Resolving Ethical Dilemmas in AI
As we plunge deeper into the era of artificial intelligence, the ethical dilemmas that accompany this technology become increasingly complex and pressing. It’s like walking a tightrope: on one side, we have the incredible potential of AI to revolutionize industries, improve lives, and enhance our understanding of the world. On the other side, we face the daunting reality of ethical challenges that could lead to significant societal harm if left unaddressed. So, how do we navigate this intricate landscape? The answer lies in understanding these dilemmas, developing robust frameworks for ethical decision-making, and ensuring that AI serves humanity positively.
At the heart of resolving ethical dilemmas in AI is the recognition that technology should not operate in a vacuum. The impact of AI extends beyond mere algorithms and codes; it touches on fundamental aspects of our society, such as privacy, human rights, and accountability. As we explore the ethical challenges posed by AI, it becomes clear that a collaborative approach is essential. This includes input from ethicists, technologists, policymakers, and the public to ensure a holistic understanding of the implications of AI deployment.
One of the primary ethical considerations in AI is the potential for bias. Imagine a world where an AI system decides who gets a job, who receives a loan, or who is deemed worthy of medical treatment. If these systems are trained on biased data or designed without inclusive perspectives, the consequences can be devastating. Therefore, addressing bias in AI is not just a technical issue; it’s a moral imperative. Developers must actively seek to understand the sources of bias—ranging from data selection to algorithm design—and implement strategies to mitigate its impact.
Moreover, accountability in AI decision-making is paramount. When an AI system makes a decision that adversely affects an individual, who is responsible? Is it the developer, the organization, or the AI itself? Establishing clear lines of accountability is essential for ethical governance. This ensures that there are mechanisms in place to address grievances and rectify injustices caused by AI systems. Without accountability, we risk eroding trust in technology, which could hinder its potential benefits.
Transparency is another critical element in resolving ethical dilemmas in AI. Users must understand how AI systems operate and make decisions. This transparency fosters trust and allows individuals to make informed choices about their interactions with technology. For instance, if a user knows that an AI system considers certain factors in its decision-making process, they can better understand the rationale behind its conclusions. Providing clear explanations for AI decisions is not just a technical requirement; it’s a fundamental aspect of ethical AI deployment.
In conclusion, resolving ethical dilemmas in AI requires a multifaceted approach. By acknowledging the significance of ethics in technology, addressing bias, establishing accountability, and promoting transparency, we can pave the way for AI systems that enhance our lives while safeguarding our values. As we move forward, it’s crucial to engage in ongoing discussions about the ethical implications of AI, ensuring that we remain vigilant and proactive in our efforts to create a fair and just technological landscape.
- What are the main ethical dilemmas in AI? The main ethical dilemmas in AI include bias in algorithms, accountability in decision-making, and transparency in AI processes.
- How can bias in AI be mitigated? Bias can be mitigated by using diverse datasets, continuous monitoring, and engaging diverse teams in the development process.
- Why is accountability important in AI? Accountability is crucial in AI to ensure that there are responsible parties when AI systems make decisions that impact individuals and society.
- What role does transparency play in AI? Transparency helps build trust between users and AI systems by providing clear communication about how decisions are made.
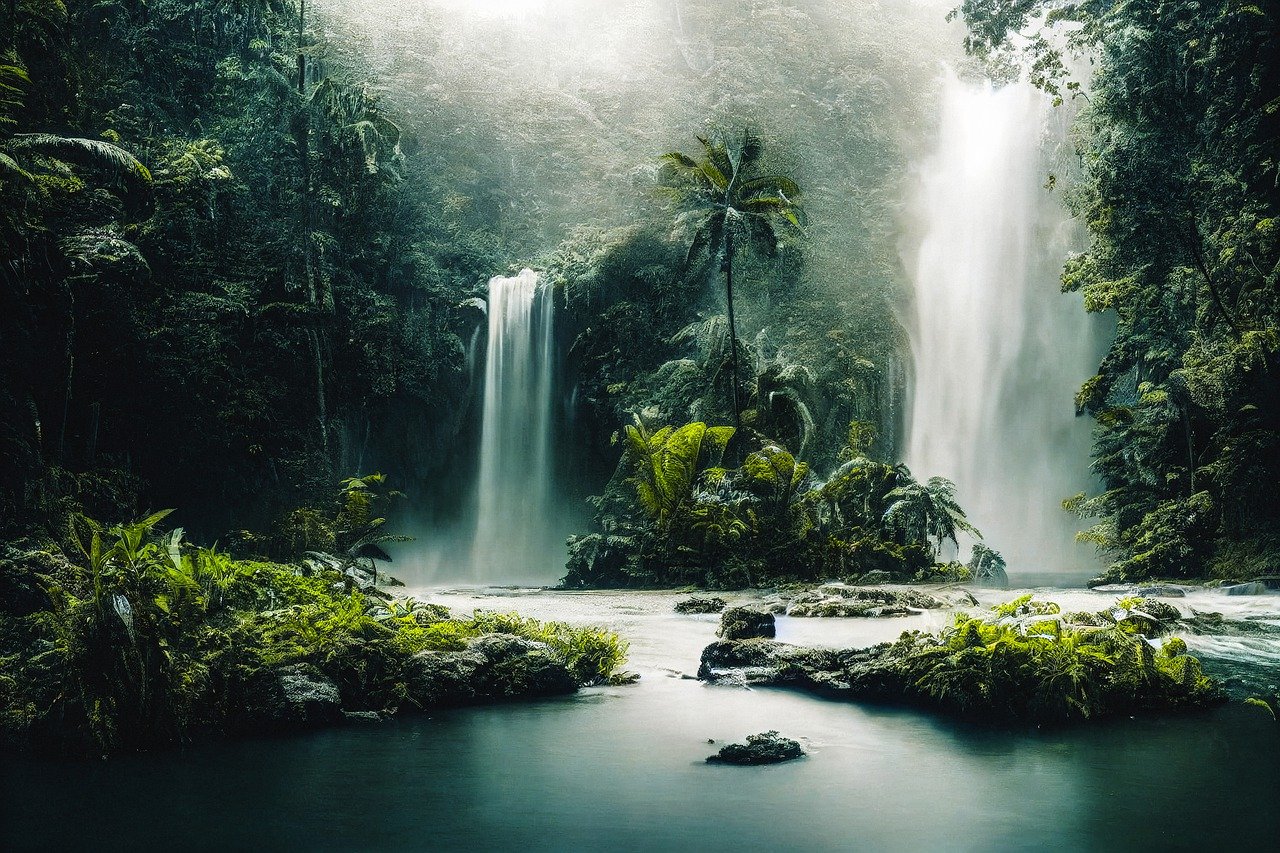
The Importance of Ethics in AI
In today's rapidly evolving technological landscape, the significance of ethics in artificial intelligence (AI) cannot be overstated. As AI systems become more integrated into our daily lives, from autonomous vehicles to recommendation algorithms, the ethical implications of these technologies are profound. Imagine a world where machines make decisions that affect our lives without any moral compass guiding them—scary, right? That's why establishing a robust ethical framework is essential to ensure that AI serves humanity positively while minimizing harm.
At the heart of this ethical discourse lies the responsibility of developers, organizations, and policymakers to create systems that are not only efficient but also fair and just. Without a clear ethical guideline, AI can perpetuate existing biases, leading to unfair treatment of individuals and groups. For example, consider a hiring algorithm that favors candidates from specific demographics simply because of biased training data. This scenario illustrates how the absence of ethical considerations can perpetuate inequality.
Moreover, the ethical implications of AI extend beyond mere bias. They encompass critical issues such as privacy, accountability, and the very essence of human rights. As AI systems gather and process vast amounts of personal data, the risk of privacy violations increases. How can we trust a system that operates in the shadows, making decisions based on information we never consented to share? This question highlights the urgent need for transparency in AI operations.
To navigate this complex landscape, it’s crucial to adopt a multifaceted approach to ethics in AI. Here are some key principles that should guide AI development:
- Fairness: Ensuring that AI systems treat all individuals equitably.
- Transparency: Making AI processes understandable and accessible to users.
- Accountability: Establishing clear responsibilities for AI decision-making.
- Privacy: Safeguarding personal data and respecting user consent.
By embracing these principles, we can create AI technologies that not only enhance our lives but also uphold our values. In doing so, we can foster a society where technology acts as a partner in progress rather than a source of division and mistrust. The ethical challenges posed by AI are not just technical issues; they are deeply human questions that demand our attention and action.
In conclusion, the importance of ethics in AI is paramount. As we continue to innovate and integrate AI into various sectors, we must remain vigilant and proactive in addressing the ethical dilemmas that arise. After all, the ultimate goal of AI should be to enhance human well-being and promote a fair and just society.
Here are some common questions regarding the importance of ethics in AI:
- Why is ethics important in AI? Ethics is crucial in AI to ensure that technology serves humanity positively, minimizes harm, and prevents bias in decision-making.
- What are the main ethical concerns in AI? Key concerns include bias, accountability, transparency, and privacy.
- How can we mitigate bias in AI? By implementing diverse datasets, continuous monitoring, and involving a wide range of stakeholders in the development process.
- What role do policymakers play in AI ethics? Policymakers are essential for establishing regulations and frameworks that guide ethical AI deployment.
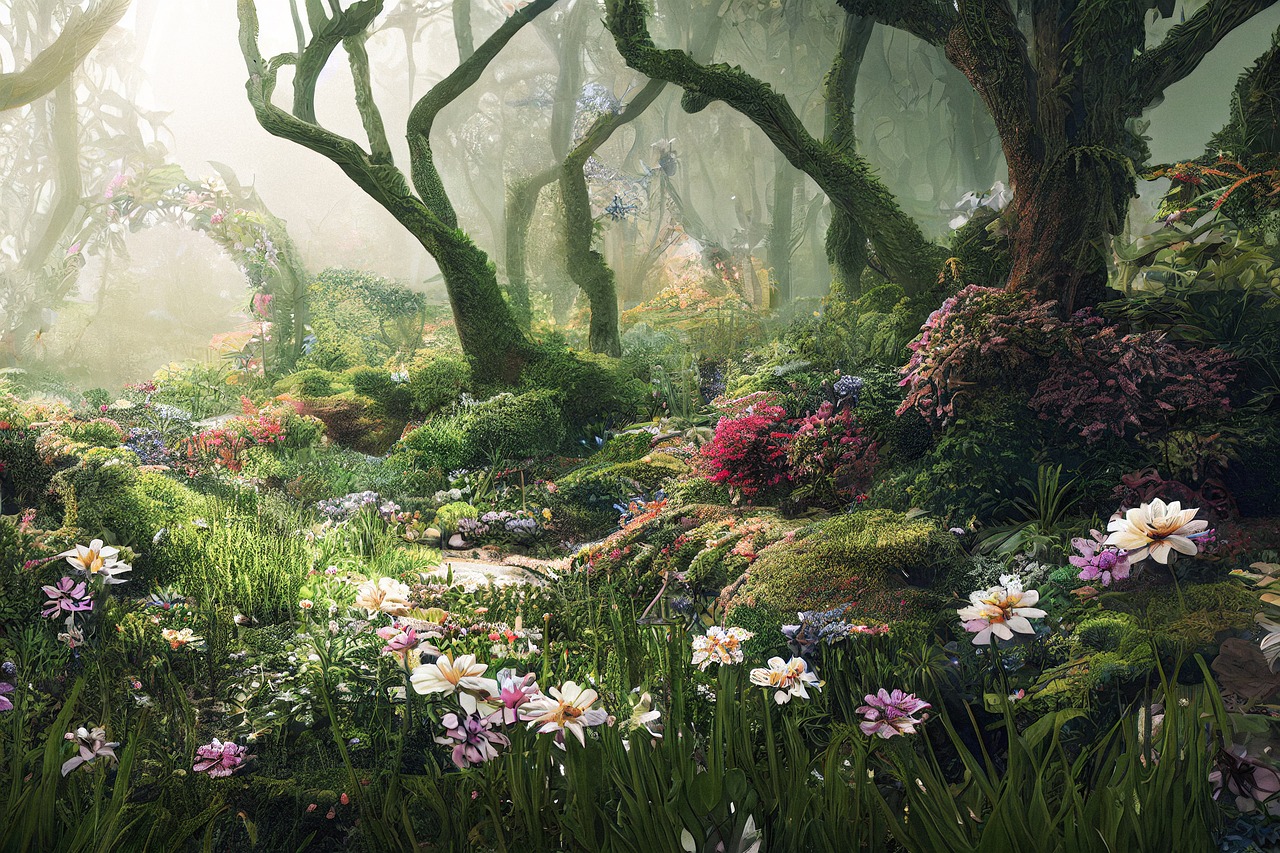
Common Ethical Dilemmas in AI
Artificial Intelligence (AI) is not just a technological marvel; it brings a plethora of ethical dilemmas that we must navigate. As we increasingly rely on AI systems to make decisions, we face profound questions about fairness, accountability, and transparency. It's like handing the keys of a high-speed car to a teenager—exciting yet fraught with potential hazards. In this section, we will delve into the most pressing ethical challenges that AI poses and how they impact both developers and users.
One of the most significant ethical dilemmas is bias in AI algorithms. Imagine a world where your chances of getting a job, a loan, or even a fair trial hinge on a computer's judgment. Unfortunately, this is the reality we face when AI systems are trained on biased data. These biases can lead to unfair treatment of individuals based on race, gender, or socioeconomic status. For instance, a hiring algorithm might favor candidates from certain backgrounds while unfairly disadvantaging others, perpetuating existing inequalities.
Bias in AI algorithms can arise from various sources. It's crucial to understand these origins to mitigate their impact effectively. Here are some common sources of bias:
- Data Selection: If the data used to train an AI system is not representative of the entire population, the outcomes will reflect those imbalances.
- Algorithm Design: The way algorithms are constructed can inadvertently favor certain groups over others.
- User Interaction: The way users interact with AI can introduce biases, as preferences may skew results based on previous data.
To combat these biases, developers must implement diverse datasets and engage in continuous monitoring. This means actively seeking out data that represents a wide range of demographics and ensuring that algorithms are tested against various scenarios to identify any potential biases that may arise. By doing so, we can work towards creating fair and equitable AI systems that serve all members of society.
Another ethical dilemma revolves around accountability. When an AI system makes a decision that negatively impacts someone's life, who is responsible? Is it the developers, the company that deployed the AI, or the AI itself? Establishing accountability in AI systems is essential for ethical governance. Without clear lines of responsibility, victims of AI-related injustices may have nowhere to turn for recourse. This dilemma raises fundamental questions about the nature of responsibility in a world increasingly dominated by machines.
As we navigate these ethical challenges, it becomes evident that the implications of AI extend far beyond the technology itself. The decisions we make regarding the development and deployment of AI will shape our society for years to come. It's not merely about what AI can do; it's about what it should do. We must strive to ensure that AI serves humanity positively, minimizing harm and bias in its decision-making processes.
Q: What are the main ethical dilemmas associated with AI?
A: The primary ethical dilemmas include bias in algorithms, accountability for decisions made by AI systems, and the need for transparency in how these systems operate.
Q: How can we mitigate bias in AI?
A: Mitigating bias involves using diverse datasets, continuous monitoring, and regularly testing algorithms to ensure fairness in outcomes.
Q: Who is responsible for decisions made by AI?
A: Accountability can be complex; it may involve developers, companies, or even regulatory bodies, depending on the context and implications of the AI's decisions.
Q: Why is transparency important in AI?
A: Transparency builds trust among users and allows for better understanding of how AI systems make decisions, which is crucial for ethical governance.
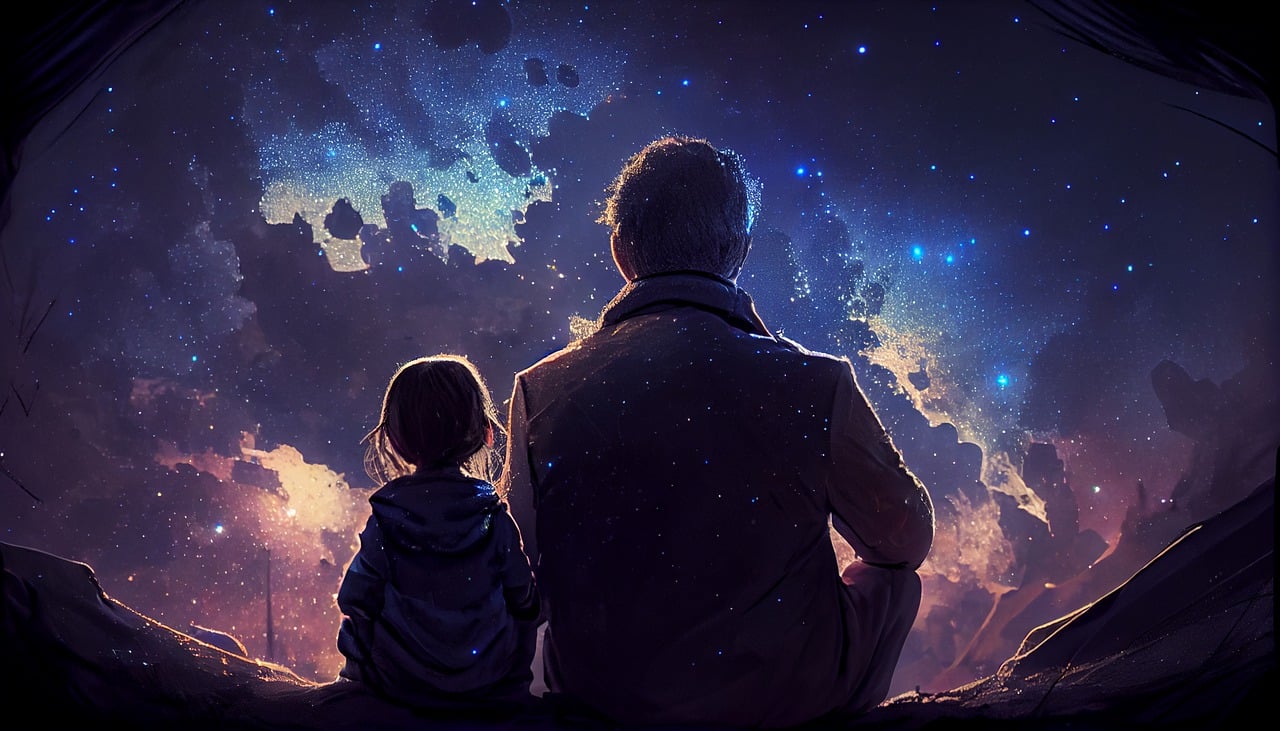
Bias in AI Algorithms
Bias in AI algorithms is a pressing issue that can have serious repercussions on individuals and communities. When we talk about bias, we're not just referring to a mere oversight; we're discussing a fundamental flaw that can skew outcomes and perpetuate inequality. Imagine a world where a computer program decides who gets a loan, who is hired for a job, or even who gets a prison sentence. If that program is biased, the consequences can be devastating. It’s like letting a biased referee officiate a game—no one wants to play under those conditions!
So, where does this bias come from? It can originate from various sources, including the data used to train the algorithms, the design of the algorithms themselves, and even the way users interact with the AI systems. For instance, if an AI is trained on historical data that reflects societal biases, it will likely replicate those biases in its decision-making processes. This is akin to teaching a child using outdated textbooks that contain inaccuracies; the child will grow up with a flawed understanding of the world.
To tackle this issue, it's crucial to understand the different sources of bias:
- Data Selection: If the data used to train AI models is not representative of the entire population, the AI will inevitably favor certain groups over others.
- Algorithm Design: The way algorithms are structured can introduce bias, especially if they prioritize certain variables over others without proper justification.
- User Interaction: User input can also skew AI behavior, especially if the system learns from feedback that reflects human biases.
Addressing these biases is not just a technical challenge; it requires a concerted effort from developers, policymakers, and society as a whole. Some practical steps to mitigate bias include:
- Implementing diverse datasets that accurately reflect the demographics of the population.
- Conducting regular audits of AI systems to identify and rectify any biases that may arise.
- Encouraging interdisciplinary collaboration among technologists, ethicists, and community representatives to foster a more holistic understanding of the implications of AI.
Ultimately, the goal is to create AI systems that are not only efficient but also fair and equitable. By acknowledging and addressing bias in AI algorithms, we can work towards a future where technology serves everyone equally, rather than perpetuating existing disparities. It's a complex challenge, but with the right approach and dedication, we can make significant strides toward a more just technological landscape.
Q1: What is bias in AI algorithms?
Bias in AI algorithms refers to systematic favoritism or discrimination that occurs when the AI makes decisions based on flawed or unrepresentative data, leading to unfair outcomes.
Q2: How can bias in AI be mitigated?
Bias can be mitigated by using diverse datasets, conducting regular audits, and fostering interdisciplinary collaboration among stakeholders.
Q3: Why is addressing bias in AI important?
Addressing bias is crucial to ensure that AI technologies are fair and equitable, preventing discrimination and ensuring that all individuals are treated justly.
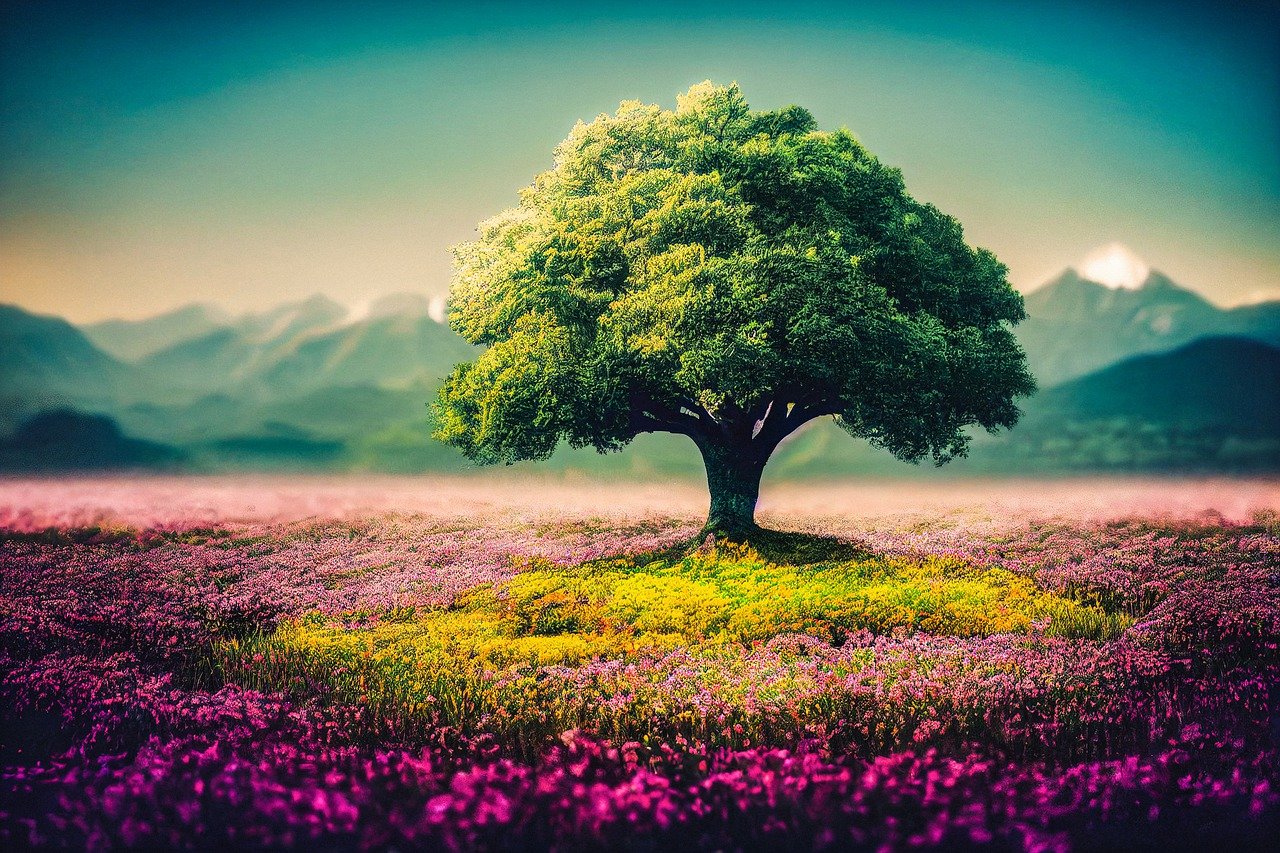
Sources of Bias
When we delve into the in artificial intelligence, it's crucial to understand that bias doesn't just appear out of thin air; it often has roots in the data, algorithms, and even the very design of the systems we create. Imagine AI as a mirror reflecting society's values, yet sometimes that mirror is fogged by preconceived notions and historical injustices. Bias can emerge from several key areas:
- Data Selection: The data used to train AI models is paramount. If the dataset is skewed or unrepresentative of the broader population, the AI will inevitably inherit those biases. For example, if an AI system is trained predominantly on data from one demographic, it may struggle to make fair decisions for individuals outside that group.
- Algorithm Design: The algorithms that process this data can also introduce bias. Choices made during the design phase—such as which variables to prioritize or how to weigh certain inputs—can significantly impact outcomes. Think of it like a recipe: if you add too much of one ingredient and not enough of another, the final dish may not taste right.
- User Interaction: The way users interact with AI systems can further exacerbate bias. For instance, if users provide feedback based on their own biases or experiences, this can skew the AI's learning process. It's a bit like teaching a dog tricks; if you reward certain behaviors while ignoring others, the dog will only learn what you reinforce.
Understanding these sources is vital for developers and stakeholders looking to create fair and equitable AI systems. By scrutinizing the datasets, questioning algorithmic choices, and considering user input, we can begin to dismantle the biases that undermine the integrity of AI. The journey towards fairness in AI is ongoing, and it requires a collective effort to ensure that technology serves all of humanity without prejudice.
- What is bias in AI? Bias in AI refers to systematic favoritism or prejudice in the outcomes produced by AI systems, often stemming from the data or design choices made during development.
- How can bias in AI be identified? Bias can be identified through rigorous testing and analysis of AI outputs across different demographics and scenarios, ensuring that the AI performs equitably.
- What steps can be taken to mitigate bias? Steps include using diverse datasets, implementing fairness algorithms, and conducting regular audits of AI systems to identify and rectify biases.
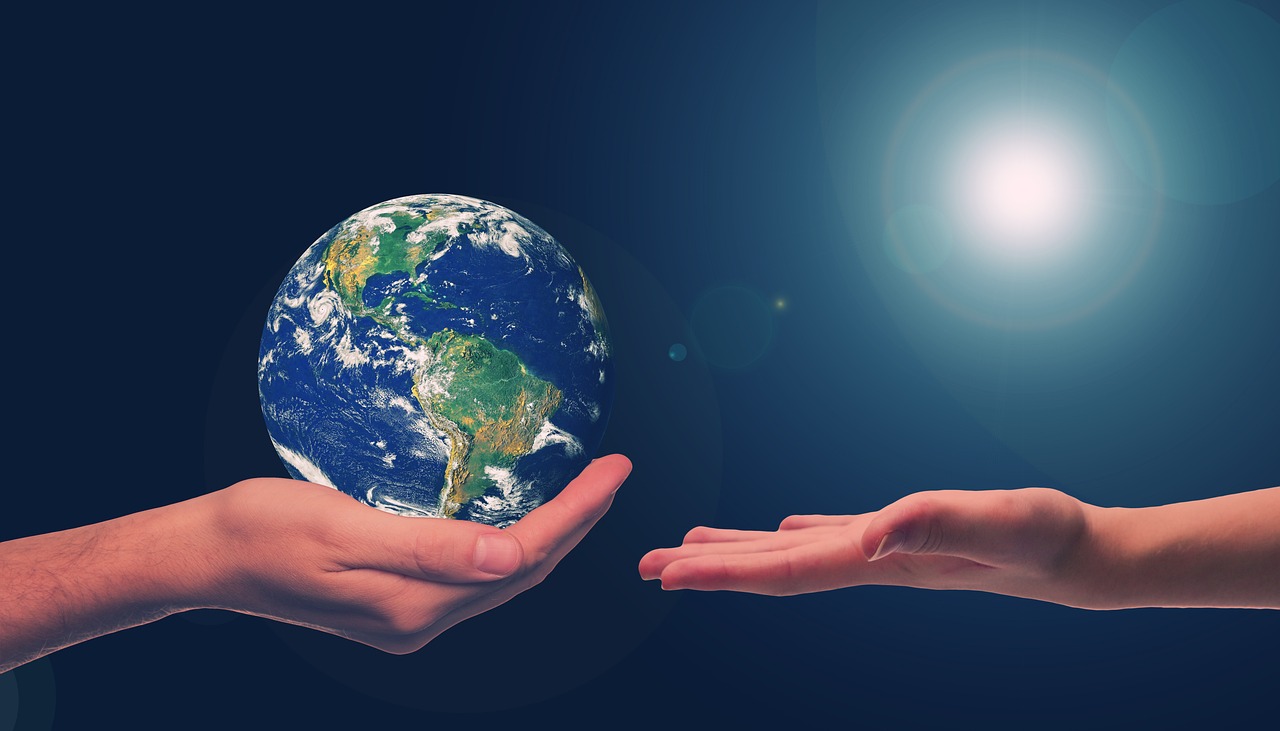
Mitigating Bias
In the quest for fairness in artificial intelligence, is not just an option; it’s a necessity. Bias in AI can manifest in various forms and lead to outcomes that unfairly disadvantage certain groups of people. Imagine a world where a job applicant is overlooked not because of their qualifications, but due to biased algorithms that favor one demographic over another. This is where the importance of actively addressing bias becomes clear.
One of the most effective strategies for mitigating bias is the use of diverse datasets. When AI systems are trained on data that reflects a wide range of experiences, backgrounds, and perspectives, the likelihood of bias decreases significantly. It’s akin to having a well-rounded team in a brainstorming session—diversity fosters creativity and innovation. However, simply having diverse data isn’t enough. Continuous monitoring and evaluation of AI outputs are crucial to ensure that the algorithms remain fair over time.
Another vital step in the bias mitigation process is to implement algorithmic audits. These audits serve as a check-and-balance mechanism, allowing developers to scrutinize their algorithms for any signs of bias. By regularly assessing how AI systems make decisions, organizations can identify and rectify biases before they cause harm. Think of it as a health check-up for your AI; just as you wouldn’t ignore your health, you shouldn’t overlook the health of your algorithms.
Moreover, engaging with stakeholders is essential. This means involving community members, advocacy groups, and other relevant parties in the development process. Their insights can illuminate potential biases that developers might overlook. It’s like having a navigator when you’re sailing through uncharted waters; their guidance can help steer clear of treacherous areas.
Here’s a quick overview of practical steps to mitigate bias:
- Utilize diverse datasets that represent various demographics.
- Conduct regular algorithmic audits to assess and address bias.
- Engage with stakeholders to gain diverse perspectives during development.
- Implement feedback loops that allow users to report perceived biases.
In conclusion, addressing bias in AI is a multifaceted endeavor that requires a combination of diverse data, rigorous audits, and community engagement. By taking these steps, we can move towards a future where AI systems are not only efficient but also fair and equitable for all. As we continue to develop and deploy AI technologies, let’s remember that our ultimate goal should be to create systems that enhance human potential rather than diminish it.
- What is bias in AI? Bias in AI refers to systematic and unfair discrimination against certain groups of people due to flawed data or algorithms.
- How can diverse datasets help mitigate bias? Diverse datasets ensure that the AI system is trained on a wide range of experiences, reducing the chance of favoring one group over another.
- What are algorithmic audits? Algorithmic audits are evaluations of AI systems to identify and correct biases in decision-making processes.
- Why is stakeholder engagement important? Engaging stakeholders provides valuable insights that can highlight potential biases and improve the fairness of AI systems.
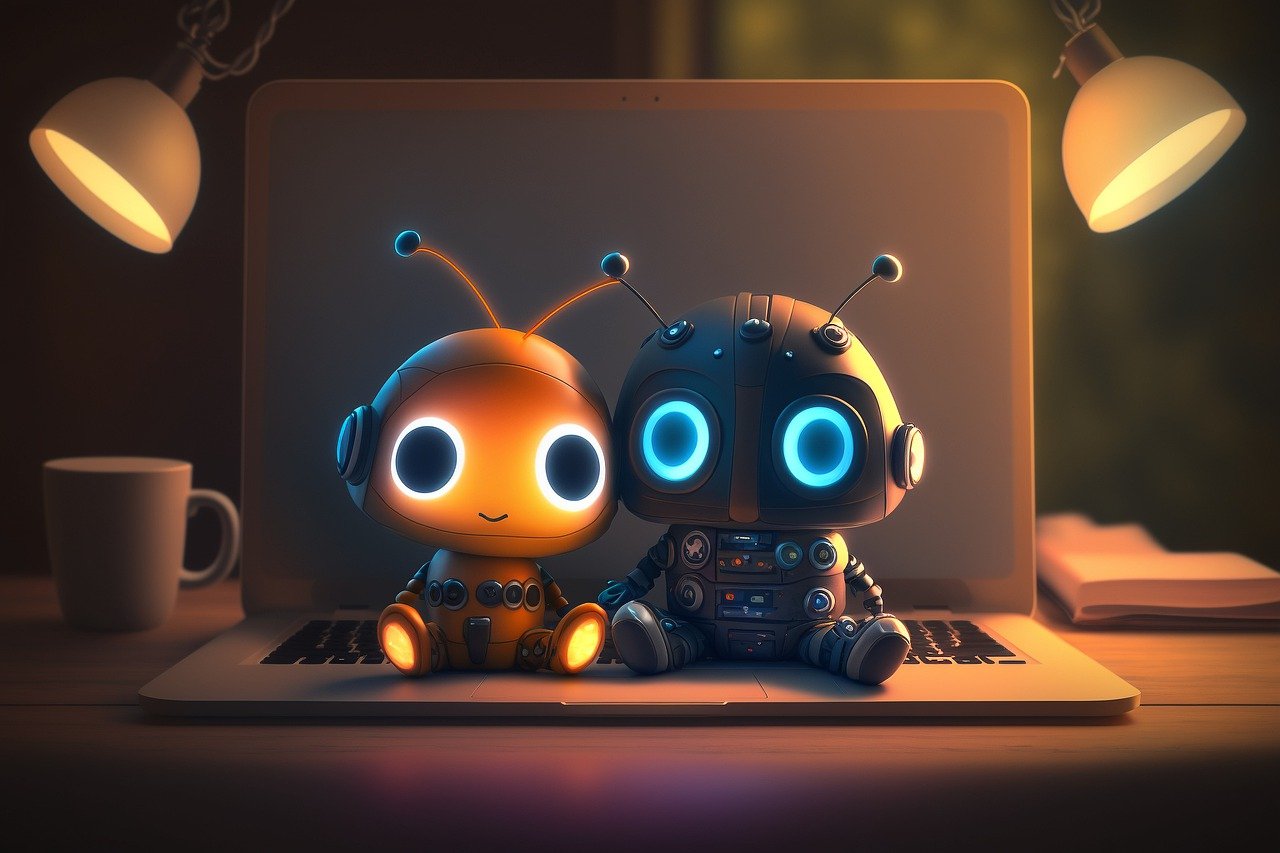
Accountability in AI Decision-Making
As artificial intelligence systems become increasingly integrated into our daily lives, the question of accountability in AI decision-making looms large. Who is responsible when an AI system makes a mistake? Is it the developers, the users, or the organizations that deploy these technologies? These questions are not merely academic; they have real-world implications for individuals and society at large. The complexity of AI systems can obscure the decision-making process, making it challenging to pinpoint accountability when things go awry.
To grasp the nuances of accountability in AI, we must first recognize that AI systems operate based on algorithms that are often trained on vast datasets. These datasets can contain biases, which can lead to flawed outcomes. When an AI system makes a biased decision, it raises ethical concerns about who should be held accountable. Should the blame fall on the data scientists who created the model, the organizations that implemented it, or the users who relied on its recommendations? The lack of clarity in this chain of responsibility can lead to a culture of evasion rather than accountability.
Moreover, establishing accountability is essential for fostering trust in AI technologies. If users feel that there is no clear path to accountability, they may become hesitant to adopt AI solutions, fearing potential negative consequences. This skepticism can hinder technological advancement and the benefits that AI can bring. Therefore, organizations must develop transparent processes that outline who is responsible for AI decisions and how accountability will be enforced.
One approach to enhancing accountability is the implementation of regulatory frameworks that clearly define the roles and responsibilities of all parties involved in AI development and deployment. Such frameworks can help ensure that organizations are held accountable for the outcomes of their AI systems. Additionally, these regulations can establish guidelines for ethical AI practices, including:
- Regular audits of AI systems to assess their performance and fairness.
- Clear documentation of the data sources and algorithms used in AI decision-making.
- Public reporting of AI outcomes to foster transparency and trust.
In conclusion, accountability in AI decision-making is not just a technical issue; it is a fundamental ethical concern that requires careful consideration. As we continue to integrate AI into various aspects of life, we must prioritize establishing clear lines of accountability to ensure that these technologies serve humanity responsibly and ethically. By doing so, we can mitigate risks and promote a future where AI contributes positively to society.
- What is accountability in AI? Accountability in AI refers to the responsibility of developers, organizations, and users for the decisions made by AI systems.
- Why is accountability important in AI? It is crucial for fostering trust and ensuring ethical governance, especially when AI systems make decisions that impact individuals and society.
- How can organizations ensure accountability in AI? Organizations can implement regulatory frameworks, conduct regular audits, and maintain clear documentation of their AI systems.

Transparency in AI Systems
In today's digital landscape, is not just a luxury; it's a necessity. As artificial intelligence continues to weave itself into the fabric of our daily lives, the need for clear and open communication regarding how these systems operate becomes paramount. Imagine if you were driving a car with a black box that dictated your every move without any explanation. Wouldn't you feel uneasy? This analogy captures the essence of why transparency is critical in AI. Users deserve to understand not only the decisions made by AI but also the reasoning behind those decisions. Without this understanding, trust erodes, and the potential benefits of AI can be overshadowed by fear and skepticism.
One of the main challenges facing AI developers today is the so-called "black box" problem, where the inner workings of AI algorithms are obscured from users. This opacity can lead to a lack of accountability and an inability to challenge or question AI decisions. To combat this, developers are increasingly focusing on creating systems that are not only effective but also interpretable. The goal is to ensure that users can grasp how decisions are made, which is crucial for fostering trust and enabling informed consent.
To enhance transparency, several strategies can be employed:
- Clear Communication: Developers should strive to communicate AI processes in a manner that is accessible to all users, regardless of their technical background.
- Visual Explanations: Utilizing visual aids, such as flowcharts or interactive demos, can help demystify complex algorithms.
- Feedback Mechanisms: Implementing channels for users to provide feedback on AI decisions can create a two-way communication street, fostering trust and collaboration.
Moreover, regulatory frameworks are beginning to emerge, emphasizing the importance of transparency in AI systems. These frameworks aim to set standards that require AI developers to disclose how their systems function and the data used in their algorithms. For instance, the European Union's General Data Protection Regulation (GDPR) has made strides towards ensuring that individuals have the right to understand how their data is processed by AI systems. This regulatory push reflects a growing recognition that transparency is not just a technical issue but a fundamental human right.
As we move forward, it is essential for both developers and users to advocate for greater transparency in AI. By doing so, we can create a landscape where artificial intelligence is not a mysterious force but a tool that enhances our lives while respecting our rights and dignity. The journey towards transparent AI is ongoing, but with concerted efforts from all stakeholders, we can pave the way for a more trustworthy and equitable future.
- Why is transparency in AI important? Transparency helps build trust between users and AI systems, ensuring that individuals understand how decisions are made.
- What are the consequences of a lack of transparency? A lack of transparency can lead to mistrust, misuse, and potential harm, as users may feel uncertain about the decisions made by AI.
- How can developers ensure transparency? Developers can ensure transparency by providing clear explanations of their algorithms, using visual aids, and allowing user feedback.
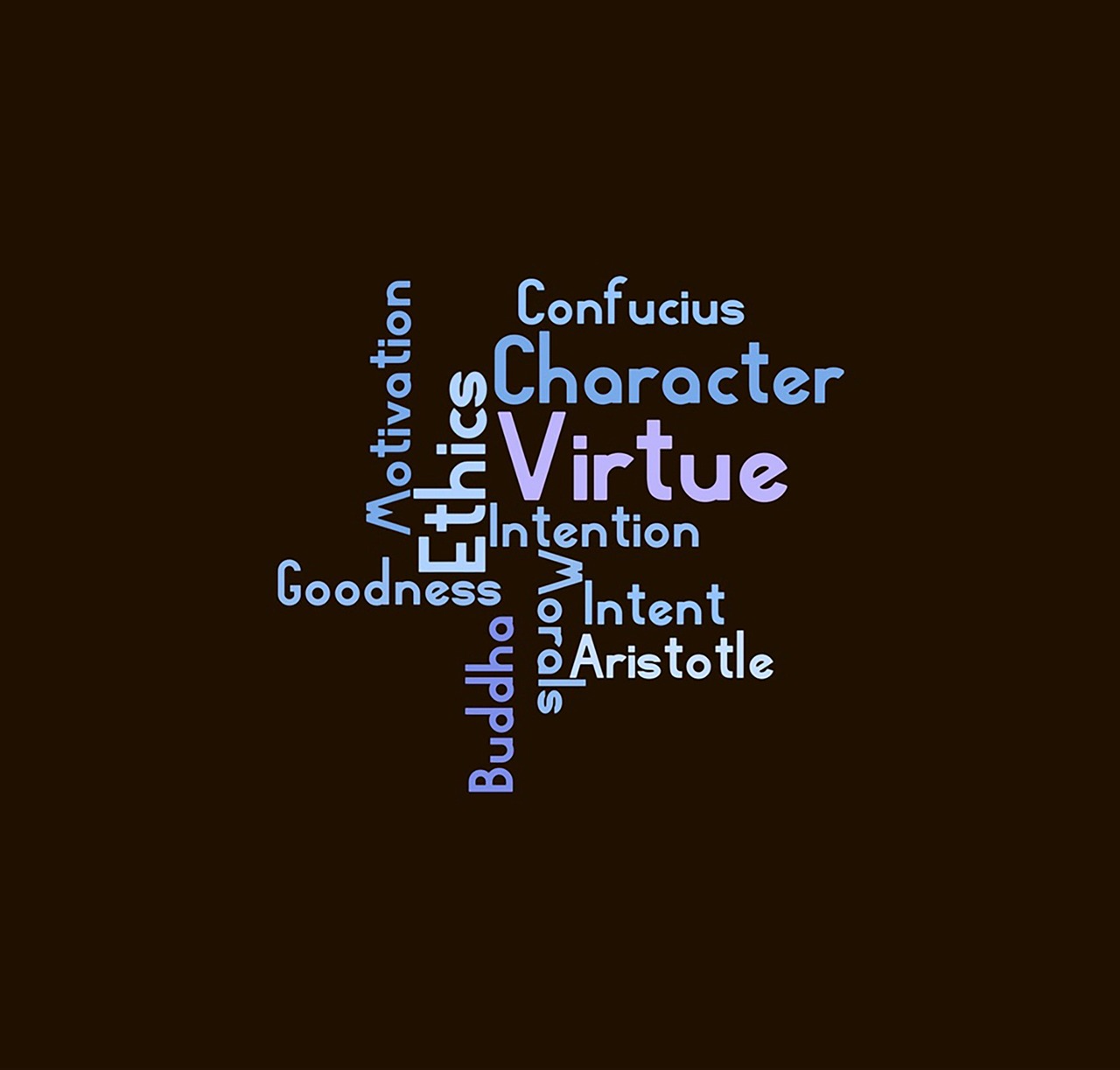
Explaining AI Decisions
In the world of artificial intelligence, the ability to explain how decisions are made is not just a luxury; it is a necessity. Imagine you're in a car that suddenly decides to brake without warning. Wouldn't you want to know why? This analogy highlights the importance of transparency in AI systems. When users understand the reasoning behind AI decisions, it fosters trust and confidence in the technology. Without this understanding, users may feel like they are in a black box, where outcomes seem random and unexplainable.
To enhance the clarity of AI decision-making processes, developers can employ various techniques. One effective method is the use of explainable AI (XAI), which aims to make the operations of AI systems more interpretable. By utilizing XAI, developers can provide insights into the algorithms and data that drive decisions. This can be achieved through:
- Feature Importance: Highlighting which factors had the most influence on a decision.
- Visualizations: Using graphs or charts to represent how input data affects outcomes.
- Case Studies: Providing examples of similar past decisions to illustrate reasoning.
Moreover, clear communication is essential. When AI systems provide explanations, they should be in a language that users can easily understand. Technical jargon can alienate users, leading to confusion and mistrust. Instead, AI explanations should be straightforward and relatable. For instance, if an AI denies a loan application, it should clearly state the reasons—such as credit score, income level, or outstanding debts—rather than just saying, "Your application was rejected."
Additionally, the context in which AI operates plays a significant role in how explanations are perceived. In high-stakes situations, such as healthcare or criminal justice, the need for transparency becomes even more critical. Users in these fields require not just an understanding of the decision but also the assurance that the AI is operating fairly and without bias. This leads to the question: how can we ensure that AI explanations are not only informative but also ethical?
One approach is to involve stakeholders in the development of AI systems. By gathering input from diverse groups—such as ethicists, domain experts, and end-users—developers can create explanations that are meaningful and relevant. This collaboration can help identify potential biases and ensure that the AI operates within ethical boundaries.
In conclusion, explaining AI decisions is fundamental to building trust and ensuring ethical practices in technology. By embracing transparency, employing explainable AI techniques, and engaging with stakeholders, we can create AI systems that not only perform effectively but also uphold the values of fairness and accountability. As we move forward in this rapidly evolving field, the emphasis on clear and understandable AI decision-making will only grow stronger.
Q1: Why is it important to explain AI decisions?
A: Explaining AI decisions builds trust among users and ensures that they understand how outcomes are determined, which is crucial for ethical governance.
Q2: What is explainable AI (XAI)?
A: Explainable AI refers to methods and techniques that make the operations of AI systems more interpretable and understandable to users.
Q3: How can developers ensure their AI explanations are clear?
A: Developers can use straightforward language, avoid technical jargon, and incorporate visual aids to make explanations accessible to all users.
Q4: What role do stakeholders play in AI decision-making?
A: Stakeholders provide valuable insights and perspectives that can help shape AI systems, ensuring they are fair, transparent, and aligned with ethical standards.
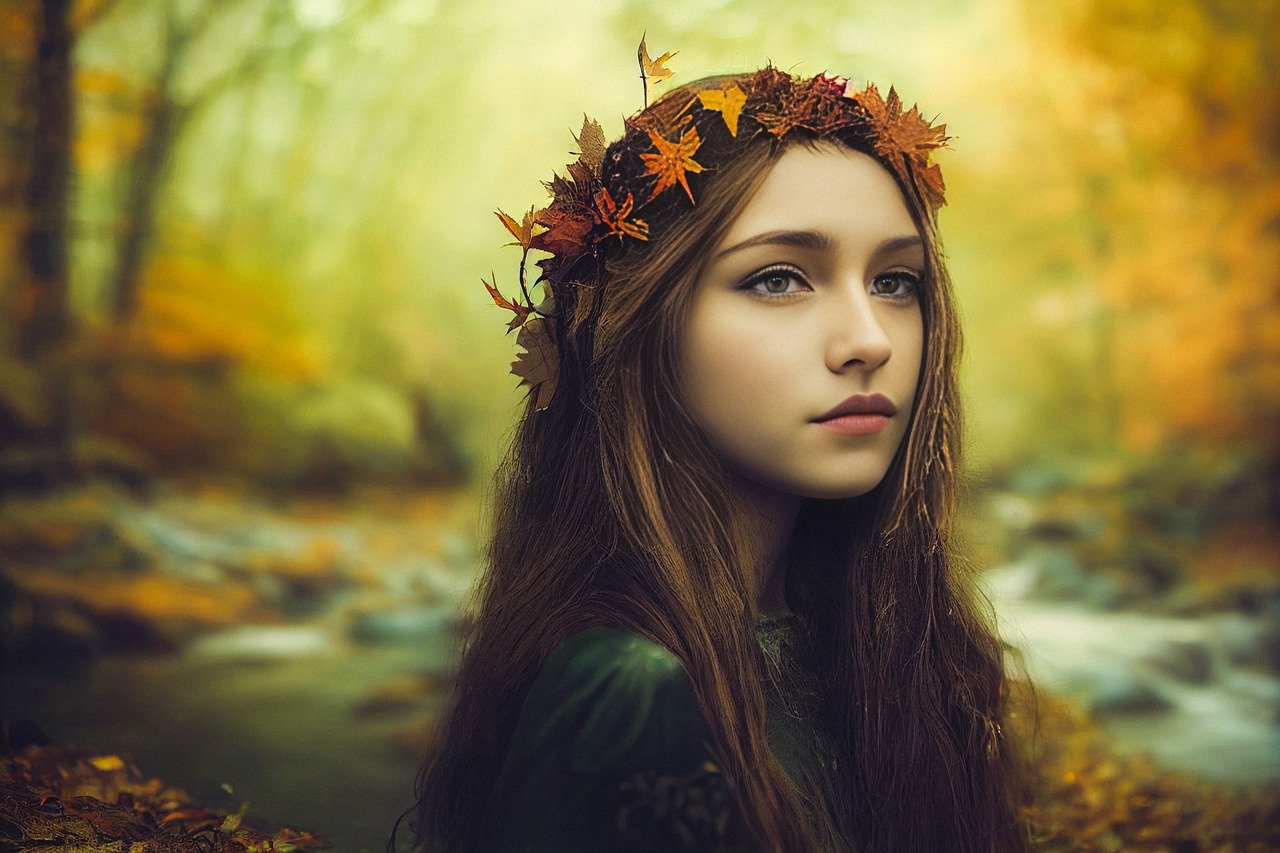
Regulatory Frameworks for AI
As artificial intelligence continues to evolve and integrate into various aspects of our daily lives, the need for robust regulatory frameworks becomes increasingly critical. These frameworks serve as the backbone for guiding the ethical deployment of AI technologies, ensuring they are utilized in ways that are not only effective but also morally sound. With the rapid pace of AI development, existing regulations often struggle to keep up, leading to a patchwork of rules that can vary significantly across different regions and industries.
Currently, several countries and organizations are working diligently to create comprehensive policies that address the unique challenges posed by AI. For instance, the European Union has proposed the AI Act, which aims to categorize AI systems based on their risk levels and impose stricter regulations on those deemed high-risk. This approach highlights the importance of proportionality in regulation—ensuring that the level of oversight corresponds to the potential risks associated with AI applications.
Moreover, regulatory frameworks should not only focus on risk management but also promote innovation. An overly rigid regulatory environment can stifle creativity and hinder technological advancements. Therefore, a balanced approach is necessary, one that encourages innovation while safeguarding public interests. This balance can be achieved through the following strategies:
- Stakeholder Engagement: Involving a diverse range of stakeholders—including technologists, ethicists, and the public—in the regulatory process can lead to more inclusive and effective policies.
- Adaptive Regulations: Regulations should be flexible enough to adapt to the fast-changing landscape of AI technology.
- International Cooperation: Given the global nature of AI, international collaboration is essential to create standards that can be universally applied.
In addition to these strategies, transparency in the regulatory process is paramount. Policymakers must clearly communicate the rationale behind regulations and the criteria used to assess AI technologies. This transparency fosters trust among users and developers, making it easier for everyone to understand their rights and responsibilities.
Furthermore, the establishment of independent oversight bodies can greatly enhance the effectiveness of regulatory frameworks. These bodies would be responsible for monitoring AI systems, ensuring compliance with regulations, and addressing any ethical concerns that arise. By having an impartial entity oversee AI deployment, we can better hold companies accountable for their actions and ensure that AI technologies are used responsibly.
Ultimately, the development of regulatory frameworks for AI is a complex but necessary endeavor. As we navigate this uncharted territory, it’s crucial to remain vigilant and proactive in our approach, ensuring that the benefits of AI are harnessed while minimizing potential harms. The future of AI regulation will not only shape the technology itself but also influence the very fabric of society, impacting privacy, human rights, and the overall quality of life.
- What are regulatory frameworks for AI? Regulatory frameworks for AI are sets of guidelines and policies designed to govern the development, deployment, and use of artificial intelligence technologies.
- Why are these frameworks important? They are important because they help to ensure that AI technologies are used ethically, protecting individuals' rights and promoting public trust.
- How can stakeholders be involved in creating these frameworks? Stakeholders can be involved through public consultations, workshops, and collaborative initiatives that allow for diverse perspectives to be considered.
- What role does international cooperation play in AI regulation? International cooperation is crucial for establishing consistent standards and practices that can be applied globally, reducing the risk of regulatory gaps.
Frequently Asked Questions
- What are the main ethical dilemmas associated with AI?
AI brings several ethical challenges to the forefront, including issues of bias, accountability, and transparency. These dilemmas can affect how AI systems are designed, implemented, and utilized in society, raising questions about fairness and ethical governance.
- How does bias manifest in AI algorithms?
Bias in AI can arise from various sources, such as data selection, algorithm design, and user interaction. When data used to train AI systems reflect societal biases, the outcomes can unintentionally discriminate against certain groups, leading to unfair treatment.
- What steps can be taken to mitigate bias in AI?
To reduce bias in AI, developers can implement diverse datasets, conduct regular audits, and engage in continuous monitoring. These strategies help ensure that AI systems produce fair and equitable results for all users.
- Who is accountable for decisions made by AI systems?
Establishing accountability in AI is crucial. Responsibility may fall on various stakeholders, including developers, companies, and even government regulators. Clear frameworks need to be in place to determine who is liable when AI systems make impactful decisions.
- Why is transparency important in AI systems?
Transparency builds trust among users by providing clear insights into how AI systems operate and make decisions. When users understand the processes behind AI, they are more likely to trust its outcomes and rely on its applications.
- How can AI decisions be effectively explained to users?
Effective communication of AI processes can be achieved through clear documentation, user-friendly interfaces, and explanatory models. These methods help users grasp the reasoning behind AI decisions, enhancing their overall experience.
- What regulatory frameworks currently exist for AI?
Various countries are developing regulatory frameworks to guide the ethical deployment of AI. These regulations aim to address issues of privacy, accountability, and bias, ensuring that AI technologies are used responsibly and ethically.