AI and Data Science: Shaping Future of Business
In today's fast-paced world, Artificial Intelligence (AI) and Data Science are not just buzzwords; they are the driving forces behind the transformation of modern business practices. Imagine a world where decisions are made not just on instinct but backed by data and predictive models. This is the reality that AI and data science are creating, revolutionizing how companies operate and interact with their customers. The integration of these technologies is akin to adding a turbocharger to a car; it enhances performance, efficiency, and speed, allowing businesses to race ahead of the competition.
But what exactly do we mean by AI and data science? At their core, AI refers to the simulation of human intelligence processes by machines, especially computer systems. This includes learning, reasoning, and self-correction. On the other hand, data science is the discipline that uses scientific methods, algorithms, and systems to extract knowledge and insights from structured and unstructured data. Together, they create a powerful synergy that enables businesses to innovate and make data-driven decisions.
As we delve deeper into the implications of AI and data science, it becomes clear that their significance extends beyond mere efficiency. They are reshaping the landscape of industries, influencing everything from marketing strategies to supply chain management. For instance, companies that harness predictive analytics can foresee market trends and customer behaviors, allowing them to adapt swiftly and effectively. This proactive approach is not just beneficial; it's essential for survival in a competitive marketplace.
Moreover, the interrelationship between AI and data science fosters a culture of continuous improvement within organizations. By leveraging vast amounts of data, businesses can identify patterns, optimize operations, and enhance customer experiences. It's like having a crystal ball that not only shows you the future but also provides insights into how to shape that future. As we explore the key applications and trends in this article, keep in mind that the journey of AI and data science is just beginning, and its potential is limited only by our imagination.
- What is the difference between AI and Data Science?
AI focuses on creating systems that can perform tasks usually requiring human intelligence, while data science involves extracting insights from data using various techniques.
- How can businesses benefit from AI and Data Science?
Businesses can improve decision-making, enhance customer engagement, optimize operations, and drive innovation through the applications of AI and data science.
- Are there challenges in implementing AI and Data Science?
Yes, common challenges include data quality issues, the need for skilled personnel, and the integration of new technologies into existing systems.
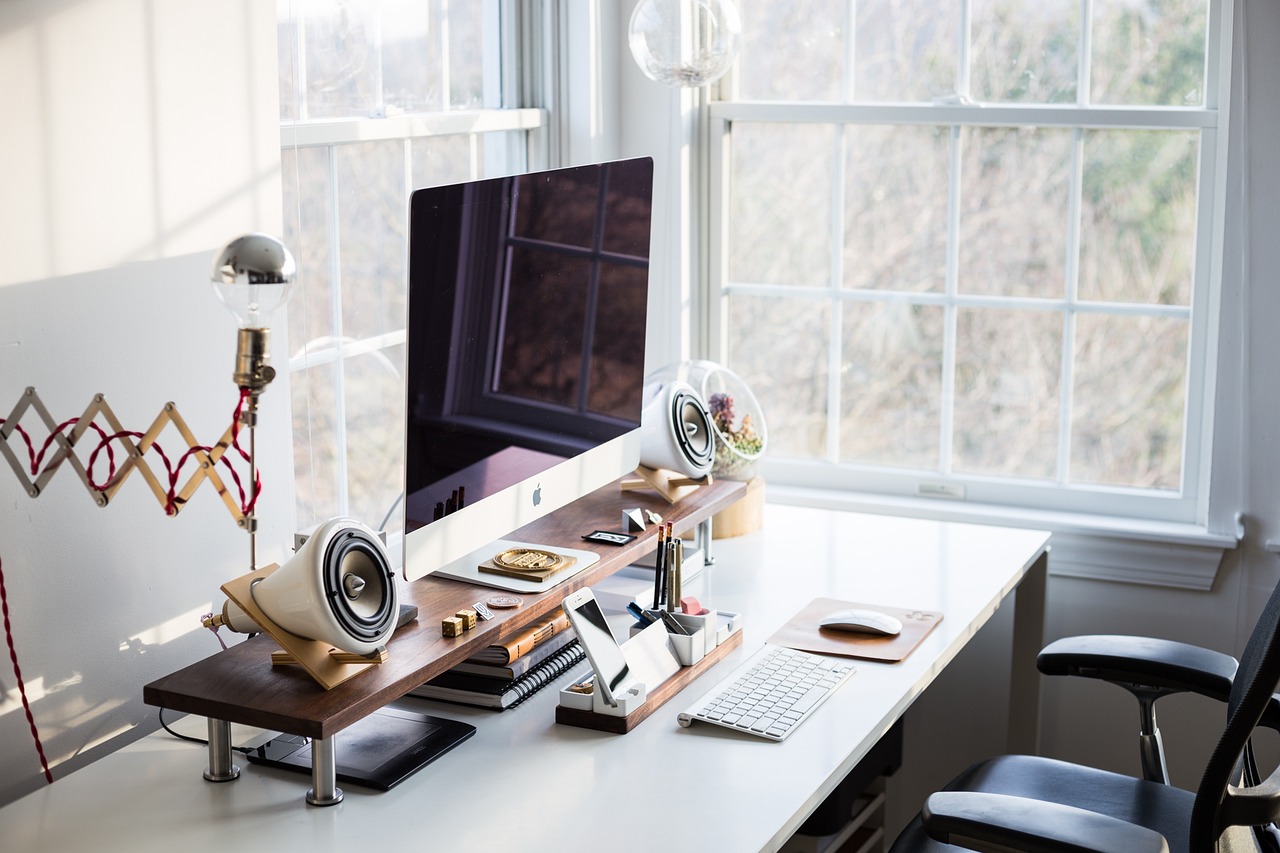
Understanding AI and Data Science
In today's fast-paced digital landscape, Artificial Intelligence (AI) and Data Science are not just buzzwords; they are the driving forces behind innovation and efficiency in business. But what exactly do these terms mean? At its core, AI refers to the simulation of human intelligence in machines that are programmed to think, learn, and adapt. This can range from simple tasks like recognizing speech to complex functions like decision-making and problem-solving. On the other hand, data science is the field that combines statistical analysis, data processing, and machine learning to extract meaningful insights from vast amounts of data. Together, they form a powerful duo that enables businesses to make data-driven decisions.
The significance of AI and data science in the business world cannot be overstated. As companies collect more data than ever before—from customer interactions to market trends—leveraging this information becomes crucial for staying competitive. Imagine trying to navigate a bustling city without a map; that’s what it feels like for businesses without AI and data science. These technologies act as the GPS, guiding organizations through the complexities of the market landscape and helping them identify opportunities and threats.
Moreover, the interrelationship between AI and data science is akin to a dance; each discipline complements the other. Data science provides the foundation of data analysis and interpretation, while AI enhances these insights by automating processes and enabling predictive capabilities. For instance, when a company collects customer data, data science techniques can help analyze purchasing patterns, while AI can predict future buying behaviors based on those patterns. This synergy not only boosts operational efficiency but also enhances customer satisfaction, leading to greater loyalty and increased revenue.
As we delve deeper into this article, we will explore various applications of AI and data science in business, showcasing their transformative impact. From predictive analytics to customer segmentation, the possibilities are endless. But before we do that, let’s take a moment to address some frequently asked questions about these technologies.
- What is the difference between AI and data science? AI focuses on creating systems that can perform tasks that typically require human intelligence, while data science is about extracting insights from data.
- How do businesses benefit from AI and data science? They help in making informed decisions, improving customer experiences, and increasing operational efficiency.
- Are there any challenges in implementing AI and data science? Yes, common challenges include data quality issues, the need for skilled personnel, and integration with existing systems.
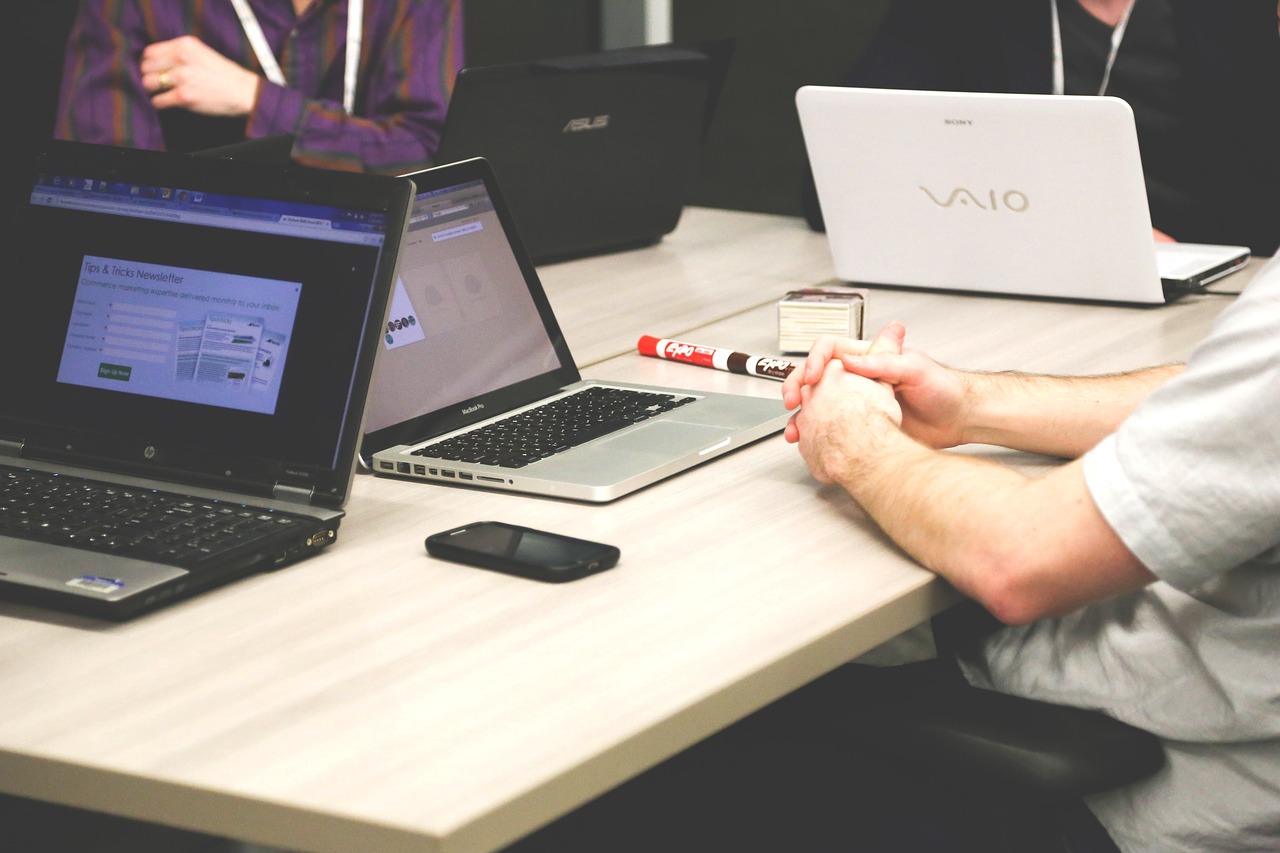
Key Applications in Business
In today's fast-paced business environment, AI and data science have emerged as pivotal tools that organizations leverage to stay ahead of the competition. From enhancing operational efficiency to improving customer satisfaction, the applications of these technologies are vast and varied. Let's dive into some of the most impactful applications that are reshaping the business landscape.
One of the most significant applications of AI and data science is in the realm of predictive analytics. This powerful tool enables businesses to sift through historical data to identify patterns and predict future outcomes. Imagine a retail store that analyzes past sales data to forecast which products will be in demand next season. By leveraging predictive analytics, they can make informed decisions on inventory management, ensuring they stock up on popular items while avoiding overstocking less popular ones.
Predictive analytics is not just about looking at numbers; it's about transforming those numbers into actionable insights. By analyzing trends and patterns, businesses can anticipate customer needs and market shifts. For instance, a financial institution might use predictive models to assess the likelihood of loan defaults, allowing them to minimize risk and optimize their lending strategies.
The benefits of incorporating predictive analytics into business strategies are substantial:
- Improved Customer Insights: Understanding customer behavior allows businesses to tailor their offerings, leading to better customer experiences.
- Enhanced Operational Efficiency: By predicting demand, companies can streamline their operations, reducing waste and optimizing resource allocation.
- Increased Revenue Potential: With accurate forecasts, businesses can capitalize on opportunities, driving sales and boosting profitability.
However, the journey to implementing predictive analytics is not without its hurdles. Common challenges include:
- Data Quality Issues: The effectiveness of predictive analytics is heavily reliant on the quality of data. Inaccurate or incomplete data can lead to misleading predictions.
- Need for Skilled Personnel: Organizations often struggle to find professionals who possess the necessary skills to analyze data effectively and interpret the results.
Another crucial application of AI and data science is customer segmentation. By analyzing customer data, businesses can identify distinct groups within their customer base, enabling them to tailor their marketing strategies effectively. For example, a company might discover that a particular demographic responds better to email marketing than social media ads. This insight allows them to allocate their marketing resources more efficiently, maximizing engagement and conversion rates.
In essence, the key applications of AI and data science in business are not just trends; they are powerful strategies that can lead to significant improvements in efficiency, customer satisfaction, and overall profitability. As businesses continue to embrace these technologies, the potential for innovation and growth is boundless.
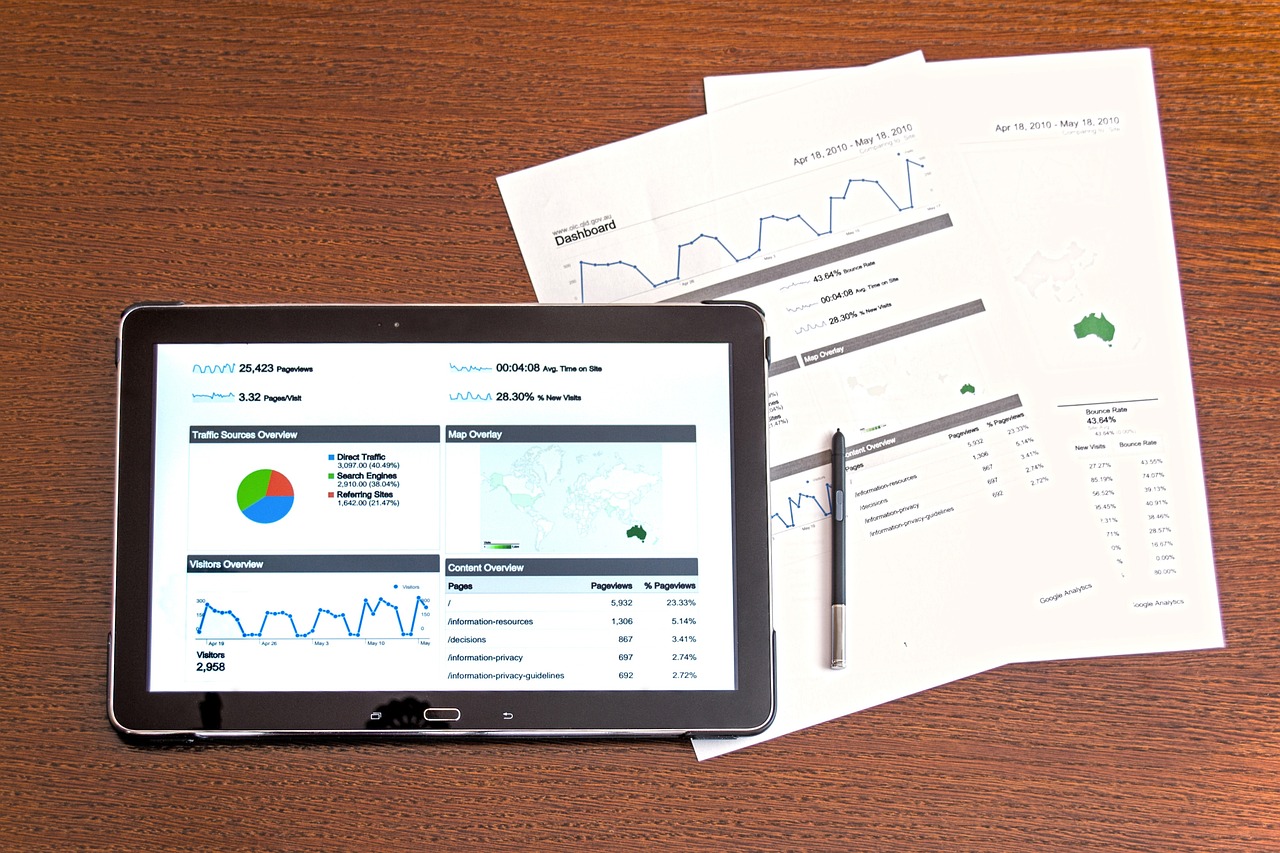
Predictive Analytics
Predictive analytics is like having a crystal ball for your business, allowing you to peek into the future based on historical data. Imagine being able to forecast customer behavior, sales trends, or even potential risks before they happen! This powerful tool utilizes statistical algorithms and machine learning techniques to analyze past data, revealing patterns that can guide decision-making. In today’s fast-paced business environment, where every second counts, having the ability to predict outcomes can mean the difference between thriving and merely surviving.
At its core, predictive analytics combines various data sources to create models that can forecast future events. For example, a retail company might analyze previous sales data, customer demographics, and seasonal trends to predict which products will be in high demand during the upcoming holiday season. By doing so, they can optimize inventory levels, reduce waste, and ensure that they meet customer expectations. This not only enhances operational efficiency but also boosts customer satisfaction—talk about a win-win!
However, implementing predictive analytics isn't just about having the right tools; it requires a strategic approach. Businesses need to ensure that they have high-quality data, as the accuracy of predictions heavily relies on the data fed into the models. Furthermore, organizations must invest in skilled personnel who can interpret the data and translate it into actionable insights. Without these elements, even the most advanced predictive models can fall flat, leading to misguided strategies and wasted resources.
To illustrate the impact of predictive analytics, let’s consider a few key benefits:
- Improved Customer Insights: By analyzing customer behavior patterns, businesses can gain a deeper understanding of their audience, allowing for more targeted marketing efforts.
- Enhanced Operational Efficiency: Predictive analytics can identify bottlenecks in processes, enabling companies to streamline operations and reduce costs.
- Increased Revenue Potential: By forecasting demand accurately, businesses can optimize pricing strategies and promotions, ultimately driving sales growth.
Despite its advantages, businesses often encounter challenges when implementing predictive analytics. Data quality issues can arise if the data is incomplete or inaccurate, leading to unreliable predictions. Additionally, there’s a growing need for skilled personnel who can navigate complex data landscapes and derive meaningful insights. Companies must invest in training or hire data scientists to ensure they can effectively harness the power of predictive analytics.
In conclusion, predictive analytics serves as a crucial component in modern business strategy. By leveraging historical data to forecast future trends, companies can make informed decisions that drive growth and efficiency. As technology continues to evolve, the potential for predictive analytics to reshape industries is limitless. Are you ready to embrace the future and unlock the secrets hidden within your data?
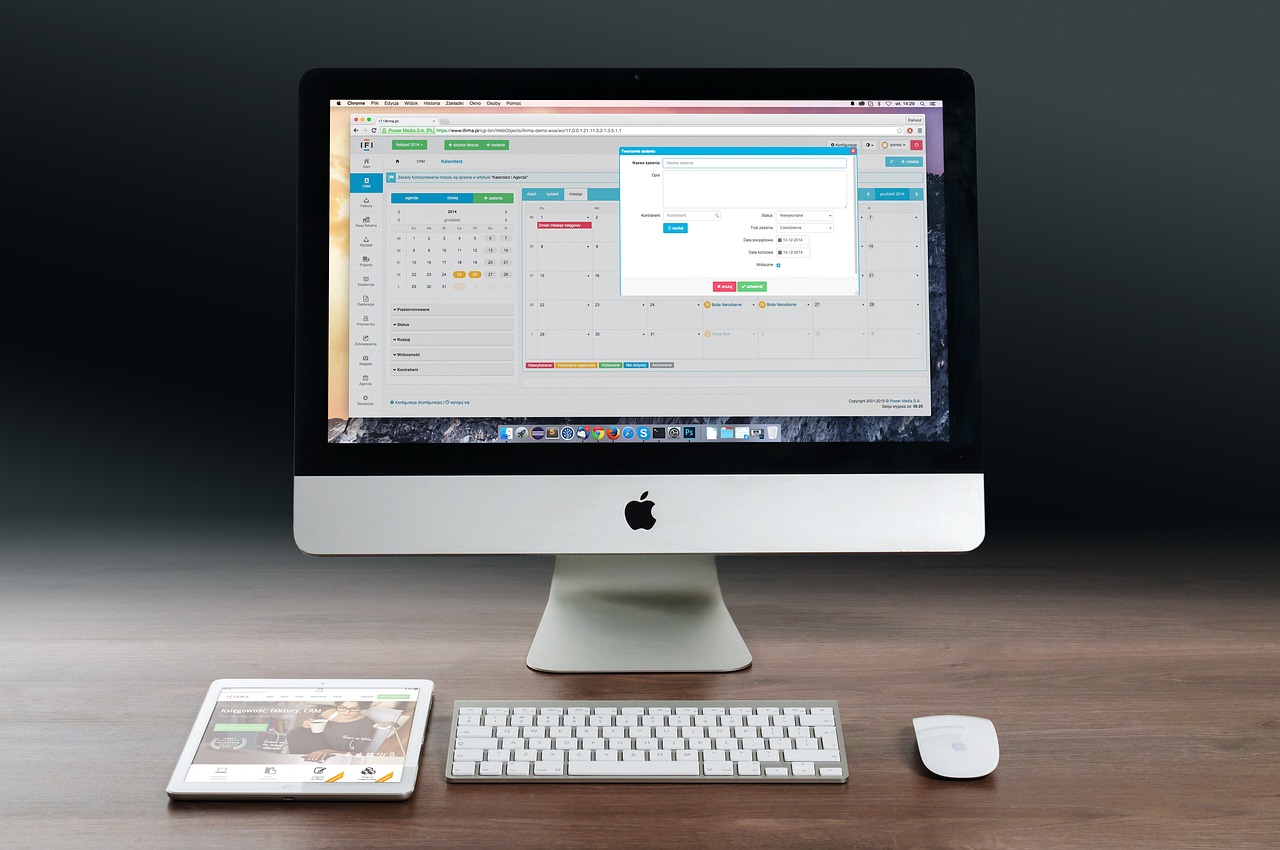
Benefits of Predictive Analytics
Predictive analytics is like having a crystal ball for your business. Imagine being able to foresee trends, anticipate customer needs, and make decisions that are backed by data rather than guesswork. This powerful tool harnesses the potential of historical data and advanced algorithms to forecast future outcomes, which can significantly enhance a company’s strategic planning. The benefits of implementing predictive analytics are numerous and impactful, making it an essential component of modern business practices.
One of the most significant advantages of predictive analytics is its ability to provide improved customer insights. By analyzing past behaviors and preferences, businesses can create highly detailed customer profiles. This allows for personalized marketing strategies that resonate with the target audience. For instance, companies can tailor their promotional offers based on individual buying patterns, leading to increased engagement and loyalty. When customers feel understood and valued, they are more likely to return, boosting overall sales.
Another key benefit is the enhancement of operational efficiency. Predictive analytics can identify bottlenecks in processes and suggest improvements. For example, a manufacturing company can use predictive models to forecast equipment failures before they occur, allowing for timely maintenance and minimizing downtime. This proactive approach not only saves costs but also ensures that resources are utilized effectively, leading to a smoother operation overall.
Moreover, businesses that leverage predictive analytics often experience an increase in revenue potential. By accurately predicting market trends and customer demands, organizations can align their inventory and marketing strategies accordingly. This not only reduces waste but also ensures that popular products are always in stock, maximizing sales opportunities. In fact, studies have shown that companies using predictive analytics can achieve up to a 20% increase in sales compared to those that do not.
However, it's essential to note that while the benefits are compelling, the implementation of predictive analytics does come with its challenges. Organizations must ensure they have access to high-quality data and the necessary infrastructure to support these advanced analytics tools. Additionally, there is a growing need for skilled personnel who can interpret the data and derive actionable insights. Despite these challenges, the potential rewards of predictive analytics make it a worthwhile investment for any forward-thinking business.
In summary, the benefits of predictive analytics are vast and varied, impacting everything from customer engagement to operational efficiency and revenue growth. As businesses continue to navigate an increasingly complex market landscape, those that embrace predictive analytics will undoubtedly have a competitive edge, transforming data into a powerful strategic asset.
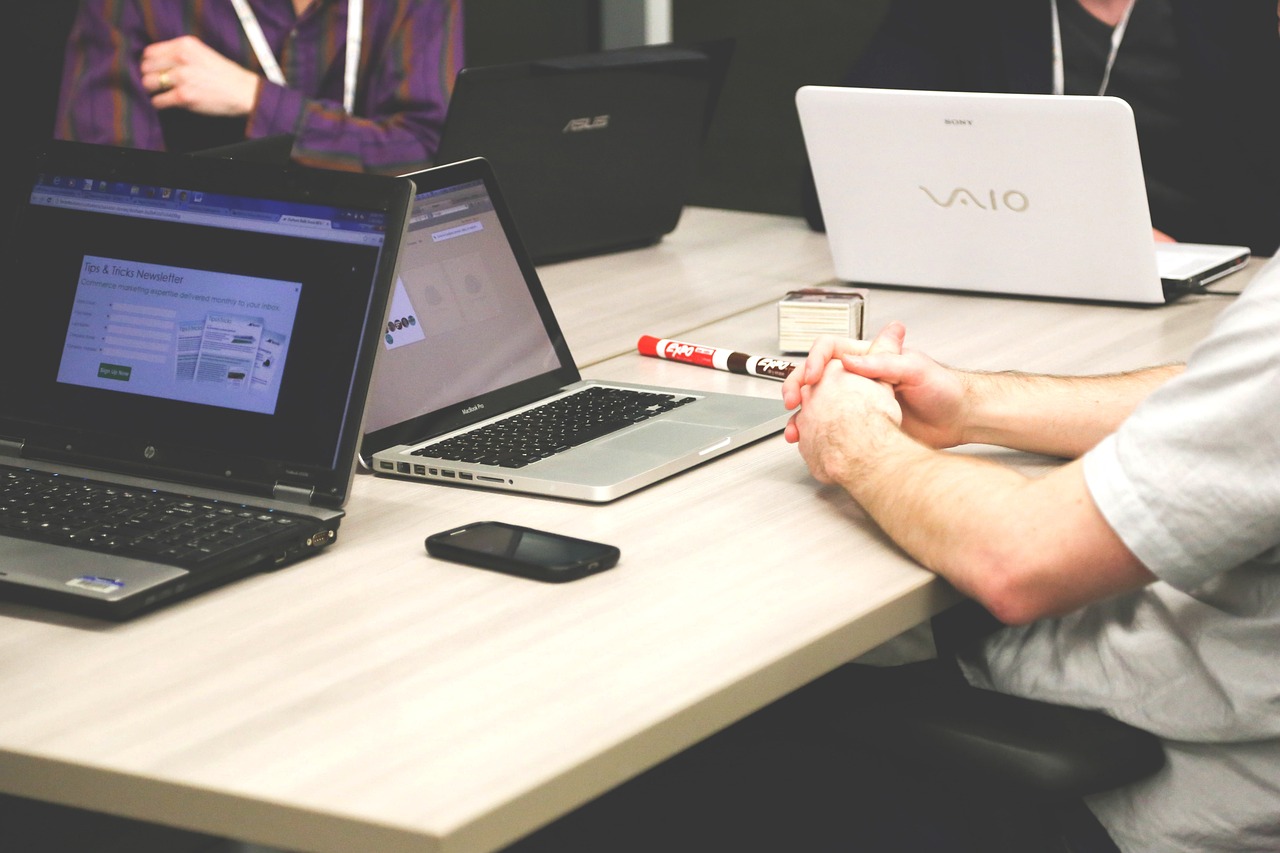
Challenges in Implementation
Implementing predictive analytics in a business setting can feel like trying to navigate a maze blindfolded. While the potential benefits are enormous, several challenges can obstruct the path to success. One of the most significant hurdles is data quality. Businesses often struggle with incomplete, inconsistent, or outdated data, which can lead to inaccurate predictions and misguided strategies. Imagine trying to build a house on a shaky foundation; the results are likely to be disastrous!
Another challenge is the need for skilled personnel. The world of AI and data science is highly technical and requires a specific skill set that not all organizations possess. Without data scientists and analysts who understand how to interpret data correctly, companies may find themselves lost in a sea of numbers, unable to make sense of the insights they collect.
Moreover, there is often a resistance to change within organizations. Employees may be accustomed to traditional methods of decision-making and may be hesitant to embrace new technologies. This cultural barrier can slow down the implementation process, making it difficult for businesses to fully leverage predictive analytics. To overcome this, companies need to foster a culture of innovation and continuous learning, encouraging their teams to adapt and grow.
Lastly, cost considerations cannot be ignored. Implementing predictive analytics tools and hiring skilled personnel can be a significant investment. For small to medium-sized businesses, this can pose a serious challenge. It's essential for organizations to weigh the potential return on investment against the costs involved, ensuring that they are making a sound financial decision.
In summary, while the journey toward implementing predictive analytics is fraught with challenges, understanding these obstacles can help businesses prepare and strategize effectively. By addressing data quality issues, investing in skilled personnel, fostering a culture of innovation, and carefully considering costs, organizations can pave the way for successful predictive analytics implementation.
- What is predictive analytics?
Predictive analytics is the use of historical data and statistical algorithms to forecast future outcomes. It helps businesses make informed decisions based on data-driven insights.
- Why is data quality important?
Data quality is crucial because inaccurate or incomplete data can lead to faulty predictions, ultimately affecting business strategies and outcomes.
- What skills are needed for predictive analytics?
Skills required include statistical analysis, data mining, programming, and a solid understanding of machine learning algorithms.
- How can businesses overcome resistance to change?
Businesses can overcome resistance by fostering a culture of innovation, providing training, and demonstrating the benefits of new technologies.
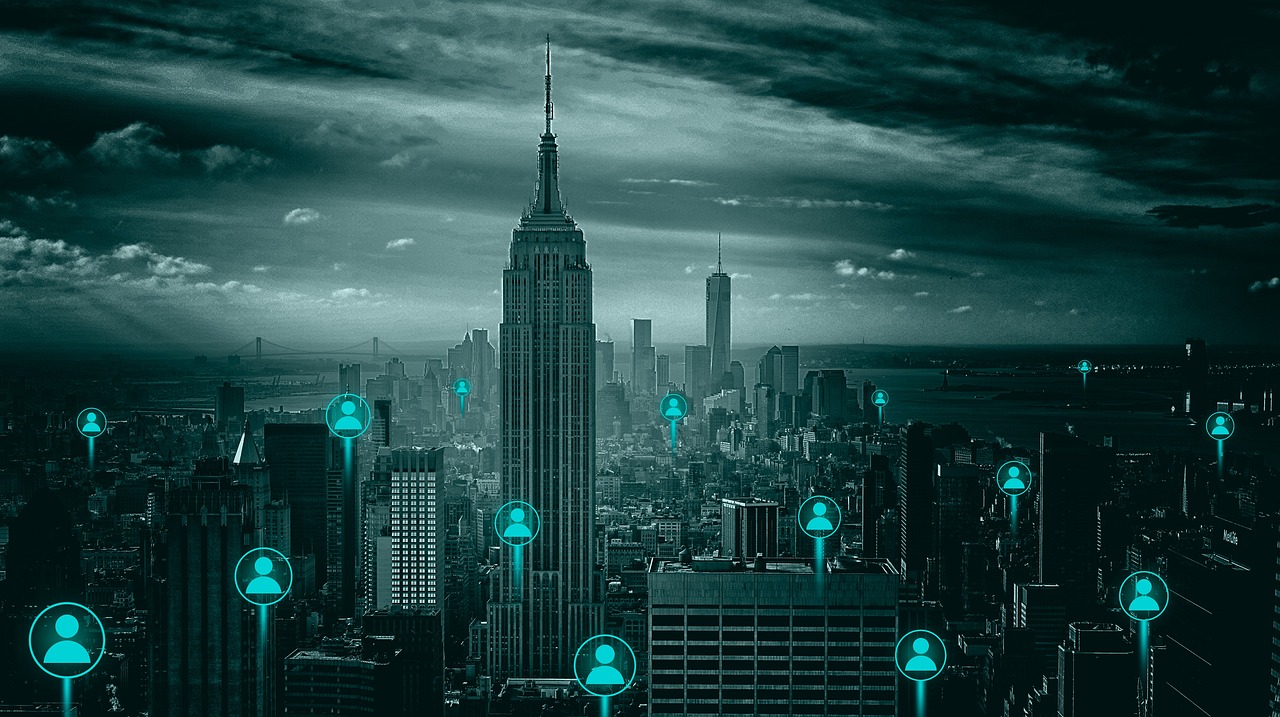
Customer Segmentation
In today's fast-paced business landscape, understanding your customers is not just an advantage; it's a necessity. is a powerful technique that allows businesses to divide their customer base into distinct groups based on various criteria, such as demographics, purchasing behavior, and preferences. This process is akin to a chef selecting specific ingredients for a dish—each segment represents a unique flavor profile that requires a tailored approach to marketing and engagement.
By leveraging AI and data science, companies can analyze vast amounts of data to identify these segments with precision. Imagine walking into a store where every item is perfectly curated for your tastes. That's the magic of customer segmentation. It enables businesses to create personalized marketing strategies that resonate with each group, ultimately driving higher engagement and conversion rates.
There are several key factors that businesses consider when segmenting their customers:
- Demographics: Age, gender, income level, and education can significantly influence purchasing decisions.
- Geographic Location: Understanding where customers live helps tailor products and marketing efforts to regional preferences.
- Behavioral Data: Analyzing how customers interact with your brand, including purchase history and website behavior, provides insights into their preferences.
- Psychographics: This involves understanding customers' lifestyles, values, and interests, enabling deeper emotional connections.
For instance, a clothing retailer might use segmentation to identify a group of environmentally conscious consumers who prefer sustainable fashion. By targeting this segment with eco-friendly product lines and marketing campaigns, the retailer can foster loyalty and enhance customer satisfaction. This tailored approach not only boosts sales but also enhances the overall customer experience.
However, effective customer segmentation isn't without its challenges. Businesses must ensure they have access to high-quality data and the right analytical tools to derive meaningful insights. Additionally, as customer behaviors and preferences evolve, ongoing analysis and adjustment of segmentation strategies are crucial. This dynamic nature of customer segmentation means that companies must remain agile, continually refining their approaches to stay relevant in an ever-changing market.
In conclusion, customer segmentation is a vital component of modern marketing strategies. By harnessing the power of AI and data science, businesses can create personalized experiences that not only meet but exceed customer expectations. The result? A more engaged customer base, increased loyalty, and ultimately, enhanced profitability.
Q: What is customer segmentation?
A: Customer segmentation is the process of dividing a customer base into distinct groups based on shared characteristics, such as demographics, behaviors, and preferences.
Q: Why is customer segmentation important?
A: It allows businesses to tailor their marketing strategies to specific groups, leading to improved customer engagement, higher conversion rates, and increased customer loyalty.
Q: How can AI help with customer segmentation?
A: AI can analyze large datasets quickly, identifying patterns and insights that inform the segmentation process, enabling businesses to create more effective marketing strategies.
Q: What are some common challenges in customer segmentation?
A: Common challenges include data quality issues, the need for skilled personnel to analyze data, and the necessity for ongoing adjustments to segmentation strategies as customer behaviors change.
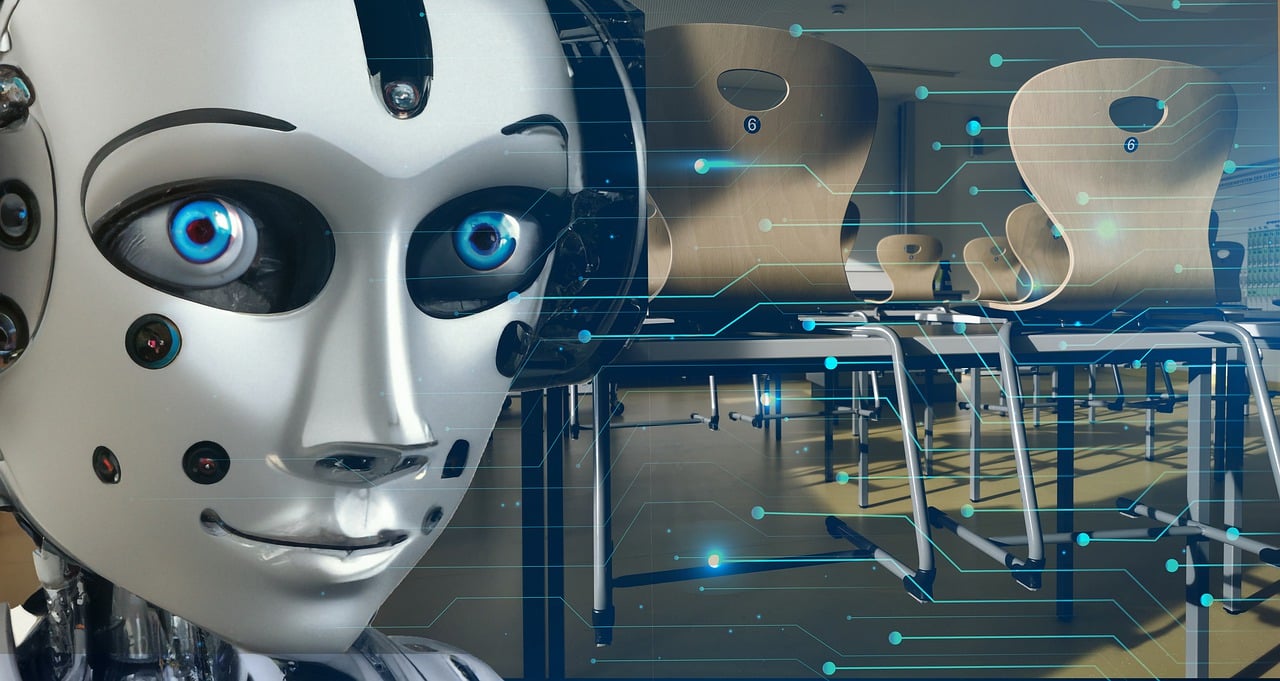
The Role of Machine Learning
Machine learning, a subset of artificial intelligence, is revolutionizing the way businesses operate by allowing them to analyze vast amounts of data and uncover hidden patterns that can significantly inform their strategies. Imagine having a crystal ball that not only predicts the future but also adapts and learns from new information—it’s pretty much what machine learning offers to modern enterprises. By leveraging algorithms that improve automatically through experience, businesses can make decisions that are not just informed but also timely and relevant.
The significance of machine learning in business cannot be overstated. It enables organizations to automate processes, enhance customer experiences, and optimize operations. For instance, consider a retail company that uses machine learning to analyze customer purchasing behavior. By examining historical data, the algorithm can identify trends and preferences, allowing the company to tailor marketing campaigns and inventory management. This not only boosts sales but also enhances customer satisfaction, as individuals receive personalized recommendations that resonate with their needs.
Furthermore, machine learning plays a critical role in risk management. Financial institutions, for example, utilize machine learning models to detect fraudulent transactions. These models are trained on historical transaction data, learning to recognize patterns indicative of fraud. As a result, they can flag suspicious activities in real-time, significantly reducing the risk of financial loss. This application exemplifies how machine learning not only enhances operational efficiency but also safeguards businesses against potential threats.
To put it simply, the role of machine learning in business is akin to having a highly skilled analyst who can sift through mountains of data at lightning speed, providing insights that would take humans days or weeks to uncover. This capability is not just a luxury; it’s becoming a necessity in an increasingly data-driven world.
When examining the applications of machine learning, it’s essential to differentiate between supervised and unsupervised learning. In supervised learning, algorithms are trained on labeled data, meaning they learn from input-output pairs. This approach is commonly used in scenarios like email filtering, where the model learns to classify emails as spam or not based on labeled examples. On the other hand, unsupervised learning deals with unlabeled data, allowing the algorithm to identify patterns and groupings without prior guidance. This is particularly useful in customer segmentation, where businesses can discover distinct customer groups based on purchasing behavior without pre-defined categories.
Real-world examples of machine learning in action abound across various industries. For instance, Netflix utilizes machine learning algorithms to analyze viewer preferences and recommend content that aligns with individual tastes. By continuously learning from user interactions, Netflix not only enhances user engagement but also drives subscription growth. Similarly, in healthcare, machine learning algorithms analyze patient data to predict disease outbreaks or identify potential health risks, enabling proactive measures that can save lives.
In conclusion, the role of machine learning in business is transformative and multifaceted. It empowers organizations to harness the power of data, leading to smarter decisions, enhanced customer experiences, and improved operational efficiencies. As businesses continue to navigate the complexities of the digital landscape, embracing machine learning will be crucial for staying competitive and innovative.
- What is machine learning? Machine learning is a branch of artificial intelligence that focuses on building systems that learn from data to improve their performance over time.
- How does machine learning benefit businesses? It allows businesses to automate processes, gain insights from data, enhance customer experiences, and optimize operations.
- What are the types of machine learning? The two main types are supervised learning, which uses labeled data, and unsupervised learning, which works with unlabeled data.
- Can machine learning help in fraud detection? Yes, machine learning algorithms can analyze transaction data to identify patterns indicative of fraudulent activities in real-time.
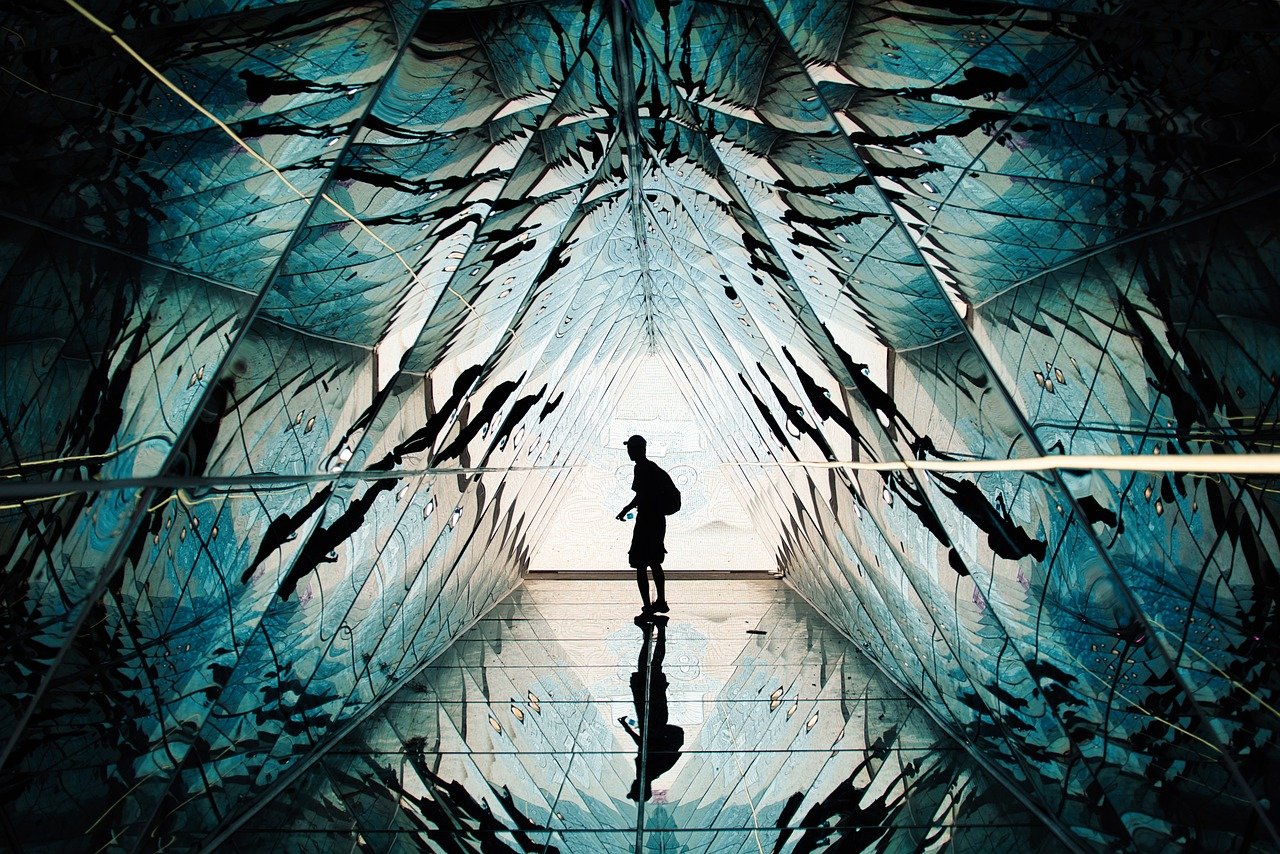
Supervised vs. Unsupervised Learning
When diving into the world of machine learning, one of the first concepts you'll encounter is the distinction between supervised and unsupervised learning. Think of it as two different paths in a vast forest of data, each leading to unique insights and applications. Supervised learning is like a teacher guiding a student through lessons. In this scenario, the model is trained on a labeled dataset, meaning that each input data point is paired with a corresponding output. This method is particularly effective for tasks where the outcome is known and can be used to train the model. For example, if you want to predict house prices based on various features such as size, location, and age, you would feed the model historical data that includes these features alongside the actual prices.
On the flip side, we have unsupervised learning, which can be likened to exploring a new city without a map. Here, the model is given data without any explicit instructions on what to do with it. Instead of learning from labeled examples, it seeks to identify patterns and groupings within the data on its own. This approach is incredibly useful for tasks like customer segmentation, where a business wants to understand different customer groups without prior knowledge of their characteristics. Imagine a retail store analyzing purchasing behavior without knowing beforehand what the segments might be. Unsupervised learning allows the model to discover hidden structures in the data, revealing insights that might not have been apparent at first glance.
To further clarify the differences, let’s look at a comparison table:
Feature | Supervised Learning | Unsupervised Learning |
---|---|---|
Data Type | Labeled Data | Unlabeled Data |
Goal | Predict outcomes based on input | Discover patterns and groupings |
Common Algorithms | Linear Regression, Decision Trees, SVM | K-Means Clustering, PCA, Hierarchical Clustering |
Use Cases | Spam detection, Image classification | Market segmentation, Anomaly detection |
Understanding these two methods is crucial for businesses looking to leverage data science effectively. Each method has its own strengths and weaknesses, and the choice between them often depends on the specific problem at hand. For instance, if a company is looking to predict customer churn based on past behavior, supervised learning would be the way to go. Conversely, if they're interested in discovering new customer segments for targeted marketing, unsupervised learning would be more appropriate.
In conclusion, both supervised and unsupervised learning play vital roles in the data science landscape. By understanding their differences and applications, businesses can harness the power of machine learning to drive innovation and make data-driven decisions. So, whether you're a seasoned data scientist or just getting started, recognizing when to use each approach can make all the difference in achieving your business goals.
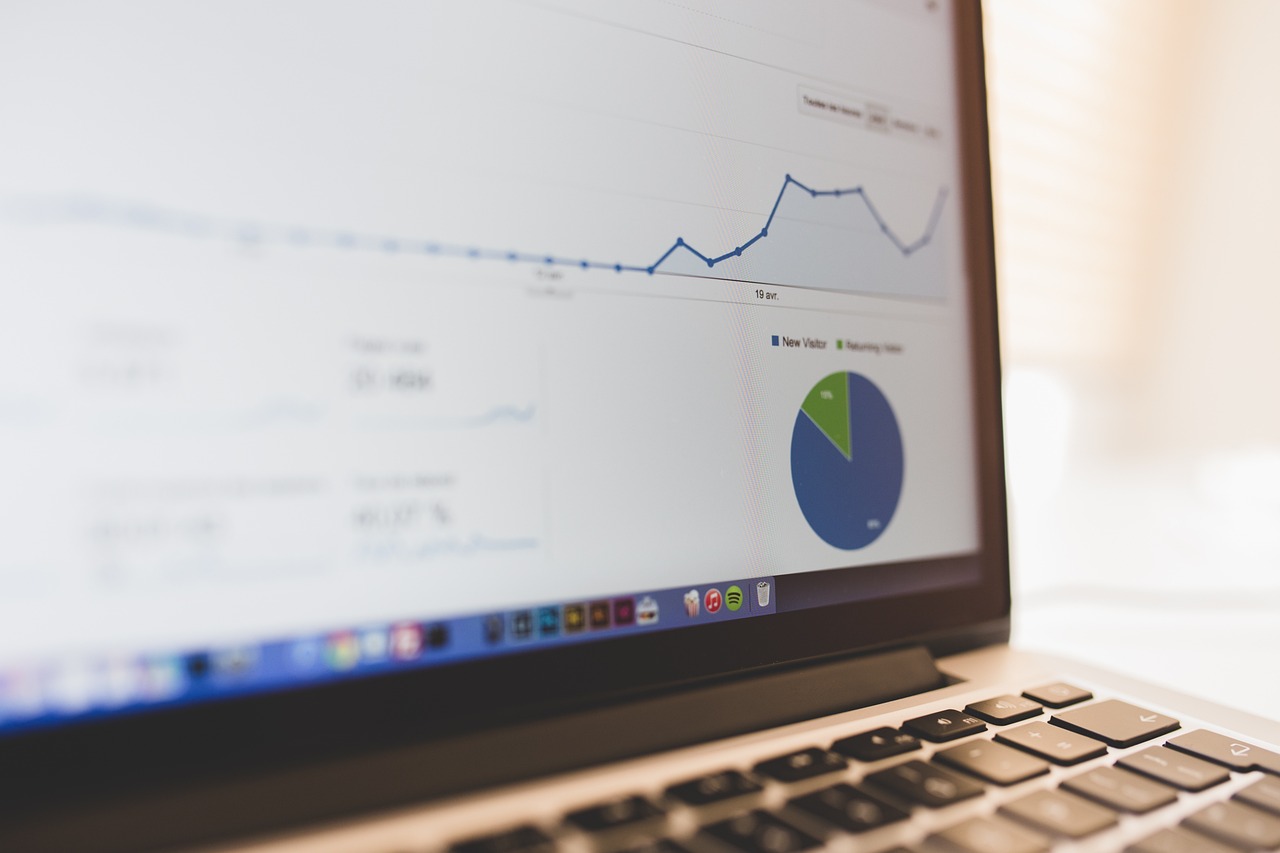
Real-World Examples
When it comes to understanding the transformative power of AI and data science in business, real-world examples serve as the best teachers. Businesses across various industries are embracing these technologies, and the results are nothing short of astounding. Take, for instance, the retail giant Walmart. By utilizing predictive analytics, Walmart can forecast customer demand with remarkable accuracy. This capability not only allows them to stock the right products at the right time but also significantly reduces waste. Imagine walking into a store where everything you want is available, simply because the business anticipated your needs!
Another fascinating example is Netflix, which has revolutionized the entertainment industry through its sophisticated use of machine learning. By analyzing user viewing patterns, Netflix can recommend shows and movies tailored to individual preferences. This personalized experience keeps users engaged and increases subscription retention. It's like having a personal movie assistant who knows your tastes better than you do!
In the financial sector, companies like American Express leverage data science to enhance fraud detection systems. By employing machine learning algorithms, they analyze transaction patterns in real-time, identifying anomalies that could indicate fraudulent activity. This proactive approach not only protects customers but also saves the company millions in potential losses. It's akin to having a vigilant security guard who never sleeps, always on the lookout for suspicious behavior.
Moreover, in healthcare, organizations like IBM Watson are harnessing the power of AI to assist in diagnosing diseases. Watson can analyze vast amounts of medical data, including patient records and clinical studies, to provide healthcare professionals with insights that can lead to better patient outcomes. Imagine a doctor who has access to an entire library of medical knowledge at their fingertips—a game changer for patient care!
Here’s a quick look at some industries and how they are benefiting from AI and data science:
Industry | Application of AI/Data Science | Impact |
---|---|---|
Retail | Predictive Analytics | Improved inventory management and customer satisfaction |
Entertainment | Personalized Recommendations | Increased user engagement and retention |
Finance | Fraud Detection | Enhanced security and reduced losses |
Healthcare | Disease Diagnosis | Improved patient outcomes and treatment efficiency |
These examples illustrate just a fraction of the incredible potential that AI and data science hold for businesses. As these technologies continue to evolve, we can expect even more innovative applications to emerge, paving the way for a future where data-driven decision-making is the norm. The question is, are you ready to embrace this change and leverage these technologies for your own business success?
Q: How can small businesses benefit from AI and data science?
A: Small businesses can leverage AI and data science to gain insights into customer behavior, optimize marketing strategies, and improve operational efficiency, often with minimal investment.
Q: What are the common challenges faced when implementing AI solutions?
A: Common challenges include data quality issues, lack of skilled personnel, and resistance to change within the organization.
Q: Is machine learning the same as AI?
A: Machine learning is a subset of AI that focuses on the development of algorithms that allow computers to learn from and make predictions based on data.
Frequently Asked Questions
- What is the difference between AI and data science?
AI, or Artificial Intelligence, refers to the simulation of human intelligence processes by machines, especially computer systems. Data science, on the other hand, involves the extraction of insights and knowledge from data using various techniques and algorithms. While AI can be a tool used within data science, data science encompasses a broader range of data manipulation and analysis techniques.
- How does predictive analytics benefit businesses?
Predictive analytics helps businesses by leveraging historical data to forecast future trends. This enables companies to make informed decisions, optimize operations, and enhance customer experiences. For instance, businesses can predict customer behavior, improve inventory management, and ultimately drive revenue growth.
- What are the common challenges in implementing predictive analytics?
Some common challenges include data quality issues, which can lead to inaccurate predictions, and the need for skilled personnel who can interpret the data effectively. Additionally, integrating predictive models into existing business processes can be complex and require substantial investment in technology and training.
- How does customer segmentation work with AI and data science?
Customer segmentation involves dividing a customer base into distinct groups based on specific characteristics, behaviors, or preferences. AI and data science facilitate this process by analyzing vast amounts of data to identify patterns that inform targeted marketing strategies, leading to improved customer engagement and satisfaction.
- What is the role of machine learning in business?
Machine learning, a subset of AI, plays a crucial role in business by enabling organizations to analyze large datasets and uncover hidden patterns. This capability allows businesses to enhance decision-making processes, optimize operations, and deliver personalized customer experiences, ultimately driving growth and efficiency.
- What is the difference between supervised and unsupervised learning?
Supervised learning involves training a model on a labeled dataset, meaning the input data is paired with the correct output. In contrast, unsupervised learning deals with unlabeled data, allowing the model to identify patterns and groupings without prior instructions. Both methods have unique applications in business, such as fraud detection and market segmentation.
- Can you provide examples of businesses using machine learning successfully?
Absolutely! Companies like Amazon and Netflix use machine learning algorithms to analyze user behavior and provide personalized recommendations. Additionally, financial institutions employ machine learning for fraud detection and risk assessment, enhancing both security and customer trust.