Steering Clear of Pitfalls in AI Ethics
Artificial Intelligence (AI) is rapidly transforming our world, revolutionizing industries and enhancing everyday life. However, as we embrace these advancements, we must also navigate the murky waters of AI ethics. It's not just about building smarter systems; it's about ensuring that these systems operate within a framework that respects human rights and societal norms. So, how do we steer clear of the ethical pitfalls that can arise in AI development? Let's dive in!
At its core, AI ethics is about understanding the implications of the technology we create. The decisions made during the development of AI can have profound effects on individuals and communities. For example, consider a self-driving car. If the algorithms prioritize speed over safety, the consequences could be catastrophic. This scenario exemplifies why ethical considerations must be at the forefront of AI development. We need to ask ourselves: are we creating technology that benefits everyone, or are we inadvertently reinforcing existing inequalities?
Moreover, the conversation around AI ethics is not just for developers and tech companies; it extends to policymakers, consumers, and society as a whole. Each stakeholder plays a crucial role in shaping the ethical landscape of AI. By fostering an environment of trust, accountability, and transparency, we can ensure that AI serves the greater good. It’s like building a bridge: every piece must fit together perfectly to support the weight of what we are trying to achieve.
As we navigate this complex terrain, we must remain vigilant against common ethical pitfalls. These include issues like bias, privacy violations, and accountability challenges. Recognizing these pitfalls is essential for mitigating risks and enhancing ethical practices. For instance, biased algorithms can lead to unfair treatment of certain groups, while privacy violations can erode public trust in AI systems. It's crucial to identify these risks early on and implement strategies to address them effectively.
In summary, steering clear of pitfalls in AI ethics requires a collective effort. By prioritizing ethical considerations in every stage of AI development, we can harness the power of technology to create a future that is equitable and just for all. So, let’s embark on this journey together, ensuring that our innovations reflect the values we cherish as a society.
- What is AI ethics? AI ethics refers to the moral implications and responsibilities associated with the development and deployment of artificial intelligence technologies.
- Why is AI ethics important? It is essential for building trust, ensuring fairness, and safeguarding individual rights in the use of AI systems.
- What are common pitfalls in AI ethics? Common pitfalls include bias in algorithms, privacy concerns, and lack of accountability in AI development.
- How can organizations ensure ethical AI practices? Organizations can adopt best practices such as stakeholder engagement, transparency, and continuous evaluation of AI systems.
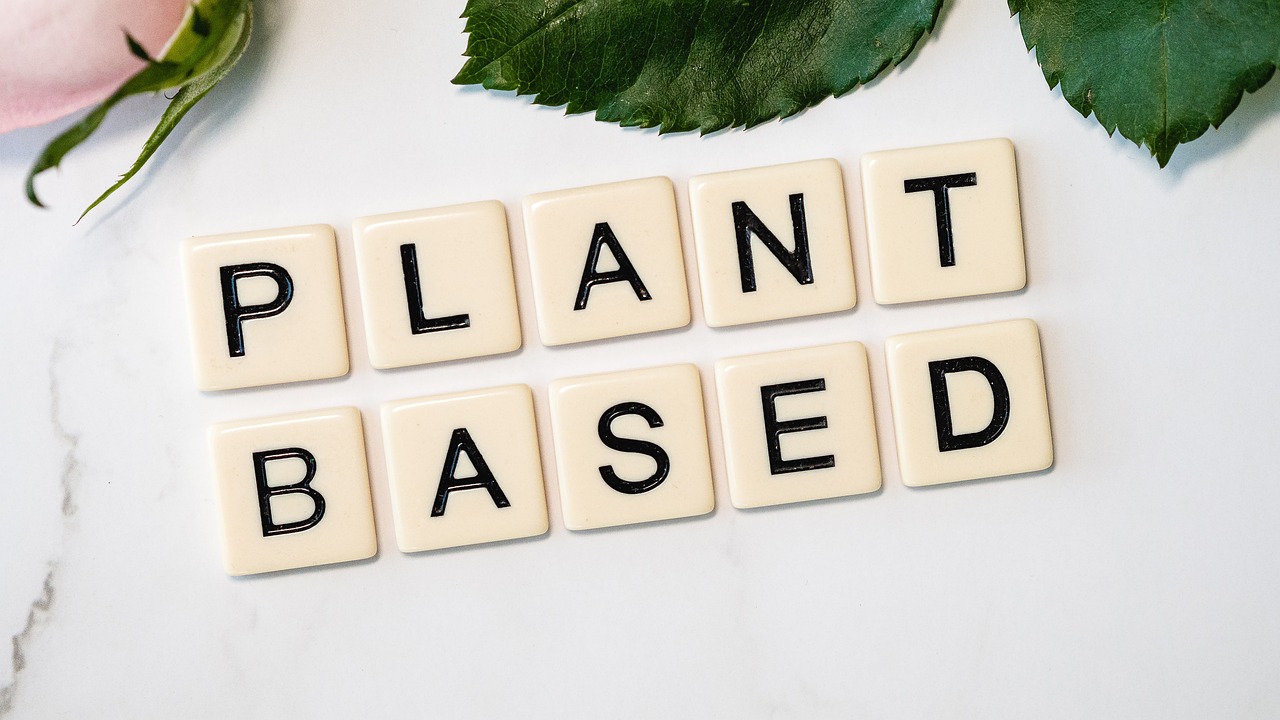
The Importance of AI Ethics
In today's rapidly evolving technological landscape, the significance of AI ethics cannot be overstated. As artificial intelligence becomes increasingly integrated into our daily lives, it is essential for developers and organizations to prioritize ethical considerations. Why is this so crucial, you might ask? Well, think of AI systems as powerful tools that can either uplift society or create chaos, much like a double-edged sword. When wielded responsibly, they can enhance productivity, streamline processes, and even solve complex problems. However, if mismanaged, they can lead to detrimental consequences that affect individuals and communities alike.
Fostering trust, accountability, and transparency in AI systems is not just a moral obligation; it's a necessity. When users know that their data is handled ethically and that decisions made by AI are fair, they are more likely to embrace these technologies. This trust is the bedrock of successful AI deployment. Imagine walking into a store where the cashier uses facial recognition to identify you. If you knew that this system respected your privacy and operated without bias, wouldn’t you feel more comfortable? This is the essence of ethical AI—creating systems that users can trust.
Moreover, the societal benefits of ethical AI practices extend beyond individual trust. They encourage innovation and growth within organizations. When companies adopt ethical guidelines, they not only protect their users but also enhance their reputation. This positive perception can lead to increased customer loyalty and even attract new clients who value responsible practices. In essence, ethical AI is not just about avoiding pitfalls; it’s about paving the way for a brighter, more equitable future.
To illustrate the importance of AI ethics, consider the following table that highlights the key benefits:
Benefit | Description |
---|---|
Trust | Users feel secure knowing their data is handled responsibly. |
Accountability | Organizations take responsibility for the outcomes of their AI systems. |
Transparency | Clear communication about how AI systems operate fosters understanding. |
Innovation | Ethical practices encourage new ideas and solutions in AI development. |
In conclusion, the importance of AI ethics cannot be ignored. As we continue to advance into an era dominated by technology, the choices we make today will shape the future of AI and its impact on society. By prioritizing ethical considerations, we can ensure that AI serves as a force for good, benefiting all members of society rather than creating divides. So, let’s commit to making ethical AI a priority, because the future is in our hands.

Common Ethical Pitfalls in AI
In the rapidly evolving landscape of artificial intelligence, ethical pitfalls are not just minor inconveniences; they can have profound implications for individuals and society as a whole. As developers and organizations dive headfirst into AI projects, it's crucial to recognize these potential hazards. Ignoring them is akin to sailing a ship without a compass—you're bound to get lost in uncharted waters.
One of the most pressing issues is bias in AI algorithms. Bias can creep into systems in various ways, often leading to unfair treatment of certain groups. Imagine a scenario where an AI system designed to screen job applicants inadvertently favors candidates from a particular demographic, simply because of biased training data. This not only undermines the integrity of the hiring process but can also perpetuate systemic inequalities. To combat this, it's essential to actively identify and address bias at every stage of AI development.
Another significant pitfall is the issue of privacy. With AI systems increasingly handling sensitive personal data, the risk of privacy violations looms large. For instance, consider a healthcare AI that processes patient records. If not properly secured, this information could be exposed, leading to severe repercussions for individuals and organizations alike. Protecting user data isn't just a regulatory requirement; it's a fundamental aspect of maintaining trust between users and AI systems.
Moreover, the lack of accountability in AI development can create a dangerous environment where no one takes responsibility for the outcomes of AI systems. Imagine a self-driving car that causes an accident. Who is to blame—the manufacturer, the software developer, or the user? Establishing clear lines of accountability is essential to ensure that ethical standards are upheld and that users' rights are protected.
To summarize, the common ethical pitfalls in AI can be categorized as follows:
- Bias in AI Algorithms: Unfair treatment due to biased training data.
- Privacy Violations: Risks associated with handling sensitive personal data.
- Lack of Accountability: Unclear responsibility for AI system outcomes.
Recognizing these pitfalls is the first step toward mitigating risks and enhancing ethical practices in AI. By being aware of these challenges, developers and organizations can take proactive measures to ensure their AI systems are not only effective but also fair and trustworthy. The journey toward ethical AI is ongoing, and it requires vigilance, collaboration, and a commitment to doing what’s right.
- What is AI bias? AI bias occurs when an algorithm produces unfair outcomes due to prejudiced training data or design flaws.
- How can organizations mitigate bias in AI? By employing diverse data sets, utilizing bias detection tools, and continuously monitoring AI systems.
- Why is privacy important in AI? Protecting user privacy is crucial to maintain trust and comply with legal regulations.
- What does accountability in AI development mean? It refers to the responsibility of organizations to oversee and manage the ethical implications of their AI systems.

Bias in AI Algorithms
Bias in AI algorithms is a critical issue that can lead to **unjust outcomes** and **inequitable treatment** of individuals or groups. Just imagine a world where your chances of getting a job, a loan, or even healthcare depend on algorithms that have been skewed by biases. It's not just a tech problem; it's a societal one. When AI systems are trained on data that reflects historical prejudices or societal inequalities, they can perpetuate these biases, often without anyone even realizing it. This can create a cycle of discrimination that is hard to break.
One of the primary reasons bias creeps into AI is the **data** used for training. If the data contains biased information, the AI will learn from it and replicate those biases in its decision-making processes. For instance, if an AI system is trained on hiring data that favors a specific demographic, it will likely continue to favor that demographic in future hiring decisions. This is not just theoretical; there have been real-world examples where AI algorithms have favored one group over another, leading to significant backlash and calls for reform.
Understanding the sources of bias is essential for developers who want to create fair AI systems. Bias can originate from several areas:
- Biased Training Data: Data that reflects existing inequalities or stereotypes can lead to biased outcomes.
- Flawed Assumptions: Developers may unknowingly introduce their own biases through assumptions made during the design process.
- Systemic Inequalities: Societal biases that exist outside of technology can seep into AI systems if not carefully monitored and addressed.
To combat bias in AI algorithms, it’s crucial to implement strategies that promote fairness. This involves:
- Diverse Data Collection: Actively seeking out diverse data sources can help mitigate bias. This means not just collecting data from a homogeneous group but ensuring that various demographics are represented.
- Bias Detection Techniques: Utilizing algorithms that can detect bias in existing models is essential. Regular audits can help identify and rectify biased outcomes.
- Continuous Monitoring: AI systems should not be set and forgotten. Continuous evaluation is necessary to ensure they remain fair and equitable over time.
In conclusion, addressing bias in AI algorithms is not merely a technical challenge; it’s a moral imperative. As we continue to integrate AI into various aspects of our lives, we must prioritize fairness and equity. Only then can we ensure that AI serves as a tool for **empowerment** rather than a mechanism for **discrimination**.
Q: What is bias in AI?
A: Bias in AI refers to systematic favoritism or discrimination that occurs when AI algorithms produce unfair outcomes based on flawed data or assumptions.
Q: How can bias in AI be detected?
A: Bias can be detected through various techniques, including algorithmic audits and statistical analyses that reveal disparities in outcomes across different demographic groups.
Q: Why is it important to mitigate bias in AI?
A: Mitigating bias is crucial to ensure fairness, accountability, and trust in AI systems, which ultimately benefits society as a whole by promoting equitable outcomes.
Q: Can bias in AI be completely eliminated?
A: While it may be challenging to completely eliminate bias, it can be significantly reduced through careful design, diverse data collection, and ongoing monitoring.

Sources of Bias
When we delve into the in AI, it's essential to recognize that bias doesn't just appear out of thin air; it often stems from various underlying factors. Imagine bias as a shadow that follows us around, shaped by the light of our experiences, data, and societal norms. In the world of AI, this shadow can become particularly problematic if not addressed properly. Let's explore some of the primary sources of bias that can infiltrate AI systems.
One significant source of bias is biased training data. AI systems learn from the data they're fed, and if that data contains biases—whether intentional or unintentional—the AI will likely replicate those biases in its decision-making processes. For instance, if an AI model is trained on historical hiring data from a company that has predominantly hired a specific demographic, it may inadvertently favor candidates from that group in future hiring decisions. This can perpetuate systemic inequalities and unfair treatment.
Another source is flawed assumptions made during the development of AI algorithms. Developers may unconsciously introduce their own biases into the algorithms they create. This could be due to a lack of diversity in the development team or simply because certain assumptions about the data or the problem being solved are taken for granted. Such assumptions can lead to skewed outcomes that do not reflect the broader population accurately.
Moreover, systemic inequalities present in society can also seep into AI systems. These inequalities often manifest in various forms, such as economic disparities, social prejudices, or even cultural biases. When AI systems are developed without considering these factors, they can inadvertently reinforce existing societal biases, making it crucial for developers to be vigilant and proactive in identifying and mitigating these influences.
To better understand these sources, consider the following table that summarizes them:
Source of Bias | Description |
---|---|
Biased Training Data | Data that reflects existing prejudices or inequalities, leading to skewed AI outcomes. |
Flawed Assumptions | Unconscious biases introduced by developers, affecting algorithm design and implementation. |
Systemic Inequalities | Societal biases that influence data and outcomes, perpetuating existing disparities. |
Addressing these sources of bias is not just a technical challenge; it's a moral imperative. By acknowledging and understanding where biases originate, developers can take significant steps toward creating more equitable and fair AI systems. This process requires a commitment to diversity in data collection, ongoing training for developers, and an openness to reevaluating existing assumptions. After all, in the quest for ethical AI, recognizing our own biases is the first step toward dismantling them.
- What is bias in AI? Bias in AI refers to systematic favoritism or prejudice in the outcomes produced by AI systems, often due to biased training data or flawed assumptions.
- How can we mitigate bias in AI? Mitigating bias involves diverse data collection, implementing bias detection techniques, and continuous monitoring of AI systems.
- Why is it important to address bias in AI? Addressing bias is crucial for ensuring fairness, accountability, and trust in AI applications, ultimately benefiting society as a whole.

Mitigating Bias
Mitigating bias in AI systems is not just a technical challenge; it's a moral imperative that can shape the future of technology and society. To effectively tackle bias, organizations must adopt a multifaceted approach that encompasses various strategies and practices. First and foremost, it’s essential to ensure that the data used to train AI models is representative of the diverse population it intends to serve. This means actively seeking out and including data from underrepresented groups to avoid skewed outcomes that could harm these communities.
One effective method for identifying bias is through bias detection techniques. These techniques can include statistical analysis to uncover disparities in how different groups are treated by the AI system. For instance, if an AI model is used for hiring and shows a preference for candidates from a particular demographic, it’s crucial to investigate the underlying data and algorithms. By employing tools such as fairness metrics, organizations can quantify bias and take corrective actions before deploying their AI systems.
Additionally, continuous monitoring of AI systems is vital. Bias is not a static issue; it can evolve as societal norms and values change. Therefore, organizations should implement a framework for ongoing evaluation that includes regular audits of AI systems. This proactive approach not only helps in identifying emerging biases but also fosters a culture of accountability. Here are some strategies that can be employed:
- Diverse Data Collection: Actively seek out data from various demographics, ensuring that no group is disproportionately represented.
- Regular Audits: Conduct periodic reviews of AI systems to assess for bias and make necessary adjustments.
- Stakeholder Feedback: Engage with diverse stakeholders to gain insights and perspectives that might highlight potential biases.
Moreover, involving ethicists and social scientists in the AI development process can provide invaluable insights into the potential societal impacts of AI technologies. These experts can help identify blind spots and suggest strategies that promote fairness and inclusivity. Ultimately, the goal is to create AI systems that not only perform efficiently but also uphold the values of equity and justice.
In summary, mitigating bias in AI is a continuous journey that requires commitment, vigilance, and collaboration. By implementing these strategies, organizations can work towards building AI systems that are not only effective but also ethical, ensuring that technology serves everyone fairly.
Q1: What is bias in AI?
A1: Bias in AI refers to systematic favoritism or prejudice in algorithms that can lead to unfair treatment of individuals or groups based on race, gender, or other characteristics.
Q2: How can organizations identify bias in their AI systems?
A2: Organizations can identify bias by using statistical analysis, fairness metrics, and conducting audits to assess how different groups are treated by their AI models.
Q3: Why is it important to mitigate bias in AI?
A3: Mitigating bias is crucial to ensure that AI systems are fair, equitable, and do not perpetuate existing inequalities, thereby fostering trust and accountability in technology.
Q4: What role do stakeholders play in mitigating AI bias?
A4: Engaging diverse stakeholders helps to bring multiple perspectives into the AI development process, ensuring that ethical implications are thoroughly examined and considered.

Privacy Concerns in AI
In the rapidly evolving landscape of artificial intelligence, privacy concerns have emerged as a critical issue that cannot be overlooked. As AI systems increasingly handle vast amounts of sensitive data—from personal information to behavioral patterns—the risk of privacy violations grows exponentially. Imagine a world where your every online move is tracked, analyzed, and potentially exploited. This scenario isn’t far from reality, and it raises a plethora of questions about how we can safeguard our personal information while still benefiting from the advancements AI offers.
One of the primary concerns is that AI systems often rely on large datasets to train their algorithms. These datasets can inadvertently include sensitive personal information, leading to unintended consequences. For instance, if an AI system is trained on data that contains identifiable information, there’s a risk that it could expose that data during its operation. Moreover, the lack of transparency in how data is collected, processed, and shared further exacerbates these privacy issues.
To illustrate the gravity of the situation, consider the following points:
- Data Breaches: High-profile data breaches have shown us that even the most secure systems can be compromised. When AI systems are involved, the impact can be even more significant due to the sensitive nature of the data they handle.
- Informed Consent: Many users are unaware of how their data is being used in AI applications. The concept of informed consent becomes murky when data is collected without clear communication about its purpose and potential risks.
- Surveillance and Monitoring: AI technologies, such as facial recognition, can lead to invasive surveillance practices. This not only raises ethical questions but also poses a threat to individual freedoms and privacy.
Furthermore, the implications of AI on privacy are not just limited to individual users. Organizations must also grapple with compliance to regulations such as the General Data Protection Regulation (GDPR) in Europe, which mandates strict guidelines on data handling and user privacy. Failing to comply can result in hefty fines and damage to an organization's reputation.
In response to these challenges, it’s essential for developers and companies to prioritize data protection measures throughout the AI development lifecycle. This includes implementing robust encryption methods, conducting regular audits, and ensuring that data collection practices are transparent and ethical. By fostering a culture of privacy awareness, organizations can build trust with their users and mitigate the risks associated with AI.
As we continue to explore the potential of AI, we must remain vigilant about the privacy implications. It’s not just about creating powerful algorithms; it’s about doing so responsibly. The future of AI can be bright, but only if we navigate the privacy concerns with care and consideration.
Q1: What are the main privacy concerns associated with AI?
A1: The main privacy concerns include data breaches, lack of informed consent, and invasive surveillance practices. These issues arise from the extensive data collection and processing that AI systems require.
Q2: How can organizations ensure user privacy when developing AI systems?
A2: Organizations can ensure user privacy by implementing robust data protection measures, being transparent about data usage, and adhering to regulations like GDPR.
Q3: Why is informed consent important in AI?
A3: Informed consent is crucial because it ensures that users understand how their data will be used, promoting trust and accountability in AI systems.

Accountability in AI Development
Establishing accountability in AI development is not just a buzzword; it’s a necessity in today’s tech-driven world. As organizations increasingly rely on artificial intelligence to make decisions that impact lives, the question arises: who is responsible when things go wrong? This is a crucial consideration that can’t be overlooked. When AI systems malfunction or produce biased outcomes, the consequences can be severe, ranging from loss of trust to legal repercussions. Therefore, it is imperative that organizations not only acknowledge their responsibility but also actively implement measures to ensure accountability throughout the AI lifecycle.
To achieve this, organizations must develop comprehensive frameworks that clarify roles and responsibilities. Imagine a well-oiled machine where every cog knows its function; this is what accountability in AI should resemble. By creating clear guidelines, organizations can establish a culture of responsibility that permeates all levels of AI development. This means that everyone involved, from data scientists to executives, understands their part in ensuring ethical AI practices. Accountability should not be an afterthought; it should be integrated into the very fabric of AI development processes.
One effective approach to fostering accountability is through the implementation of audit trails. These are systematic records that track the decision-making processes of AI systems. By maintaining detailed logs, organizations can provide transparency regarding how AI decisions are made. This not only helps in identifying potential issues but also builds trust with users who may be wary of automated systems. When users know that their data and decisions are being handled responsibly, they are more likely to engage with AI technologies.
Moreover, organizations should consider incorporating regular ethical audits into their AI development processes. These audits can serve as checkpoints to evaluate whether AI systems are operating within ethical boundaries. During these audits, organizations can assess various aspects, such as:
- The fairness of algorithms
- Compliance with privacy regulations
- The impact of AI decisions on different demographic groups
By conducting these audits, organizations can proactively identify potential risks and take corrective actions before issues escalate. This not only protects users but also shields the organization from potential backlash.
Finally, accountability in AI development also requires fostering a culture of open communication. Encouraging team members to voice concerns and share insights can lead to a more ethical development process. When individuals feel empowered to speak up, it creates an environment where ethical considerations are at the forefront of AI development. This culture can be reinforced through training programs that emphasize the importance of ethics in technology, ensuring that everyone is aligned with the organization's values.
In conclusion, accountability in AI development is essential for fostering trust and ensuring ethical practices. By implementing clear frameworks, maintaining audit trails, conducting regular ethical audits, and promoting open communication, organizations can navigate the complexities of AI responsibly. This not only protects users but also enhances the overall integrity of AI systems, paving the way for a future where technology serves humanity ethically and effectively.
- What is accountability in AI development? Accountability in AI development refers to the responsibility organizations have in ensuring that their AI systems operate ethically and transparently, addressing any issues that may arise.
- Why is accountability important? Accountability is crucial because it helps build trust with users, mitigates risks, and ensures that AI systems are developed and deployed in a responsible manner.
- How can organizations ensure accountability? Organizations can ensure accountability by creating clear guidelines, maintaining audit trails, conducting ethical audits, and fostering a culture of open communication.

Frameworks for Accountability
Establishing accountability in AI development is not just a good practice; it's a necessity. As AI systems become increasingly integrated into our daily lives, the consequences of their decisions can be profound. This is where come into play. They serve as a structured approach to ensure that organizations take responsibility for their AI systems, promoting ethical practices and protecting users' rights.
To create effective accountability frameworks, organizations need to focus on three key components: clear guidelines, defined roles, and responsibilities. These elements help in delineating who is accountable at various stages of AI development and deployment. For instance, having a dedicated ethics board can oversee AI projects, ensuring that ethical considerations are front and center throughout the process. This board can also serve as a point of contact for stakeholders, fostering transparency and trust.
Moreover, organizations should implement regular audits of their AI systems. These audits can help identify any ethical lapses or unintended consequences that may arise from AI decisions. By being proactive, companies can address issues before they escalate, thus maintaining user trust and compliance with legal standards. A well-structured audit process might look something like this:
Audit Phase | Description |
---|---|
Preparation | Gather relevant data and set objectives for the audit. |
Execution | Conduct the audit, analyzing AI outputs and decision-making processes. |
Reporting | Document findings and recommendations for improvement. |
Follow-up | Implement changes and monitor for effectiveness. |
Another vital aspect of accountability frameworks is stakeholder engagement. Involving diverse stakeholders, including ethicists, technologists, and end-users, can provide a broader perspective on the ethical implications of AI systems. This engagement can take the form of workshops, discussions, or public forums, where various viewpoints can be explored and considered. By integrating feedback from a wide range of sources, organizations can better anticipate the societal impact of their AI technologies.
Ultimately, the goal of these frameworks is to create a culture of accountability within organizations. When everyone understands their role in upholding ethical standards, it fosters a sense of ownership and responsibility. This culture can be reinforced through training programs that educate employees about ethical AI practices, ensuring that accountability is not just a checkbox but a fundamental aspect of the organization's ethos.
- What is the importance of accountability in AI? Accountability in AI ensures that organizations take responsibility for their systems, promoting ethical practices and protecting users' rights.
- How can organizations implement accountability frameworks? Organizations can implement accountability frameworks by establishing clear guidelines, defining roles and responsibilities, and conducting regular audits of their AI systems.
- Why is stakeholder engagement crucial in AI development? Engaging stakeholders provides diverse perspectives, helping organizations to better understand the ethical implications of their AI technologies.

Best Practices for Ethical AI
In the ever-evolving landscape of artificial intelligence, adhering to best practices is not just a recommendation; it's a necessity. As organizations strive to innovate and leverage the power of AI, they must also navigate the ethical complexities that come with it. So, how can we ensure that AI serves humanity positively and responsibly? The answer lies in a combination of transparency, stakeholder engagement, and continuous evaluation.
First and foremost, transparency is key. When AI systems operate like a black box, it creates an environment of distrust. Users and stakeholders need to understand how decisions are made. This means organizations should be open about their algorithms, data sources, and the rationale behind their AI models. By providing clear documentation and explanations, companies can demystify AI processes, making it easier for everyone to grasp the implications of AI decisions. Imagine trying to solve a puzzle without knowing what the picture looks like; that’s how users feel when AI lacks transparency.
Next, engaging stakeholders throughout the AI development process is crucial. This includes not just developers and engineers, but also ethicists, legal experts, and representatives from diverse communities. By fostering a collaborative environment, organizations can gather a wider array of perspectives, ensuring that ethical implications are thoroughly examined. For instance, consider a healthcare AI that predicts patient outcomes. Involving medical professionals, patients, and ethicists in the design process can help identify potential biases and improve the overall effectiveness of the system. Stakeholder engagement acts as a safety net, catching potential ethical oversights before they escalate into significant issues.
Moreover, the practice of continuous evaluation cannot be overlooked. AI systems should not be set and forgotten; they require ongoing scrutiny to adapt to changing societal norms and values. This means regularly revisiting the data used for training, the outcomes produced, and the feedback from users. Organizations can implement a feedback loop where users can report issues or concerns, allowing developers to make necessary adjustments. To illustrate, think of a gardener who regularly prunes and waters their plants. Without this care, the garden may become overgrown with weeds, much like an AI system that becomes outdated or biased without proper maintenance.
In addition to these principles, organizations should also consider establishing a set of ethical guidelines tailored to their specific AI applications. This can include:
- Conducting regular audits of AI systems to check for bias and ethical compliance.
- Providing training for employees on ethical AI development practices.
- Creating an ethics board to oversee AI projects and ensure adherence to ethical standards.
By integrating these best practices into their AI development processes, organizations can not only mitigate risks but also enhance the overall impact of their AI systems. The goal is to build AI that is not only efficient but also equitable, fostering a future where technology and ethics go hand in hand.
Q: Why is transparency important in AI?
A: Transparency helps build trust with users by allowing them to understand how AI systems make decisions and the data they rely on. It reduces the fear of the unknown and promotes accountability.
Q: How can organizations engage stakeholders effectively?
A: Organizations can engage stakeholders by forming diverse teams that include ethicists, community representatives, and industry experts. Regular meetings, workshops, and feedback sessions can facilitate open communication and collaboration.
Q: What should be included in continuous evaluation?
A: Continuous evaluation should involve regular audits of AI systems, user feedback collection, and updates to algorithms and data sources to ensure fairness and relevance over time.
Q: How can ethical guidelines be established for AI?
A: Ethical guidelines can be established by reviewing existing frameworks, consulting with experts, and tailoring them to the organization's specific AI applications, ensuring they address potential ethical challenges unique to their context.

Engaging Stakeholders
Engaging stakeholders in the AI development process is not just a good practice; it’s a necessity for fostering ethical AI systems. Imagine building a house without consulting an architect or the people who will live in it. It’s a recipe for disaster! Similarly, when developing AI technologies, involving a diverse group of stakeholders ensures that multiple perspectives are considered, which can lead to more balanced and responsible outcomes.
Stakeholders can include a variety of individuals and groups such as end-users, industry experts, ethicists, and community representatives. Each of these voices brings unique insights that can illuminate potential ethical implications and unintended consequences of AI systems. For instance, a developer might focus solely on efficiency and functionality, while a community representative might highlight potential social impacts or privacy concerns that could arise from an AI application.
To facilitate effective engagement, organizations should create structured opportunities for stakeholder involvement. This can take the form of workshops, focus groups, or public forums where stakeholders can voice their opinions and concerns. By actively listening to these diverse viewpoints, organizations can not only identify ethical issues early in the development process but also build trust with the communities they serve. After all, trust is the cornerstone of any successful relationship, and in the realm of AI, it’s essential for user acceptance and compliance.
Moreover, organizations should consider establishing a Stakeholder Advisory Board. This board can serve as a continuous feedback loop, providing ongoing insights and recommendations throughout the AI lifecycle. The table below illustrates the potential composition and roles of such a board:
Stakeholder Group | Role |
---|---|
End-users | Provide insights on usability and real-world applications. |
Ethicists | Identify potential ethical dilemmas and suggest frameworks for resolution. |
Industry Experts | Offer knowledge on technical feasibility and market implications. |
Community Representatives | Bring in the concerns and needs of the broader community affected by AI. |
In conclusion, engaging stakeholders is not just a checkbox on a project management list; it’s a vital component of ethical AI development. By fostering a culture of collaboration and transparency, organizations can better navigate the complex landscape of AI ethics and ensure that their technologies serve the greater good. After all, the ultimate goal of AI should be to enhance human capabilities and improve lives, not to create new challenges or exacerbate existing ones.
- Why is stakeholder engagement important in AI development?
Engaging stakeholders ensures diverse perspectives are considered, which helps identify potential ethical issues and fosters trust. - Who should be involved as stakeholders?
Stakeholders can include end-users, ethicists, industry experts, and community representatives, among others. - How can organizations effectively engage stakeholders?
Organizations can hold workshops, focus groups, and public forums to facilitate open dialogue and feedback. - What is a Stakeholder Advisory Board?
A Stakeholder Advisory Board is a group that provides ongoing insights and recommendations throughout the AI development lifecycle.
Frequently Asked Questions
- What is AI ethics and why is it important?
AI ethics refers to the moral principles and guidelines that govern the development and deployment of artificial intelligence technologies. It's important because it fosters trust, accountability, and transparency in AI systems, ensuring that these technologies benefit society while minimizing risks and harms.
- What are some common ethical pitfalls in AI?
Common ethical pitfalls in AI include bias, privacy violations, and a lack of accountability. These challenges can lead to unfair treatment, unauthorized data usage, and a failure to take responsibility for AI outcomes, making it crucial to identify and address them.
- How does bias manifest in AI algorithms?
Bias in AI algorithms can manifest in various ways, such as through biased training data or flawed assumptions made during development. This can result in unfair treatment of individuals or groups, highlighting the need for developers to actively recognize and mitigate these biases.
- What are some sources of bias in AI?
Sources of bias can include biased training data, flawed assumptions, and existing systemic inequalities. Understanding these sources is essential for creating fairer AI systems that do not perpetuate discrimination or unfair practices.
- How can organizations mitigate bias in AI?
Organizations can mitigate bias by employing strategies such as diverse data collection, utilizing bias detection techniques, and engaging in continuous monitoring of AI systems to promote fairness and inclusivity throughout the development process.
- What privacy concerns are associated with AI?
AI systems often handle sensitive data, raising significant privacy concerns. Safeguarding user information is paramount to maintaining trust and compliance with regulations, as mishandling data can lead to serious consequences for users and organizations alike.
- Why is accountability important in AI development?
Establishing accountability in AI development ensures that organizations take responsibility for the consequences of their AI systems. This promotes ethical practices and protects users' rights, creating a safer and more trustworthy environment for AI applications.
- What frameworks can be developed for accountability in AI?
Frameworks for accountability involve creating clear guidelines, defining roles, and establishing responsibilities within organizations. This ensures that ethical AI practices are upheld throughout the development lifecycle, fostering a culture of responsibility.
- What are some best practices for ensuring ethical AI?
Best practices for ethical AI include promoting transparency, engaging with stakeholders, and conducting continuous evaluations. These practices help organizations navigate ethical challenges effectively and responsibly, leading to better outcomes for all.
- How can stakeholders be engaged in the AI development process?
Engaging diverse stakeholders in the AI development process allows for a broader perspective, ensuring that various viewpoints are considered. This involvement helps to thoroughly examine the ethical implications of AI technologies and promotes more responsible development.