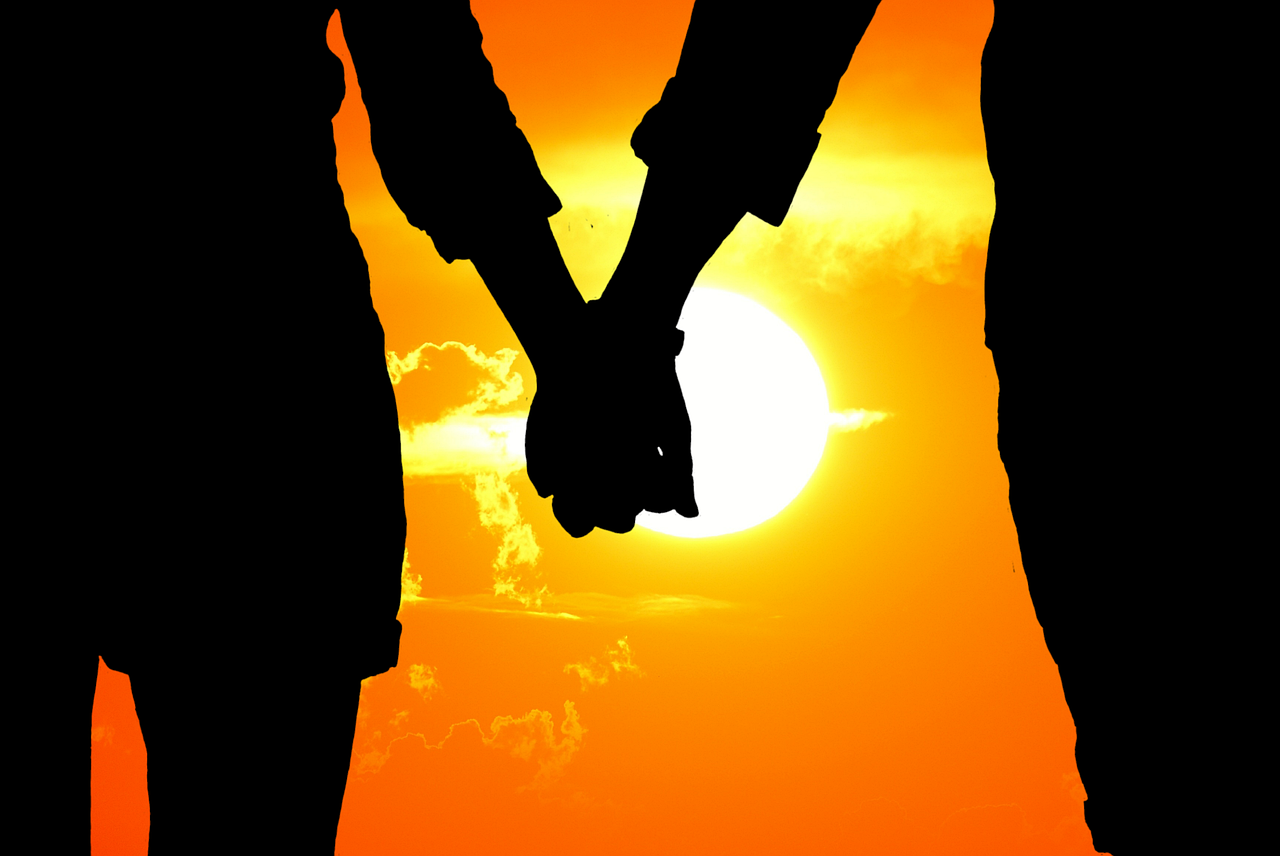
The Future of AI in Supply Chains
As we gaze into the crystal ball of supply chain management, it's clear that the future of AI is not just bright—it's dazzling. Imagine a world where every link in the supply chain is interconnected and optimized, driven by advanced technologies that can predict, adapt, and respond to changes in real-time. This is not science fiction; it's the emerging reality shaped by artificial intelligence. Companies are already leveraging AI to create more resilient and agile supply chains, ensuring they can meet the ever-evolving demands of consumers.
One of the most exciting aspects of AI in supply chains is its potential for enhanced collaboration. By integrating machine learning algorithms with blockchain technology, businesses can create a transparent and secure environment for sharing data across the supply chain. This not only improves trust among partners but also facilitates quicker decision-making. For instance, a manufacturer can instantly access real-time data from suppliers, allowing them to adjust production schedules based on current market demands.
Furthermore, the incorporation of AI-driven predictive analytics will continue to revolutionize demand forecasting. Companies will be able to analyze vast amounts of data from various sources—ranging from social media trends to weather patterns—to anticipate customer needs with unprecedented accuracy. This capability will not only enhance inventory management but also minimize waste, leading to a more sustainable supply chain. In fact, studies suggest that organizations utilizing AI for demand forecasting can reduce excess inventory by up to 30%.
However, the journey towards an AI-integrated supply chain is not without its challenges. As organizations adopt these technologies, they must also navigate complex issues such as data privacy and cybersecurity. With sensitive information flowing through digital channels, ensuring robust security measures is crucial to protect against potential breaches. Companies will need to invest in advanced security protocols to safeguard their data while remaining compliant with regulations.
Another significant aspect of the future is the evolution of the workforce. As AI takes over repetitive tasks, there will be a pressing need for reskilling employees to adapt to new roles that require a higher level of expertise. This shift presents an opportunity for companies to foster a culture of continuous learning, ensuring their workforce is equipped to thrive in an AI-driven landscape. By investing in training programs, organizations can empower their employees to embrace technology rather than fear it.
In conclusion, the future of AI in supply chains is not just about automation; it's about creating a more intelligent, responsive, and interconnected ecosystem. As companies continue to explore the potential of AI, we can expect to see innovations that redefine logistics and distribution, making supply chains smarter and more efficient than ever before. The horizon is filled with possibilities, and those who are willing to embrace these changes will undoubtedly lead the charge into a new era of supply chain management.
- What are the main benefits of AI in supply chains? AI enhances efficiency, reduces costs, and improves decision-making through data analysis.
- How does predictive analytics improve demand forecasting? It allows companies to anticipate demand fluctuations and optimize inventory levels.
- What challenges do companies face when implementing AI? Challenges include data quality issues, workforce displacement, and data privacy concerns.
- How will AI impact the workforce in supply chains? AI may lead to job displacement, necessitating reskilling and transitioning employees to new roles.
- What role will blockchain play in the future of AI in supply chains? Blockchain can enhance collaboration and data transparency, improving trust among supply chain partners.
Frequently Asked Questions
- What is the role of AI in supply chain optimization?
AI plays a crucial role in supply chain optimization by enhancing efficiency and reducing costs. It leverages data analysis and predictive modeling to improve decision-making processes, allowing businesses to operate more smoothly and responsively.
- How does predictive analytics improve demand forecasting?
Predictive analytics uses AI to analyze historical data and identify patterns, enabling companies to anticipate demand fluctuations. This helps in optimizing inventory levels and ensuring that products are available when customers need them, ultimately boosting customer satisfaction.
- What challenges do businesses face when implementing AI in supply chains?
While AI offers significant benefits, businesses may encounter challenges such as data quality issues, the need for skilled personnel, and resistance to change within the organization. These hurdles can complicate the adoption of AI technologies in supply chain processes.
- How does AI facilitate real-time inventory management?
AI enables real-time inventory management by providing businesses with the tools to track stock levels accurately and streamline replenishment processes. This leads to reduced waste and better visibility across the supply chain, ensuring that resources are utilized efficiently.
- What innovations are being made in last-mile delivery using AI?
AI technologies are revolutionizing last-mile delivery through the use of autonomous vehicles and drones. These innovations optimize delivery routes, enhance speed, and improve reliability, making the logistics of getting products to customers faster and more efficient.
- What ethical considerations should be kept in mind with AI in supply chains?
As AI becomes more integrated into supply chains, ethical considerations such as data privacy, job displacement, and the implications of automated decision-making must be addressed. Companies need to ensure they are compliant with regulations and have strategies in place for workforce transitions.
- What does the future hold for AI in supply chains?
The future of AI in supply chains is promising, with ongoing advancements in machine learning, blockchain integration, and enhanced collaboration among supply chain partners. These developments are expected to drive further innovation and efficiency in logistics and distribution.