Artificial Intelligence in Healthcare: Emerging Possibilities
In recent years, the healthcare industry has witnessed a remarkable transformation, primarily driven by the advent of artificial intelligence (AI). This groundbreaking technology is not just a buzzword; it’s reshaping how we approach patient care, diagnostics, and even drug discovery. Imagine a world where diseases are detected before symptoms even appear, or where treatment plans are tailored specifically to your genetic makeup. Sounds like science fiction, right? But this is the reality that AI is bringing to the forefront of healthcare. With the ability to analyze vast amounts of data at lightning speed, AI technologies are paving the way for enhanced accuracy, efficiency, and ultimately, better patient outcomes.
One of the most exciting aspects of AI in healthcare is its potential to revolutionize diagnostics. Traditional diagnostic methods can be time-consuming and often rely heavily on human interpretation, which can lead to errors. However, AI algorithms are capable of analyzing medical images and patient data with unparalleled precision. For instance, in the realm of oncology, AI can help radiologists identify tumors that may not be visible to the naked eye, enabling earlier and more effective interventions. This capability is not just limited to cancer; cardiovascular diseases and other conditions can also be diagnosed more accurately, potentially saving countless lives.
Furthermore, the concept of personalized medicine is gaining traction thanks to AI. Rather than adopting a one-size-fits-all approach, AI allows healthcare providers to tailor treatments based on an individual's unique genetic profile and medical history. This not only enhances the effectiveness of treatments but also minimizes the risk of adverse side effects that can occur with traditional therapies. Imagine being prescribed a medication that is specifically designed for your genetic makeup—this is the future that AI is helping to create.
Additionally, AI's role in predictive analytics is another game-changer in patient care. By examining historical patient data, AI can identify trends and patterns that might indicate a patient is at risk for certain health conditions. This proactive approach allows healthcare providers to implement preventive measures before issues escalate, leading to better health management. For example, if a patient’s data suggests a high risk of diabetes, healthcare teams can intervene early, offering lifestyle changes and monitoring that could prevent the disease from developing.
Moreover, risk stratification models powered by AI categorize patients based on their likelihood of developing specific health issues. This categorization enables healthcare providers to allocate resources more effectively and focus on high-risk patients who may benefit the most from targeted interventions. It’s like having a crystal ball that helps healthcare teams see who needs their attention the most, thus optimizing patient care and improving overall health outcomes.
Another significant application of AI is in clinical decision support systems. These systems assist healthcare professionals by providing evidence-based recommendations during the decision-making process. By integrating AI into clinical workflows, providers can reduce diagnostic errors and enhance treatment strategies, ultimately leading to improved patient outcomes. It’s as if AI acts as a trusted advisor, offering insights that complement the expertise of healthcare providers.
Lastly, AI is making waves in the field of drug discovery. Traditionally, the process of bringing a new drug to market is lengthy and expensive. However, AI can analyze vast datasets to identify potential drug candidates much faster than traditional methods. This not only shortens the time it takes to develop new medications but also significantly reduces costs, benefiting both pharmaceutical companies and patients alike. It’s like having a supercharged research assistant that can sift through mountains of data in seconds!
- What is artificial intelligence in healthcare?
AI in healthcare refers to the use of machine learning algorithms and software to analyze complex medical data, improving diagnostics, treatment planning, and patient outcomes. - How does AI improve diagnostics?
AI enhances diagnostics by analyzing medical images and patient data quickly and accurately, leading to earlier detection of diseases. - What is personalized medicine?
Personalized medicine tailors treatment plans to individual patients based on their genetic information and medical history, improving effectiveness and reducing side effects. - What are predictive analytics?
Predictive analytics uses historical data to anticipate patient needs and outcomes, allowing for proactive healthcare interventions. - What challenges does AI face in healthcare?
Challenges include data privacy concerns, integration with existing systems, and the need for regulatory frameworks to ensure safety and efficacy.
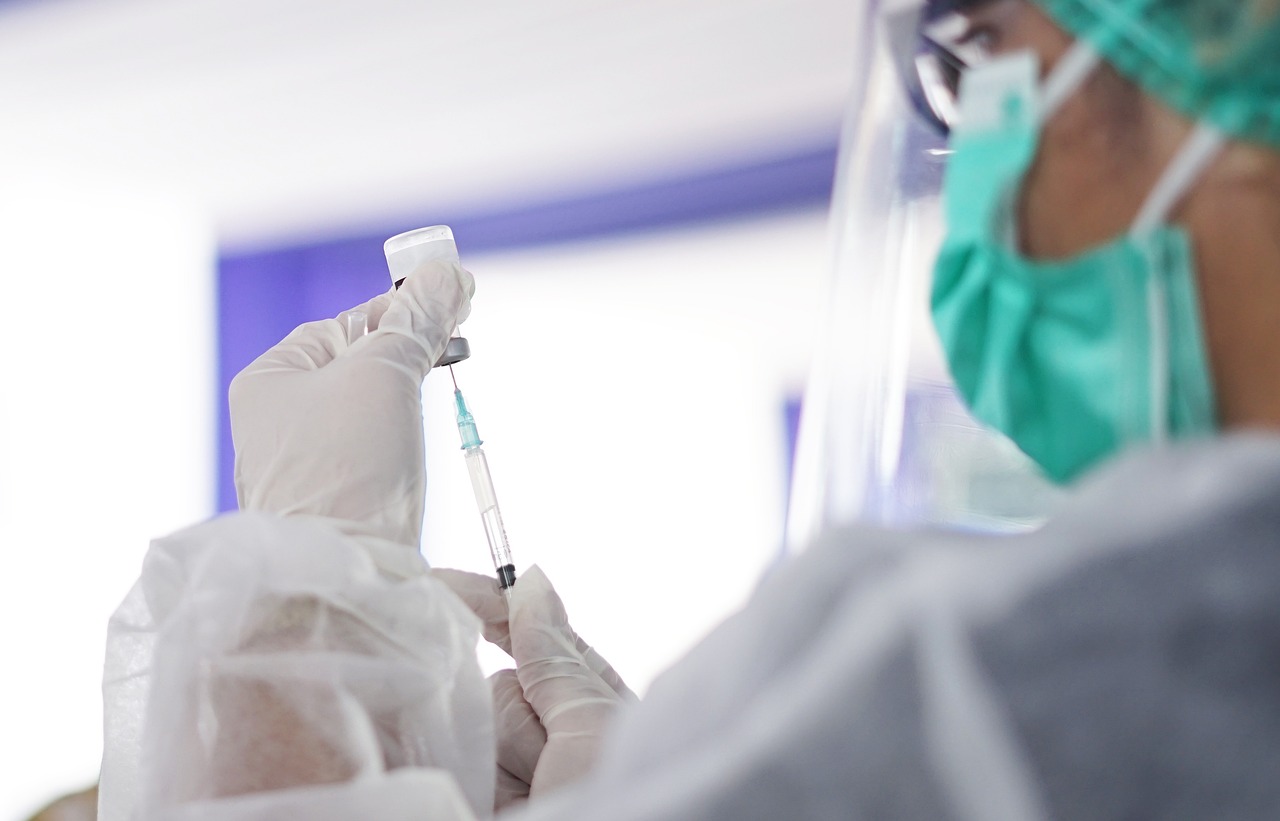
AI Applications in Diagnostics
Artificial Intelligence (AI) technologies are revolutionizing diagnostics in the healthcare sector, leading to remarkable improvements in both accuracy and speed. Imagine a world where machines can analyze medical images and vast datasets faster than a human ever could. This transformation is not just a futuristic dream; it's happening right now! Machine learning algorithms are at the forefront of this innovation, allowing for earlier detection of diseases that can be life-threatening if not caught in time, such as cancer and cardiovascular conditions.
One of the most exciting aspects of AI in diagnostics is its ability to process and analyze complex medical images, such as MRIs, CT scans, and X-rays. Traditional methods often rely on the expertise of radiologists, who may miss subtle signs of disease due to fatigue or the sheer volume of images they need to review. With AI, we can enhance the diagnostic process by providing additional insights that might go unnoticed. For instance, studies have shown that AI systems can achieve accuracy rates comparable to, or even exceeding, those of seasoned radiologists in detecting certain types of tumors.
Furthermore, AI's capability to analyze patient data extends beyond just imaging. By integrating various data sources, including electronic health records (EHRs), lab results, and genetic information, AI can provide a comprehensive overview of a patient's health status. This holistic approach allows healthcare providers to make better-informed decisions, ultimately leading to improved patient outcomes. For example, AI can help identify patterns in patient data that indicate a higher risk of developing chronic conditions, enabling proactive interventions.
To illustrate the impact of AI in diagnostics, consider the following table that summarizes key benefits:
Benefit | Description |
---|---|
Enhanced Accuracy | AI algorithms can analyze images and data with high precision, reducing the likelihood of misdiagnosis. |
Speed of Diagnosis | AI can process large volumes of data in a fraction of the time it takes human professionals, facilitating quicker treatment decisions. |
Predictive Insights | By analyzing historical data, AI can predict potential health issues before they manifest, allowing for early intervention. |
Resource Optimization | AI can help prioritize cases based on urgency, ensuring that critical patients receive timely attention. |
As we delve deeper into the realm of AI applications in diagnostics, it's crucial to address the challenges that come with this technology. While the benefits are significant, the integration of AI into diagnostic practices must be approached with caution. Issues such as data privacy, the need for robust training datasets, and the potential for algorithmic bias must be carefully considered. However, the potential rewards are too great to ignore. With ongoing advancements in AI, we are on the brink of a new era in healthcare diagnostics that promises to save lives and enhance the quality of care.
- How does AI improve diagnostic accuracy? AI improves diagnostic accuracy by analyzing complex medical images and data quickly and efficiently, often identifying patterns that human eyes might miss.
- What types of diseases can AI help detect? AI can assist in detecting a wide range of diseases, including cancer, cardiovascular conditions, and various chronic illnesses.
- Are there any risks associated with using AI in diagnostics? Yes, risks include potential data privacy concerns, algorithmic bias, and the need for comprehensive regulatory frameworks to ensure safety and efficacy.
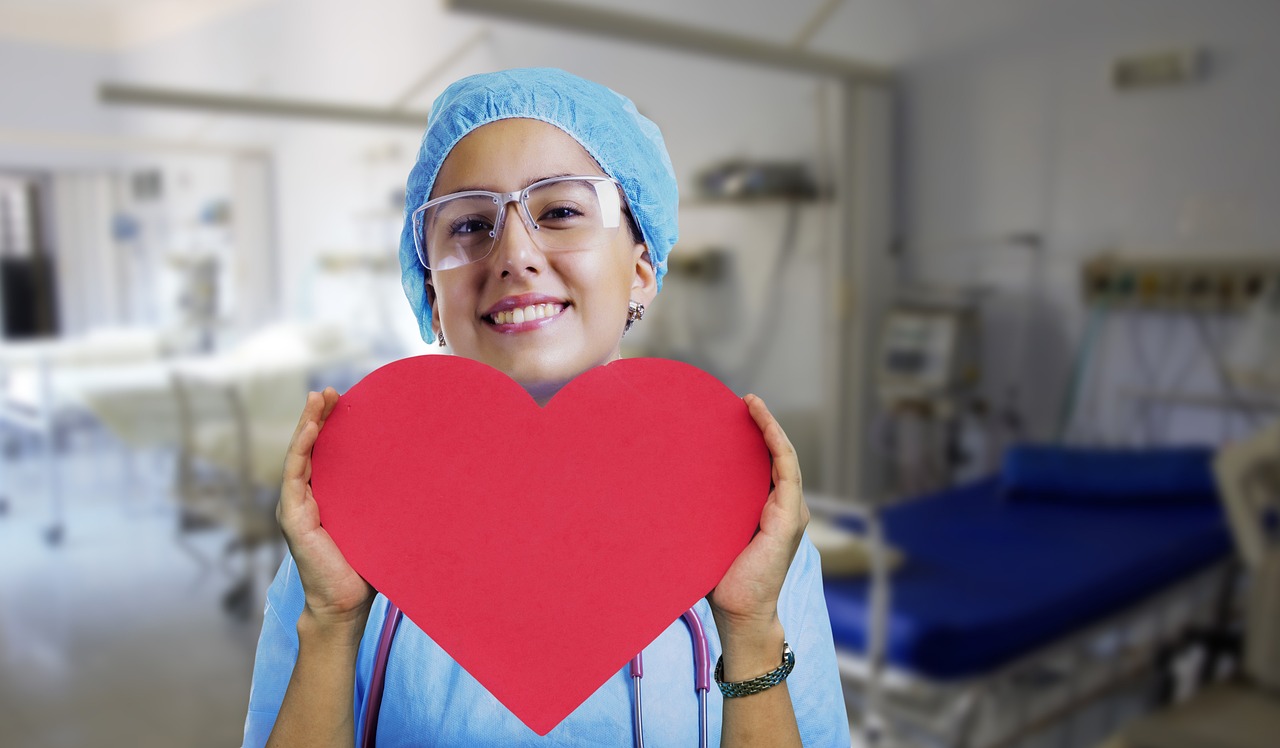
Personalized Medicine Through AI
Imagine a world where your treatment plan is as unique as your DNA. Personalized medicine is not just a futuristic dream; it's becoming a reality thanks to the transformative power of artificial intelligence (AI). By analyzing a patient’s genetic makeup alongside their medical history, AI can tailor treatments specifically designed for individual needs. This approach is a game-changer, moving away from the traditional one-size-fits-all model that often leads to ineffective treatments and unnecessary side effects.
At the heart of personalized medicine is the ability of AI to process vast amounts of data quickly and accurately. For instance, AI algorithms can sift through thousands of genetic variations to identify markers that predict how a patient will respond to a particular medication. This not only enhances the effectiveness of treatments but also minimizes the risk of adverse reactions. Think of it as having a personal health assistant that knows your body better than anyone else!
Moreover, AI’s capability in predictive analytics plays a crucial role in personalizing treatment plans. By examining historical data from similar patient profiles, AI can forecast potential health issues and suggest preemptive measures. This proactive approach ensures that healthcare providers can act before problems escalate, leading to better health outcomes. For example, if a patient has a family history of diabetes, AI can recommend lifestyle changes or preventative medications tailored to that individual’s risk factors.
To illustrate the impact of AI in personalized medicine, consider the following table that summarizes key benefits:
Benefit | Description |
---|---|
Improved Treatment Efficacy | AI tailors treatments to individual genetic profiles, enhancing effectiveness. |
Reduced Side Effects | Personalized approaches minimize adverse reactions commonly seen in standard treatments. |
Proactive Health Management | Predictive analytics allows for early intervention based on individual risk factors. |
Furthermore, AI can assist in the development of targeted therapies. For instance, in oncology, AI algorithms analyze tumor genetics to identify which treatments are most likely to succeed for specific cancer types. This means that instead of treating all patients with the same chemotherapy regimen, doctors can select targeted therapies that are more effective for the unique genetic profile of each tumor. This shift not only improves survival rates but also enhances the quality of life for patients undergoing treatment.
However, while the prospects of personalized medicine through AI are exciting, it’s essential to address the challenges that come with it. Issues such as data privacy, ethical considerations, and the need for robust regulatory frameworks must be navigated carefully. After all, as we embrace this advanced technology, we must ensure that patient safety and confidentiality remain a top priority.
In conclusion, the integration of AI into personalized medicine is revolutionizing healthcare. By harnessing the power of data, AI is paving the way for treatments that are not only more effective but also safer for patients. As we continue to explore these possibilities, one thing is clear: the future of healthcare is personal, and AI is at the forefront of this remarkable evolution.
- What is personalized medicine? Personalized medicine is a medical model that tailors healthcare, with decisions and treatments customized to the individual patient based on their genetic makeup, lifestyle, and environment.
- How does AI improve personalized medicine? AI enhances personalized medicine by analyzing large datasets to identify patterns and predict how patients will respond to specific treatments, leading to more effective and targeted therapies.
- What are the challenges associated with AI in healthcare? Challenges include data privacy concerns, the integration of AI with existing healthcare systems, and the need for regulatory frameworks to ensure the safety and efficacy of AI applications.
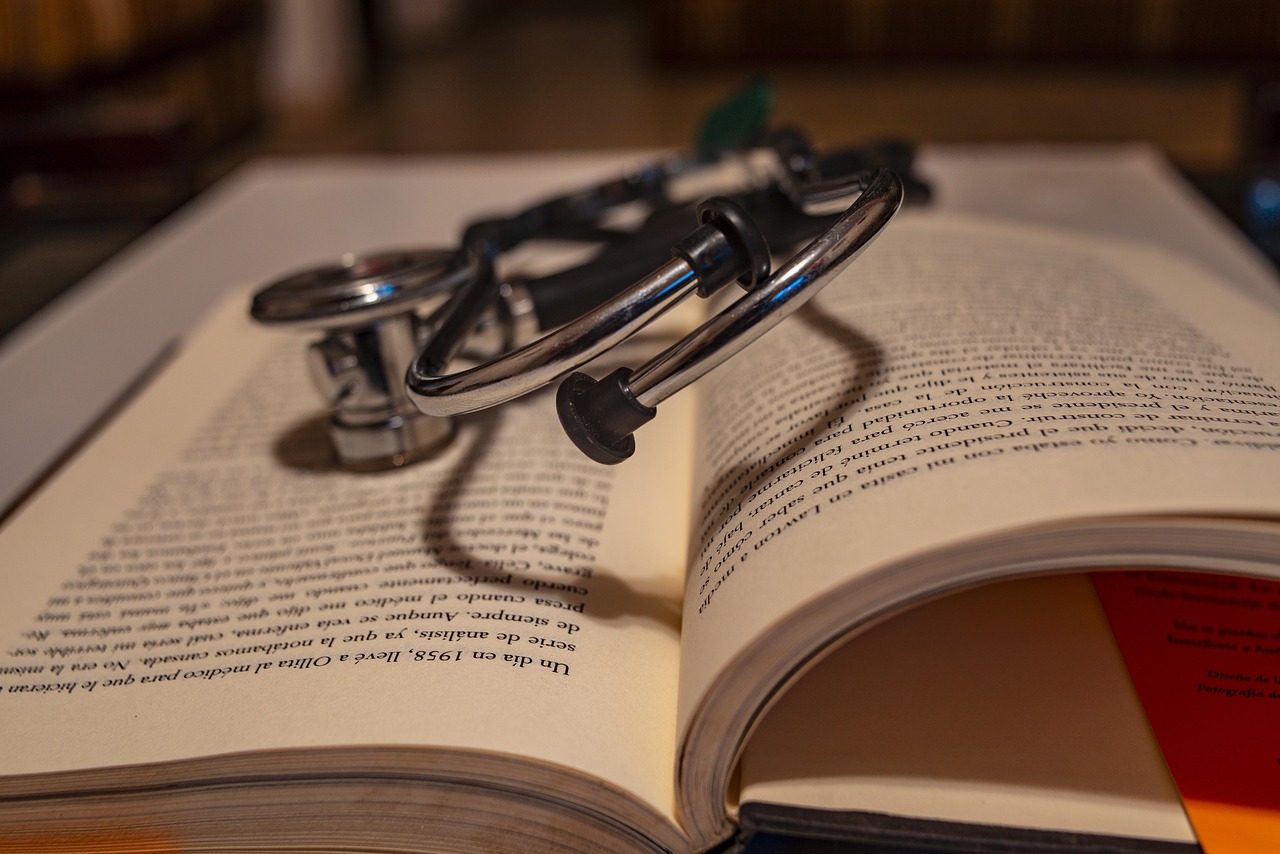
Predictive Analytics in Patient Care
Predictive analytics in patient care is like having a crystal ball that helps healthcare providers foresee potential health issues before they escalate. Imagine being able to predict a storm before it hits; that's what predictive analytics does for patient health. By leveraging historical data and advanced algorithms, healthcare professionals can identify patterns and trends that indicate which patients are at risk for certain conditions. This proactive approach not only enhances patient outcomes but also optimizes resource allocation within healthcare systems.
One of the most exciting aspects of predictive analytics is its ability to analyze vast amounts of data from various sources, such as electronic health records (EHRs), lab results, and even social determinants of health. For example, if a patient has a history of heart disease and certain lifestyle factors like obesity and smoking, predictive models can flag them as at risk for a heart attack. This early warning system allows providers to implement preventive measures, such as lifestyle interventions or increased monitoring, tailored to the individual's needs.
Furthermore, predictive analytics can significantly enhance chronic disease management. For patients with conditions like diabetes or hypertension, continuous monitoring and analysis can lead to timely interventions that improve quality of life and reduce hospitalizations. By predicting potential complications, healthcare teams can engage patients in their care more effectively, empowering them to make informed decisions about their health.
To illustrate the impact of predictive analytics, consider the following table that outlines its benefits in patient care:
Benefit | Description |
---|---|
Early Detection | Identifies at-risk patients before symptoms appear, allowing for timely intervention. |
Resource Optimization | Allocates healthcare resources more efficiently by focusing on high-risk patients. |
Improved Patient Engagement | Encourages patients to take an active role in their healthcare through tailored recommendations. |
Cost Reduction | Reduces unnecessary hospitalizations and emergency visits through proactive care. |
The beauty of predictive analytics lies in its ability to transform raw data into actionable insights. However, it’s important to note that while predictive models can provide valuable information, they are not infallible. Healthcare providers must combine these insights with their clinical judgment to make the best decisions for their patients. In doing so, they can create a more personalized and effective healthcare experience that not only anticipates needs but also fosters a deeper connection between patients and providers.
As we move forward, the integration of predictive analytics in patient care will likely become more sophisticated. With advancements in machine learning and artificial intelligence, the accuracy and reliability of these predictions will continue to improve, paving the way for a healthcare system that is not only reactive but also proactive. Just as a skilled navigator uses the stars to chart a course, healthcare providers will increasingly rely on predictive analytics to guide their decisions, ensuring that patients receive the care they need, when they need it.
- What is predictive analytics in healthcare?
Predictive analytics in healthcare refers to the use of statistical techniques and algorithms to analyze historical data and predict future outcomes, enabling healthcare providers to anticipate patient needs and improve care.
- How does predictive analytics improve patient care?
By identifying at-risk patients early, predictive analytics allows for timely interventions, better management of chronic diseases, and overall enhanced patient engagement and outcomes.
- Are there any challenges associated with predictive analytics?
Yes, challenges include data privacy concerns, the need for high-quality data, and the necessity for healthcare providers to integrate predictive insights with their clinical judgment.

Risk Stratification Models
In the realm of healthcare, are becoming increasingly vital as they enable healthcare providers to categorize patients based on their risk of developing specific health conditions. Imagine walking into a crowded room and being able to immediately identify which individuals might need help the most. This is precisely what risk stratification models achieve, allowing for a more focused and effective approach to patient care.
These models utilize advanced artificial intelligence algorithms to analyze a plethora of data points, including medical history, demographic information, and lifestyle factors. By sifting through this information, AI can identify patterns and predict which patients are at the highest risk for diseases such as diabetes, heart disease, or even certain types of cancer. This not only allows for early intervention but also empowers healthcare providers to allocate resources more efficiently.
For instance, consider a hospital that has implemented a risk stratification model. With the help of AI, they can classify their patient population into different risk categories:
Risk Category | Criteria | Recommended Interventions |
---|---|---|
High Risk | Multiple chronic conditions, recent hospitalizations | Intensive monitoring and personalized care plans |
Moderate Risk | Single chronic condition, lifestyle factors | Regular follow-ups and lifestyle modification programs |
Low Risk | No significant health issues | Standard preventive care and wellness programs |
This categorization not only streamlines the care process but also enhances patient outcomes by ensuring that those who need the most attention receive it promptly. Furthermore, it allows healthcare systems to forecast demand for services and manage their resources accordingly. However, implementing these models is not without challenges. Data accuracy and the integration of diverse datasets are critical to the success of risk stratification efforts.
Moreover, healthcare providers must also consider the ethical implications of using AI in risk stratification. Questions surrounding bias in algorithms and the potential for exacerbating health disparities must be addressed. As we delve deeper into the age of AI in healthcare, it becomes essential to ensure that these systems are designed with fairness and inclusivity in mind.
In conclusion, risk stratification models represent a significant leap forward in how we approach patient care. By harnessing the power of AI, healthcare providers can not only improve patient outcomes but also create a more efficient and effective healthcare system. As we continue to explore the capabilities of artificial intelligence, the potential for enhancing patient care is truly limitless.
- What are risk stratification models? Risk stratification models are tools that categorize patients based on their likelihood of developing specific health conditions, enabling targeted interventions.
- How does AI enhance risk stratification? AI analyzes large datasets to identify patterns and predict patient risks, allowing for earlier interventions and better resource allocation.
- What challenges exist with risk stratification models? Key challenges include data accuracy, integration with existing systems, and addressing ethical concerns related to bias and health disparities.

Clinical Decision Support Systems
Clinical Decision Support Systems (CDSS) are transforming the landscape of healthcare by providing healthcare professionals with powerful tools to enhance their decision-making processes. Imagine having a knowledgeable assistant by your side, ready to sift through mountains of medical data and literature to provide you with the most relevant information at your fingertips. That's precisely what CDSS does. These systems utilize artificial intelligence to analyze patient data, medical histories, and clinical guidelines, offering evidence-based recommendations that can significantly improve patient outcomes.
One of the most remarkable aspects of CDSS is their ability to integrate seamlessly into the clinical workflow. For instance, when a physician inputs a patient's symptoms and medical history, the CDSS can quickly cross-reference this information against a vast database of clinical knowledge. This allows the system to highlight potential diagnoses, suggest appropriate tests, and even recommend treatment options tailored to the patient's specific needs. In this way, CDSS acts as a safety net, reducing the likelihood of diagnostic errors and ensuring that patients receive timely and appropriate care.
Moreover, CDSS can be particularly beneficial in managing complex cases where multiple conditions coexist. In such scenarios, the system can analyze interactions between different medications and suggest alternatives that minimize adverse effects. This capability is crucial in today's healthcare environment, where patients often present with multiple comorbidities. By leveraging AI, CDSS can help healthcare providers navigate these complexities, ultimately leading to better patient management.
However, it’s essential to acknowledge that while CDSS offers numerous benefits, they are not a substitute for the healthcare professional's expertise. Instead, they are designed to complement clinical judgment. The most effective use of CDSS occurs when healthcare providers utilize the system's recommendations as a starting point for further investigation and discussion with their patients. This collaborative approach fosters a more comprehensive understanding of the patient's condition and promotes shared decision-making.
To illustrate the impact of CDSS, consider the following table that outlines some key benefits:
Benefit | Description |
---|---|
Improved Accuracy | Reduces diagnostic errors by providing evidence-based recommendations. |
Time Efficiency | Speeds up decision-making by quickly analyzing patient data. |
Enhanced Patient Safety | Identifies potential drug interactions and contraindications. |
Personalized Care | Offers tailored treatment options based on individual patient profiles. |
In conclusion, Clinical Decision Support Systems represent a significant advancement in healthcare technology, harnessing the power of AI to support healthcare professionals in their vital work. As these systems continue to evolve, they will undoubtedly play an increasingly critical role in enhancing patient care and operational efficiency within healthcare settings.
- What is a Clinical Decision Support System?
A Clinical Decision Support System (CDSS) is a health information technology system that analyzes data to help healthcare providers make clinical decisions.
- How does CDSS improve patient outcomes?
By providing evidence-based recommendations and reducing diagnostic errors, CDSS improves the accuracy of diagnoses and treatment plans, leading to better patient outcomes.
- Is CDSS a replacement for healthcare professionals?
No, CDSS is not a replacement but a tool that complements the expertise of healthcare professionals, aiding them in making informed decisions.
- Can CDSS handle complex medical cases?
Yes, CDSS can analyze multiple conditions and medications to provide recommendations that consider potential interactions and contraindications.

AI in Drug Discovery
Artificial Intelligence is making waves in the field of drug discovery, transforming how new medications are identified and developed. Traditionally, the process of discovering a new drug could take over a decade and cost billions of dollars. However, with the power of AI technologies, this timeline is being significantly shortened. Imagine having a super-smart assistant that can sift through mountains of data in seconds, pinpointing potential drug candidates that would have taken humans years to discover. That’s what AI brings to the table!
One of the most exciting aspects of AI in drug discovery is its ability to analyze vast datasets, including genetic information, clinical trial results, and even scientific literature. This capability allows researchers to uncover hidden patterns and relationships that might not be immediately obvious. For instance, AI algorithms can identify how certain compounds interact with biological targets, leading to the discovery of new therapies for diseases that currently have limited treatment options.
Moreover, AI can simulate how different drugs will behave in the body, which is a game-changer. This means that researchers can conduct virtual trials before moving to actual human testing, saving both time and resources. Not only does this speed up the process, but it also minimizes the risks associated with drug development.
Here’s a quick breakdown of how AI is reshaping drug discovery:
- Data Mining: AI algorithms can analyze existing data to find new uses for existing drugs, a process known as drug repurposing.
- Predictive Modeling: By predicting how different drugs will interact with various biological systems, AI helps in selecting the most promising candidates for further testing.
- Clinical Trial Optimization: AI can streamline the recruitment process for clinical trials, ensuring that the right patients are selected based on their genetic profiles and health histories.
As we look to the future, the integration of AI into drug discovery is expected to continue growing, with pharmaceutical companies increasingly relying on these technologies to enhance their research processes. The potential for faster, cheaper, and more effective drug development is not just a dream; it’s becoming a reality. However, it’s essential to navigate the challenges that come with these advancements, including ethical considerations and the need for robust regulatory frameworks to ensure patient safety.
In conclusion, AI is not just a tool; it’s a revolution in drug discovery that promises to change the landscape of medicine as we know it. With its ability to analyze complex data sets and predict outcomes, AI is paving the way for breakthroughs that could lead to life-saving treatments for diseases that affect millions of people worldwide.
- What is AI in drug discovery? AI in drug discovery refers to the use of artificial intelligence technologies to streamline and enhance the process of identifying and developing new medications.
- How does AI improve drug discovery? AI improves drug discovery by analyzing large datasets to identify potential drug candidates, predicting their effects, and optimizing clinical trials.
- What are the challenges of using AI in drug discovery? Challenges include data privacy concerns, the need for regulatory frameworks, and integrating AI with existing healthcare systems.
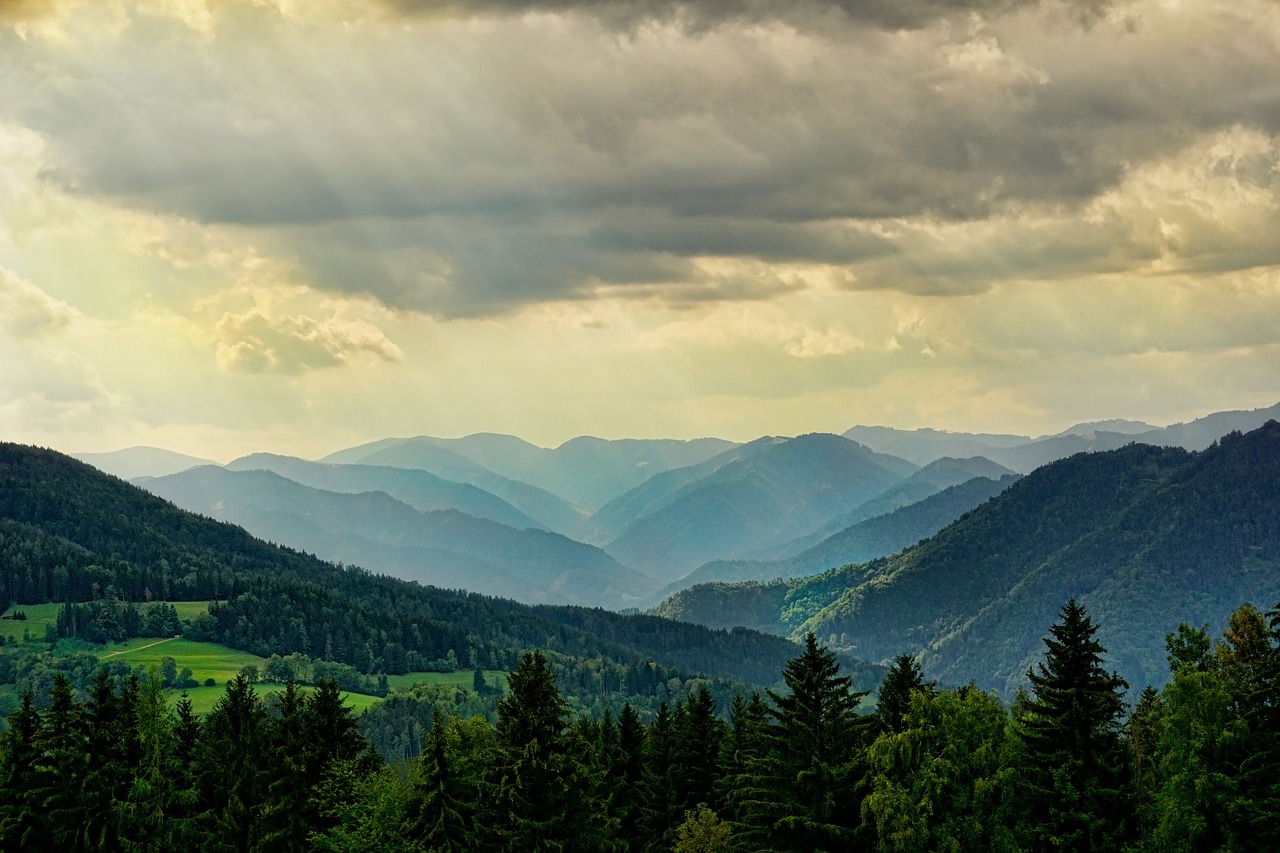
Challenges in Implementing AI
Despite the breathtaking potential of artificial intelligence (AI) in healthcare, the road to its full implementation is not without its **challenges**. One of the most pressing issues is the concern surrounding data privacy and security. With healthcare data being extremely sensitive, the integration of AI systems must ensure that patient information is protected from breaches. This is not just a technical hurdle; it's also a matter of trust. Patients need to feel confident that their data is safe, and healthcare providers must comply with stringent regulations like HIPAA in the United States.
Another significant challenge is the integration of AI with legacy systems. Many healthcare institutions still rely on outdated technology that can be difficult to upgrade or replace. The process of integrating AI solutions with these existing systems can be complex and often requires substantial investment in both time and resources. It's akin to trying to fit a square peg into a round hole; without careful planning and execution, the benefits of AI can be lost in translation.
Moreover, there is a need for regulatory frameworks that ensure the safety and efficacy of AI in healthcare. As AI technologies evolve, so too must the regulations that govern them. Regulatory bodies need to keep pace with innovations to create guidelines that not only protect patients but also encourage the adoption of AI technologies. This balancing act is crucial to foster an environment where innovation can thrive while safeguarding public health.
Additionally, there is the issue of training healthcare professionals to effectively use AI tools. Many practitioners may feel overwhelmed by new technologies or skeptical of their reliability. Providing adequate training and resources is essential for healthcare professionals to embrace AI, ensuring they understand its capabilities and limitations. After all, AI is not a replacement for human expertise; rather, it is a tool designed to enhance it.
Finally, the financial aspect cannot be ignored. Implementing AI solutions often requires significant investment, and not all healthcare providers have the budget to support such initiatives. This financial barrier can lead to disparities in access to cutting-edge technologies, particularly between large hospitals and smaller clinics. In essence, while the potential of AI to revolutionize healthcare is immense, overcoming these challenges requires a concerted effort from all stakeholders involved.
- What are the main challenges in implementing AI in healthcare? The main challenges include data privacy and security concerns, integration with legacy systems, the need for regulatory frameworks, training healthcare professionals, and financial barriers.
- How can data privacy be ensured when using AI? Ensuring data privacy involves implementing robust security measures, complying with regulations, and maintaining transparency with patients regarding how their data is used.
- What role do healthcare professionals play in AI adoption? Healthcare professionals need to be trained to effectively use AI tools, ensuring they can leverage these technologies to enhance patient care.
- Are there financial barriers to implementing AI in healthcare? Yes, the cost of implementing AI can be significant, creating disparities in access between larger hospitals and smaller clinics.
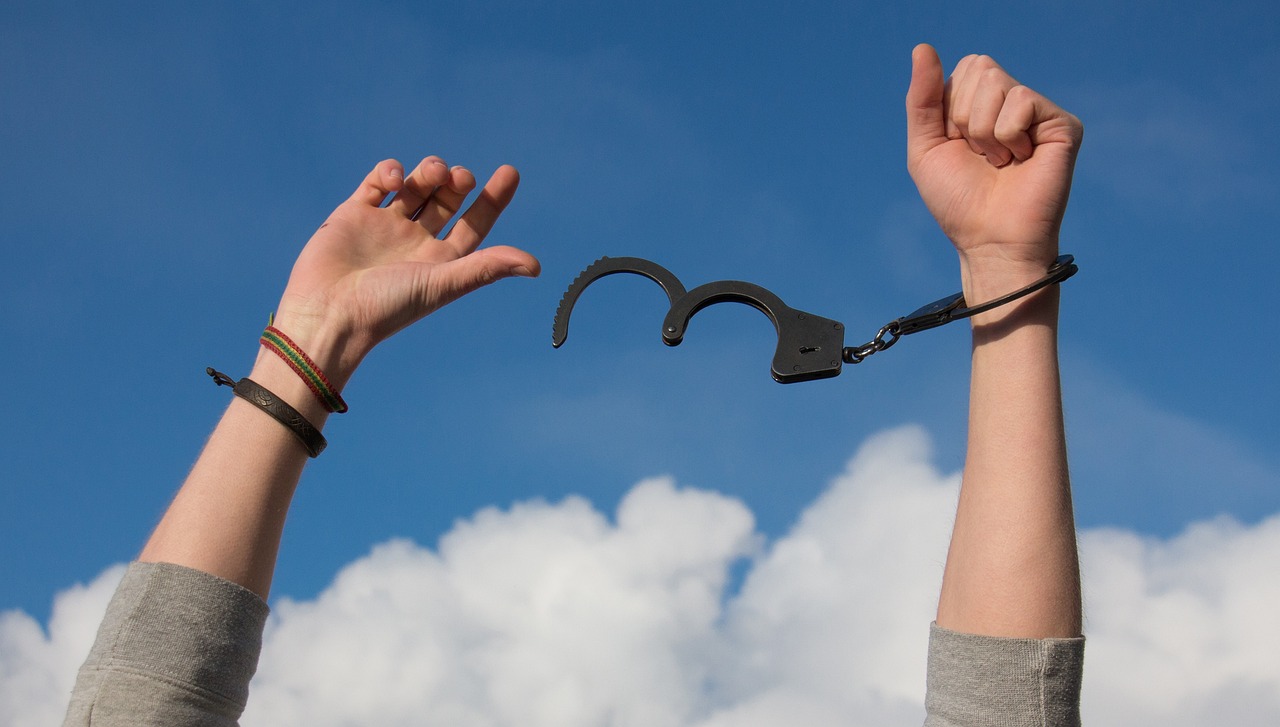
Data Privacy and Security
The integration of artificial intelligence (AI) into healthcare brings forth a myriad of opportunities, but it also raises significant concerns regarding . As healthcare providers increasingly rely on AI technologies to process sensitive patient information, the need to safeguard this data becomes paramount. Imagine your personal health records being analyzed by a machine learning algorithm to predict your future health outcomes; while this can lead to remarkable advancements in patient care, it also poses a risk if that data falls into the wrong hands.
One of the primary challenges in ensuring data privacy is the sheer volume of data that AI systems require. These systems often need access to extensive datasets, which may include personal health information, genetic data, and even lifestyle choices. To mitigate risks, healthcare organizations must implement robust security measures that comply with regulations such as HIPAA (Health Insurance Portability and Accountability Act) in the United States. This not only helps maintain patient trust but also protects organizations from potential legal repercussions.
Moreover, the use of AI in healthcare can lead to unintended consequences if data is not handled properly. For instance, if an AI algorithm is trained on biased data, it may produce skewed results that could adversely affect patient care. Therefore, it is essential to ensure that the data used for training these systems is both diverse and representative of the population. Transparency in how data is collected, stored, and utilized is crucial for fostering trust among patients and healthcare providers alike.
To address these privacy and security challenges, healthcare organizations can adopt several best practices:
- Data Encryption: Implementing strong encryption protocols to protect sensitive data both in transit and at rest.
- Access Controls: Limiting access to patient data to only those who need it for legitimate purposes.
- Regular Audits: Conducting routine audits of data access and usage to identify potential vulnerabilities.
- Employee Training: Providing ongoing training for staff on data privacy and security practices.
In conclusion, while AI holds the potential to revolutionize healthcare, it is essential to prioritize to ensure that patient information remains protected. By adopting stringent security measures and fostering a culture of transparency, healthcare organizations can harness the power of AI while maintaining the trust of the patients they serve.
1. What are the main concerns regarding AI and data privacy in healthcare?
The main concerns include the risk of unauthorized access to sensitive patient information, the potential for biased algorithms leading to unfair treatment, and the need for compliance with regulations like HIPAA.
2. How can healthcare organizations protect patient data when using AI?
Organizations can protect patient data by implementing encryption, access controls, regular audits, and employee training focused on data privacy and security.
3. Why is transparency important in AI healthcare applications?
Transparency is crucial as it builds trust between patients and healthcare providers, ensuring that patients understand how their data is being used and protected.
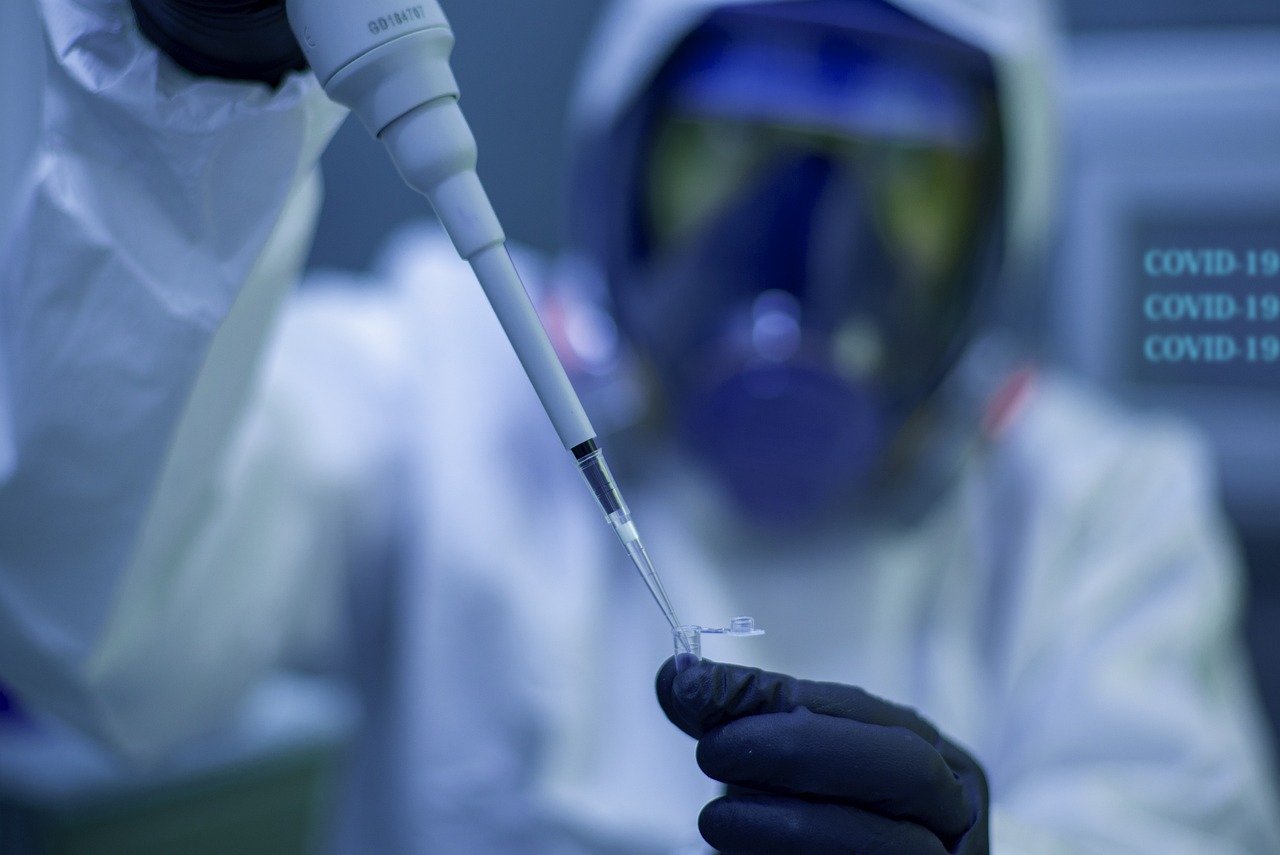
Integration with Legacy Systems
Integrating artificial intelligence (AI) solutions with existing healthcare systems is a complex yet crucial task. Many healthcare institutions are still relying on legacy systems, which were not designed to accommodate the rapid advancements in technology. This creates a significant barrier to the adoption of AI, as these older systems often lack the necessary interoperability to communicate effectively with modern AI applications.
The challenge is not just about plugging in new technology; it’s about ensuring that these systems can work together seamlessly. Imagine trying to fit a new piece of software into a puzzle where the pieces were designed decades ago. The result can be a frustrating mismatch that hinders rather than helps. The integration process requires careful planning, robust infrastructure, and a clear understanding of the data flow.
One of the primary issues healthcare providers face is the data silos created by legacy systems. These silos can lead to incomplete patient records and hinder the comprehensive analysis that AI thrives on. When AI algorithms are fed incomplete or inconsistent data, the accuracy of their predictions and recommendations can be compromised. Therefore, addressing data silos is essential for maximizing the benefits of AI in healthcare.
To facilitate successful integration, healthcare organizations can consider the following strategies:
- Conduct a thorough assessment of existing systems to identify compatibility issues.
- Invest in middleware solutions that can bridge the gap between legacy systems and new AI technologies.
- Provide training for staff to ensure they understand both the legacy systems and the new AI tools.
- Collaborate with technology partners who specialize in healthcare IT integration.
Moreover, regulatory compliance plays a significant role in this integration process. Healthcare providers must navigate a maze of regulations that govern data privacy and security. This is where a well-thought-out integration plan can make a world of difference. By ensuring that all systems comply with regulations such as HIPAA in the United States, healthcare organizations can protect patient data while benefiting from AI technologies.
In summary, while integrating AI with legacy systems presents challenges, it is not insurmountable. With the right strategies, healthcare organizations can create a cohesive environment where both legacy systems and AI solutions work in harmony, ultimately leading to improved patient care and operational efficiency.
- What are legacy systems in healthcare?
Legacy systems refer to outdated technology or software that is still in use within healthcare organizations. These systems often lack the capabilities to integrate easily with modern technologies like AI. - Why is integration with legacy systems important for AI?
Integration is crucial because it allows AI tools to access comprehensive and accurate data, which enhances their ability to provide valuable insights and recommendations for patient care. - What challenges do healthcare providers face when integrating AI?
Challenges include dealing with data silos, ensuring compliance with regulations, and addressing compatibility issues between old and new systems. - How can healthcare organizations improve integration?
Organizations can improve integration by assessing their current systems, investing in middleware solutions, providing staff training, and collaborating with IT specialists.
Frequently Asked Questions
- What is the role of AI in diagnostics?
AI plays a crucial role in diagnostics by enhancing the accuracy and speed of disease detection. Machine learning algorithms can analyze medical images and patient data, leading to earlier identification of conditions such as cancer and heart disease, ultimately saving lives.
- How does AI contribute to personalized medicine?
AI contributes to personalized medicine by analyzing genetic information and individual patient data. This allows healthcare providers to tailor treatments specifically to each patient, improving outcomes and reducing the side effects commonly associated with standard treatments.
- What are predictive analytics in patient care?
Predictive analytics involves using AI to analyze historical patient data to anticipate future health needs and outcomes. By identifying at-risk patients, healthcare providers can implement preventive measures, enhancing overall health management.
- What are risk stratification models?
AI-driven risk stratification models categorize patients based on their likelihood of developing certain health conditions. This categorization enables healthcare professionals to deliver targeted interventions, which optimizes resource allocation and improves patient care.
- How do clinical decision support systems work?
Clinical decision support systems powered by AI assist healthcare professionals by providing evidence-based recommendations during the decision-making process. These systems help reduce diagnostic errors and improve patient outcomes by ensuring that providers have access to the most relevant information.
- How is AI transforming drug discovery?
AI is transforming drug discovery by analyzing vast datasets to identify potential drug candidates more quickly and cost-effectively. This innovation helps accelerate the process of bringing new medications to market, benefiting both patients and pharmaceutical companies.
- What challenges does AI face in healthcare?
Despite its benefits, AI in healthcare faces challenges such as data privacy concerns, the complexity of integrating with existing systems, and the need for regulatory frameworks to ensure that AI technologies are safe and effective.
- How important is data privacy in AI healthcare applications?
Data privacy is critically important in AI healthcare applications. Protecting patient information while leveraging AI technologies is essential for maintaining trust and compliance with privacy regulations.
- What issues arise with integrating AI into legacy systems?
Integrating AI solutions with legacy healthcare systems can be complex due to interoperability issues. Addressing these challenges is vital for maximizing the benefits of AI and ensuring seamless workflows in clinical settings.