Crafting an Ethical AI Framework: Challenges and Opportunities
The rapid advancement of artificial intelligence (AI) technology has ushered in a new era of possibilities, but it also brings with it a myriad of ethical dilemmas that we must confront. As we delve into the complexities of developing an ethical AI framework, it's crucial to recognize that this journey is not merely about technology; it's about the people who create, use, and are affected by these systems. The challenge lies in crafting guidelines that not only ensure responsible AI deployment but also foster public trust and societal benefit. So, how do we navigate this intricate landscape? Let's explore the fundamental principles, key challenges, and exciting opportunities that await us in the realm of ethical AI.
At its core, ethical AI is about embedding values such as fairness, transparency, and accountability into the design and deployment of AI systems. But what do these terms really mean? Fairness ensures that AI systems treat all individuals equitably, avoiding discrimination based on race, gender, or socioeconomic status. Transparency involves making AI decision-making processes understandable to users and stakeholders, while accountability requires that there are mechanisms in place to hold developers and organizations responsible for the outcomes of their AI systems. The integration of these principles is not just a technical challenge; it's a philosophical one, requiring us to rethink how we define and measure success in AI.
Implementing an ethical AI framework is fraught with challenges that can feel overwhelming. From technological limitations to regulatory hurdles, the path is anything but straightforward. Each obstacle presents unique complications that must be addressed to establish a universally accepted standard for ethical AI. For instance, how do we ensure that the algorithms we develop are free from bias? And what about the legal implications of using personal data in AI training? These are just a few of the pressing questions that require our attention.
One of the most significant hurdles in the quest for ethical AI is the technological constraints we face. Current algorithms often fall short in addressing critical issues such as bias, privacy, and security. For example, many machine learning models are trained on datasets that may not accurately represent the diversity of the population, leading to biased outcomes. To combat this, we need innovative solutions and ongoing research to develop algorithms that can adapt and learn from diverse data sources, ensuring equitable outcomes across various contexts. This is akin to teaching a child; if they only learn from one perspective, their understanding of the world will be limited.
Algorithmic bias is a significant concern in AI ethics, as it can perpetuate existing inequalities and create new forms of discrimination. Developers must implement strategies to identify and mitigate bias in their systems. This involves not only refining the algorithms themselves but also critically examining the data used for training. By employing techniques such as diversity audits and bias detection tools, we can work towards ensuring that AI systems deliver equitable outcomes for all populations.
Data privacy is another critical concern in ethical AI. As AI systems require vast amounts of data to function effectively, balancing the need for information with the protection of personal privacy is paramount. Robust measures must be in place to safeguard sensitive information, while also allowing for the necessary data flow to train AI systems. This delicate balance is akin to walking a tightrope; one misstep could lead to significant repercussions for individuals and organizations alike.
Navigating the regulatory landscape is essential for ethical AI development. The differing laws and guidelines across jurisdictions can create confusion and impede progress toward standardized practices. For instance, while the European Union has established strict regulations surrounding data protection, other regions may lack comprehensive frameworks. This inconsistency can complicate the efforts of organizations striving to develop ethical AI solutions. Therefore, it is vital for stakeholders to engage in dialogue and collaborate on creating cohesive regulatory guidelines that promote ethical practices across the board.
Despite the challenges, the landscape of ethical AI is ripe with opportunities. By fostering collaboration among stakeholders and leveraging emerging technologies, we can enhance ethical practices in AI development. Imagine a world where governments, businesses, and civil society work together to create a framework that not only addresses ethical concerns but also promotes innovation. This collaborative approach can lead to the sharing of best practices and resources, ultimately resulting in more effective ethical AI frameworks.
Building partnerships among various stakeholders is crucial for the advancement of ethical AI. When governments, businesses, and civil society unite, they can share insights, resources, and best practices that contribute to a more comprehensive understanding of ethical AI. This collaboration can take many forms, including joint research initiatives, public-private partnerships, and community engagement efforts. By pooling our knowledge and expertise, we can create a more robust ethical AI ecosystem that benefits everyone.
Emerging technologies such as blockchain and federated learning offer innovative solutions to enhance transparency and accountability in AI systems. Blockchain, for example, can provide a secure and immutable record of AI decision-making processes, allowing stakeholders to trace the origins of decisions. Federated learning enables AI models to be trained across decentralized data sources, enhancing privacy while still allowing for collaborative learning. These technologies pave the way for more ethical applications of AI, transforming the way we think about accountability in the digital age.
- What is ethical AI? Ethical AI refers to the design and deployment of artificial intelligence systems that prioritize fairness, transparency, and accountability.
- Why is addressing algorithmic bias important? Addressing algorithmic bias is crucial to ensure that AI systems do not perpetuate existing inequalities and provide equitable outcomes for all individuals.
- How can emerging technologies improve ethical AI? Technologies like blockchain and federated learning can enhance transparency and accountability in AI systems, leading to more ethical applications.
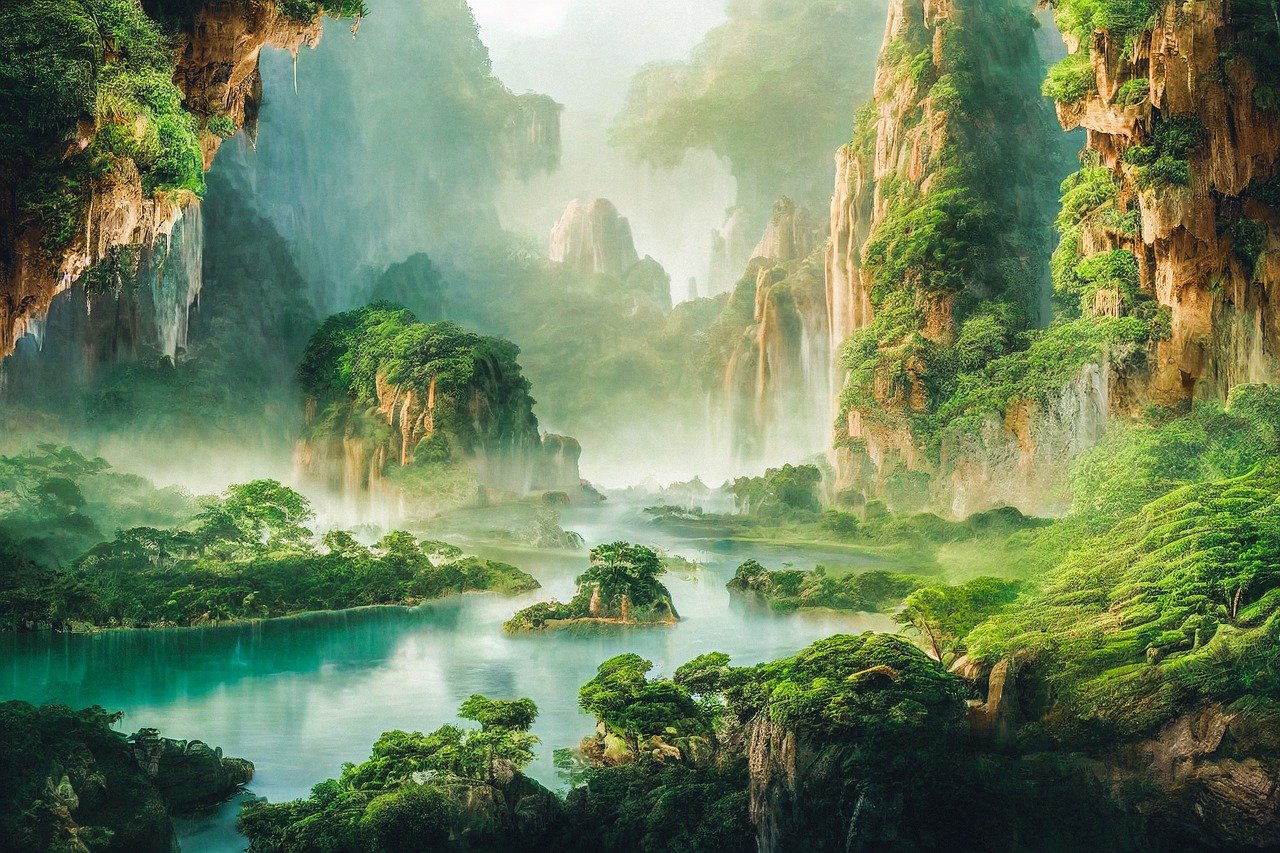
Understanding Ethical AI
When we talk about ethical AI, we're diving into a realm that’s not just about technology, but about values and principles. At its core, ethical AI aims to ensure that artificial intelligence serves humanity in a way that is fair, transparent, and accountable. Imagine AI as a powerful tool—a double-edged sword, if you will. It has the potential to revolutionize our lives, but without proper ethical guidelines, it can also lead to significant harm. So, what does it mean to create an ethical AI framework?
First off, let’s break down the fundamental principles that guide ethical AI:
- Fairness: This principle emphasizes that AI systems should be designed to treat all individuals and groups equitably. No one should be disadvantaged because of biased algorithms or data.
- Transparency: Ethical AI should operate in a manner that is open and understandable. Users should know how decisions are made, which helps build trust in AI systems.
- Accountability: Developers and organizations must be responsible for the outcomes of their AI systems. If an AI makes a mistake, there should be a clear path to address the issue.
Now, why are these principles so crucial? Let’s consider fairness as an example. Think about a hiring algorithm that unintentionally favors one demographic over another. If we don’t address this bias, we’re not just making an unfair decision; we’re perpetuating inequality. The implications can ripple through society, affecting job opportunities and economic stability. This is why embedding fairness into AI systems is not just a technical challenge but a moral imperative.
Next up is transparency. Picture this: you’re using a navigation app that suddenly reroutes you without explanation. Frustrating, right? Now, imagine if AI systems operated in the same opaque manner. Users would be left in the dark, leading to distrust. Transparency allows users to understand the reasoning behind AI decisions, which is essential for fostering a positive relationship between humans and machines.
Lastly, let’s talk about accountability. In the world of AI, accountability means that when an AI system fails, there should be a clear mechanism to address the failure. This could involve human oversight or regulatory frameworks that ensure AI systems are regularly audited for compliance with ethical standards. Without accountability, we risk creating a technology that operates without checks and balances, which can lead to dire consequences.
In conclusion, understanding ethical AI is about more than just adhering to a set of rules; it’s about embracing a mindset that prioritizes human welfare. As we continue to innovate and integrate AI into our daily lives, we must keep these principles at the forefront. After all, the goal is to create AI that not only enhances our capabilities but also aligns with our shared values as a society.

Key Challenges in Implementation
Implementing an ethical AI framework is no walk in the park. In fact, it’s more like navigating a complex maze filled with twists and turns. The journey is fraught with **numerous obstacles** that can trip up even the most seasoned developers and organizations. From technological limitations to regulatory hurdles, the path to establishing a universally accepted standard for ethical AI is anything but straightforward. Each challenge presents its own set of complications, making it essential for stakeholders to remain vigilant and adaptable.
One of the most pressing issues in the realm of ethical AI is the **technological constraints** that developers face. Current algorithms often fall short when it comes to adequately addressing critical concerns such as bias, privacy, and security. Imagine trying to build a skyscraper on a shaky foundation; without a solid technological base, the entire structure risks collapsing under its own weight. To overcome these limitations, we need **innovative solutions** and continuous research to improve the algorithms and systems we rely on. This means investing time and resources into developing technologies that can handle the complexities of ethical AI.
Algorithmic bias is a significant challenge that developers must confront. It’s like trying to bake a cake with the wrong ingredients; no matter how skilled the baker is, the result will be off. Bias can seep into AI systems through various channels, including skewed training data or flawed algorithm design. To combat this, developers should implement strategies that actively mitigate bias and promote equitable outcomes. This involves rigorous testing, diverse data collection, and the establishment of best practices to ensure fairness across different populations and contexts.
Data privacy is another critical concern that cannot be overlooked. In today’s digital age, personal information is more vulnerable than ever, and ethical AI must prioritize the protection of this data. It’s a balancing act—while we need data to train AI systems effectively, we must also safeguard individuals' privacy. Developers must adopt robust measures that not only comply with existing regulations but also foster user trust. This could mean implementing advanced encryption techniques, anonymizing data, or even utilizing federated learning approaches that allow for data analysis without compromising individual privacy.
Navigating the regulatory landscape is essential for ethical AI development. With differing laws and guidelines across jurisdictions, organizations can find themselves in a state of confusion. It’s like trying to follow a recipe that keeps changing mid-way; it’s frustrating and can lead to disastrous results. The lack of standardized practices can impede progress and stifle innovation. Therefore, it’s crucial for stakeholders to engage in dialogue with lawmakers and regulatory bodies to advocate for clear, coherent guidelines that support ethical AI development. Building a collaborative environment can help streamline regulations and promote a unified approach to ethical standards.
In summary, while the challenges in implementing an ethical AI framework are daunting, they are not insurmountable. By acknowledging these obstacles and working collaboratively, stakeholders can pave the way for responsible and ethical artificial intelligence that benefits society as a whole.
- What is ethical AI? Ethical AI refers to the development and deployment of artificial intelligence systems that prioritize fairness, accountability, and transparency.
- Why is addressing algorithmic bias important? Addressing algorithmic bias is crucial to ensure that AI systems deliver equitable outcomes and do not perpetuate existing inequalities.
- How can organizations enhance data privacy in AI? Organizations can enhance data privacy by implementing robust encryption methods, anonymizing data, and adopting federated learning techniques.
- What role do regulations play in ethical AI? Regulations help establish clear guidelines and standards for ethical AI development, ensuring that organizations adhere to best practices and protect users' rights.
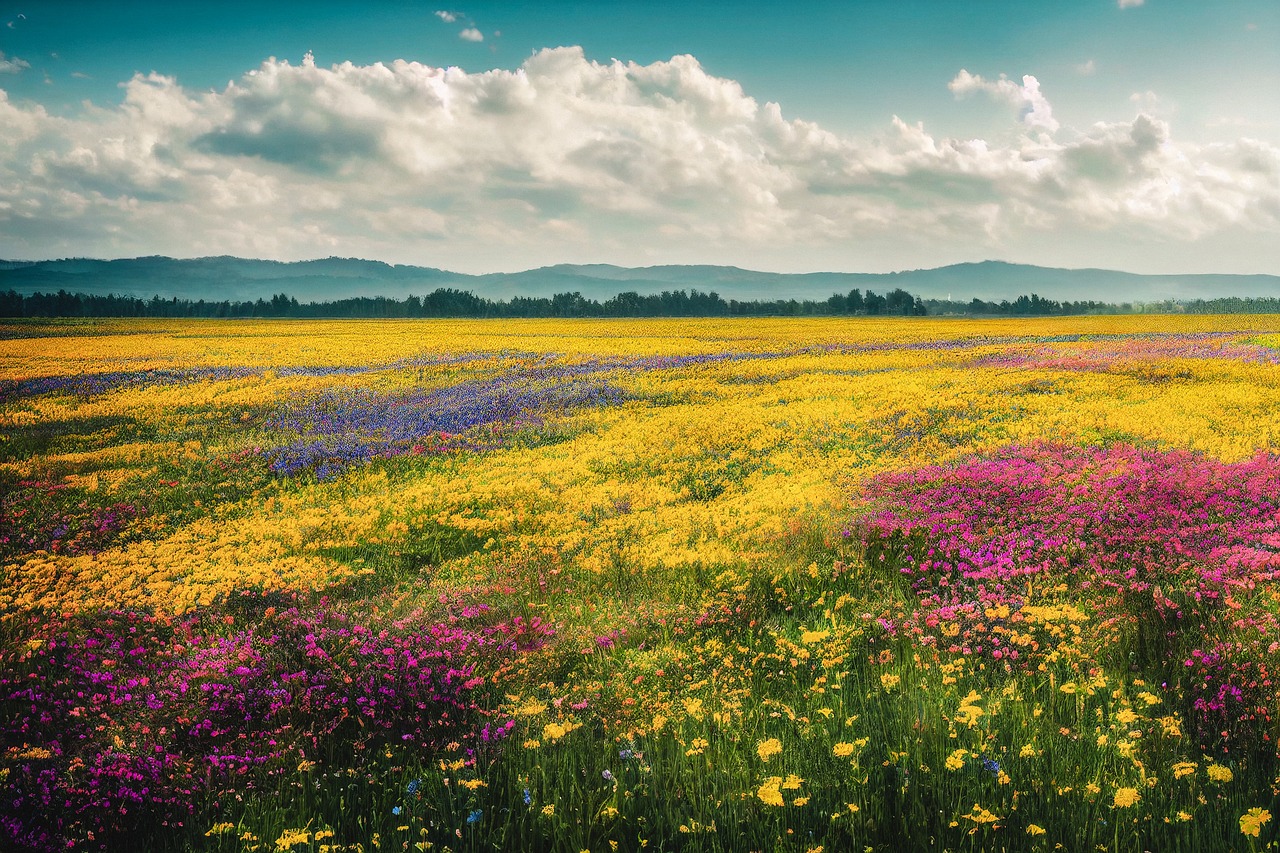
Technological Limitations
The journey towards ethical AI is not a smooth sail; it’s more like navigating through a stormy sea filled with hidden rocks and unpredictable waves. One of the most significant hurdles we face is the that exist within current AI systems. These limitations can severely hinder our ability to create AI that is not only effective but also ethical. Imagine trying to build a house on a shaky foundation; no matter how beautiful the design, it’s likely to crumble. Similarly, if the algorithms we rely on are flawed, the ethical framework we aim to construct will be unstable and potentially harmful.
One of the primary issues is algorithmic bias. Algorithms are designed based on the data fed into them, and if that data is biased, the outcomes will be too. For instance, if a facial recognition system is trained predominantly on images of one demographic, it may struggle to accurately identify individuals from other backgrounds. This not only raises ethical questions but also poses real-world risks, such as misidentifying individuals in critical situations. Developers must actively seek to identify and mitigate these biases. This can be achieved through techniques such as:
- Conducting regular audits of AI systems
- Utilizing diverse datasets for training
- Implementing continuous feedback loops to refine algorithms
Moreover, the issue of data privacy cannot be overlooked. In our quest for more sophisticated AI, we often require vast amounts of personal data. However, collecting and utilizing this data raises serious concerns about how personal information is handled. Striking a balance between the need for data and the imperative to protect individual privacy is a tightrope walk. Developers must implement robust security measures to safeguard personal information, which can sometimes conflict with the need for transparency in AI operations. It’s a classic case of needing to have your cake and eat it too.
Lastly, the rapid pace of technological advancement means that existing solutions may quickly become obsolete. As we strive to enhance AI systems, we must also be prepared for the challenges that arise from integrating new technologies. For example, while machine learning models can improve over time, they can also become more complex and less interpretable, complicating our efforts to ensure ethical standards are met. The challenge lies in ensuring that as we innovate, we do not lose sight of the ethical implications of these advancements.
In summary, the road to ethical AI is fraught with technological challenges that require ongoing research, innovative solutions, and a commitment to continuous improvement. By addressing these limitations head-on, we can move closer to a future where AI is not only powerful but also responsible and fair.
- What is algorithmic bias? Algorithmic bias occurs when an AI system produces results that are systematically prejudiced due to erroneous assumptions in the machine learning process.
- How can we mitigate data privacy concerns in AI? Implementing strong data protection measures, anonymizing data, and ensuring compliance with regulations like GDPR are crucial steps in addressing data privacy.
- Why is transparency important in AI? Transparency helps build trust between users and AI systems, allowing individuals to understand how decisions are made and ensuring accountability.
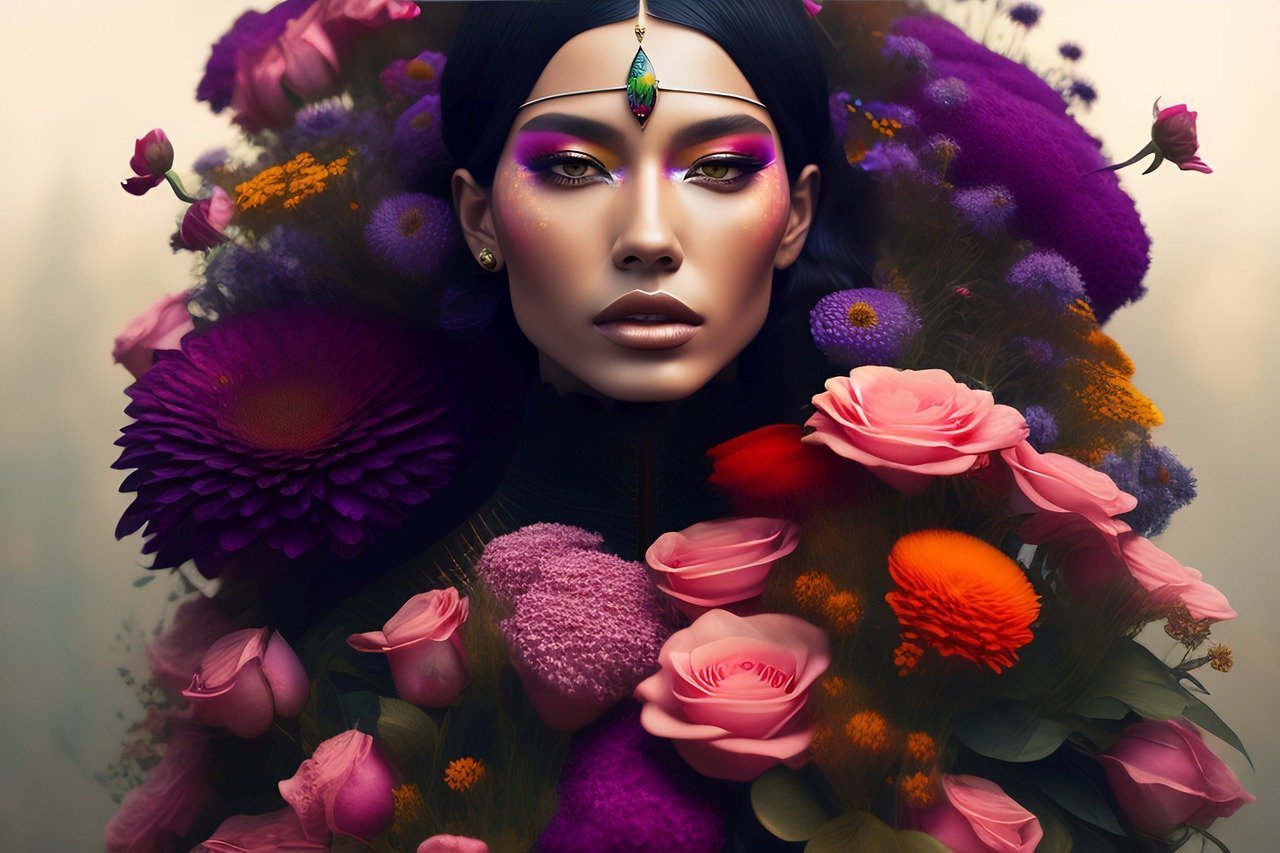
Addressing Algorithmic Bias
Algorithmic bias is one of the most pressing challenges in the realm of ethical AI. It refers to the systematic and unfair discrimination that can occur when AI systems make decisions based on biased data. Imagine a world where your chances of getting a loan, a job, or even a medical diagnosis depend on flawed algorithms that have been trained on skewed data. This is not just a hypothetical scenario; it’s a reality that many individuals face today. Therefore, addressing algorithmic bias is not merely an option; it’s a necessity.
To tackle this issue, developers and organizations must first recognize that bias can infiltrate AI systems at various stages, from data collection to algorithm design. For instance, if the training data used to develop an AI model is not representative of the entire population, the outcomes can disproportionately favor one group over another. This can lead to significant disparities in treatment, opportunities, and outcomes. A recent study highlighted that facial recognition systems had a higher error rate for individuals with darker skin tones compared to those with lighter skin tones. Such discrepancies highlight the urgent need for strategies that mitigate bias.
One effective approach to address algorithmic bias involves implementing diverse data sets. By ensuring that the data used to train AI models includes a wide range of perspectives and demographics, developers can create more equitable systems. Additionally, continuous monitoring and auditing of AI systems can help identify and rectify biases that may emerge post-deployment. It’s akin to having a regular health check-up; just because a system is performing well today doesn’t mean it will remain unbiased tomorrow.
Moreover, organizations can adopt the following strategies to combat algorithmic bias:
- Bias Audits: Regularly conduct audits of AI systems to identify and address any biases that may exist.
- Inclusive Design: Involve diverse teams in the development process, ensuring that multiple perspectives are considered.
- User Feedback: Encourage feedback from users to identify potential biases and areas for improvement.
Ultimately, addressing algorithmic bias is not just about improving technology; it’s about fostering trust and accountability in AI systems. As we navigate this complex landscape, it’s crucial for developers, organizations, and stakeholders to work collaboratively, sharing insights and best practices to create a more just and equitable future. The journey towards ethical AI is multifaceted, but by prioritizing the mitigation of algorithmic bias, we can take significant strides towards achieving it.
- What is algorithmic bias? Algorithmic bias refers to systematic and unfair discrimination in AI systems that can result from biased training data or flawed algorithms.
- How can bias be identified in AI systems? Bias can be identified through regular audits, analyzing outcomes across different demographics, and soliciting user feedback.
- What role does diverse data play in mitigating bias? Diverse data sets ensure that AI models are trained on a wide range of perspectives, reducing the likelihood of biased outcomes.

Enhancing Data Privacy
In the rapidly evolving landscape of artificial intelligence, data privacy has emerged as a paramount concern. As AI systems increasingly rely on vast amounts of personal data to function effectively, the challenge lies in balancing the need for this data with the imperative to protect individuals' privacy. Think of it like a tightrope walk; on one side, we have the benefits of AI that can revolutionize industries, and on the other, the risk of infringing on personal privacy. This delicate balance is crucial for fostering trust among users and ensuring the responsible use of AI technologies.
To enhance data privacy in AI systems, organizations must adopt a multi-faceted approach that encompasses several key strategies. These strategies not only safeguard personal information but also promote ethical AI practices. Here are some essential measures that can be implemented:
- Data Minimization: Collect only the data that is necessary for the AI system to function. This reduces the risk associated with storing excessive personal information.
- Anonymization Techniques: Employ methods to anonymize data, ensuring that individuals cannot be easily identified from the datasets used in AI training.
- Robust Consent Mechanisms: Implement clear and transparent consent processes, allowing users to understand how their data will be used and giving them control over their information.
- Regular Audits: Conduct regular audits of AI systems to ensure compliance with data privacy regulations and to identify potential vulnerabilities.
Moreover, organizations should consider integrating privacy-preserving technologies such as federated learning. This approach allows AI models to be trained on decentralized data sources without the need to share sensitive information. Imagine a scenario where multiple hospitals collaborate to improve a medical AI model without ever sharing patient data. This not only enhances data privacy but also leads to better, more generalized AI outcomes.
Furthermore, the legal landscape surrounding data privacy is continually shifting, with regulations like the General Data Protection Regulation (GDPR) setting stringent standards. Companies must stay ahead of these regulations, ensuring that their AI frameworks are compliant while also advocating for policies that protect user privacy. This proactive stance not only mitigates legal risks but also positions organizations as leaders in ethical AI development.
In conclusion, enhancing data privacy in AI is not just about compliance; it's about establishing a foundation of trust with users. By implementing robust privacy measures and leveraging innovative technologies, organizations can pave the way for a future where AI can thrive without compromising individual rights. This commitment to data privacy will ultimately drive the responsible and ethical deployment of AI systems, benefiting both businesses and society as a whole.
- What is data privacy in the context of AI?
Data privacy refers to the protection of personal information collected and used by AI systems, ensuring that individuals' rights are respected and their data is handled responsibly. - Why is data privacy important for AI?
Data privacy is crucial for building trust among users, complying with regulations, and preventing misuse of personal information, which can lead to serious ethical and legal implications. - How can organizations enhance data privacy?
Organizations can enhance data privacy by implementing data minimization practices, anonymization techniques, robust consent mechanisms, and conducting regular audits of their AI systems. - What role does federated learning play in data privacy?
Federated learning allows AI models to be trained on decentralized data, enhancing privacy by ensuring that sensitive information does not need to be shared or stored centrally.

Regulatory and Legal Hurdles
When it comes to crafting an ethical AI framework, navigating the regulatory and legal landscape can feel like trying to find your way through a maze blindfolded. Different countries and regions have their own sets of laws and regulations governing technology and data privacy, which can create a patchwork of compliance requirements. This inconsistency can lead to confusion for developers and organizations looking to implement ethical AI practices. Imagine trying to build a house with bricks that come in various sizes and shapes; it’s a challenge!
One of the key issues is the lack of a universal standard. While some regions, such as the European Union, have made strides with regulations like the General Data Protection Regulation (GDPR), other areas may lack comprehensive legal frameworks to address AI ethics. This disparity makes it challenging for companies operating globally to adhere to ethical practices without running afoul of local laws. For instance, a company might develop an AI tool that complies with European regulations but inadvertently violate privacy laws in another country.
Moreover, the rapidly evolving nature of technology means that laws often lag behind innovation. This creates a scenario where developers are left in a state of uncertainty, unsure whether their AI systems will comply with future regulations. In many cases, organizations may find themselves investing heavily in compliance measures, only to discover that the rules have changed or that new regulations have been introduced. This constant state of flux can stifle innovation and lead to a hesitance among businesses to fully embrace AI technologies.
To illustrate the complexity of these challenges, consider the following table showing the differences in AI regulations across various regions:
Region | Key Regulations | Focus Areas |
---|---|---|
European Union | GDPR, AI Act (proposed) | Data protection, accountability |
United States | Varies by state (e.g., CCPA in California) | Consumer privacy, data security |
China | Personal Information Protection Law (PIPL) | Data sovereignty, security |
As we can see, the regulatory environment is not only diverse but also constantly changing. This creates a significant hurdle for organizations aiming to implement ethical AI. Additionally, differing stakeholder interests can complicate the dialogue around regulation. For example, while consumers may prioritize data privacy, businesses might focus on operational flexibility, leading to conflicting priorities. It's crucial for all stakeholders to engage in open discussions to bridge these gaps and work towards a common understanding of ethical AI.
In conclusion, addressing these regulatory and legal hurdles is essential for the successful development of ethical AI. Organizations must remain agile and proactive, continuously adapting to the evolving legal landscape while advocating for clearer, more consistent regulations. By fostering collaboration among stakeholders and pushing for comprehensive legal frameworks, we can pave the way for a future where ethical AI thrives.
- What are the main challenges in implementing ethical AI? The main challenges include technological limitations, regulatory hurdles, and differing stakeholder interests.
- How do regulations impact AI development? Regulations can create confusion and compliance challenges, especially when laws differ across regions.
- What can organizations do to navigate these hurdles? Organizations should stay informed about regulatory changes and actively engage with stakeholders to advocate for clearer laws.
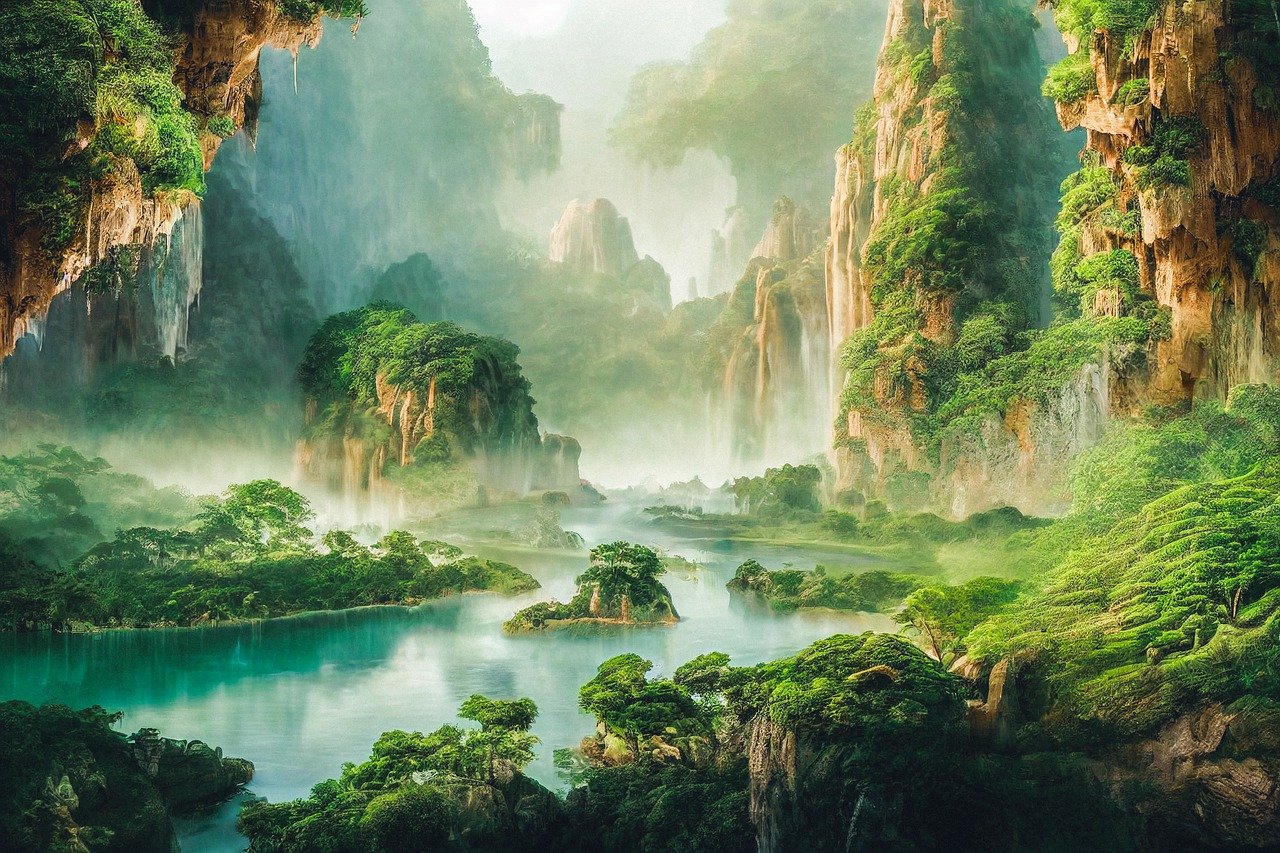
Opportunities for Ethical AI
Despite the myriad challenges that accompany the development of ethical AI, there are significant opportunities that can drive progress and innovation in this field. As we navigate through the complexities of artificial intelligence, one of the most promising avenues lies in fostering collaboration among various stakeholders. Imagine a world where governments, businesses, and civil society join forces to create a united front in ethical AI practices. This collaboration can lead to the sharing of best practices, resources, and knowledge, ultimately resulting in more comprehensive and effective ethical frameworks. By working together, these entities can address common concerns and establish guidelines that promote fairness and accountability.
Furthermore, the advent of emerging technologies presents a unique chance to enhance ethical practices in AI development. Technologies such as blockchain and federated learning are not just buzzwords; they hold the potential to revolutionize how we approach transparency and accountability in AI systems. For instance, blockchain can create immutable records of AI decision-making processes, allowing stakeholders to trace how decisions are made and ensuring that they are fair and just. Similarly, federated learning enables AI models to be trained on decentralized data, which can significantly enhance data privacy and security. This means that organizations can develop powerful AI systems without compromising the personal information of individuals.
Moreover, as society becomes more aware of the implications of AI, there is a growing demand for ethical considerations in technology. This shift in public perception creates a fertile ground for businesses to differentiate themselves by adopting ethical AI practices. Companies that prioritize ethical AI can build trust with their customers, leading to increased loyalty and a competitive edge in the market. In this landscape, ethical AI isn't just a moral obligation; it’s also a strategic advantage.
To summarize, the opportunities for ethical AI are vast and varied. By embracing collaboration among stakeholders and leveraging emerging technologies, we can pave the way for a future where AI is developed and deployed responsibly. As we stand on the brink of this exciting frontier, the question remains: are we ready to seize these opportunities and transform the landscape of artificial intelligence for the better?
- What is ethical AI?
Ethical AI refers to the development and deployment of artificial intelligence systems that adhere to principles such as fairness, transparency, and accountability. - Why is collaboration important in developing ethical AI?
Collaboration among stakeholders helps share best practices, resources, and knowledge, leading to more effective ethical frameworks and solutions. - How can emerging technologies enhance ethical AI?
Technologies like blockchain and federated learning can improve transparency, accountability, and data privacy in AI systems. - What are the benefits of adopting ethical AI practices for businesses?
Businesses that prioritize ethical AI can build trust with customers, leading to increased loyalty and a competitive edge in the market.
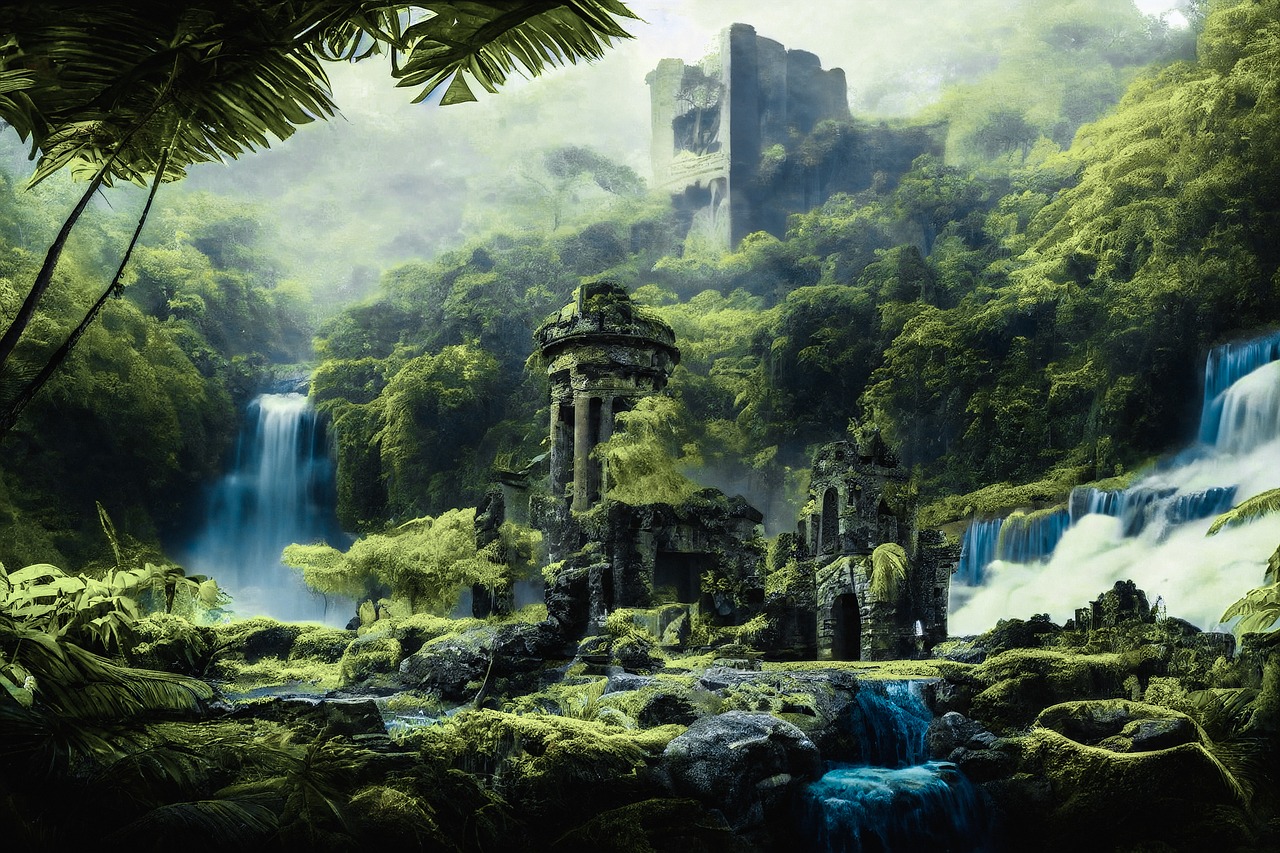
Collaboration Among Stakeholders
In the rapidly evolving landscape of artificial intelligence, the importance of cannot be overstated. As we navigate the complexities of ethical AI, it becomes clear that no single entity can tackle these challenges alone. Instead, a concerted effort involving governments, businesses, academia, and civil society is essential to create a robust ethical framework that is both effective and comprehensive.
Imagine trying to solve a jigsaw puzzle where each piece represents a different stakeholder's perspective—each piece is unique, and together they create a complete picture of ethical AI. By fostering collaboration, we can ensure that diverse viewpoints are considered, leading to solutions that are more inclusive and representative of the society we live in. This collaborative approach not only enhances the development of ethical guidelines but also encourages the sharing of best practices and resources.
One of the most significant benefits of stakeholder collaboration is the ability to address the multifaceted nature of AI ethics. For instance, when governments, businesses, and non-profit organizations come together, they can pool their expertise and insights to tackle issues such as algorithmic bias and data privacy. This collective intelligence can lead to innovative strategies that might not have been possible in isolation. Furthermore, by engaging in open dialogues and partnerships, stakeholders can build trust, which is crucial for the successful implementation of ethical AI practices.
Moreover, collaboration can pave the way for the development of standardized practices across different jurisdictions. When stakeholders from various regions work together, they can identify common challenges and create frameworks that are adaptable yet consistent. This is particularly vital in a globalized world where AI systems are deployed across borders. A unified approach can help mitigate the confusion caused by differing regulations and promote a more harmonious adoption of ethical AI principles.
To illustrate the potential of stakeholder collaboration, consider the following table showcasing successful initiatives:
Initiative | Stakeholders Involved | Outcome |
---|---|---|
AI Ethics Consortium | Governments, Tech Companies, NGOs | Developed guidelines for ethical AI deployment |
Global AI Partnership | International Organizations, Academics | Shared research on algorithmic fairness |
Data Privacy Coalition | Businesses, Civil Rights Groups | Established best practices for data handling |
As we move forward, it is crucial to recognize that collaboration is not just a beneficial approach; it is a necessity for the responsible development of AI. By working together, stakeholders can create a more equitable and ethical AI landscape that serves the interests of all members of society. The road ahead may be challenging, but with a united front, we can turn obstacles into opportunities, ensuring that the future of AI is not only innovative but also ethical.
Q1: Why is collaboration among stakeholders important for ethical AI?
A1: Collaboration is vital because it brings together diverse perspectives, enabling a more comprehensive approach to addressing the complexities of ethical AI.
Q2: Who are the key stakeholders in ethical AI?
A2: Key stakeholders include governments, businesses, academia, and civil society organizations, all of which play a role in shaping ethical AI practices.
Q3: How can stakeholders share best practices?
A3: Stakeholders can share best practices through initiatives, workshops, and collaborative projects that focus on common challenges and solutions in AI ethics.

Leveraging Emerging Technologies
In the rapidly evolving landscape of artificial intelligence, emerging technologies play a pivotal role in shaping the future of ethical AI. By harnessing these innovations, we can address some of the most pressing challenges associated with AI development. For instance, technologies like blockchain and federated learning are not just buzzwords; they hold the potential to revolutionize how we ensure transparency and accountability in AI systems.
Take blockchain, for example. This decentralized ledger technology is renowned for its ability to provide a secure and transparent way to record transactions. When applied to AI, it can create a verifiable audit trail for AI decision-making processes. Imagine a world where every decision made by an AI system can be traced back to its origin, allowing stakeholders to understand how and why decisions were made. This level of transparency not only builds trust among users but also ensures that AI systems adhere to ethical guidelines. By integrating blockchain into AI frameworks, organizations can enhance their credibility and demonstrate their commitment to ethical practices.
On the other hand, federated learning offers a unique approach to data privacy. Instead of centralizing data in one location, federated learning allows AI models to be trained across multiple decentralized devices or servers. This means that sensitive data never leaves its original location, significantly reducing the risk of data breaches. As a result, organizations can develop robust AI systems without compromising user privacy. This technology is particularly beneficial in sectors like healthcare, where patient confidentiality is paramount. By leveraging federated learning, developers can create AI solutions that respect privacy while still delivering valuable insights.
Moreover, the integration of these technologies can foster collaboration among various stakeholders, including governments, businesses, and academia. By working together, these entities can share knowledge and resources, leading to the development of more comprehensive ethical AI frameworks. For instance, regulatory bodies can collaborate with tech companies to establish guidelines that promote ethical practices while still encouraging innovation. This synergy can pave the way for a future where ethical AI is not just an aspiration but a reality.
In conclusion, the potential of emerging technologies to enhance ethical AI cannot be overstated. By leveraging innovations like blockchain and federated learning, we can address critical issues such as transparency and data privacy, creating AI systems that are not only effective but also responsible. As we continue to explore these possibilities, it's crucial for all stakeholders to engage in open dialogue and collaboration, ensuring that the future of AI aligns with our ethical values.
- What is ethical AI? Ethical AI refers to the development and deployment of artificial intelligence systems that prioritize fairness, transparency, and accountability.
- How does blockchain enhance AI ethics? Blockchain provides a secure and transparent way to record AI decision-making processes, ensuring accountability and trust.
- What is federated learning? Federated learning is a decentralized approach to training AI models, allowing data to remain on local devices while still contributing to model improvement.
- Why is collaboration important in ethical AI? Collaboration among stakeholders fosters the sharing of best practices and resources, leading to more effective ethical AI frameworks.
Frequently Asked Questions
- What is ethical AI?
Ethical AI refers to the development and deployment of artificial intelligence systems that prioritize fairness, transparency, and accountability. It aims to ensure that AI technologies are designed to respect human rights and promote social good, while minimizing harm to individuals and communities.
- Why is addressing algorithmic bias important?
Addressing algorithmic bias is crucial because biased AI systems can lead to unfair outcomes, perpetuating discrimination against certain groups. By implementing strategies to mitigate bias, developers can create more equitable AI applications that serve diverse populations effectively.
- What are the main challenges in implementing ethical AI?
There are several key challenges in implementing ethical AI, including technological limitations, regulatory hurdles, and differing stakeholder interests. These obstacles complicate the establishment of universally accepted standards and practices for AI ethics.
- How can data privacy be ensured in AI systems?
Ensuring data privacy in AI systems requires robust measures such as data encryption, anonymization, and strict access controls. Balancing the need for data to train AI while protecting personal information is a critical aspect of ethical AI development.
- What role do stakeholders play in ethical AI?
Stakeholders, including governments, businesses, and civil society, play a vital role in shaping ethical AI practices. By collaborating and sharing best practices, they can help create comprehensive frameworks that promote responsible AI development and usage.
- How can emerging technologies enhance ethical AI?
Emerging technologies like blockchain and federated learning can significantly enhance ethical AI by improving transparency and accountability. These technologies provide innovative solutions that help ensure AI systems operate fairly and responsibly.
- Is there a universal standard for ethical AI?
Currently, there is no universally accepted standard for ethical AI. However, ongoing discussions among stakeholders aim to establish common guidelines and best practices that can be adapted across different jurisdictions and industries.