Avoiding Ethical Traps in AI Advances
As we stand on the brink of a technological revolution, the rise of artificial intelligence (AI) brings both incredible opportunities and daunting challenges. The landscape of AI is like a double-edged sword; it can either pave the way for groundbreaking advancements or lead us into ethical quagmires that could have lasting repercussions on society. So, how do we navigate this complex terrain without falling into ethical traps? The answer lies in a combination of awareness, responsibility, and proactive measures.
First and foremost, it’s essential to recognize that the decisions made during the development of AI systems can have profound impacts on individuals and communities. For instance, consider a self-driving car. If the algorithms guiding its decisions are flawed or biased, the consequences could be catastrophic. This isn’t just about technology; it’s about human lives. Therefore, understanding the ethical implications of AI isn't merely a theoretical exercise; it’s a pressing necessity for developers, businesses, and policymakers alike.
Moreover, the ethical challenges posed by AI are not isolated incidents; they are interconnected issues that require a holistic approach. As we delve deeper into the realm of AI, we must ask ourselves: Are we prioritizing fairness? Are we safeguarding privacy? Are we ensuring transparency in decision-making? By addressing these questions head-on, we can create AI systems that not only meet technological advancements but also align with our core human values.
One of the most effective ways to avoid ethical traps is to foster a culture of ethical responsibility within organizations. This involves not just compliance with regulations, but a genuine commitment to ethical practices. Encouraging open discussions about the ethical implications of AI can empower teams to identify potential pitfalls early in the development process. It’s like having a compass that guides you through a dense forest; it helps you stay on the right path even when the journey gets tough.
In this article, we will explore the importance of ethical considerations in AI, common dilemmas faced by developers, and existing regulatory frameworks aimed at ensuring responsible practices. By the end, you’ll not only understand the complexities of ethical AI but also be equipped with strategies to navigate these challenges effectively.
Understanding why ethical considerations are crucial in AI development helps stakeholders prioritize responsible practices that align with societal values and promote trust in technology. When we think about AI, it’s easy to get caught up in the technical aspects—algorithms, data sets, and machine learning models. However, the human element is just as important, if not more so. Ethical AI is about ensuring that the technology serves humanity, rather than the other way around.
Imagine a world where AI systems are designed with empathy and fairness at their core. How transformative would that be? It would mean that technology is not just a tool for efficiency but a partner in fostering social good. This shift in perspective can significantly alter how AI is perceived and utilized, ultimately leading to greater societal acceptance and trust.
This section delves into prevalent ethical dilemmas faced by AI developers, including bias, privacy concerns, and decision-making transparency, highlighting the need for awareness and proactive solutions. These dilemmas are not just theoretical; they manifest in real-world scenarios that affect people's lives daily. For instance, biased algorithms in hiring processes can lead to discrimination against qualified candidates based on race or gender. This is not just an ethical issue; it’s a social justice issue that demands immediate attention.
Bias in AI algorithms can lead to unfair outcomes, which is why addressing this issue is paramount. This subsection examines how biases are introduced and the impact they have on marginalized communities, emphasizing the importance of fairness in AI systems. Bias can creep in at various stages of AI development, often stemming from biased data sets or flawed assumptions made during model training.
Recognizing the various sources of bias, including data selection and model training, is essential for developing fair AI solutions that do not perpetuate existing inequalities. For example, if an AI system is trained on historical data that reflects societal biases, it will likely reproduce those biases in its outputs. This is akin to teaching a child based on outdated textbooks; the knowledge imparted will be skewed and incomplete.
Strategies for mitigating algorithmic bias include diverse data sets, regular audits, and inclusive design practices, ensuring AI systems are equitable and just for all users. By actively seeking out diverse perspectives and data sources, developers can create more robust and fair AI systems. It’s about building a foundation that reflects the diversity of the world we live in.
Privacy concerns are paramount in AI development. This subsection discusses the ethical implications of data collection and usage, advocating for robust data protection measures to safeguard individuals' rights. In an age where data is often referred to as the new oil, it’s crucial to handle it with care. Just as we wouldn’t spill oil on the ground, we must ensure that personal data is treated with respect and integrity.
- What are the main ethical concerns in AI? The primary concerns include bias, privacy, transparency, and accountability.
- How can organizations ensure ethical AI practices? By adopting diverse data sets, conducting regular audits, and fostering a culture of ethical responsibility.
- Why is transparency important in AI? Transparency builds trust and allows stakeholders to understand how decisions are made, ensuring accountability.
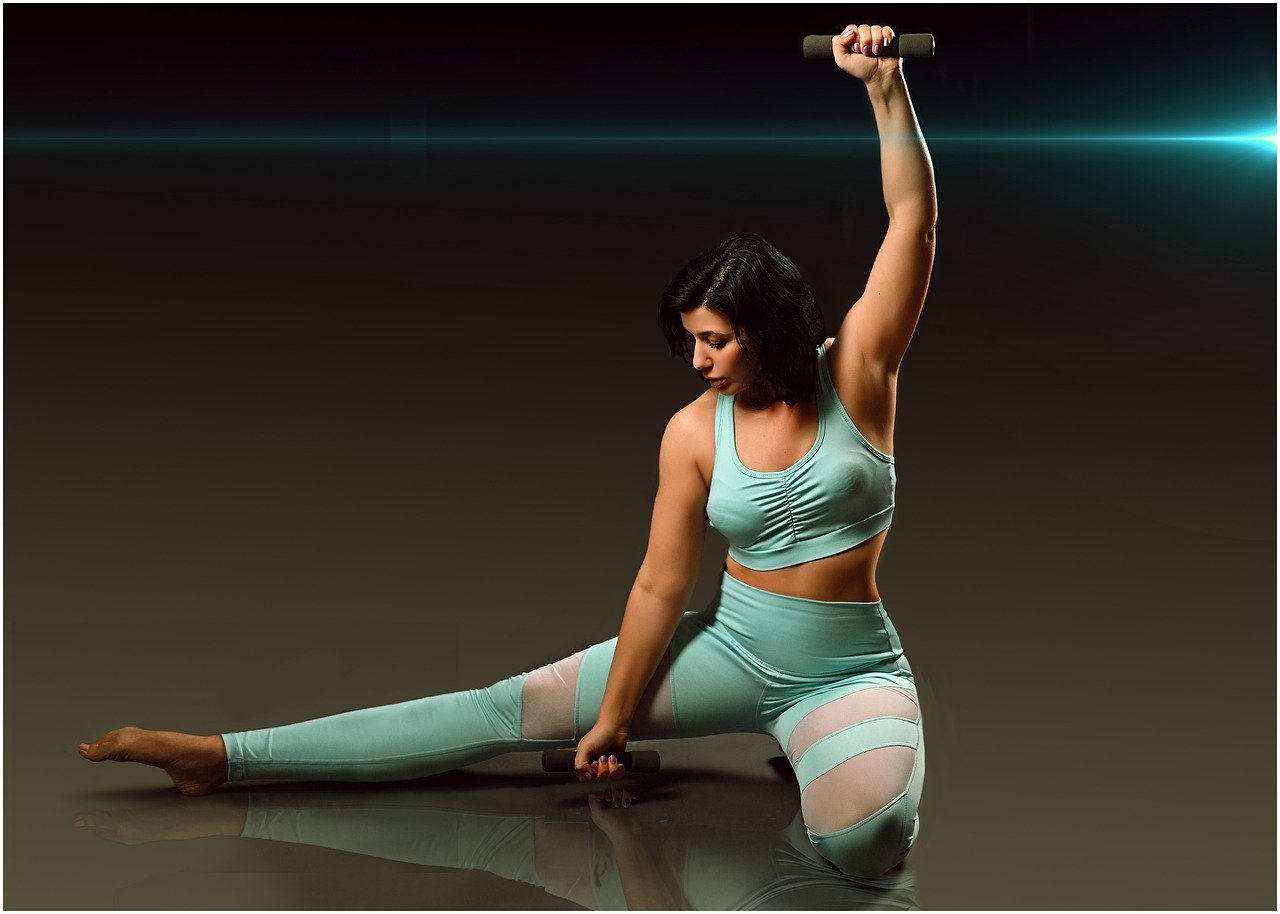
The Importance of Ethical AI
As we venture further into the realm of artificial intelligence, the need for ethical considerations becomes more critical than ever. Why, you ask? Well, think of AI as a powerful tool—much like a double-edged sword. On one side, it has the potential to revolutionize industries, enhance productivity, and improve our daily lives. On the flip side, if not handled responsibly, it can lead to unintended consequences that may harm individuals and society at large. This is where the importance of ethical AI comes into play.
Understanding the ethical implications of AI is not just a matter of compliance; it's about fostering trust and ensuring that technology aligns with our core values as a society. When stakeholders prioritize ethical practices in AI development, they create systems that are not only effective but also responsible and transparent. This alignment is crucial because it helps to build confidence among users and mitigates the risks associated with misuse of technology.
Moreover, ethical AI practices can lead to better decision-making processes. When developers consider ethical guidelines, they are more likely to create algorithms that are fair and just. This is especially important in applications like hiring, lending, and law enforcement, where biased algorithms can lead to significant inequalities. By prioritizing ethics, we can steer AI development towards outcomes that benefit everyone, not just a select few.
To illustrate the importance of ethical AI, consider the following points:
- Accountability: Ethical AI practices ensure that developers and organizations are held accountable for their creations, fostering a culture of responsibility.
- Public Trust: When AI systems operate transparently and fairly, they gain public trust, which is essential for widespread adoption.
- Long-term Sustainability: Ethical considerations lead to sustainable practices that can adapt to future technological advancements without compromising societal values.
In conclusion, the importance of ethical AI cannot be overstated. As we continue to innovate and integrate AI into various aspects of life, we must remember that our choices today will shape the future. By embedding ethical considerations into the very fabric of AI development, we can ensure that this powerful technology serves humanity in a way that is equitable and beneficial for all.
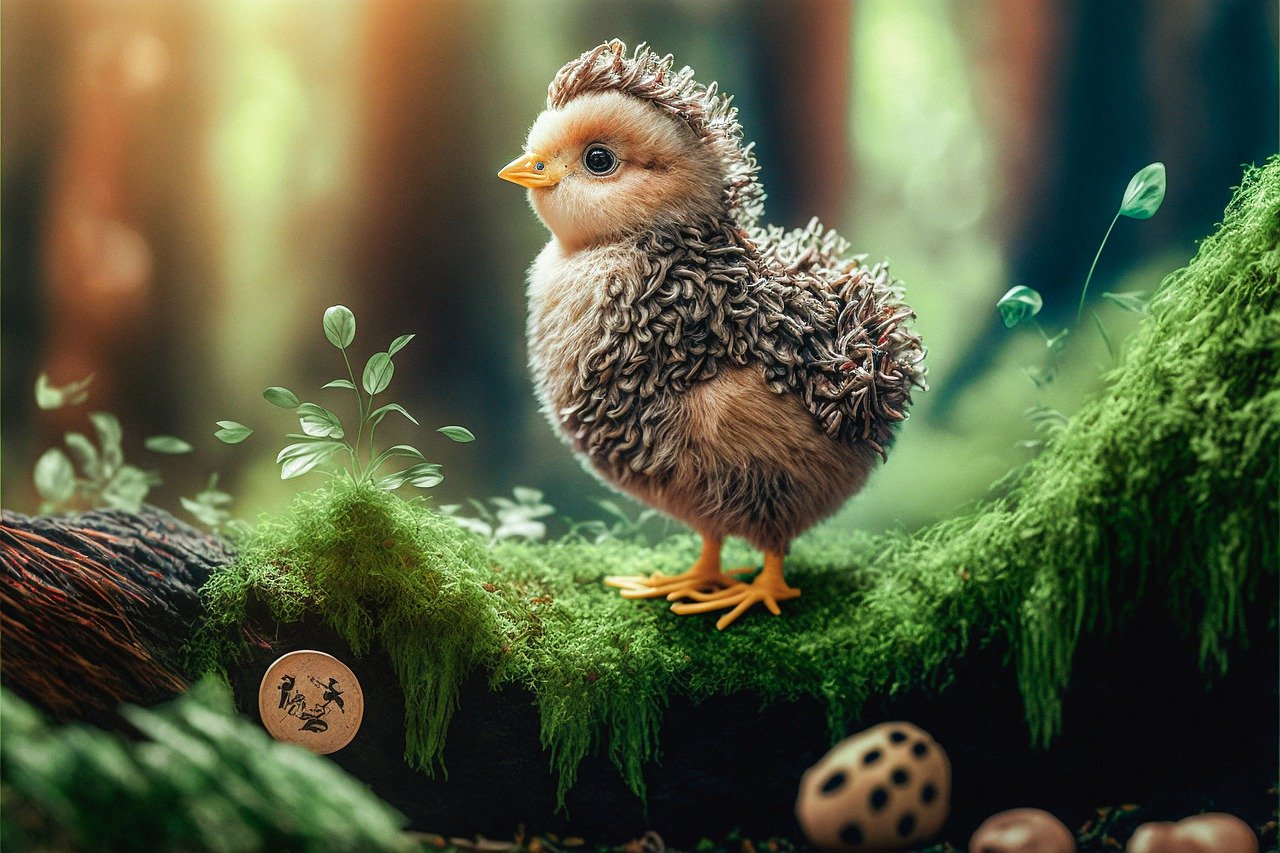
As artificial intelligence continues to evolve at a breakneck pace, it brings with it a plethora of ethical dilemmas that developers and stakeholders must navigate. These dilemmas are not just abstract concepts; they directly impact individuals and communities. One of the most pressing issues is the presence of bias in AI algorithms, which can lead to unfair treatment of certain groups. Imagine a world where a machine learning model is trained on biased data, leading to decisions that reinforce existing inequalities. This is not just a theoretical concern; it’s a reality that we must address.
Another significant ethical challenge is privacy and data protection. With AI systems often relying on vast amounts of personal data, the question arises: how do we ensure that individuals' rights are respected? The potential for misuse of data is alarming, and without robust protections, individuals may find their personal information exploited. As we delve deeper into these dilemmas, it's essential to recognize the intricate balance between innovation and ethical responsibility.
Moreover, the transparency of AI decision-making processes poses another ethical hurdle. Many AI systems operate as "black boxes," where the rationale behind their decisions is obscured. This lack of transparency can erode trust and make it difficult for users to understand how and why certain decisions are made. For instance, consider an AI system used in hiring processes—if candidates are evaluated without clear criteria, it can lead to questions about fairness and accountability.
To further illustrate these dilemmas, let's take a closer look at some of the most common ethical challenges faced by AI developers:
Ethical Dilemma | Description | Impact |
---|---|---|
Bias in Algorithms | Unintentional biases in training data leading to unfair outcomes. | Marginalized communities face discrimination. |
Privacy Concerns | Collection and usage of personal data without consent. | Potential misuse of sensitive information. |
Lack of Transparency | Opaque decision-making processes in AI systems. | Decreased trust and accountability. |
These ethical dilemmas highlight the urgent need for developers and organizations to adopt a proactive stance. By understanding and addressing these issues, we can work towards creating AI systems that are not only effective but also ethical. As we move forward, it’s crucial for all stakeholders to engage in open discussions about these challenges, ensuring that the development of AI aligns with our shared values and societal norms.
- What is bias in AI? Bias in AI refers to the tendency of algorithms to produce unfair outcomes due to prejudiced training data.
- Why is privacy a concern in AI? AI systems often require large amounts of personal data, raising concerns about how this data is collected, used, and protected.
- How can transparency be improved in AI? By implementing clear guidelines and frameworks that require AI systems to provide understandable explanations for their decisions.
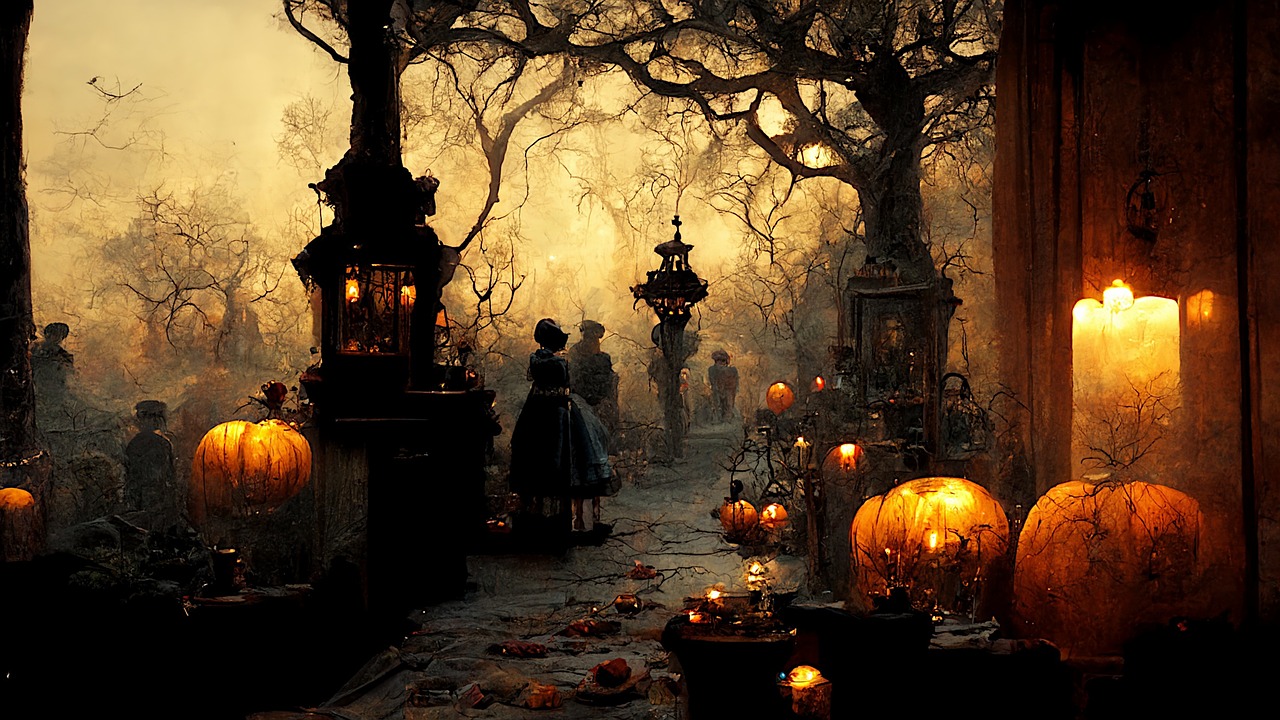
Bias in AI Algorithms
Bias in AI algorithms is a pressing concern that can lead to unfair outcomes across various sectors, from hiring practices to law enforcement. Imagine a world where a machine decides your job prospects or your legal fate based on flawed data—sounds scary, right? This is the reality we face if we don’t address the biases inherent in AI systems. Bias can creep in through various channels, often unnoticed, and its impact can disproportionately affect marginalized communities, perpetuating existing inequalities and injustices.
To truly understand how bias infiltrates AI, we must first look at the data used to train these systems. If the training data reflects societal biases—whether related to race, gender, or socioeconomic status—the AI is likely to learn and replicate those biases. For example, if an AI system is trained on historical hiring data that favors male candidates over female candidates, it may continue to favor men, regardless of the actual qualifications of the applicants. This not only undermines the principles of fairness but also erodes public trust in technology.
Recognizing the sources of bias is crucial for developing fair AI solutions. Bias can stem from:
- Data Selection: Choosing data sets that lack diversity can lead to skewed outcomes.
- Model Training: The algorithms may learn from biased patterns present in the training data.
- Human Oversight: Developers' own biases can unintentionally influence the AI's design and functionality.
Mitigating algorithmic bias is not just a technical challenge; it’s a moral imperative. Here are some strategies that can help:
- Diverse Data Sets: Incorporating a wide range of data sources can help ensure that the AI learns from a more balanced perspective.
- Regular Audits: Conducting frequent assessments of AI systems can help identify and correct biases before they cause harm.
- Inclusive Design Practices: Engaging diverse teams in the development process can lead to more equitable AI outcomes.
Ultimately, addressing bias in AI algorithms is about more than just improving technology; it’s about creating a future where technology serves everyone fairly. As we continue to integrate AI into our daily lives, we must remain vigilant and proactive in ensuring that these systems promote equity rather than perpetuate discrimination.
Q: What is bias in AI algorithms?
A: Bias in AI algorithms refers to systematic errors that lead to unfair outcomes, often influenced by the data used to train the systems.
Q: How can bias in AI affect marginalized communities?
A: Bias can result in discriminatory practices, such as unfair hiring decisions or biased law enforcement actions, adversely impacting marginalized groups.
Q: What are some strategies to mitigate bias in AI?
A: Strategies include using diverse data sets, conducting regular audits, and implementing inclusive design practices.
Q: Why is it important to address bias in AI?
A: Addressing bias is essential to ensure fairness, build public trust, and create equitable outcomes for all individuals affected by AI technologies.
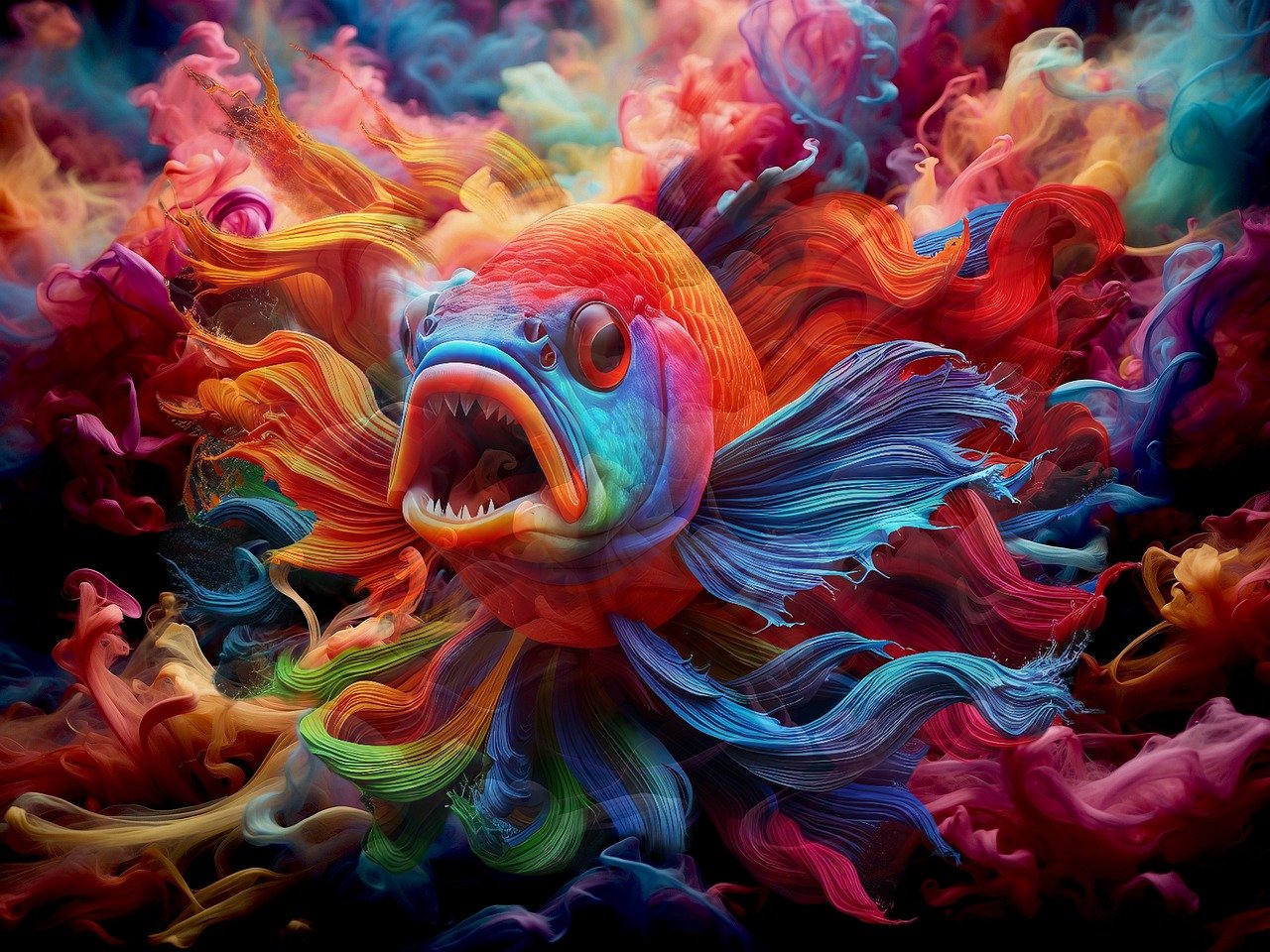
Identifying Sources of Bias
When we talk about bias in AI, it’s crucial to first understand where these biases originate. Bias can creep into AI systems through various channels, often unnoticed until it manifests in harmful ways. One of the primary sources of bias is the data itself. If the data used to train an AI model reflects existing societal prejudices, the model is likely to inherit and even amplify those biases. For instance, if a facial recognition system is trained predominantly on images of individuals from one demographic, it may struggle to accurately identify people from other backgrounds, leading to significant disparities in accuracy.
Moreover, the selection of training data plays a pivotal role. If developers unintentionally curate datasets that lack diversity, the AI will not be equipped to understand or respond appropriately to a broader range of scenarios. This is akin to teaching a child with only a handful of books; their worldview will be limited, and they may form skewed perceptions of reality. Additionally, the algorithms themselves can introduce bias during the model training process. If the mathematical functions used to process the data favor certain outcomes over others, it can lead to skewed results that reinforce stereotypes.
Another significant factor is the human element. The biases of the developers and the stakeholders involved in creating AI systems can inadvertently seep into the technology. For instance, if a team lacks diversity, they may overlook crucial perspectives that could highlight potential biases in their work. This is why fostering a diverse team is not just a moral imperative but also a practical necessity in the development of fair AI systems.
To truly combat bias, organizations must adopt a proactive approach by implementing regular audits of their AI systems. This means examining the data, algorithms, and outcomes to identify any signs of bias. By doing so, they can make informed adjustments to ensure fairness. Here’s a brief overview of some common sources of bias:
- Data Selection: The choice of data used for training can lead to skewed results.
- Model Training: Algorithms may favor certain outcomes based on how they are structured.
- Human Bias: The personal biases of developers can influence AI design and function.
Ultimately, recognizing these sources of bias is the first step toward creating AI that is not only effective but also equitable. It’s about building technology that reflects the diversity of the world we live in and serves everyone equally, rather than perpetuating existing inequalities. By being vigilant and proactive, we can pave the way for a future where AI is a tool for fairness and justice.
- What is algorithmic bias? Algorithmic bias refers to systematic and unfair discrimination in the outputs of AI systems, often stemming from biased training data or flawed algorithms.
- How can organizations mitigate bias in AI? Organizations can mitigate bias by using diverse datasets, conducting regular audits, and involving diverse teams in the development process.
- Why is diversity important in AI development? Diversity in AI development teams helps ensure a wider range of perspectives, reducing the likelihood of overlooking potential biases in the technology.
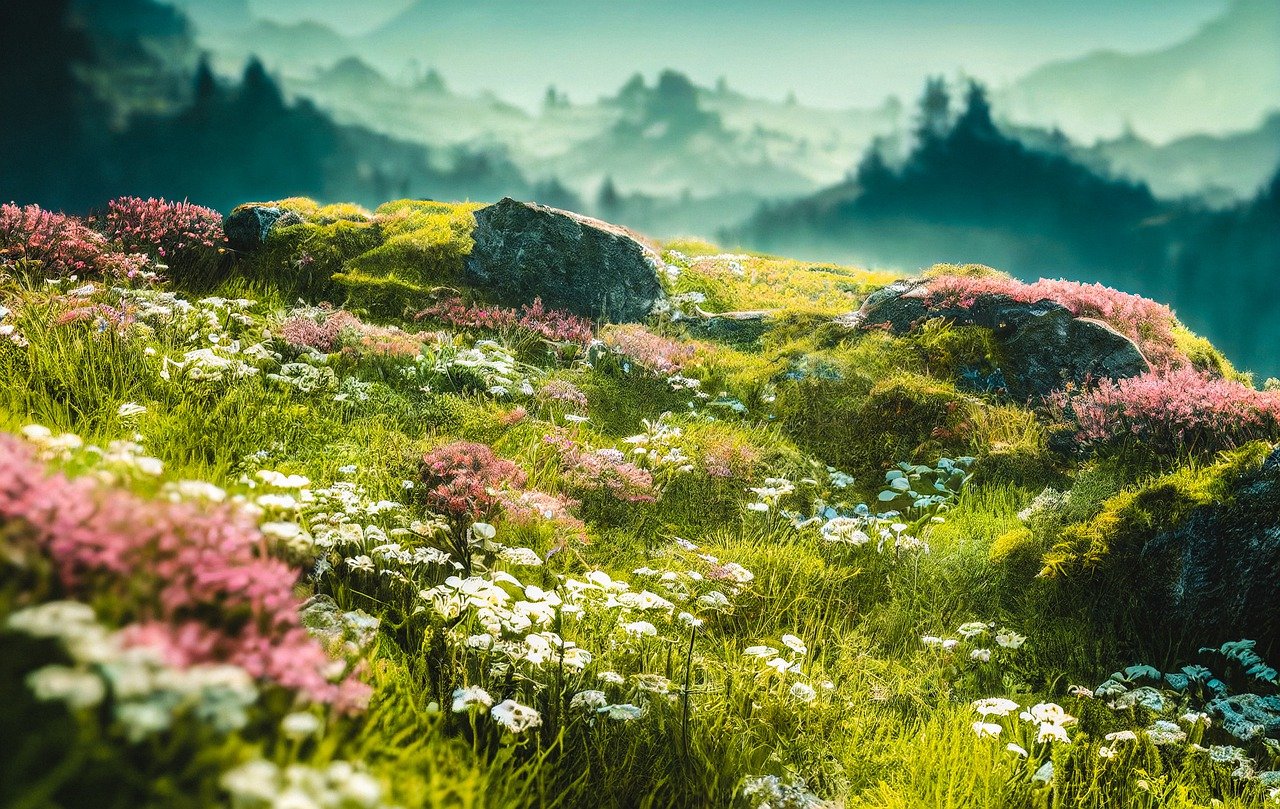
Mitigating Algorithmic Bias
In the realm of artificial intelligence, the presence of algorithmic bias can lead to significant social injustices, impacting the lives of many individuals, particularly those from marginalized communities. To combat this pressing issue, it is crucial to adopt a multi-faceted approach that not only addresses the root causes of bias but also promotes fairness and equity in AI systems. So, how can we effectively mitigate algorithmic bias and ensure that our AI technologies serve everyone equally?
One of the most effective strategies for reducing bias is the use of diverse data sets. When training AI models, it’s essential to include data that represents a wide range of demographics, experiences, and perspectives. This diversity helps to ensure that the algorithms do not favor one group over another, thereby minimizing the risk of biased outcomes. For instance, if an AI system is developed primarily using data from one demographic, it may not perform well or fairly for individuals outside that group. Therefore, actively seeking out and incorporating varied data sources is a foundational step in creating equitable AI systems.
Another critical aspect is conducting regular audits of AI algorithms. Just like a financial audit ensures that a company's books are in order, algorithmic audits help identify and rectify biases that may have crept into AI systems over time. These audits can involve testing the AI against various scenarios and demographic groups to observe its performance and make necessary adjustments. By establishing a routine for these audits, organizations can proactively address biases before they manifest in real-world applications.
Moreover, adopting inclusive design practices during the development phase can significantly reduce the likelihood of bias. This involves engaging a diverse group of stakeholders, including individuals from different backgrounds, to contribute to the design and development of AI systems. By incorporating a variety of viewpoints, developers can better anticipate potential biases and create more robust solutions. This collaborative approach not only enhances the quality of the AI systems but also fosters a sense of ownership and accountability among all participants.
Lastly, it’s important to cultivate an organizational culture that prioritizes ethical responsibility in AI development. This means training developers and stakeholders to recognize the implications of bias and equipping them with the tools and knowledge to address these issues effectively. Organizations should encourage open discussions about ethical concerns and provide resources for continuous learning about bias mitigation strategies. By embedding ethics into the core values of the organization, we can create a sustainable framework for responsible AI development.
In conclusion, mitigating algorithmic bias is not just a technical challenge; it’s a moral imperative. By employing diverse data sets, conducting regular audits, adopting inclusive design practices, and fostering a culture of ethical responsibility, we can pave the way for a future where AI technologies are fair, transparent, and beneficial for all.
- What is algorithmic bias? Algorithmic bias refers to systematic and unfair discrimination in AI algorithms, which can lead to unequal treatment of different demographic groups.
- How can diverse data sets help mitigate bias? Diverse data sets ensure that AI systems learn from a wide range of experiences and perspectives, reducing the likelihood of favoring one group over another.
- Why are regular audits important? Regular audits help identify and address biases in AI systems, ensuring that they remain fair and equitable over time.
- What role does organizational culture play in bias mitigation? A culture that prioritizes ethical responsibility encourages awareness and proactive measures to address bias, leading to more equitable AI outcomes.
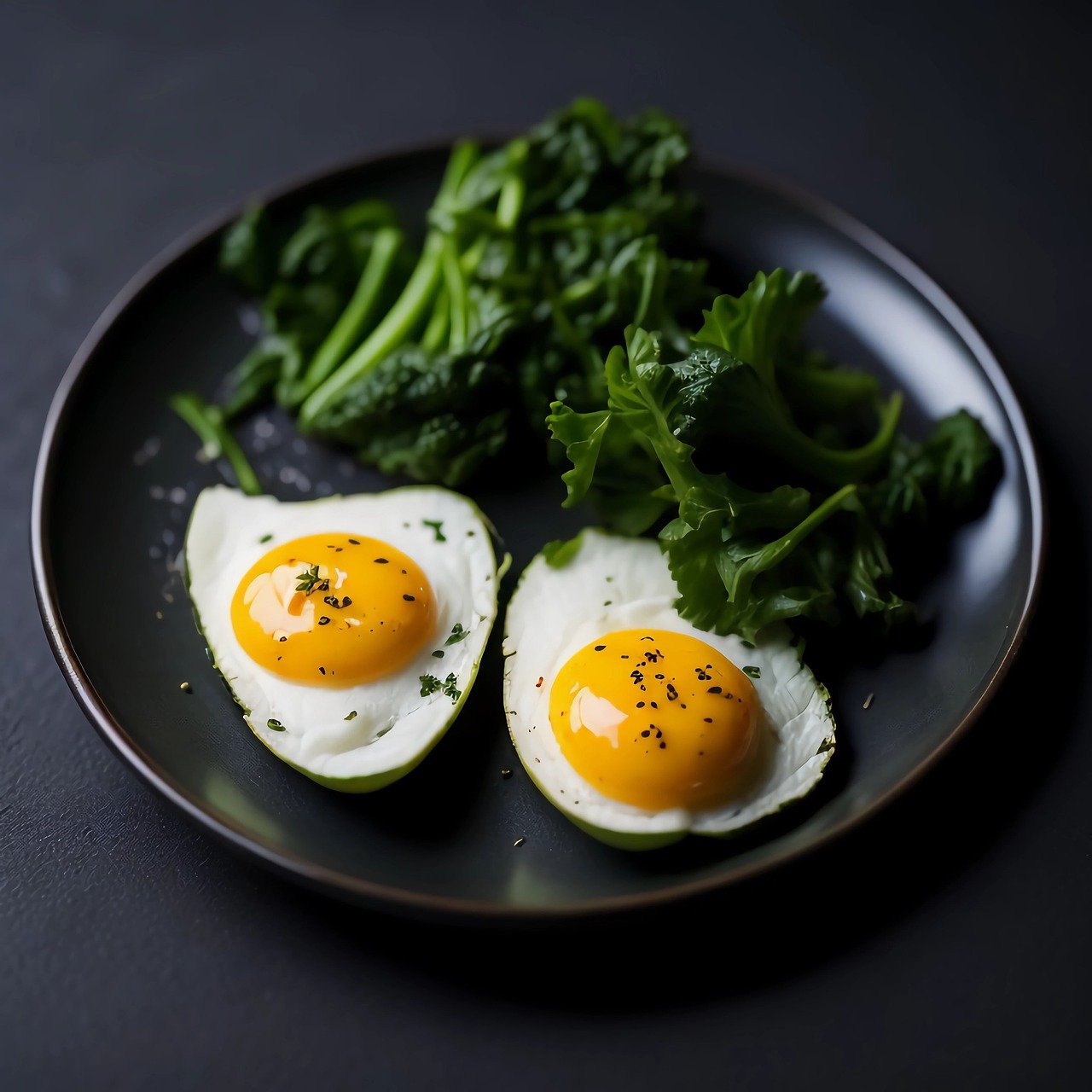
Privacy and Data Protection
In the rapidly evolving landscape of artificial intelligence, privacy concerns have emerged as a pivotal issue that cannot be ignored. As AI systems become more integrated into our daily lives, they often rely on vast amounts of data, which can include sensitive personal information. This raises a critical question: how do we balance the benefits of AI with the need to protect individual privacy? The ethical implications of data collection and usage are profound, and they demand our immediate attention.
First and foremost, it's essential to understand that the data used to train AI systems can come from various sources, including social media, online transactions, and even health records. This data, when not handled responsibly, can lead to significant breaches of privacy. Imagine a world where your personal information is not just stored but analyzed and utilized without your consent. It’s a chilling prospect, isn't it?
To navigate these murky waters, organizations must adopt robust data protection measures. This involves not only compliance with existing regulations, such as the General Data Protection Regulation (GDPR) in Europe but also a commitment to ethical practices that prioritize user consent and data security. Here are some key strategies that organizations can implement:
- Data Minimization: Collect only the data that is absolutely necessary for the AI system to function.
- User Consent: Ensure that users are fully informed about what data is being collected and how it will be used.
- Transparency: Provide clear information on data handling practices to foster trust among users.
- Regular Audits: Conduct periodic reviews of data practices to ensure compliance with ethical standards.
Moreover, the ethical use of data goes beyond just compliance; it’s about creating a culture that values privacy as a fundamental human right. Organizations should strive to be transparent about their data practices and actively engage with users to build trust. This means not only informing users about data usage but also empowering them with choices regarding their information.
As we move forward, the conversation around in AI must evolve. Stakeholders, including developers, policymakers, and users, need to collaborate to create frameworks that not only protect individual rights but also promote innovation. After all, the goal of AI should not be to infringe on privacy but to enhance our lives while respecting our personal boundaries.
In conclusion, as AI continues to advance, the importance of privacy and data protection will only grow. By prioritizing ethical practices and fostering a culture of responsibility, we can ensure that AI technologies serve humanity without compromising our fundamental rights.
Q1: What are the main privacy concerns related to AI?
A1: The main privacy concerns include unauthorized data collection, lack of user consent, and potential misuse of personal information.
Q2: How can organizations ensure they are protecting user privacy?
A2: Organizations can protect user privacy by implementing data minimization practices, ensuring user consent, and conducting regular audits of their data handling procedures.
Q3: What role do regulations like GDPR play in AI privacy?
A3: Regulations like GDPR set legal standards for data protection, requiring organizations to be transparent about data collection and usage while giving users more control over their personal information.
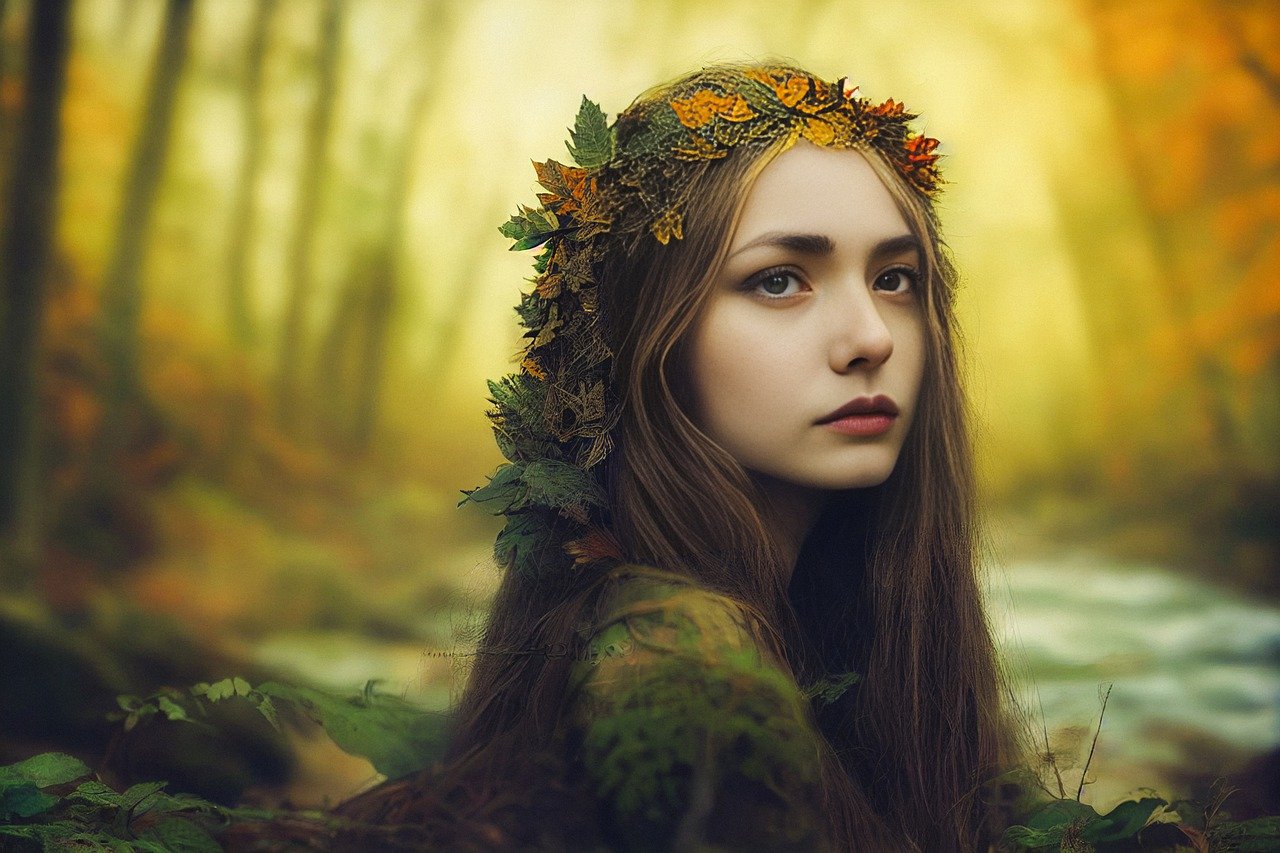
Regulatory Frameworks for Ethical AI
As artificial intelligence continues to evolve at a breakneck pace, the need for robust regulatory frameworks becomes increasingly evident. These frameworks are not just a set of rules; they are the backbone of responsible AI development, ensuring that innovations align with societal values and ethical standards. Without such regulations, we risk creating a technological landscape that operates without accountability, potentially leading to severe consequences for individuals and communities.
One of the primary purposes of regulatory frameworks is to establish clear guidelines that govern the development and deployment of AI technologies. These guidelines help organizations navigate the complex ethical landscape by providing a structured approach to identifying and mitigating risks associated with AI. For instance, regulations can mandate transparency in AI algorithms, ensuring that stakeholders understand how decisions are made. This transparency is crucial because it fosters trust, which is essential for the widespread acceptance of AI technologies.
Currently, several international standards and guidelines exist that offer a roadmap for ethical AI development. Organizations such as the IEEE and the ISO have developed frameworks that outline best practices for AI ethics. These frameworks cover various aspects, including fairness, accountability, and data protection. By adhering to these standards, companies can not only enhance their credibility but also contribute to a more equitable technological landscape.
However, the implementation of these regulatory frameworks is not without its challenges. One significant hurdle is the lack of a unified approach across different jurisdictions. As AI technology transcends borders, the disparity in regulations can create confusion and compliance issues for multinational companies. For example, what is permissible in one country may be strictly prohibited in another, leading to a patchwork of regulations that can hinder innovation.
Moreover, enforcement of compliance with ethical standards poses its own set of challenges. Organizations often struggle to keep up with the rapidly changing technological landscape, making it difficult to ensure that their AI systems remain compliant with existing regulations. This is where fostering a culture of ethical responsibility within organizations becomes crucial. By prioritizing ethics in their core values, companies can proactively address compliance issues and create a more responsible AI ecosystem.
In summary, regulatory frameworks for ethical AI are essential for guiding the development of responsible technology. They provide a necessary structure that promotes accountability and transparency while helping to mitigate risks associated with AI. As stakeholders work together to refine and implement these regulations, the focus must remain on fostering a culture of ethical responsibility that ensures AI serves the greater good.
- What are regulatory frameworks for AI? Regulatory frameworks for AI are guidelines and standards that govern the ethical development and deployment of artificial intelligence technologies.
- Why are ethical regulations important? Ethical regulations are important because they ensure that AI technologies are developed responsibly, promoting transparency, accountability, and trust among users.
- What challenges do organizations face in complying with AI regulations? Organizations face challenges such as varying regulations across jurisdictions, rapidly changing technologies, and the need to foster a culture of ethical responsibility.
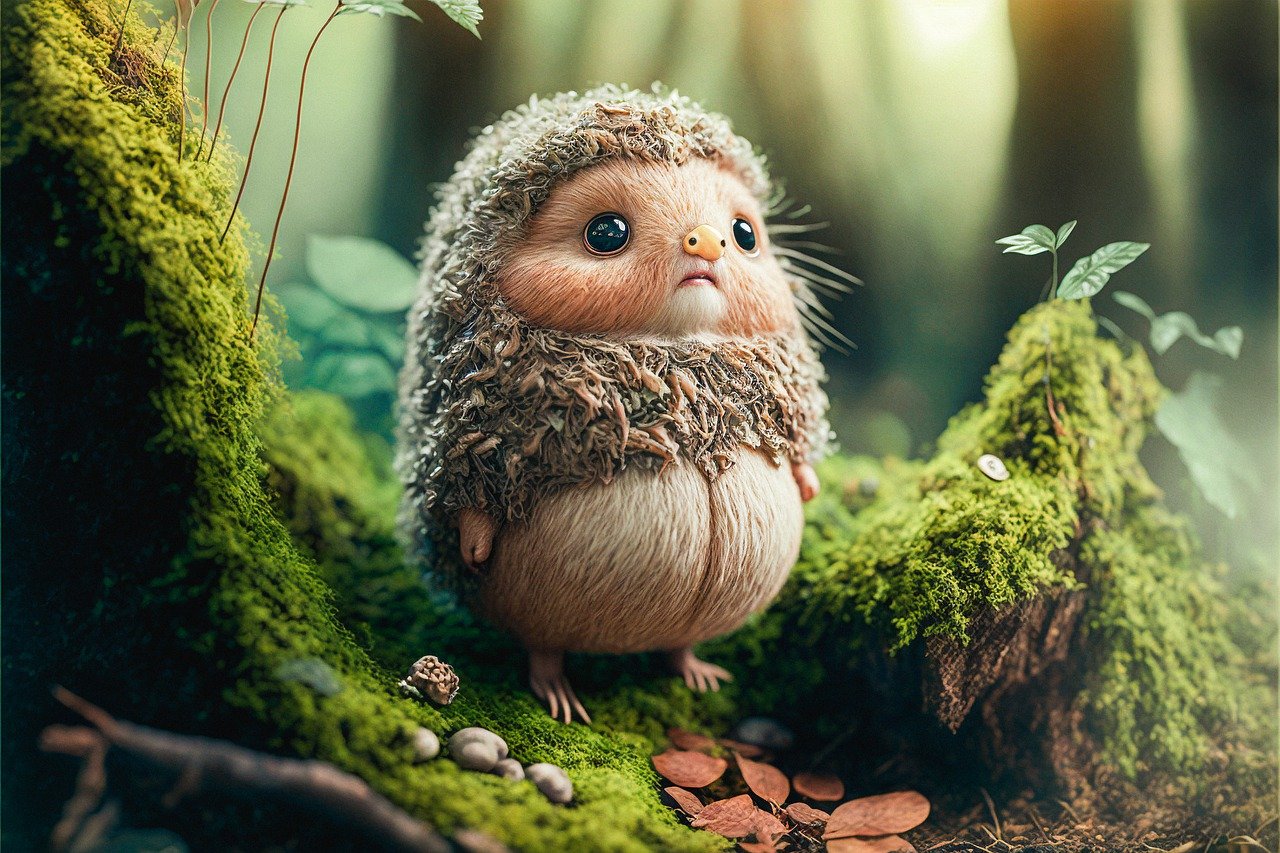
International Standards and Guidelines
In the rapidly evolving landscape of artificial intelligence, serve as crucial navigational tools for organizations striving to develop ethical AI systems. These frameworks are designed to ensure that AI technologies are not only innovative but also respect human rights and societal values. Without these guidelines, the risk of ethical lapses increases significantly, potentially leading to harmful consequences for individuals and communities.
One of the most recognized frameworks is the ISO/IEC JTC 1/SC 42, which focuses on artificial intelligence. This standard emphasizes the need for transparency, accountability, and ethical considerations in AI development. By adhering to these standards, organizations can create systems that are not only effective but also trustworthy. The guidelines encourage developers to consider the implications of their AI systems on users and society at large, prompting them to ask critical questions about fairness, accountability, and transparency.
Furthermore, the OECD Principles on Artificial Intelligence provide a comprehensive set of recommendations that countries can adopt to foster responsible AI use. These principles advocate for:
- Inclusive growth: Ensuring that AI benefits all segments of society.
- Human-centered values: Prioritizing human rights and democratic values in AI decision-making.
- Robustness and safety: Guaranteeing that AI systems are secure and resilient against failures.
In addition to these frameworks, several organizations, including the IEEE and the European Commission, have developed guidelines that emphasize ethical considerations in AI. For instance, the IEEE's Ethically Aligned Design initiative encourages developers to consider the ethical implications of their technologies from the ground up. This approach not only enhances the ethical integrity of AI systems but also builds public trust in these technologies.
However, the implementation of these international standards is not without challenges. Organizations often struggle with compliance due to the complexity of regulations and the rapidly changing technological landscape. It is crucial for companies to foster a culture of ethical responsibility within their teams, ensuring that all members understand the importance of these guidelines and are equipped to implement them effectively.
In summary, international standards and guidelines play a pivotal role in shaping the ethical landscape of artificial intelligence. By adhering to these frameworks, organizations can navigate the complexities of AI development responsibly, ensuring that their innovations align with societal values and contribute positively to the world. As AI continues to advance, the importance of these standards will only grow, highlighting the need for ongoing dialogue and collaboration among stakeholders.
What are international standards for AI?
International standards for AI are guidelines and frameworks developed by organizations such as ISO, IEEE, and OECD to promote ethical practices in AI development. They emphasize transparency, accountability, and respect for human rights.
Why are these standards important?
These standards are crucial because they help ensure that AI technologies are developed responsibly, minimizing risks of bias, discrimination, and other ethical issues. They foster public trust and encourage organizations to prioritize ethical considerations in their AI systems.
How can organizations implement these standards?
Organizations can implement these standards by educating their teams about the guidelines, conducting regular audits of their AI systems, and fostering a culture of ethical responsibility. Collaboration with stakeholders and adherence to best practices are also essential for effective implementation.
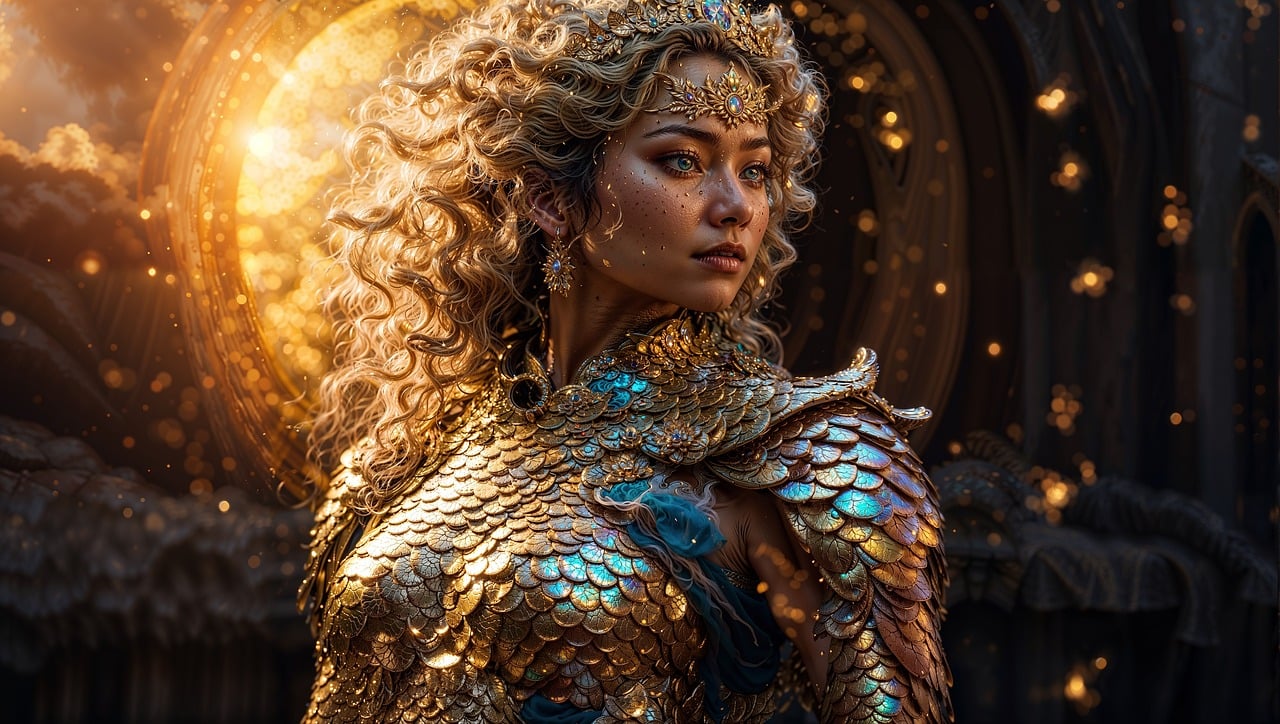
Enforcement and Compliance Challenges
When it comes to the enforcement of ethical standards in artificial intelligence, the road is anything but smooth. Organizations often find themselves navigating a complex web of regulations that can vary significantly from one jurisdiction to another. This inconsistency can create confusion, making it challenging for companies to know what is expected of them. Moreover, the rapid pace of AI advancement means that regulations can quickly become outdated, leaving a gap between technological capabilities and legal frameworks.
One of the major hurdles in compliance is the lack of clear guidelines. Many companies struggle with understanding how to implement ethical practices effectively. For instance, while some regulations may emphasize transparency, they may not provide specific metrics or methodologies for achieving it. This ambiguity can lead to a “check-the-box” mentality, where organizations do the bare minimum to comply rather than genuinely embracing ethical AI practices.
Furthermore, the cost of compliance can be daunting. Smaller companies, in particular, may lack the resources to implement rigorous compliance programs. This creates an uneven playing field where larger corporations can absorb the costs of compliance while smaller firms may cut corners, potentially leading to ethical breaches. As a result, the very essence of fairness and accountability in AI can be compromised.
Another significant challenge is the enforcement of these regulations. Regulatory bodies often lack the necessary tools and expertise to monitor AI systems effectively. For example, how can a regulator assess an AI algorithm's fairness if they do not have access to the underlying data or model? This lack of transparency can lead to a situation where unethical practices go unchecked, further eroding public trust in AI technologies.
To address these challenges, organizations need to foster a culture of ethical responsibility from the top down. This involves not just compliance with regulations but a commitment to ethical principles that guide decision-making. Companies can implement training programs that educate employees about the importance of ethical AI, emphasizing that ethical considerations should be integrated into every stage of the AI development process.
In addition, collaboration between industry stakeholders, regulatory bodies, and academia can lead to the development of more robust enforcement mechanisms. By working together, these groups can create a unified approach to ethical AI that balances innovation with accountability. For instance, establishing a shared framework for ethical AI can help standardize practices across the industry, making it easier for organizations to comply with regulations.
Ultimately, while the challenges of enforcement and compliance in AI are significant, they are not insurmountable. By prioritizing ethical considerations and fostering collaboration, organizations can navigate these complexities effectively. The journey towards ethical AI is ongoing, but with commitment and cooperation, we can pave the way for a future where technology serves humanity responsibly.
- What are the main challenges in enforcing ethical AI standards?
Enforcement challenges include regulatory inconsistencies, lack of clear guidelines, high compliance costs, and limited monitoring capabilities. - How can organizations foster a culture of ethical responsibility?
Organizations can implement training programs, encourage open discussions about ethics, and integrate ethical considerations into all stages of AI development. - Why is collaboration important in addressing ethical AI challenges?
Collaboration among stakeholders can lead to the creation of unified frameworks and standards that facilitate compliance and accountability.
Frequently Asked Questions
- What are the main ethical challenges in AI development?
The main ethical challenges in AI development include bias in algorithms, privacy concerns, and the need for transparency in decision-making processes. These challenges require careful consideration to ensure that AI technologies are developed responsibly and ethically.
- How can bias be introduced in AI systems?
Bias can be introduced in AI systems through various means, such as biased data selection, unrepresentative training datasets, or flawed algorithmic design. This can lead to unfair outcomes, particularly for marginalized communities, making it essential to identify and address these biases early in the development process.
- What strategies can be implemented to mitigate algorithmic bias?
To mitigate algorithmic bias, developers can use diverse datasets, conduct regular audits of their AI systems, and adopt inclusive design practices. By ensuring that a wide range of perspectives is considered, AI solutions can be made more equitable and just for all users.
- Why is privacy a significant concern in AI?
Privacy is a significant concern in AI because these systems often rely on vast amounts of personal data for training and operation. Without robust data protection measures, individuals' rights can be compromised, leading to potential misuse of their information and erosion of trust in AI technologies.
- What existing regulatory frameworks address ethical AI?
There are several regulatory frameworks and guidelines aimed at promoting ethical AI practices, such as the EU's General Data Protection Regulation (GDPR) and various international standards. These frameworks help organizations implement responsible AI practices while ensuring accountability and transparency.
- What challenges do organizations face in complying with ethical standards?
Organizations often face challenges such as a lack of clear guidelines, insufficient resources for compliance, and cultural resistance to change. These obstacles can hinder the effective implementation of ethical standards, making it crucial to foster a culture of ethical responsibility within organizations.
- How can stakeholders promote ethical AI practices?
Stakeholders can promote ethical AI practices by prioritizing transparency, engaging in ongoing education about ethical implications, and advocating for inclusive design processes. Collaboration among developers, policymakers, and users is key to ensuring that AI technologies align with societal values.