Navigating the Future: AI Innovations in GIS Technology
In a world that is constantly evolving, the integration of artificial intelligence (AI) into Geographic Information Systems (GIS) is nothing short of a revolution. Imagine a landscape where data is not just collected but transformed into actionable insights that drive decision-making across industries. This transformation is not just a trend; it's a fundamental shift in how we perceive and utilize geographic data. With AI at the helm, GIS technology is becoming smarter, faster, and more intuitive, enabling us to tackle complex challenges in urban planning, environmental management, and disaster response with unprecedented efficiency.
The marriage of AI and GIS is akin to pairing a fine wine with gourmet food—it enhances the overall experience. As we delve deeper into the impact of AI on GIS, we uncover innovations that are reshaping our understanding of spatial data and its applications. From predictive analytics that forecast urban growth to natural language processing that allows users to interact with GIS systems using everyday language, the possibilities are limitless. This article will explore these exciting advancements, highlighting how they are not only improving efficiency but also democratizing access to geographic information.
As we navigate through this transformative journey, it’s essential to recognize that the implications of AI in GIS extend far beyond mere technological enhancements. They are fundamentally altering how we think about data, making it more accessible and actionable for a broader audience. So, buckle up as we explore the rise of AI in GIS, enhanced data analysis techniques, real-time data integration, and the future trends that promise to take us even further into this brave new world.
Artificial intelligence is revolutionizing GIS by enhancing data processing capabilities, enabling more accurate spatial analysis, and improving decision-making processes across various sectors, including urban planning, environmental management, and disaster response. The rise of AI in GIS is not just about automation; it’s about enhancing human capabilities. By leveraging AI, GIS professionals can focus on strategic decision-making rather than getting bogged down by data processing tasks. This shift allows for a more efficient workflow, where insights can be derived faster and with greater accuracy.
Moreover, AI's ability to analyze vast amounts of data at lightning speed means that organizations can respond to changes in real-time. For instance, in urban planning, AI can analyze traffic patterns, population growth, and environmental impacts simultaneously, providing planners with a comprehensive view of potential developments. This capability is invaluable, particularly in today’s fast-paced world where timely decisions can make all the difference.
AI-driven algorithms are enabling more sophisticated data analysis techniques in GIS, allowing for the extraction of meaningful insights from vast datasets and improving predictive modeling for various applications. With machine learning at the forefront, GIS can now automate tasks that were once tedious and time-consuming. Imagine a system that learns from historical data and can predict future trends—this is the power of AI in action.
Machine learning is a subset of AI that provides powerful tools for GIS, enabling automated pattern recognition, anomaly detection, and classification of spatial data to enhance overall analysis and visualization. For example, by training algorithms on historical data, GIS can identify patterns that might not be immediately visible to human analysts. This capability not only saves time but also uncovers insights that can lead to better decision-making.
Deep learning techniques are particularly effective in processing satellite imagery and aerial photographs, facilitating accurate land cover classification, object detection, and change detection over time. This is crucial for applications such as environmental monitoring and urban development, where understanding changes in land use is vital. The ability to automatically classify and detect changes in imagery allows for quicker responses to environmental issues and more informed urban planning decisions.
Predictive analytics powered by AI can forecast future trends in urban development, environmental changes, and resource management, providing valuable insights for strategic planning and policy-making. By analyzing current and historical data, AI can help stakeholders anticipate challenges and opportunities, making it an essential tool for effective governance and resource allocation.
Natural language processing (NLP) is enhancing GIS by enabling users to query spatial data using everyday language, making geographic information more accessible and user-friendly for non-experts. Imagine being able to ask a GIS system, "Where are the nearest parks?" instead of having to navigate complex menus and technical jargon. This accessibility opens the doors for more people to engage with GIS technology, fostering a greater understanding of spatial data and its implications.
AI innovations facilitate real-time data integration from various sources, including IoT devices and social media, allowing GIS applications to respond dynamically to changing conditions and improve situational awareness. This capability is particularly beneficial in scenarios where timely information is critical, such as in emergency management or urban traffic control.
AI-enhanced GIS is playing a crucial role in smart city initiatives, optimizing urban infrastructure, traffic management, and public services through real-time data analysis and visualization. By integrating various data sources, cities can improve their responsiveness to citizen needs and enhance overall quality of life.
The integration of AI in GIS is transforming disaster management by providing timely and accurate information for emergency responders, enhancing risk assessment, and improving recovery strategies after natural disasters. In the face of climate change and increasing natural disasters, the ability to predict and respond effectively can save lives and resources.
The future of AI in GIS holds exciting possibilities, including advancements in automation, increased accuracy in spatial analysis, and the development of more intuitive user interfaces that will further democratize geographic information access. As technology continues to evolve, we can expect even more innovative solutions that will empower individuals and organizations alike to make informed decisions based on geographic data.
- What is GIS? GIS stands for Geographic Information Systems, a framework for gathering, managing, and analyzing spatial and geographic data.
- How does AI enhance GIS? AI enhances GIS by automating data analysis, improving accuracy, and enabling real-time decision-making.
- What are some applications of AI in GIS? Applications include urban planning, environmental monitoring, disaster response, and smart city initiatives.
- Is AI in GIS accessible for non-experts? Yes, advancements in natural language processing are making GIS more user-friendly for non-experts.
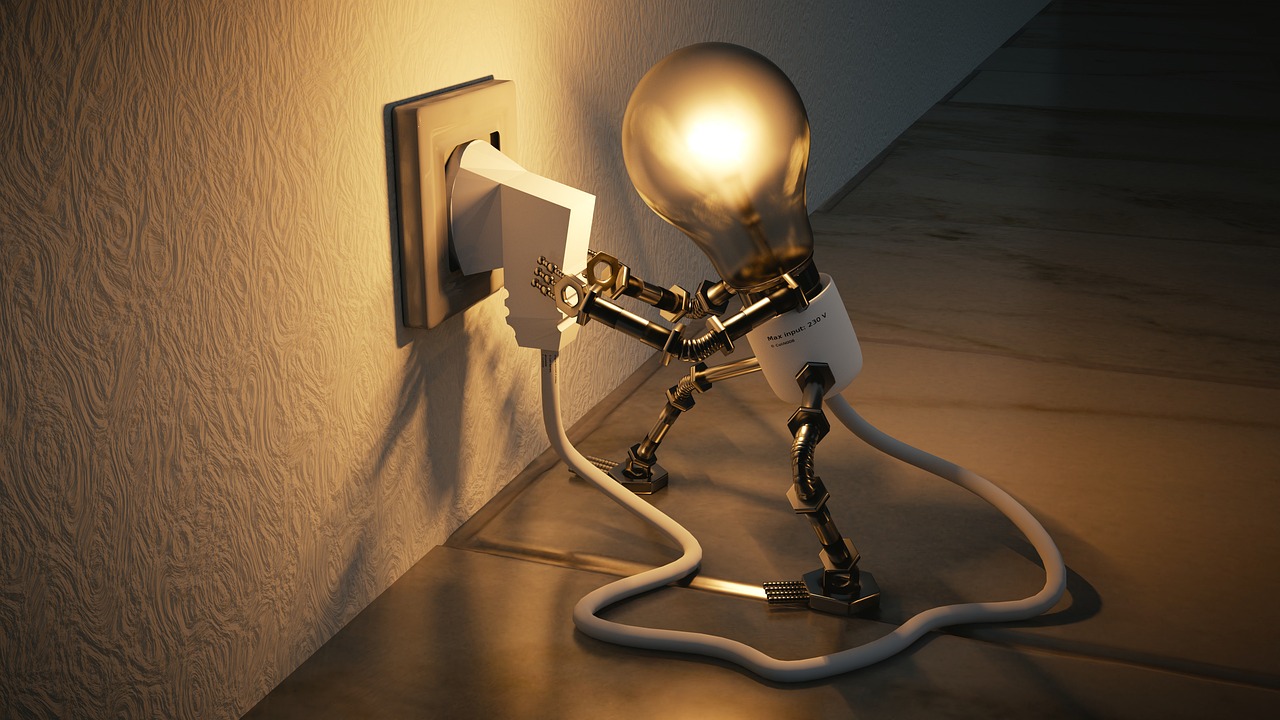
[The Rise of AI in GIS]
Artificial intelligence is not just a buzzword; it’s a game-changer in the realm of Geographic Information Systems (GIS). Imagine having the ability to sift through massive amounts of geographical data in mere seconds, uncovering patterns and insights that would take a human analyst days, if not weeks, to discover. This is the power of AI, and it is revolutionizing how we understand and interact with our world. From urban planning to environmental management, AI is enhancing the way we analyze spatial data, making it more accurate and efficient.
The integration of AI into GIS is akin to adding a turbocharger to an already powerful engine. It amplifies the capabilities of traditional GIS by enabling more sophisticated data processing techniques. For instance, AI algorithms can analyze satellite imagery to identify land use changes over time, detect illegal logging in rainforests, or even predict traffic patterns in urban areas. These advancements are not just theoretical; they are being applied in real-world scenarios, transforming decision-making processes across various sectors.
One of the most significant benefits of AI in GIS is its ability to enhance decision-making processes. By utilizing advanced algorithms, GIS can now provide insights that were previously unattainable. For example, in urban planning, AI can analyze demographic data, land use, and environmental factors to suggest optimal locations for new developments. This not only saves time but also leads to more informed decisions that can positively impact communities.
The rise of AI in GIS is also democratizing access to geographic information. With user-friendly interfaces and natural language processing capabilities, even those without technical expertise can engage with complex spatial data. This shift is crucial in making geographic information more accessible to a broader audience, allowing community members to participate in planning and decision-making processes.
In summary, the rise of AI in GIS is a transformative force that is reshaping the landscape of geographic data analysis. By enhancing data processing capabilities, improving accuracy, and facilitating better decision-making, AI is paving the way for a future where spatial analysis is not just for experts, but for everyone. As we continue to explore the intersection of AI and GIS, the possibilities seem endless, promising a more informed and connected world.
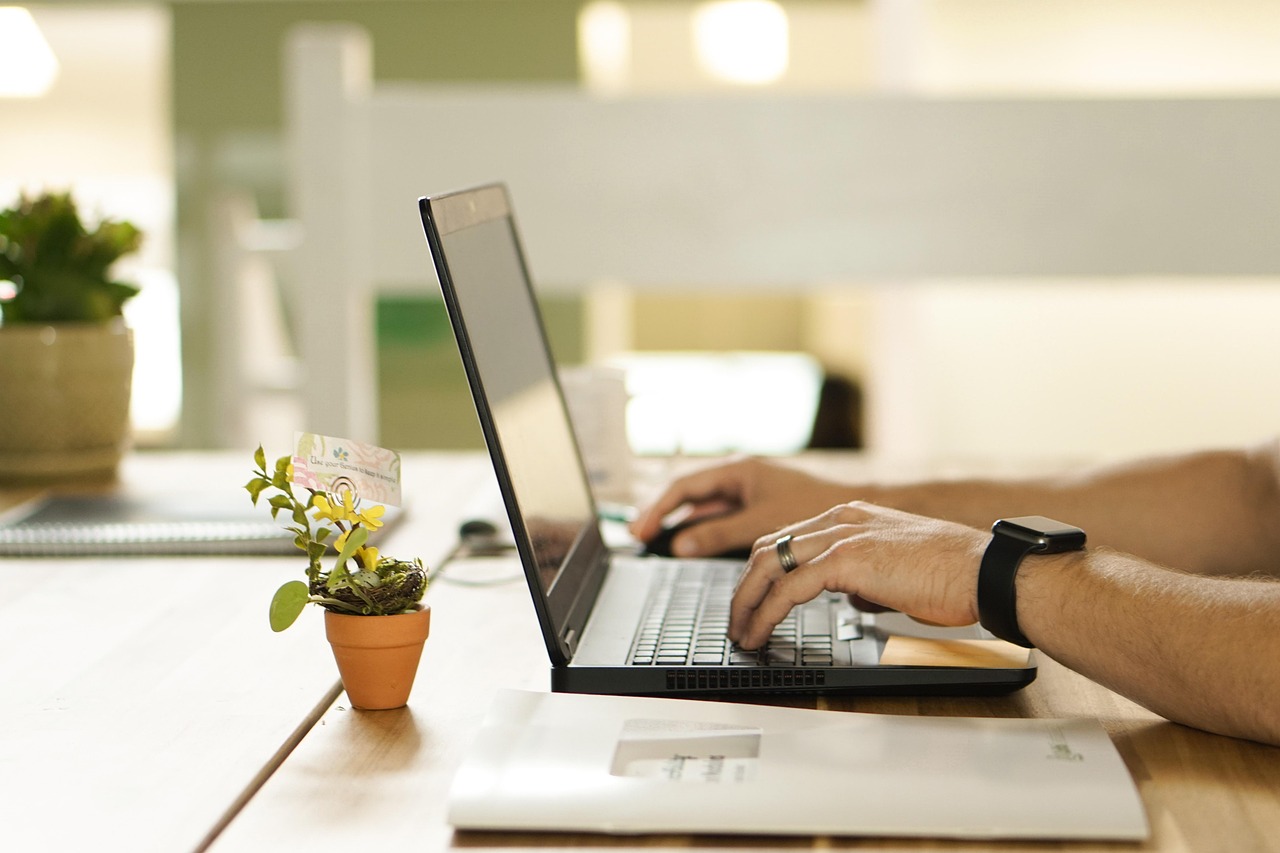
[Enhanced Data Analysis Techniques]
Artificial Intelligence is not just a buzzword; it's a game-changer in the realm of Geographic Information Systems (GIS). The integration of AI-driven algorithms is reshaping how we analyze data, enabling us to extract meaningful insights from vast datasets that were once overwhelming. Imagine sifting through mountains of information, trying to find that one crucial piece of data. With AI, this process becomes not only faster but also more accurate. It's like having a supercharged assistant who can quickly pinpoint what you need, allowing you to focus on making informed decisions rather than getting lost in the data jungle.
One of the most significant advancements in this field is the use of machine learning. This subset of AI provides powerful tools that automate complex tasks, such as pattern recognition and anomaly detection. For instance, consider a city planner trying to identify traffic congestion patterns. Traditionally, this would require hours of manual analysis. However, with machine learning, algorithms can analyze historical traffic data, identify trends, and even predict future congestion points. This not only saves time but also enhances the overall analysis and visualization of spatial data, making it easier for decision-makers to act swiftly.
Machine learning applications in GIS are vast and varied. They can be employed in numerous sectors, including:
- Urban Planning: Optimizing land use and infrastructure development.
- Environmental Monitoring: Tracking changes in ecosystems and biodiversity.
- Public Health: Analyzing spatial data related to disease outbreaks.
By leveraging these applications, organizations can enhance their operational efficiency and effectiveness in addressing complex challenges.
Deep learning, a more advanced form of machine learning, has made significant strides in image processing within GIS. It excels at analyzing satellite imagery and aerial photographs, facilitating tasks such as land cover classification, object detection, and change detection over time. For example, deep learning algorithms can automatically classify images to distinguish between urban areas, forests, and water bodies. This capability is crucial for environmental management, urban planning, and disaster response, as it provides accurate and timely information that can be acted upon quickly.
Another exciting aspect of AI in GIS is predictive analytics. Imagine being able to forecast future trends in urban development or environmental changes with a high degree of accuracy. AI-powered predictive analytics can analyze historical data and current trends to provide insights that are invaluable for strategic planning and policy-making. For instance, city officials can use predictive models to anticipate population growth and its impact on infrastructure needs, allowing them to proactively address challenges before they become crises.
In summary, the enhanced data analysis techniques driven by AI are revolutionizing GIS. By automating complex analysis, improving accuracy, and providing predictive insights, organizations can make better decisions that lead to more effective urban planning, environmental management, and disaster response strategies.
Q1: How does AI improve data analysis in GIS?
A1: AI enhances data analysis in GIS by automating complex tasks, enabling faster processing of large datasets, and providing predictive insights that inform decision-making.
Q2: What are some applications of machine learning in GIS?
A2: Machine learning is used in urban planning, environmental monitoring, and public health to analyze spatial data and identify patterns, trends, and anomalies.
Q3: How does deep learning differ from traditional machine learning in GIS?
A3: Deep learning is a more advanced subset of machine learning that excels at processing and analyzing complex data types, such as images, making it particularly effective for tasks like land cover classification and object detection.
Q4: What role does predictive analytics play in GIS?
A4: Predictive analytics in GIS allows organizations to forecast future trends, enabling proactive decision-making in areas like urban development and environmental management.
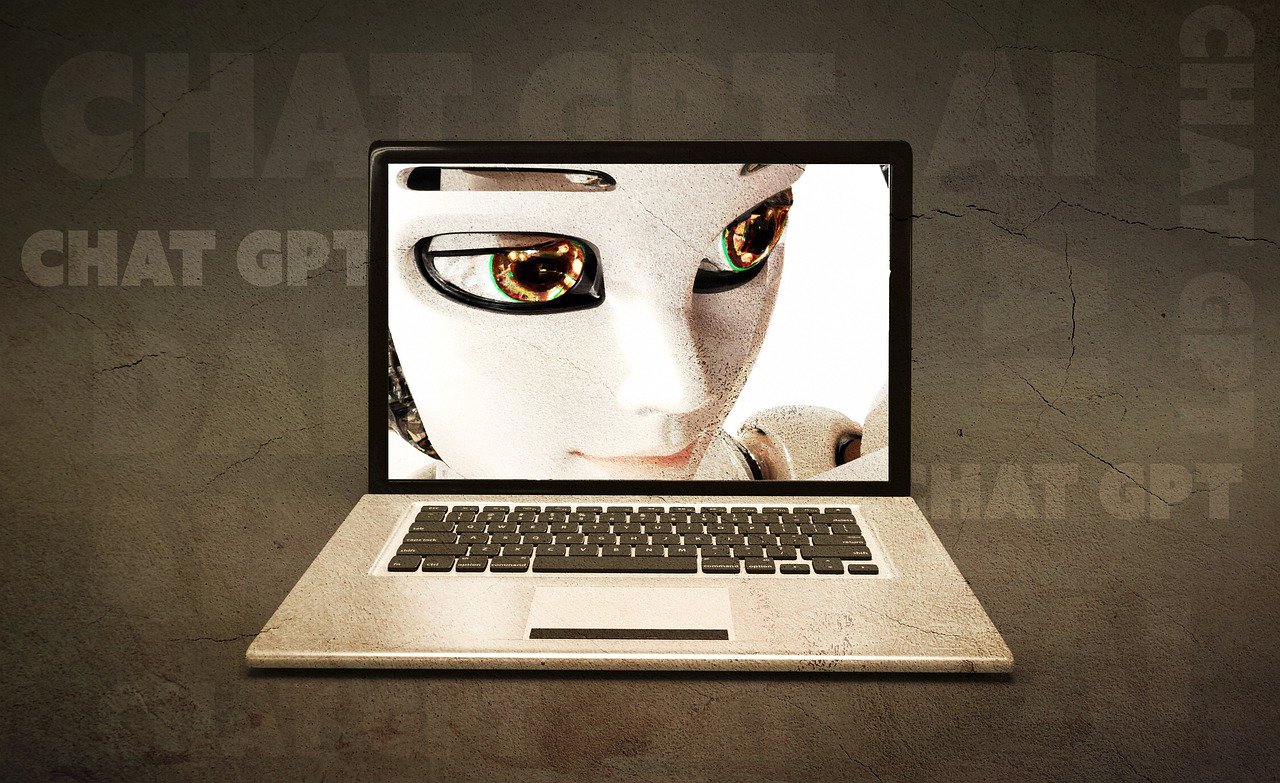
[Machine Learning Applications]
Machine learning is not just a buzzword; it’s a game-changer in the realm of Geographic Information Systems (GIS). Imagine having a super-smart assistant that can sift through mountains of data, identify patterns, and highlight anomalies without breaking a sweat. That’s precisely what machine learning does for GIS. By leveraging sophisticated algorithms, it allows for the automated recognition of patterns in spatial data, which is crucial for various applications ranging from urban planning to environmental monitoring.
But how does this actually work? Well, machine learning algorithms are trained using historical data, enabling them to learn from past instances and make predictions about future occurrences. For example, in urban planning, these algorithms can analyze traffic patterns and predict congestion points, helping city planners devise more efficient traffic management strategies. This predictive capability is not just limited to traffic; it extends to environmental changes, resource allocation, and even public health initiatives.
One of the most fascinating aspects of machine learning in GIS is its ability to enhance data visualization. Imagine a city’s heat map that dynamically updates based on real-time data inputs. Machine learning can analyze this data to provide insights into areas that require urgent attention, such as high pollution zones or regions prone to flooding. This is where the magic happens: it transforms raw data into actionable insights that can guide decision-making.
Moreover, machine learning facilitates the classification of spatial data, making it easier to categorize different land uses, detect changes over time, and even identify illegal activities such as deforestation or urban encroachment. For instance, by training algorithms on satellite imagery, GIS can automatically classify land cover types with remarkable accuracy. This not only saves time but also reduces human error, leading to more reliable outcomes.
To give you a clearer picture, here’s a simple breakdown of how machine learning enhances GIS:
Application | Description |
---|---|
Pattern Recognition | Identifies trends and anomalies in spatial data. |
Predictive Modeling | Forecasts future scenarios based on historical data. |
Data Classification | Automates the categorization of land use and cover types. |
Change Detection | Monitors alterations in the landscape over time. |
In essence, machine learning is like a magnifying glass for GIS—allowing us to zoom in on the details that matter most. It empowers us to make informed decisions based on data-driven insights, ultimately leading to smarter, more sustainable solutions. As we continue to harness the power of machine learning, the possibilities for GIS applications are practically endless.
- What is machine learning? Machine learning is a subset of artificial intelligence that uses algorithms to analyze data, learn from it, and make predictions or decisions without being explicitly programmed.
- How does machine learning improve GIS? It enhances GIS by enabling automated pattern recognition, predictive modeling, and data classification, making spatial analysis more efficient and accurate.
- Can machine learning predict environmental changes? Yes, machine learning algorithms can analyze historical data to forecast future environmental changes, aiding in resource management and urban planning.
- Is machine learning accessible for non-experts? With advancements in natural language processing and user-friendly interfaces, machine learning applications in GIS are becoming increasingly accessible to non-experts.
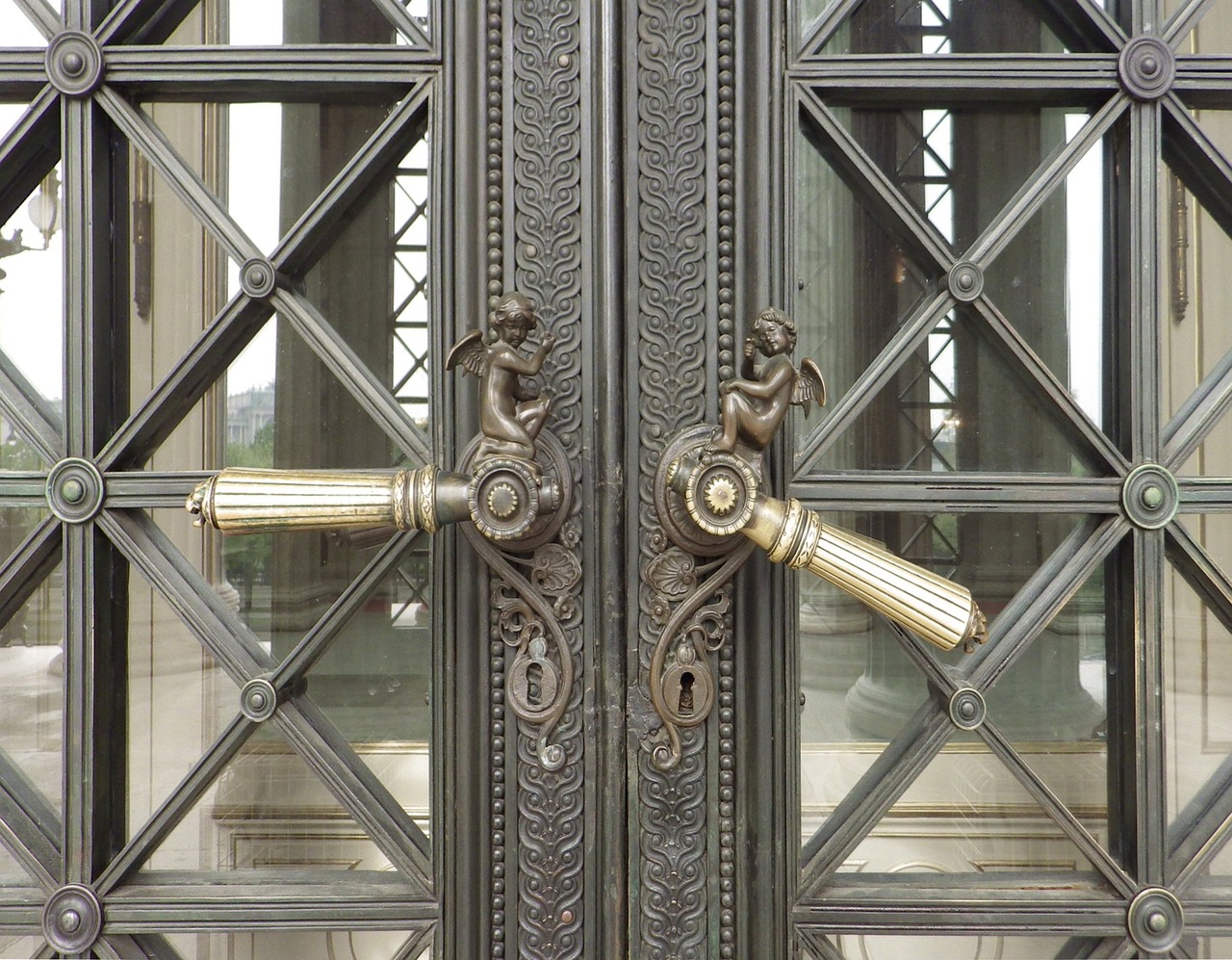
[Deep Learning in Image Processing]
Deep learning has emerged as a game-changer in the realm of image processing, especially within Geographic Information Systems (GIS). This technology utilizes neural networks that mimic the human brain's functioning, allowing computers to learn from vast amounts of data. Imagine teaching a child to recognize different animals by showing them thousands of pictures; similarly, deep learning algorithms process countless images to identify patterns and features. This capability is particularly beneficial when analyzing satellite imagery and aerial photographs, where the details can be overwhelming for traditional methods.
One of the most significant applications of deep learning in GIS is land cover classification. For instance, when a city plans to expand, understanding the current land use—whether it's residential, commercial, agricultural, or forested—is crucial. Deep learning models can accurately classify these land types with remarkable precision, enabling planners to make informed decisions. By training on diverse datasets, these models can also adapt to different geographical contexts, ensuring that the analysis remains relevant across various regions.
Moreover, deep learning excels in object detection, which involves identifying and locating specific features within an image. This can include anything from buildings and roads to natural features like rivers and mountains. The ability to automatically detect these objects not only speeds up the analysis process but also enhances the accuracy of spatial data interpretation. In disaster response scenarios, for example, quickly identifying damaged infrastructure can significantly improve the effectiveness of rescue operations.
Change detection is another critical area where deep learning shines. By comparing images taken at different times, algorithms can detect alterations in land use, vegetation cover, or urban development. This capability is invaluable for environmental monitoring and urban planning. For instance, a city may want to track how much green space has been lost over the years due to urbanization. Deep learning models can automatically analyze this data, providing insights that would be labor-intensive and time-consuming to gather manually.
The integration of deep learning in image processing is not without challenges, however. Training these models requires substantial computational power and access to high-quality datasets. Additionally, there are concerns regarding the interpretability of deep learning models; understanding how these algorithms make decisions can be complex. Yet, as technology continues to advance, the benefits of deep learning in GIS are becoming increasingly apparent, paving the way for more sophisticated and accessible geographic analysis.
In summary, deep learning is revolutionizing image processing in GIS by enhancing land cover classification, improving object detection, and facilitating effective change detection. As we embrace these innovations, the potential for more informed decision-making in urban planning, environmental management, and disaster response grows exponentially.
- What is deep learning? Deep learning is a subset of machine learning that uses neural networks to analyze and learn from large amounts of data.
- How does deep learning improve image processing in GIS? It enhances accuracy in identifying and classifying features in images, enabling better analysis and decision-making.
- What are some applications of deep learning in GIS? Applications include land cover classification, object detection, and change detection in satellite and aerial imagery.
- What are the challenges of implementing deep learning in GIS? Challenges include the need for large datasets, computational resources, and the complexity of model interpretability.
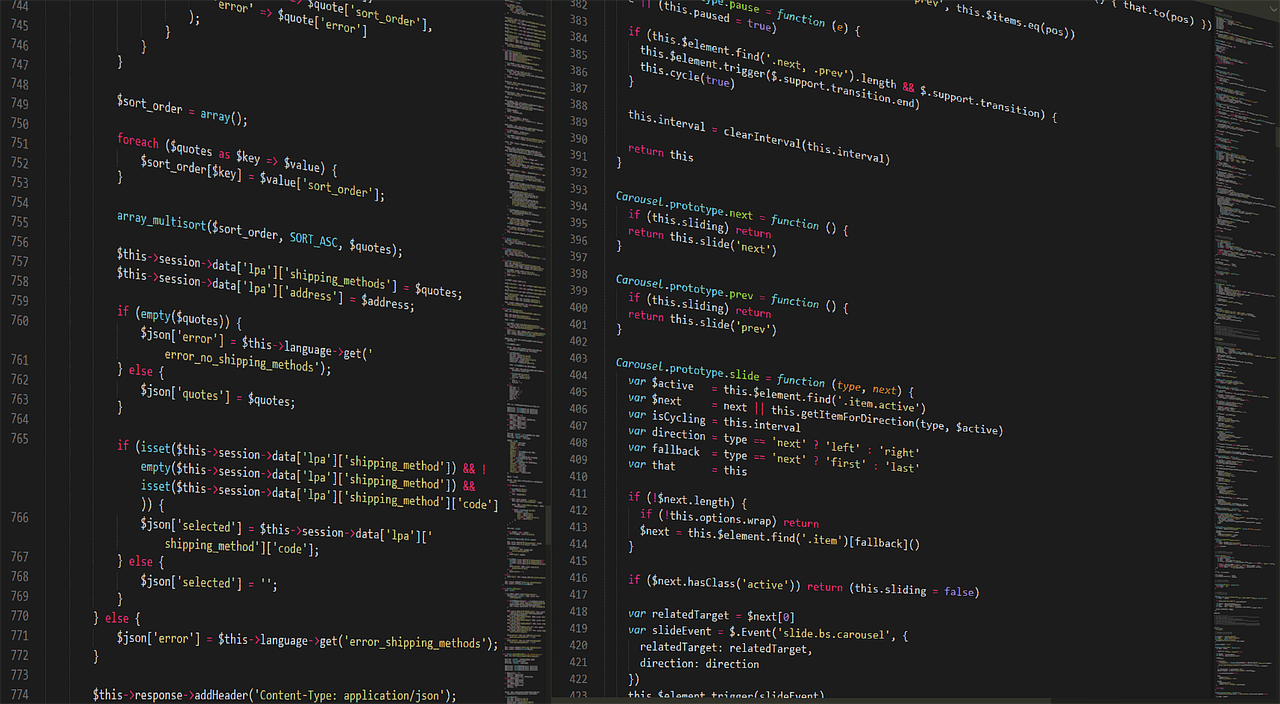
[Predictive Analytics]
Predictive analytics is rapidly becoming a cornerstone of Geographic Information Systems (GIS) thanks to the power of artificial intelligence. Imagine having a crystal ball that not only helps you see the future but also guides you in making informed decisions based on data. That’s the essence of predictive analytics in GIS—it’s about leveraging historical data and advanced algorithms to forecast future trends and outcomes. This capability is invaluable across various sectors, from urban planning to environmental conservation.
At its core, predictive analytics involves using statistical algorithms and machine learning techniques to identify patterns in historical data. By analyzing these patterns, GIS professionals can make educated predictions about future scenarios. For instance, urban planners can predict traffic congestion patterns based on previous data, allowing for more effective road designs and public transport routes. Similarly, environmental scientists can anticipate changes in ecosystems due to climate change, enabling proactive measures to protect vulnerable habitats.
One of the most exciting aspects of predictive analytics in GIS is its ability to integrate with real-time data. This means that as new data comes in—be it from sensors, social media, or satellite imagery—the predictive models can be updated to reflect the most current information. This dynamic approach allows for timely interventions in various fields. For example, during a natural disaster, predictive analytics can help emergency responders anticipate areas that will be affected next, thereby optimizing resource allocation and response strategies.
To illustrate the impact of predictive analytics in GIS, consider the following table that highlights some key applications and their benefits:
Application | Description | Benefits |
---|---|---|
Urban Planning | Forecasting traffic patterns and population growth | Improved infrastructure planning and reduced congestion |
Environmental Monitoring | Predicting changes in land use and natural resources | Enhanced conservation efforts and sustainable management |
Disaster Preparedness | Anticipating the impact of natural disasters | Better resource allocation and quicker response times |
As we look to the future, the potential for predictive analytics in GIS continues to expand. With advancements in AI and machine learning, we can expect even more sophisticated models that will enhance accuracy and reliability. Imagine being able to predict not just where a flood might happen, but also how it will affect different communities, infrastructure, and ecosystems. The implications are profound, offering a pathway to not only react to but also proactively manage challenges before they escalate.
In conclusion, predictive analytics is not just a trend; it’s a transformative tool that is reshaping how we interact with geographic data. By harnessing the power of AI, GIS professionals can create smarter, more responsive systems that benefit society as a whole. The future is bright, and as we continue to innovate, the possibilities for predictive analytics in GIS are limitless.
- What is predictive analytics in GIS?
Predictive analytics in GIS refers to the use of statistical algorithms and machine learning techniques to analyze historical data and predict future trends related to geographic information. - How does AI enhance predictive analytics?
AI enhances predictive analytics by automating data processing, improving pattern recognition, and enabling real-time updates to predictive models based on new incoming data. - What are some applications of predictive analytics in GIS?
Applications include urban planning, environmental monitoring, and disaster preparedness, where predictive analytics helps in making informed decisions based on forecasts. - Can predictive analytics help in disaster management?
Yes, predictive analytics can significantly improve disaster management by forecasting potential impacts and helping emergency responders allocate resources more effectively.
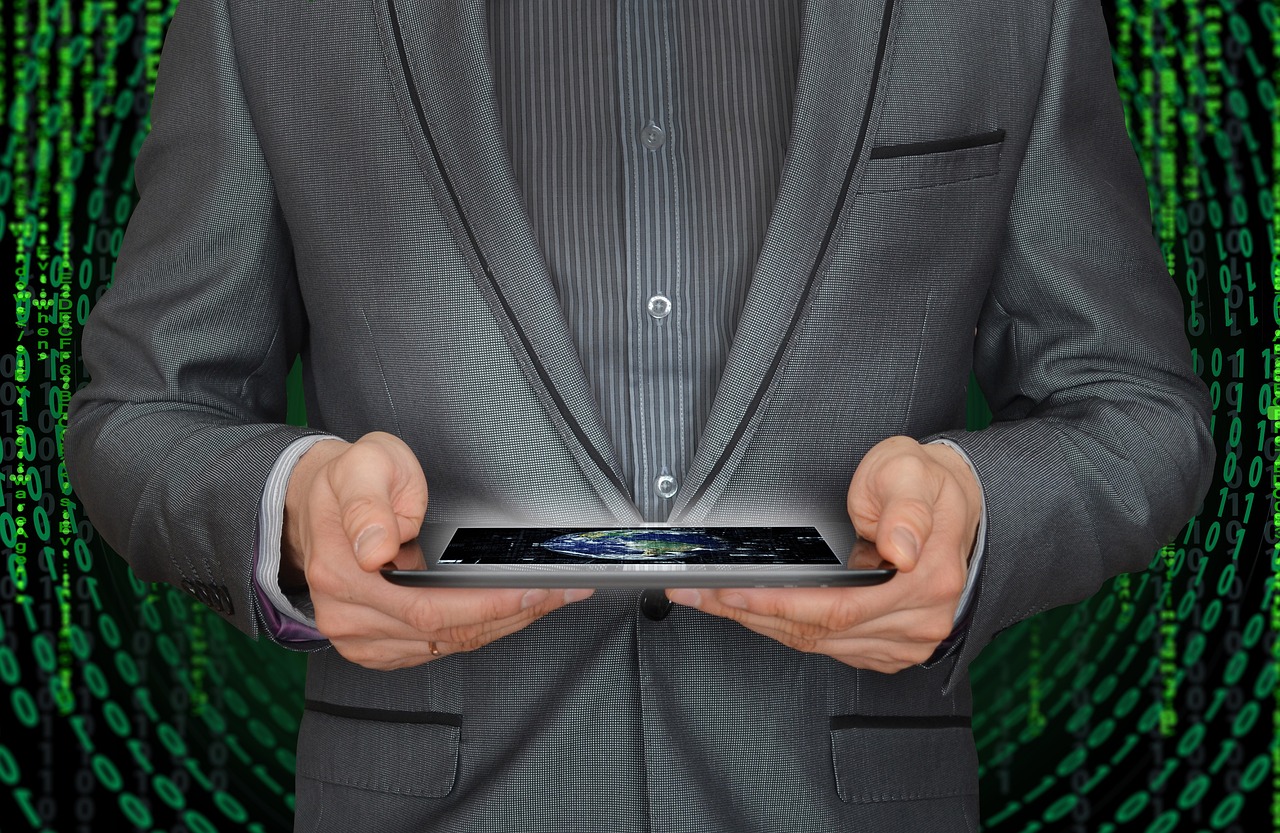
[Natural Language Processing in GIS]
Natural Language Processing, or NLP, is rapidly becoming a game-changer in the world of Geographic Information Systems (GIS). Imagine being able to interact with complex spatial data using nothing but your everyday language. Sounds like science fiction, right? Well, it's not! With NLP, users can now query geographic information without needing to master complex technical jargon or coding languages. This accessibility is crucial in democratizing GIS, making it usable for everyone—from city planners to community activists.
One of the most exciting aspects of NLP in GIS is its ability to transform how we retrieve and analyze spatial data. For instance, instead of having to sift through layers of data and technical specifications, a user could simply ask, “What are the flood zones in my area?” The system, powered by NLP algorithms, can interpret this query, understand the context, and deliver precise information in a matter of seconds. This not only saves time but also makes GIS more intuitive and user-friendly, allowing non-experts to harness the power of geographic data.
Moreover, NLP can enhance the searchability of spatial data. By enabling users to input queries in natural language, GIS systems can improve their search algorithms to return more relevant results. Imagine a scenario where urban planners could type in, “Show me areas with high traffic congestion during rush hour,” and receive detailed maps and analyses that highlight the most affected zones. This capability can significantly improve decision-making processes, making urban planning more responsive to real-time needs.
Another fascinating application of NLP in GIS is its role in sentiment analysis. By analyzing social media feeds and public comments, NLP can help GIS professionals understand public opinion on various geographic issues, such as urban development projects or environmental concerns. This can lead to more informed decision-making that considers community sentiment and needs. For example, if a city is planning to develop a new park, analyzing tweets and posts about local green spaces can provide valuable insights into what the community values most.
To summarize, the integration of NLP within GIS is not just a technological advancement; it represents a fundamental shift in how we interact with geographic data. As NLP continues to evolve, we can expect even more innovative applications that will further enhance the accessibility and usability of GIS. The future is bright, and the potential for NLP in GIS is truly limitless!
- What is Natural Language Processing (NLP)?
NLP is a branch of artificial intelligence that focuses on the interaction between computers and humans through natural language. - How does NLP improve GIS?
NLP allows users to query spatial data using everyday language, making GIS more accessible and easier to use for non-experts. - Can NLP analyze social media data?
Yes, NLP can analyze social media feeds to gauge public sentiment on geographic issues, providing valuable insights for decision-making. - Is NLP only beneficial for experts?
No, NLP is designed to make GIS accessible to everyone, including those without technical expertise.
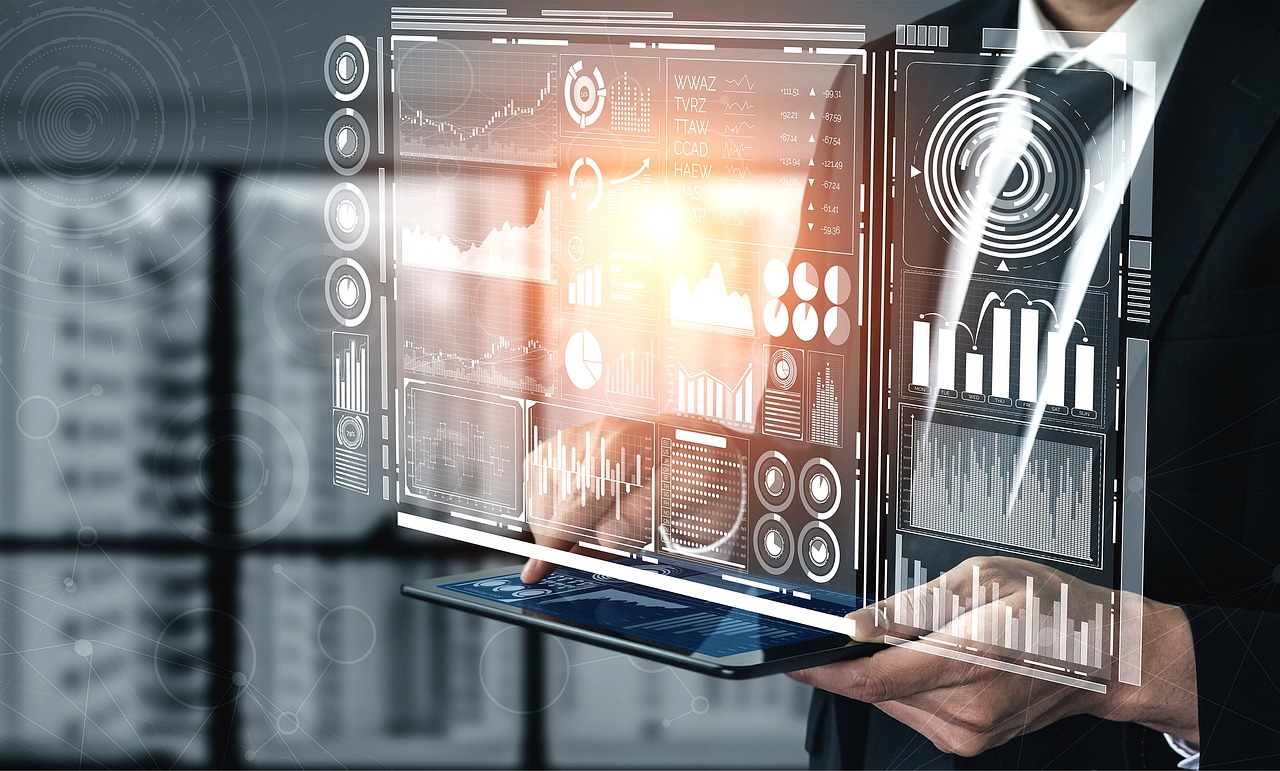
[Real-Time Data Integration]
In today’s fast-paced world, the ability to integrate data in real-time is more crucial than ever. Geographic Information Systems (GIS) are stepping up to the plate, thanks to the remarkable advancements brought about by artificial intelligence (AI). Imagine a city where traffic lights adjust based on real-time traffic conditions or where emergency responders have immediate access to the latest data during a crisis. This is not just a dream—it's becoming a reality through AI-enhanced GIS technology.
Real-time data integration allows GIS applications to pull information from a myriad of sources, including Internet of Things (IoT) devices, social media platforms, and even satellite feeds. This dynamic influx of data enables organizations to respond proactively to evolving situations. For example, during a natural disaster, real-time data can help assess damage, monitor the movement of people, and optimize resource allocation. Think of it as having a live map that updates every second, giving you the most accurate picture of what’s happening.
Furthermore, the integration of AI with GIS not only enhances situational awareness but also streamlines decision-making processes. Consider a scenario where a city experiences a sudden influx of rainfall. With AI-driven GIS, city planners can instantly analyze flood-prone areas, predict potential hazards, and deploy emergency services where they are needed most. This level of responsiveness can save lives and minimize damage.
To illustrate the impact of real-time data integration in GIS, here’s a quick comparison of traditional methods versus AI-enhanced approaches:
Aspect | Traditional GIS | AI-Enhanced GIS |
---|---|---|
Data Processing Speed | Slower, often requiring manual updates | Instantaneous, with automated data feeds |
Decision Making | Reactive, based on historical data | Proactive, based on real-time insights |
User Accessibility | Complex, requiring specialized knowledge | User-friendly, accessible to non-experts via natural language queries |
Moreover, the integration of real-time data is not limited to urban planning or disaster management. It extends to various sectors, including environmental monitoring, public health, and transportation. For instance, public health officials can track disease outbreaks by analyzing real-time data from hospitals and clinics, allowing for quicker interventions. Similarly, transportation agencies can monitor traffic patterns and adjust public transit schedules accordingly, ensuring smoother commutes for everyone.
In conclusion, the integration of real-time data in GIS, powered by AI, is revolutionizing how we interact with our environment. It’s about making informed decisions faster and with greater accuracy. As we continue to harness the power of AI, the possibilities for real-time data integration in GIS are boundless, paving the way for smarter, safer, and more efficient communities.
- What is real-time data integration in GIS? Real-time data integration in GIS refers to the process of aggregating and analyzing data from various sources as it becomes available, allowing for immediate insights and decision-making.
- How does AI enhance real-time data integration? AI enhances real-time data integration by automating data processing, improving accuracy in analysis, and enabling proactive responses to changing conditions.
- What are some applications of real-time data integration in GIS? Applications include urban planning, disaster management, environmental monitoring, and public health tracking.
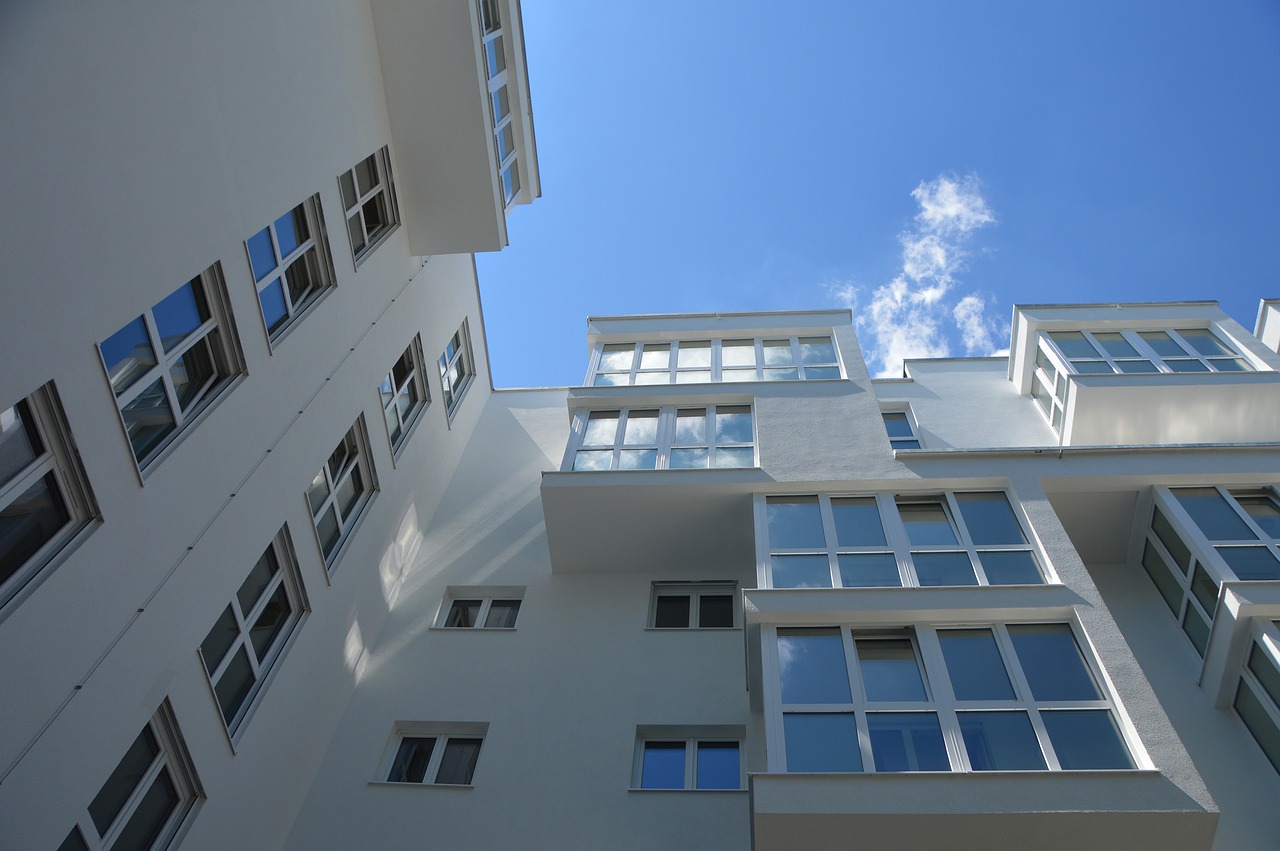
[Smart City Applications]
In the rapidly evolving landscape of urban development, smart city applications powered by AI-enhanced GIS are becoming the backbone of modern urban management. Imagine a city where traffic flows seamlessly, public services are optimized, and resources are allocated efficiently—all thanks to the integration of cutting-edge technology. This is not just a futuristic dream; it's happening right now. AI-driven GIS technologies are transforming how cities operate, making them more livable, sustainable, and responsive to the needs of their residents.
One of the most significant benefits of AI in smart city applications is its ability to analyze vast amounts of data in real-time. For instance, consider how traffic management systems utilize AI algorithms to predict congestion patterns. By analyzing data from various sources, including traffic cameras, sensors, and social media, these systems can adjust traffic signals dynamically, reducing wait times and improving overall traffic flow. This not only enhances the commuting experience but also contributes to lower emissions and reduced fuel consumption.
Moreover, AI-enhanced GIS plays a crucial role in public safety and emergency response. Imagine a scenario where a natural disaster strikes a city. With real-time data integration, emergency responders can access up-to-date information about affected areas, including population density, infrastructure status, and available resources. This capability enables them to make informed decisions quickly, ensuring that help reaches those in need as efficiently as possible. The integration of AI also aids in risk assessment, helping city planners to identify vulnerable areas and develop strategies to mitigate potential disasters.
Another fascinating application lies in urban planning and resource management. AI-driven GIS can analyze historical data and current trends to forecast future urban growth. City planners can visualize potential developments and assess their impact on existing infrastructure, ensuring that new projects align with sustainability goals. For example, by examining data on land use, population growth, and environmental factors, planners can make informed decisions about where to build new housing, parks, or transportation networks.
To illustrate the impact of AI in smart cities, consider the following table that highlights key applications and their benefits:
Application | Benefits |
---|---|
Traffic Management | Reduced congestion, improved travel times, lower emissions |
Public Safety | Enhanced emergency response, better resource allocation |
Urban Planning | Informed decision-making, sustainable development |
Environmental Monitoring | Real-time pollution tracking, effective resource management |
As cities continue to grow and face complex challenges, the role of AI in GIS will only become more critical. By harnessing the power of AI, urban planners and city officials can create environments that not only meet the demands of today but also anticipate the needs of future generations. The integration of smart city applications is paving the way for a more efficient, sustainable, and resilient urban future.
- What are smart cities? Smart cities use technology and data to improve urban living and enhance public services.
- How does AI improve traffic management? AI analyzes real-time data to optimize traffic signals and reduce congestion.
- What role does GIS play in smart city applications? GIS provides spatial analysis and visualization tools to support urban planning and resource management.
- Can AI help in disaster management? Yes, AI can provide real-time data for emergency responders to enhance decision-making during disasters.
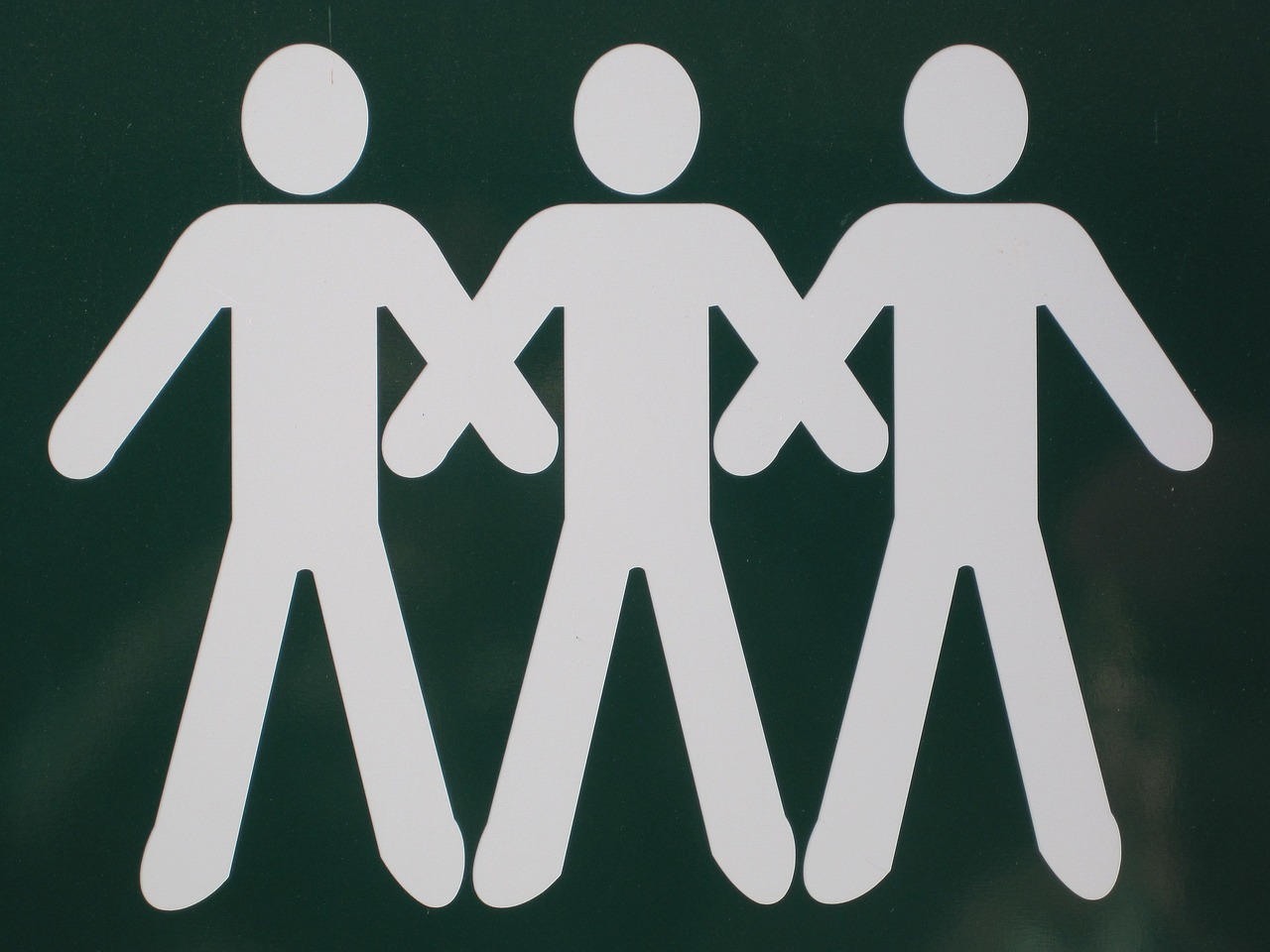
[Disaster Management and Response]
In an era where natural disasters seem to be on the rise, the integration of artificial intelligence (AI) into Geographic Information Systems (GIS) is proving to be a game-changer for disaster management and response. Imagine being able to predict a storm's path or assess the damage from a wildfire in real-time; this is no longer a distant fantasy but a reality made possible through AI-enhanced GIS technologies. By harnessing the power of AI, emergency responders can access timely and accurate information, which is crucial for effective disaster management.
One of the most significant advantages of using AI in GIS for disaster response is its ability to improve risk assessment. Through advanced algorithms and data analytics, AI can analyze historical data, weather patterns, and geographical features to identify areas that are most vulnerable to disasters. For instance, AI can evaluate flood risks by examining rainfall data, river flow rates, and topographical information. This predictive capability allows authorities to allocate resources more efficiently and prepare communities ahead of time.
Furthermore, AI-driven GIS systems can facilitate real-time monitoring during disasters. By integrating data from various sources, such as satellite imagery, social media feeds, and IoT devices, these systems can provide a comprehensive view of the situation on the ground. For example, during a hurricane, AI can process live data to track the storm's intensity and trajectory, enabling emergency services to issue timely evacuation orders. This rapid response capability can save lives and minimize damage.
Moreover, after a disaster strikes, AI-enhanced GIS plays a crucial role in recovery efforts. By utilizing change detection algorithms, emergency teams can assess the extent of the damage quickly. These algorithms can compare pre- and post-disaster images to highlight affected areas, allowing for targeted aid distribution. For instance, if a wildfire has ravaged a forest area, GIS can help identify which communities need immediate assistance, ensuring that resources are directed where they are most needed.
To illustrate the impact of AI in disaster management, consider the following table that summarizes key applications:
Application | Description | Benefits |
---|---|---|
Risk Assessment | Analyzing data to identify vulnerable areas | Improved resource allocation and preparedness |
Real-Time Monitoring | Integrating live data for situational awareness | Timely decision-making and evacuation orders |
Damage Assessment | Using change detection to evaluate impact | Efficient recovery and aid distribution |
It's also worth noting that the collaboration between various stakeholders, including government agencies, NGOs, and technology providers, is essential for maximizing the potential of AI in disaster response. By sharing data and resources, these entities can create a more robust framework for disaster management. The future looks promising, as advancements in AI continue to evolve, paving the way for even more innovative solutions in GIS.
As we move forward, the question arises: how can we further enhance the integration of AI in GIS for disaster management? Continuous research and development, along with community engagement, will be vital in shaping the next generation of disaster response technologies. The goal is to create systems that not only respond to disasters but also empower communities to better prepare for them.
- How does AI improve disaster response? AI enhances disaster response by providing real-time data analysis, improving risk assessment, and enabling efficient resource allocation.
- What role does GIS play in disaster management? GIS provides a spatial context for understanding and managing disasters, allowing for better planning and response strategies.
- Can AI predict natural disasters? While AI cannot predict disasters with absolute certainty, it can analyze patterns and trends to provide forecasts that improve preparedness.
- What technologies are integrated with AI in GIS? AI in GIS often integrates with satellite imagery, IoT devices, and social media data to enhance situational awareness.
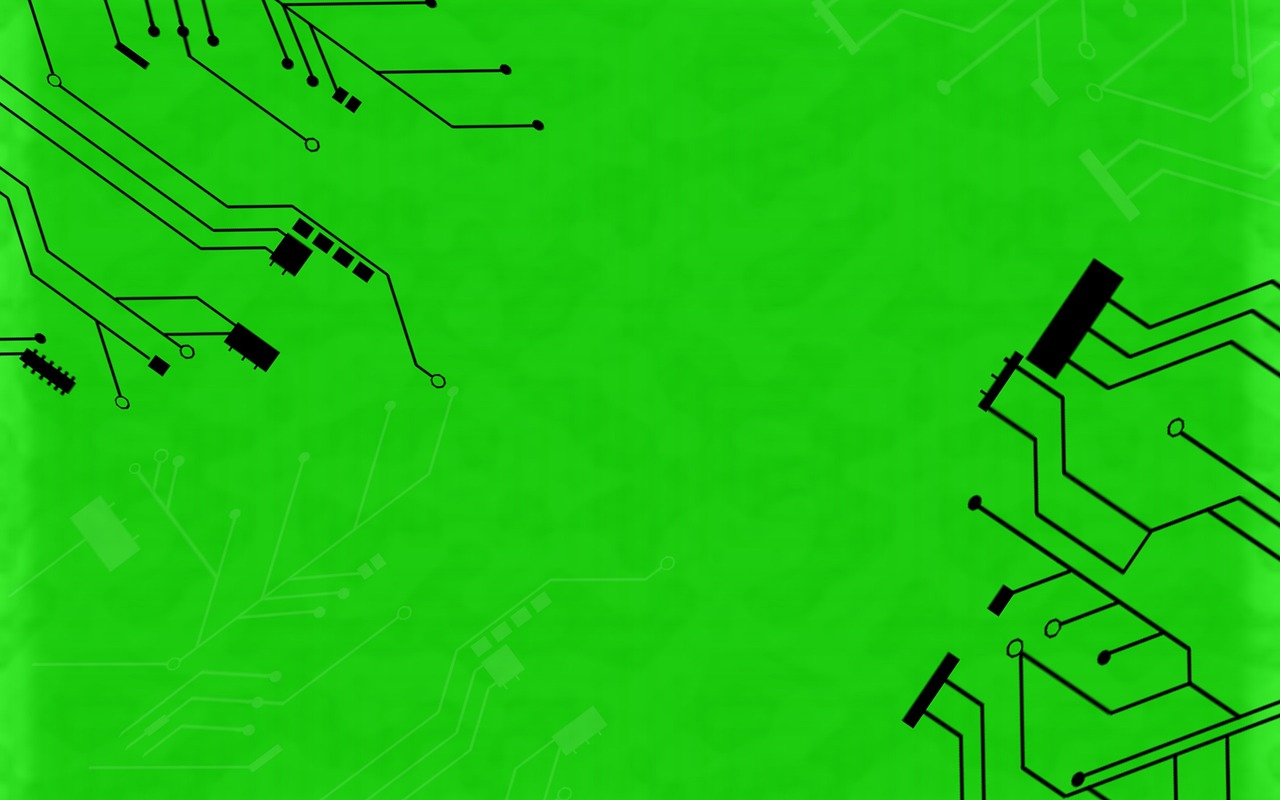
[Future Trends in AI and GIS]
The future of artificial intelligence (AI) in Geographic Information Systems (GIS) is not just bright; it's downright dazzling! As we look ahead, several exciting trends are emerging that promise to reshape how we interact with geographic data. One of the most significant advancements is the push toward greater automation. Imagine a world where GIS systems can automatically analyze vast amounts of spatial data without human intervention. This level of automation could drastically reduce the time it takes to gather insights, allowing professionals to focus more on strategy and less on data crunching.
In addition to automation, we can expect to see an increase in accuracy in spatial analysis. With the continuous improvement of AI algorithms, the precision of data interpretation will enhance significantly. This means that decisions made based on GIS data will be more reliable, providing critical support for urban planning, environmental monitoring, and resource management. For example, AI could help predict urban sprawl with higher accuracy, allowing city planners to make informed decisions that can lead to sustainable development.
Another trend to watch is the development of intuitive user interfaces. As GIS technology evolves, so does the need for accessibility. Future interfaces will likely leverage natural language processing (NLP) and other user-friendly features, enabling even those without technical expertise to navigate complex spatial data effortlessly. Imagine asking your GIS software a question in plain English and receiving a comprehensive analysis in seconds. This democratization of geographic information access will empower more people to utilize GIS in their work, from local governments to community organizations.
Furthermore, the integration of augmented reality (AR) and virtual reality (VR) with GIS is on the horizon. These technologies will allow users to visualize spatial data in immersive environments, enhancing understanding and engagement. For instance, city planners could use VR to walk through a virtual model of a proposed development, assessing its impact on the surrounding area before any physical construction begins. This innovative approach could lead to better decision-making and community involvement in urban planning processes.
As we embrace these trends, it's essential to consider the ethical implications of AI in GIS. With great power comes great responsibility, and as AI becomes more integrated into our decision-making processes, ensuring fairness and transparency in data usage will be crucial. Stakeholders must collaborate to establish guidelines that protect user privacy and promote equitable access to information.
In summary, the future of AI in GIS is poised for remarkable advancements that will enhance automation, accuracy, accessibility, and visualization. As these trends unfold, they will not only transform how we analyze geographic data but also how we engage with our environments. The possibilities are endless, and the journey ahead is sure to be as thrilling as it is transformative.
- What role does AI play in GIS?
AI enhances GIS by improving data processing, enabling predictive analytics, and automating complex analysis tasks. - How can AI improve decision-making in urban planning?
AI can analyze vast datasets to identify trends and provide insights that support strategic planning and resource allocation. - What are the benefits of using natural language processing in GIS?
NLP makes GIS more user-friendly, allowing non-experts to query spatial data using everyday language. - Will AR and VR change the way we use GIS?
Yes, AR and VR will provide immersive experiences that enhance understanding and engagement with geographic data.
Frequently Asked Questions
- What is the role of AI in GIS technology?
AI plays a transformative role in GIS technology by enhancing data processing capabilities, improving spatial analysis accuracy, and streamlining decision-making processes across various sectors like urban planning and environmental management.
- How does machine learning improve GIS applications?
Machine learning enhances GIS applications by automating pattern recognition, enabling anomaly detection, and classifying spatial data, which leads to better analysis and visualization of complex datasets.
- What are some examples of deep learning in GIS?
Deep learning is particularly effective in processing satellite imagery and aerial photographs for tasks such as land cover classification, object detection, and monitoring changes over time, providing more accurate and detailed insights.
- Can you explain predictive analytics in the context of GIS?
Predictive analytics in GIS uses AI to forecast future trends in areas like urban development and resource management, offering valuable insights that assist in strategic planning and policy-making.
- How does natural language processing (NLP) enhance GIS?
NLP makes GIS more user-friendly by allowing users to query spatial data using everyday language, thus making geographic information accessible to non-experts and enhancing user engagement.
- What is the significance of real-time data integration in GIS?
Real-time data integration allows GIS applications to dynamically respond to changing conditions by incorporating data from various sources, such as IoT devices and social media, which improves situational awareness and decision-making.
- How is AI used in smart city applications?
AI-enhanced GIS is crucial for smart city initiatives, optimizing urban infrastructure, traffic management, and public services through real-time data analysis, ultimately leading to more efficient city operations.
- What impact does AI have on disaster management?
AI integration in GIS significantly improves disaster management by providing timely and accurate information for emergency responders, enhancing risk assessment, and aiding recovery strategies after natural disasters.
- What are the future trends for AI in GIS?
The future of AI in GIS includes exciting advancements in automation, increased accuracy in spatial analysis, and the creation of more intuitive user interfaces, all of which will further democratize access to geographic information.