AI in Neurology: Understanding the Impact on Brain Disorders
In recent years, artificial intelligence (AI) has emerged as a groundbreaking force in the field of neurology, fundamentally changing how we diagnose and treat brain disorders. Imagine a world where neurological conditions like Alzheimer's, Parkinson's, and multiple sclerosis are detected at their earliest stages, allowing for timely interventions that can significantly improve patient outcomes. This is not just a dream; it's becoming a reality thanks to the rapid advancements in AI technologies. But how exactly is AI reshaping neurology, and what does this mean for the future of healthcare? Let's dive deeper into this fascinating intersection of technology and medicine.
The integration of AI into neurology is akin to giving neurologists a powerful new set of tools that can enhance their clinical capabilities. By leveraging advanced imaging techniques and sophisticated machine learning algorithms, healthcare professionals are now able to make more accurate diagnoses in a fraction of the time it used to take. For instance, traditional methods of diagnosing brain disorders often involve lengthy assessments and numerous tests. In contrast, AI can sift through vast amounts of data quickly, identifying patterns and anomalies that might be missed by the human eye. This not only speeds up the diagnostic process but also reduces the chances of misdiagnosis, which can have serious implications for patient care.
Consider the case of brain imaging. With AI, radiologists can utilize algorithms that analyze MRI and CT scans to detect subtle changes in brain structure that could indicate the onset of a neurological disorder. This capability is particularly crucial for conditions that require early intervention. The sooner a disorder is identified, the better the chances of successful treatment. In this context, AI acts as a second pair of eyes—one that never tires and can constantly learn from new data. As we continue to develop these technologies, the potential for improving diagnostic accuracy becomes even more promising.
Furthermore, the role of AI extends beyond just diagnosis; it also plays a significant part in treatment personalization. By employing machine learning algorithms, healthcare providers can analyze individual patient data to tailor treatment plans that are more effective. This personalized approach is a game-changer, especially for patients with complex neurological conditions that respond differently to various therapies. Imagine being able to predict which treatment will work best for a specific patient based on their unique genetic makeup and medical history. This level of customization not only enhances treatment efficacy but also minimizes the trial-and-error approach that often accompanies neurological care.
As we look toward the future, the implications of AI in neurology are boundless. It's not just about enhancing current practices; it's about redefining what is possible in brain disorder management. However, with great power comes great responsibility. As AI technologies continue to evolve, we must also address the ethical considerations that accompany them. Issues such as data privacy, algorithmic bias, and the potential for AI to make life-altering decisions in healthcare must be carefully navigated. Ensuring that these technologies are used responsibly is critical to maintaining trust in healthcare systems and safeguarding patient welfare.
In summary, the impact of AI on neurology is profound and transformative. It promises to enhance diagnostic accuracy, personalize treatment plans, and ultimately improve patient outcomes. Yet, as we embrace these advancements, we must remain vigilant about the ethical implications and ensure that the technology is used in a way that upholds the highest standards of care. The future of neurology is bright, and with AI at the helm, we are on the brink of significant breakthroughs that could change the landscape of brain disorder management forever.
- What is AI in neurology? AI in neurology refers to the use of artificial intelligence technologies to improve the diagnosis, treatment, and management of brain disorders.
- How does AI improve diagnosis in neurology? AI enhances diagnosis by analyzing medical data, such as imaging scans, to identify patterns that may indicate neurological conditions more accurately and quickly than traditional methods.
- Can AI personalize treatment plans for neurological patients? Yes, AI can analyze individual patient data to tailor treatment plans, improving the likelihood of positive outcomes based on the patient's specific needs.
- What ethical concerns are associated with AI in healthcare? Ethical concerns include patient data privacy, algorithmic bias, and the implications of AI decision-making in clinical settings.
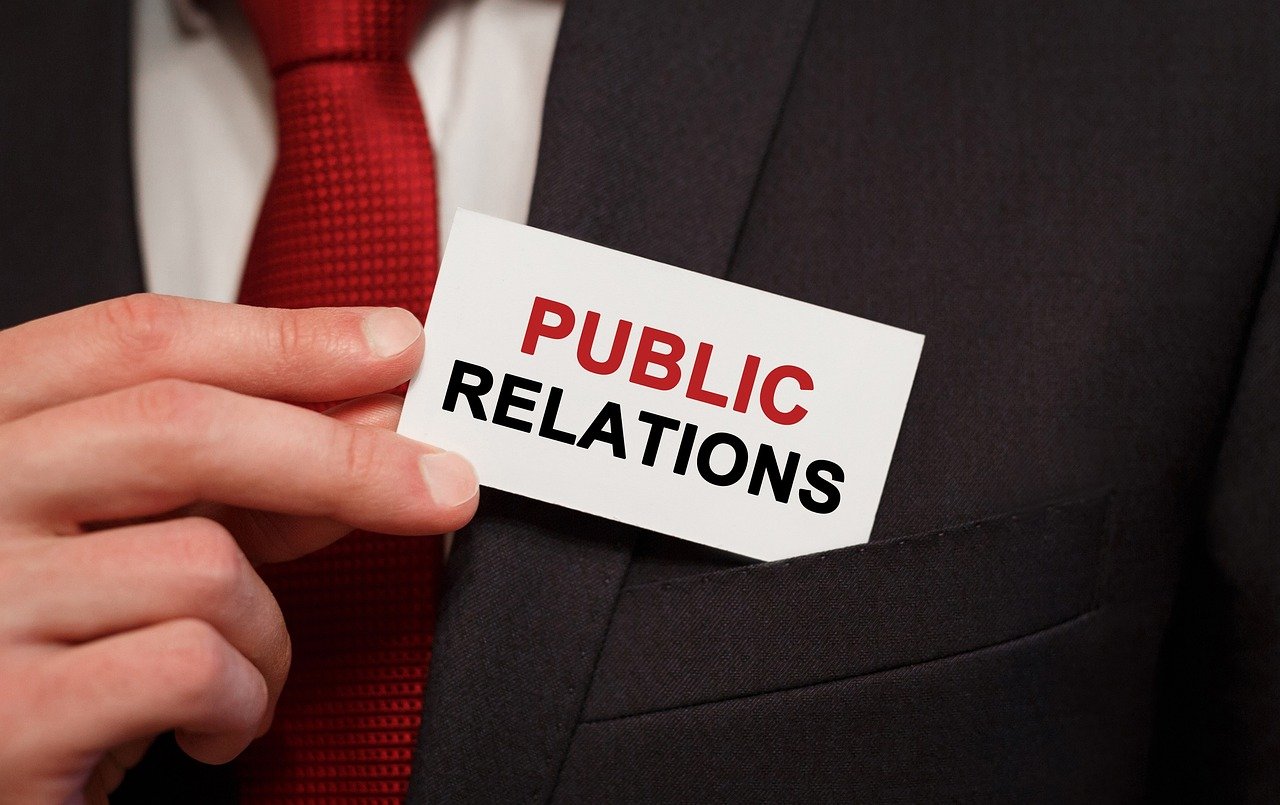
AI Applications in Diagnosis
Artificial intelligence is revolutionizing the diagnostic process in neurology, enabling faster and more accurate identification of brain disorders through advanced imaging techniques and machine learning algorithms. Imagine a world where a patient walks into a clinic, and within minutes, their brain scan is analyzed by an AI system that can detect anomalies with precision that even the most experienced neurologist might miss. This is not just a futuristic dream; it is happening right now. AI technologies, powered by deep learning and neural networks, are transforming how we perceive and treat neurological conditions.
One of the most exciting applications of AI in diagnosis is in medical imaging. Techniques such as MRI and CT scans generate vast amounts of data, and analyzing these images manually can be time-consuming and prone to human error. AI algorithms, however, can process these images rapidly, identifying patterns and potential issues that may indicate conditions like Alzheimer's, Parkinson's, or multiple sclerosis. For instance, a recent study found that AI could detect early signs of Alzheimer's with an accuracy rate of over 90%, significantly outperforming traditional methods.
Furthermore, AI systems are being developed to assist in electroencephalogram (EEG) interpretation. EEGs are crucial for diagnosing epilepsy and other neurological disorders, but interpreting the data can be complex. AI can help by analyzing the brain's electrical activity, detecting irregular patterns, and even predicting seizure occurrences. This not only aids in timely diagnosis but also helps in monitoring the effectiveness of ongoing treatments.
Additionally, AI applications extend beyond imaging. Natural Language Processing (NLP) is another area where AI is making waves. By analyzing patient histories, clinical notes, and even patient conversations, NLP algorithms can extract vital information that might be overlooked during a routine examination. This technology helps in creating a more comprehensive picture of a patient’s health, leading to more accurate diagnoses.
Moreover, the integration of AI in diagnostic processes is not just about speed; it’s also about enhancing the accuracy of diagnoses. Traditional diagnostic methods often rely on subjective interpretations and can vary from one clinician to another. AI, with its data-driven approach, offers a level of consistency that is hard to achieve in human assessments. This consistency is particularly important in neurology, where the stakes are high, and the consequences of misdiagnosis can be severe.
In summary, the application of AI in the diagnostic realm of neurology is a game-changer. It combines speed, accuracy, and the ability to analyze vast amounts of data to improve patient outcomes. As we continue to embrace these advanced technologies, we can look forward to a future where diagnosing brain disorders is not only faster but also more reliable, ultimately leading to better treatment plans and improved quality of life for patients.
- How does AI improve the accuracy of brain disorder diagnoses? AI analyzes large datasets and identifies patterns that may not be visible to human practitioners, leading to more accurate results.
- Can AI replace neurologists in diagnosing brain disorders? While AI can significantly aid in diagnosis, it is not a replacement for neurologists. Instead, it serves as a powerful tool to enhance their capabilities.
- Are there any risks associated with using AI in medical diagnostics? Yes, there are concerns regarding data privacy, algorithmic bias, and the need for thorough validation of AI systems before widespread implementation.
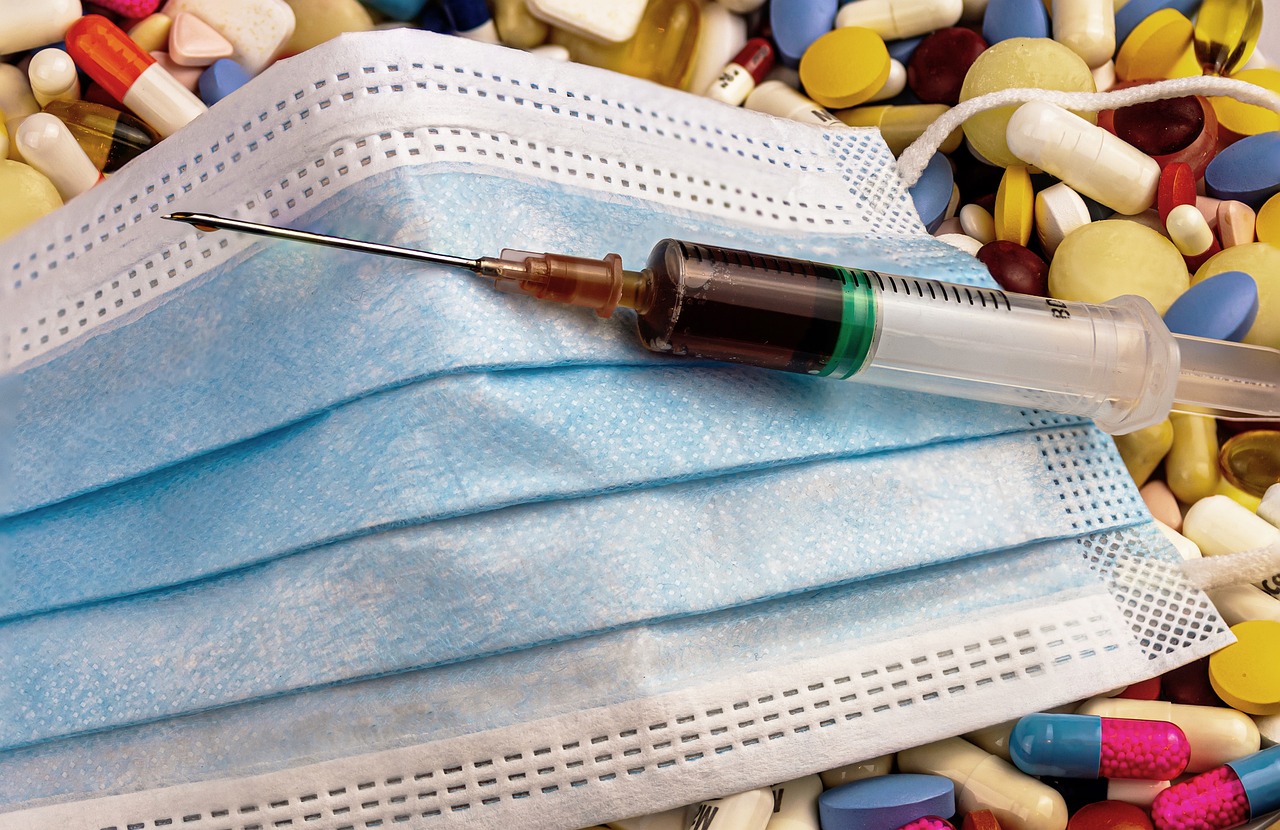
Machine Learning in Treatment
Machine learning is not just a buzzword; it’s a transformative force in the treatment of neurological conditions. Imagine walking into a clinic and knowing that your treatment plan is tailored specifically for you, based on a sophisticated analysis of your unique health data. This is the reality that machine learning is creating in neurology, where algorithms analyze vast amounts of patient information to predict how individuals will respond to various therapies. By leveraging data from countless cases, machine learning can identify patterns that may not be obvious to even the most experienced neurologists.
One of the most exciting aspects of machine learning in treatment is its ability to personalize patient care. Instead of a one-size-fits-all approach, machine learning models can suggest the most effective treatment options based on a patient’s specific characteristics, such as genetic markers, medical history, and even lifestyle factors. For instance, a patient with epilepsy might benefit from a different medication than another patient with the same condition, depending on their individual response to previous treatments. This tailored approach not only enhances the likelihood of successful outcomes but also minimizes the trial-and-error process that often accompanies neurological treatments.
Furthermore, machine learning algorithms can continuously learn and improve over time. As more data becomes available, these systems can refine their predictions, leading to even better treatment recommendations. This adaptability is crucial in the field of neurology, where new research and treatment modalities are constantly emerging. For example, recent studies have shown that machine learning can predict the likelihood of a patient responding to a specific drug based on their previous reactions. This predictive capability is a game-changer, allowing neurologists to make informed decisions that can significantly impact patient care.
To illustrate the impact of machine learning in treatment, consider the following table that summarizes some of the key benefits:
Benefit | Description |
---|---|
Personalization | Treatment plans are tailored to individual patient needs, improving outcomes. |
Predictive Analytics | Algorithms forecast responses to treatments, enhancing decision-making. |
Continuous Improvement | Machine learning systems adapt and refine recommendations as new data is available. |
Efficiency | Reduces the time spent on trial-and-error in finding effective treatments. |
However, it’s not just about the technology; the human element remains crucial. Neurologists play a vital role in interpreting the data provided by machine learning systems. They have the expertise to assess whether the suggested treatment aligns with the patient’s overall health strategy. This collaboration between AI and healthcare professionals is where the magic happens, combining the computational power of machines with the empathy and judgement of human practitioners.
In conclusion, machine learning is revolutionizing treatment in neurology by offering personalized, data-driven insights that enhance patient care. As we continue to embrace these advancements, we can expect to see even more innovative solutions that will improve the lives of those affected by neurological disorders.
- What is machine learning in neurology? Machine learning in neurology refers to the use of algorithms to analyze patient data, allowing for personalized treatment plans and improved outcomes.
- How does machine learning improve treatment? It enhances treatment by predicting patient responses to therapies based on individual characteristics and past data.
- Are there risks associated with machine learning in treatment? Yes, risks include potential algorithmic bias and the need for strict data privacy measures.
- What role do neurologists play in machine learning? Neurologists interpret machine learning data and integrate it into patient care, ensuring a balanced approach to treatment.
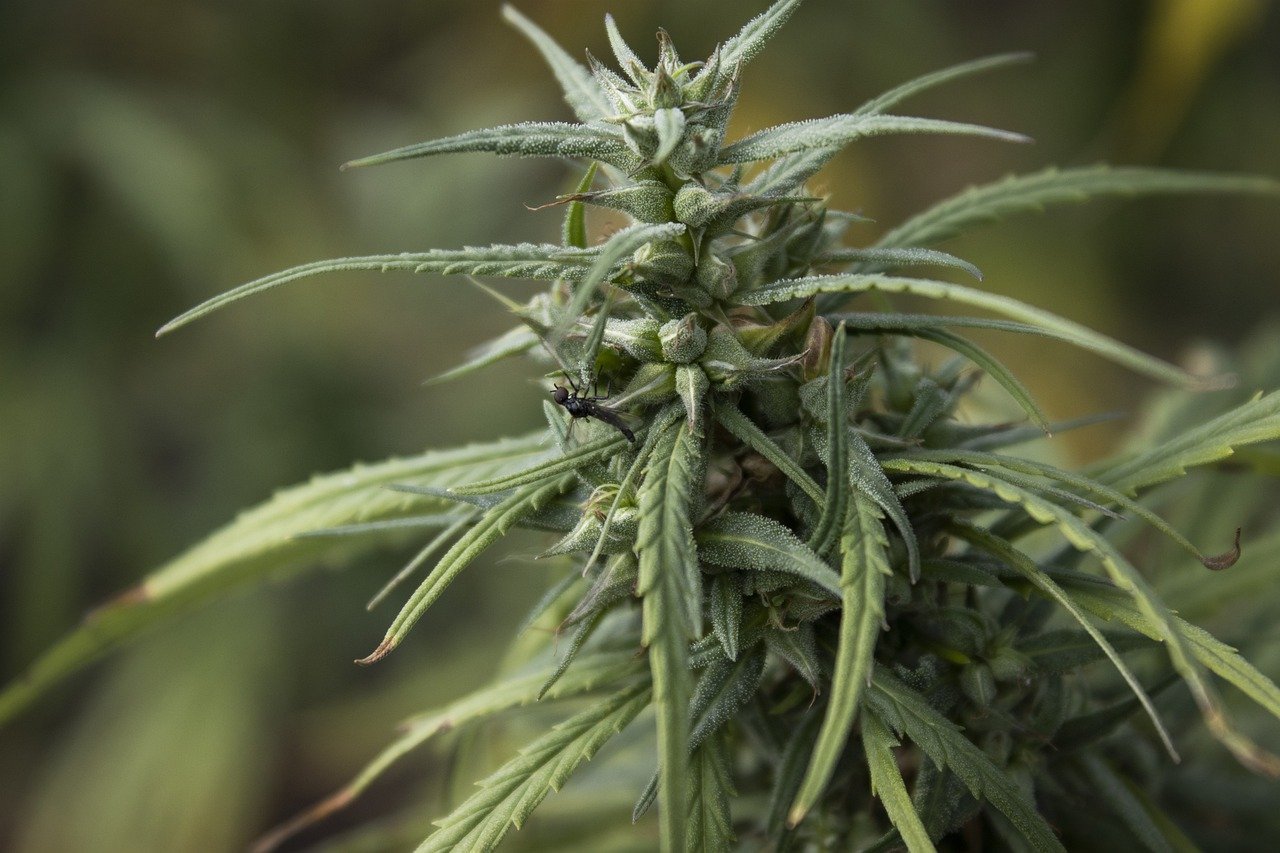
Predictive Analytics for Patient Outcomes
In the ever-evolving landscape of neurology, predictive analytics stands out as a game-changer, offering neurologists a powerful tool to forecast patient outcomes with remarkable precision. But what exactly does this mean for patients and their treatment journeys? Imagine having the ability to predict how a patient will respond to a specific therapy before they even begin treatment. This is not just a dream; it’s becoming a reality thanks to the integration of artificial intelligence and machine learning in healthcare.
At its core, predictive analytics utilizes vast amounts of data—ranging from patient histories to genetic information—to identify patterns and trends that can influence treatment outcomes. By analyzing this data, neurologists can make informed decisions that are tailored to each patient’s unique circumstances. For instance, if a patient has a history of certain neurological disorders, the algorithm can suggest the most effective treatment options based on similar cases. This personalized approach not only enhances the effectiveness of treatments but also minimizes the risk of adverse effects.
Moreover, predictive analytics can also play a crucial role in risk stratification. By categorizing patients into different risk groups, healthcare providers can prioritize interventions for those at higher risk of complications. This proactive approach is akin to having a weather forecast that warns you of an impending storm, allowing you to take necessary precautions ahead of time. For example, if data indicates that a patient is at a higher risk of developing severe symptoms, neurologists can implement early interventions that could potentially alter the disease's trajectory.
To illustrate the impact of predictive analytics, consider the following table showcasing the potential benefits:
Benefits of Predictive Analytics | Description |
---|---|
Improved Treatment Outcomes | Personalized treatment plans based on predictive models lead to higher success rates. |
Early Intervention | Identifying high-risk patients allows for timely preventive measures. |
Resource Optimization | Efficient allocation of healthcare resources by focusing on patients who need them most. |
As we delve deeper into the world of predictive analytics, it’s essential to recognize that while the technology is promising, it’s not without its challenges. Data quality and integration remain significant hurdles. Inaccurate or incomplete data can lead to misleading predictions, which could adversely affect patient care. Therefore, ongoing efforts to enhance data collection methods and ensure comprehensive datasets are crucial for the success of predictive analytics in neurology.
In conclusion, predictive analytics is set to redefine how neurologists approach patient care. By leveraging data-driven insights, healthcare providers can enhance their decision-making processes, ultimately leading to better outcomes for patients with neurological disorders. As we continue to explore the potential of AI in neurology, the future looks brighter than ever for those seeking effective and personalized treatment options.
- What is predictive analytics in neurology? Predictive analytics involves using data analysis to forecast patient outcomes and tailor treatment plans based on individual risk factors.
- How does predictive analytics improve patient care? It allows for personalized treatment plans, early interventions, and better resource allocation, enhancing overall patient outcomes.
- What challenges does predictive analytics face? Data quality and integration issues can lead to inaccurate predictions, which is why robust data collection methods are essential.
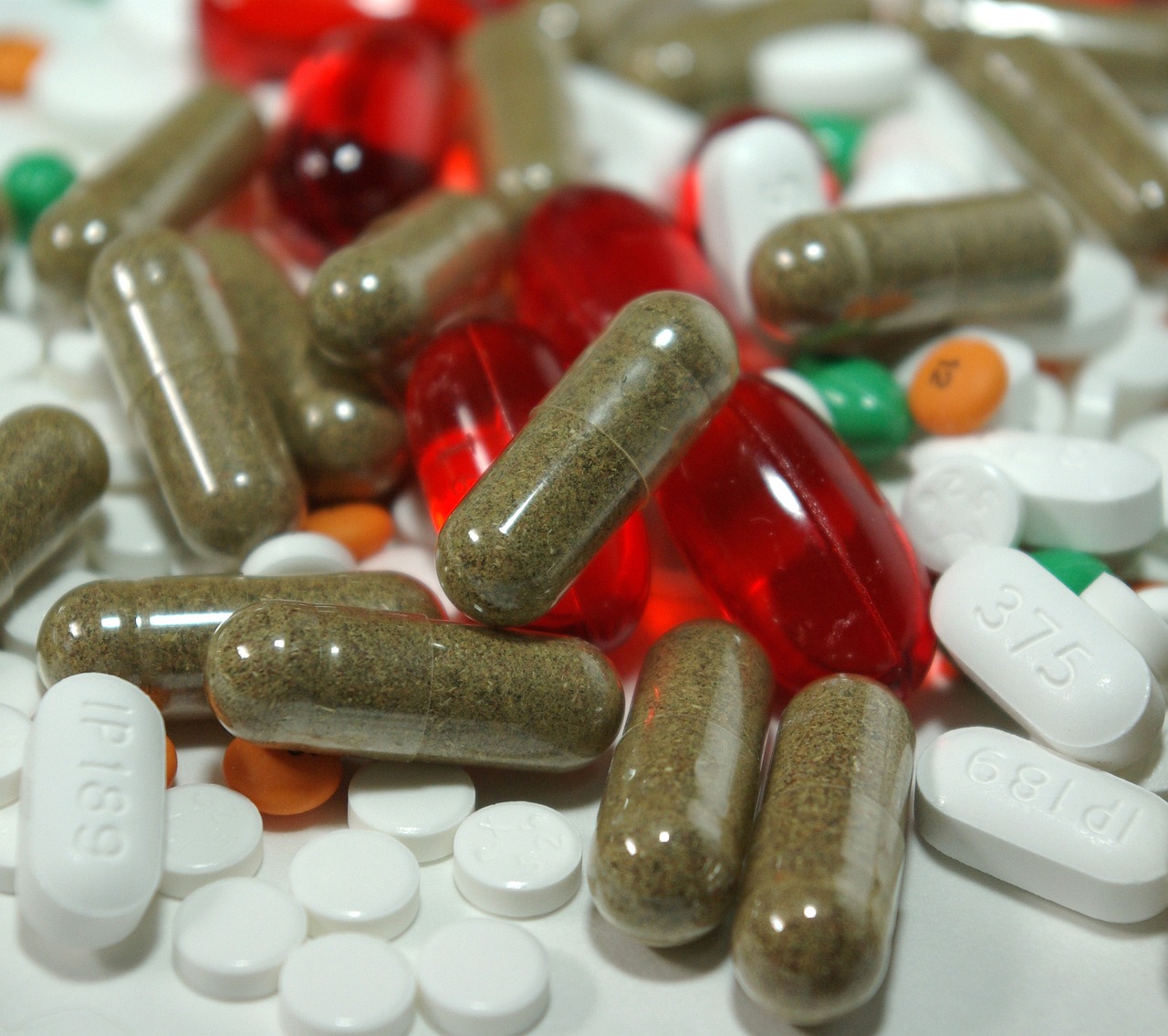
Risk Assessment Models
In the realm of neurology, AI-driven risk assessment models are emerging as game-changers, providing neurologists with the tools they need to identify patients at high risk for developing severe neurological disorders. Imagine having a crystal ball that can predict the future of your health; that's essentially what these models aim to do. By analyzing various data points, including genetic information, lifestyle choices, and medical history, these models can forecast potential health issues before they manifest. This proactive approach is akin to having a smoke detector in your home—it alerts you to potential dangers before they become a full-blown crisis.
These models utilize sophisticated algorithms to sift through vast amounts of data, identifying patterns that might escape the naked eye. For instance, a patient's family history of neurological disorders combined with their current health metrics can signal a higher risk for conditions such as Alzheimer's or Parkinson's disease. The beauty of this technology lies in its ability to tailor interventions specifically designed for at-risk individuals. By pinpointing who might need early intervention, healthcare providers can implement strategies that not only improve patient outcomes but also significantly enhance the quality of life.
Furthermore, the incorporation of machine learning within these risk assessment models allows for continuous learning and adaptation. As more data is collected, the algorithms refine their predictions, becoming increasingly accurate over time. This dynamic nature of AI means that the models are not static; they evolve with new research findings and emerging health trends. In a field as complex as neurology, where every patient's journey is unique, this adaptability is crucial.
To illustrate the effectiveness of these models, consider the following table that summarizes some key factors involved in risk assessment for neurological disorders:
Risk Factor | Impact Level | Potential Intervention |
---|---|---|
Family History | High | Regular screenings and genetic counseling |
Age | Medium | Preventive lifestyle changes |
Chronic Conditions (e.g., diabetes) | High | Integrated care and monitoring |
Environmental Factors | Medium | Education on risk reduction |
Ultimately, the goal of these risk assessment models is to shift the focus from reactive treatment to proactive management of neurological health. By identifying at-risk patients early on, neurologists can implement preventive measures that not only aim to delay or prevent the onset of disorders but also empower patients with knowledge about their health. This approach fosters a collaborative relationship between patients and healthcare providers, creating a shared responsibility for health outcomes.
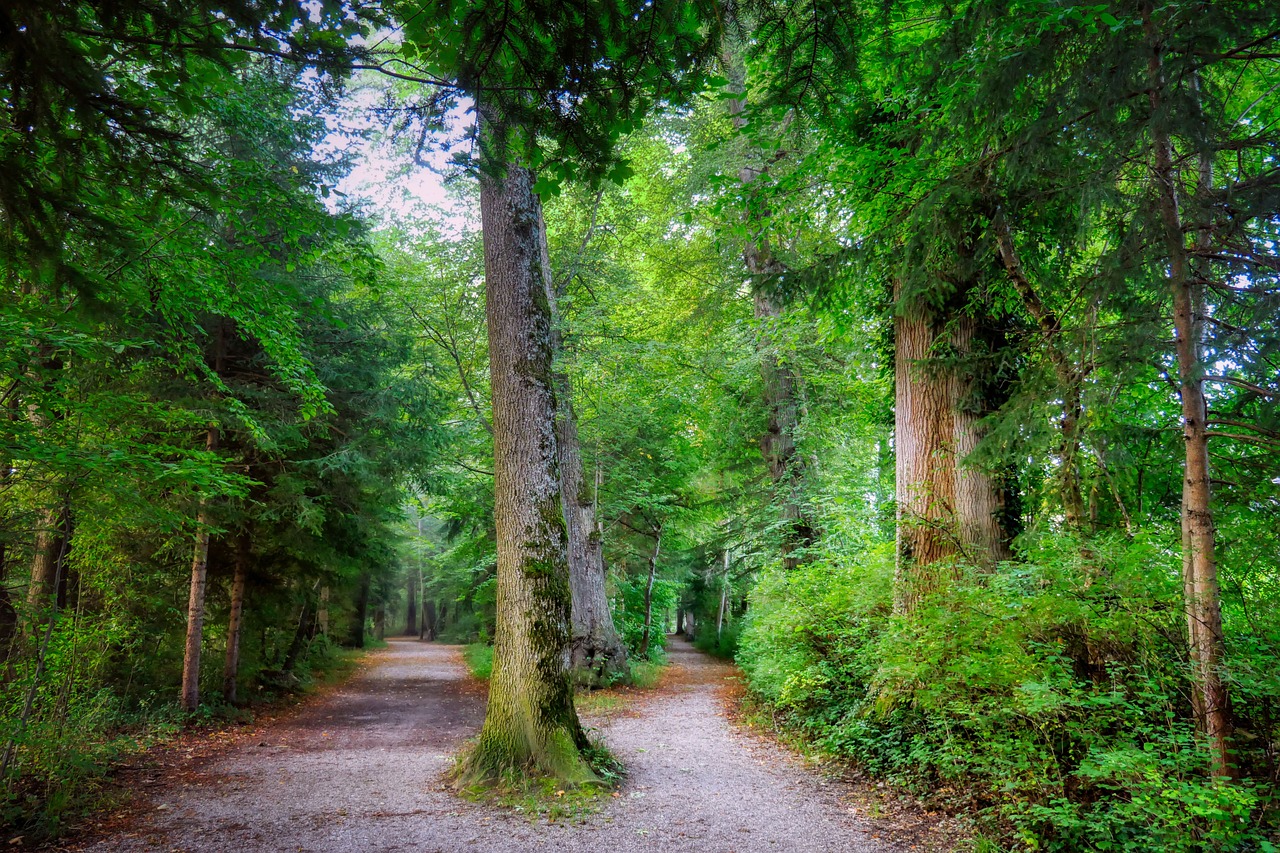
Data-Driven Insights
In the ever-evolving landscape of neurology, are becoming the cornerstone of effective diagnosis and treatment strategies. The integration of artificial intelligence into neurological research allows specialists to sift through vast amounts of data, uncovering patterns and trends that were previously hidden from view. Imagine trying to find a needle in a haystack; AI is like a magnet that not only finds the needle but also shows you where more needles might be hiding. This capability is particularly crucial in understanding the complexities of brain disorders, where every data point can make a significant difference.
By analyzing large datasets from various sources—such as electronic health records, imaging studies, and genetic information—AI can provide neurologists with valuable insights into disease progression and treatment efficacy. For instance, machine learning algorithms can identify which patients are more likely to respond positively to specific treatments based on their unique biological markers. This level of personalization is a game-changer, allowing for tailored therapies that maximize effectiveness while minimizing side effects.
Moreover, the insights derived from data analysis can lead to the discovery of new biomarkers for early diagnosis. Neurologists can use these biomarkers to detect conditions like Alzheimer’s or Parkinson’s disease at much earlier stages than was previously possible. Early detection is critical, as it opens up a window for intervention that can significantly alter the course of these debilitating diseases.
To illustrate the impact of data-driven insights, consider the following table that outlines the benefits of using AI in analyzing neurological data:
Benefit | Description |
---|---|
Enhanced Diagnosis | AI algorithms analyze imaging data to identify abnormalities that may be missed by the human eye. |
Personalized Treatment | Machine learning predicts patient responses to therapies based on historical data, improving treatment outcomes. |
Early Detection | AI identifies new biomarkers, allowing for earlier diagnosis of neurological disorders. |
Improved Research | Data analytics accelerates the discovery of new treatments and understanding of disease mechanisms. |
As we move forward, the role of AI in providing data-driven insights will only expand. Neurologists will be equipped with the tools to not only treat conditions more effectively but also to engage in proactive healthcare management. This shift from reactive to proactive care is akin to switching from a fire-fighting approach to fire prevention—it's all about anticipating issues before they escalate. In this way, AI is not just a tool; it is a partner in the quest for better brain health.
- What is the role of AI in neurology? AI assists in diagnosing and treating neurological disorders through data analysis and predictive modeling.
- How does machine learning improve treatment plans? Machine learning analyzes individual patient data to tailor treatment plans based on predicted responses.
- What are the ethical concerns regarding AI in healthcare? Issues include patient data privacy, algorithmic bias, and the impact of AI decision-making on patient care.
- Can AI help in early detection of brain disorders? Yes, AI can identify biomarkers that allow for earlier diagnosis of conditions like Alzheimer's and Parkinson's disease.
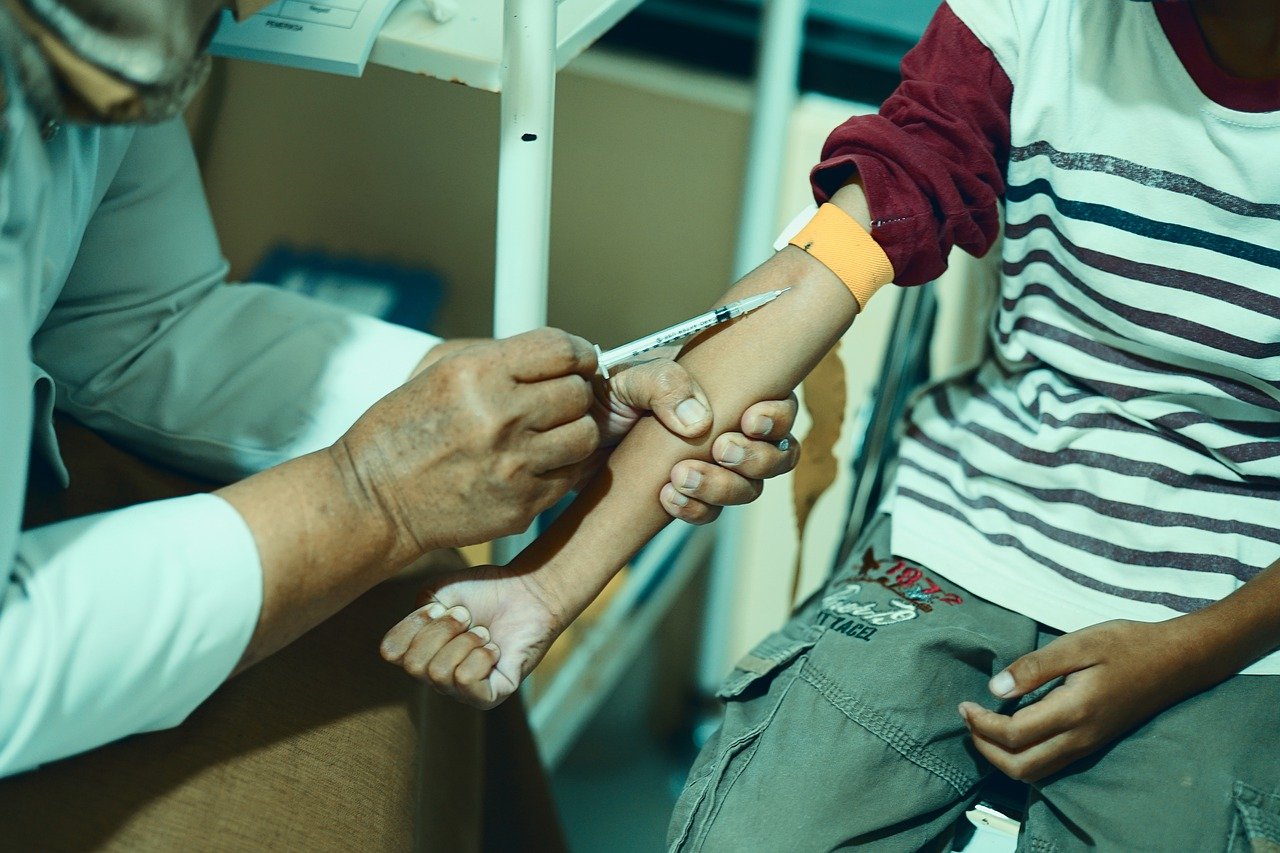
Clinical Trials and AI
Artificial Intelligence (AI) is not just a buzzword; it’s a game changer in the realm of clinical trials, particularly in the field of neurology. Imagine a world where the arduous process of recruiting patients, analyzing data, and interpreting results is streamlined through cutting-edge technology. That's precisely what AI brings to the table! By leveraging sophisticated algorithms, AI can identify suitable candidates for clinical trials more efficiently than traditional methods. This means that researchers can recruit participants who meet specific criteria quickly, ensuring that trials are not only faster but also more representative of diverse populations.
One of the most exciting aspects of AI in clinical trials is its ability to analyze vast amounts of data in real-time. Think of it as having a super-intelligent assistant that can sift through mountains of information to find patterns and insights that might take human researchers months or even years to uncover. This capability allows researchers to make data-driven decisions that enhance the quality and reliability of the trials. For instance, AI can help in identifying early indicators of success or failure in drug efficacy, enabling timely modifications to trial protocols.
Moreover, AI can significantly enhance patient monitoring during clinical trials. With the advent of wearable technology and mobile health applications, AI can continuously collect data on patients' vital signs and symptoms. This real-time monitoring allows neurologists to adjust treatment regimens promptly, ensuring that patients receive the most effective care throughout the trial. It’s akin to having a personal health coach who keeps track of your progress, offering insights and adjustments as needed.
However, the integration of AI in clinical trials is not without its challenges. Ethical considerations, such as data privacy and the potential for algorithmic bias, must be addressed to ensure that the benefits of AI are realized without compromising patient safety or fairness. Researchers must establish robust frameworks to protect patient information while harnessing the power of AI. This includes implementing stringent data encryption methods and ensuring transparency in how AI algorithms are developed and utilized.
In conclusion, the intersection of AI and clinical trials in neurology represents a promising frontier in medical research. By improving patient recruitment, enhancing data analysis, and facilitating real-time monitoring, AI is paving the way for more efficient and effective clinical trials. As we continue to explore these advancements, the potential for breakthroughs in neurological treatments becomes even more attainable, ultimately leading to better health outcomes for patients worldwide.
- How does AI improve patient recruitment for clinical trials?
AI analyzes patient databases to identify individuals who meet specific trial criteria, streamlining the recruitment process. - What role does AI play in data analysis during clinical trials?
AI can process large datasets quickly, identifying patterns and insights that aid in making informed decisions about trial protocols. - Are there ethical concerns associated with using AI in clinical trials?
Yes, issues such as data privacy and algorithmic bias need to be addressed to ensure responsible use of AI technology. - Can AI enhance patient monitoring during clinical trials?
Absolutely! AI can utilize wearable technology to monitor patients' health metrics in real-time, allowing for timely adjustments to treatment.
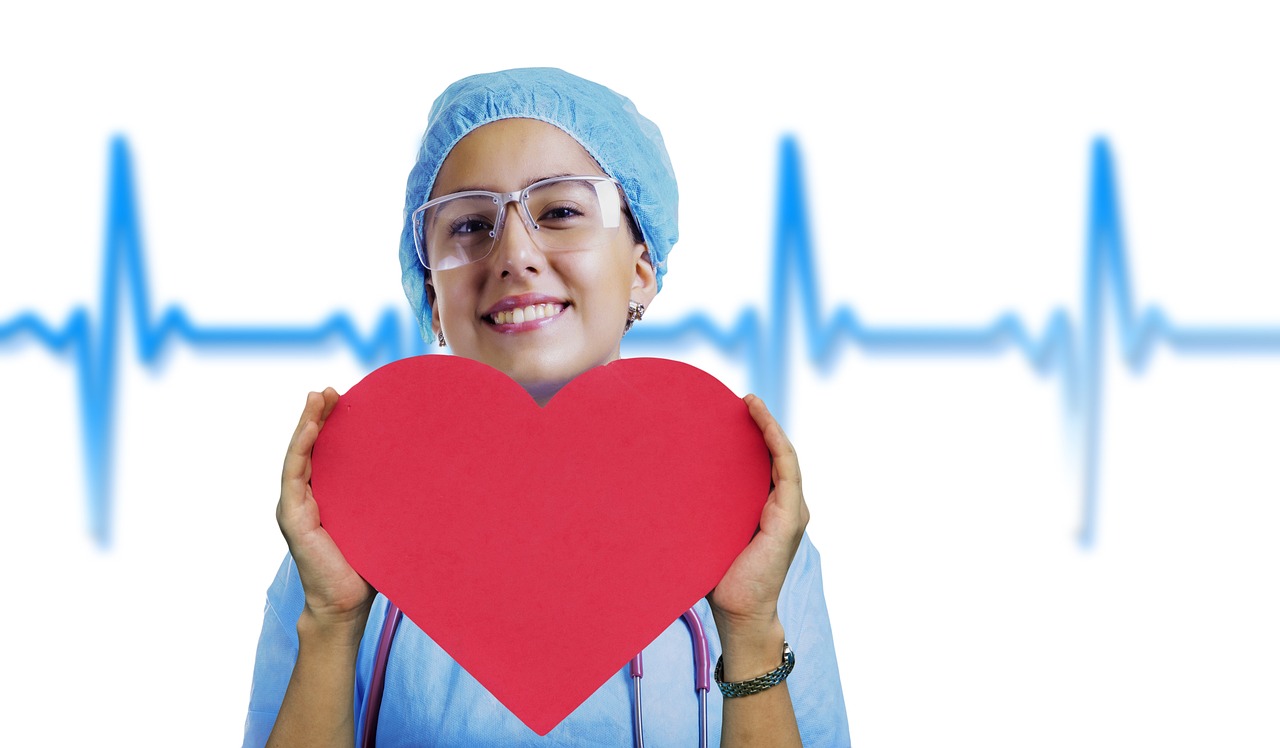
Ethical Considerations in AI
As we delve deeper into the integration of artificial intelligence in neurology, it becomes increasingly clear that we must navigate a landscape fraught with ethical dilemmas. The rapid advancement of AI technologies prompts us to ask some tough questions: How do we ensure patient data privacy? What safeguards are in place to prevent algorithmic bias? And how do we maintain human oversight in decision-making processes? These questions are not just academic; they have real-world implications for patients and healthcare providers alike.
One of the most pressing concerns is data privacy. With AI systems requiring vast amounts of patient data to function effectively, the risk of sensitive information being compromised is heightened. Neurologists and healthcare institutions must implement robust security measures to protect patient confidentiality. This includes using advanced encryption methods and ensuring compliance with regulations such as HIPAA in the United States. The balance between leveraging AI for improved patient outcomes and safeguarding personal data is a tightrope walk that requires constant vigilance.
Moreover, the potential for algorithmic bias poses another significant ethical challenge. If the data used to train AI systems is not diverse or representative of the broader population, there is a risk that certain demographic groups may receive subpar treatment recommendations. This can lead to disparities in healthcare outcomes, which is particularly concerning in neurology, where conditions like Alzheimer’s and Parkinson’s disease can manifest differently across various populations. Therefore, it is crucial for developers and healthcare professionals to continuously assess the fairness of AI algorithms and ensure they are trained on inclusive datasets.
To tackle these ethical issues, a multi-faceted approach is necessary. Here are some key strategies:
- Establish clear guidelines for data usage and patient consent.
- Conduct regular audits of AI algorithms to identify and rectify biases.
- Incorporate diverse datasets in the training of AI systems to enhance fairness.
- Engage with ethicists, patients, and healthcare professionals in the development of AI technologies.
Lastly, the implications of AI decision-making in healthcare cannot be overstated. While AI can assist in diagnosing conditions and suggesting treatments, the ultimate responsibility for patient care should always rest with human clinicians. The idea of a machine making critical health decisions raises ethical concerns about accountability and the human touch in medicine. As we embrace AI in neurology, we must ensure that it serves as a tool to augment human expertise rather than replace it.
In conclusion, the ethical considerations surrounding AI in neurology are complex and multifaceted. As we continue to explore the possibilities that AI offers, we must remain committed to addressing these challenges head-on. By prioritizing data privacy, mitigating algorithmic bias, and maintaining a human-centered approach to healthcare, we can harness the transformative power of AI while upholding the ethical standards that protect patients and promote equitable care.
- What are the main ethical concerns regarding AI in neurology?
The primary concerns include data privacy, algorithmic bias, and the need for human oversight in AI decision-making. - How can healthcare providers ensure patient data privacy?
By implementing robust security measures, using encryption, and complying with regulations like HIPAA. - What steps can be taken to address algorithmic bias in AI systems?
Regular audits, using diverse datasets for training, and engaging stakeholders in the development process are crucial. - Is AI likely to replace neurologists in the future?
No, AI is intended to augment human expertise, not replace it. The human touch remains essential in patient care.
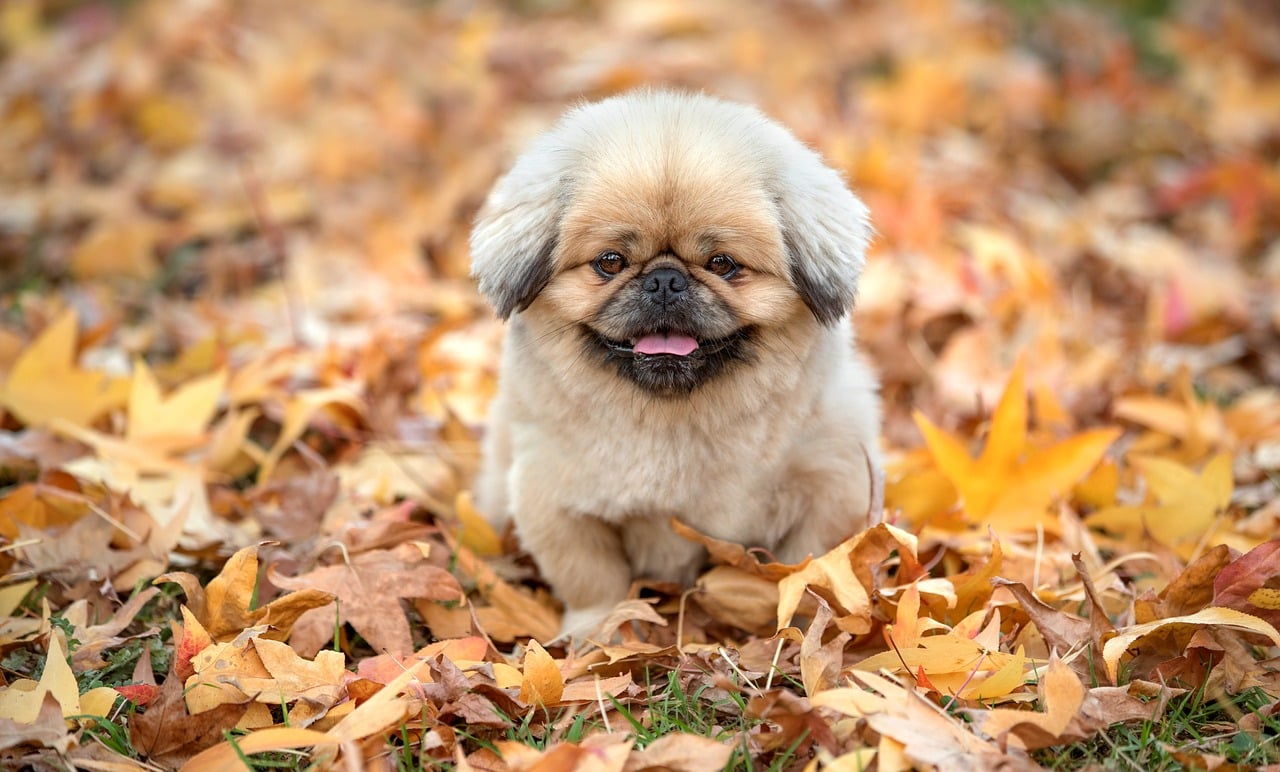
Data Privacy Concerns
The integration of artificial intelligence in neurology is not without its challenges, particularly when it comes to data privacy. As healthcare providers increasingly rely on AI to analyze sensitive patient information, the need to safeguard this data has never been more crucial. Imagine your personal health records being processed by algorithms designed to predict neurological disorders—sounds efficient, right? But what happens to that data once it's in the hands of machines? This question raises significant concerns that must be addressed to ensure patient trust and safety.
One of the primary issues is the potential for data breaches. With cyberattacks becoming more sophisticated, the risk of unauthorized access to sensitive health information looms large. Hospitals and clinics are tasked with implementing robust cybersecurity measures to protect patient data from falling into the wrong hands. According to recent studies, nearly 60% of healthcare organizations have experienced a data breach in the past few years, highlighting the urgency of this issue.
Moreover, the anonymization of data is another critical concern. While AI systems often utilize de-identified data to train algorithms, there's always a risk that individuals could be re-identified through advanced data mining techniques. This potential for re-identification poses a significant threat to patient privacy. For instance, if a patient's data is used in a research study and later becomes linked back to them, it could lead to unwanted exposure of their medical history.
To tackle these challenges, healthcare providers must establish strict data governance policies that outline how patient information is collected, stored, and shared. This includes implementing encryption technologies and ensuring that only authorized personnel have access to sensitive data. Additionally, patients should be made aware of their rights regarding their health information, fostering a culture of transparency and trust.
Furthermore, regulatory bodies are stepping in to create guidelines and frameworks that govern the use of AI in healthcare. The Health Insurance Portability and Accountability Act (HIPAA) in the United States, for example, sets the standard for protecting sensitive patient information. Compliance with such regulations is essential for healthcare organizations to maintain the integrity of their data practices.
In conclusion, while the potential benefits of AI in neurology are immense, the importance of addressing data privacy concerns cannot be overstated. As we navigate this new frontier, it is imperative that we prioritize the protection of patient information to ensure that technological advancements do not come at the cost of individual privacy. After all, in the world of healthcare, trust is everything.
- What are the main data privacy concerns related to AI in neurology? The main concerns include data breaches, re-identification risks, and the need for robust data governance policies.
- How can healthcare providers protect patient data? By implementing encryption technologies, ensuring limited access to sensitive information, and adhering to regulatory guidelines like HIPAA.
- What role do patients play in protecting their own data? Patients should be informed about their rights regarding their health information and advocate for transparency in how their data is used.
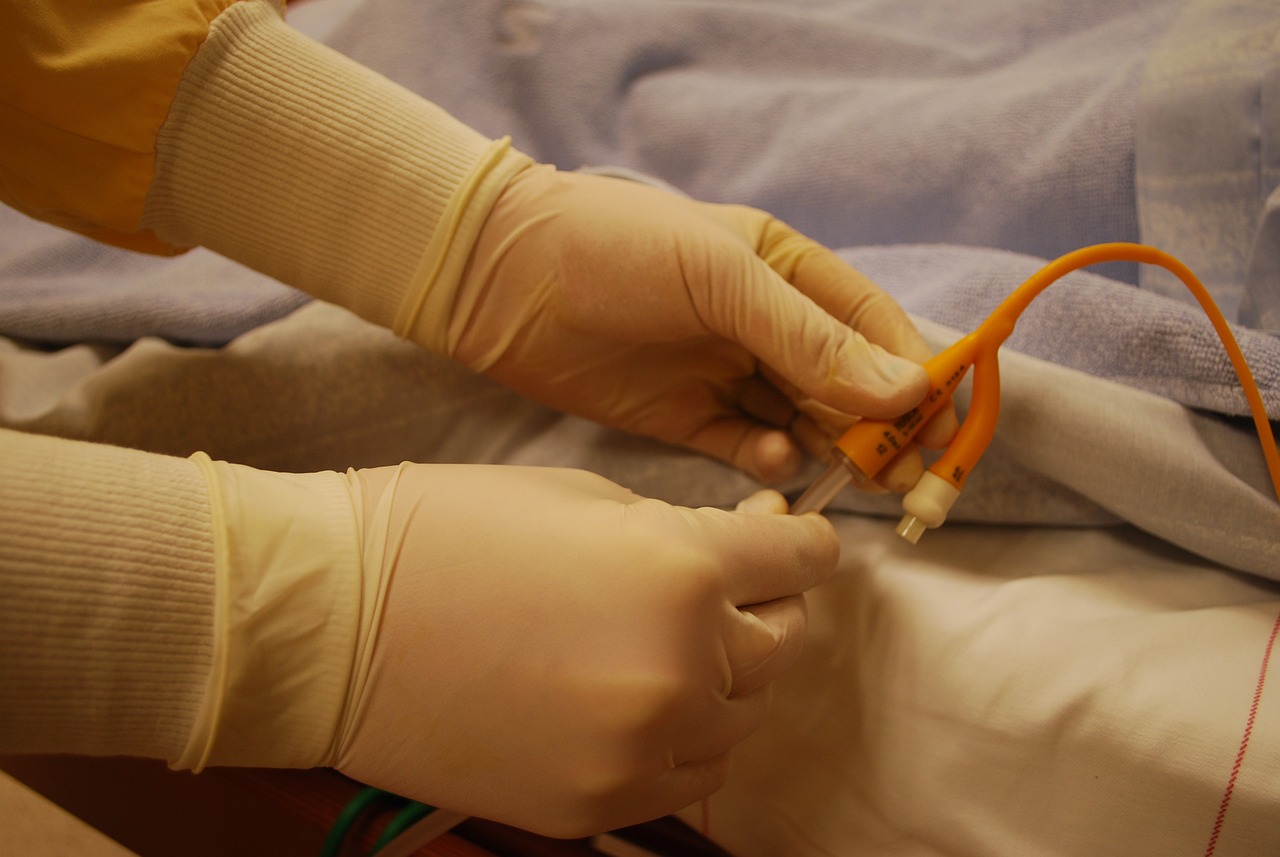
Addressing Algorithmic Bias
As we delve deeper into the integration of artificial intelligence in neurology, one of the most pressing issues that arises is algorithmic bias. This bias can manifest in various ways, often leading to disparities in treatment outcomes among different patient demographics. Imagine a world where a life-saving treatment is less effective for certain groups simply because the AI that recommended it was trained on data that didn't represent them adequately. This is not just a hypothetical situation; it's a reality that we must confront as we advance in this field.
Algorithmic bias occurs when the algorithms used in AI systems reflect the prejudices present in the data they were trained on. For instance, if a machine learning model is developed using predominantly data from one demographic, it may not perform as well for patients outside that group. This can result in misdiagnoses or ineffective treatment plans for those individuals, ultimately affecting their health outcomes. To combat this issue, it is crucial to implement several strategies:
- Diverse Data Collection: Ensuring that the data used to train AI models is representative of the entire population is fundamental. This means actively seeking out data from various ethnicities, ages, and genders to create a more balanced dataset.
- Regular Audits: Conducting regular audits of AI systems can help identify and rectify any biases that may have developed over time. These audits should assess the performance of AI models across different demographic groups.
- Transparent Algorithms: Developing algorithms that are transparent and explainable can foster trust. When healthcare providers understand how an AI system makes decisions, they can better assess its recommendations and recognize potential biases.
Moreover, involving a diverse group of stakeholders in the development process of AI systems can provide invaluable perspectives that help address bias. This includes not only data scientists and neurologists but also ethicists, patients, and community representatives. By fostering a collaborative environment, we can ensure that the AI tools we develop serve everyone equitably.
Ultimately, addressing algorithmic bias in AI is not just a technical challenge; it is a moral imperative. The healthcare field has a responsibility to ensure that all patients receive fair and effective treatment. By committing to ongoing education, rigorous testing, and community involvement, we can mitigate the risks associated with algorithmic bias and harness the full potential of AI in neurology.
- What is algorithmic bias?
Algorithmic bias refers to systematic and unfair discrimination that can occur when AI systems make decisions based on biased data or flawed algorithms. - How can we prevent algorithmic bias in AI?
Preventing algorithmic bias involves using diverse datasets, conducting regular audits, and ensuring transparency in algorithms. - Why is addressing algorithmic bias important in neurology?
Addressing algorithmic bias is crucial to ensure that all patients receive equitable treatment and that AI tools are effective across different demographics.
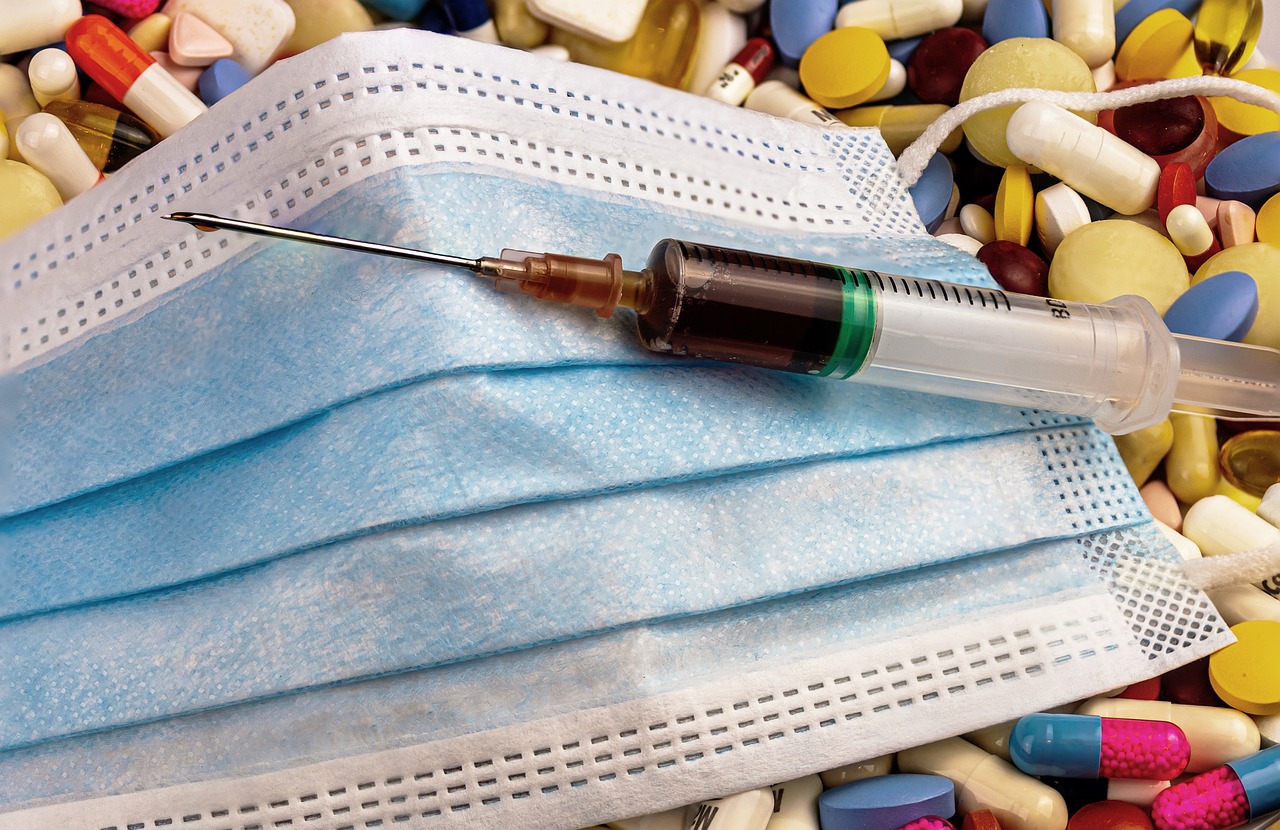
The Future of AI in Neurology
The future of AI in neurology is nothing short of exhilarating! As we stand on the brink of a technological revolution, the integration of artificial intelligence into the field of neurology promises to reshape how we understand, diagnose, and treat brain disorders. Imagine a world where neurologists have instant access to a wealth of data and insights at their fingertips, allowing them to make decisions that are not only faster but also more informed. This is not just a dream; it’s becoming a reality.
One of the most exciting prospects is the development of AI-driven diagnostic tools that can analyze brain scans with a level of precision that surpasses human capability. These tools utilize advanced algorithms to detect subtle changes in brain structure and function that may indicate the early stages of disorders such as Alzheimer's or Parkinson's disease. As these technologies evolve, we can expect a significant reduction in misdiagnosis rates, leading to timely interventions that can change the course of a patient's life.
Furthermore, AI has the potential to revolutionize treatment protocols. By harnessing machine learning, healthcare providers can create personalized treatment plans tailored to individual patient profiles. This means that instead of a one-size-fits-all approach, patients will receive therapies that are specifically designed based on their unique genetic makeup and health history. For instance, AI can analyze a patient's response to previous treatments and predict which new therapies are likely to be the most effective, thus enhancing the overall success rate of neurological interventions.
Moreover, the role of predictive analytics cannot be overstated. By leveraging vast amounts of data, AI can forecast patient outcomes with remarkable accuracy. This allows neurologists to not only choose the best treatment options but also to anticipate potential complications before they arise. Imagine a scenario where a doctor can foresee a patient's response to a medication and adjust the treatment plan proactively, rather than reactively. This level of foresight could significantly improve the quality of care patients receive.
As we look ahead, we must also consider the ethical implications of these advancements. The integration of AI into neurology raises critical questions about data privacy and algorithmic bias. It’s essential that as we embrace these technologies, we also establish robust frameworks to protect patient data and ensure that AI systems are fair and equitable. After all, the goal is to enhance patient care, not to introduce new disparities.
To sum up, the future of AI in neurology is bright and full of possibilities. With ongoing research and development, we can expect to see groundbreaking innovations that will not only improve diagnostic accuracy and treatment efficacy but also transform the patient experience. As we navigate this exciting frontier, it is crucial to remain vigilant about the ethical considerations that accompany these advancements, ensuring that the benefits of AI are accessible to all.
- What role does AI play in diagnosing neurological disorders?
AI enhances diagnostic accuracy by analyzing imaging data and identifying patterns that may be missed by human eyes, leading to earlier and more precise diagnoses.
- How can machine learning improve treatment plans for neurological patients?
Machine learning algorithms can analyze individual patient data to predict how they will respond to various treatments, allowing for personalized and effective care strategies.
- What ethical concerns are associated with AI in neurology?
Key concerns include data privacy, algorithmic bias, and the implications of AI decision-making, which must be addressed to ensure responsible use of technology in healthcare.
Frequently Asked Questions
- What role does AI play in diagnosing brain disorders?
AI significantly enhances the diagnostic process in neurology by utilizing advanced imaging techniques and machine learning algorithms. This technology allows for faster and more accurate identification of brain disorders, enabling healthcare professionals to make better-informed decisions.
- How is machine learning used in the treatment of neurological conditions?
Machine learning algorithms are being employed to personalize treatment plans for patients with neurological conditions. By analyzing individual patient data, these algorithms can predict how patients will respond to various therapies, ultimately improving treatment outcomes.
- What is predictive analytics, and how does it benefit patient outcomes?
Predictive analytics uses AI to forecast patient outcomes, which helps neurologists tailor treatment strategies to meet the unique needs of each patient. This proactive approach enables better management of brain disorders and enhances overall patient care.
- How do AI-driven risk assessment models work?
AI-driven risk assessment models identify patients at high risk for developing severe neurological disorders. By recognizing these individuals early, healthcare providers can implement preventive measures and interventions to improve long-term health prospects.
- What insights can AI provide regarding disease progression?
AI analyzes large datasets to offer neurologists valuable insights into disease progression and treatment efficacy. This data-driven approach fosters a deeper understanding of brain disorders, allowing for more effective management and care.
- How is AI optimizing clinical trials for neurological treatments?
AI plays a crucial role in optimizing clinical trials by streamlining patient recruitment and improving data analysis. This acceleration in the development of new therapies can lead to quicker and more effective treatment options for patients.
- What are the ethical considerations surrounding AI in neurology?
As AI technology advances in neurology, ethical concerns about patient data privacy, algorithmic bias, and the implications of AI decision-making must be addressed. It’s vital to ensure responsible use of AI while protecting sensitive patient information.
- What are the data privacy concerns associated with AI in neurology?
The integration of AI in neurology raises significant concerns regarding the privacy of patient data. It is essential to establish robust protocols that protect sensitive information while still leveraging the benefits of advanced technology.
- How is algorithmic bias addressed in AI systems?
Addressing algorithmic bias is crucial to ensure fairness and equity in AI applications within neurology. Ongoing efforts are necessary to monitor and correct any disparities in treatment outcomes that may arise from biased algorithms.
- What does the future hold for AI in neurology?
The future of AI in neurology is bright, with immense potential for advancements in managing brain disorders. Innovations promise to enhance diagnostic accuracy and treatment efficacy, ultimately benefiting patients worldwide.