Predictive Analysis: Changing the Face of Business
In today’s fast-paced business environment, making decisions based on gut feelings is becoming a thing of the past. Instead, companies are turning to predictive analysis to help them navigate the complexities of the market. This powerful tool harnesses the power of data-driven insights to forecast future trends and behaviors, allowing organizations to make informed decisions that lead to growth and efficiency. Imagine being able to see into the future of your business; that’s what predictive analysis offers—a glimpse into what’s next and how to prepare for it.
So, what exactly is predictive analysis? At its core, it involves using statistical algorithms and machine learning techniques to sift through historical data. By identifying patterns and trends, businesses can predict potential outcomes with remarkable accuracy. This is not just a buzzword; it’s a revolutionary approach that is reshaping business strategies across various industries. From anticipating customer needs to optimizing supply chains, the applications are vast and impactful.
One of the most exciting aspects of predictive analysis is its ability to enhance decision-making processes. Think of it as having a crystal ball that not only shows you potential outcomes but also provides the data needed to back up those predictions. This level of insight enables businesses to act proactively rather than reactively, which is crucial in today’s competitive landscape. For instance, a retail company can use predictive analysis to forecast sales trends, allowing them to stock up on popular items before the rush, thus maximizing profits and minimizing waste.
Furthermore, predictive analysis is not limited to any one sector. It is a versatile tool that can be tailored to meet the unique needs of various industries. For example, in healthcare, it assists in managing patient care by predicting disease outbreaks and optimizing resource allocation. In finance, it helps in risk assessment and fraud detection, empowering companies to make strategic financial decisions. The potential for growth and improvement is limitless, making predictive analysis an essential part of modern business strategy.
However, it’s important to acknowledge that the journey to implementing predictive analysis is not without its challenges. Organizations must ensure that they have high-quality data, as poor data quality can lead to misleading conclusions. Additionally, integrating predictive analysis tools with existing systems can be complex and time-consuming. Nevertheless, the benefits far outweigh the hurdles, and businesses that embrace this technology stand to gain a significant competitive edge.
As we delve deeper into the applications of predictive analysis in various industries, we’ll uncover how it is revolutionizing operations and enhancing customer satisfaction. Stay tuned as we explore the transformative power of predictive analysis and its role in shaping the future of business.
- What is predictive analysis?
Predictive analysis is the use of statistical algorithms and machine learning techniques to identify the likelihood of future outcomes based on historical data. - How does predictive analysis benefit businesses?
It helps businesses make informed decisions, optimize operations, and anticipate customer needs, leading to increased efficiency and profitability. - What industries can benefit from predictive analysis?
Predictive analysis can be applied in various sectors, including healthcare, finance, retail, and more. - What challenges are associated with predictive analysis?
Challenges include ensuring data quality, integrating with existing systems, and the need for skilled personnel to interpret complex data models.
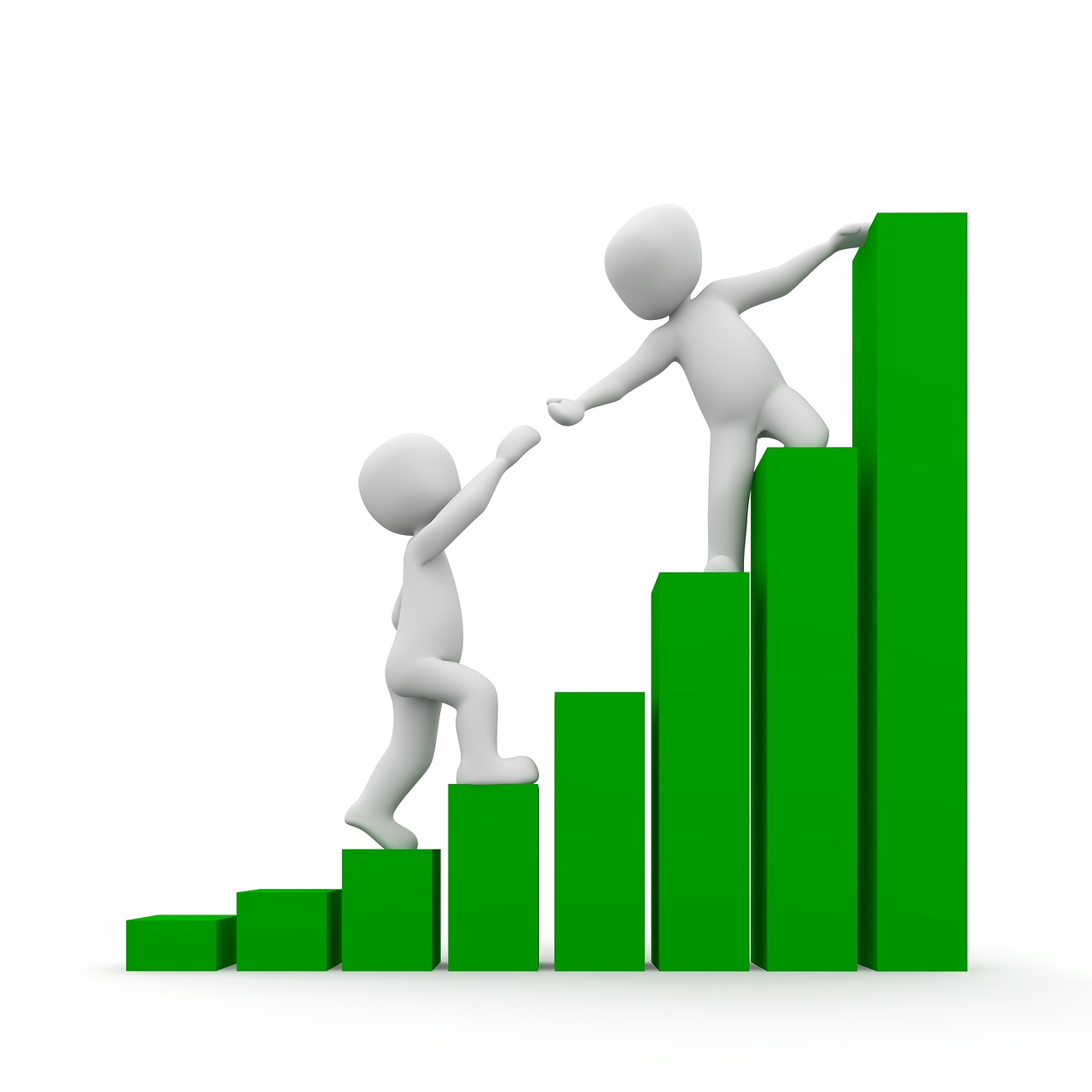
Understanding Predictive Analysis
This article explores how predictive analysis is transforming business strategies, enhancing decision-making, and driving growth through data-driven insights and forecasting techniques that anticipate future trends and behaviors.
Predictive analysis is a powerful tool that combines statistics, data mining, and machine learning to forecast future events based on historical data. Imagine you’re trying to predict the weather; you wouldn’t just look at today’s temperature, right? You’d consider past weather patterns, current atmospheric conditions, and various other factors. Similarly, businesses use predictive analysis to sift through vast amounts of data, uncovering patterns and trends that inform future decisions.
At its core, predictive analysis helps organizations to make informed decisions by identifying the likelihood of different outcomes. This process involves several steps:
- Data Collection: Gathering relevant historical data from various sources.
- Data Processing: Cleaning and organizing the data to ensure accuracy.
- Model Building: Creating statistical models to analyze the data.
- Prediction: Using these models to predict future trends or behaviors.
By leveraging these steps, businesses can gain insights that drive strategic initiatives, optimize operations, and enhance customer experiences. For instance, a retail company might analyze past sales data to predict which products will be in demand during the upcoming holiday season, allowing them to stock inventory accordingly. This not only maximizes sales but also minimizes waste.
Moreover, predictive analysis is not just about looking into the future; it’s about enhancing decision-making in the present. Organizations can identify potential risks and opportunities, enabling them to act proactively rather than reactively. For example, a bank might use predictive analysis to assess the likelihood of loan defaults, allowing them to adjust their lending criteria and mitigate financial risks.
As businesses continue to embrace data-driven strategies, the role of predictive analysis becomes increasingly vital. It empowers organizations to not only anticipate market shifts but also to adapt their strategies accordingly. In a world where change is the only constant, having the ability to foresee potential challenges and opportunities can be the difference between success and failure.
Different industries utilize predictive analysis to improve efficiency and profitability. From healthcare to finance, this technology offers tailored solutions that enhance operational performance and customer satisfaction.
In healthcare, predictive analysis helps in patient care management by forecasting disease outbreaks and optimizing resource allocation, ultimately improving patient outcomes and reducing costs.
By analyzing patient data, healthcare providers can anticipate complications and tailor treatments, leading to better health outcomes and more personalized care strategies.
Predictive models assist healthcare organizations in effectively managing resources, ensuring that staff and equipment are allocated where they are needed most, thereby increasing operational efficiency.
In the finance sector, predictive analysis is used for risk assessment, fraud detection, and investment forecasting, enabling companies to make strategic financial decisions based on data insights.
Despite its benefits, implementing predictive analysis poses challenges such as data quality, integration issues, and the need for skilled personnel to interpret complex data models.
Ensuring high-quality, reliable data is crucial for accurate predictive analysis. Poor data quality can lead to misleading conclusions and ineffective business strategies.
Integrating predictive analysis tools with existing business systems can be complex, requiring significant time and resources to ensure seamless operation and data flow.
What is predictive analysis?
Predictive analysis is a technique that uses statistical algorithms and machine learning to analyze historical data and predict future outcomes.
How can businesses benefit from predictive analysis?
Businesses can use predictive analysis to enhance decision-making, optimize operations, and improve customer satisfaction by anticipating future trends and behaviors.
What industries use predictive analysis?
Predictive analysis is applied in various industries, including healthcare, finance, retail, and marketing, to improve efficiency and profitability.
What are the challenges of implementing predictive analysis?
Challenges include ensuring data quality, integrating with existing systems, and the need for skilled personnel to interpret complex data models.
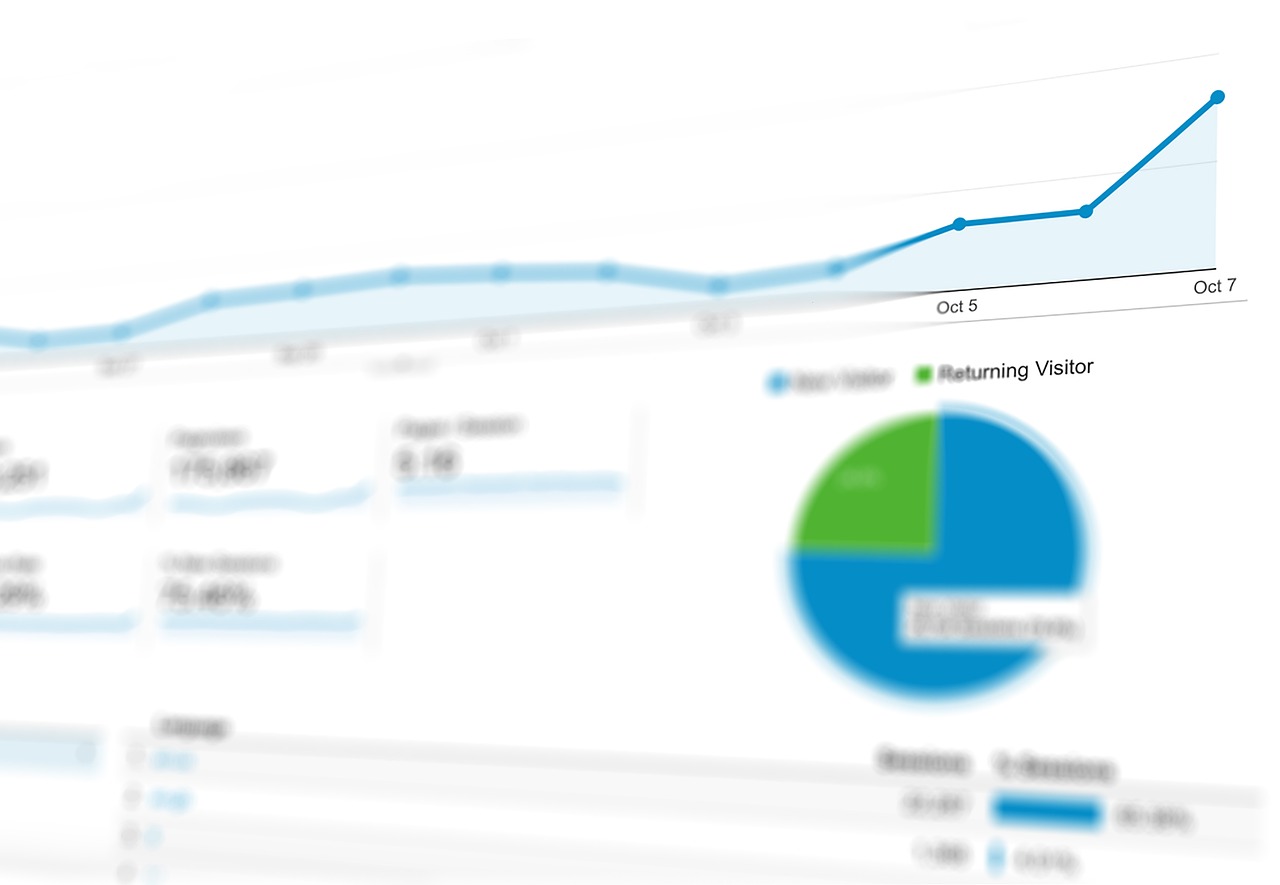
Applications in Various Industries
This article explores how predictive analysis is transforming business strategies, enhancing decision-making, and driving growth through data-driven insights and forecasting techniques that anticipate future trends and behaviors.
Predictive analysis involves using statistical algorithms and machine learning techniques to identify the likelihood of future outcomes based on historical data, helping businesses make informed decisions and optimize operations.
Predictive analysis is not just a buzzword; it's a game-changer across various industries. Imagine being able to forecast customer preferences before they even realize what they want! This technology is revolutionizing how businesses operate, driving efficiency and profitability in ways we never thought possible. Here’s how different sectors are harnessing the power of predictive analysis:
In the healthcare realm, predictive analysis is a lifesaver—literally! By leveraging vast amounts of patient data, healthcare providers can anticipate disease outbreaks, manage patient care more effectively, and allocate resources where they are needed most. This not only improves patient outcomes but also streamlines operations, ultimately reducing costs. For instance, hospitals can predict patient admission rates based on historical data, ensuring they have enough staff and resources ready to handle surges in demand.
By analyzing patient data, healthcare providers can anticipate complications before they arise. This proactive approach allows for tailored treatments that cater to individual patient needs. Imagine a world where doctors can predict a patient's risk of developing a chronic illness and intervene early—this is the power of predictive analysis in action!
Predictive models assist healthcare organizations in effectively managing resources. By forecasting patient inflow, hospitals can ensure that staff and equipment are allocated where they are needed most. This not only increases operational efficiency but also enhances the overall patient experience. A well-staffed hospital is a happy hospital, and predictive analysis is the key to making that happen.
The finance sector is another area where predictive analysis shines. From risk assessment to fraud detection, this technology enables companies to make strategic financial decisions based on data insights. For example, by analyzing transaction patterns, financial institutions can identify potentially fraudulent activities before they escalate, saving millions in losses.
Moreover, predictive analysis aids in investment forecasting. By examining market trends and historical performance, investors can make informed decisions about where to allocate their funds. This isn't just guesswork; it's a calculated approach that minimizes risks and maximizes returns.
Despite its benefits, implementing predictive analysis poses challenges such as data quality, integration issues, and the need for skilled personnel to interpret complex data models.
Ensuring high-quality, reliable data is crucial for accurate predictive analysis. Poor data quality can lead to misleading conclusions and ineffective business strategies. Businesses must invest in data cleansing and management to ensure that the insights derived from predictive analysis are valid and actionable.
Integrating predictive analysis tools with existing business systems can be complex, requiring significant time and resources to ensure seamless operation and data flow. Companies must be prepared to tackle these challenges head-on to reap the full benefits of predictive analysis.
- What is predictive analysis?
Predictive analysis is a technique that uses statistical algorithms and machine learning to predict future outcomes based on historical data.
- How is predictive analysis used in healthcare?
In healthcare, predictive analysis helps in forecasting disease outbreaks, optimizing resource allocation, and enhancing patient care.
- What are the benefits of predictive analysis in finance?
It aids in risk assessment, fraud detection, and investment forecasting, allowing companies to make informed financial decisions.
- What challenges come with implementing predictive analysis?
Challenges include ensuring data quality, integrating with existing systems, and the need for skilled personnel to interpret data.
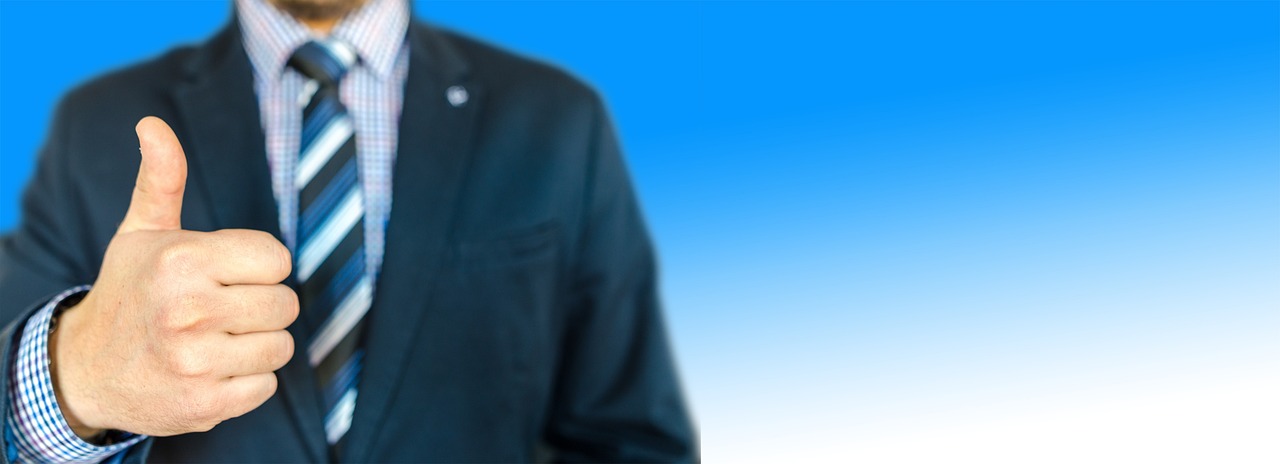
Predictive Analysis in Healthcare
In the rapidly evolving landscape of healthcare, predictive analysis is emerging as a game-changer. By leveraging vast amounts of historical data, healthcare providers can anticipate patient needs, forecast disease outbreaks, and enhance overall operational efficiency. Imagine being able to predict a flu outbreak before it happens, allowing hospitals to prepare and allocate resources effectively. This isn't science fiction; it's the power of predictive analysis at work.
One of the most significant applications of predictive analysis in healthcare is in patient care management. By analyzing data from various sources such as electronic health records, wearable devices, and population health metrics, healthcare professionals can identify patterns that might indicate potential health issues. For example, if data shows a spike in respiratory infections in a specific area, healthcare providers can take proactive measures to educate the public on preventive care and increase staffing in clinics.
Moreover, predictive models are instrumental in enhancing patient outcomes. They allow healthcare providers to tailor treatments based on individual patient data, leading to more personalized care strategies. For instance, a predictive model might analyze a patient's genetic information alongside their medical history to determine the most effective treatment plan for a chronic condition. This not only improves health outcomes but also fosters a stronger patient-provider relationship, as patients feel more understood and cared for.
Additionally, predictive analysis plays a crucial role in resource allocation. In a healthcare setting, resources such as staff, equipment, and facilities need to be managed efficiently to ensure optimal patient care. Predictive models can analyze historical data to forecast peak times for patient admissions, allowing hospitals to schedule staff accordingly and ensure that they are prepared for fluctuations in patient volume. This proactive approach helps to minimize wait times and enhances the overall patient experience.
To illustrate the impact of predictive analysis in healthcare, consider the following table that highlights key benefits:
Benefit | Description |
---|---|
Improved Patient Outcomes | Tailored treatments based on predictive data analysis. |
Efficient Resource Management | Optimized allocation of staff and equipment based on patient forecasts. |
Disease Prevention | Proactive measures to prevent outbreaks through data insights. |
In conclusion, the integration of predictive analysis in healthcare not only revolutionizes patient care but also enhances operational efficiency. By anticipating future trends and behaviors, healthcare providers can make informed decisions that ultimately lead to better health outcomes and a more responsive healthcare system. It's clear that predictive analysis is not just a tool; it's a vital component in the quest for improved healthcare delivery.
- What is predictive analysis in healthcare? Predictive analysis in healthcare refers to the use of statistical algorithms and machine learning techniques to analyze historical data and predict future outcomes, helping healthcare providers make informed decisions.
- How does predictive analysis improve patient care? It allows for personalized treatment plans by analyzing patient data, leading to better health outcomes and proactive care strategies.
- What are some challenges in implementing predictive analysis? Challenges include ensuring data quality, integrating with existing systems, and the need for skilled personnel to interpret data effectively.
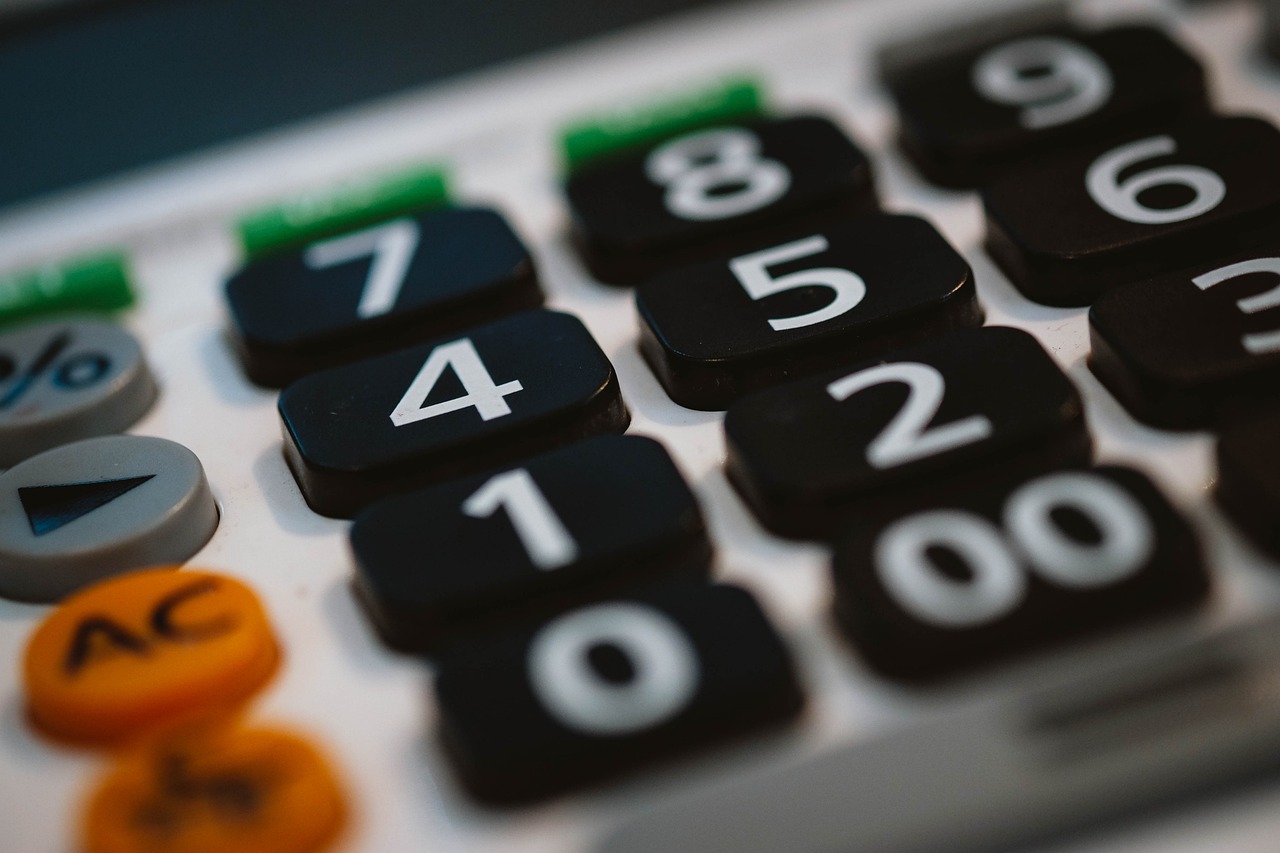
Enhancing Patient Outcomes
In the realm of healthcare, the stakes are incredibly high, and every decision can significantly impact patient lives. Predictive analysis plays a pivotal role in enhancing patient outcomes by leveraging data to foresee potential health complications before they arise. Imagine a world where doctors can anticipate health issues rather than merely reacting to them; this is the promise of predictive analytics. By analyzing historical patient data, healthcare providers can identify patterns that might indicate risks for specific patients. For instance, a patient with a history of heart disease might be flagged for closer monitoring when predictive models indicate a higher likelihood of complications.
One of the most powerful aspects of predictive analysis is its ability to facilitate personalized treatment plans. When healthcare providers can foresee potential issues, they can tailor interventions to meet individual patient needs. This means that rather than applying a one-size-fits-all approach, treatments can be adjusted based on a patient's unique risk profile. For example, if predictive models suggest that a certain demographic is at higher risk for diabetes, healthcare systems can proactively implement screening programs targeted at those individuals, thus catching the disease early and improving long-term outcomes.
Furthermore, predictive analysis enables healthcare organizations to manage resources more effectively. By forecasting patient inflow and potential complications, hospitals can ensure that they have adequate staffing and equipment available. This not only enhances operational efficiency but also ensures that patients receive timely care. For example, if predictive models indicate a surge in flu cases during the winter months, hospitals can prepare by increasing staffing levels and stocking up on necessary supplies, ultimately leading to better patient care during peak times.
To illustrate the impact of predictive analysis on patient outcomes, consider the following table that outlines the benefits:
Benefit | Description |
---|---|
Early Intervention | Identifying at-risk patients allows for timely medical interventions, reducing the severity of health issues. |
Personalized Care | Treatment plans are tailored to individual patient needs, improving the effectiveness of interventions. |
Resource Optimization | Predictive models help allocate resources efficiently, ensuring that care is delivered promptly. |
Improved Patient Satisfaction | Timely and personalized care leads to better patient experiences and outcomes. |
In summary, the integration of predictive analysis into healthcare is not just a technological advancement; it is a transformation that enhances patient outcomes significantly. By anticipating health issues and personalizing care, healthcare providers can not only improve the quality of life for their patients but also streamline operations to foster a more effective healthcare system. As we continue to harness the power of data, the future of healthcare looks promising, with predictive analysis at the forefront of this evolution.
- What is predictive analysis in healthcare? Predictive analysis in healthcare involves using data and statistical algorithms to forecast patient outcomes, enabling proactive care and personalized treatment plans.
- How does predictive analysis improve patient care? By identifying at-risk patients and anticipating potential health issues, predictive analysis allows healthcare providers to intervene early and tailor treatments to individual needs.
- What challenges exist in implementing predictive analysis? Challenges include ensuring data quality, integrating predictive tools with existing systems, and the need for skilled professionals to interpret complex data.
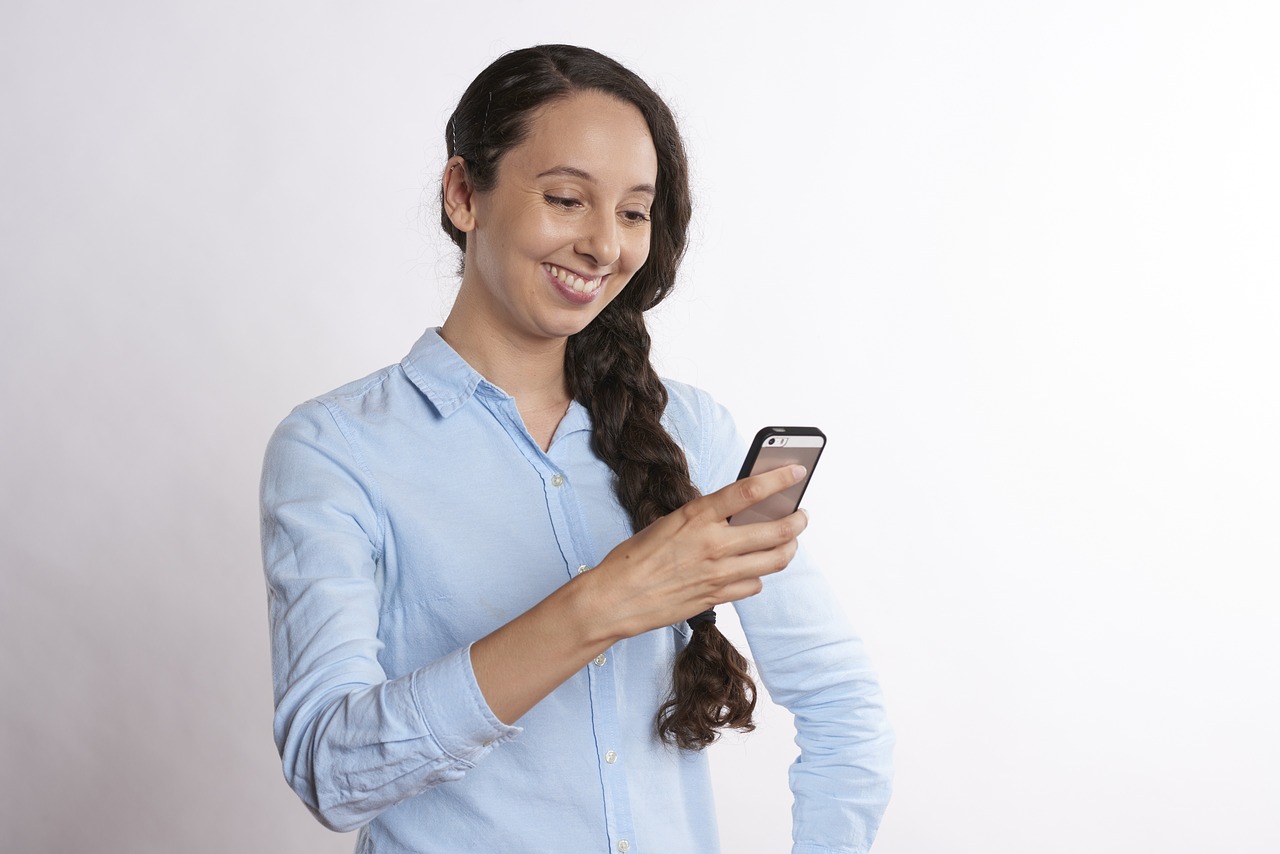
Resource Allocation
In today's fast-paced healthcare environment, is more critical than ever. Hospitals and clinics are constantly faced with the challenge of utilizing their resources—be it staff, equipment, or facilities—efficiently. Predictive analysis plays a pivotal role in this arena, allowing healthcare organizations to forecast demand and allocate resources accordingly. Imagine a hospital that can predict a surge in patient admissions due to seasonal flu outbreaks; this foresight enables them to prepare in advance, ensuring that they have enough staff on hand and that critical equipment is available when needed.
By leveraging historical data and advanced algorithms, predictive models can highlight trends and patterns that may not be immediately apparent. For instance, a healthcare provider might analyze past patient admission rates and correlate them with various factors such as weather patterns, local events, or even social media trends. This data-driven approach allows for a more proactive stance in managing resources, rather than a reactive one, which can often lead to chaos and inefficiency.
Moreover, effective resource allocation isn't just about having enough beds or staff; it also involves optimizing the use of existing resources. For example, predictive analysis can help identify which departments are likely to experience high patient volumes at certain times of the day or week. This information can be invaluable for scheduling staff shifts, ensuring that the right number of healthcare professionals is available during peak times while avoiding overstaffing during quieter periods. This not only enhances operational efficiency but also contributes to better patient care as staff can focus on providing quality service rather than being stretched thin.
To illustrate the impact of predictive analysis on resource allocation, consider the following table:
Scenario | Traditional Approach | Predictive Analysis Approach |
---|---|---|
Flu Season | Reactively hire temporary staff during peak weeks. | Predictive modeling forecasts patient influx, allowing for proactive staffing adjustments. |
Emergency Room Visits | Staffing based on historical averages. | Analyze trends to adjust staffing levels dynamically based on predicted demand. |
Resource Utilization | General allocation of equipment without analysis. | Allocate equipment based on predictive insights into patient needs and treatment plans. |
Ultimately, the integration of predictive analysis into resource allocation strategies not only enhances operational efficiency but also leads to improved patient experiences. When healthcare providers can anticipate needs and allocate resources effectively, they create an environment where patient care can thrive. This proactive approach reduces wait times, optimizes staff workloads, and ensures that patients receive the attention and care they deserve when they need it the most.
- What is predictive analysis? Predictive analysis uses historical data and statistical algorithms to forecast future outcomes, helping businesses make informed decisions.
- How does predictive analysis benefit healthcare? It improves patient outcomes, optimizes resource allocation, and enhances operational efficiency.
- What challenges does predictive analysis face? Data quality, integration with existing systems, and the need for skilled personnel to interpret data are significant challenges.
- Can predictive analysis be used in other industries? Yes, it is widely used in finance, retail, marketing, and many other sectors to improve decision-making and operational performance.
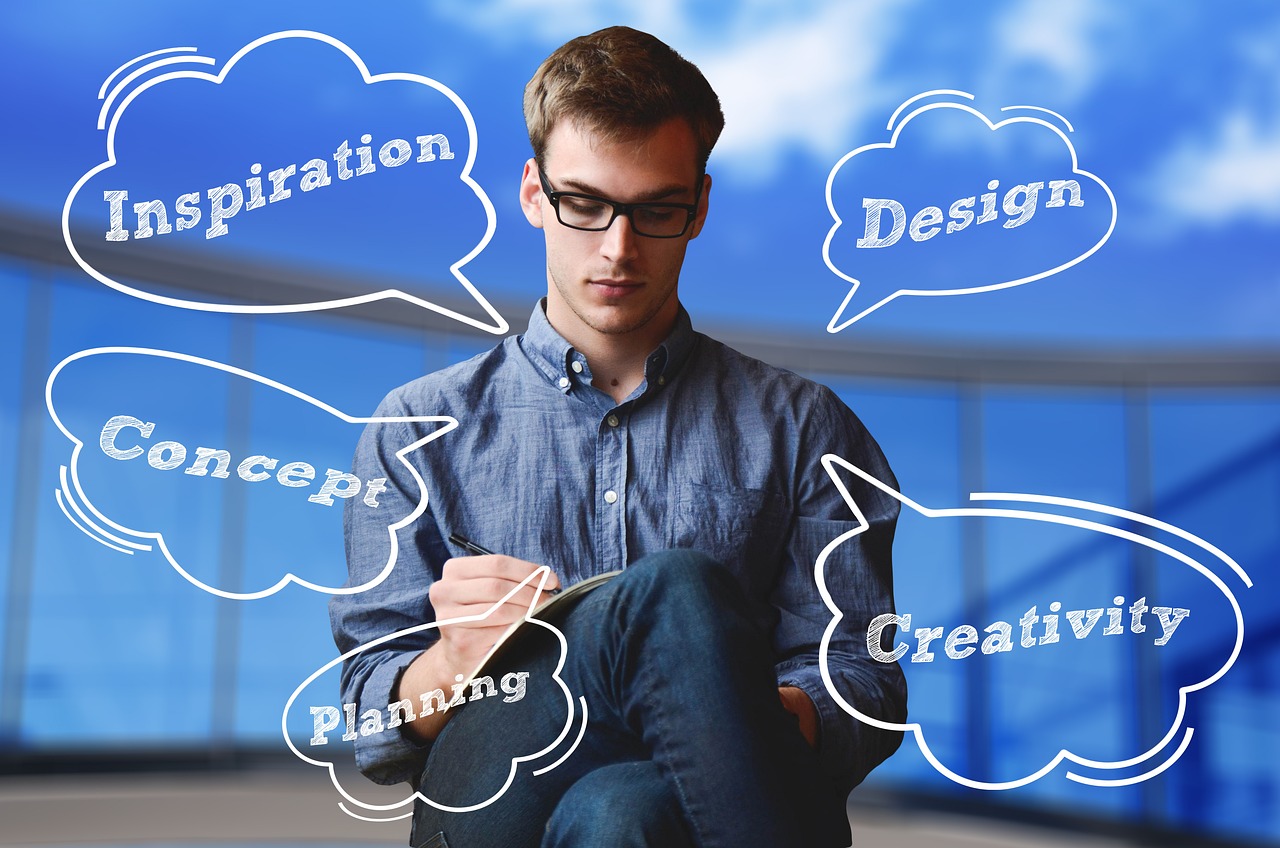
Predictive Analysis in Finance
In the ever-evolving world of finance, predictive analysis has emerged as a game-changer, allowing organizations to navigate complex financial landscapes with confidence. By leveraging historical data and advanced statistical algorithms, financial institutions can anticipate market trends and make informed decisions that significantly impact their bottom line. Imagine having the ability to foresee potential risks before they materialize or to identify lucrative investment opportunities before they become mainstream. This is the power of predictive analysis in finance.
One of the primary applications of predictive analysis in finance is risk assessment. Financial institutions utilize predictive models to evaluate the likelihood of default on loans and credit products. By analyzing various factors such as credit history, income levels, and economic indicators, banks can better understand the risk associated with lending to specific individuals or businesses. This not only helps in minimizing potential losses but also allows for the creation of tailored financial products that suit the needs of different customer segments.
Another vital area where predictive analysis shines is in fraud detection. With the rise of digital transactions, the financial sector has become increasingly vulnerable to fraudulent activities. Predictive analysis employs machine learning algorithms to detect unusual patterns and behaviors in transaction data. For instance, if a customer's spending behavior suddenly changes dramatically—say, a series of high-value purchases in a foreign country—predictive models can flag this activity for further investigation. This proactive approach not only protects the institution from financial losses but also enhances customer trust and satisfaction.
Moreover, predictive analysis plays a crucial role in investment forecasting. Investors are always on the lookout for insights that can give them an edge over the competition. By analyzing historical market data, economic indicators, and even social media sentiment, predictive models can forecast stock price movements and market trends. This enables investors to make data-driven decisions, optimizing their portfolios and maximizing returns. For example, a predictive model might suggest that a particular stock is likely to rise based on positive sentiment and historical performance, prompting investors to act swiftly.
To illustrate the impact of predictive analysis in finance, consider the following table that summarizes its key applications and benefits:
Application | Description | Benefits |
---|---|---|
Risk Assessment | Evaluating the likelihood of loan defaults. | Minimized losses, tailored financial products. |
Fraud Detection | Identifying unusual transaction patterns. | Enhanced security, customer trust. |
Investment Forecasting | Predicting stock price movements. | Optimized portfolios, maximized returns. |
In conclusion, the integration of predictive analysis in finance is not merely a trend; it is a necessity for organizations aiming to thrive in a competitive environment. By harnessing the power of data, financial institutions can make smarter decisions, mitigate risks, and ultimately drive profitability. As technology continues to advance, the potential for predictive analysis to transform the finance sector will only grow, paving the way for innovative solutions that enhance both operational efficiency and customer experiences.
- What is predictive analysis? Predictive analysis is a statistical technique that uses historical data to forecast future outcomes.
- How is predictive analysis used in finance? It is used for risk assessment, fraud detection, and investment forecasting.
- What are the benefits of predictive analysis? Benefits include improved decision-making, enhanced efficiency, and reduced risks.
- Can predictive analysis be applied to other industries? Yes, predictive analysis is widely used in various sectors, including healthcare, retail, and marketing.
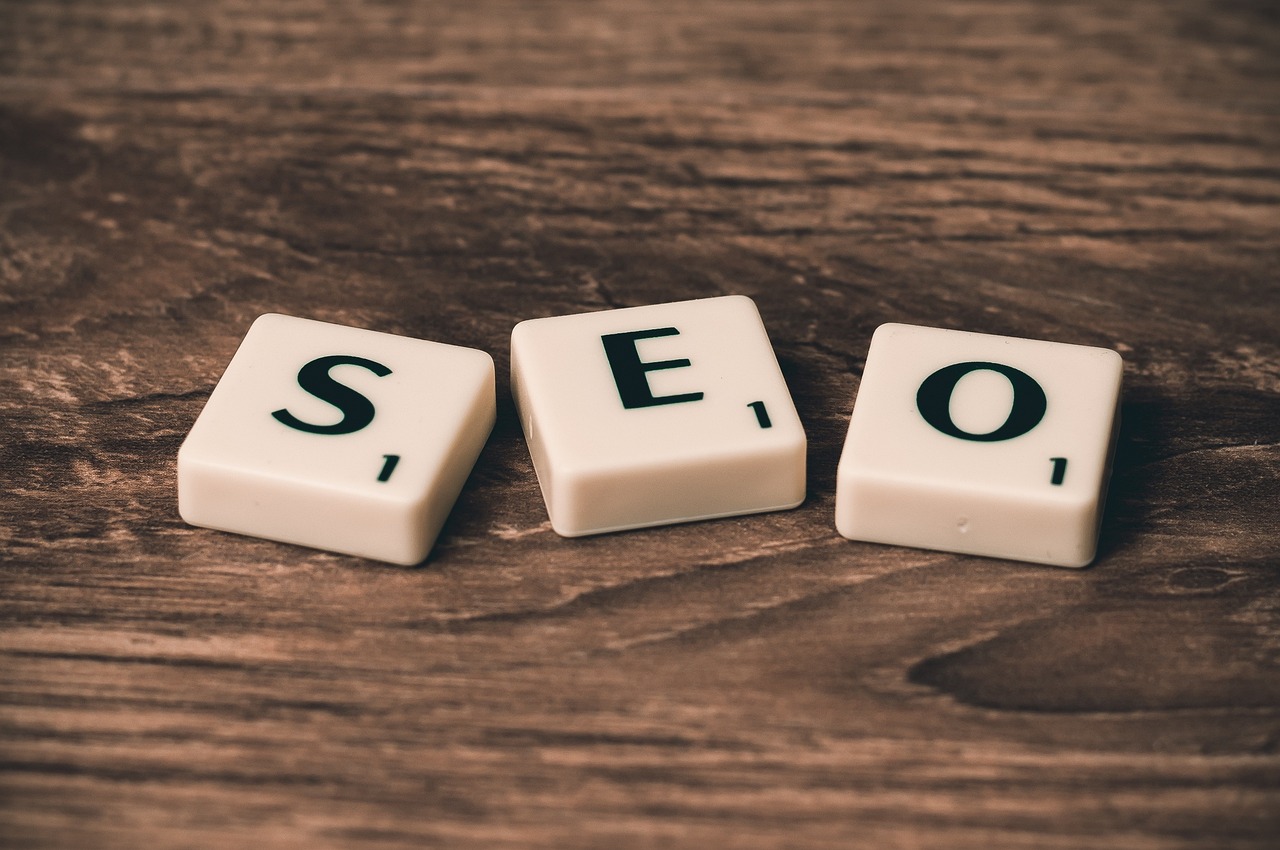
Challenges in Implementing Predictive Analysis
While the benefits of predictive analysis are clear, the road to implementation is often riddled with challenges that can hinder progress. One of the primary obstacles is data quality. Without high-quality, reliable data, the insights derived from predictive models can be misleading at best, and at worst, detrimental to business strategies. Imagine trying to predict the weather with faulty instruments; the results would be chaotic and unreliable, much like using poor data for predictive analysis.
Another significant hurdle is the integration with existing systems. Businesses often have legacy systems that are not designed to work with advanced predictive tools. This can lead to a complex web of integration issues that require not just time, but also substantial financial investment. Companies need to ensure that their data flows seamlessly between systems to leverage predictive analysis effectively. The complexity of this integration can be compared to trying to fit a square peg into a round hole; without the right adjustments, it simply won’t work.
Moreover, there’s the pressing need for skilled personnel who can interpret the complex data models and transform them into actionable insights. This is not just about having data scientists on board; it’s about fostering a culture where data-driven decision-making is valued and understood across all levels of the organization. Companies may find themselves in a situation where they have the technology but lack the human resources to utilize it effectively.
In addition to these challenges, organizations must also consider the cost implications. Implementing predictive analysis tools can require significant upfront investment in technology and training. Businesses need to weigh these costs against the potential benefits, which can sometimes feel like a gamble. However, the long-term advantages, such as improved efficiency and enhanced decision-making, often outweigh the initial expenditures.
To summarize, the challenges in implementing predictive analysis can be categorized into several key areas:
- Data Quality: Ensuring reliable data is crucial for accurate predictions.
- Integration Issues: Legacy systems may complicate the integration of new tools.
- Skilled Personnel: A lack of qualified personnel can hinder effective utilization.
- Cost Implications: Initial investments can be significant, requiring careful consideration.
Addressing these challenges requires a strategic approach, one that not only focuses on the technology but also on the people and processes involved. By investing in quality data, ensuring seamless integration, fostering a skilled workforce, and carefully evaluating costs, businesses can navigate the complexities of predictive analysis and unlock its full potential.
- What is predictive analysis?
Predictive analysis involves using statistical algorithms and machine learning techniques to forecast future outcomes based on historical data. - How can predictive analysis benefit my business?
It can enhance decision-making, improve operational efficiency, and drive growth by providing valuable data-driven insights. - What industries use predictive analysis?
Industries such as healthcare, finance, retail, and marketing utilize predictive analysis to optimize their operations and enhance customer satisfaction. - What are the main challenges of implementing predictive analysis?
The main challenges include data quality, integration with existing systems, the need for skilled personnel, and cost implications.
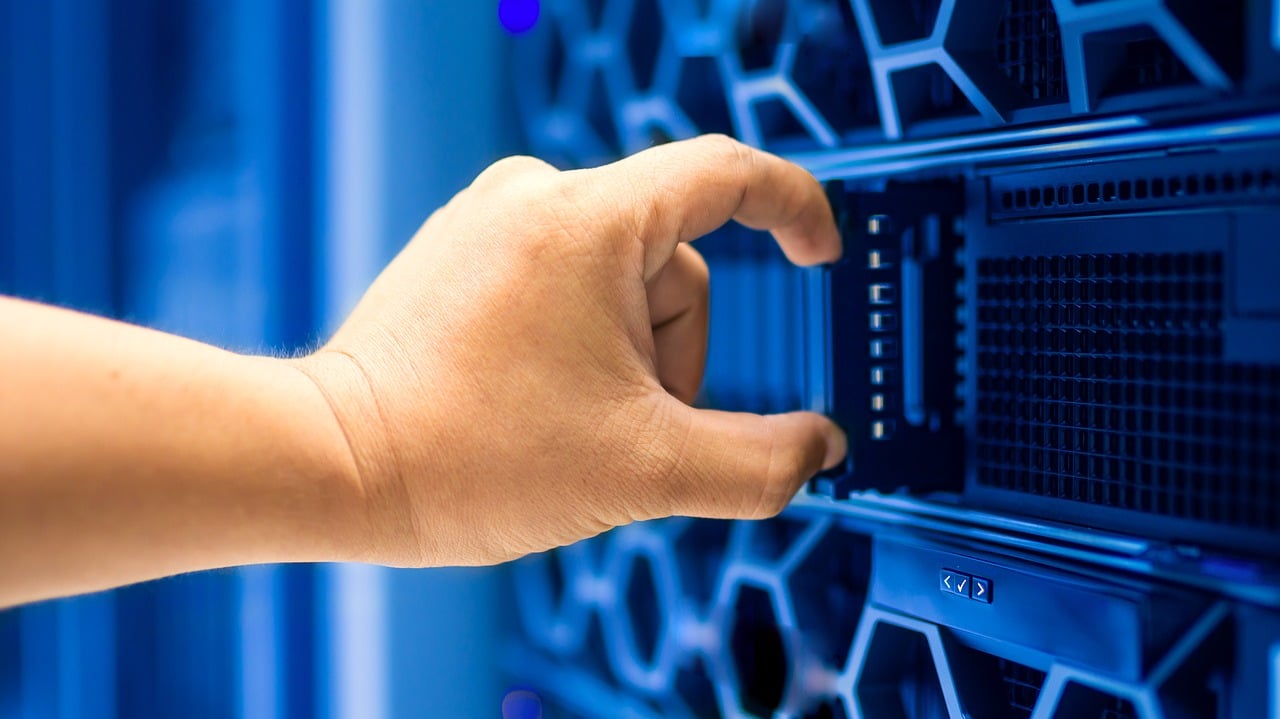
Data Quality and Integrity
When it comes to predictive analysis, the phrase “garbage in, garbage out” couldn't be more accurate. The quality and integrity of data serve as the foundation upon which all predictive insights are built. If the data fed into predictive models is flawed, the outcomes will be equally unreliable. This is a significant challenge for many businesses, as they often deal with vast amounts of data collected from various sources.
Imagine trying to navigate a ship through fog without a reliable compass. That's what businesses face when they rely on poor-quality data for predictive analysis. A lack of accuracy can lead to misguided strategies, wasted resources, and missed opportunities. Therefore, ensuring that data is not only accurate but also consistent and complete is crucial for effective predictive analysis.
Data quality can be assessed through several key dimensions, including:
- Accuracy: Are the data values correct and reliable?
- Completeness: Is all the necessary data available?
- Consistency: Are there discrepancies in data across different sources?
- Timeliness: Is the data current and relevant for decision-making?
To tackle these challenges, organizations need to invest in robust data management practices. This includes implementing data cleansing processes to remove inaccuracies, standardizing data formats to ensure consistency, and regularly updating data to maintain its relevance. Furthermore, employing advanced technologies, such as machine learning algorithms, can assist in identifying anomalies and ensuring data integrity.
Moreover, organizations should foster a culture of data governance, where every team member understands the importance of data quality and their role in maintaining it. Training programs can be instrumental in educating employees about best practices in data entry and management.
In summary, the integrity of data is paramount for successful predictive analysis. By focusing on improving data quality, businesses can enhance their predictive capabilities, leading to more informed decision-making and ultimately, better outcomes. Investing time and resources into data quality not only mitigates risks but also maximizes the potential of predictive analysis to drive growth.
- What is predictive analysis? Predictive analysis involves using statistical algorithms and machine learning techniques to forecast future outcomes based on historical data.
- Why is data quality important in predictive analysis? High-quality data ensures accurate predictions and effective business strategies, while poor data can lead to misleading conclusions.
- How can businesses improve data quality? Businesses can improve data quality by implementing data cleansing processes, standardizing formats, and fostering a culture of data governance.
- What are the common challenges in implementing predictive analysis? Challenges include data quality issues, integration with existing systems, and the need for skilled personnel to interpret complex data models.
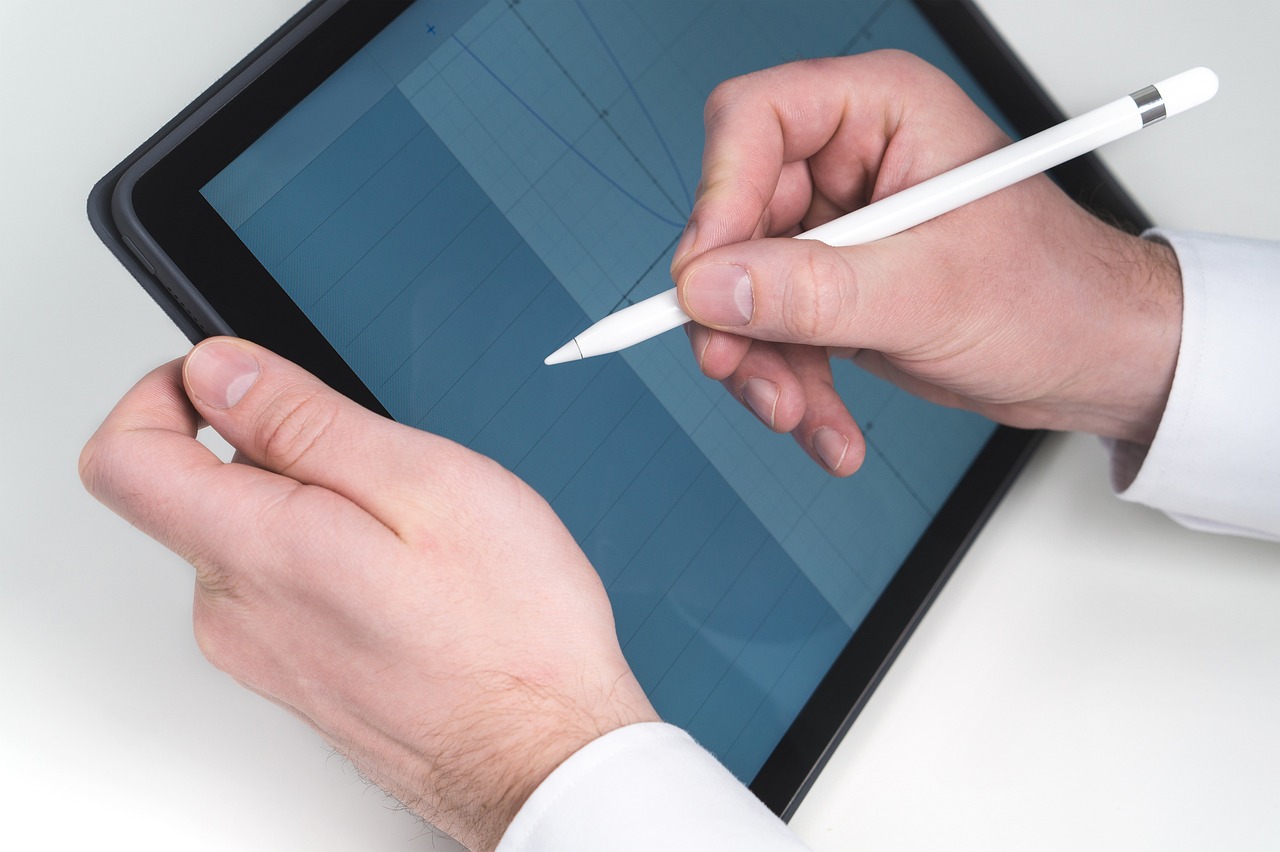
Integration with Existing Systems
Integrating predictive analysis tools with existing business systems can feel like trying to fit a square peg in a round hole. It’s not just about slapping a new tool on top of your current processes; it’s about ensuring that everything works together seamlessly. Businesses often face a myriad of challenges during this integration phase, and understanding these hurdles is crucial for a successful transition.
One of the primary challenges is the data silos that many organizations operate with. Different departments may use varied systems that don’t communicate well with each other. When predictive analysis tools are introduced, they often require access to data from multiple sources. If these sources are isolated, it can lead to incomplete datasets, which ultimately hampers the effectiveness of predictive insights. Think of it like trying to assemble a puzzle without all the pieces; you might get a partial picture, but it's unlikely to be complete or accurate.
Moreover, training and skill gaps present significant obstacles. Many organizations lack personnel who are proficient in both the technical aspects of predictive analysis and the specific nuances of their existing systems. This gap can lead to underutilization of the predictive tools, as employees may struggle to interpret the data or apply it effectively in their decision-making processes. To mitigate this, companies should consider investing in training programs that empower their staff with the necessary skills to leverage these tools fully.
Another critical factor is the cost of integration. Integrating new systems often requires a substantial financial investment, not only in technology but also in time and human resources. Businesses must assess whether the potential benefits of predictive analysis justify these costs. A well-planned integration strategy can help minimize expenses and maximize return on investment. Here’s a simple breakdown of potential costs associated with integration:
Cost Category | Estimated Cost |
---|---|
Software Licensing | $5,000 - $50,000 |
Consultation Services | $1,000 - $20,000 |
Training Programs | $500 - $10,000 |
Maintenance and Support | $2,000 - $15,000 annually |
Lastly, change management plays a vital role in the successful integration of predictive analysis tools. Employees may resist changes to their workflows, fearing that new tools could disrupt their established routines. To counter this, organizations should foster a culture of openness and communication, highlighting the benefits of predictive analysis in enhancing their daily tasks. By involving employees in the integration process and addressing their concerns, businesses can create a more receptive environment for these transformative technologies.
In summary, while the integration of predictive analysis tools with existing systems presents several challenges, understanding these hurdles can pave the way for a smoother transition. By focusing on data connectivity, investing in training, managing costs effectively, and embracing change management, businesses can unlock the full potential of predictive analysis and drive their growth forward.
- What is predictive analysis? Predictive analysis is the practice of using historical data and statistical algorithms to forecast future outcomes.
- How can predictive analysis benefit my business? It can enhance decision-making, improve operational efficiency, and drive growth by providing data-driven insights.
- What industries benefit most from predictive analysis? Industries such as healthcare, finance, retail, and manufacturing utilize predictive analysis to optimize their operations and improve customer satisfaction.
- What are the main challenges in implementing predictive analysis? Key challenges include data quality issues, integration with existing systems, and the need for skilled personnel.
Frequently Asked Questions
- What is predictive analysis?
Predictive analysis is a technique that uses statistical algorithms and machine learning to analyze historical data and predict future outcomes. It helps businesses understand potential trends and make informed decisions based on data-driven insights.
- How does predictive analysis benefit businesses?
By leveraging predictive analysis, businesses can enhance their decision-making processes, optimize operations, and drive growth. It allows companies to anticipate customer behaviors, manage resources efficiently, and improve overall performance.
- In which industries is predictive analysis commonly used?
Predictive analysis is utilized across various industries including healthcare, finance, retail, and manufacturing. Each sector applies this technology to improve efficiency, profitability, and customer satisfaction tailored to their specific needs.
- Can predictive analysis improve patient outcomes in healthcare?
Absolutely! In healthcare, predictive analysis can forecast disease outbreaks and help tailor treatments by analyzing patient data. This leads to better health outcomes and more personalized care strategies, ultimately enhancing patient satisfaction.
- What are the challenges of implementing predictive analysis?
Implementing predictive analysis can be challenging due to factors like data quality, integration with existing systems, and the need for skilled personnel to interpret complex data models. Addressing these challenges is crucial for successful implementation.
- How important is data quality in predictive analysis?
Data quality is paramount in predictive analysis. High-quality, reliable data ensures accurate predictions. Poor data can lead to misleading conclusions, which can negatively impact business strategies and outcomes.
- What role does predictive analysis play in finance?
In finance, predictive analysis is essential for risk assessment, fraud detection, and investment forecasting. It enables financial institutions to make strategic decisions based on comprehensive data insights, ultimately enhancing their performance.
- Is it difficult to integrate predictive analysis tools with existing systems?
Yes, integrating predictive analysis tools with existing business systems can be complex. It often requires significant time and resources to ensure a seamless operation and effective data flow, but the long-term benefits can outweigh these initial challenges.