Artificial Intelligence and Ethics: A New Frontier
In today's rapidly evolving technological landscape, the intersection of artificial intelligence (AI) and ethics has become a topic of profound significance. As AI systems are increasingly integrated into various aspects of our daily lives—from healthcare to finance and beyond—the ethical implications of these technologies are coming to the forefront. Imagine a world where machines not only make decisions but also influence the very fabric of our society. It’s both exhilarating and daunting, isn't it? The potential for AI to enhance human capabilities is immense, yet it raises a plethora of questions about responsibility, fairness, and transparency.
As we delve deeper into this new frontier, we must ask ourselves: What happens when algorithms make choices that affect our lives? Who is accountable when these systems fail or exhibit bias? The answers are not straightforward, but they are critical to ensuring that AI serves humanity positively. The ethical considerations surrounding AI are not just theoretical; they have real-world implications that can shape our future. For instance, consider the role of AI in hiring processes. If an algorithm is trained on biased data, it may inadvertently perpetuate existing inequalities, leading to unfair treatment of candidates. This scenario underscores the urgent need for ethical guidelines and frameworks to govern AI development.
Moreover, the conversation around AI ethics is not limited to developers and policymakers; it involves every one of us. Users must be informed and empowered to understand how these technologies operate and the potential risks they carry. The more we engage in discussions about AI ethics, the better equipped we will be to navigate the challenges ahead. It’s like embarking on a journey into uncharted territory; the path may be fraught with obstacles, but with collective awareness and action, we can steer towards a future where AI enhances human life rather than detracts from it.
Understanding why ethical considerations are crucial in AI development helps ensure technologies serve humanity positively, fostering trust and accountability among users and developers alike. As we explore this topic, it becomes evident that ethical AI is not just a buzzword; it's a necessity. Without a solid ethical foundation, the deployment of AI could lead to unintended consequences that may harm individuals and society at large.
This section outlines fundamental ethical principles, such as fairness, transparency, and accountability, that should guide the development and deployment of artificial intelligence systems. These principles serve as a compass, directing developers and organizations towards responsible AI practices.
Examining how biases can be inadvertently introduced into AI algorithms, this section discusses the importance of fairness in decision-making processes and the steps needed to mitigate bias. Fairness is not just a desirable trait; it is essential for building trust in AI systems.
This subheading focuses on methods to identify and address biases present in training datasets, emphasizing the need for diverse and representative data to improve AI outcomes. A diverse dataset is akin to a well-rounded education; it equips AI systems with the knowledge to make informed decisions that reflect the complexities of real-world scenarios.
Here, we explore various techniques and frameworks designed to enhance algorithmic fairness, ensuring that AI systems treat all individuals equitably regardless of background. These techniques are crucial in building AI systems that not only perform well but also uphold ethical standards.
This section delves into the significance of transparency in AI systems, highlighting the need for explainable AI to foster user understanding and trust in automated decisions. When users can see how decisions are made, they are more likely to trust the technology, leading to broader acceptance and integration of AI solutions in society.
Discussing the critical issue of accountability, this section emphasizes the need for clear responsibility structures in AI development and deployment to address ethical concerns effectively. Accountability is not just about assigning blame when things go wrong; it’s about creating a culture of responsibility that encourages ethical practices from the outset.
Exploring existing and proposed regulatory frameworks, this subheading discusses how legislation can guide ethical AI practices and protect user rights in a rapidly evolving technological landscape. Regulatory frameworks are like guardrails on a highway; they help keep the development of AI on a safe and ethical path.
This section highlights the role of companies in ensuring ethical AI practices, advocating for corporate responsibility and ethical guidelines to govern AI development and usage. Companies must recognize that with great power comes great responsibility, and they should strive to lead by example in the ethical deployment of AI technologies.
As AI technology evolves, new ethical challenges will emerge. This section anticipates future dilemmas and the importance of ongoing dialogue and adaptation in ethical AI practices. The journey of ethical AI is ongoing, and it requires continuous reflection and adaptation to meet the challenges of a changing world.
- What is ethical AI? Ethical AI refers to the development and deployment of artificial intelligence systems that prioritize fairness, accountability, and transparency.
- Why is fairness important in AI? Fairness is crucial to ensure that AI systems do not perpetuate biases and treat all individuals equitably.
- How can we ensure AI systems are transparent? By implementing explainable AI techniques, we can help users understand how decisions are made by AI systems.
- What role do companies play in AI ethics? Companies are responsible for establishing ethical guidelines and practices to govern the development and use of AI technologies.
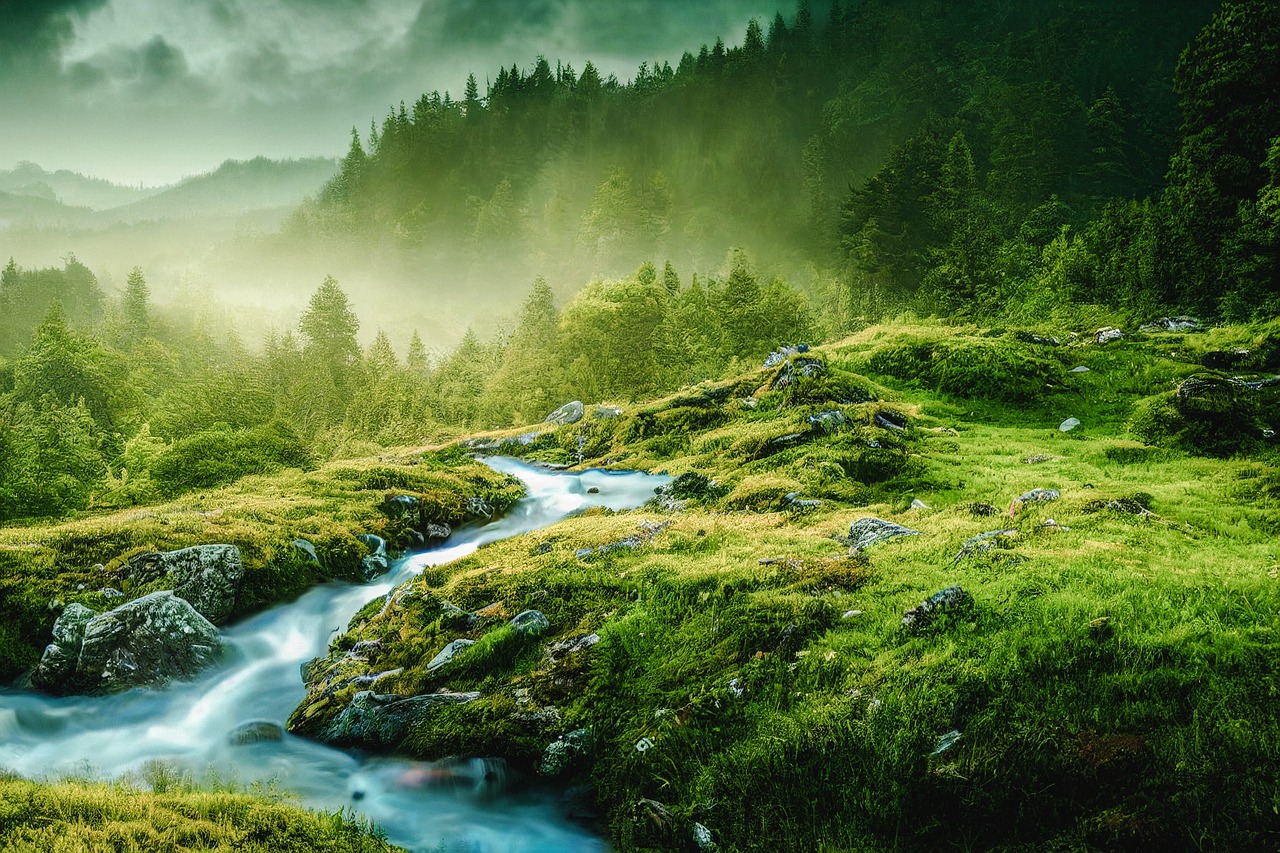
The Importance of Ethical AI
In today's rapidly advancing technological landscape, the significance of ethical AI cannot be overstated. As artificial intelligence becomes increasingly integrated into our daily lives, it is crucial to understand why ethical considerations are at the forefront of AI development. Imagine a world where machines make decisions that directly impact our lives—everything from hiring practices to healthcare diagnostics. Wouldn't you want to ensure that these decisions are made fairly and transparently? This is where the importance of ethical AI comes into play.
Ethical AI serves as a guiding principle, ensuring that technologies are developed and utilized in ways that positively impact society. It fosters trust and accountability among users and developers alike, acting as a safeguard against potential misuse or unintended consequences. Without a strong ethical framework, there's a risk that AI technologies could perpetuate existing biases, infringe on privacy, or even lead to harmful outcomes. Therefore, embracing ethical AI is not just a moral obligation; it is essential for the sustainable growth of technology in society.
Moreover, ethical AI encourages collaboration and dialogue among stakeholders. This includes developers, policymakers, and the general public. By engaging in open discussions about the implications of AI, we can collectively shape a future where technology serves humanity's best interests. It’s like building a bridge—everyone must contribute to ensure it stands strong and safe. Just as a bridge needs solid foundations, so too does AI need ethical principles to support its development and deployment.
To illustrate the importance of ethical AI, consider the following key aspects:
- Promotes Fairness: Ethical AI aims to eliminate biases that can arise from flawed algorithms or unrepresentative training data.
- Enhances Transparency: By making AI systems more understandable, users can trust the decisions made by these technologies.
- Encourages Accountability: Establishing clear responsibility structures ensures that developers and organizations are held accountable for their AI systems.
In summary, the importance of ethical AI cannot be ignored. As we continue to innovate and push the boundaries of what is possible with artificial intelligence, we must prioritize ethical considerations to ensure that these advancements benefit society as a whole. By doing so, we not only protect individual rights but also pave the way for a future where technology and humanity coexist harmoniously.

Key Ethical Principles in AI
As we delve into the realm of artificial intelligence, it's imperative to anchor our discussions around key ethical principles that guide the development and deployment of these technologies. Without a solid ethical foundation, AI can easily veer off course, leading to unintended consequences that could harm individuals and society. So, what are these principles, and why do they matter? Let's explore the essential pillars of ethical AI: fairness, transparency, and accountability.
Fairness is the cornerstone of ethical AI. Imagine a world where algorithms make decisions about hiring, lending, or even sentencing without bias. Unfortunately, the reality is that biases can seep into AI systems, often reflecting historical inequalities. This is not just a technical issue; it’s a moral one. For instance, if an AI system trained on biased data decides who gets a job, it could perpetuate systemic discrimination. Therefore, ensuring fairness in AI means actively working to eliminate these biases and create systems that treat all individuals equitably, regardless of their background.
Now, how do we achieve this fairness? One approach is through diverse and representative data. By ensuring that training datasets include a wide range of perspectives and experiences, we can mitigate the risk of bias. Additionally, implementing algorithmic fairness techniques such as bias detection tools and fairness-aware algorithms can help create a more balanced approach to AI decision-making. For example, using techniques like re-weighting data or adjusting algorithm outputs can significantly enhance fairness in AI systems.
Next, we turn our attention to transparency and explainability. In a world increasingly governed by AI, understanding how these systems make decisions is crucial. If users don’t understand the rationale behind an AI's decision, how can they trust it? This is where transparency comes into play. AI developers must strive to create systems that are not only effective but also comprehensible. This might involve providing clear explanations of how algorithms function, the data they use, and the decision-making processes involved.
To illustrate this, consider a healthcare AI that suggests treatment options. If a doctor cannot explain why a particular treatment was recommended, it could lead to mistrust among patients. Therefore, fostering a culture of transparency not only enhances user trust but also encourages accountability among developers. The more we understand AI, the better equipped we are to hold its creators responsible for any missteps.
Finally, let’s discuss accountability. With great power comes great responsibility, and AI is no exception. Developers and organizations must establish clear accountability structures to address ethical concerns effectively. This means defining who is responsible for AI decisions and outcomes, as well as ensuring compliance with ethical standards and regulations. As AI technology continues to evolve, we must also consider the role of regulatory frameworks in guiding ethical practices. Existing and proposed regulations can help set boundaries and protect user rights, ensuring that AI serves humanity positively.
In conclusion, the key ethical principles of fairness, transparency, and accountability are not just buzzwords; they are essential components of responsible AI development. By prioritizing these principles, we can create AI systems that not only enhance our lives but also uphold our shared values. As we navigate this new frontier, let’s commit to ensuring that technology serves as a force for good in society.

Fairness and Bias in AI
In the ever-evolving landscape of artificial intelligence, fairness is a crucial pillar that underpins the ethical deployment of AI systems. But what does fairness really mean in this context? It goes beyond just the absence of discrimination; it encompasses the idea that AI should make decisions that are equitable and just for all individuals involved. However, the challenge lies in the fact that AI systems often inherit biases from the data they are trained on, leading to outcomes that can be skewed and unfair. Imagine training a model on historical hiring data where certain demographics were favored over others. The model may learn to replicate these biased patterns, perpetuating inequality rather than alleviating it.
To effectively address bias in AI, it's essential to first understand how it creeps into algorithms. Bias can manifest in various forms, such as historical bias, where past prejudices are reflected in the data, or representation bias, where certain groups are underrepresented in the training datasets. This is why it’s vital to scrutinize the data used for training AI. For instance, if a facial recognition system is predominantly trained on images of light-skinned individuals, it may perform poorly on darker-skinned faces. This not only raises ethical concerns but also poses significant risks to individuals who may be unfairly treated based on flawed AI assessments.
To combat these biases, several techniques can be employed. One effective approach is to implement diversity in datasets. By ensuring that training data is representative of the entire population, AI systems can be trained to make fairer decisions. Additionally, organizations can utilize algorithmic fairness techniques, which include methods such as re-weighting training samples or adjusting decision thresholds to promote fairness across different demographic groups. These strategies are not just technical fixes; they require a commitment to ethical practices and a willingness to challenge the status quo.
Moreover, the conversation around fairness in AI isn't just about mitigating bias; it's also about fostering a culture of accountability. Developers and organizations must take responsibility for the outcomes of their AI systems. This means conducting regular audits and assessments to identify and rectify biases that may arise post-deployment. Just as we wouldn’t allow a faulty machine to operate without oversight, we must ensure that AI systems are continuously monitored for fairness and equity.
In summary, fairness and bias in AI are complex issues that necessitate a multifaceted approach. By prioritizing diversity in data, employing algorithmic fairness techniques, and establishing accountability measures, we can work towards creating AI systems that not only enhance efficiency but also promote justice and equity in our society.
- What is bias in AI? Bias in AI refers to systematic favoritism or discrimination that occurs when algorithms produce unfair outcomes based on the data they are trained on.
- How can we identify bias in AI? Bias can be identified through data analysis, performance evaluation across different demographic groups, and regular audits of AI systems.
- What are some techniques to ensure fairness in AI? Techniques include diversifying training datasets, applying algorithmic fairness methods, and conducting ongoing assessments of AI outcomes.
- Why is accountability important in AI? Accountability ensures that developers and organizations take responsibility for the impacts of their AI systems, fostering trust and ethical practices.

Identifying Bias in Data
In the rapidly evolving landscape of artificial intelligence, one of the most pressing challenges is . Bias can creep into AI systems through various channels, often unnoticed until it manifests in problematic outcomes. This can lead to unfair treatment of individuals based on race, gender, or socioeconomic status, undermining the very foundations of equality and justice that we strive to uphold. So, how do we pinpoint these biases lurking in our datasets?
First and foremost, it’s essential to understand that data is not merely a collection of numbers or entries; it is a reflection of the world around us. If the data we feed into AI systems is skewed or unrepresentative, the outputs will inevitably mirror these flaws. For instance, if an AI system is trained predominantly on data from a specific demographic, it may fail to accurately represent or serve others. This is why conducting a thorough data audit is crucial. By examining the sources of data, we can identify potential biases and take steps to rectify them.
Additionally, we can employ various techniques to uncover biases in datasets. Some of these methods include:
- Statistical Analysis: By applying statistical tests, we can determine if certain groups are underrepresented or misrepresented in the data.
- Visualization Tools: Utilizing data visualization can help in spotting anomalies and patterns that suggest bias.
- Feedback Loops: Engaging with community feedback can provide insights into how data is perceived and used, allowing for adjustments that mitigate bias.
Moreover, it’s not just about identifying bias; it’s about understanding its origins. Bias can stem from:
Source of Bias | Description |
---|---|
Historical Bias | Data that reflects past inequalities or prejudices. |
Representation Bias | Data that does not adequately represent the diversity of the population. |
Measurement Bias | Errors in how data is collected or measured, leading to skewed results. |
By recognizing these sources, developers can take proactive measures to ensure that their AI systems are trained on data that is as fair and representative as possible. This not only improves the accuracy of AI predictions but also builds trust with users who are increasingly concerned about the ethical implications of technology.
In conclusion, identifying bias in data is a multifaceted challenge that requires ongoing vigilance and a commitment to ethical practices. By employing robust auditing techniques and fostering an inclusive approach to data collection, we can pave the way for AI systems that truly serve the needs of all individuals, regardless of their background.
- What is bias in AI? Bias in AI refers to systematic errors that lead to unfair outcomes for certain groups of people, often due to flawed data or algorithms.
- How can I identify bias in my dataset? You can identify bias through statistical analysis, data visualization, and community feedback.
- Why is it important to address bias in AI? Addressing bias is crucial for ensuring fairness, accountability, and trust in AI systems, which ultimately affects societal equity.

Algorithmic Fairness Techniques
When we talk about algorithmic fairness, we're diving into a vital aspect of artificial intelligence that ensures AI systems operate without bias. Imagine a world where decisions about loans, job applications, or even criminal sentencing are made by machines that only see numbers and data, yet somehow still reflect the biases of the society that created them. It's a bit like handing over the keys to a car to someone who doesn't know how to drive; the potential for disaster is high. So, what can we do to ensure that these algorithms are fair and just?
One of the most effective approaches to achieving algorithmic fairness is through the application of specific techniques that can help identify and mitigate bias. These techniques can be broadly categorized into three phases: pre-processing, in-processing, and post-processing. Each phase plays a crucial role in ensuring that the data fed into AI systems, the algorithms themselves, and the outcomes they produce are as unbiased as possible. Let's break this down further:
Phase | Description | Example Techniques |
---|---|---|
Pre-processing | Modifying the training data to eliminate bias before it is used. | Data augmentation, re-weighting, and data cleaning. |
In-processing | Adjusting the algorithm during training to promote fairness. | Fairness constraints, adversarial training, and regularization techniques. |
Post-processing | Altering the output of the algorithm to ensure fair outcomes. | Equalized odds, calibration, and threshold adjustment. |
These techniques are not just theoretical; they have been implemented in various real-world applications to great effect. For instance, consider a hiring algorithm that initially favored candidates from specific universities. By employing pre-processing techniques, developers can adjust the data set to ensure a wider representation of educational backgrounds. This is not just about fairness in hiring; it's about creating a diverse workforce that brings different perspectives and ideas to the table, ultimately benefiting everyone.
Moreover, the in-processing techniques can be particularly powerful. By integrating fairness constraints directly into the model training process, developers can ensure that the algorithm learns to prioritize fairness alongside accuracy. This is akin to teaching a child not just to win a game but to play fair and respect others. The long-term benefits of such practices can lead to a more just society where technology enhances, rather than undermines, equality.
Finally, the post-processing techniques serve as a safety net. Even after an algorithm has been trained, adjustments can be made to its outputs to ensure fairness. This is like a referee in a sports game who steps in to correct a wrong call, ensuring the game remains fair. By applying these techniques, we can strive for a future where AI systems act as allies in promoting fairness rather than perpetuating existing biases.
In conclusion, algorithmic fairness is not just a technical challenge; it's a moral imperative. As we continue to develop and deploy AI technologies, we must remain vigilant and proactive in applying these fairness techniques. The responsibility lies with us, the creators and users of AI, to ensure that these systems reflect the values of equity and justice that we hold dear.

Transparency and Explainability
In the rapidly evolving world of artificial intelligence, the concepts of transparency and explainability are becoming increasingly critical. But what do these terms really mean? Transparency refers to the clarity with which an AI system operates, while explainability is about how well the system can articulate its decision-making processes. Imagine trying to navigate a labyrinth without a map; that's how users often feel when interacting with AI systems that lack transparency. They’re left in the dark, unsure of how decisions are made, and this can lead to a significant erosion of trust.
Consider this: when you receive a recommendation from a streaming service or a decision from a credit algorithm, wouldn't you want to know why? This is where explainability comes into play. It’s not just about getting a result; it’s about understanding the reasoning behind that result. Users deserve to know why a particular decision was made, especially in high-stakes scenarios such as healthcare or criminal justice, where lives can be profoundly affected by an algorithm's output.
Furthermore, the lack of transparency can lead to a phenomenon known as the “black box” problem. In many AI systems, especially those utilizing deep learning, the inner workings can be so complex that even the developers may struggle to explain how the system arrived at a specific conclusion. This opacity can result in biases going unchecked, as stakeholders cannot identify or rectify issues without insight into the decision-making process. The implications are serious: without transparency, it becomes challenging to hold AI systems accountable for their outcomes.
To foster a culture of transparency and explainability, several approaches can be adopted:
- Model Interpretability: Using simpler models or techniques that inherently provide better insights into how decisions are made.
- Documentation: Thoroughly documenting the AI system's development process, including decision points and data sources, can provide clarity.
- User-Centric Design: Designing AI systems with the end-user in mind, ensuring that they can easily understand and interact with the technology.
In addition to these strategies, regulatory bodies are increasingly recognizing the need for guidelines that mandate transparency in AI systems. For instance, the European Union's proposed regulations on AI emphasize not only the need for ethical considerations but also the importance of making AI systems explainable to users. This regulatory push is essential for creating a framework where users can trust AI technologies, knowing they are designed with their best interests in mind.
Ultimately, the journey toward transparency and explainability in AI is not just a technical challenge; it’s a societal imperative. As AI continues to permeate various aspects of our lives, ensuring that these systems are transparent will foster trust, enhance user experience, and promote ethical practices. In a world where technology is advancing at breakneck speed, we must not forget the human element. After all, technology should serve humanity, not the other way around.
- What is the difference between transparency and explainability in AI?
Transparency refers to how open and clear an AI system's operations are, while explainability focuses on the ability of the system to articulate its decision-making process. - Why is transparency important in AI?
Transparency is crucial because it builds trust between users and AI systems, allowing users to understand how decisions are made, which is especially important in sensitive applications. - How can AI developers ensure transparency?
Developers can ensure transparency by adopting model interpretability techniques, thorough documentation, and user-centric design principles.

Accountability in AI Development
As we dive deeper into the world of artificial intelligence, the question of accountability looms larger than ever. Who is responsible when an AI system makes a mistake? Is it the developers, the companies, or the users? The rapid pace of AI development has outstripped our ability to create clear accountability structures, which can lead to significant ethical dilemmas. In a society increasingly reliant on AI for critical decisions—ranging from healthcare diagnostics to job recruitment—the need for a robust framework of responsibility is paramount.
Accountability in AI development is not just a legal issue; it’s a moral imperative. Developers and companies must recognize that their creations have real-world implications. When AI systems fail or behave in ways that are harmful, the repercussions can be devastating. For instance, a biased algorithm in hiring can perpetuate discrimination, while an autonomous vehicle malfunction can lead to accidents. These scenarios highlight the urgent need for clear lines of accountability.
To address these challenges, we need to establish a comprehensive accountability framework that includes various stakeholders. This framework should encompass:
- Developers: They must ensure their algorithms are free from bias and function as intended.
- Companies: Organizations should implement ethical guidelines and oversight mechanisms to monitor AI systems continuously.
- Regulators: Governments and regulatory bodies need to create policies that enforce accountability standards and protect users' rights.
Furthermore, transparency plays a crucial role in accountability. When AI systems operate as black boxes, it becomes nearly impossible to determine who is at fault when something goes wrong. Therefore, developers must prioritize creating systems that are not only effective but also explainable. This means that the decision-making processes of AI should be understandable to users, allowing them to grasp how and why decisions are made.
To illustrate the importance of accountability, consider a hypothetical scenario involving an AI-driven healthcare application. If this application misdiagnoses a patient due to flawed algorithms, the consequences could be life-threatening. In such a case, accountability must be clear:
Stakeholder | Responsibilities |
---|---|
Developers | Ensure algorithms are tested for accuracy and bias. |
Healthcare Provider | Verify AI recommendations and maintain patient safety. |
Regulatory Body | Establish guidelines for AI usage in healthcare. |
In conclusion, as we forge ahead into the AI-driven future, establishing accountability in AI development is not just a technical requirement but a societal necessity. It is crucial for fostering trust among users and ensuring that AI technologies serve humanity positively. By creating clear responsibility structures, prioritizing transparency, and engaging multiple stakeholders, we can address ethical concerns effectively and pave the way for responsible AI innovation.
Q: Why is accountability important in AI development?
A: Accountability is essential to ensure that AI systems operate ethically, and it helps to establish trust among users and developers. When clear lines of responsibility are in place, it becomes easier to address issues that arise from AI decisions.
Q: Who is responsible if an AI system causes harm?
A: Responsibility can fall on multiple stakeholders, including developers, companies, and regulatory bodies. It is crucial to have a framework that defines the roles and responsibilities of each party involved in AI development.
Q: How can transparency improve accountability in AI?
A: Transparency allows users to understand how AI systems make decisions, which can help identify and rectify issues. When users are informed about the processes behind AI decisions, it fosters trust and accountability.
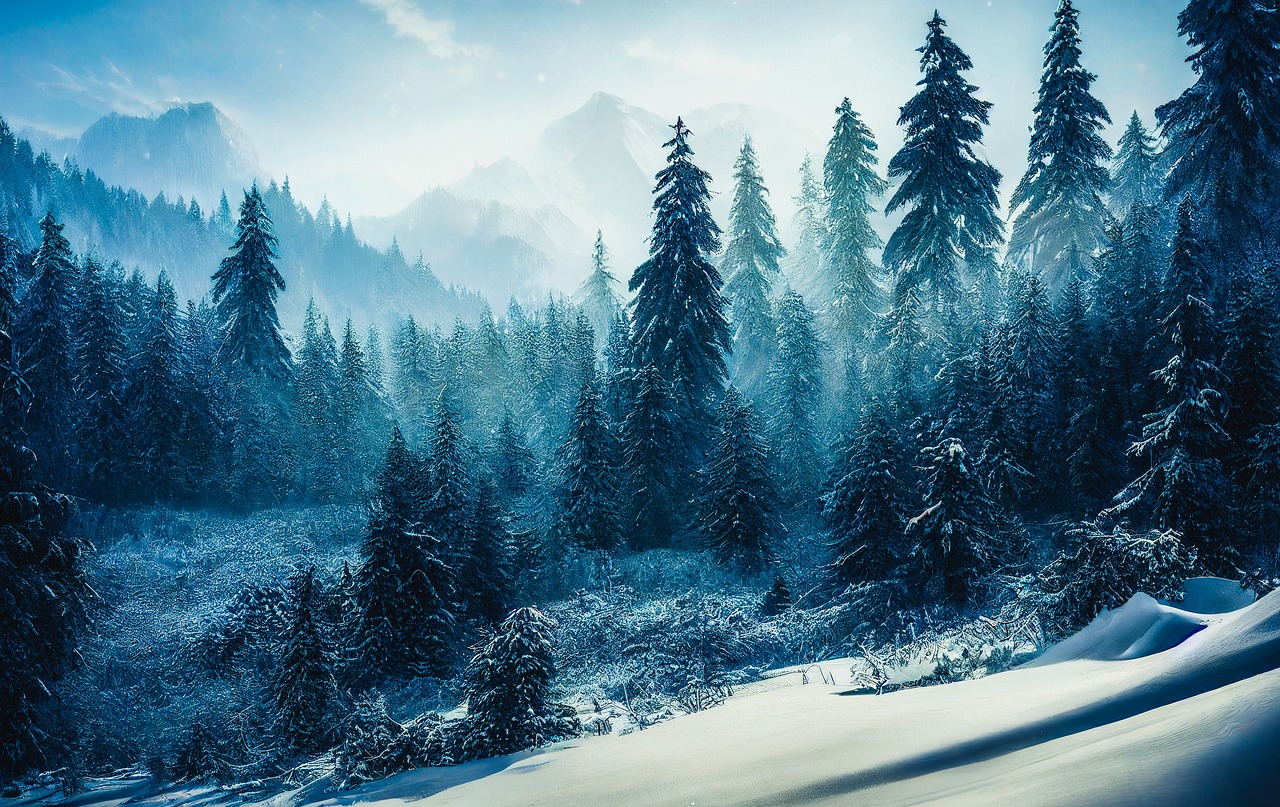
Regulatory Frameworks for AI
As the world becomes increasingly reliant on artificial intelligence, the need for robust regulatory frameworks has never been more pressing. These frameworks serve as a guiding light, ensuring that the development and deployment of AI technologies are aligned with ethical standards and societal values. Without clear regulations, we risk falling into a chaotic landscape where AI systems could operate without accountability, leading to potential harm and misuse.
Regulatory frameworks for AI can take various forms, ranging from government legislation to industry self-regulation. Countries around the globe are beginning to recognize the importance of establishing guidelines that govern how AI is created and used. For instance, the European Union has proposed the AI Act, which aims to create a comprehensive legal framework for AI that categorizes systems based on risk levels. This approach not only protects users but also encourages innovation by providing clear rules of engagement.
However, creating effective regulatory frameworks is not a straightforward task. Policymakers must grapple with several challenges, including:
- Rapid Technological Advancements: AI technology evolves at a breakneck pace, making it difficult for regulations to keep up. What is considered ethical today may not hold true tomorrow.
- Global Cooperation: AI operates on a global scale, yet regulatory approaches can vary significantly between countries, leading to inconsistencies and potential loopholes.
- Balancing Innovation and Regulation: Striking the right balance between fostering innovation and ensuring safety is a delicate dance. Overly stringent regulations could stifle creativity, while lax rules could lead to ethical breaches.
To navigate these challenges, various stakeholders—including governments, tech companies, and civil society—must work together to create a shared understanding of ethical AI practices. This collaboration can take the form of public consultations, where diverse voices are heard, and insights are gathered to shape effective regulations. Furthermore, the establishment of independent oversight bodies could help monitor compliance and ensure that AI systems are developed and deployed responsibly.
In addition to government regulations, corporate responsibility plays a crucial role in the ethical deployment of AI. Companies must not only adhere to legal requirements but also adopt ethical guidelines that go beyond mere compliance. By fostering a culture of accountability and transparency within their organizations, tech companies can contribute to building trust with users and stakeholders alike.
In conclusion, the establishment of regulatory frameworks for AI is essential for promoting ethical practices and protecting user rights. It requires a collective effort from all sectors of society to ensure that AI technologies not only advance but do so in a manner that is fair, transparent, and accountable. As we move forward, ongoing dialogue and adaptation will be crucial in addressing the evolving ethical challenges posed by AI.
Q: Why are regulatory frameworks important for AI?
A: Regulatory frameworks ensure that AI technologies are developed and deployed ethically, protecting users from potential harm and fostering trust in AI systems.
Q: What challenges do regulators face in creating AI regulations?
A: Regulators face challenges such as rapid technological advancements, the need for global cooperation, and balancing innovation with safety.
Q: How can companies ensure ethical AI practices?
A: Companies can ensure ethical AI practices by adhering to legal requirements, adopting ethical guidelines, and fostering a culture of accountability and transparency.

Corporate Responsibility in AI
In today's rapidly evolving technological landscape, the notion of corporate responsibility in artificial intelligence (AI) has become more critical than ever. As companies harness the power of AI to drive innovation and efficiency, they must also recognize the profound impact these technologies can have on society. With great power comes great responsibility, and organizations must be vigilant in ensuring that their AI systems are developed and deployed ethically.
One of the primary roles of corporations in the AI space is to establish and adhere to ethical guidelines that govern the development and deployment of these technologies. This involves a commitment to principles such as transparency, fairness, and accountability. By embedding these values into their corporate culture, companies can foster trust among users and stakeholders, ultimately leading to a more positive perception of AI technologies.
Moreover, corporations must actively engage in the ongoing dialogue surrounding AI ethics. This means not only participating in discussions about best practices but also being open to feedback from diverse communities. For instance, companies can create advisory boards that include ethicists, sociologists, and representatives from various demographics to provide insights on the societal implications of their AI systems. This collaborative approach can help ensure that AI technologies are designed to serve the broader interests of society rather than just corporate profits.
Another aspect of corporate responsibility is the commitment to diversity and inclusion in AI development teams. Diverse teams are more likely to recognize and mitigate biases that can inadvertently creep into AI algorithms. When people from different backgrounds come together, they bring unique perspectives that can lead to more equitable outcomes. Companies should prioritize hiring practices that promote diversity, ensuring that their AI systems reflect the richness of human experience.
To illustrate how corporate responsibility can be effectively implemented in AI, consider the following table that outlines key actions companies can take:
Action | Description |
---|---|
Establish Ethical Guidelines | Create a framework that outlines the ethical principles guiding AI development and usage. |
Engage Stakeholders | Involve diverse groups in discussions about AI ethics to gather a wide range of perspectives. |
Promote Diversity in Teams | Ensure that AI development teams are diverse to minimize biases in AI systems. |
Implement Accountability Measures | Establish clear accountability structures for AI decisions to ensure responsible use of technology. |
Ultimately, the responsibility of corporations extends beyond mere compliance with regulations; it encompasses a proactive approach to ethical AI development. This means being willing to invest in research and development that prioritizes ethical considerations, even if it requires sacrificing short-term profits. Companies that embrace this mindset will not only mitigate risks but also position themselves as leaders in the AI industry, earning the trust and loyalty of their customers.
As we look to the future, it is clear that the challenges surrounding AI ethics will only grow more complex. However, by prioritizing corporate responsibility, companies can navigate these challenges effectively, ensuring that AI technologies are a force for good in society. After all, the true measure of success in AI isn't just about technological advancement; it's about how that advancement positively impacts the world around us.
- What is corporate responsibility in AI? Corporate responsibility in AI refers to the ethical obligations companies have to ensure their AI technologies are developed and used in ways that are fair, transparent, and accountable.
- Why is diversity important in AI development? Diversity in AI development teams helps to identify and mitigate biases in algorithms, leading to more equitable and effective AI systems.
- How can companies ensure accountability in AI? Companies can ensure accountability by establishing clear responsibility structures and guidelines for AI decision-making processes.

Future Challenges in AI Ethics
As we propel ourselves into the future, the landscape of artificial intelligence continues to evolve at breakneck speed, and with it, a myriad of ethical challenges loom on the horizon. One of the most pressing issues is the **rapid pace of technological advancement**, which often outstrips our ability to fully understand and regulate these innovations. Can we keep up with the ethical implications of AI when it seems to evolve almost overnight? This question is at the heart of the ongoing discourse surrounding AI ethics.
Another significant challenge is the **global disparity in AI governance**. Different countries have varying levels of resources, technological capabilities, and regulatory frameworks, leading to a patchwork of ethical standards. This inconsistency can create scenarios where AI technologies developed in one part of the world are deployed in another without adequate oversight, raising concerns about **exploitation and misuse**. For instance, how do we ensure that AI systems designed in a well-regulated environment are not used in regions with lax ethical standards? This disparity calls for a **unified global approach** to AI ethics, fostering collaboration among nations to establish universal guidelines.
Moreover, the question of **data privacy** continues to be a hot-button issue. With the increasing reliance on vast datasets to train AI systems, the potential for misuse of personal information grows. How do we balance the need for data to improve AI performance with the right to privacy? This dilemma necessitates a robust framework that not only protects users' data but also ensures that AI technologies do not infringe upon individual rights. The challenge lies in creating policies that are both comprehensive and adaptable, as the nature of data and technology is ever-changing.
Furthermore, the **impact of AI on employment** cannot be overlooked. As AI systems become more capable, there is a growing fear that they will replace human jobs, leading to widespread unemployment and economic inequality. This raises ethical questions about the responsibility of companies and governments to ensure a **just transition** for workers affected by automation. Should there be a mandate for retraining programs, or is it up to individuals to adapt? The answers to these questions are not straightforward and require a nuanced approach that considers both the economic implications and the human element.
Finally, as AI systems become more autonomous, the issue of **moral responsibility** comes to the forefront. If an AI makes a decision that leads to harm, who is held accountable? Is it the developer, the user, or the AI itself? This conundrum complicates the ethical landscape significantly and necessitates a re-evaluation of our legal frameworks to address these emerging realities. Establishing clear lines of accountability is crucial for fostering trust in AI technologies.
In summary, the future of AI ethics is fraught with challenges that require **ongoing dialogue**, collaboration, and adaptability. As we continue to integrate AI into various facets of society, we must remain vigilant and proactive in addressing these ethical dilemmas. The conversation around AI ethics is not just about technology; it's about the very fabric of our society and how we choose to navigate the complexities of our digital future.
- What are the main ethical challenges in AI today? The main challenges include data privacy, bias in algorithms, accountability for decisions made by AI, and the impact on employment.
- How can we ensure fairness in AI systems? By using diverse datasets, implementing fairness frameworks, and regularly auditing AI systems for bias.
- What role do governments play in regulating AI? Governments can establish regulatory frameworks that guide ethical AI development and protect user rights.
- Why is transparency important in AI? Transparency fosters trust and allows users to understand how decisions are made, which is crucial for accountability.
Frequently Asked Questions
- What is the significance of ethical considerations in AI development?
Ethical considerations in AI development are crucial because they ensure that the technology serves humanity positively. By focusing on ethics, developers can build systems that foster trust and accountability, ultimately leading to better societal outcomes.
- How can bias be identified and mitigated in AI systems?
Bias in AI systems can be identified through rigorous analysis of training datasets, looking for patterns that may disadvantage certain groups. Mitigation techniques include using diverse and representative data, as well as implementing algorithmic fairness frameworks to ensure equitable treatment for all individuals.
- Why is transparency important in AI?
Transparency is vital in AI because it helps users understand how decisions are made. When AI systems are explainable, users are more likely to trust and accept automated outcomes, which is essential for fostering a positive relationship between humans and technology.
- What role do regulatory frameworks play in ethical AI?
Regulatory frameworks guide ethical AI practices by establishing clear guidelines and responsibilities for developers. These regulations help protect user rights and ensure that AI technologies are developed and deployed responsibly, minimizing potential harm.
- How can companies ensure they are practicing ethical AI?
Companies can ensure ethical AI practices by adopting corporate responsibility measures, developing ethical guidelines, and promoting a culture of accountability. This involves regular assessments of AI systems and their impacts, as well as engaging with stakeholders to address ethical concerns.
- What future challenges might arise in AI ethics?
As AI technology continues to evolve, new ethical challenges will likely emerge, such as issues related to privacy, security, and the potential for misuse. Ongoing dialogue and adaptation of ethical practices will be essential to navigate these dilemmas effectively.