Considering the Ethics of AI in Cybersecurity
In today’s digital landscape, the integration of artificial intelligence (AI) in cybersecurity is not just a trend; it's a necessity. As cyber threats become more sophisticated, the need for advanced security measures has never been more critical. However, with great power comes great responsibility. The use of AI in cybersecurity brings forth a myriad of ethical considerations that must be addressed to ensure that the benefits do not come at the cost of individual rights and societal norms. This article delves into the complex interplay between AI technologies and ethical practices, shedding light on how we can navigate this evolving landscape.
AI significantly enhances cybersecurity measures by automating threat detection and response. Imagine having a vigilant guardian that never sleeps, constantly monitoring your digital assets. AI technologies utilize machine learning algorithms to analyze vast amounts of data, identifying patterns that may indicate a potential threat. This capability not only improves the efficiency of security protocols but also boosts their effectiveness. Organizations can respond to threats in real-time, drastically reducing the window of vulnerability. However, while the benefits are substantial, they also raise questions about the implications of relying heavily on automated systems.
The integration of AI raises several ethical questions that demand our attention. For instance, how do we balance the need for security with the right to privacy? What happens when AI systems make biased decisions? These concerns are not trivial; they have profound implications for how cybersecurity practices are shaped. The following subsections will delve deeper into these pressing issues, starting with the critical aspect of privacy and data protection.
AI systems often require access to vast amounts of data, which can lead to potential privacy violations. The challenge lies in finding the right balance between effective security measures and individual privacy rights. Organizations must tread carefully, ensuring that their data collection practices do not infringe upon the personal liberties of individuals. For instance, while monitoring network traffic for suspicious activity may be necessary, it should not come at the cost of monitoring personal communications without consent.
The methods used to collect data for AI systems can often be intrusive. Companies must adopt ethical data collection practices that respect user privacy while ensuring cybersecurity effectiveness. This could involve implementing anonymization techniques and minimizing data collection to what is strictly necessary. By doing so, organizations can enhance their security postures without compromising the privacy of their users.
Ensuring informed consent and transparency in AI operations is crucial. Users should be fully aware of how their data is being used in cybersecurity applications. Clear communication about data usage not only fosters trust but also empowers individuals to make informed decisions about their personal information. Organizations that prioritize transparency are more likely to build long-lasting relationships with their customers, which can ultimately lead to a stronger security framework.
Another significant concern is the potential for bias in AI algorithms. AI systems can inadvertently perpetuate existing biases, leading to unfair treatment of certain individuals or groups. This is particularly concerning in cybersecurity, where biased algorithms could result in disproportionate scrutiny of specific demographics. Addressing these biases requires a concerted effort to ensure fairness in AI development, including diverse datasets and inclusive testing processes.
Determining responsibility for AI-driven decisions is a complex issue that poses significant challenges. When an AI system makes a mistake, such as misidentifying a threat, who is held accountable? This section discusses the intricate web of accountability in cybersecurity incidents involving AI technologies. The question of liability for AI failures is crucial, as it shapes the legal and ethical responsibilities of developers, organizations, and users alike.
When AI systems fail, questions arise about who is liable. Is it the developers who created the algorithm, the organizations that deployed it, or the users who relied on it? This ambiguity can lead to significant legal and ethical dilemmas, particularly in the event of cybersecurity breaches. It’s essential to establish clear guidelines that define accountability and ensure that all parties understand their responsibilities in the AI ecosystem.
The need for regulations governing AI in cybersecurity is becoming increasingly apparent. As technology evolves, so too must our regulatory approaches. Potential frameworks should aim to ensure ethical practices and accountability in the use of AI technologies. These regulations could include guidelines for data usage, bias mitigation strategies, and accountability measures that protect both organizations and individuals.
- What are the main ethical concerns regarding AI in cybersecurity?
Privacy violations, algorithmic bias, and accountability are primary concerns. - How can organizations ensure ethical AI use?
By adopting transparent data practices, obtaining informed consent, and regularly auditing algorithms for bias. - Who is responsible for AI failures in cybersecurity?
Liability can be complex and may involve developers, organizations, and users.
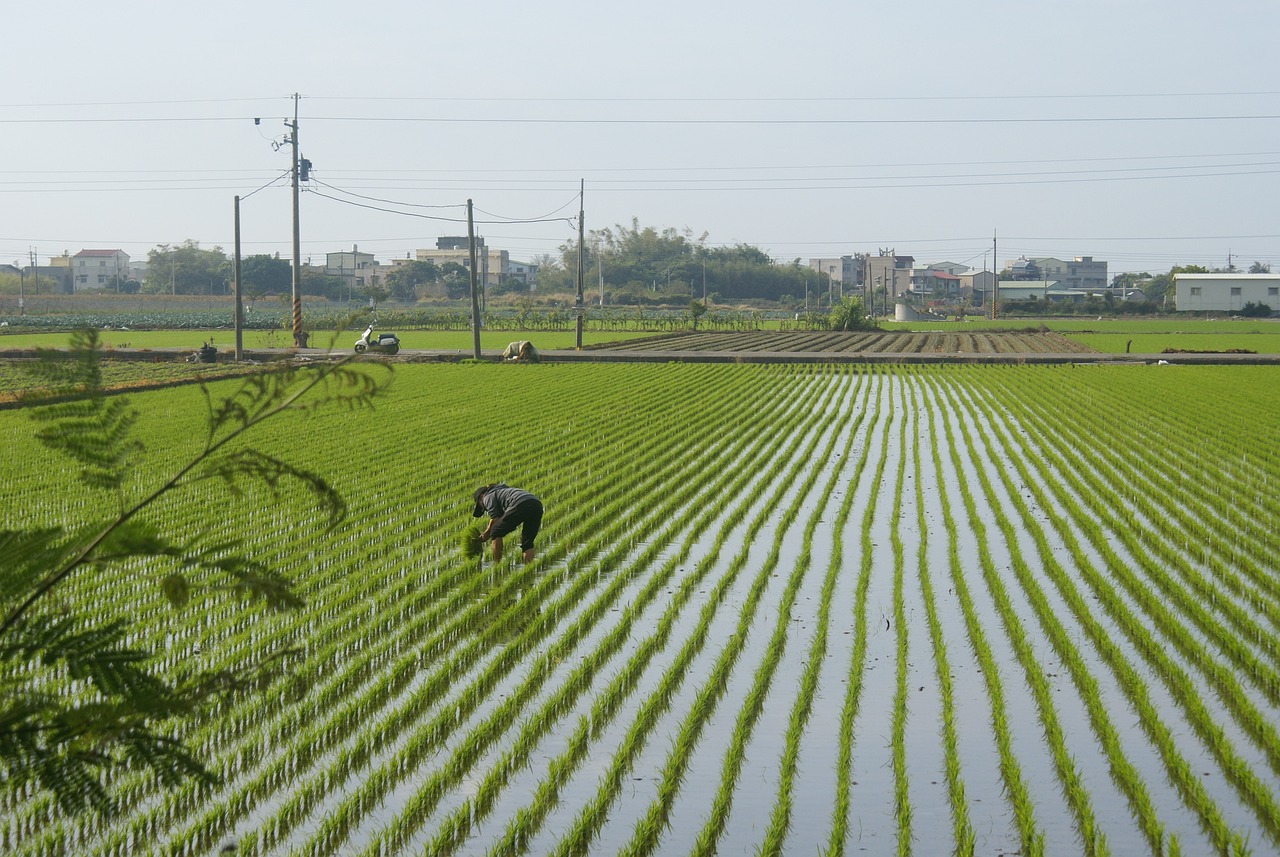
The Role of AI in Cybersecurity
In today's digital landscape, where cyber threats are becoming increasingly sophisticated, the role of artificial intelligence (AI) in cybersecurity cannot be overstated. AI technologies are revolutionizing how organizations protect their sensitive information and systems, making them more resilient against attacks. Imagine having a vigilant guard that never sleeps—this is essentially what AI brings to the table. It automates threat detection and response, allowing security teams to focus on more complex issues while AI handles the mundane yet critical tasks.
One of the most significant advantages of AI in cybersecurity is its ability to analyze vast amounts of data in real-time. Traditional security systems often struggle to keep up with the sheer volume of information generated daily. However, AI algorithms can sift through this data, identifying patterns and anomalies that may indicate a potential threat. For instance, AI can detect unusual login attempts or unexpected data transfers, flagging them for further investigation. This proactive approach not only enhances security but also minimizes the time it takes to respond to incidents, potentially preventing breaches before they occur.
Furthermore, AI can adapt and learn from new threats. As cybercriminals develop more advanced techniques, AI systems can update their algorithms based on previous attacks, thereby improving their defensive capabilities. This machine learning aspect is crucial because it ensures that security measures evolve alongside emerging threats. In a world where cyberattacks are constantly changing, having a system that can learn and adapt is like having a security expert who is always up-to-date with the latest tactics.
Moreover, AI-driven tools can assist in automating repetitive tasks such as log analysis and incident response. This not only speeds up the process but also reduces human error, which is often a significant factor in security breaches. By automating these processes, organizations can allocate their resources more effectively, allowing cybersecurity professionals to concentrate on strategic planning and risk management rather than getting bogged down by routine tasks.
To illustrate the impact of AI in cybersecurity, consider the following table that highlights some key benefits:
Benefit | Description |
---|---|
Real-Time Threat Detection | AI systems can monitor network activity continuously, identifying threats as they arise. |
Adaptive Learning | AI can learn from past incidents, improving its response to new threats. |
Automation of Tasks | AI can automate repetitive security tasks, reducing the workload on human analysts. |
Enhanced Decision-Making | AI can provide insights and recommendations based on data analysis, aiding in strategic decisions. |
In summary, the integration of AI in cybersecurity is not just a trend; it's a necessity in an era where cyber threats are more prevalent than ever. By leveraging AI technologies, organizations can enhance their security posture, respond to incidents more efficiently, and ultimately protect their critical assets from malicious attacks. As we delve deeper into the ethical implications of AI in this field, it’s essential to recognize the profound impact it has on shaping the future of cybersecurity.
Q: How does AI improve threat detection?
A: AI improves threat detection by analyzing large volumes of data in real-time to identify patterns and anomalies that may indicate a security threat.
Q: Can AI adapt to new cyber threats?
A: Yes, AI systems can learn from previous attacks and adapt their algorithms to improve their defenses against emerging threats.
Q: What are the benefits of automating cybersecurity tasks?
A: Automating cybersecurity tasks reduces human error, speeds up response times, and allows security professionals to focus on more strategic issues.
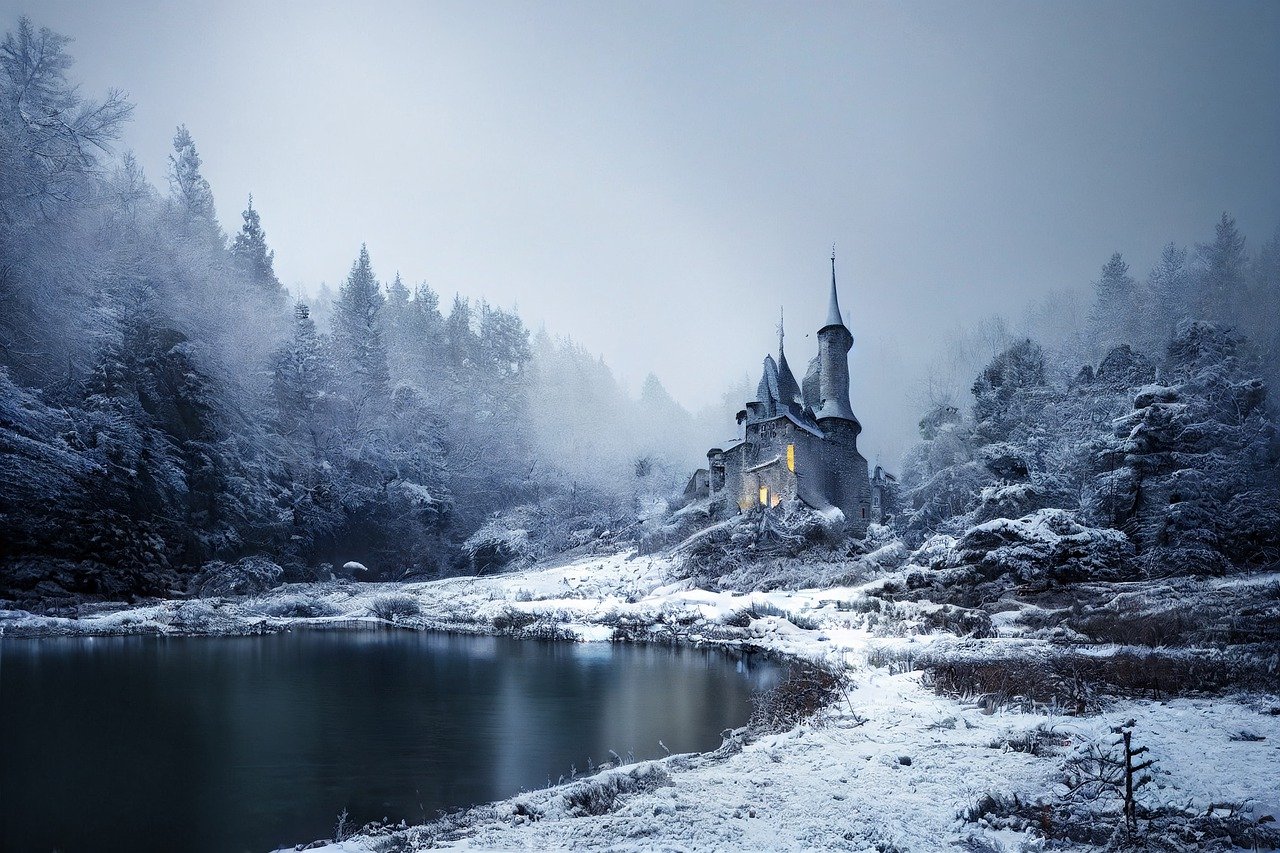
Ethical Concerns of AI Usage
The integration of artificial intelligence into cybersecurity isn't just about enhancing efficiency; it brings with it a whirlwind of ethical dilemmas that we can’t afford to ignore. As we embrace these advanced technologies, we must also grapple with the implications of their use. From privacy violations to algorithmic bias, the ethical concerns surrounding AI in cybersecurity are as complex as they are critical. So, what are the key issues we need to consider?
First and foremost, we have to talk about privacy and data protection. AI systems often require access to vast amounts of data to function effectively. This data can include sensitive personal information, which raises the question: at what cost are we willing to protect our systems? The balance between effective security measures and individual privacy rights is a delicate one. Organizations must ensure that while they are safeguarding their digital assets, they are not infringing on the privacy of individuals. This is a tightrope walk that requires meticulous attention to ethical data practices.
When we consider data collection practices, it becomes evident that the methods used can often be intrusive. For instance, companies may resort to scraping data from various online platforms, leading to potential violations of user privacy. Ethical data collection should prioritize user consent and respect for individual rights. It’s essential to implement practices that not only enhance cybersecurity but also foster trust among users. Organizations should focus on transparent data collection methods that clearly communicate what data is being collected and how it will be used.
Moreover, the concepts of consent and transparency cannot be overstated. Users must be informed about how their data is being utilized in AI-driven cybersecurity applications. This means that organizations must provide clear communication and ensure that consent is not just a checkbox on a form but a genuine agreement. The more transparent organizations are about their data usage, the more likely they are to gain the trust of their users. This trust is crucial, especially in a landscape where data breaches are becoming alarmingly common.
Another pressing ethical concern is the potential for bias in AI algorithms. These algorithms can inadvertently perpetuate existing biases, leading to unfair treatment of individuals or groups. Imagine a security system that flags certain demographics as more suspicious based solely on historical data; this could lead to discriminatory practices that harm innocent individuals. The ethical implications of biased AI in cybersecurity contexts are profound and require rigorous scrutiny. It’s vital for developers to actively work towards creating algorithms that are fair and equitable, ensuring that AI serves as a tool for justice rather than a mechanism of discrimination.
In conclusion, while the benefits of AI in cybersecurity are significant, the ethical concerns associated with its usage are equally important. Organizations must navigate these waters carefully, balancing the need for robust security with the imperative to uphold ethical standards. As we move forward, it’s crucial that we prioritize ethics in our discussions about AI in cybersecurity, ensuring that we are not only protecting our systems but also respecting the rights and dignity of individuals.
- What are the main ethical concerns regarding AI in cybersecurity?
The main concerns include privacy violations, data collection practices, consent and transparency, and bias in algorithms. - How can organizations ensure ethical data collection?
Organizations can ensure ethical data collection by prioritizing user consent, being transparent about data usage, and implementing practices that respect individual privacy rights. - What role does bias play in AI algorithms?
Bias in AI algorithms can lead to unfair treatment of certain groups, perpetuating discrimination and compromising the integrity of cybersecurity measures. - Why is transparency important in AI usage?
Transparency builds trust with users, ensuring they are informed about how their data is used and fostering a sense of security regarding their personal information.
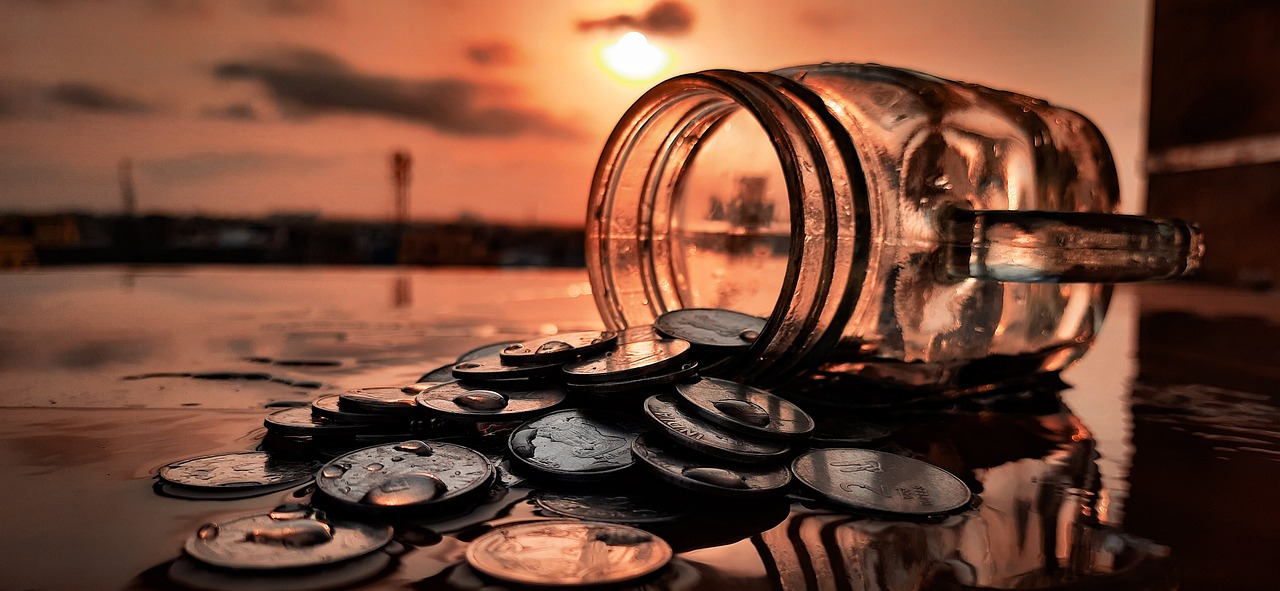
Privacy and Data Protection
As we dive deeper into the realm of artificial intelligence in cybersecurity, one of the most pressing concerns that surfaces is . With AI systems often requiring access to vast amounts of data to function effectively, the delicate balance between robust security and individual privacy rights becomes increasingly challenging. Think of it this way: while a security guard is essential for keeping a building safe, if that guard starts rummaging through personal belongings without consent, trust is shattered. Similarly, AI must tread carefully to avoid infringing on personal privacy while safeguarding against cyber threats.
One critical aspect of this discussion is the methods used to collect data for AI systems. Many organizations employ techniques that can be perceived as intrusive, such as monitoring user behavior, analyzing communication patterns, and aggregating data from various sources. While these practices can enhance the effectiveness of cybersecurity measures, they may also lead to significant privacy violations. For instance, if a company collects data without informing users, it can create a sense of unease and distrust among its clientele. Thus, ethical data collection practices must be established to respect user privacy while ensuring cybersecurity effectiveness.
To navigate this complex landscape, organizations can adopt a few principles:
- Minimization: Collect only the data necessary for specific security functions.
- Transparency: Clearly communicate to users what data is being collected and how it will be used.
- Consent: Ensure that users are informed and give explicit permission before data collection begins.
Moreover, the importance of informed consent and transparency cannot be overstated. Users should be made aware of how their data is utilized in cybersecurity applications, fostering an environment of trust and cooperation. This transparency not only enhances the ethical standing of an organization but also strengthens its overall cybersecurity posture. When users feel secure in the knowledge that their data is handled responsibly, they are more likely to engage with and support the cybersecurity measures in place.
In conclusion, as we embrace the benefits of AI in cybersecurity, we must not lose sight of the ethical implications regarding privacy and data protection. Striking the right balance is crucial, and organizations must prioritize ethical data collection practices, informed consent, and transparency to build a secure and trustworthy environment for all users.
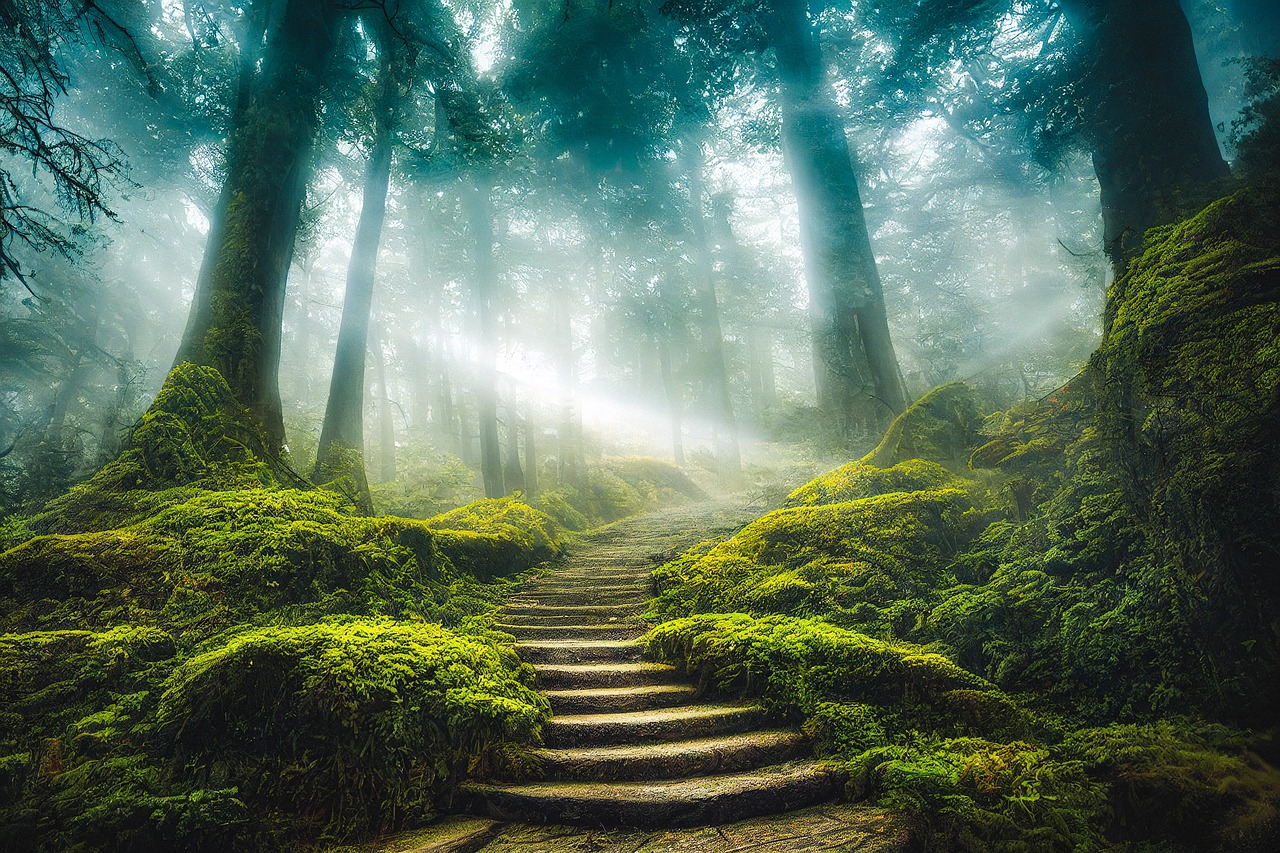
Data Collection Practices
When it comes to the integration of artificial intelligence in cybersecurity, one of the most pressing issues is how data is collected. The methods used for data collection can often be intrusive and raise significant ethical concerns. Imagine a scenario where your personal data is harvested without your knowledge. It’s a bit like someone rummaging through your belongings while you’re not looking—definitely uncomfortable, right? This discomfort stems from the fact that AI systems often require access to vast amounts of data to function effectively. However, this necessity must be balanced with the need to respect individual privacy.
To ensure that data collection practices are ethical and transparent, organizations should adopt a set of guiding principles. These principles can help navigate the murky waters of data ethics while still leveraging the power of AI. Here are a few key considerations:
- Minimization: Only collect data that is absolutely necessary for the intended purpose. This reduces the risk of privacy violations.
- Anonymization: Whenever possible, data should be anonymized to protect the identity of individuals. This way, even if data is compromised, personal information remains safe.
- Purpose Limitation: Clearly define and communicate the purpose for which data is being collected. Users should know how their data will be used and for how long it will be retained.
Moreover, organizations must consider the implications of their data collection practices on user trust. If users feel that their data is being collected and used without their consent, it can lead to a significant erosion of trust. This is particularly critical in cybersecurity, where trust is paramount. A breach of trust can have far-reaching consequences, not just for the individual but also for the organization’s reputation.
Additionally, ethical data collection practices should include mechanisms for user consent. This means that organizations need to ensure that users are fully informed about what data is being collected, how it will be used, and who it will be shared with. Transparency is key here. Users should feel empowered to make informed decisions about their data. Providing clear and accessible privacy policies can go a long way in achieving this goal.
In conclusion, while the use of AI in cybersecurity can greatly enhance security measures, it is crucial to approach data collection with a strong ethical framework. By prioritizing user privacy and maintaining transparency in data practices, organizations can create a safer environment for users while still reaping the benefits of advanced AI technologies.
- What is ethical data collection? Ethical data collection involves gathering information in a manner that respects individuals' privacy rights and obtains informed consent.
- Why is user consent important? User consent is important because it empowers individuals to control how their personal data is used and shared.
- How can organizations ensure transparency in data practices? Organizations can ensure transparency by providing clear privacy policies and communicating openly about data usage.
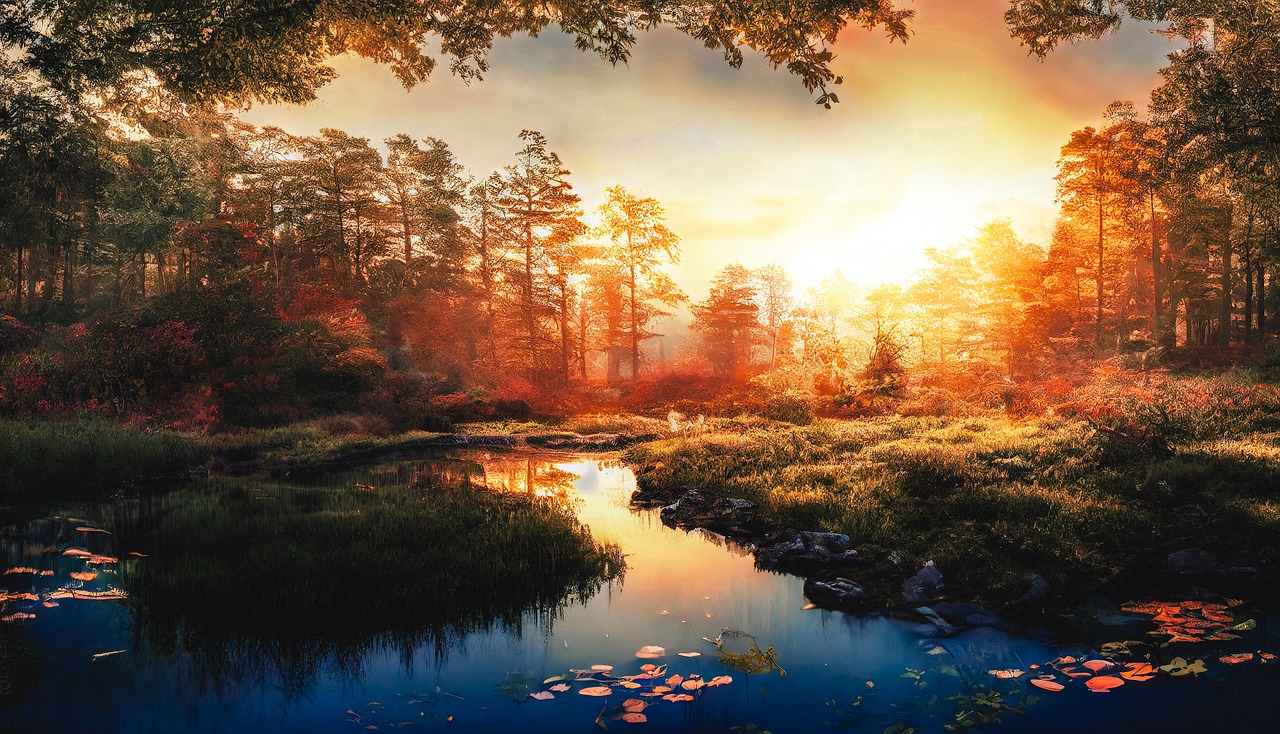
Consent and Transparency
In the realm of cybersecurity, where artificial intelligence (AI) plays an increasingly pivotal role, the concepts of consent and transparency are not just buzzwords; they are essential pillars that uphold ethical standards. Imagine walking into a store where the owner knows everything about you without asking—your preferences, your past purchases, even your online behavior. It’s unsettling, right? This analogy reflects the discomfort many feel regarding AI systems that collect and analyze vast amounts of personal data without explicit consent.
When organizations deploy AI technologies for cybersecurity, they often require access to sensitive information to identify potential threats effectively. However, this raises a fundamental question: Are users fully aware of what data is being collected and how it is being utilized? Transparency is crucial here. Users should not only be informed about data collection practices but also understand the purpose behind it. This fosters trust and ensures that individuals feel secure in the knowledge that their information is being handled responsibly.
Moreover, consent should be informed, meaning that individuals need to be provided with clear details about the data collection process. This includes:
- The type of data being collected
- How the data will be used
- The duration for which the data will be retained
- Who will have access to this data
Without this level of clarity, organizations risk not only breaching ethical boundaries but also facing potential legal repercussions. The General Data Protection Regulation (GDPR) in Europe, for instance, emphasizes the importance of obtaining explicit consent from users before processing their personal data. This regulatory framework serves as a reminder that organizations must prioritize transparency in their cybersecurity practices.
Ultimately, achieving a balance between robust cybersecurity measures and respecting individual privacy rights is vital. Organizations must strive to create an environment where users feel empowered to give or withhold consent based on a comprehensive understanding of how their data will be used. By prioritizing transparency and consent, companies can build a solid foundation of trust, which is essential in today’s digital landscape.
- What is the importance of consent in AI-driven cybersecurity?
Consent ensures that users are aware of and agree to the data collection practices, fostering trust and ethical responsibility. - How can organizations ensure transparency?
By clearly communicating data collection practices and purposes, organizations can help users understand how their information is being used. - What are the consequences of not obtaining consent?
Failure to obtain consent can lead to legal issues, loss of trust, and potential damage to an organization's reputation.
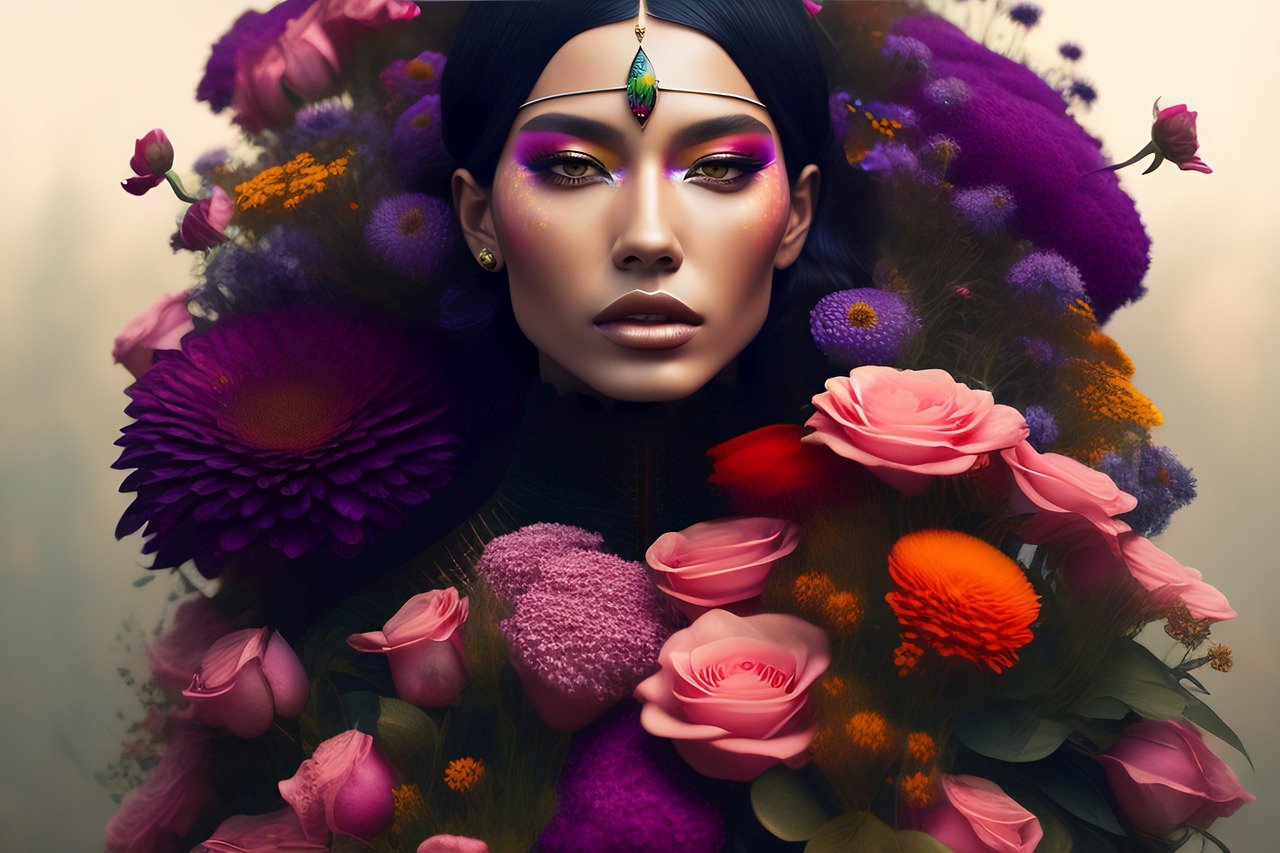
Bias and Fairness in AI Algorithms
As we dive deeper into the world of artificial intelligence, one of the most pressing issues that emerges is the bias inherent in AI algorithms. This isn't just a technical glitch; it's a significant ethical concern that can have real-world implications. Imagine a security system that, due to biased algorithms, mistakenly flags a particular demographic as a higher risk for cyber threats. This can lead to unfair treatment and discrimination, raising serious questions about the fairness of AI in cybersecurity.
AI systems learn from data, and if that data is biased, the results will be too. For instance, if an AI model is trained primarily on data from a specific demographic, it may not perform well when applied to other groups. This can create a scenario where certain individuals or communities are unfairly targeted or overlooked, ultimately undermining the trust that users place in these technologies. To illustrate this point, consider the following examples:
Scenario | Potential Bias | Impact |
---|---|---|
Facial Recognition in Security Systems | Underrepresentation of certain ethnic groups in training data | Higher false positive rates for those groups |
Spam Detection Algorithms | Training on biased email datasets | Over-blocking legitimate emails from certain users |
Fraud Detection Systems | Historical data reflecting societal biases | Disproportionate scrutiny of specific demographics |
These examples highlight the critical need for fairness in AI algorithms. Organizations must be proactive in addressing bias by ensuring that their data sets are diverse and representative. This involves not only gathering data from a variety of sources but also regularly auditing algorithms to identify and rectify any biases that may arise. The goal is to create AI systems that are not only effective but also equitable.
Moreover, transparency plays a vital role in mitigating bias. Users should be informed about how AI systems make decisions and what data they rely on. By fostering an environment of open communication, organizations can help build trust and accountability in their AI implementations. As we navigate this complex landscape, it's essential to ask ourselves: How can we ensure that AI serves everyone fairly? The answer lies in a commitment to continuous improvement and ethical practices.
In conclusion, addressing bias and fairness in AI algorithms is not just a technical challenge; it is a moral imperative. As we continue to integrate AI into cybersecurity, we must prioritize fairness to protect all users equitably. Only then can we truly harness the power of AI to create a safer digital environment for everyone.
- What is AI bias? AI bias refers to the tendency of AI algorithms to produce unfair outcomes due to biased training data or flawed design.
- How can organizations prevent AI bias? Organizations can prevent AI bias by using diverse data sets, conducting regular audits, and ensuring transparency in their AI systems.
- Why is fairness important in AI? Fairness in AI is crucial to ensure that all individuals are treated equitably, preventing discrimination and fostering trust in AI technologies.
- What are the consequences of biased AI? Biased AI can lead to unfair treatment, loss of trust, and potential legal consequences for organizations that fail to address these issues.
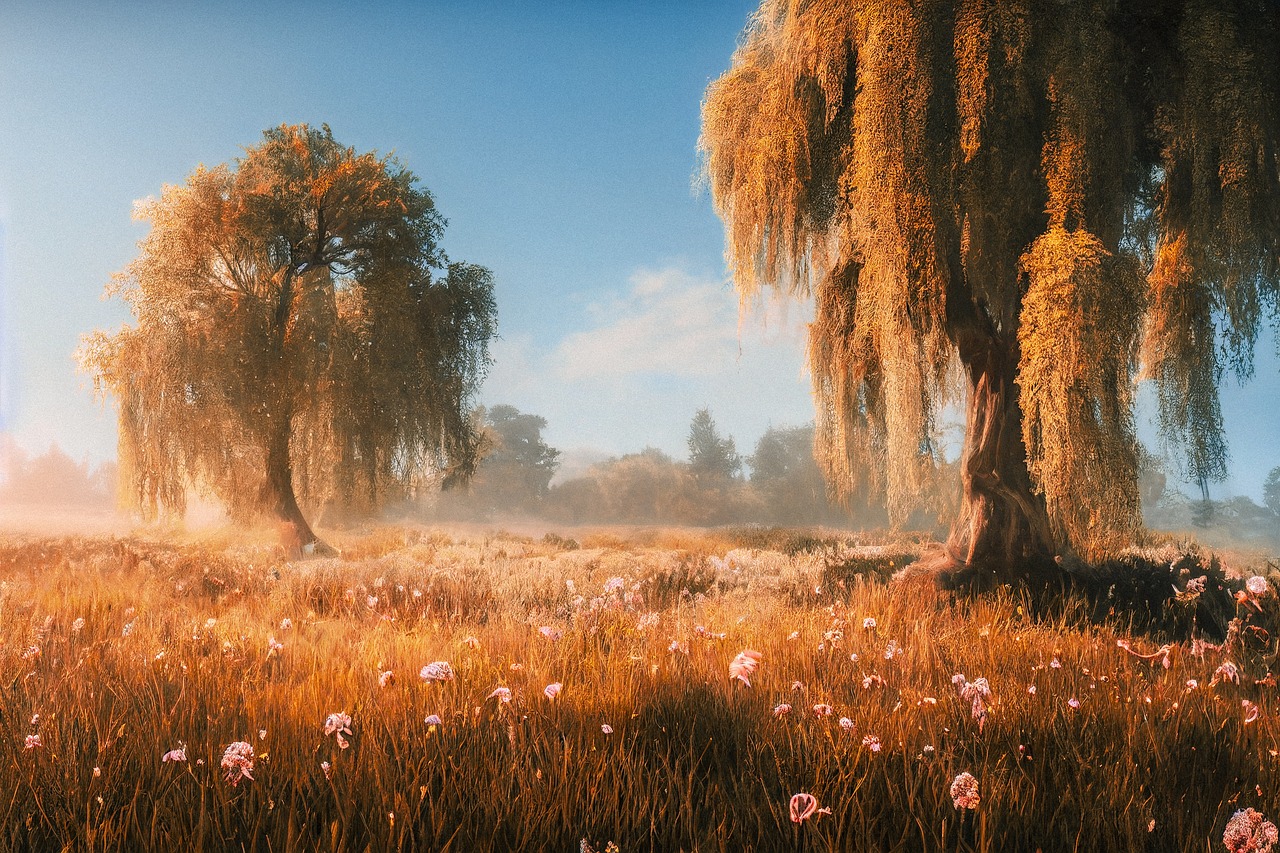
Accountability in AI Decision-Making
In today's digital landscape, the integration of artificial intelligence (AI) into cybersecurity practices has transformed how organizations protect their sensitive data and systems. However, with great power comes great responsibility. The question of accountability in AI decision-making has become a pressing issue, as the complexity of these technologies often obscures the lines of responsibility. When an AI system makes a decision that leads to a cybersecurity breach, who is held accountable? Is it the developers who created the algorithm, the organizations that deployed it, or the users who relied on its recommendations? These questions are crucial as they determine how we navigate the ethical landscape of AI in cybersecurity.
One of the fundamental challenges in establishing accountability in AI decision-making is understanding the opacity of many AI systems. Often, these algorithms operate as "black boxes," meaning their decision-making processes are not fully transparent. This lack of clarity can make it difficult to trace back the origins of a decision, leading to a situation where accountability is diffuse. For instance, if an AI system mistakenly flags a legitimate transaction as fraudulent, causing significant financial loss, pinpointing who is responsible can be like trying to find a needle in a haystack. The developers might argue that they provided the best tools available, while organizations might claim they were following the AI's recommendations. This diffusion of responsibility raises ethical concerns that cannot be ignored.
Another layer to this complexity is the legal framework surrounding AI technologies. Currently, there is no universal standard that dictates who is liable for AI failures, leaving many organizations in a gray area. As AI systems become more autonomous, the question of liability becomes even more critical. For example, if an AI-driven security system fails to prevent a data breach, the organization may face significant legal repercussions. However, if the AI's failure was due to a flaw in its design or training data, should the developers be held responsible? This ambiguity highlights the urgent need for clear regulatory frameworks that define accountability in AI decision-making.
To address these challenges, stakeholders must engage in a collaborative dialogue to establish guidelines that ensure ethical practices in AI usage. Here are some potential strategies to enhance accountability:
- Transparent Algorithms: Developers should strive to create more transparent AI systems that allow stakeholders to understand how decisions are made.
- Clear Responsibility Assignments: Organizations should establish clear lines of responsibility for AI-driven decisions, ensuring that all parties understand their roles.
- Robust Testing and Validation: Regular testing and validation of AI systems can help identify potential issues before they lead to significant consequences.
- Legal Framework Development: Policymakers should work towards creating legal frameworks that define liability and accountability in AI applications.
In conclusion, the journey towards accountability in AI decision-making is fraught with challenges, but it is a necessary path to ensure ethical practices in cybersecurity. As AI continues to evolve, it is imperative that we establish clear guidelines and frameworks that protect not only organizations but also the individuals whose data they safeguard. By fostering transparency, assigning responsibility, and developing robust legal frameworks, we can navigate the complexities of AI in cybersecurity and build a safer digital future.
- What is accountability in AI decision-making?
Accountability in AI decision-making refers to the responsibility that individuals or organizations have for the actions taken by AI systems, especially in the context of cybersecurity. - Why is accountability important in AI?
Accountability is crucial to ensure ethical practices, protect users' rights, and maintain trust in AI technologies, particularly in sensitive areas like cybersecurity. - Who is liable when an AI system fails?
Liability can fall on various parties, including developers, organizations, and users, depending on the circumstances surrounding the AI's failure and the legal frameworks in place. - How can organizations ensure accountability in AI?
Organizations can ensure accountability by establishing clear responsibility assignments, promoting transparency in AI algorithms, and adhering to robust testing and validation practices.
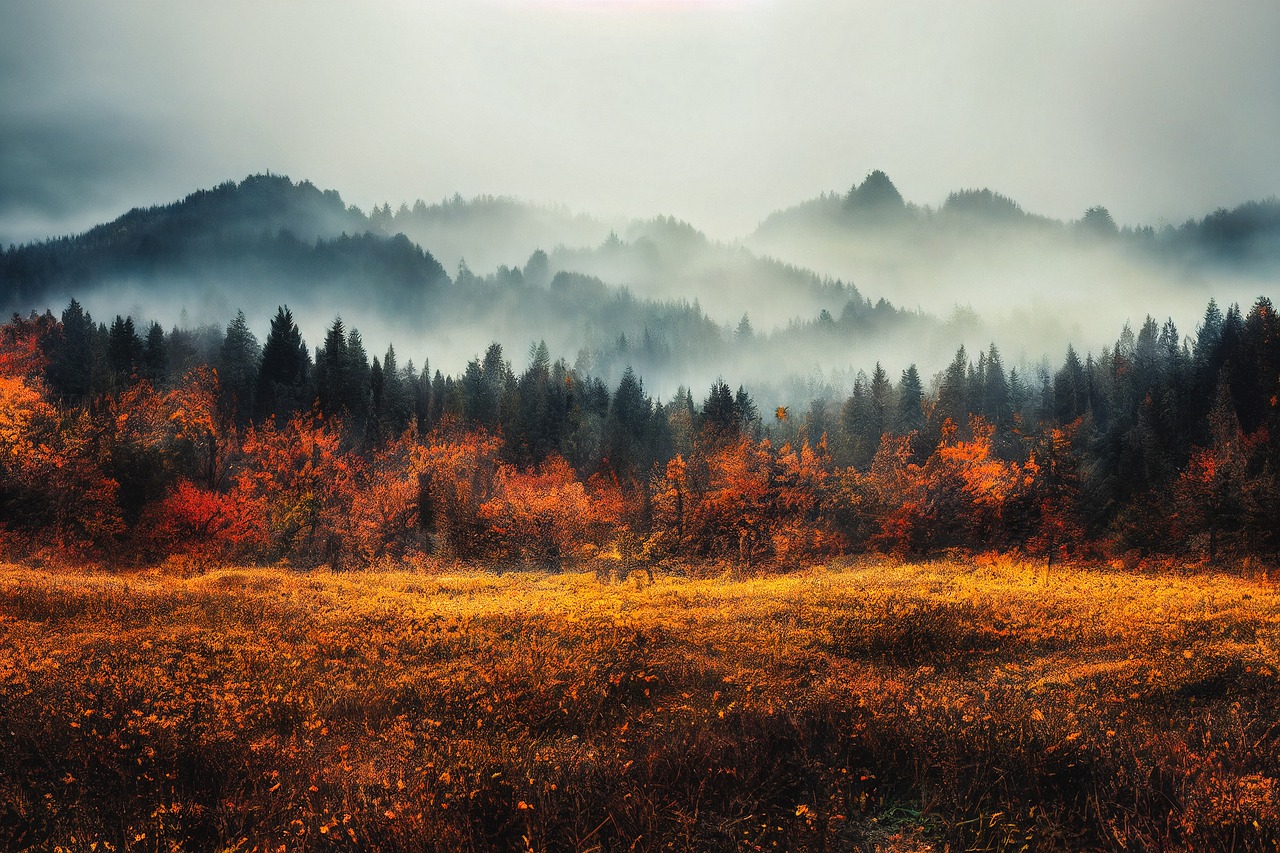
Liability for AI Failures
When it comes to the realm of artificial intelligence (AI) in cybersecurity, the question of liability for AI failures is a hot topic that sparks intense debate. Imagine this: you've entrusted a sophisticated AI system to safeguard your organization’s sensitive data, only to find out that it failed to detect a significant breach. Now, who is to blame? Is it the developers who created the AI, the organization that deployed it, or the users who relied on its predictions? The complexity of AI technology complicates the issue of accountability.
In many cases, AI systems operate as black boxes, making it difficult to trace back decisions to specific inputs or programming. This lack of transparency can lead to a tangled web of responsibility. For instance, if an AI mistakenly flags a legitimate transaction as fraudulent, the repercussions can be severe—loss of revenue, damaged reputation, and even legal consequences. Therefore, establishing clear lines of liability is crucial for organizations that leverage AI in their cybersecurity strategies.
To understand the landscape of liability, we can categorize potential responsible parties:
- Developers: They design and create the algorithms that power AI systems. If the AI fails due to a flaw in its design, developers may bear some responsibility.
- Organizations: When businesses implement AI solutions, they must ensure that these systems are adequately tested and monitored. Failure to do so could lead to liability on their part.
- Users: In some cases, users may also share the blame, especially if they misuse the technology or ignore alerts generated by the AI.
Furthermore, the legal landscape surrounding AI liability is still evolving. Courts and lawmakers are grappling with how to address these challenges. Some argue for the establishment of a new legal category specifically for AI-related incidents, while others suggest adapting existing laws to cover AI failures. The key takeaway here is that as AI continues to integrate into cybersecurity, the need for a robust framework to address liability becomes increasingly urgent.
In conclusion, the issue of liability for AI failures in cybersecurity is multifaceted and requires collaboration among developers, organizations, and regulators. Each party must play their part in ensuring that AI technologies are not only effective but also ethically sound. As we move forward, it’s imperative to create a system that holds the right parties accountable, thereby fostering trust in AI-driven cybersecurity solutions.
- Who is responsible if an AI system fails to protect sensitive data?
Responsibility can fall on developers, organizations, or users, depending on the circumstances of the failure. - Are there existing laws that govern AI liability?
The legal framework is still developing, with ongoing discussions about how to adapt current laws to cover AI-related incidents. - What steps can organizations take to mitigate liability risks?
Organizations should ensure thorough testing of AI systems, continuous monitoring, and clear documentation of AI decision-making processes.
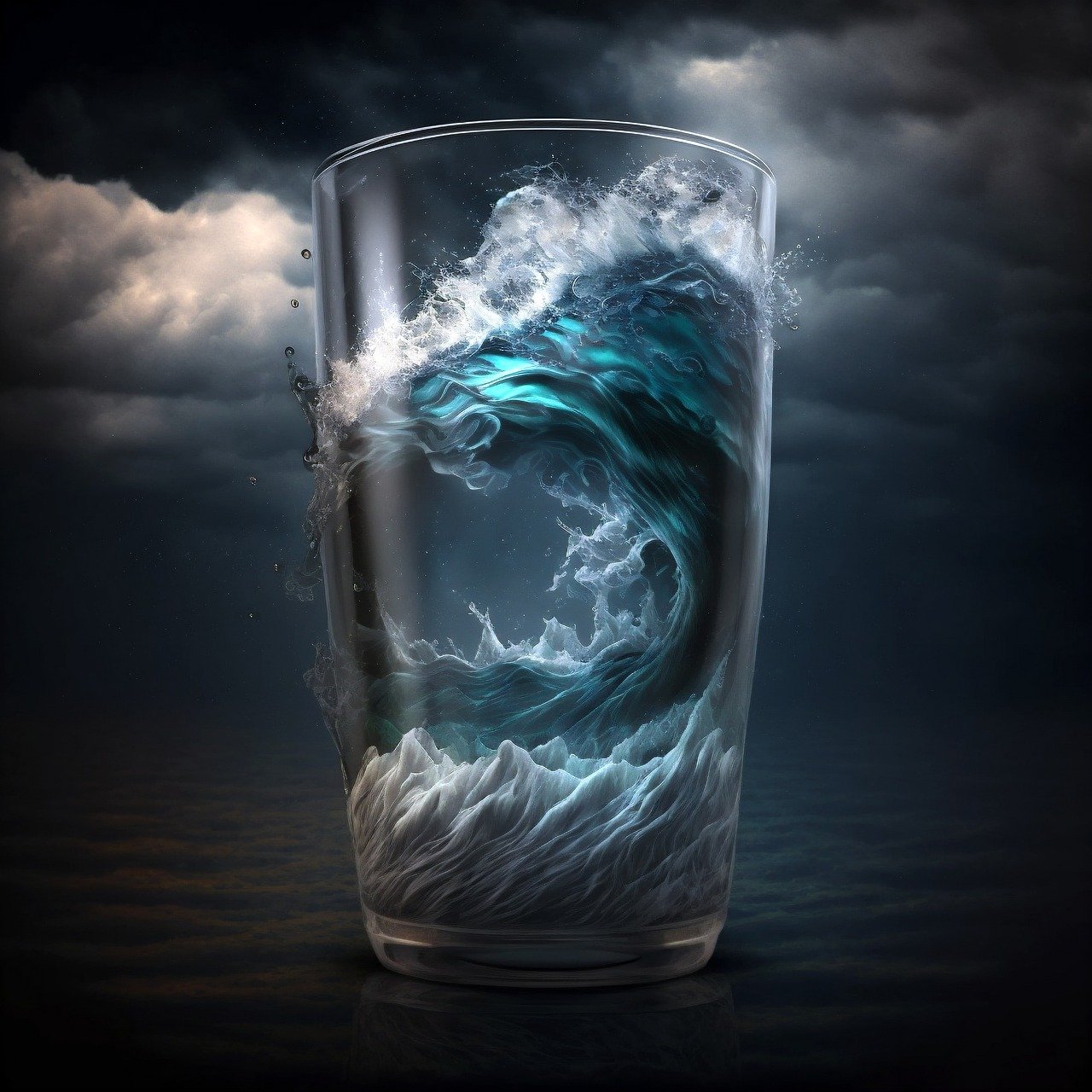
Regulatory Frameworks
The rapid integration of artificial intelligence (AI) into cybersecurity has prompted a pressing need for robust that govern its use. As organizations increasingly rely on AI technologies to protect sensitive data and systems, the potential for misuse or unintended consequences grows. This reality calls for a comprehensive approach to regulation that not only addresses the technical aspects of AI but also considers the ethical implications of its deployment in cybersecurity environments.
One of the primary challenges in creating effective regulatory frameworks is the dynamic nature of technology itself. AI is constantly evolving, which makes it difficult for regulations to keep pace. Therefore, regulators must adopt a flexible approach that allows for ongoing updates and adaptations as new technologies and methods emerge. This could involve establishing adaptive regulatory bodies that focus on continuous learning and feedback from the cybersecurity landscape.
Moreover, these frameworks should encompass various aspects of AI usage, including:
- Data Privacy: Regulations should ensure that AI systems adhere to strict data privacy standards, safeguarding individual rights while enabling effective security measures.
- Accountability: Clear guidelines about who is responsible for AI-driven decisions are crucial, particularly in cases of security breaches or failures.
- Transparency: Organizations must be transparent about how AI algorithms operate and the data they utilize, fostering trust among users and stakeholders.
Additionally, international cooperation is essential in developing regulatory frameworks that can be applied globally. Cyber threats often transcend borders, making it imperative for countries to collaborate on establishing consistent standards and practices. This could involve creating international treaties or agreements that outline the ethical use of AI in cybersecurity, ensuring that all parties are held to the same standards.
In light of these considerations, several key elements should be integrated into any regulatory framework for AI in cybersecurity:
Element | Description |
---|---|
Risk Assessment | Regular evaluations of AI systems to identify potential risks and vulnerabilities. |
Ethical Guidelines | Clear principles that guide the ethical use of AI, ensuring fairness and accountability. |
Compliance Monitoring | Ongoing oversight to ensure adherence to established regulations and standards. |
Public Engagement | Involving stakeholders in the regulatory process to foster transparency and trust. |
Ultimately, the establishment of effective regulatory frameworks for AI in cybersecurity is not just about compliance; it’s about fostering a culture of responsibility and ethical behavior. As organizations navigate this complex landscape, they must prioritize the development of systems that not only protect data but also respect the rights and privacy of individuals. By doing so, they can build a more secure and trustworthy digital environment for everyone.
- What are the main ethical concerns regarding AI in cybersecurity?
Ethical concerns include privacy violations, algorithmic bias, and accountability for AI-driven decisions. - How can regulatory frameworks help mitigate risks associated with AI?
Regulatory frameworks can establish guidelines for data privacy, accountability, and transparency, ensuring responsible AI usage. - Why is international cooperation important in regulating AI?
Cyber threats are global, so consistent international standards are necessary to effectively combat these challenges.
Frequently Asked Questions
- What is the role of AI in cybersecurity?
AI plays a crucial role in enhancing cybersecurity by automating threat detection and response. It helps organizations identify potential threats more efficiently and respond to them in real-time, thus improving overall security protocols.
- Are there ethical concerns associated with AI in cybersecurity?
Yes, there are several ethical concerns, including privacy violations, potential biases in algorithms, and issues surrounding accountability. These concerns must be addressed to ensure that AI is used responsibly in cybersecurity.
- How does AI impact privacy and data protection?
AI systems often require access to large amounts of data, which can lead to privacy issues. It's essential to strike a balance between effective security measures and respecting individual privacy rights.
- What are ethical data collection practices for AI?
Ethical data collection practices involve gathering data in a way that respects user privacy and complies with regulations. Organizations should ensure transparency and obtain informed consent from users when collecting their data.
- How do biases in AI algorithms affect cybersecurity?
Biases in AI algorithms can lead to unfair treatment of individuals or groups, potentially resulting in discriminatory practices. It's vital to address these biases to ensure fair and equitable cybersecurity measures.
- Who is accountable for AI-driven decisions in cybersecurity?
Determining accountability for AI-driven decisions can be complex. It involves understanding the roles of developers, organizations, and users, especially in the event of cybersecurity breaches or failures.
- What happens if an AI system fails?
If an AI system fails, questions of liability arise. Developers, organizations, and users may all share responsibility, depending on the circumstances surrounding the failure and the legal frameworks in place.
- Is there a need for regulations governing AI in cybersecurity?
Absolutely! As AI technologies continue to evolve, there is an increasing need for regulatory frameworks that ensure ethical practices and accountability in their use within cybersecurity.