Artificial Intelligence in the Future of GIS and Mapping
In today's rapidly evolving technological landscape, artificial intelligence (AI) is not just a buzzword; it's a game changer, especially in the field of Geographic Information Systems (GIS) and mapping. Imagine a world where maps are not just static images but dynamic tools that can predict future trends, optimize resource allocation, and enhance decision-making processes. This is not science fiction; it's the reality we are stepping into as AI integrates more deeply into GIS. The transformative role of AI in GIS is set to redefine how we understand and interact with spatial data, making our world more connected and efficient.
As we delve into the future, it's essential to recognize that GIS has come a long way. From its humble beginnings as a tool for cartographers to a sophisticated system that combines spatial analysis with real-time data, GIS has evolved to meet the complex demands of modern society. The integration of AI technologies is the next logical step in this evolution, promising to enhance data analysis capabilities and improve mapping accuracy.
AI's potential in GIS is vast, but it’s crucial to understand how specific algorithms contribute to this transformation. For instance, machine learning and neural networks are at the forefront of this revolution. These AI algorithms are not just enhancing the way we process data; they are fundamentally changing the landscape of spatial analysis. By leveraging these technologies, GIS can now perform tasks that were once thought to be the domain of human experts, such as predictive modeling and complex image classification.
Moreover, the impact of AI on urban planning is profound. Imagine cities that can adapt to the needs of their residents in real-time, where data-driven insights lead to sustainable development and smarter city designs. AI's role in promoting community engagement is equally significant, as it enables participatory planning processes that ensure every voice is heard. However, with great power comes great responsibility. As we embrace these advancements, we must also navigate the challenges and ethical considerations that arise, such as data privacy and algorithmic bias.
Looking ahead, the future of AI in GIS is filled with promise. New innovations will continue to emerge, offering solutions to pressing global challenges and enhancing our ability to analyze and visualize spatial data. As we stand on the brink of this exciting future, the question remains: how will we harness the power of AI to create a more informed and equitable world?
- What is GIS? Geographic Information Systems (GIS) are systems designed to capture, store, manipulate, analyze, manage, and present spatial or geographic data.
- How does AI enhance GIS? AI enhances GIS by improving data analysis, enabling predictive modeling, and automating complex tasks like image classification.
- What are the ethical concerns surrounding AI in GIS? Ethical concerns include data privacy, algorithmic bias, and the implications of automated decision-making in public policy.
- What is the future of AI in GIS? The future promises further advancements in technology, offering new opportunities for innovation and improved efficiency in tackling global challenges.
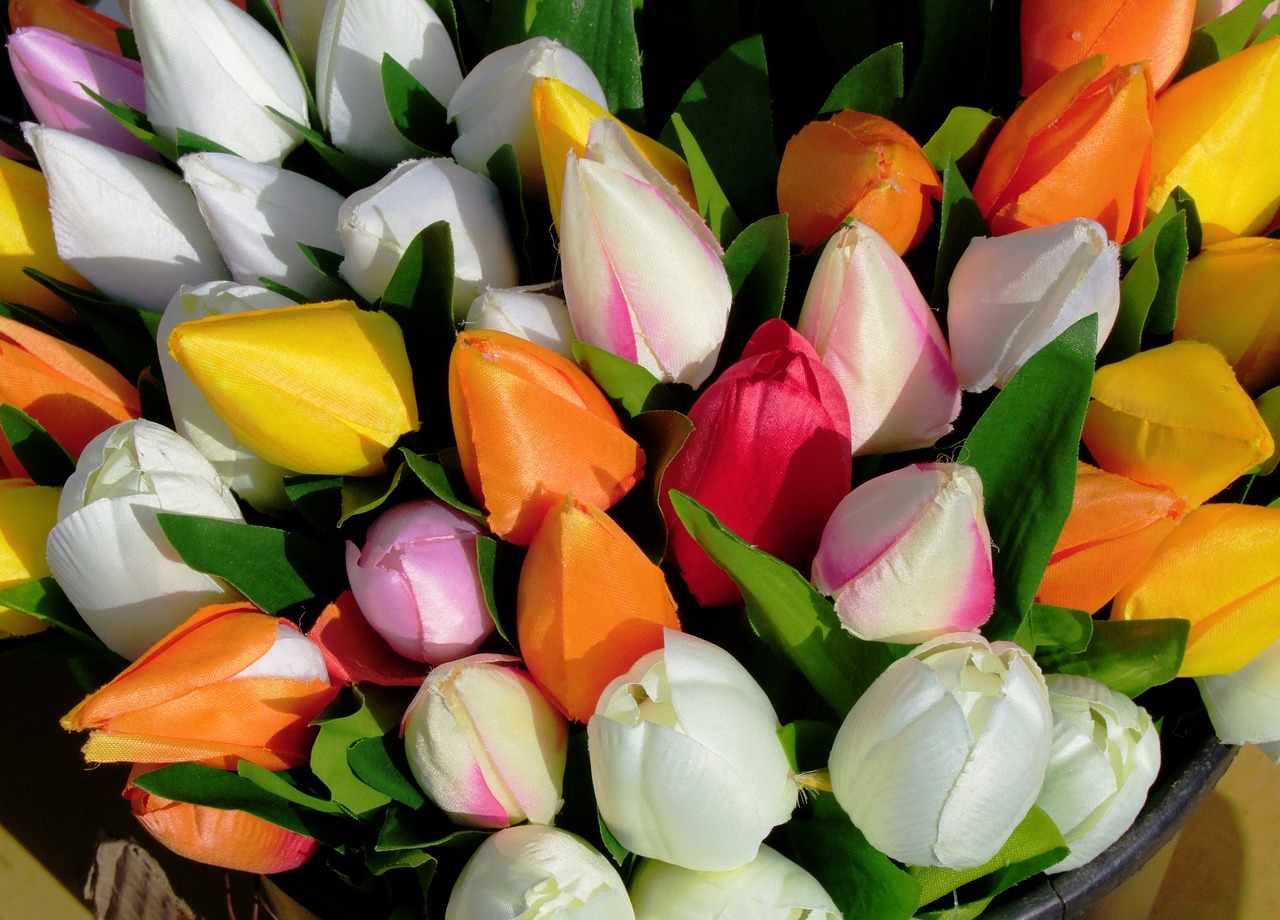
The Evolution of GIS
The journey of Geographic Information Systems (GIS) is nothing short of fascinating. From its humble beginnings to the sophisticated systems we see today, GIS has evolved dramatically to meet the growing demands of data analysis and mapping. Initially, GIS was primarily a tool for cartographers and geographers, allowing them to create maps and visualize spatial data. However, as technology advanced, so did the capabilities of GIS. The integration of computer technology in the 1960s marked a significant turning point, enabling users to manipulate and analyze geographic data with greater efficiency.
As we moved into the 1980s and 1990s, the advent of personal computers and software applications made GIS more accessible to a broader audience. This democratization of GIS technology allowed various sectors, including urban planning, environmental management, and transportation, to leverage spatial data for better decision-making. The introduction of the internet in the late 1990s further revolutionized GIS by enabling real-time data sharing and collaboration across geographic boundaries.
Today, GIS is not just about mapping; it’s about understanding the world through data. The incorporation of advanced technologies like Artificial Intelligence (AI) and machine learning has taken GIS to new heights. These innovations allow for more complex data analysis, predictive modeling, and enhanced spatial analysis capabilities. For instance, AI algorithms can process vast amounts of data in real-time, identifying patterns and trends that would be impossible for humans to discern. This capability is crucial for applications ranging from urban planning to environmental monitoring.
To illustrate the evolution of GIS, consider the following table that outlines key milestones in its development:
Year | Milestone |
---|---|
1960s | Introduction of computer-based mapping |
1980s | Personal computers make GIS accessible |
1990s | Internet enables real-time data sharing |
2000s | Integration of GPS technology into GIS |
2010s | AI and machine learning transform data analysis |
In essence, the evolution of GIS reflects a broader trend towards data-driven decision-making across various fields. As we continue to generate vast amounts of spatial data, the role of GIS will only become more critical. The integration of AI technologies into GIS not only enhances its capabilities but also opens up new avenues for innovation and efficiency. The future of GIS is bright, and it promises to play a pivotal role in addressing some of the world’s most pressing challenges.
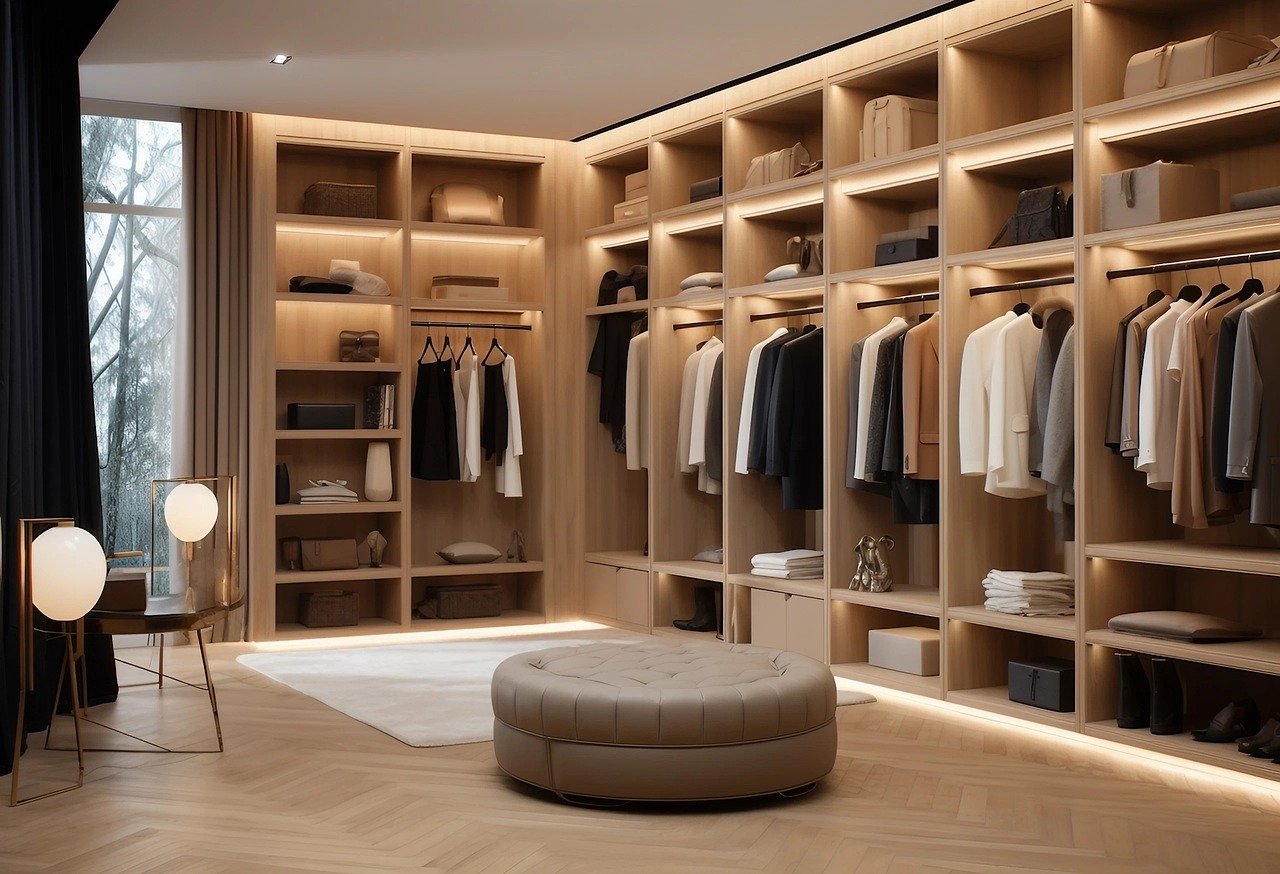
AI Algorithms in Mapping
The integration of artificial intelligence (AI) into Geographic Information Systems (GIS) has revolutionized the way we analyze and interpret spatial data. AI algorithms, particularly machine learning and neural networks, are at the forefront of this transformation. These technologies not only enhance data processing capabilities but also significantly improve spatial analysis, allowing for more informed decision-making across various sectors. Imagine being able to predict urban growth or environmental changes with remarkable accuracy—this is the promise of AI in mapping.
Machine learning algorithms are particularly effective in processing large datasets quickly and efficiently. By identifying patterns and trends within the data, these algorithms can make predictions about future developments. For instance, in urban planning, machine learning can analyze traffic patterns, population density, and land use to forecast how a city will evolve over the next decade. This predictive capability is invaluable for city planners who aim to create sustainable and livable environments.
Neural networks, on the other hand, take this a step further by mimicking the way the human brain works. They excel in complex data analysis tasks, such as image recognition and classification. In the context of GIS, neural networks can process satellite imagery to identify land cover types, detect changes over time, and even classify various features such as roads, buildings, and vegetation. This level of analysis is crucial for applications like remote sensing and environmental monitoring.
To illustrate the impact of these AI algorithms, consider the following table that outlines their applications in GIS:
AI Algorithm | Application in GIS | Benefits |
---|---|---|
Machine Learning | Predictive modeling | Improved accuracy in forecasting urban growth |
Machine Learning | Pattern recognition | Enhanced resource management |
Neural Networks | Image classification | Advanced remote sensing capabilities |
Neural Networks | Data analysis | Efficient processing of complex datasets |
Moreover, the synergy between machine learning and neural networks in GIS can lead to even more sophisticated applications. For example, combining these technologies can facilitate real-time data analysis, enabling cities to respond dynamically to changing conditions, such as traffic congestion or environmental hazards. This adaptability is essential for creating smart cities that can efficiently manage resources and enhance the quality of life for their residents.
In summary, the deployment of AI algorithms in mapping not only enhances the accuracy of spatial data analysis but also empowers decision-makers with insights that were previously unattainable. As we continue to explore the capabilities of AI in GIS, the potential for innovation and improved efficiency is limitless. The future of mapping is not just about what we can see on a map; it's about understanding the story behind the data and making informed choices that shape our world.
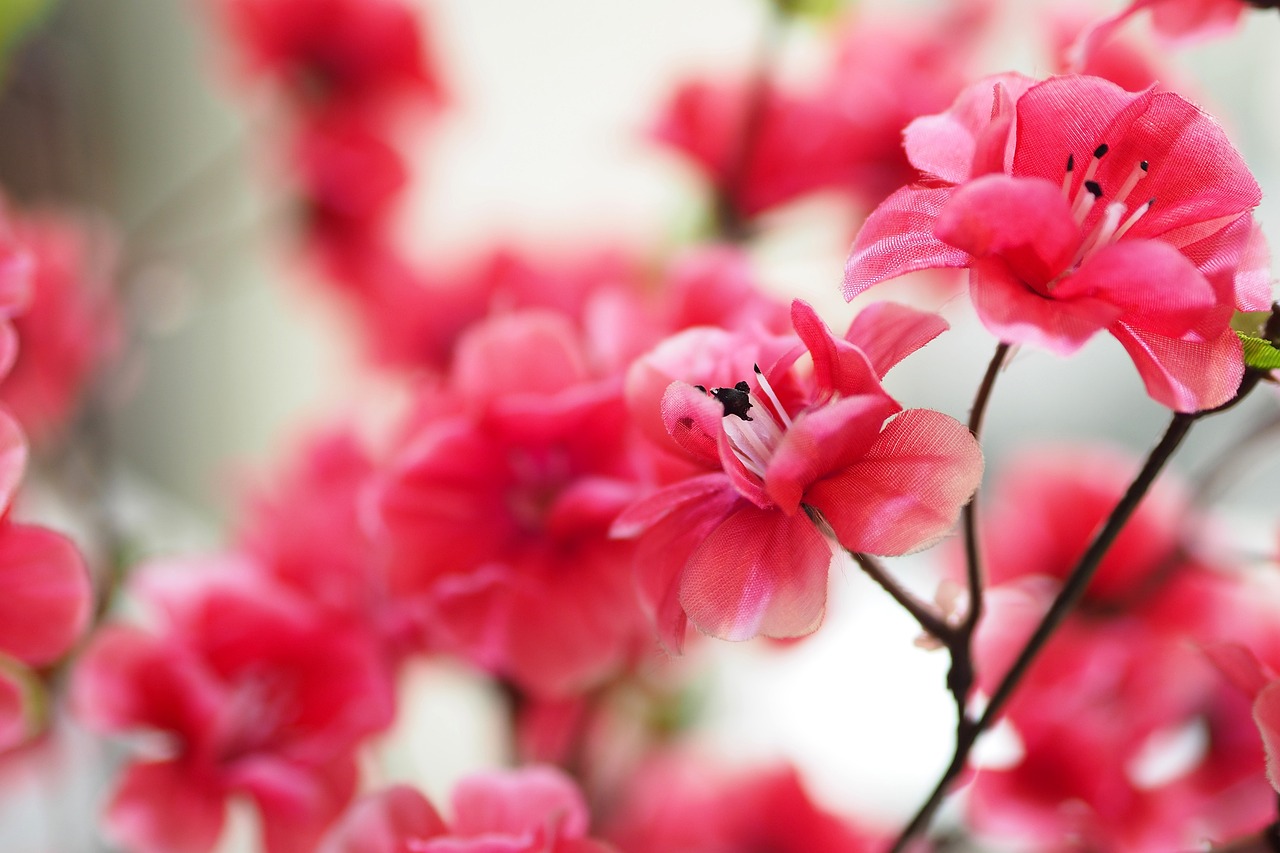
Machine Learning Applications
Machine learning is truly transforming the landscape of Geographic Information Systems (GIS). Imagine having the power to predict future trends or recognize complex patterns in vast datasets—this is precisely what machine learning brings to the table. By leveraging algorithms that can learn from data, GIS professionals can now make decisions that are not just based on historical data but also on predictive insights. This capability is a game-changer across various sectors, including urban planning, environmental monitoring, and disaster management.
One of the most significant applications of machine learning in GIS is in predictive modeling. This involves analyzing past data to forecast future events. For instance, urban planners can utilize predictive analytics to anticipate population growth in specific areas, which can inform decisions about infrastructure development. By understanding where people are likely to move, cities can allocate resources more effectively, ensuring they are prepared for future demands. The implications are profound; it’s like having a crystal ball that helps in shaping smarter, more resilient cities.
Another exciting application is pattern recognition. Machine learning algorithms excel at identifying trends and anomalies in data that might not be readily apparent to human analysts. For example, in environmental monitoring, machine learning can analyze satellite imagery to detect changes in land use or deforestation patterns. This capability not only enhances the accuracy of mapping but also provides critical insights for conservation efforts. The ability to process and analyze spatial data at an unprecedented scale allows for a more informed approach to environmental stewardship.
Furthermore, machine learning enhances resource management. By utilizing algorithms that can optimize resource allocation based on real-time data, organizations can improve efficiency and reduce waste. For instance, in agriculture, machine learning can analyze soil conditions, weather patterns, and crop health to recommend optimal planting strategies. This not only increases yield but also promotes sustainable practices, ensuring that resources are used judiciously.
To illustrate the impact of machine learning applications in GIS, consider the following table that summarizes key benefits:
Application | Benefit |
---|---|
Predictive Modeling | Anticipates future trends for better resource allocation |
Pattern Recognition | Identifies anomalies in spatial data for enhanced analysis |
Resource Management | Optimizes allocation and reduces waste in various sectors |
In conclusion, the integration of machine learning into GIS is not just a technical advancement; it is a paradigm shift. It empowers decision-makers with data-driven insights that can lead to more sustainable and efficient practices across various domains. As we continue to harness the power of machine learning, the possibilities for enhancing our understanding of spatial data are limitless. With each new development, we move closer to a future where GIS is not only a tool for mapping but a vital component in addressing the world’s most pressing challenges.
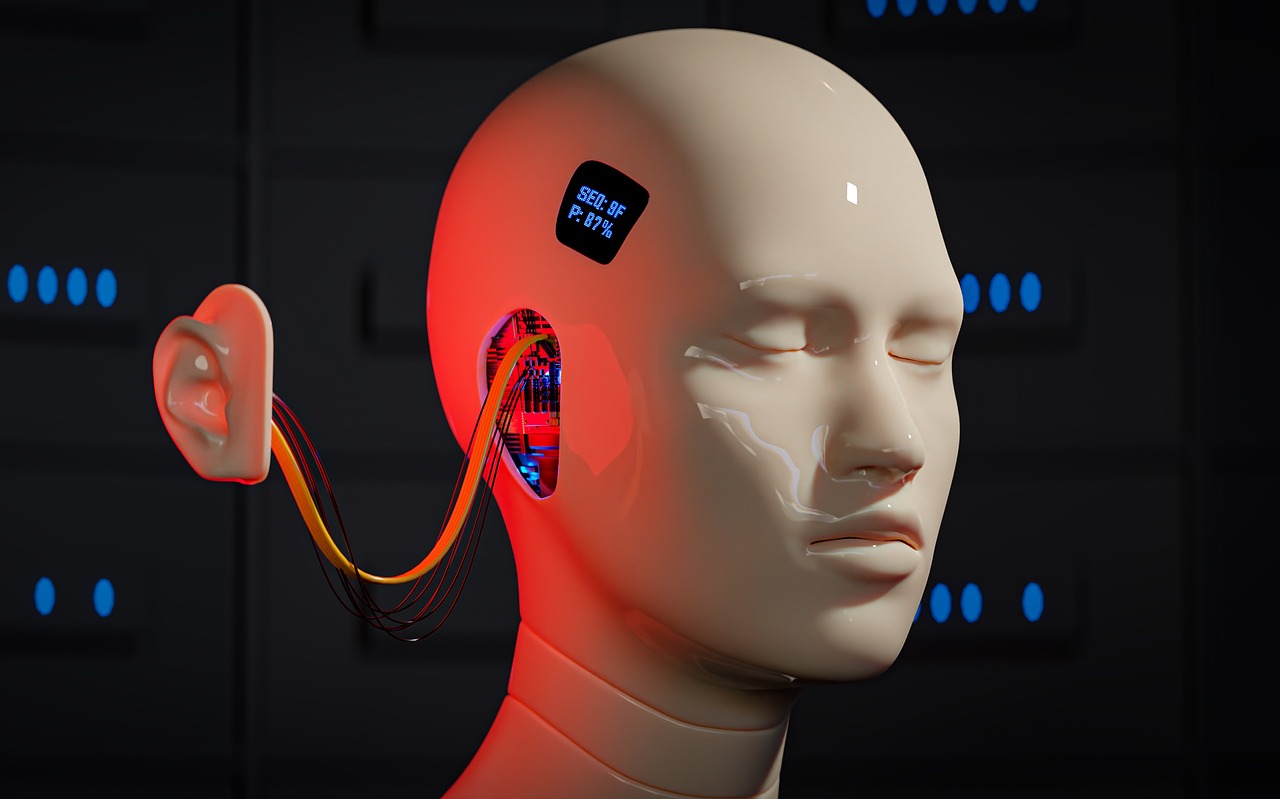
Predictive Analytics
Predictive analytics is a game-changer in the realm of Geographic Information Systems (GIS). By leveraging historical data, algorithms can identify patterns and trends that might not be immediately evident to the human eye. Imagine trying to predict the weather; you wouldn't just look at today's temperature, would you? You'd analyze past weather patterns, temperature fluctuations, and even atmospheric pressure to make an educated guess about what tomorrow might bring. Similarly, predictive analytics in GIS allows urban planners, environmentalists, and businesses to forecast future scenarios, giving them a powerful tool to make informed decisions.
For instance, consider urban development. Cities are constantly evolving, and with that comes the need for sustainable planning. Predictive analytics can help forecast population growth, traffic patterns, and even the future demand for public services. By analyzing historical data, city planners can anticipate where new schools, parks, or hospitals might be needed. This proactive approach not only saves time and resources but also enhances the quality of life for residents.
Moreover, predictive analytics plays a crucial role in disaster management. By analyzing data from previous natural disasters, such as floods or hurricanes, GIS can help predict the areas most at risk. This information is invaluable for emergency responders and city officials, allowing them to allocate resources more effectively and plan evacuation routes in advance. It's like having a crystal ball that helps communities prepare for the worst, ultimately saving lives and minimizing damage.
To illustrate the impact of predictive analytics in various sectors, consider the following table:
Sector | Application of Predictive Analytics | Benefits |
---|---|---|
Urban Planning | Forecasting population growth and infrastructure needs | Efficient resource allocation, improved quality of life |
Environmental Monitoring | Predicting environmental changes and risks | Proactive management of natural resources |
Disaster Management | Anticipating disaster-prone areas | Enhanced preparedness and response strategies |
Transportation | Analyzing traffic patterns to optimize routes | Reduced congestion and improved public transport efficiency |
In addition to these applications, predictive analytics can also enhance the effectiveness of public health initiatives. By analyzing data on disease outbreaks and population health trends, GIS can help health officials predict where interventions might be needed most. This not only helps in allocating resources efficiently but also ensures that communities receive timely support.
In conclusion, predictive analytics is not just a buzzword; it’s a powerful tool that transforms how we understand and interact with our environment. By harnessing the power of data, we can make smarter decisions that lead to better outcomes for communities, businesses, and the planet as a whole. As technology continues to evolve, the potential for predictive analytics in GIS will only expand, paving the way for a future where data-driven insights lead the charge in solving some of society's most pressing challenges.
- What is predictive analytics in GIS? Predictive analytics in GIS involves using historical data and algorithms to forecast future trends and scenarios, helping decision-makers plan effectively.
- How does predictive analytics improve urban planning? It allows urban planners to anticipate future needs for infrastructure and services, leading to more sustainable and efficient city development.
- Can predictive analytics help in disaster management? Yes, by identifying areas at risk based on historical data, it enables better preparedness and resource allocation during emergencies.
- What are some benefits of using predictive analytics? Benefits include improved decision-making, enhanced efficiency, and the ability to proactively address challenges before they escalate.
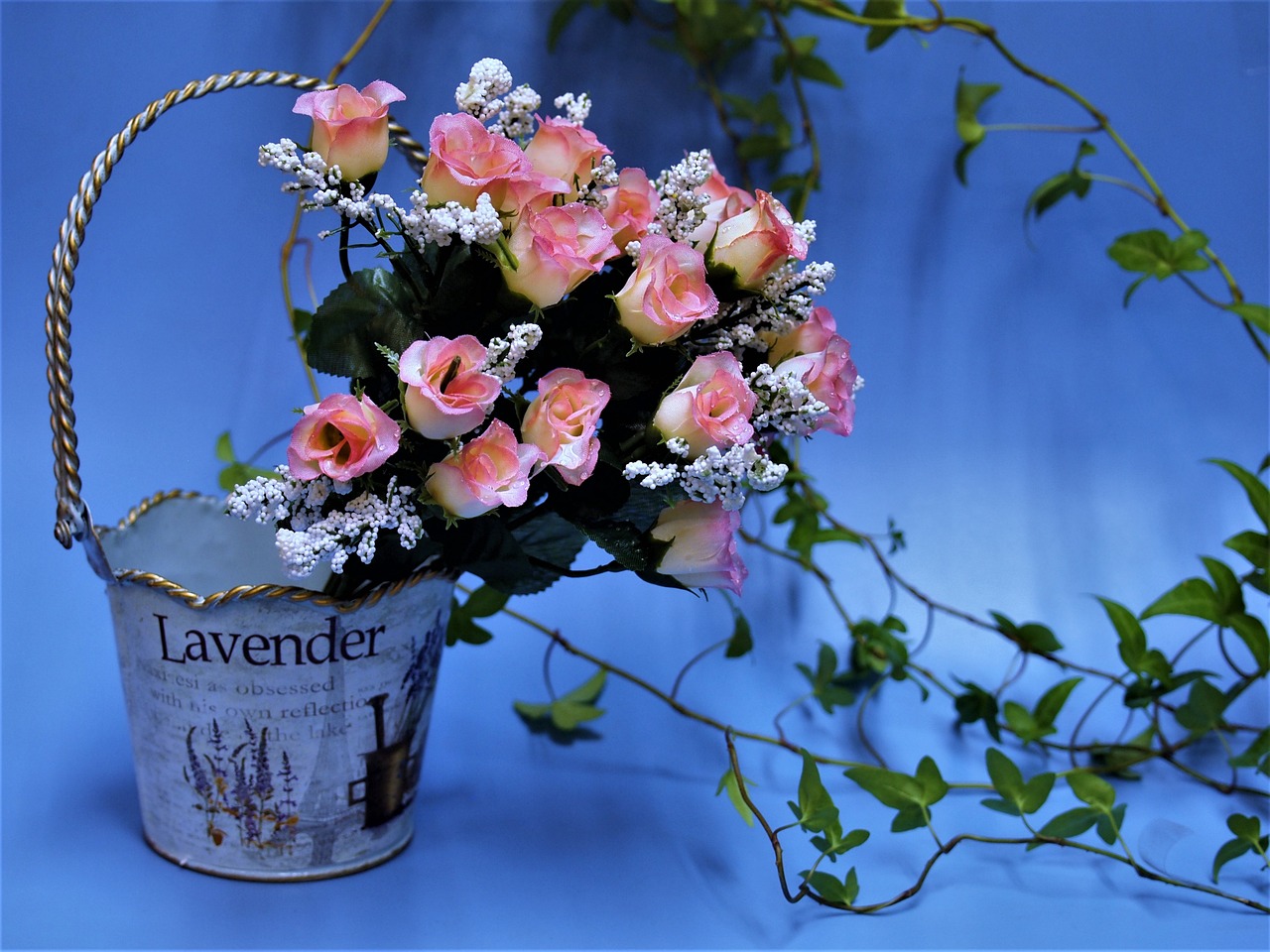
Spatial Data Classification
In the realm of Geographic Information Systems (GIS), is a crucial process that enhances our understanding of geographic phenomena. By employing advanced AI techniques, particularly those rooted in machine learning, GIS professionals can categorize vast amounts of spatial data with remarkable accuracy. This classification not only streamlines data analysis but also significantly improves the quality of insights derived from geographic datasets.
Imagine you’re trying to paint a detailed picture of a city's landscape. You need to differentiate between residential areas, commercial zones, parks, and industrial sites. This is where spatial data classification comes into play. It acts like a skilled artist, helping to segment different land uses based on various attributes and characteristics.
AI-driven classification algorithms utilize complex mathematical models to analyze spatial data, identifying patterns and relationships that might be invisible to the naked eye. For instance, a neural network can be trained to recognize the subtle differences between urban and rural land uses, or between various types of vegetation in a forested area. This process is not just about sorting data; it’s about enhancing decision-making capabilities for urban planners, environmentalists, and resource managers.
To illustrate the power of spatial data classification, consider the following table that summarizes some common classification techniques used in GIS:
Classification Technique | Description | Applications |
---|---|---|
Supervised Classification | Uses labeled training data to classify new data. | Land cover mapping, urban planning |
Unsupervised Classification | Clusters data into groups based on inherent characteristics. | Natural resource management, environmental monitoring |
Deep Learning | Employs neural networks to analyze complex datasets. | Image recognition, remote sensing |
Moreover, the integration of spatial data classification into GIS enables the creation of more sophisticated models for predicting future trends. For instance, urban planners can utilize classified data to forecast population growth patterns, allowing them to allocate resources more effectively. This predictive capability is invaluable in developing sustainable cities that can adapt to changing demographics and environmental conditions.
However, it’s essential to recognize that the accuracy of spatial data classification hinges on the quality of the input data. Poor quality or biased data can lead to inaccurate classifications, which may result in misguided decisions. Therefore, ensuring high-quality data collection and preprocessing is vital for effective spatial analysis.
In conclusion, spatial data classification powered by AI is transforming the way we analyze and interpret geographic information. By enhancing our ability to categorize and understand spatial data, we are not only improving the efficiency of GIS applications but also paving the way for more informed decision-making in various sectors.
- What is spatial data classification?
Spatial data classification is the process of categorizing geographic data into different classes or categories based on specific attributes, often using AI techniques. - Why is spatial data classification important?
It enhances the accuracy of data analysis, improves decision-making processes, and allows for better resource management in urban planning and environmental monitoring. - What role does AI play in spatial data classification?
AI algorithms, particularly machine learning and neural networks, enable more accurate classification of spatial data by identifying complex patterns and relationships.
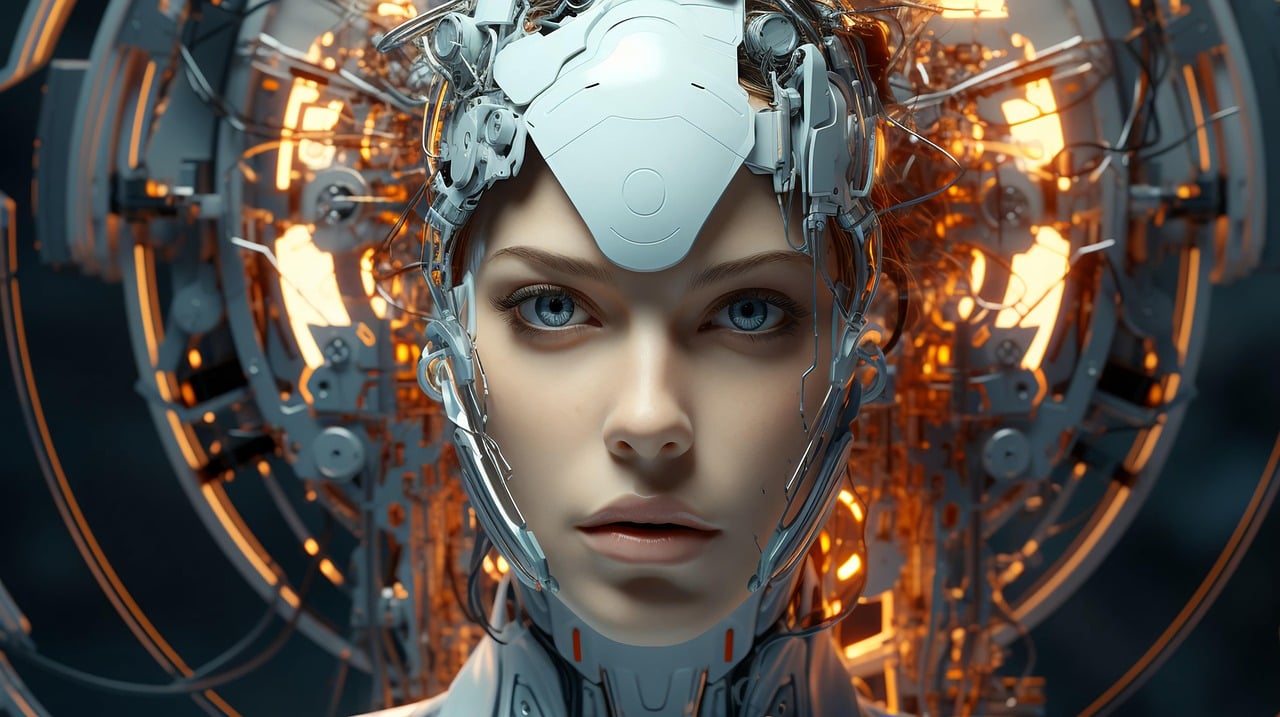
Neural Networks in GIS
Neural networks are a groundbreaking technology that has transformed the landscape of Geographic Information Systems (GIS). By mimicking the way human brains operate, these networks can analyze complex data sets with remarkable efficiency. Imagine trying to identify patterns in a massive collection of satellite images; this is where neural networks shine. They can process thousands of images in a fraction of the time it would take a human, making them invaluable for applications like remote sensing and environmental monitoring.
One of the most exciting aspects of neural networks in GIS is their ability to perform advanced image recognition. For example, when analyzing aerial photographs, neural networks can distinguish between different land uses, such as forests, urban areas, and agricultural lands. This capability enables urban planners and environmental scientists to make informed decisions based on accurate data. The implications are vast, from tracking deforestation rates to assessing urban sprawl.
Furthermore, neural networks excel at classification tasks. They can categorize geographic data into various classes, which enhances the accuracy of mapping processes. For instance, when working with multispectral satellite imagery, neural networks can identify and classify different vegetation types, soil conditions, and water bodies. This classification is crucial for effective resource management and environmental conservation.
To illustrate the impact of neural networks in GIS, consider the following table that highlights some key applications:
Application | Description | Benefits |
---|---|---|
Land Use Classification | Identifying and categorizing different land uses from satellite images. | Improved urban planning and resource allocation. |
Change Detection | Monitoring changes in land cover over time. | Early detection of environmental changes and urban expansion. |
Environmental Monitoring | Assessing ecological conditions using remote sensing data. | Enhanced conservation efforts and policy-making. |
As we delve deeper into the integration of neural networks in GIS, it becomes evident that these technologies are not just tools; they are catalysts for innovation. They enable more sophisticated analyses and empower decision-makers with the insights they need to tackle complex challenges. However, as with any technology, there are challenges to consider. The quality of the input data is crucial; if the training data for a neural network is biased or incomplete, the output will be too. This highlights the importance of using high-quality, representative data sets in GIS applications.
In conclusion, neural networks are reshaping how we approach geographic data analysis. Their ability to process and classify vast amounts of information quickly and accurately opens up new avenues for research and application in various fields. As we continue to explore the potential of AI in GIS, the future looks promising, with neural networks leading the charge in transforming our understanding of the world around us.
- What are neural networks?
Neural networks are computational models inspired by the human brain, designed to recognize patterns and make decisions based on data. - How do neural networks improve GIS?
They enhance GIS by enabling advanced data analysis, such as image recognition and classification, leading to more accurate mapping and resource management. - What are some challenges of using neural networks in GIS?
Challenges include data quality, algorithmic bias, and the need for substantial computational resources. - Can neural networks be used for real-time data analysis?
Yes, with advancements in technology, neural networks can process data in real-time, providing timely insights for decision-making.
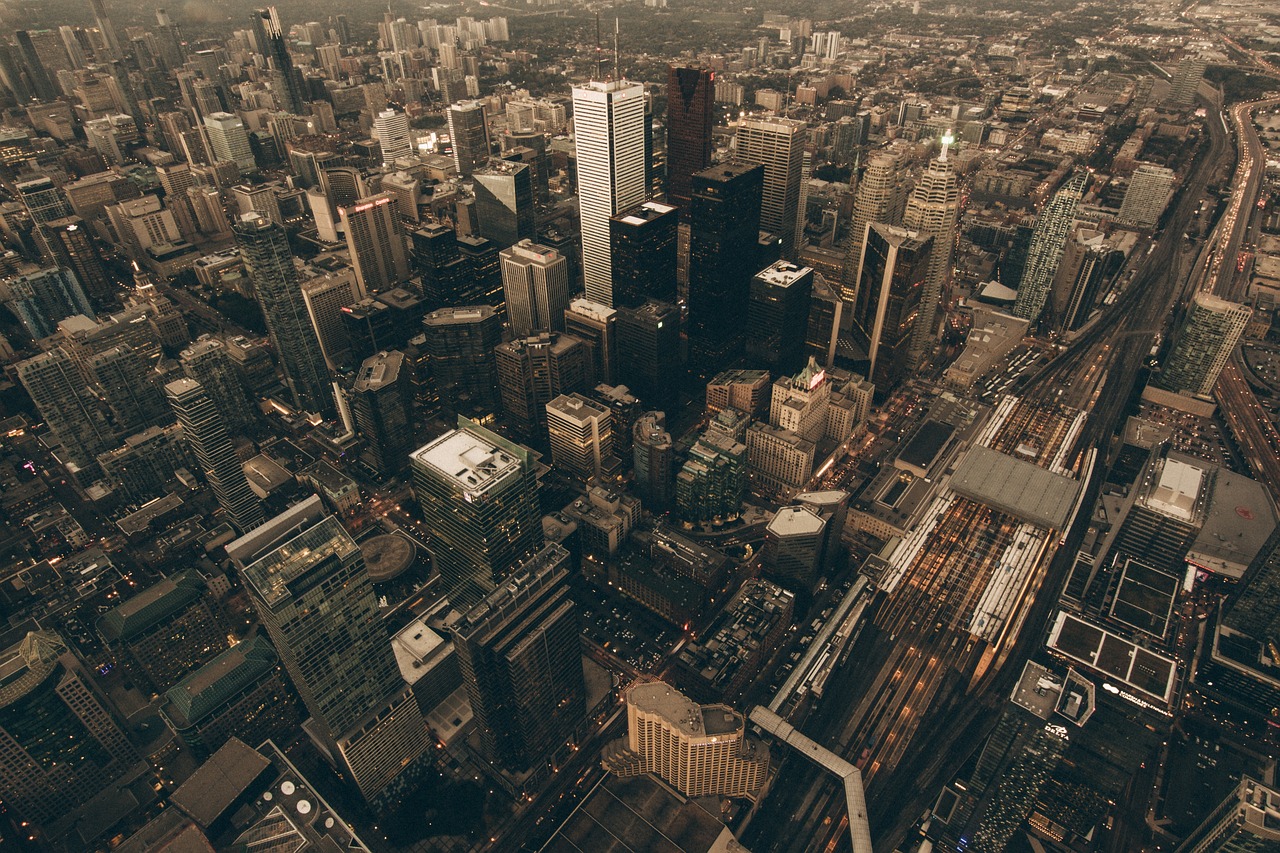
Impact on Urban Planning
Artificial Intelligence (AI) is not just a buzzword; it's a game-changer in the realm of urban planning. Imagine a city where decisions are backed by data, where resources are allocated efficiently, and where community needs are prioritized. AI's integration into Geographic Information Systems (GIS) is paving the way for such cities, transforming the way planners approach urban development. By harnessing the power of AI, urban planners can analyze vast amounts of data more effectively, leading to smarter, more sustainable urban environments.
One of the most significant impacts of AI in urban planning is its ability to provide data-driven insights. Traditional planning often relied on historical trends and human intuition, but with AI, planners can now utilize real-time data to make informed decisions. For instance, AI can analyze traffic patterns, demographic shifts, and environmental factors, allowing planners to anticipate the needs of a growing population. This predictive capability is crucial for designing infrastructure that can adapt to future demands.
Moreover, AI enhances the concept of smart cities. These are urban areas that use digital technology to improve performance, well-being, and sustainability. AI-powered GIS tools can optimize resource management in several ways:
- Transportation Systems: AI can analyze traffic data to optimize public transport routes, reducing congestion and improving commute times.
- Public Safety: By analyzing crime data, AI can help law enforcement agencies allocate resources more effectively, ensuring safer neighborhoods.
- Energy Management: Smart grids powered by AI can predict energy usage patterns, helping cities to manage resources efficiently and reduce waste.
Another vital aspect of AI's impact on urban planning is its role in community engagement. Involving residents in the planning process is crucial for creating spaces that reflect their needs and desires. AI tools can facilitate this engagement by providing platforms for feedback and participation. For example, virtual reality simulations can allow community members to visualize proposed developments and offer their input, ensuring that their voices are heard in the decision-making process.
However, while AI brings exciting possibilities, it also presents challenges. Urban planners must navigate the complexities of data privacy and ethical considerations. As cities become more interconnected, the data collected can be sensitive, raising concerns about how it's used and who has access to it. Ensuring that AI applications are transparent and equitable is essential to prevent reinforcing existing inequalities.
As we look ahead, the future of urban planning with AI at the helm is bright. The potential to create more livable, efficient, and sustainable cities is within reach, but it requires a collaborative approach that embraces innovation while addressing ethical challenges. Urban planners, technologists, and community members must work together to harness AI's full potential for the benefit of all.
Q: How does AI improve urban planning?
A: AI improves urban planning by providing data-driven insights, optimizing resource management, and enhancing community engagement through participatory processes.
Q: What are smart cities?
A: Smart cities are urban areas that leverage digital technology and data analytics to enhance performance, sustainability, and quality of life for residents.
Q: What challenges does AI pose in urban planning?
A: Some challenges include data privacy concerns, algorithmic bias, and the need for transparency in how AI-driven decisions are made.
Q: How can communities engage in the urban planning process?
A: Communities can engage through AI tools that facilitate feedback, such as virtual reality simulations and online platforms that allow residents to share their opinions on proposed developments.
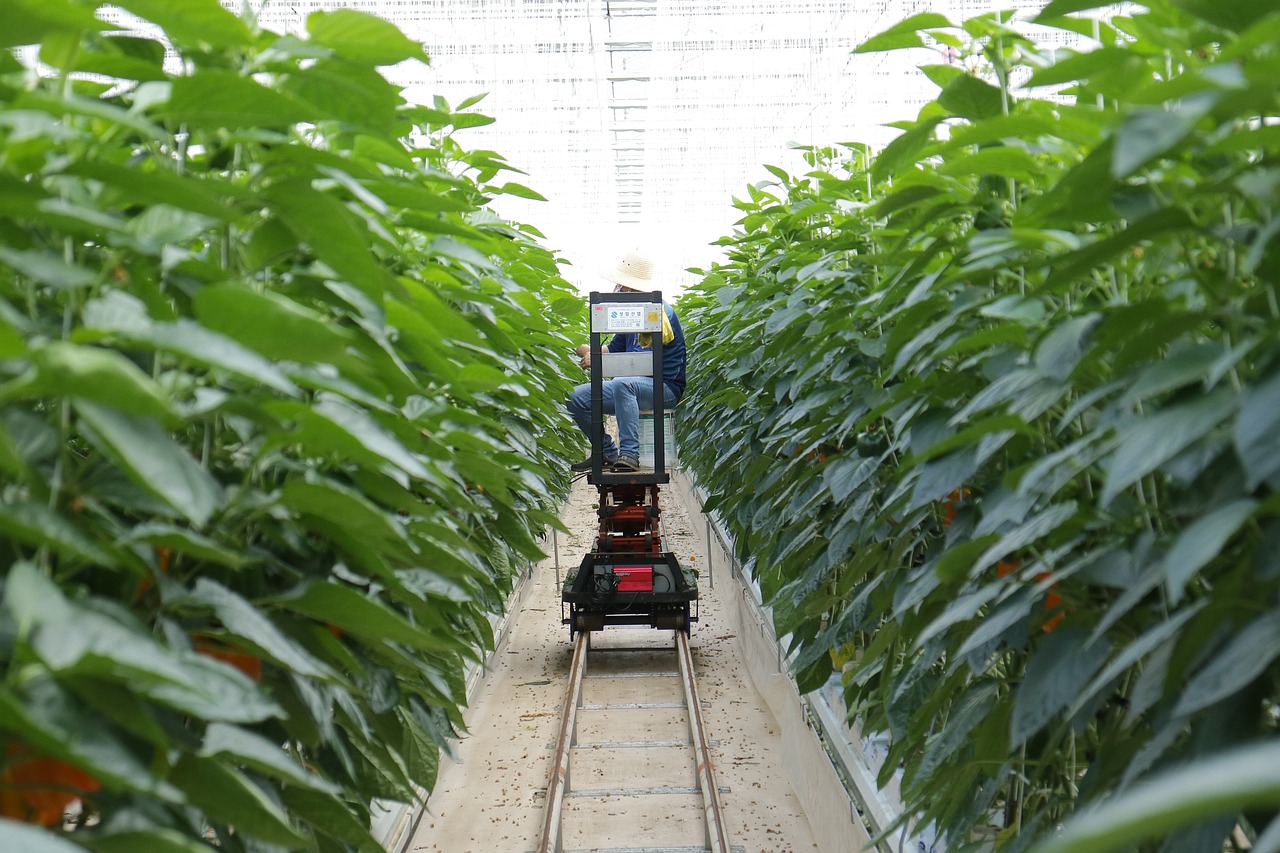
Smart City Initiatives
As cities around the globe continue to expand, the concept of smart cities is becoming increasingly vital. These urban areas leverage technology, particularly artificial intelligence (AI), to enhance the quality of life for their residents while promoting sustainable development. By integrating AI into Geographic Information Systems (GIS), smart city initiatives are transforming how urban spaces are planned and managed. Imagine a city where traffic flows seamlessly, public services are optimized, and resources are allocated efficiently—this is the promise of smart city initiatives.
One of the primary goals of smart city initiatives is to utilize data-driven insights to improve urban infrastructure. For instance, AI can analyze traffic patterns and predict congestion points, enabling city planners to make informed decisions about road design and public transportation routes. Additionally, AI can optimize energy consumption in buildings, leading to reduced costs and a smaller carbon footprint. This optimization is not just about saving money; it's about creating a more livable and environmentally friendly urban space.
Moreover, smart city initiatives often focus on enhancing public safety. With the help of AI and GIS, cities can deploy resources more effectively during emergencies. For example, real-time data can inform first responders about the most efficient routes to take, ensuring they arrive at the scene as quickly as possible. This proactive approach to urban safety not only saves lives but also builds community trust in public services.
Another critical aspect of smart city initiatives is community engagement. AI tools in GIS facilitate participatory planning processes, allowing residents to voice their opinions and contribute to urban development strategies. By incorporating feedback from the community, cities can ensure that their initiatives meet the actual needs of the people who live there. This engagement fosters a sense of ownership among residents and encourages them to be active participants in shaping their environment.
In summary, smart city initiatives represent a significant leap forward in urban planning and management. By harnessing the power of AI and GIS, cities can create more efficient, safe, and sustainable environments for their inhabitants. As we look to the future, the integration of technology in urban settings will only continue to grow, paving the way for smarter, more adaptive cities that can meet the challenges of tomorrow.
- What is a smart city?
A smart city uses technology and data to improve the quality of life for its residents, enhance public services, and promote sustainability. - How does AI contribute to smart city initiatives?
AI helps analyze data for better decision-making in areas like traffic management, resource allocation, and public safety. - What role does community engagement play in smart cities?
Community engagement ensures that urban development strategies reflect the needs and desires of residents, fostering a sense of ownership. - What are the benefits of integrating AI in urban planning?
Benefits include improved efficiency, enhanced public safety, better resource management, and a more sustainable urban environment.
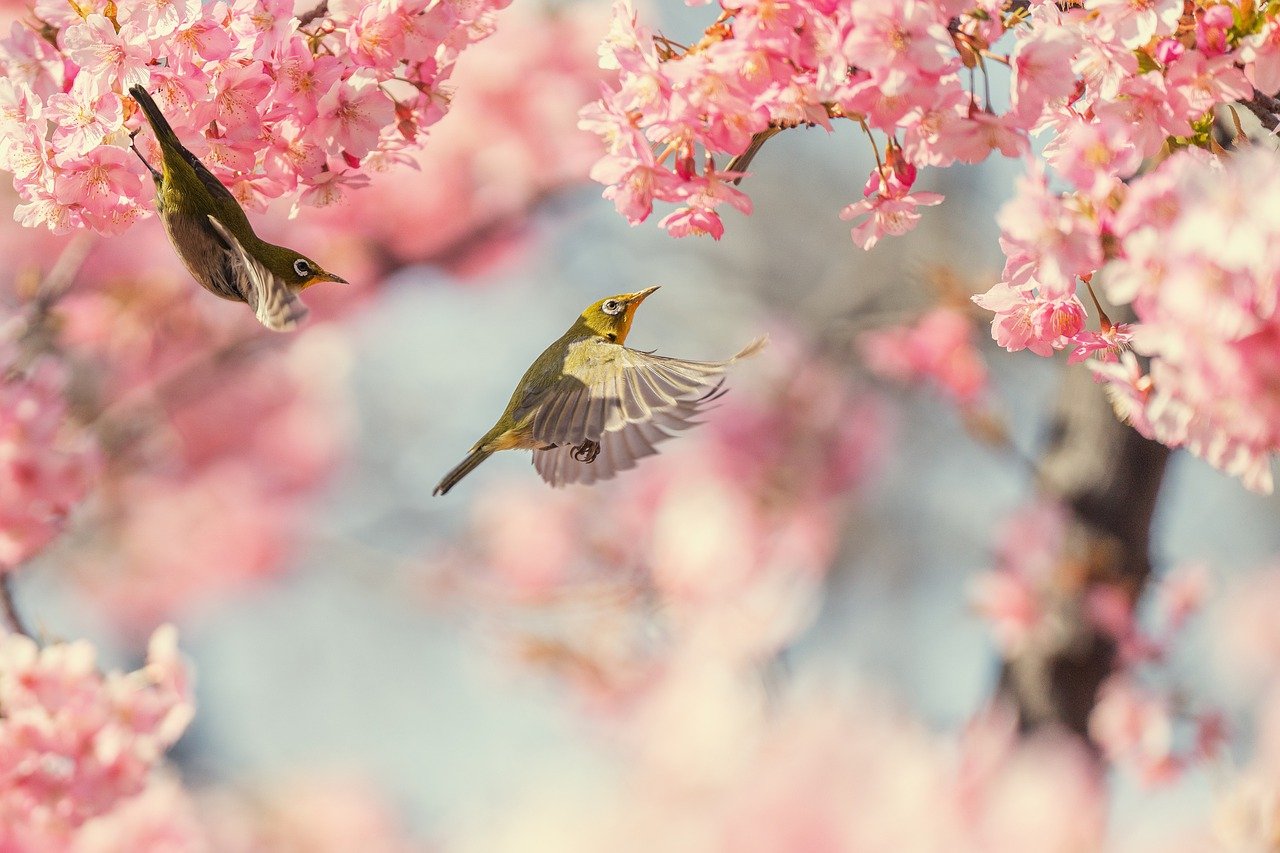
Community Engagement
In today's rapidly changing urban landscapes, has become a cornerstone of successful urban planning. With the integration of artificial intelligence in Geographic Information Systems (GIS), the way communities interact with and influence their environments is evolving dramatically. AI tools empower residents to participate actively in the planning process, ensuring their voices are not just heard but are integral to the decision-making framework.
Imagine a neighborhood where residents can visualize proposed developments right from their smartphones. With AI-enhanced GIS platforms, this is not just a dream—it's a reality. These tools allow for real-time feedback and interaction, creating a dynamic dialogue between planners and community members. This participatory planning process not only fosters a sense of ownership among residents but also leads to more informed and equitable decision-making.
Moreover, AI can analyze community sentiments through social media data, surveys, and public forums. By processing this information, planners can identify common concerns and preferences, tailoring their strategies to meet the actual needs of the community. For example, if a significant number of residents express interest in green spaces, AI can help prioritize parks and recreational areas in urban development plans. In this way, technology acts as a bridge between planners and the public, enhancing transparency and trust.
To illustrate the impact of AI on community engagement, consider the following table that outlines various AI tools and their applications:
AI Tool | Application | Benefit |
---|---|---|
Sentiment Analysis | Analyzing public opinion from social media | Identifies community concerns and preferences |
Interactive Mapping | Visualizing proposed developments | Enhances public understanding and feedback |
Predictive Modeling | Forecasting community needs | Informs resource allocation and planning |
However, while AI offers powerful tools for engagement, it is essential to ensure that these technologies are accessible to all community members. Digital divides can exacerbate existing inequalities, so planners must strive to create inclusive platforms that reach diverse populations. This may involve community workshops, training sessions, or partnerships with local organizations to ensure that everyone has a voice in shaping their environment.
In conclusion, the integration of AI in GIS not only enhances community engagement but also transforms the way urban planning is approached. By leveraging technology to foster collaboration, planners can create more livable, sustainable, and equitable communities. The future of urban development lies not just in the hands of experts but in the collective wisdom of the communities they serve.
- How does AI improve community engagement in urban planning?
AI tools enable real-time feedback and analysis of community sentiments, allowing planners to tailor their strategies based on actual resident needs. - What are some examples of AI tools used in community engagement?
Examples include sentiment analysis for public opinion, interactive mapping for visualizing developments, and predictive modeling for forecasting community needs. - How can planners ensure inclusivity in AI-driven community engagement?
Planners can conduct workshops, provide training, and partner with local organizations to reach diverse populations and ensure everyone has a voice.
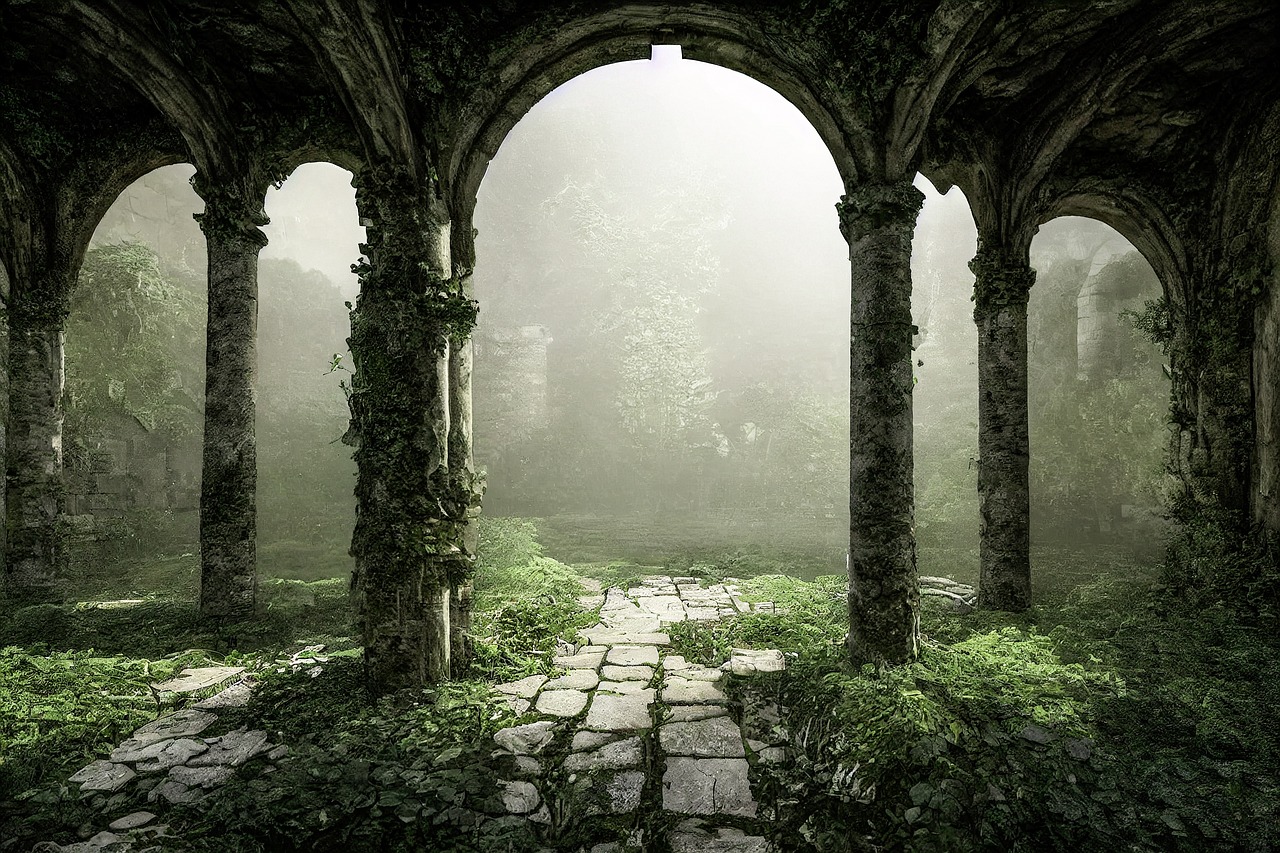
Challenges and Ethical Considerations
The integration of artificial intelligence into Geographic Information Systems (GIS) is not without its challenges and ethical dilemmas. As we embrace the power of AI to enhance our mapping and data analysis capabilities, we must also confront the complexities that come with it. One of the most pressing issues is data privacy. Geographic data is often sensitive, and the potential for misuse looms large. Imagine a scenario where personal information collected through GIS is exploited for commercial gain or surveillance. This concern necessitates stringent measures to protect individual privacy while still leveraging the benefits of AI.
Moreover, the issue of algorithmic bias cannot be overlooked. AI systems learn from historical data, and if that data reflects existing societal biases, the outcomes produced by these systems may perpetuate those biases. For example, if a GIS application used for urban planning relies on biased data, it could lead to inequitable resource distribution, ultimately harming marginalized communities. This is why addressing algorithmic bias is crucial. It’s not just about enhancing technology; it’s about ensuring fairness and equity in decision-making processes.
Another significant challenge lies in the realm of automated decision-making. As AI systems begin to take on more responsibility in public policy and urban planning, the implications of these automated decisions can be profound. Who is accountable when an AI-driven decision leads to negative outcomes? This question highlights the need for clear guidelines and frameworks governing AI use in GIS. We must ensure that human oversight remains a critical component of any automated system, especially when it comes to decisions that affect the lives of individuals and communities.
To further illustrate these challenges, consider the following table that outlines some of the key ethical issues associated with AI in GIS:
Challenge | Description |
---|---|
Data Privacy | Concerns about the misuse of sensitive geographic data and the need for protective measures. |
Algorithmic Bias | The risk of reinforcing societal inequalities through biased data and AI outcomes. |
Automated Decision-Making | The implications of AI-driven decisions and the need for accountability and human oversight. |
In conclusion, while the integration of AI into GIS offers exciting possibilities, it also brings forth a myriad of challenges that require careful consideration. It’s essential for stakeholders—governments, developers, and communities—to engage in an ongoing dialogue about these ethical implications. By doing so, we can harness the power of AI responsibly, ensuring that it serves to enhance our world rather than complicate it.
- What are the main ethical concerns regarding AI in GIS? The main concerns include data privacy, algorithmic bias, and the implications of automated decision-making.
- How can we ensure data privacy in GIS applications? Implementing strict data protection measures and transparent data usage policies can help safeguard individual privacy.
- What is algorithmic bias, and why is it important? Algorithmic bias occurs when AI systems produce skewed results due to biased training data, which can lead to unfair outcomes in urban planning and resource allocation.
- Why is human oversight necessary in AI decision-making? Human oversight is crucial to maintain accountability and ensure that AI-driven decisions align with ethical standards and community needs.
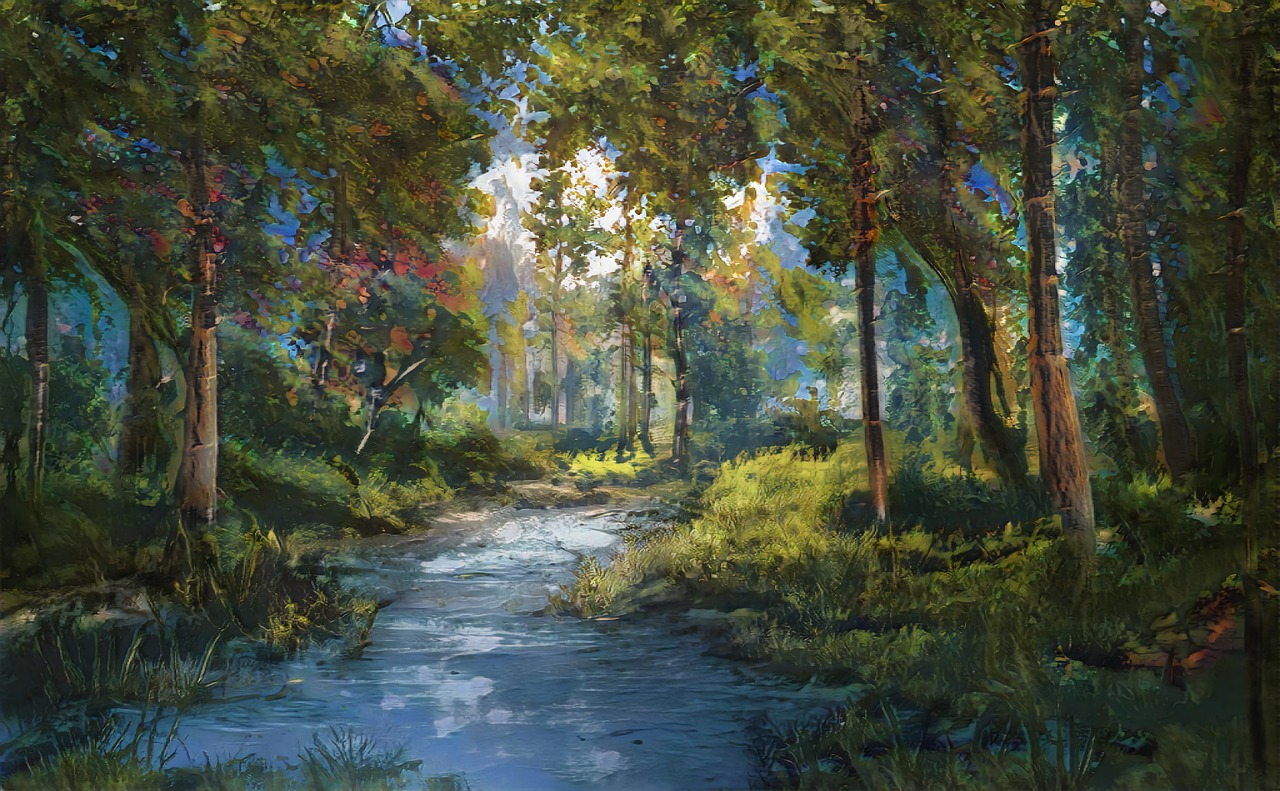
Data Privacy Concerns
As we dive deeper into the integration of artificial intelligence within Geographic Information Systems (GIS), one of the most pressing issues that arises is data privacy. With the vast amounts of geographic data being collected, analyzed, and utilized, the potential for misuse becomes a serious concern. Imagine a world where your every move is tracked and recorded; this is the reality that many fear as AI technologies advance. The data that GIS systems collect can include sensitive information about individuals, their habits, and their locations. This raises the question: how can we ensure that this data is handled responsibly?
Furthermore, as cities become smarter and more connected, the amount of data generated will only increase. This leads to a complex web of data privacy issues that must be addressed. For instance, when AI algorithms analyze geographic data, there is a risk that they could inadvertently expose personal information or lead to surveillance practices that infringe on individual privacy. It's essential to find a balance between leveraging the power of AI for societal benefits and protecting the rights of individuals.
To tackle these concerns, organizations and governments must implement robust data protection policies. Here are a few strategies that can help mitigate privacy risks:
- Data Anonymization: Before using geographic data for analysis, it should be anonymized to prevent the identification of individuals.
- Transparency: Organizations should be transparent about how data is collected, used, and shared.
- Regulatory Compliance: Adhering to data protection regulations, such as GDPR, is crucial in ensuring that privacy rights are respected.
Additionally, public awareness and education about data privacy are vital. The more people understand how their data is used and the potential risks involved, the better equipped they will be to protect themselves. As technology continues to evolve, so too must our approaches to safeguarding privacy. The future of AI in GIS holds great promise, but it must not come at the expense of individual rights.
In summary, while AI enhances the capabilities of GIS, it also brings forth significant challenges regarding data privacy. Addressing these concerns is not just a technical issue, but a societal one that requires collaboration between technologists, policymakers, and the public. Only through a concerted effort can we harness the power of AI in GIS while ensuring that data privacy remains a top priority.
- What are the main data privacy concerns related to AI in GIS?
The main concerns include the potential misuse of sensitive geographic data, risks of surveillance, and the need for robust data protection policies. - How can organizations protect individuals' data privacy?
Organizations can implement data anonymization, ensure transparency in data usage, and comply with regulatory frameworks such as GDPR. - Why is public awareness important in data privacy?
Public awareness helps individuals understand the risks associated with their data, enabling them to take proactive measures to protect their privacy.
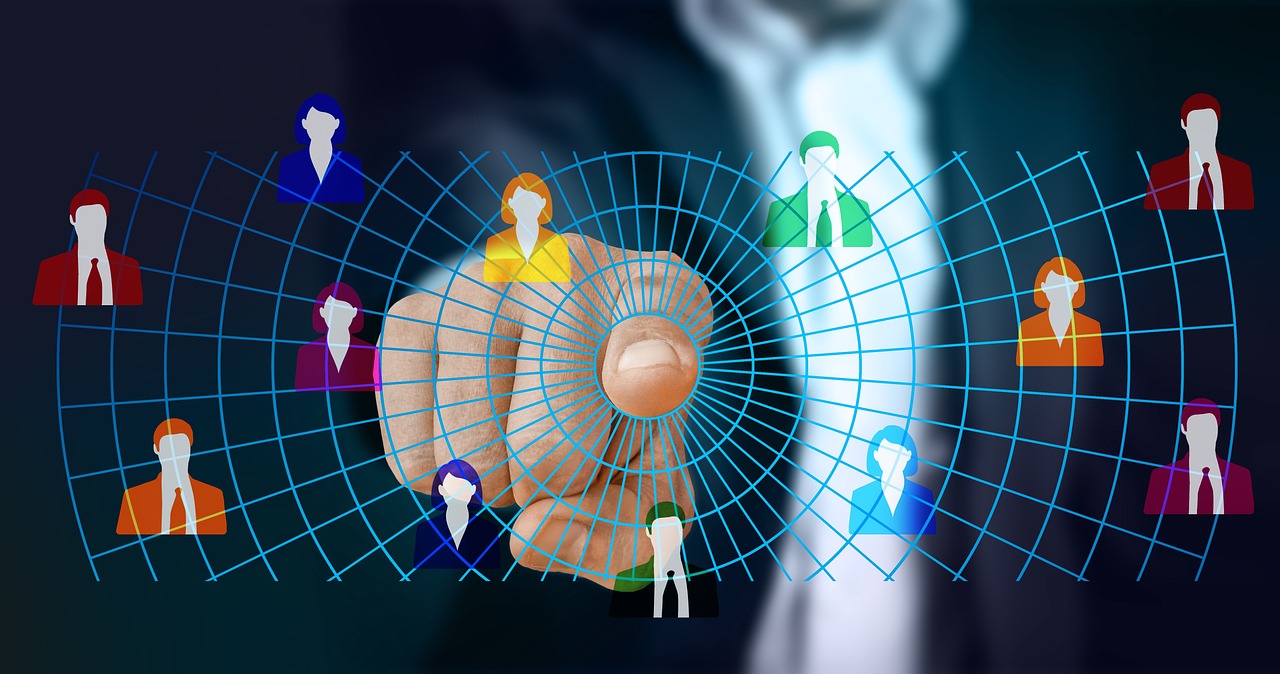
Addressing Algorithmic Bias
As we delve deeper into the integration of artificial intelligence within Geographic Information Systems (GIS), one of the most pressing issues that emerges is the concern of algorithmic bias. This bias can arise from various sources, including the data used to train AI models and the inherent assumptions made during the design of these algorithms. If not addressed, algorithmic bias can lead to skewed results that disproportionately affect marginalized communities, ultimately reinforcing existing inequalities in urban planning and resource distribution.
To tackle this issue, it is essential to adopt a multi-faceted approach that includes:
- Diverse Data Collection: Ensuring that the data used for training AI models is representative of all demographic groups is crucial. This means actively seeking out data from underrepresented communities to avoid skewed outcomes.
- Transparent Algorithms: Making the workings of AI algorithms transparent can help stakeholders understand how decisions are made. This transparency fosters accountability and allows for scrutiny that can highlight potential biases.
- Continuous Monitoring: Implementing mechanisms for ongoing evaluation of AI systems is vital. By regularly assessing the outputs of these systems, organizations can identify and rectify biases as they arise.
Moreover, it is essential to engage with community stakeholders in the development and deployment of AI-driven GIS applications. By involving those who are most affected by these technologies, we can ensure that their perspectives and needs are adequately represented. This participatory approach not only enhances the fairness of the outcomes but also builds trust between communities and decision-makers.
In addition to community engagement, fostering a culture of ethical AI development within organizations is paramount. This includes training developers and data scientists to recognize and mitigate bias in their work actively. Workshops, seminars, and collaborative projects can create awareness and facilitate the sharing of best practices in addressing algorithmic bias.
Ultimately, addressing algorithmic bias in GIS and AI is not merely a technical challenge but a societal one. It requires a commitment from all stakeholders to ensure that the benefits of these technologies are shared equitably. By taking proactive steps, we can harness the full potential of AI in GIS while safeguarding against the risks of bias and inequality.
- What is algorithmic bias?
Algorithmic bias refers to systematic and unfair discrimination that can occur when AI systems make decisions based on biased data or flawed algorithms.
- How can algorithmic bias affect GIS applications?
In GIS applications, algorithmic bias can lead to misrepresentation of certain demographics, skewed resource allocation, and unfair urban planning outcomes.
- What steps can organizations take to mitigate algorithmic bias?
Organizations can mitigate bias by ensuring diverse data collection, promoting transparency in algorithms, and engaging with affected communities throughout the development process.
- Why is community engagement important in addressing algorithmic bias?
Community engagement is crucial as it ensures that the needs and perspectives of those most affected by AI applications are considered, leading to more equitable outcomes.
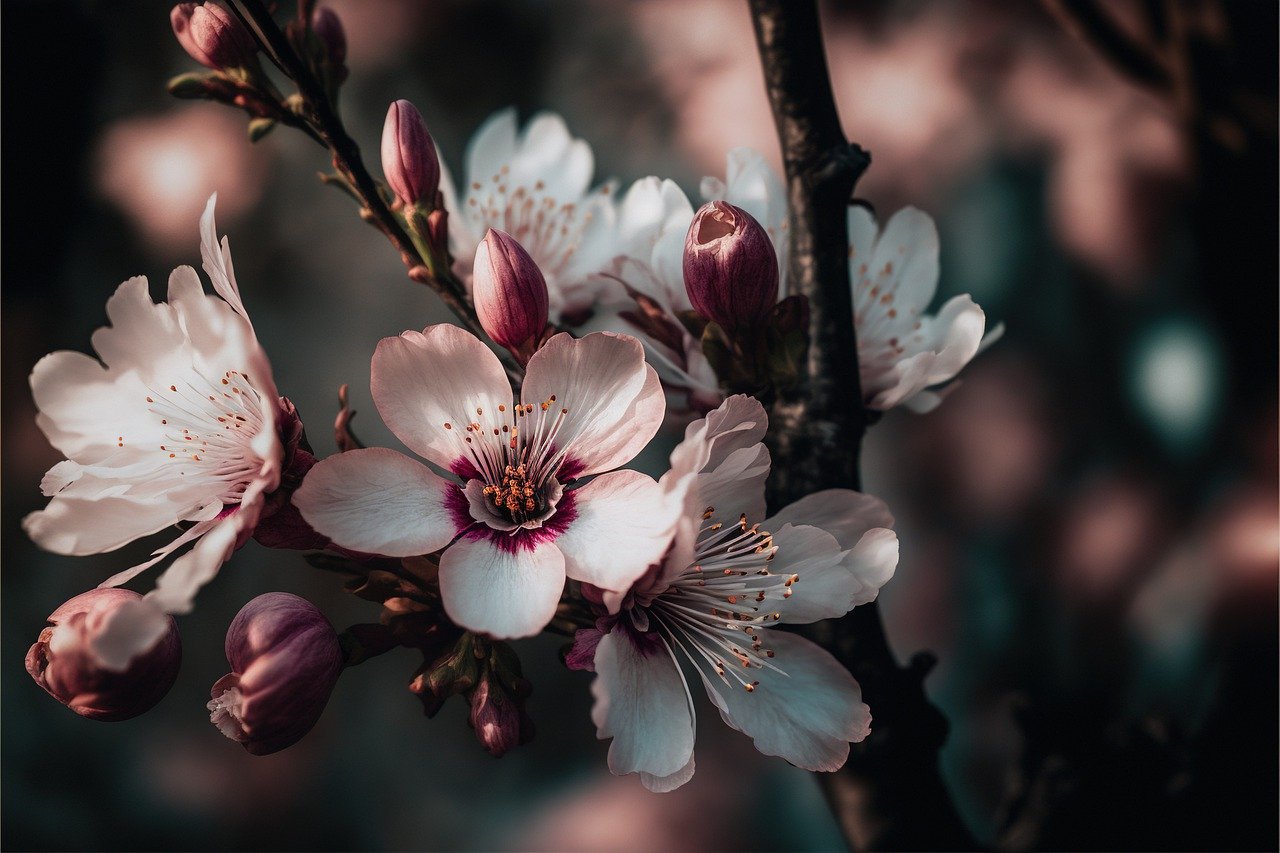
The Future of AI in GIS
As we look toward the horizon, the future of Artificial Intelligence (AI) in Geographic Information Systems (GIS) is nothing short of exhilarating. Imagine a world where mapping and data analysis are not just reactive but proactive, where decisions are informed by real-time data, and where urban landscapes are shaped by intelligent insights rather than guesswork. This is the promise of AI in GIS—transforming how we understand and interact with our environments.
One of the most compelling aspects of this future is the potential for enhanced efficiency in data processing. Traditional GIS methods can be time-consuming and labor-intensive, often requiring extensive manual input. However, with the integration of AI, we can expect automated data collection and analysis, significantly reducing the time required to generate valuable insights. For instance, AI algorithms can quickly sift through vast datasets, identifying patterns and anomalies that would take humans much longer to detect.
Moreover, the advent of real-time analytics is set to revolutionize various sectors, from urban planning to disaster response. Imagine city planners being able to visualize the impact of their decisions instantly, or emergency responders accessing up-to-the-minute information during a crisis. This level of responsiveness not only enhances operational efficiency but also improves public safety and resource allocation.
Another exciting development is the use of predictive modeling powered by AI. This technology allows us to forecast future scenarios based on historical data, enabling urban planners to make informed decisions that anticipate growth and challenges. For instance, by analyzing trends in population growth, traffic patterns, and environmental changes, planners can design cities that are not only sustainable but also adaptable to future needs.
In addition to predictive capabilities, the future of AI in GIS also promises to enhance collaborative efforts among stakeholders. With AI-driven platforms, community members, government agencies, and private organizations can share insights and data seamlessly. This collaboration fosters a more inclusive approach to urban development, ensuring that all voices are heard and considered in the planning process.
However, with great promise comes great responsibility. As we embrace the future of AI in GIS, we must also be vigilant about the ethical implications that arise. Issues such as data privacy, algorithmic bias, and the transparency of AI decision-making processes must be addressed proactively. For instance, as AI systems become more integral to public policy, ensuring that these systems operate fairly and equitably will be crucial to avoid reinforcing existing inequalities.
Ultimately, the future of AI in GIS is bright and full of potential. By leveraging advanced technologies, we can not only enhance our understanding of geographic data but also create smarter, more sustainable communities. As we continue to innovate and explore the possibilities, the question remains: how will we harness this power responsibly to shape the world of tomorrow?
- What is the role of AI in GIS? AI enhances GIS by automating data analysis, improving predictive modeling, and facilitating real-time decision-making.
- How does AI improve urban planning? AI provides data-driven insights that help urban planners anticipate future trends and make informed decisions for sustainable development.
- What are the ethical considerations of using AI in GIS? Key concerns include data privacy, algorithmic bias, and the need for transparency in AI decision-making processes.
- Can AI help in disaster management? Yes, AI can analyze real-time data to provide critical insights during emergencies, improving response times and resource allocation.
Frequently Asked Questions
- What is the role of AI in GIS?
AI plays a transformative role in Geographic Information Systems (GIS) by enhancing data analysis, improving decision-making processes, and increasing overall efficiency across various applications. With AI, GIS can process vast amounts of data more effectively, leading to better insights and outcomes.
- How has GIS evolved over the years?
The evolution of GIS has been marked by advancements in technology and an increasing demand for data analysis. Initially, GIS focused on manual mapping and data collection. However, with the introduction of digital technologies and AI, GIS has become more sophisticated, allowing for real-time data analysis and improved spatial analysis capabilities.
- What are some AI algorithms used in GIS?
Some of the key AI algorithms utilized in GIS include machine learning and neural networks. These algorithms enhance data processing capabilities, enabling predictive modeling, pattern recognition, and advanced image classification, which are crucial for applications like urban planning and environmental monitoring.
- How does machine learning improve GIS?
Machine learning improves GIS by enabling predictive analytics and spatial data classification. This allows for accurate forecasting in urban development, disaster management, and resource allocation, ultimately leading to more informed decision-making.
- What are the benefits of neural networks in GIS?
Neural networks facilitate complex data analysis in GIS, particularly in tasks like image recognition and classification. This is essential for applications such as remote sensing and environmental monitoring, where advanced analytical capabilities are required.
- What impact does AI have on urban planning?
AI significantly influences urban planning by providing data-driven insights that promote sustainable development. It helps optimize resource management, improve transportation systems, and enhance public safety, leading to smarter city designs.
- How does AI foster community engagement in urban planning?
AI tools in GIS enhance community engagement by enabling participatory planning processes. This ensures that the voices of residents are heard and integrated into urban development strategies, making planning more inclusive and effective.
- What ethical considerations arise from using AI in GIS?
Using AI in GIS raises several ethical concerns, particularly regarding data privacy and algorithmic bias. It's crucial to address these issues to ensure that the applications of AI are fair, equitable, and do not lead to the misuse of geographic data.
- How can data privacy concerns be addressed in AI-driven GIS?
Data privacy concerns can be addressed by implementing strict data governance policies, ensuring transparency in data collection and usage, and employing techniques such as data anonymization to protect individuals' information while still benefiting from AI technologies.
- What does the future hold for AI in GIS?
The future of AI in GIS promises further advancements in technology, offering new opportunities for innovation and enhanced efficiency. As AI continues to evolve, it has the potential to tackle pressing global challenges through improved mapping and spatial analysis techniques.