Decoding the Future of AI Ethics
As we navigate through the digital age, artificial intelligence (AI) is becoming an integral part of our everyday lives. From the way we shop online to how healthcare is managed, AI is reshaping our world. However, with this rapid advancement comes a pressing need to address the ethical implications of these technologies. The question arises: how do we ensure that AI serves humanity positively and equitably? This article dives deep into the evolving landscape of AI ethics, shedding light on the challenges we face and the frameworks we can adopt to create a future where technology aligns with our core values.
The significance of AI ethics cannot be overstated. As AI systems become more autonomous, the potential for unintended consequences increases. Imagine a world where decisions affecting your life are made by algorithms without any oversight—sounds daunting, right? This is why establishing a robust ethical framework is crucial. It ensures that AI technologies are developed and deployed with principles of fairness, accountability, and transparency.
At the heart of AI ethics lies a set of key ethical principles that guide the development of these technologies. These principles are not just abstract ideas; they are the bedrock upon which we can build trust in AI systems. For instance, consider the principle of fairness. It is essential that AI does not perpetuate existing biases or create new forms of discrimination. This means that developers must actively work to eliminate biases in AI algorithms, ensuring that all individuals, regardless of their background, receive equitable treatment. But how do we achieve this?
One effective approach to ensuring fairness in AI is through the implementation of bias detection techniques. These techniques help identify and mitigate biases that may exist in AI systems. For instance, developers can use statistical methods to analyze data sets for skewed representations or employ machine learning algorithms designed to flag biased outcomes. By proactively addressing these issues, we can foster a more inclusive AI environment. In fact, many organizations are already implementing these techniques, leading to notable improvements in their AI systems.
Moreover, examining real-world case studies can provide valuable insights into the challenges and successes of implementing fairness in AI systems. For example, a recent study on AI in hiring practices revealed that many algorithms favored certain demographics over others. By analyzing these outcomes, organizations were able to revise their algorithms and ensure more equitable hiring practices. These case studies not only highlight the importance of fairness but also demonstrate that it is achievable with the right strategies in place.
Another critical aspect of AI ethics revolves around privacy concerns. As AI systems often rely on vast amounts of personal data, the implications for individual privacy cannot be ignored. With every click and interaction, data is collected, analyzed, and utilized, raising questions about how this information is protected. Are individuals aware of how their data is being used? Do they have control over their personal information? Addressing these concerns is vital to maintaining public trust in AI technologies.
Establishing accountability in AI systems is equally important. Who is responsible when an AI system makes a mistake? Is it the developer, the organization, or the policymakers? To navigate these complex questions, we must create a clear framework that delineates the roles and responsibilities of all stakeholders involved in AI deployment. This framework should promote a culture of responsibility and ethical governance, ensuring that AI technologies are used for the greater good.
To facilitate this accountability, regulatory frameworks are essential. They guide ethical AI practices by establishing standards that organizations must adhere to. For example, existing regulations like the General Data Protection Regulation (GDPR) in Europe set stringent guidelines for data usage and privacy, promoting accountability in AI systems. As we look to the future, it’s crucial that we continue to develop and refine these regulations to keep pace with technological advancements.
In addition to regulations, industry best practices play a significant role in promoting ethical AI solutions. Organizations should adopt strategies that prioritize ethical considerations throughout the AI development lifecycle. This includes conducting regular audits of AI systems, investing in training for developers on ethical AI principles, and fostering a culture of transparency within the organization. By adhering to these best practices, companies can ensure that their AI technologies are not only innovative but also responsible.
- What is AI ethics? AI ethics refers to the principles and guidelines that govern the development and deployment of artificial intelligence technologies to ensure they are fair, accountable, and transparent.
- Why is fairness important in AI? Fairness is crucial in AI to prevent biases that can lead to discrimination and unequal treatment of individuals from different backgrounds.
- How can organizations ensure accountability in AI? Organizations can ensure accountability by establishing clear roles for developers, implementing regulatory frameworks, and adopting industry best practices.
- What are some common privacy concerns with AI? Common privacy concerns include data collection without consent, misuse of personal information, and lack of transparency in how data is used.
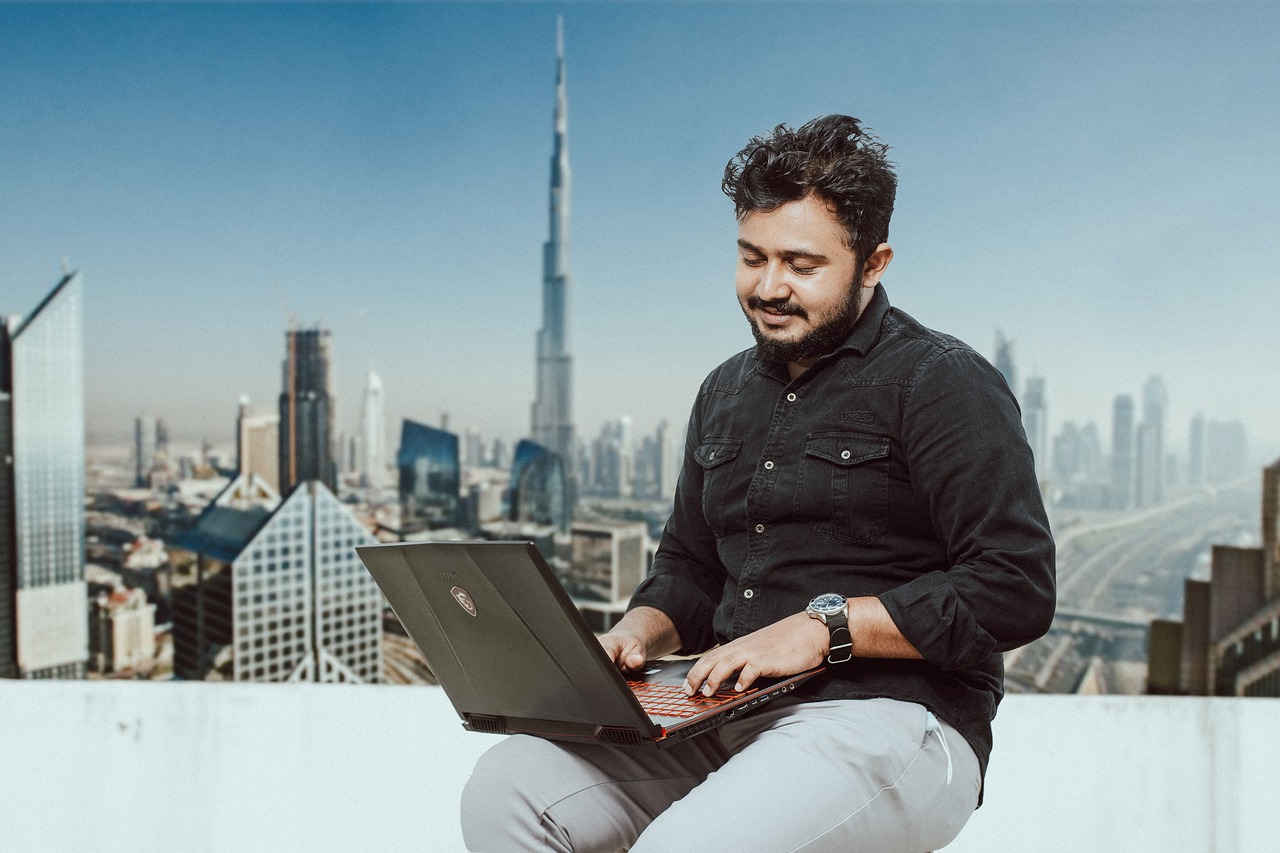
The Importance of AI Ethics
Understanding the significance of AI ethics is crucial as it shapes how technology impacts human lives. As artificial intelligence becomes more integrated into our daily routines, from healthcare to finance, the need for ethical considerations is more pressing than ever. Imagine a world where decisions affecting your life are made by algorithms without any oversight. Sounds unsettling, right? This is why AI ethics is not just a buzzword; it’s a necessity.
At its core, AI ethics aims to ensure fairness, accountability, and transparency in AI systems. These principles act as the guiding lights for developers and organizations, helping them navigate the complex landscape of technology. Without a solid ethical foundation, AI can perpetuate existing biases, invade personal privacy, and operate in a black box where users have no idea how decisions are made. Therefore, embedding ethics into AI development isn’t merely a box-ticking exercise; it’s about fostering trust and ensuring that technology serves humanity, not the other way around.
Moreover, consider the implications of failing to address ethical concerns. When AI systems malfunction or make biased decisions, the consequences can be dire. For instance, biased algorithms in hiring processes can lead to discrimination, while AI in law enforcement can exacerbate racial profiling. In these scenarios, the absence of ethical guidelines can result in lost opportunities and even harm to individuals and communities. This is why having a robust framework for AI ethics is not just important; it’s essential for the future of society.
In addition to the ethical principles mentioned, the importance of AI ethics extends to regulatory compliance. Governments and organizations are increasingly recognizing the need for regulations that govern AI practices. These regulations are designed to protect citizens and ensure that AI technologies are developed and deployed responsibly. Without such regulations, there’s a risk that organizations might prioritize profit over ethical considerations, leading to a potential crisis of trust between the public and technology.
In summary, the importance of AI ethics cannot be overstated. It is the backbone of responsible AI development and deployment. As we continue to innovate and harness the power of artificial intelligence, we must ensure that ethical principles are at the forefront of our efforts. Only then can we truly unlock the potential of AI while safeguarding our values and rights as individuals.
- What are AI ethics? AI ethics refers to the moral principles guiding the development and implementation of artificial intelligence technologies.
- Why is fairness important in AI? Fairness in AI ensures that algorithms do not discriminate against any group, promoting equity and justice in decision-making.
- How can organizations ensure accountability in AI? Organizations can establish clear guidelines, conduct audits, and foster a culture of transparency to ensure accountability in AI systems.
- What role do regulations play in AI ethics? Regulations help enforce ethical standards, protect individuals' rights, and ensure responsible AI practices across industries.
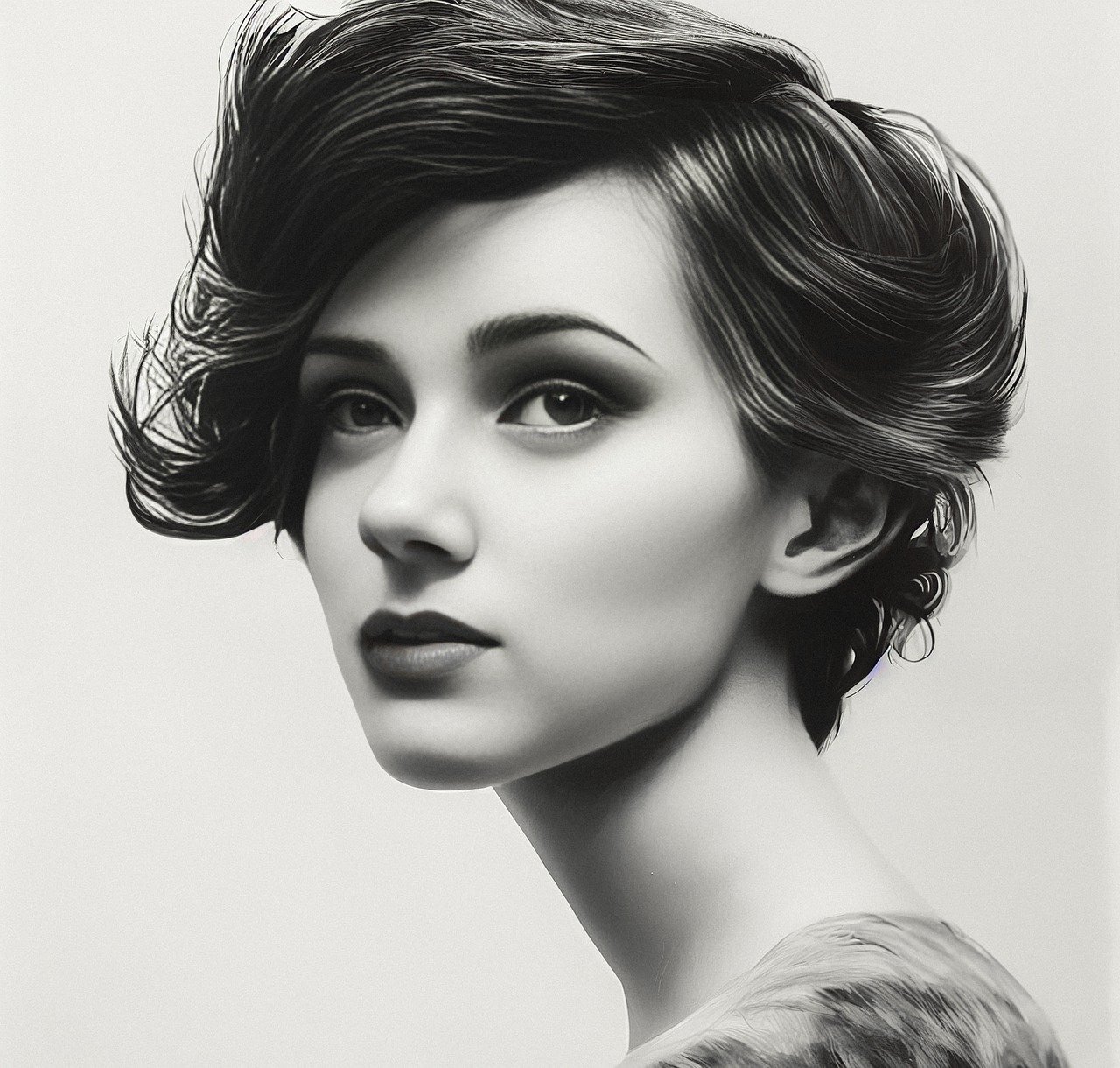
Key Ethical Principles
In the rapidly evolving world of artificial intelligence, understanding the that guide its development is not just important—it's essential. These principles form the bedrock of responsible AI innovation, ensuring that technology serves humanity rather than the other way around. With AI systems making decisions that can significantly impact our lives, from hiring processes to healthcare diagnostics, the need for a robust ethical framework is more pressing than ever. So, what are these guiding principles?
At the core of AI ethics are three fundamental principles: fairness, privacy, and accountability. Each of these principles plays a crucial role in shaping how AI technologies are developed and deployed, ultimately influencing the trust users place in these systems. Let's explore these principles in detail and see how they interconnect to create a responsible AI ecosystem.
Fairness is all about ensuring that AI systems treat all individuals equitably, regardless of their background. Imagine a world where algorithms are like judges—if they are biased, the verdicts they deliver can lead to unjust outcomes. This is why eliminating biases from AI algorithms is paramount. Developers must actively work to identify and mitigate any biases present in their data sets and models. Strategies for achieving fairness include:
- Conducting thorough audits of data sets to identify potential biases.
- Implementing algorithmic adjustments that promote equitable outcomes.
- Engaging with diverse stakeholder groups to gather insights and perspectives.
By focusing on fairness, we can foster a more inclusive technological landscape that respects and uplifts all voices.
Identifying biases in AI systems is not a one-time task; it's an ongoing process. Various bias detection techniques have emerged to help developers assess their models. These techniques can range from statistical analyses to more complex machine learning methods aimed at uncovering hidden biases. For instance, tools like Fairness Indicators and AIF360 can be employed to evaluate how different groups are impacted by AI decisions. The goal here is to continuously refine models to ensure they reflect a fair representation of society.
Real-world case studies serve as invaluable resources for understanding the challenges and successes of implementing fairness in AI systems. For example, a notable case involved a major tech company that faced backlash for a hiring algorithm that disproportionately favored male candidates. By revisiting their data and employing fairness-enhancing interventions, they managed to recalibrate their system, resulting in a more balanced hiring process. Such examples not only highlight the importance of fairness but also provide valuable insights into best practices and lessons learned for future AI developments.
As AI systems become more pervasive, the concerns surrounding privacy cannot be overstated. With vast amounts of personal data being collected and analyzed, the potential for misuse is significant. Imagine giving a stranger access to your diary—this is akin to what happens when AI systems process personal information without adequate safeguards. Developers and organizations must prioritize data protection and ensure that user consent is obtained transparently. This involves implementing robust security measures and adhering to regulations like GDPR to protect individuals' privacy rights.
Establishing accountability in AI systems is essential for ethical governance. It’s not enough to create advanced technologies; we must also ensure that there are mechanisms in place for holding developers and organizations responsible for their AI's actions. This accountability can take various forms, from internal audits to third-party evaluations, ensuring that AI systems are not only effective but also ethical.
Regulatory frameworks play a crucial role in guiding ethical AI practices. Governments and organizations are increasingly recognizing the need for regulations that promote accountability in AI technologies. Existing regulations, like the EU's AI Act, aim to set standards for high-risk AI applications, ensuring they meet ethical guidelines before deployment. Proposed policies are also being discussed globally, emphasizing the need for a collaborative approach to AI governance.
Highlighting industry best practices can help organizations implement ethical AI solutions effectively. Key strategies for responsible AI development include:
- Adopting a multidisciplinary approach that involves ethicists, engineers, and stakeholders.
- Regularly updating AI systems to reflect changing societal norms and values.
- Creating transparent processes that allow users to understand how decisions are made.
By following these best practices, organizations can foster trust and ensure that their AI technologies serve the greater good.
Q1: What are the main ethical concerns surrounding AI?
A1: The primary ethical concerns include fairness, privacy, accountability, and transparency in AI systems.
Q2: How can organizations ensure fairness in AI?
A2: Organizations can ensure fairness by conducting bias audits, involving diverse teams in development, and continuously monitoring algorithms for equitable outcomes.
Q3: Why is privacy important in AI ethics?
A3: Privacy is crucial because AI systems often handle sensitive personal data, and protecting this information is essential for maintaining user trust and compliance with regulations.
Q4: What role do regulatory frameworks play in AI ethics?
A4: Regulatory frameworks establish guidelines and standards for ethical AI development, ensuring that technologies are safe and beneficial for society.
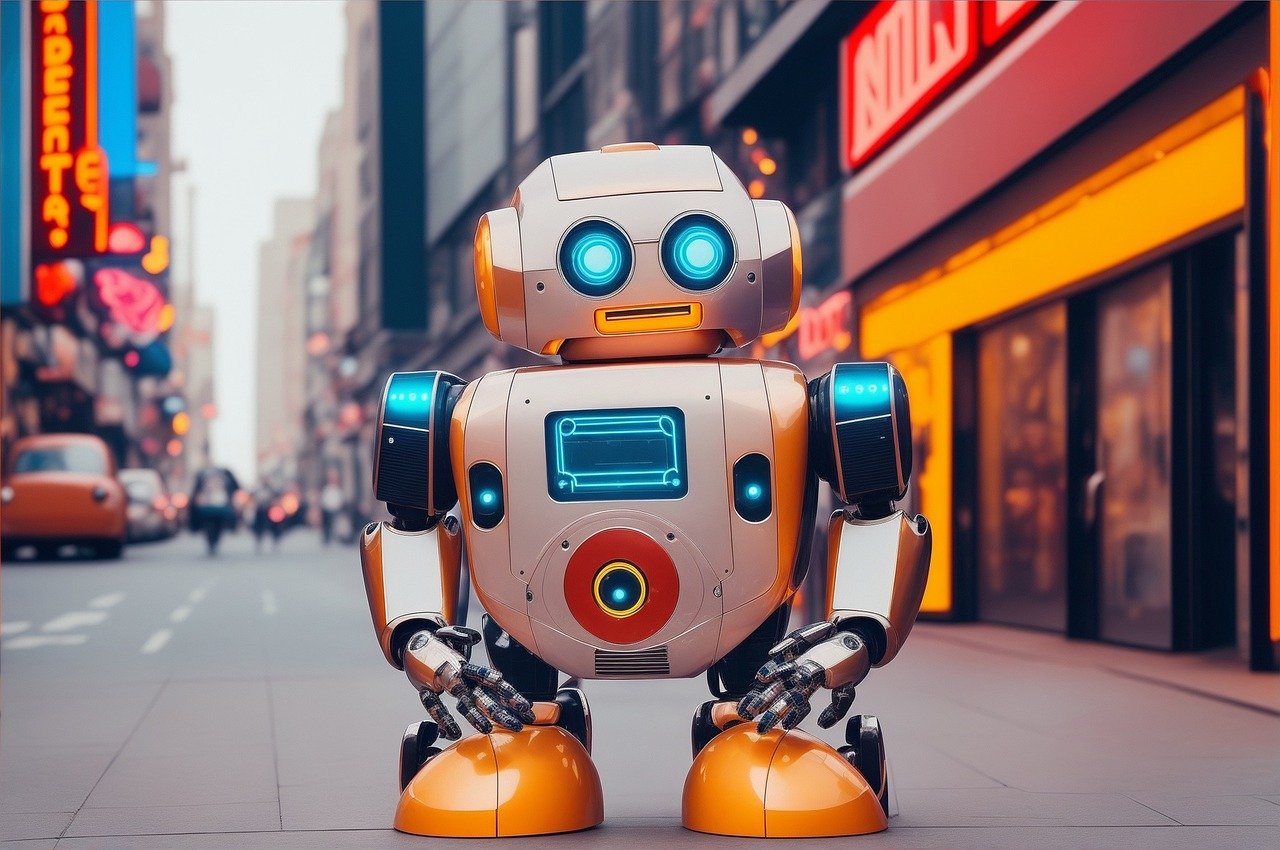
Fairness in AI
When we talk about , we're diving into a fundamental aspect that can make or break the trust people have in technology. Imagine a world where decisions affecting your life—like job opportunities, loan approvals, or even legal judgments—are made by algorithms. If these algorithms are biased, the consequences can be devastating. Fairness in AI isn't just a technical requirement; it's a moral obligation. It involves eliminating biases and ensuring that AI systems treat everyone equitably, regardless of their background, ethnicity, or gender.
To achieve this, developers and researchers are employing a variety of strategies. One effective approach is the implementation of diverse training datasets. By ensuring that the data used to train AI models is representative of all demographic groups, we can significantly reduce the risk of bias. Another strategy is the use of algorithmic auditing, where AI systems are regularly evaluated to identify and rectify any unfair outcomes. These audits can help pinpoint where biases may exist and how they can be mitigated.
Furthermore, transparency plays a crucial role in fostering fairness. When users understand how AI systems make decisions, they are more likely to trust the outcomes. This transparency can be achieved through clear documentation and open communication about how algorithms function. For instance, organizations can publish reports detailing the data sources, methodologies, and performance metrics of their AI systems. This not only builds trust but also encourages accountability.
To illustrate the importance of fairness, consider the following table that summarizes key strategies for achieving fairness in AI:
Strategy | Description |
---|---|
Diverse Training Datasets | Using data that represents various demographic groups to train AI models. |
Algorithmic Auditing | Regular evaluations of AI systems to identify and mitigate biases. |
Transparency | Clear communication about how AI systems make decisions and their data sources. |
Real-world case studies serve as a testament to the challenges and successes in achieving fairness in AI. For instance, a notable case involved a hiring algorithm that favored candidates from certain backgrounds over others. After identifying the bias, the company took steps to diversify its training data and implemented an auditing process. The result? A more equitable hiring process that opened doors for a wider range of candidates.
Ultimately, fairness in AI is not a one-time fix but an ongoing commitment. As technology evolves, so do the challenges associated with it. By continuously striving for fairness, we can ensure that AI serves as a tool for empowerment rather than oppression. So, the next time you interact with an AI system, remember that its fairness is a reflection of our collective values and ethics.
- What is fairness in AI? Fairness in AI refers to the principle of ensuring that AI systems operate without bias and treat all individuals equitably.
- Why is fairness important in AI? Fairness is crucial because biased AI systems can lead to unjust outcomes that negatively impact individuals and communities.
- How can we achieve fairness in AI? Achieving fairness involves using diverse training datasets, conducting algorithmic audits, and maintaining transparency about how AI systems function.
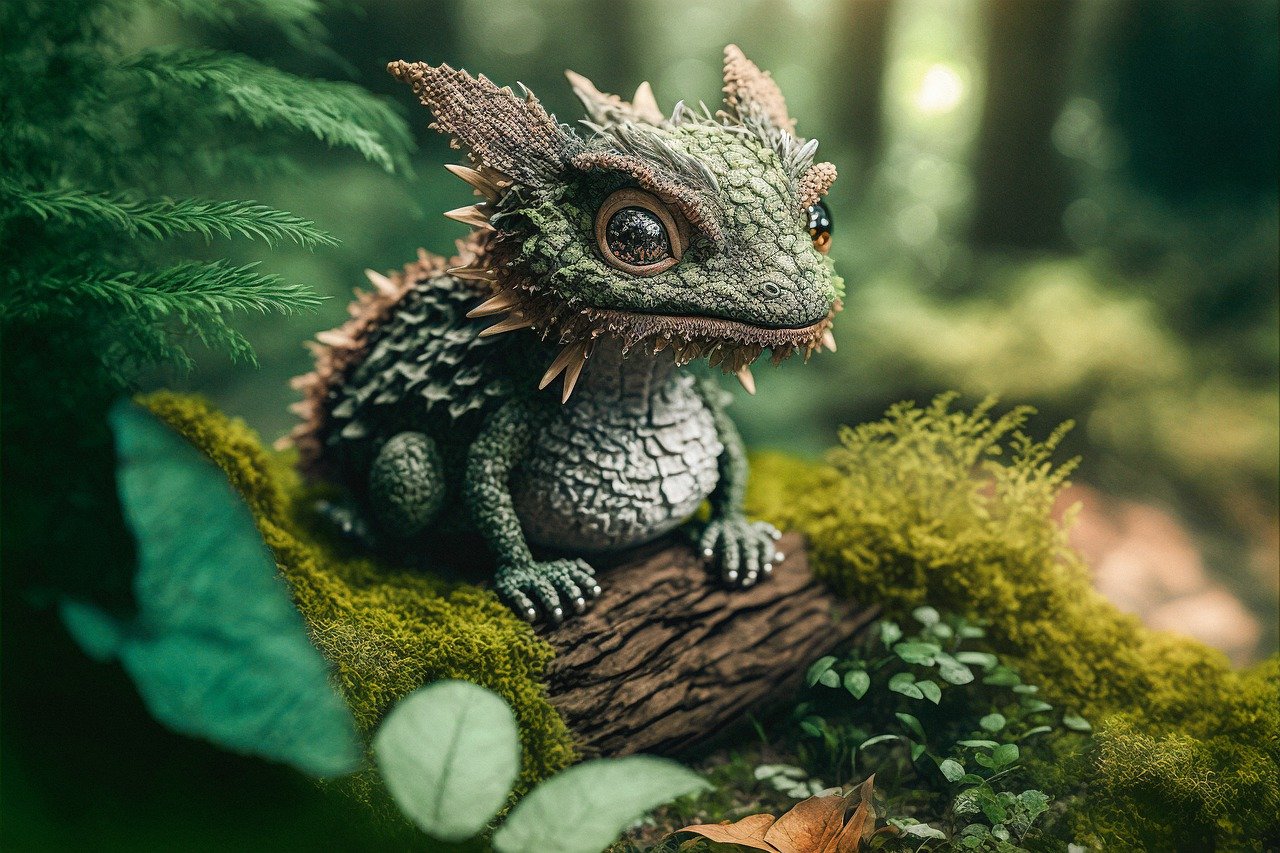
Bias Detection Techniques
In the realm of artificial intelligence, ensuring fairness is not just a goal; it’s a necessity. One of the most pressing challenges is the detection of bias within AI systems. Bias can creep into AI algorithms through various channels, including the data used for training, the design of the algorithms themselves, and even the societal norms that influence these processes. Therefore, employing effective is crucial for identifying and mitigating these biases before they lead to unfair outcomes.
One of the primary methods for detecting bias is through statistical analysis. This involves examining the outcomes of AI algorithms to identify any discrepancies in how different demographic groups are treated. For instance, if an AI system is used to screen job applicants, a statistical analysis can reveal whether candidates from certain backgrounds are consistently receiving lower scores compared to others, even when their qualifications are similar. Such an analysis can highlight potential biases and prompt further investigation.
Another effective technique is algorithmic auditing. This process entails a thorough examination of the algorithms themselves, looking for any inherent biases in their design. Auditors may use tools that simulate various scenarios to see how the algorithm responds under different conditions. For example, an audit might reveal that an algorithm disproportionately favors one gender over another in loan approvals, prompting developers to adjust the underlying logic. This proactive approach is essential for ensuring that AI systems operate fairly.
Moreover, the use of adversarial testing has emerged as a powerful technique in bias detection. This method involves introducing controlled variations into the input data to observe how the AI system reacts. By deliberately manipulating certain factors—like age, race, or gender—developers can assess whether the AI system remains consistent in its decision-making. If significant discrepancies arise, it signals the presence of bias that needs to be addressed.
To illustrate the importance of these techniques, consider the following table that summarizes some common bias detection methods and their applications:
Technique | Description | Application |
---|---|---|
Statistical Analysis | Examine outcomes for demographic discrepancies. | Job applicant screening, credit scoring. |
Algorithmic Auditing | Review algorithms for inherent biases. | Loan approval systems, hiring algorithms. |
Adversarial Testing | Manipulate input data to test algorithm responses. | Facial recognition, predictive policing. |
In addition to these methods, it’s vital to foster a culture of transparency and collaboration in AI development. By encouraging diverse teams to work together, organizations can leverage various perspectives to identify biases that may have been overlooked. This collaborative approach not only enhances the detection of bias but also contributes to the overall fairness of AI systems.
As we continue to navigate the complexities of AI ethics, employing robust bias detection techniques will be integral to building trust in AI technologies. By actively seeking out and addressing biases, we can ensure that AI serves as a tool for equity rather than a source of division.
- What is bias in AI? Bias in AI refers to systematic favoritism or prejudice in the outcomes produced by AI systems, often resulting from flawed data or algorithms.
- Why is bias detection important? Detecting bias is crucial to ensure fairness and accountability in AI applications, which can significantly impact individuals and communities.
- What are some common bias detection techniques? Common techniques include statistical analysis, algorithmic auditing, and adversarial testing.
- How can organizations promote fairness in AI? Organizations can promote fairness by employing diverse teams, implementing bias detection techniques, and fostering a culture of transparency.
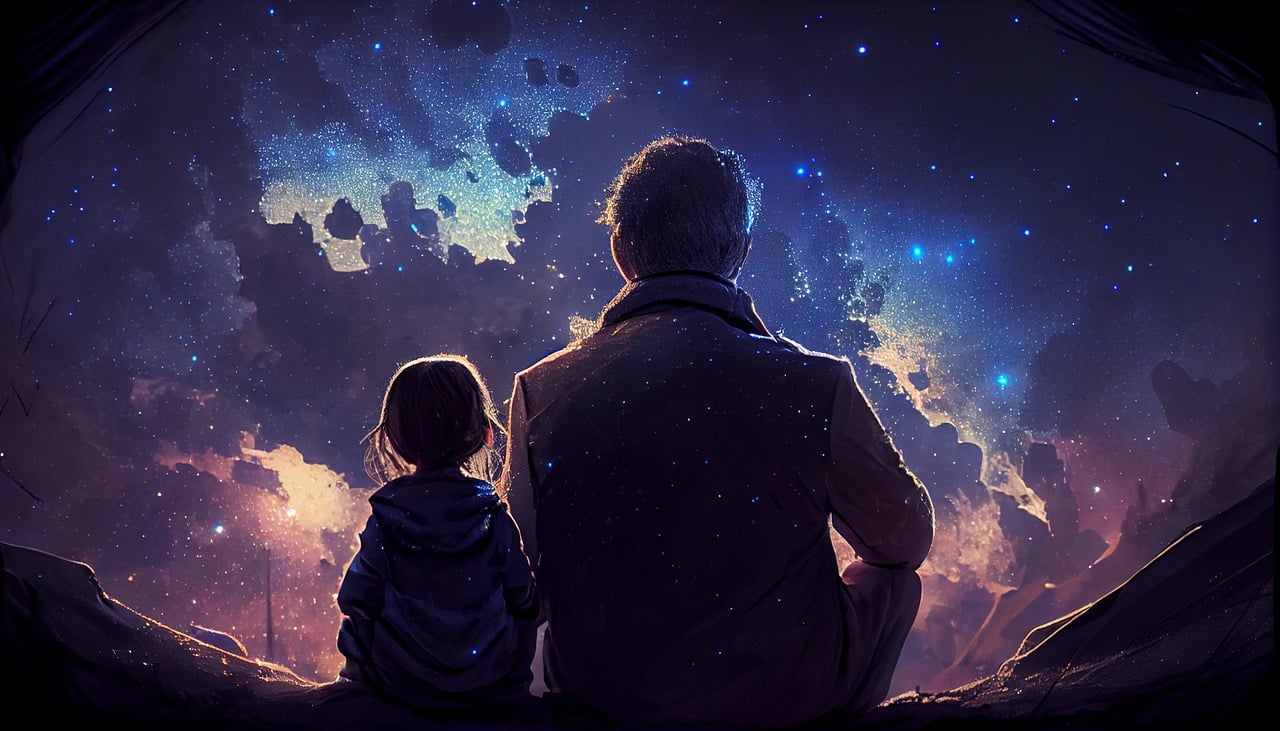
Case Studies on Fairness
When we talk about fairness in artificial intelligence, it's not just a theoretical concept; it's a real-world challenge that many organizations are grappling with. To truly understand the implications of fairness in AI, let's dive into some compelling case studies that illustrate both the challenges and the successes in implementing fair AI systems.
One notable example is the use of AI in hiring processes. Companies like Amazon initially developed an AI recruiting tool that was intended to streamline the hiring process. However, they discovered that the algorithm was biased against women. The AI had been trained on resumes submitted over a ten-year period, predominantly from male candidates, leading to a model that favored male applicants. This case highlights the importance of diverse training data and the need for continuous monitoring to ensure fairness in AI applications.
Another fascinating case study comes from the healthcare sector. Researchers developed an AI system to predict patient outcomes based on historical data. However, they found that the algorithm disproportionately favored white patients over black patients, leading to significant disparities in treatment recommendations. This situation prompted the healthcare provider to implement bias detection techniques, including regular audits and the incorporation of more representative data sets. By actively addressing these biases, the organization made strides toward a more equitable healthcare solution.
In the realm of facial recognition technology, the city of San Francisco made headlines by banning the use of facial recognition software by city agencies. This decision stemmed from concerns about racial bias and the potential for misidentification, particularly among minority groups. The city recognized that the technology was not only flawed but also posed ethical dilemmas regarding privacy and civil liberties. This case serves as a powerful reminder that regulatory measures can play a crucial role in promoting fairness and accountability in AI systems.
Moreover, the financial industry is not immune to these challenges. A major bank implemented an AI-driven credit scoring system that inadvertently discriminated against certain demographics. Upon realizing this, the bank collaborated with external auditors to reassess the algorithm's fairness. They employed a variety of bias detection techniques, such as testing for disparate impact and conducting sensitivity analyses. This proactive approach not only improved their AI model but also restored trust among their customers.
These case studies underscore the necessity of integrating fairness into AI development from the outset. Organizations must prioritize ethical considerations and actively seek to eliminate biases in their algorithms. By learning from the experiences of others, companies can foster an environment of accountability and transparency, paving the way for a more equitable future in AI.
As we continue to explore the impact of AI on our lives, it’s evident that the journey toward fairness is ongoing. Each case study offers valuable insights into best practices and lessons learned, emphasizing the importance of vigilance and commitment to ethical standards in AI development.
- What is AI fairness? AI fairness refers to the principle that AI systems should operate without bias, ensuring equitable treatment for all individuals, regardless of race, gender, or socioeconomic status.
- Why are case studies important in understanding AI ethics? Case studies provide real-world examples of how ethical principles are applied or violated, offering critical insights into the challenges and solutions that organizations face in promoting fairness.
- How can organizations ensure fairness in AI? Organizations can ensure fairness by using diverse training data, implementing bias detection techniques, and conducting regular audits of their AI systems.
- What role do regulations play in AI ethics? Regulations help establish guidelines and standards for ethical AI practices, promoting accountability and transparency in AI deployment.
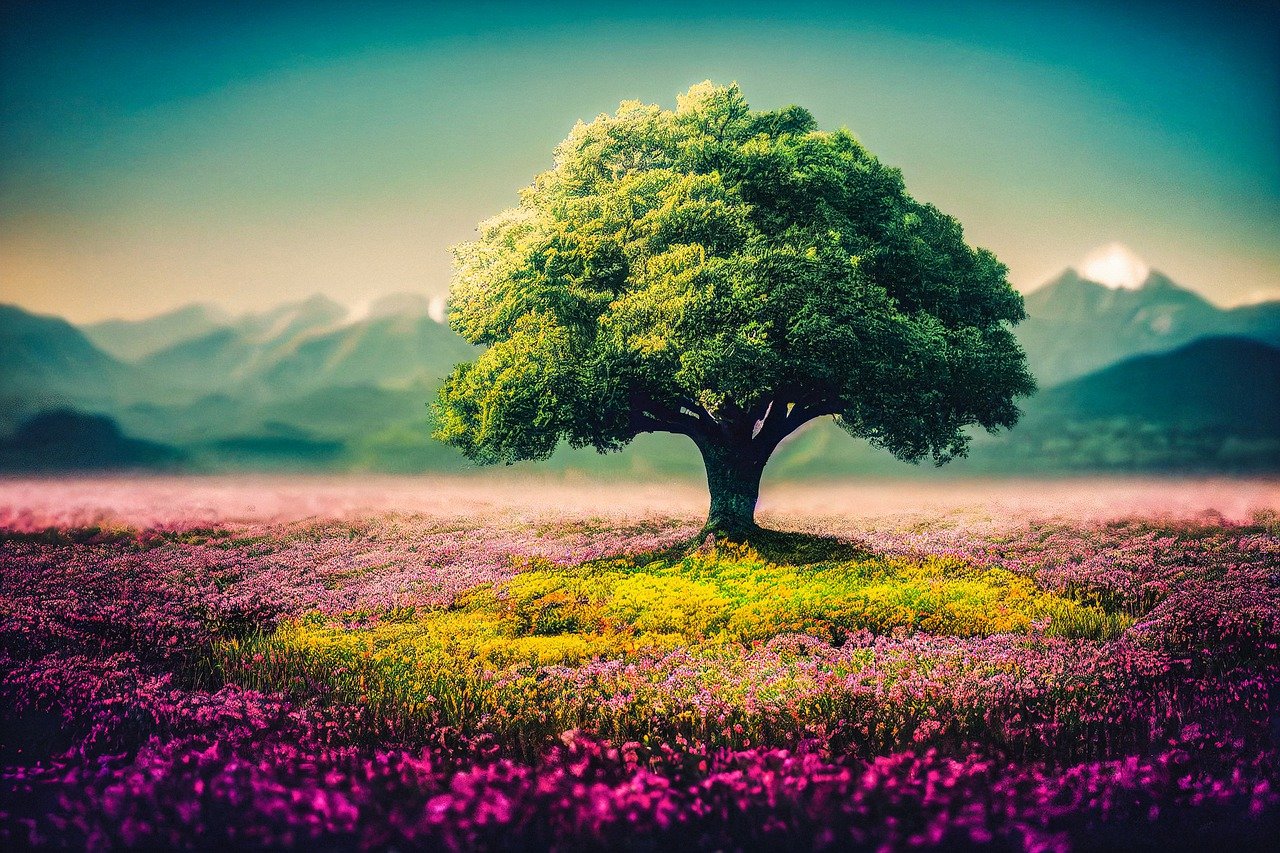
Privacy Concerns
In the age of artificial intelligence, privacy has emerged as one of the most pressing ethical challenges. As AI systems increasingly rely on vast amounts of data to function effectively, the implications for personal privacy become more complex and concerning. Imagine your every online interaction being monitored and analyzed—sounds a bit like a sci-fi dystopia, right? Yet, this is the reality we face as AI continues to permeate our daily lives.
At the heart of privacy concerns is the issue of data collection. AI systems often require access to personal information, which can include everything from your browsing history to your shopping habits. This data is then used to train algorithms that can predict behavior, tailor advertisements, and even influence decisions. While this can enhance user experience, it raises significant questions about consent and ownership. Do we truly understand what we are agreeing to when we click "Accept" on those lengthy terms and conditions?
Moreover, the storage and security of this data is another critical aspect. Once our data is collected, it needs to be stored somewhere. This creates a treasure trove for hackers and cybercriminals. A breach can lead to sensitive information being exposed, resulting in identity theft, financial loss, and a breach of trust between users and organizations. The recent surge in data breaches has highlighted the need for robust security measures to protect personal information.
Furthermore, the question of data usage cannot be overlooked. How is our data being utilized once it's collected? AI systems can analyze this data to create detailed profiles, which can then be used for targeted advertising or even surveillance. This raises ethical questions about how much control individuals have over their own data. Are we comfortable with the idea that our personal information can be used to manipulate our choices?
To tackle these privacy concerns, several strategies can be employed:
- Transparency: Organizations must be open about what data they collect and how it is used. Clear communication can help users make informed decisions.
- Data Minimization: Collecting only the data that is absolutely necessary can help reduce privacy risks. Less data means less exposure.
- Robust Security Measures: Implementing strong encryption and security protocols can protect data from unauthorized access.
In conclusion, as AI continues to evolve, addressing privacy concerns becomes imperative. Striking a balance between innovation and ethical responsibility is key to building trust in AI technologies. The conversation around privacy is not just about protecting data; it's about respecting individuals and their rights in an increasingly interconnected world.
- What are the main privacy concerns related to AI? The primary concerns include data collection practices, data security, and the potential misuse of personal information.
- How can individuals protect their privacy when using AI technologies? Individuals can protect their privacy by being cautious about the information they share, reading privacy policies, and using privacy-focused tools.
- What role do governments play in regulating AI privacy? Governments can establish regulations and guidelines to ensure that organizations adhere to ethical data practices and protect citizens' privacy rights.
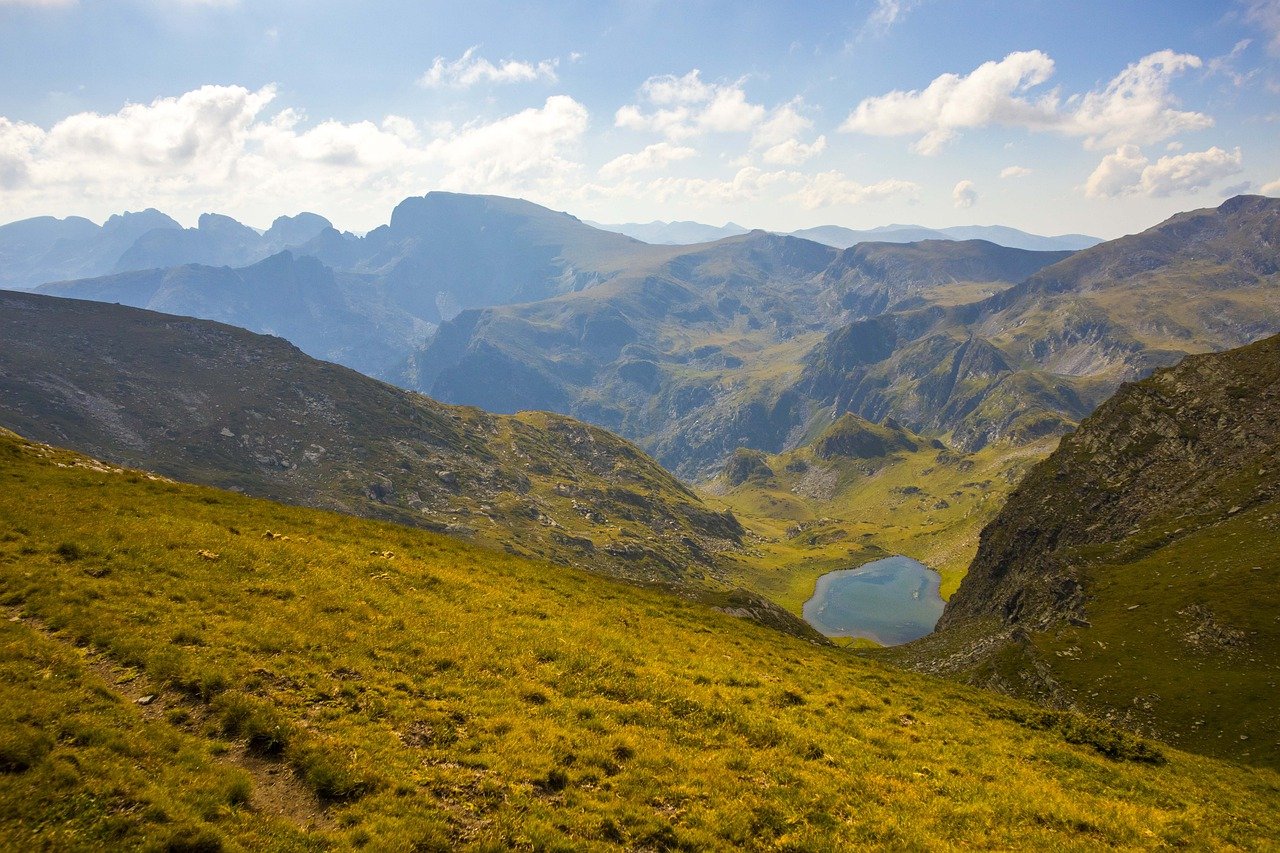
Accountability in AI Systems
When we talk about , we're diving into a crucial aspect of how artificial intelligence interacts with our lives. It's not just about creating advanced algorithms; it's about ensuring that these systems operate with a sense of responsibility. Imagine a world where decisions made by AI can be traced back to the people or organizations that developed them. Wouldn’t that add a layer of trust? Establishing accountability is essential for ethical governance, as it holds developers, organizations, and policymakers responsible for the outcomes of AI implementations.
One of the primary challenges in ensuring accountability is the complexity of AI systems themselves. They often function as "black boxes," where the decision-making process is not transparent. This lack of clarity can lead to significant ethical dilemmas, particularly when AI systems make decisions that affect people's lives—like hiring, lending, or even law enforcement. So, how can we tackle this challenge? Well, it starts with clear guidelines and frameworks that delineate roles and responsibilities.
To build a robust accountability framework, we must consider the following key players:
- Developers: They are responsible for designing and coding algorithms that are fair and unbiased.
- Organizations: Companies must implement ethical guidelines and ensure their AI systems comply with regulations.
- Policymakers: Governments should create laws that protect citizens while promoting innovation.
These roles are interconnected, and when one fails, the entire system can be compromised. For instance, if developers ignore bias detection, organizations may deploy flawed AI systems, leading to unfair outcomes. This domino effect highlights the need for collaboration among all stakeholders to foster an environment of accountability.
The regulatory landscape is also evolving to address these challenges. Various countries are developing frameworks that govern AI usage, focusing on transparency and accountability. For example, the European Union's AI Act aims to categorize AI applications based on risk levels, imposing stricter regulations on high-risk systems. This proactive approach is a step in the right direction, ensuring that AI technologies are not just innovative but also ethically sound.
Moreover, industry best practices can serve as a roadmap for organizations striving to implement accountable AI solutions. By adopting guidelines such as regular audits of AI systems, establishing ethics boards, and promoting a culture of transparency, organizations can significantly enhance accountability. These practices not only mitigate risks but also build trust with users, ultimately leading to a more responsible AI ecosystem.
- What is accountability in AI? Accountability in AI refers to the responsibility of developers, organizations, and policymakers to ensure that AI systems operate ethically and transparently.
- Why is accountability important in AI systems? It is crucial because it helps build trust, ensures fairness, and mitigates the risks associated with AI decision-making.
- How can organizations ensure accountability in AI? Organizations can implement regular audits, establish ethics boards, and adhere to regulatory frameworks to promote accountability.
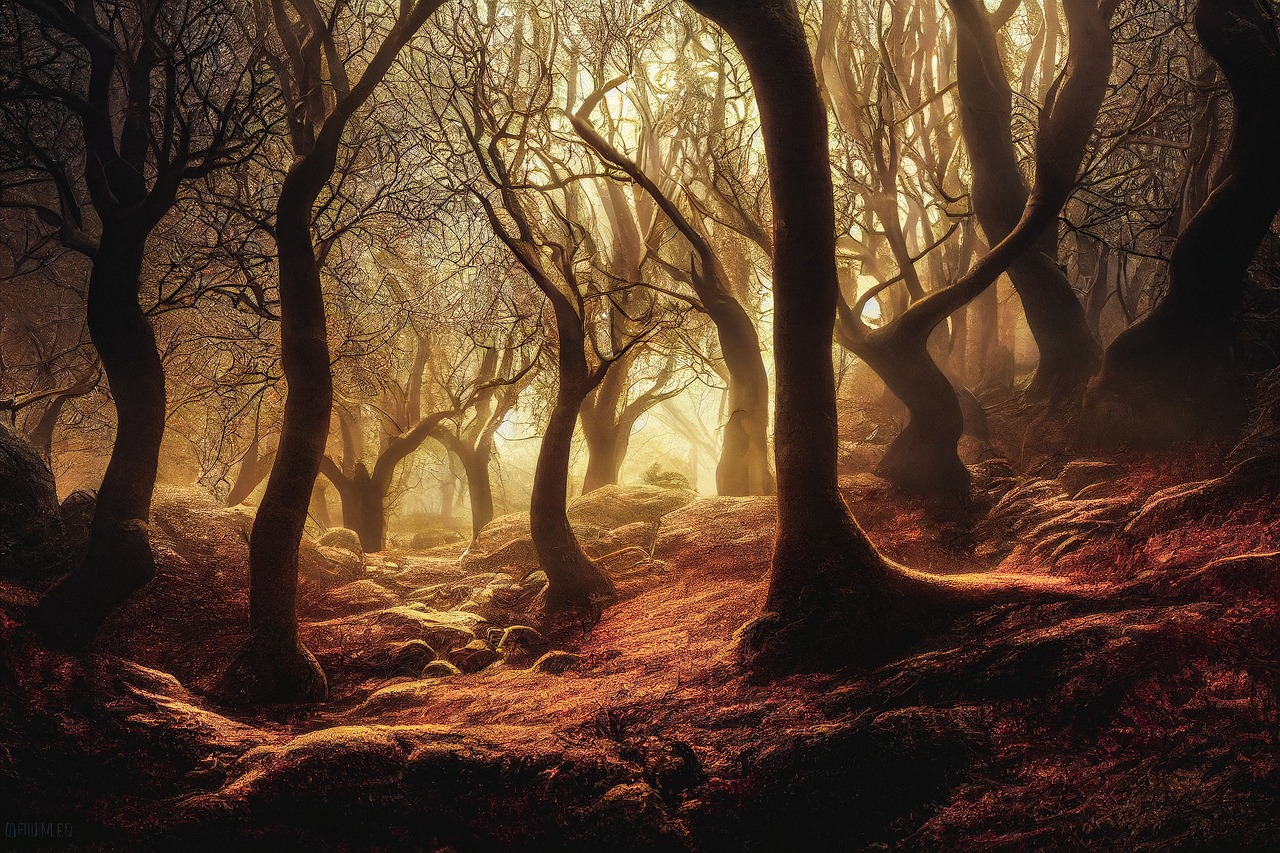
Regulatory Frameworks
In the rapidly evolving world of artificial intelligence, establishing robust is not just important; it’s essential. With AI technologies permeating various sectors—from healthcare to finance—regulations are needed to ensure that these systems operate ethically and responsibly. But what exactly do we mean by regulatory frameworks in the context of AI? Essentially, they are a set of guidelines and rules designed to govern the development, deployment, and use of AI technologies. These frameworks aim to protect individuals and society as a whole from potential harm while fostering innovation.
As we dive deeper into the regulatory landscape, it becomes evident that different regions are approaching AI governance in unique ways. For instance, the European Union has taken a proactive stance by proposing the Artificial Intelligence Act, which categorizes AI systems based on risk levels and imposes stricter requirements on high-risk applications. On the other hand, the United States has been more fragmented, with various states implementing their own regulations, leading to a patchwork of laws that can be challenging to navigate.
One of the core objectives of these regulatory frameworks is to ensure accountability in AI systems. This means that developers and organizations must be held responsible for the outcomes of their AI technologies. To facilitate this, many frameworks emphasize the need for transparency in AI operations. For example, organizations may be required to disclose how their algorithms make decisions, allowing stakeholders to understand and challenge those decisions when necessary.
Moreover, the conversation around regulatory frameworks often includes discussions on data privacy and protection. With AI systems relying heavily on vast amounts of data, it is crucial that regulations enforce strict data handling practices. This includes ensuring that personal data is collected, stored, and utilized in a manner that respects individual privacy rights. For instance, the General Data Protection Regulation (GDPR) in Europe sets a high standard for data protection, influencing how AI systems operate within its jurisdiction.
To illustrate the differences in regulatory approaches, here’s a brief comparison:
Region | Key Regulation | Focus Areas |
---|---|---|
European Union | Artificial Intelligence Act | Risk assessment, accountability, transparency |
United States | Varied State Regulations | Data privacy, ethical use, transparency |
China | AI Development Guidelines | National security, societal benefit |
As we continue to navigate this complex landscape, it’s important for stakeholders—including developers, policymakers, and the public—to engage in ongoing dialogue about the implications of AI regulations. This conversation is vital not only for shaping effective policies but also for ensuring that AI technologies serve the greater good without infringing on individual rights.
In conclusion, regulatory frameworks are not just bureaucratic hurdles; they are necessary safeguards that can help steer the development of AI towards ethical and responsible practices. As these regulations evolve, they will likely play a pivotal role in determining how AI impacts our lives in the future.
- What are regulatory frameworks in AI?
Regulatory frameworks are guidelines and rules designed to govern the ethical development, deployment, and use of AI technologies. - Why are these frameworks important?
They help ensure accountability, transparency, and protection of individual rights, fostering trust in AI systems. - How do regulations differ across regions?
Different regions have varying approaches; for example, the EU has the Artificial Intelligence Act, while the US has a more fragmented regulatory landscape. - What role does data privacy play in AI regulations?
Data privacy is a critical focus area, ensuring that personal data is handled ethically and in compliance with privacy laws like GDPR.
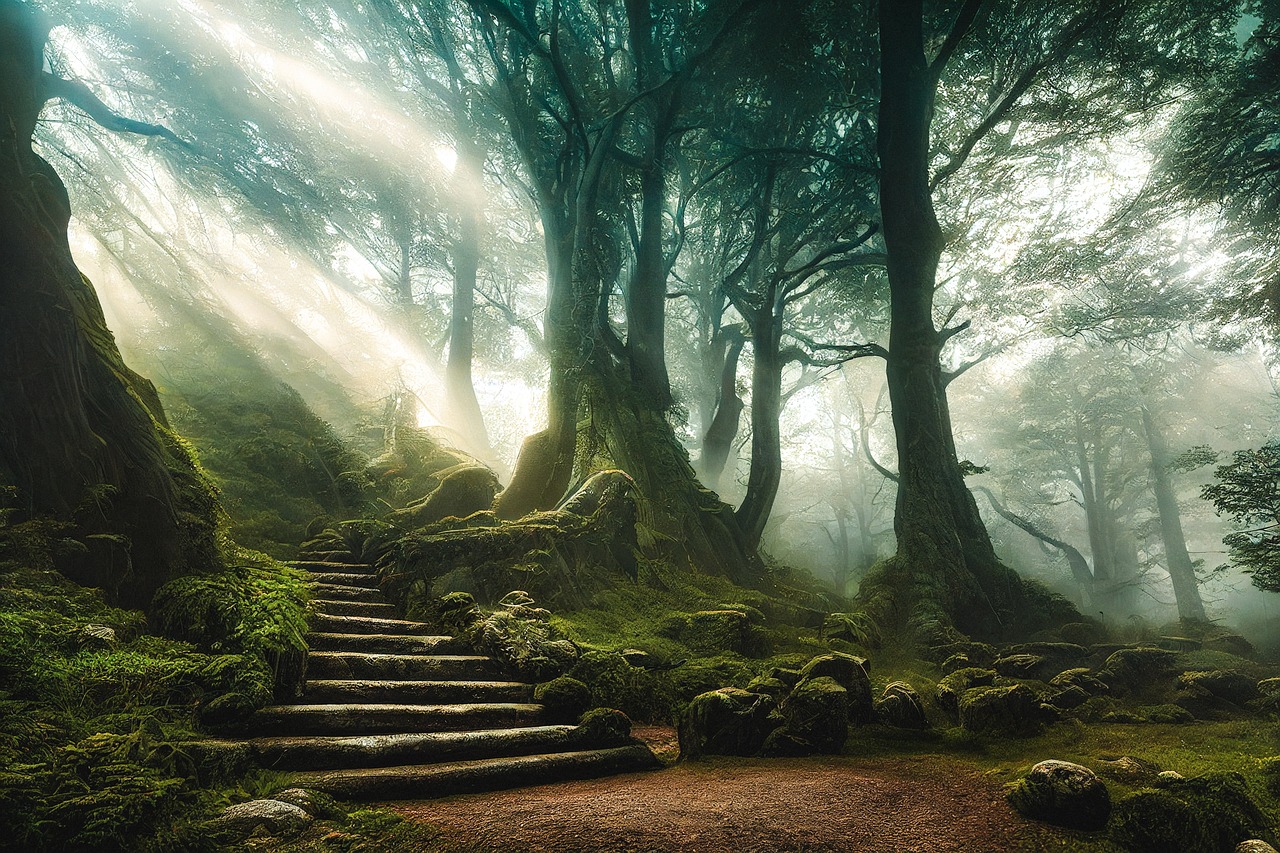
Industry Best Practices
In the rapidly evolving landscape of artificial intelligence, adhering to best practices is essential for ensuring that AI technologies are developed and deployed ethically. Organizations must prioritize responsible AI practices to foster trust and mitigate potential risks. One of the primary best practices is to implement a robust ethical framework that guides every stage of AI development, from conception through deployment. This framework should encompass principles such as fairness, accountability, and transparency, ensuring that all stakeholders are aligned with ethical standards.
Moreover, organizations should conduct thorough impact assessments before launching AI systems. These assessments evaluate the potential social, economic, and environmental impacts of AI applications, allowing organizations to identify and address any ethical concerns proactively. By engaging with diverse stakeholders, including ethicists, community representatives, and industry experts, companies can gain multifaceted insights that enhance the ethical integrity of their AI solutions.
Another critical aspect of best practices in AI is the establishment of interdisciplinary teams. By bringing together professionals from various fields—such as data science, law, ethics, and sociology—organizations can ensure that different perspectives are considered during the AI development process. This diversity of thought helps to identify potential biases and ethical dilemmas early on, allowing teams to create more inclusive and fair AI systems.
Furthermore, organizations should prioritize continuous monitoring and evaluation of their AI systems post-deployment. This practice involves regularly assessing the performance of AI technologies to ensure they function as intended and do not perpetuate biases or cause unintended harm. By employing techniques such as algorithmic auditing and user feedback mechanisms, organizations can make necessary adjustments and improvements to their systems, thereby reinforcing their commitment to ethical AI.
To illustrate these best practices, consider a table that outlines key strategies and their corresponding benefits:
Best Practice | Description | Benefits |
---|---|---|
Ethical Framework | Establishing guidelines for ethical AI development. | Ensures alignment with ethical standards and fosters trust. |
Impact Assessments | Evaluating potential impacts of AI applications. | Identifies ethical concerns proactively. |
Interdisciplinary Teams | Bringing together diverse expertise. | Enhances inclusivity and reduces biases. |
Continuous Monitoring | Regular assessment of AI systems after deployment. | Ensures systems function as intended and adapt to changes. |
By embracing these industry best practices, organizations can not only improve the ethical standards of their AI technologies but also enhance their reputation and build lasting relationships with users and stakeholders. In a world where AI is becoming increasingly integrated into our daily lives, the responsibility to act ethically lies with all of us—developers, organizations, and policymakers alike. So, let’s work together to ensure that the future of AI is not just intelligent but also responsible.
- What are the main ethical principles in AI?
The main ethical principles in AI include fairness, accountability, transparency, and privacy. These principles guide the development and implementation of AI technologies to ensure they are used responsibly.
- Why is fairness important in AI?
Fairness is crucial in AI to prevent biases that can lead to discriminatory outcomes. Ensuring equitable treatment across different populations fosters trust and promotes social justice.
- How can organizations monitor AI systems post-deployment?
Organizations can monitor AI systems through algorithmic auditing, user feedback, and performance evaluations to ensure that the systems operate as intended and do not cause harm.
- What role do interdisciplinary teams play in AI ethics?
Interdisciplinary teams bring together diverse expertise, which helps identify potential biases and ethical dilemmas during AI development, leading to more inclusive and fair technologies.
Frequently Asked Questions
- What is AI ethics and why is it important?
AI ethics refers to the moral principles and guidelines that govern the development and use of artificial intelligence. It's crucial because it helps ensure that AI technologies are designed and implemented in ways that are fair, accountable, and transparent, ultimately protecting individuals and society from potential harm.
- How can fairness be achieved in AI systems?
Achieving fairness in AI involves identifying and eliminating biases in algorithms. This can be done through various strategies, such as using diverse training data, employing bias detection techniques, and continuously monitoring AI outputs to ensure equitable treatment across different demographics.
- What are some common bias detection techniques?
Some common bias detection techniques include statistical analysis, auditing algorithms for disparate impact, and employing fairness metrics. These methods help identify areas where AI systems may be inadvertently favoring one group over another, allowing developers to make necessary adjustments.
- Can you provide examples of fairness issues in AI?
Certainly! For instance, facial recognition systems have been shown to misidentify individuals from certain racial and ethnic backgrounds more frequently than others. Another example is hiring algorithms that may favor candidates from specific demographics, leading to unequal job opportunities.
- What privacy concerns arise with AI technologies?
Privacy concerns in AI typically stem from the extensive data collection and analysis required for AI systems to function effectively. This can lead to unauthorized data usage, surveillance, and potential breaches of personal privacy, making it essential to implement strong data protection measures.
- Who is responsible for ensuring accountability in AI?
Accountability in AI systems involves multiple stakeholders, including developers, organizations, and policymakers. Each plays a critical role in establishing ethical standards, ensuring compliance with regulations, and fostering a culture of responsibility within the AI ecosystem.
- What regulatory frameworks exist for AI ethics?
Various regulatory frameworks are being developed globally to address AI ethics. These include guidelines from government bodies, industry standards, and international agreements aimed at promoting ethical AI practices and ensuring that technologies are used responsibly.
- What are some best practices for ethical AI development?
Best practices for ethical AI development include conducting thorough impact assessments, involving diverse teams in the design process, ensuring transparency in AI decision-making, and engaging with stakeholders to gather feedback and address concerns throughout the development lifecycle.