Quantum Computing and AI: A Powerful Duo
Welcome to the fascinating world where quantum computing meets artificial intelligence! This dynamic duo is not just a technological trend; it's a revolutionary force that has the potential to reshape our lives in ways we can barely imagine. Think of quantum computing as a supercharged engine, capable of processing complex calculations at lightning speed, while AI acts as the smart navigator, using this power to solve problems, make predictions, and enhance decision-making across various industries.
Imagine trying to solve a massive jigsaw puzzle. Classical computing is like working on it with a limited number of pieces at a time, while quantum computing allows you to see all the pieces simultaneously, making it easier to find where each piece fits. This synergy between quantum computing and AI opens up new frontiers, enabling advancements that could lead to breakthroughs in fields such as healthcare, finance, and climate science.
As we delve deeper into this article, we will explore the fundamentals of quantum computing, understand the core components of AI, and examine how their combination can lead to unprecedented innovations. From optimizing algorithms to enhancing data security, the implications are vast and exciting. So, buckle up as we embark on this journey into the future of technology!
Understanding quantum computing fundamentals is essential for grasping its implications. This section delves into qubits, superposition, and entanglement, laying the groundwork for how quantum systems differ from classical computing.
This section provides a concise overview of artificial intelligence, discussing its core components such as machine learning, neural networks, and natural language processing, and how these technologies are reshaping industries.
Machine learning is a vital aspect of AI, enabling systems to learn from data. This subsection explores its techniques, applications, and how it drives advancements in various fields.
This part distinguishes between supervised and unsupervised learning, discussing their methodologies, advantages, and specific use cases in AI applications across different domains.
Deep learning, a subset of machine learning, has achieved remarkable success in tasks like image and speech recognition. This subsection highlights its impact and future potential in AI development.
Quantum computing holds promise for numerous applications, from cryptography to drug discovery. This section examines various sectors where quantum technology can create significant advancements.
The combination of quantum computing and AI presents unique opportunities. This section discusses how they complement each other, enhancing computational power and enabling breakthroughs in data processing and analysis.
Quantum computing can optimize AI algorithms, allowing for faster and more efficient data processing. This subsection explores specific examples of how quantum techniques enhance machine learning performance.
The fusion of quantum computing and AI can revolutionize data security. This part discusses how quantum algorithms can strengthen encryption methods and protect sensitive information from cyber threats.
Q1: What is quantum computing?
Quantum computing is a type of computation that uses quantum bits (qubits) to process information in ways that classical computers cannot, leveraging principles of quantum mechanics.
Q2: How does AI benefit from quantum computing?
AI benefits from quantum computing by gaining the ability to process vast amounts of data more quickly, leading to faster learning and improved decision-making capabilities.
Q3: Are there practical applications of quantum computing and AI today?
Yes, practical applications include drug discovery, optimizing logistics, enhancing cybersecurity, and improving financial modeling, among others.
Q4: What industries will be most impacted by this technology?
Industries such as healthcare, finance, logistics, and cybersecurity are expected to see significant impacts from the integration of quantum computing and AI.
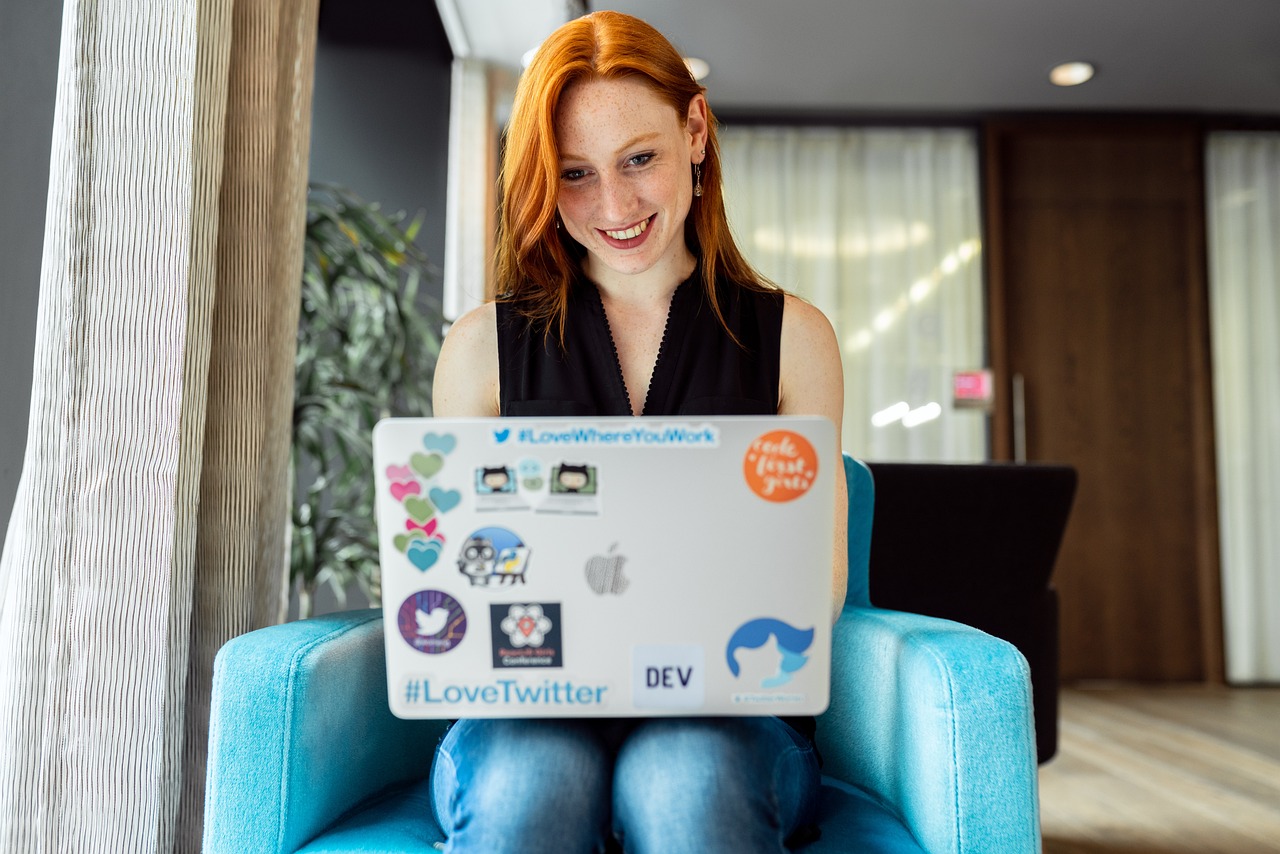
The Basics of Quantum Computing
Understanding the fundamentals of quantum computing is crucial for appreciating its transformative potential. At the heart of quantum computing lies the concept of qubits, which are the quantum analogs of classical bits. Unlike traditional bits that can exist in one of two states—0 or 1—qubits can exist in multiple states simultaneously, thanks to a phenomenon known as superposition. This ability to hold multiple states at once allows quantum computers to process vast amounts of information at an unprecedented speed.
Moreover, qubits can be interconnected through entanglement, another fundamental principle of quantum mechanics. When qubits become entangled, the state of one qubit becomes dependent on the state of another, no matter the distance between them. This interconnectedness enables quantum computers to perform complex calculations that would be infeasible for classical computers. Imagine trying to solve a massive maze: a classical computer would methodically explore each path one at a time, while a quantum computer could explore multiple paths simultaneously, reaching the exit much faster.
To illustrate the differences between classical and quantum computing, consider the following table:
Aspect | Classical Computing | Quantum Computing |
---|---|---|
Basic Unit | Bit | Qubit |
State Representation | 0 or 1 | 0, 1, or both (superposition) |
Processing Capability | Sequential | Parallel |
Interconnectivity | No correlation | Entangled states |
This table highlights the revolutionary aspects of quantum computing and how they differ from classical systems. The implications of these features are enormous, especially when we consider the potential applications in fields like cryptography, optimization problems, and drug discovery. For instance, in cryptography, quantum computers could break traditional encryption methods, prompting a significant shift in how we secure our data.
As we delve deeper into the world of quantum computing, it becomes clear that this technology is not just a theoretical concept but a rapidly evolving field with real-world applications. The next step is understanding how these quantum principles can be harnessed, particularly in conjunction with artificial intelligence. This intersection holds the key to unlocking new levels of problem-solving capabilities and innovation across various industries.
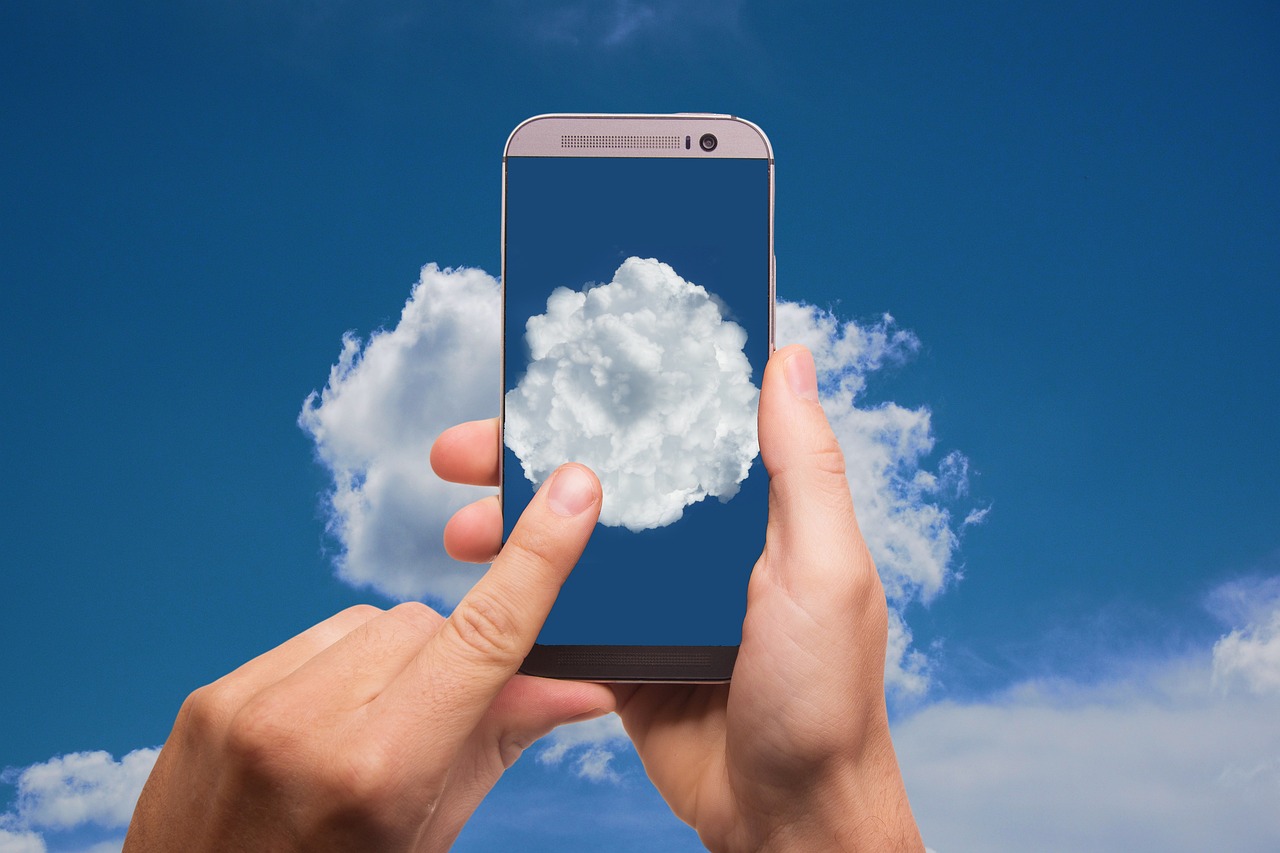
Artificial Intelligence Overview
Artificial Intelligence (AI) is transforming the world as we know it, acting as a catalyst for innovation and change across multiple sectors. At its core, AI refers to the simulation of human intelligence processes by machines, especially computer systems. This encompasses a variety of functionalities, including learning, reasoning, and self-correction. The beauty of AI lies in its ability to analyze vast amounts of data, recognize patterns, and make decisions with minimal human intervention, which ultimately leads to enhanced efficiency and productivity.
One of the fundamental components of AI is machine learning, a subset that focuses on the development of algorithms that enable computers to learn from and make predictions based on data. Imagine teaching a child to recognize different animals. You would show them various pictures of cats and dogs, and over time, they would learn to distinguish between the two. Similarly, machine learning algorithms learn from data inputs to improve their accuracy over time.
Another critical aspect of AI is neural networks, which are designed to mimic the way the human brain operates. These networks consist of interconnected nodes (or neurons) that process information in layers. Just like our brains, neural networks can recognize complex patterns and relationships within data. This capability has led to breakthroughs in fields such as image and speech recognition, where systems can now identify objects and interpret spoken language with remarkable precision.
Moreover, natural language processing (NLP) is a vital facet of AI that allows machines to understand, interpret, and respond to human language in a way that is both meaningful and contextually relevant. Think of digital assistants like Siri or Alexa; they use NLP to comprehend your commands and provide you with the information you seek. This technology is not just limited to voice recognition; it also powers chatbots and customer service applications that enhance user experience by providing instant support.
To further illustrate the core components of AI, here’s a brief table summarizing the key aspects:
Component | Description |
---|---|
Machine Learning | Algorithms that allow computers to learn from data and improve over time. |
Neural Networks | Systems that simulate the human brain's interconnected neuron structure to process information. |
Natural Language Processing | Technologies that enable machines to understand and respond to human language. |
As we delve deeper into the realms of AI, it becomes evident that these technologies are not merely tools but rather transformative forces that are reshaping industries. From healthcare to finance, AI applications are streamlining operations, enhancing decision-making processes, and delivering personalized experiences to users. However, with great power comes great responsibility. It’s crucial to address ethical considerations surrounding AI, including data privacy, bias in algorithms, and the potential for job displacement.
In summary, the overview of artificial intelligence reveals a dynamic landscape filled with potential and challenges. As we continue to explore and harness the capabilities of AI, the possibilities for innovation are boundless. How we navigate this journey will determine the future of technology and its impact on society as a whole.
- What is AI? AI stands for artificial intelligence, which refers to the simulation of human intelligence processes by machines.
- How does machine learning work? Machine learning algorithms analyze data to identify patterns and make predictions without being explicitly programmed.
- What are neural networks? Neural networks are computational models inspired by the human brain, designed to recognize complex patterns in data.
- What is natural language processing? NLP is a field of AI that focuses on the interaction between computers and humans through natural language.

Machine Learning in AI
Machine learning is often described as the beating heart of artificial intelligence, and for good reason. It’s the technology that allows systems to learn from data, adapt over time, and make decisions with minimal human intervention. Think of it like teaching a child how to ride a bike; at first, they might wobble and fall, but with practice and feedback, they become proficient. In the same way, machine learning algorithms analyze data, learn from it, and improve their performance over time.
At its core, machine learning can be broken down into a few key techniques and methodologies. These include supervised learning, unsupervised learning, and reinforcement learning. Each of these approaches has its own unique applications and advantages:
- Supervised Learning: This is where the algorithm is trained on a labeled dataset, meaning that the input data is paired with the correct output. It’s like having a teacher guide you through a lesson. Common applications include spam detection in emails and image recognition.
- Unsupervised Learning: In contrast, unsupervised learning deals with unlabeled data. The algorithm tries to learn the underlying patterns without any guidance. Imagine exploring a new city without a map; you’ll discover interesting places and connections on your own! This method is often used for clustering and association tasks.
- Reinforcement Learning: This is akin to training a pet. You reward the algorithm for making the right decisions and penalize it for the wrong ones. This approach is widely used in robotics and game development, where the AI learns to navigate complex environments.
Machine learning is not just a buzzword; it’s a transformative force across various industries. For instance, in healthcare, algorithms can analyze medical images and assist in diagnosing diseases with remarkable accuracy. In finance, machine learning models can predict market trends and detect fraudulent transactions. The retail sector uses these algorithms to personalize shopping experiences, recommending products based on previous purchases.
As we delve deeper into this technology, we see that machine learning is driving innovation at an unprecedented pace. Companies are investing heavily in research and development, and the landscape is evolving rapidly. The ability to process vast amounts of data and extract meaningful insights is becoming a game-changer. Imagine the possibilities: self-driving cars navigating through traffic, smart assistants that understand our preferences, and predictive analytics that can foresee market shifts before they happen.
However, with great power comes great responsibility. The ethical implications of machine learning are a hot topic. Issues such as data privacy, algorithmic bias, and transparency must be addressed to ensure that these technologies are used for good. As we harness the potential of machine learning, it’s crucial to create frameworks that promote fairness and accountability.
In summary, machine learning is a vital aspect of artificial intelligence that empowers systems to learn, adapt, and make decisions. Its applications are vast and varied, with the potential to revolutionize industries and improve our daily lives. As we continue to explore this exciting field, we must also be mindful of the ethical considerations that accompany such powerful technologies.
- What is machine learning? Machine learning is a subset of artificial intelligence that enables systems to learn from data and improve over time without being explicitly programmed.
- How is machine learning used in everyday life? Machine learning is used in various applications such as email filtering, recommendation systems, voice recognition, and more.
- What are the main types of machine learning? The main types include supervised learning, unsupervised learning, and reinforcement learning.
- What are the ethical concerns surrounding machine learning? Ethical concerns include data privacy, algorithmic bias, and the need for transparency in how algorithms make decisions.

Supervised vs. Unsupervised Learning
When diving into the fascinating world of artificial intelligence, one of the first things you’ll encounter is the concept of machine learning. Within this realm, two primary methodologies stand out: supervised learning and unsupervised learning. But what exactly sets them apart, and why should you care? Let's break it down!
Supervised learning is like having a knowledgeable mentor guiding you through a complex maze. In this approach, the model is trained on a labeled dataset, which means that each input data point is paired with the correct output. This training process enables the model to learn the relationship between the input and output, making predictions on new, unseen data. For instance, think of teaching a child to recognize animals: you show them pictures of dogs and cats, telling them which is which. Over time, they learn to identify these animals on their own.
On the flip side, we have unsupervised learning, which is akin to exploring that same maze without a map. Here, the model is presented with data that has no labels or predefined outcomes. The goal is to uncover hidden patterns or groupings within the data. For example, imagine a group of people at a party. Without knowing anyone’s name, you might start to notice that certain individuals tend to cluster together based on shared interests, like sports or music. This is the essence of unsupervised learning—finding structure in chaos.
To illustrate the differences more clearly, let’s take a look at the following table:
Aspect | Supervised Learning | Unsupervised Learning |
---|---|---|
Data Type | Labeled Data | Unlabeled Data |
Goal | Predict outcomes based on input | Discover patterns or groupings |
Examples | Classification, Regression | Clustering, Association |
Both supervised and unsupervised learning have their unique advantages and applications. Supervised learning excels in scenarios where you have a clear target variable and a wealth of labeled data, such as in email spam detection or medical diagnosis. On the other hand, unsupervised learning shines in exploratory data analysis, where the goal is to identify trends or anomalies without prior knowledge, like customer segmentation in marketing.
In conclusion, understanding the differences between supervised and unsupervised learning can empower you to choose the right approach for your AI projects. Whether you're looking to make predictions or uncover hidden insights, both methodologies offer powerful tools to harness the potential of data.
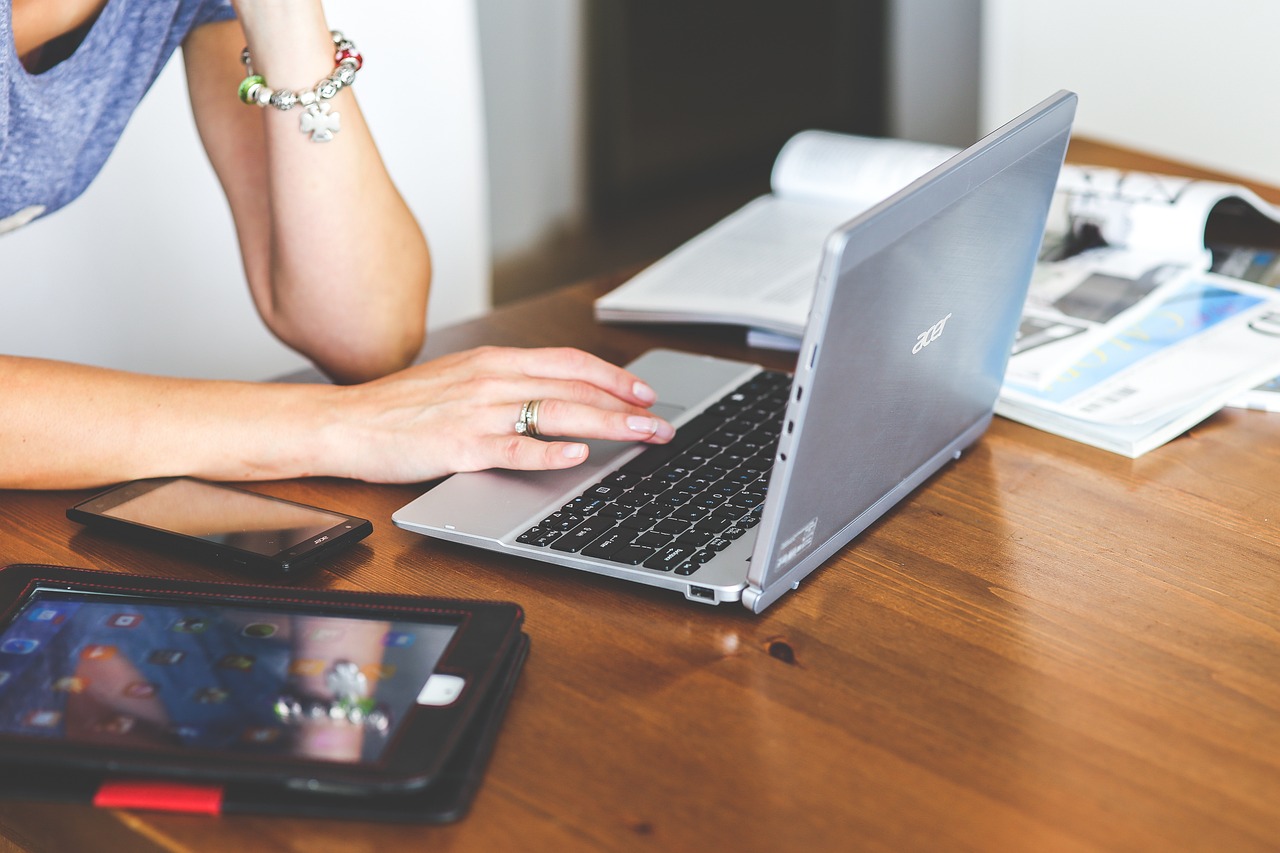
Deep Learning Breakthroughs
Deep learning, a subfield of artificial intelligence, has taken the tech world by storm, achieving breakthroughs that were once thought to be the stuff of science fiction. Imagine teaching a computer to recognize your face, understand your voice, or even predict your next move! This is not just a dream anymore; it’s a reality thanks to the advancements in deep learning algorithms.
At its core, deep learning mimics the way the human brain operates through a network of neurons. These artificial neural networks are designed to learn from vast amounts of data, making them incredibly powerful tools for various applications. For instance, in the realm of image recognition, deep learning has enabled systems to identify objects with astonishing accuracy. A well-known example is Google's DeepMind, which developed a system that can beat human players at complex games like Go and StarCraft II. This level of proficiency exemplifies how deep learning can outperform traditional algorithms.
Moreover, deep learning has made significant strides in natural language processing (NLP), allowing machines to understand and generate human language. Chatbots and virtual assistants, such as Siri and Alexa, leverage deep learning to interpret user queries and provide relevant responses. This has transformed customer service industries, making interactions faster and more efficient. The ability to analyze sentiment and context in conversations has also opened doors for businesses to engage with their customers in more personalized ways.
One of the most exciting applications of deep learning is in the field of healthcare. By analyzing medical images, deep learning algorithms can assist doctors in diagnosing diseases like cancer at an early stage, which can be crucial for patient outcomes. For example, researchers have developed systems that can detect tumors in mammograms with a precision that rivals experienced radiologists. This not only enhances diagnostic accuracy but also speeds up the process, allowing for quicker treatment decisions.
But let's not forget the role of deep learning in autonomous vehicles. Companies like Tesla and Waymo are utilizing deep learning to enable cars to navigate through complex environments, recognize pedestrians, and make split-second decisions. This technology is paving the way for safer and more efficient transportation systems, potentially reducing accidents caused by human error.
As we look to the future, the potential of deep learning seems limitless. With ongoing research and development, we can expect even more breakthroughs that will redefine our interaction with technology. However, it's crucial to approach these advancements with caution, considering ethical implications and the need for transparency in AI systems. The journey of deep learning is just beginning, and its impact on society will be profound.
- What is deep learning?
Deep learning is a subset of machine learning that uses neural networks to analyze various forms of data, enabling systems to learn and make decisions based on that data.
- How does deep learning differ from traditional machine learning?
While traditional machine learning relies on feature extraction by humans, deep learning automates this process through multiple layers of neural networks, allowing for more complex pattern recognition.
- What are some real-world applications of deep learning?
Deep learning is used in a variety of fields, including healthcare for disease diagnosis, automotive for self-driving cars, and finance for fraud detection.
- Is deep learning the same as artificial intelligence?
No, deep learning is a specific approach within the broader field of artificial intelligence, which encompasses various techniques and methods for creating intelligent systems.

Quantum Computing Applications
Quantum computing is not just a futuristic concept; it is rapidly evolving into a practical technology with a wide range of applications that could transform various industries. Imagine a world where complex problems that would take classical computers years to solve can be tackled in mere seconds. This is the promise of quantum computing, and its potential applications are as exciting as they are diverse.
One of the most significant areas where quantum computing can make a huge impact is in cryptography. Traditional encryption methods rely on the difficulty of certain mathematical problems, such as factoring large numbers. However, quantum computers can solve these problems exponentially faster, leading to the development of quantum-resistant encryption methods. As cyber threats become more sophisticated, the need for robust security measures becomes paramount. Quantum cryptography, particularly Quantum Key Distribution (QKD), offers a way to secure communications in a manner that classical systems cannot.
Another promising application lies in drug discovery. The pharmaceutical industry is notoriously slow and expensive when it comes to developing new medications. Quantum computers can simulate molecular interactions at an unprecedented scale and speed, allowing researchers to identify potential drug candidates much quicker than before. This capability can not only reduce the time it takes to bring new drugs to market but also lower the costs associated with research and development.
Moreover, quantum computing holds the potential to revolutionize optimization problems. Industries such as logistics, finance, and manufacturing often deal with complex optimization challenges. For instance, a logistics company may need to determine the most efficient route for deliveries. Quantum algorithms can process vast amounts of data and provide optimal solutions much faster than classical algorithms, leading to significant cost savings and efficiency improvements.
In the field of artificial intelligence, quantum computing can enhance machine learning models by processing large datasets more efficiently. By leveraging quantum mechanics, AI algorithms can be optimized to learn from data more quickly and accurately. This could lead to breakthroughs in areas such as natural language processing, image recognition, and predictive analytics, enabling AI systems to perform tasks that were previously thought to be impossible.
Finally, financial modeling is another area where quantum computing can shine. The financial industry relies heavily on complex models to predict market trends and assess risk. Quantum computers can analyze vast datasets and run simulations that are currently beyond the reach of classical computers. This capability can lead to better investment strategies, more accurate risk assessments, and ultimately, a more stable financial system.
In summary, the applications of quantum computing span a wide array of fields, each with the potential to revolutionize how we approach complex problems. From enhancing security in communications to accelerating drug discovery and optimizing logistics, the future of quantum computing is bright and full of possibilities.
- What is quantum computing? Quantum computing utilizes the principles of quantum mechanics to process information in ways that classical computers cannot, allowing for faster and more efficient problem-solving.
- How does quantum computing improve drug discovery? By simulating molecular interactions quickly, quantum computers can help researchers identify potential drug candidates much faster than traditional methods.
- Can quantum computing enhance AI? Yes, quantum computing can optimize machine learning algorithms, enabling AI systems to learn from data more efficiently and accurately.
- What are the security implications of quantum computing? Quantum computers can potentially break traditional encryption methods, necessitating the development of quantum-resistant encryption to secure sensitive information.

The Synergy Between Quantum Computing and AI
The intersection of quantum computing and artificial intelligence is not just a buzzword; it’s a game changer that holds the potential to redefine how we understand technology. Imagine a world where computers can solve complex problems in seconds that would take classical computers years. This is the promise of quantum computing, and when combined with AI, it creates a synergy that can enhance our computational capabilities to unprecedented levels. The collaboration between these two fields can lead to breakthroughs that were previously thought impossible, opening doors to new innovations and solutions across various industries.
At the heart of this synergy is the ability of quantum computing to process vast amounts of data at lightning speed. Traditional computers operate on bits that can either be 0 or 1, but quantum computers use qubits, which can exist in multiple states simultaneously thanks to the principles of superposition. This allows quantum computers to handle complex calculations at a scale and speed that classical systems simply cannot match. When AI algorithms leverage this power, they can analyze and interpret data with a level of accuracy and efficiency that can revolutionize sectors such as healthcare, finance, and logistics.
Moreover, quantum computing can enhance AI by improving the performance of machine learning algorithms. For instance, quantum techniques can be used to optimize neural networks, allowing them to learn from data faster and more effectively. Traditional machine learning models often struggle with large datasets, but with quantum computing, we can expect significant improvements in training times and predictive accuracy. This means that AI can become not just smarter but also more adaptable to changing environments and requirements.
One of the most exciting prospects of this synergy is in the realm of data security. As cyber threats become increasingly sophisticated, the need for robust security measures is paramount. Quantum computing can enhance data encryption methods, making it nearly impossible for malicious actors to breach sensitive information. By integrating AI into this quantum framework, we can develop adaptive security systems that not only respond to threats in real-time but also predict potential vulnerabilities before they can be exploited.
In summary, the collaboration between quantum computing and AI is more than just a technological advancement; it’s a fusion of capabilities that can transform industries. By harnessing the strengths of both fields, we can tackle some of the most pressing challenges of our time, from climate change to healthcare disparities. The future is bright for those who embrace this powerful duo, as they stand on the brink of a new technological era.
- What is quantum computing? Quantum computing is a type of computing that uses quantum bits (qubits) to perform calculations at speeds and efficiencies that traditional computers cannot achieve.
- How does AI benefit from quantum computing? AI can leverage the speed and computational power of quantum computing to process large datasets more efficiently, improving machine learning algorithms and predictive analytics.
- Can quantum computing improve data security? Yes, quantum computing can enhance encryption methods, making it more difficult for cyber threats to compromise sensitive information.
- What industries will be most affected by this synergy? Industries such as healthcare, finance, logistics, and cybersecurity are likely to see significant advancements due to the combined power of quantum computing and AI.

Improving AI Algorithms
Imagine a world where artificial intelligence (AI) can learn and adapt at lightning speed, making decisions faster than ever before. This is not just a dream; it’s becoming a reality through the incredible synergy between quantum computing and AI. Quantum computing has the potential to enhance AI algorithms significantly, allowing them to process vast amounts of data more efficiently and accurately. By leveraging the unique properties of quantum mechanics, such as superposition and entanglement, we can unlock new levels of computational power that traditional computers simply cannot match.
One of the most exciting aspects of quantum computing is its ability to tackle complex optimization problems that are often at the core of AI algorithms. For instance, consider the challenge of training a machine learning model. This process typically involves adjusting numerous parameters to improve performance. Quantum algorithms can explore multiple possibilities simultaneously, dramatically speeding up the training process. Instead of taking days or weeks, we could see results in mere hours or even minutes!
Let’s delve into a few specific ways quantum computing can improve AI algorithms:
- Enhanced Data Processing: Quantum systems can analyze large datasets at unprecedented speeds. This capability allows AI to learn from more data, leading to better predictions and insights.
- Improved Feature Selection: Quantum algorithms can help identify the most relevant features in a dataset more efficiently, which is crucial for building effective machine learning models.
- Faster Convergence: In machine learning, the goal is often to minimize a loss function. Quantum computing can accelerate this process, allowing algorithms to find optimal solutions quicker.
Moreover, the integration of quantum computing into AI can lead to breakthroughs in natural language processing (NLP). Imagine an AI that can understand and generate human language with the same nuance and context as a person. Quantum algorithms can enhance the training of NLP models, making them more adept at handling complex language structures and semantics.
To illustrate this further, let's look at a simple comparison of traditional AI training versus quantum-enhanced AI training:
Aspect | Traditional AI Training | Quantum-Enhanced AI Training |
---|---|---|
Speed | Days/Weeks | Hours/Minutes |
Data Utilization | Limited | Extensive |
Feature Selection | Manual/Time-Consuming | Automated/Quick |
Model Accuracy | Moderate | High |
As we continue to explore the intersection of quantum computing and AI, the possibilities seem endless. The potential for improved algorithms not only enhances the capabilities of AI but also opens doors to new applications across various fields, including healthcare, finance, and transportation. Imagine AI systems that can predict disease outbreaks, optimize supply chains, or even manage smart cities with unparalleled efficiency.
In conclusion, the marriage of quantum computing and AI is set to revolutionize how we approach problem-solving in the digital age. By improving AI algorithms, we are not just enhancing technology; we are paving the way for innovations that can transform our everyday lives. The future is bright, and with quantum computing, we are just scratching the surface of what is possible.
Q1: How does quantum computing differ from classical computing?
A1: Quantum computing utilizes qubits, which can represent multiple states simultaneously, allowing for parallel processing. In contrast, classical computing relies on bits that can be either 0 or 1, limiting its processing capabilities.
Q2: Can quantum computing solve all AI problems?
A2: While quantum computing significantly enhances certain AI algorithms, it is not a one-size-fits-all solution. It excels in specific areas, particularly those involving complex optimization and large datasets.
Q3: What industries will benefit most from this technology?
A3: Industries such as healthcare, finance, logistics, and cybersecurity are expected to see substantial advancements from the integration of quantum computing and AI.
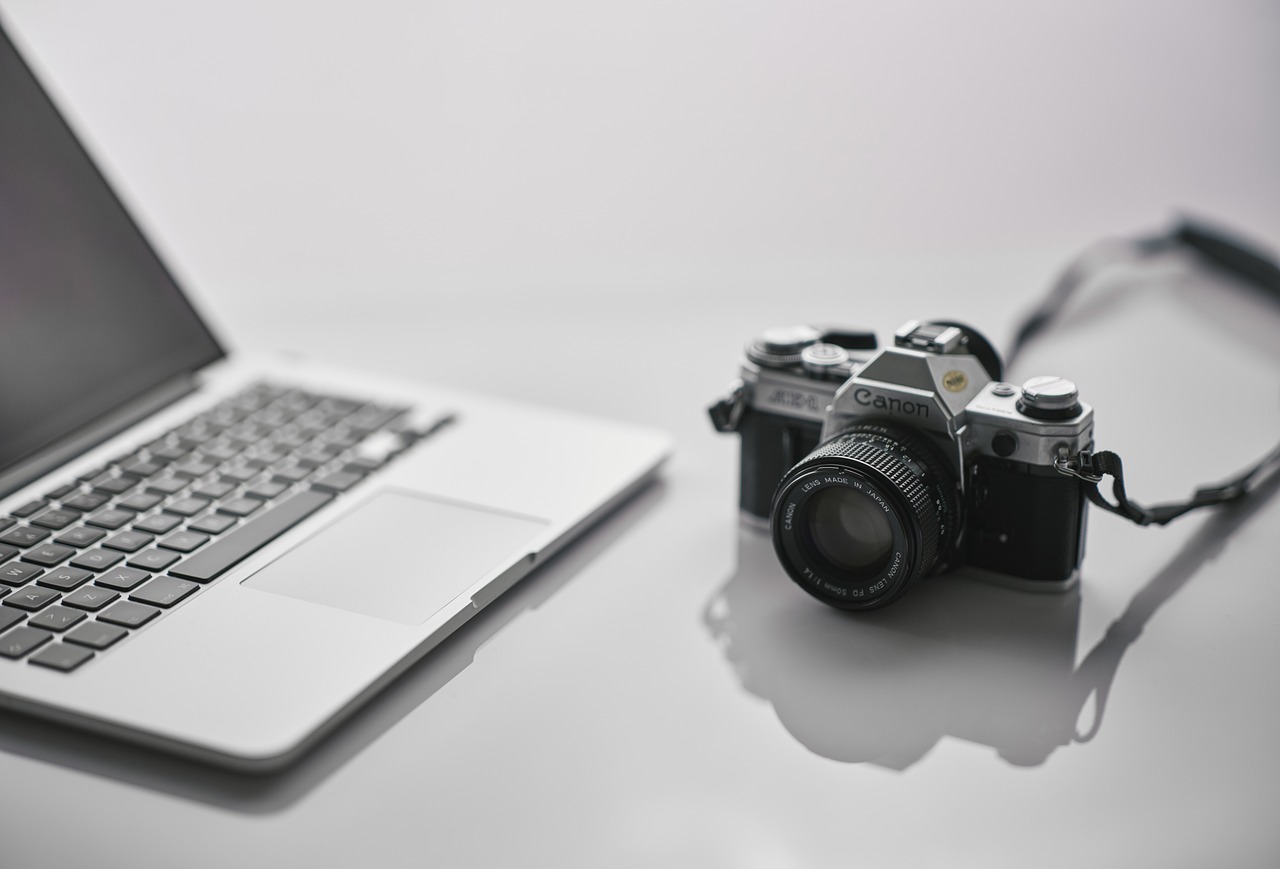
Data Security Enhancements
In today's digital landscape, where data breaches and cyber threats loom large, the fusion of quantum computing and artificial intelligence (AI) is poised to revolutionize data security. Imagine a world where your sensitive information is safeguarded by the most advanced technologies available—this is no longer a distant dream but an impending reality. Quantum computing, with its unique principles, can enhance traditional security measures, creating a formidable barrier against malicious attacks.
At the heart of this transformation are quantum algorithms, which possess the potential to strengthen encryption methods significantly. Traditional encryption techniques, such as RSA and AES, rely on mathematical problems that are challenging to solve with classical computers. However, quantum computers can tackle these problems with remarkable efficiency, rendering conventional encryption methods vulnerable. This is where AI steps in, working alongside quantum computing to develop new algorithms that can withstand the power of quantum attacks.
For instance, quantum key distribution (QKD) is a groundbreaking approach that leverages the principles of quantum mechanics to create secure communication channels. Unlike traditional methods, QKD ensures that any attempt at eavesdropping can be detected instantly, allowing users to take immediate action. By integrating AI, we can analyze communication patterns and detect anomalies in real-time, enhancing the overall security framework.
Furthermore, the implementation of quantum-enhanced machine learning algorithms can significantly improve threat detection capabilities. These algorithms can sift through vast amounts of data at unprecedented speeds, identifying potential vulnerabilities and threats before they escalate into full-blown attacks. The synergy between quantum computing and AI not only enhances the speed of data processing but also increases the accuracy of threat assessments, making it a game-changer in cybersecurity.
In addition to protecting sensitive information, quantum computing can also aid in developing robust security protocols across various industries. For example, in the financial sector, quantum algorithms can help secure transactions and protect against fraud by ensuring that data integrity is maintained. In healthcare, patient records can be encrypted with quantum-level security, safeguarding personal information from unauthorized access.
As we look to the future, the combination of quantum computing and AI presents a new frontier in the quest for data security. By harnessing the strengths of both technologies, we can build a more secure digital ecosystem that not only protects individual privacy but also fortifies the integrity of entire industries. The implications are vast, and the potential for innovation is boundless.
- What is quantum key distribution?
Quantum key distribution (QKD) is a method for secure communication that uses quantum mechanics to create a shared, secret key between two parties, ensuring that any eavesdropping attempts can be detected.
- How does AI enhance data security?
AI enhances data security by analyzing vast amounts of data to identify patterns, detect anomalies, and predict potential threats, allowing for proactive measures to be taken against cyber attacks.
- Can quantum computing break current encryption methods?
Yes, quantum computing has the potential to break many current encryption methods, which is why developing quantum-resistant algorithms is crucial for future data security.
- What industries can benefit from quantum computing and AI in data security?
Industries such as finance, healthcare, and telecommunications can greatly benefit from the enhanced security measures provided by the combination of quantum computing and AI.
Frequently Asked Questions
- What is quantum computing?
Quantum computing is a revolutionary technology that uses the principles of quantum mechanics to process information. Unlike classical computers that use bits (0s and 1s), quantum computers use qubits, which can exist in multiple states at once due to superposition. This allows them to solve complex problems much faster than traditional computers.
- How does artificial intelligence relate to quantum computing?
Artificial intelligence (AI) and quantum computing are two powerful technologies that can complement each other. Quantum computing can enhance AI algorithms by providing greater computational power, enabling faster data processing and more efficient learning from vast datasets. This synergy can lead to breakthroughs in various fields, from healthcare to finance.
- What are the main types of machine learning?
Machine learning, a crucial aspect of AI, primarily consists of two types: supervised learning and unsupervised learning. In supervised learning, algorithms learn from labeled data, while unsupervised learning involves finding patterns in data without pre-existing labels. Each has its unique applications and advantages in solving different problems.
- Can quantum computing improve AI algorithms?
Absolutely! Quantum computing can significantly optimize AI algorithms by allowing them to process and analyze data more efficiently. For instance, quantum techniques can help in training machine learning models faster, leading to quicker insights and better decision-making capabilities.
- What industries can benefit from quantum computing and AI?
Many industries stand to gain from the integration of quantum computing and AI, including healthcare, finance, cybersecurity, and pharmaceuticals. These technologies can enhance drug discovery, improve financial modeling, and strengthen data security through advanced encryption methods, creating new opportunities for innovation.
- What challenges do quantum computing and AI face?
While both technologies are promising, they also face challenges. Quantum computing is still in its infancy, with issues like qubit stability and error rates to overcome. Meanwhile, AI faces challenges related to data privacy, bias in algorithms, and the need for vast amounts of data to learn effectively. Addressing these challenges is crucial for their successful integration.
- How can quantum computing enhance data security?
Quantum computing can revolutionize data security by developing advanced encryption methods that are virtually unbreakable. Quantum algorithms can help create secure communication channels, protecting sensitive information from cyber threats and ensuring data integrity in a world where cyberattacks are increasingly sophisticated.