Role of AI in Detecting and Combating Fake News
In our fast-paced digital world, the spread of fake news has become a pressing issue that affects how we perceive reality. With the click of a button, misinformation can travel across the globe, influencing opinions, swaying elections, and even inciting violence. It’s like a wildfire, consuming everything in its path before anyone has a chance to extinguish it. Fortunately, we have a powerful ally in this battle: artificial intelligence (AI). This article explores how AI is revolutionizing the fight against misinformation, detailing its applications, benefits, challenges, and the future of digital information integrity.
Fake news refers to misinformation deliberately created to mislead readers. Its origins can be traced back to the dawn of media, but with the rise of social media platforms, it has proliferated like never before. The impact on society is profound, as individuals often fall prey to false narratives due to psychological factors such as confirmation bias and emotional appeal. Have you ever shared a post that sounded too outrageous to be true? Many people do, driven by the desire to belong or to feel informed. This section delves into the intricacies of fake news and its societal implications.
Artificial intelligence has evolved rapidly, providing tools that can analyze vast amounts of data. AI technology has become a game-changer in identifying patterns and detecting fake news effectively. Imagine having a super-intelligent assistant that can sift through millions of articles in seconds! With advancements in machine learning, natural language processing, and data mining techniques, AI is now equipped to tackle the complexities of misinformation.
Machine learning algorithms are essential for training AI systems to recognize fake news. These algorithms learn from data, improving over time in distinguishing between credible and false information. They operate like a student who learns from past mistakes; the more data they process, the better they become. For instance, an algorithm might analyze thousands of articles, identifying features that are common in fake news, such as sensationalist headlines or lack of credible sources. This continuous learning process allows AI to adapt and enhance its detection capabilities.
Natural language processing (NLP) allows AI to understand and interpret human language. It’s like teaching a computer to speak our language! NLP techniques are utilized to analyze the content of news articles for authenticity and reliability. By breaking down sentences, recognizing context, and even understanding sentiment, AI can evaluate whether an article is likely to be trustworthy. This technology is crucial in the fight against fake news, as it allows for a nuanced understanding of language and tone.
Data mining techniques enable AI to sift through large datasets to find trends and anomalies. Picture a detective piecing together clues from a mountain of evidence; that’s what AI does with data. These methods help identify sources of misinformation and track the spread of fake news across different platforms. By analyzing patterns in how information is shared, AI can pinpoint where misinformation is originating and how it evolves over time.
Various AI tools have been developed to combat fake news. Some of the most effective tools available today include:
- Fact-Checking Bots: These bots can quickly verify claims made in articles against a database of known facts.
- Content Analysis Tools: These analyze the language and structure of articles to assess their credibility.
- Social Media Monitoring Tools: These track the spread of information across social platforms to identify viral misinformation.
These tools work in tandem to create a robust defense against the onslaught of fake news, ensuring that readers have access to accurate information.
While AI presents significant advantages in combating fake news, challenges remain. Issues such as biases in algorithms, the evolving nature of misinformation, and the need for human oversight complicate the landscape. For instance, algorithmic bias can lead to inaccuracies in detecting fake news. If an AI system is trained on biased data, it may misidentify legitimate news as fake, or vice versa. This is a critical concern that researchers are actively working to address.
Algorithmic bias can lead to inaccuracies in detecting fake news. Biases are often introduced in AI systems through the data they are trained on. If the training data contains inaccuracies or reflects societal prejudices, the AI will likely perpetuate these issues. This can have serious implications for misinformation detection, as it may overlook legitimate news while flagging credible information as false.
Misinformation tactics continually evolve, posing challenges for AI. As new methods of spreading false information emerge, AI systems must adapt and improve. This is akin to a game of whack-a-mole; just when you think you’ve addressed one issue, another pops up. Continuous updates and refinements to AI algorithms are essential to keep pace with the changing landscape of misinformation.
As technology advances, the potential for AI in news verification grows. Future developments in AI capabilities could lead to more sophisticated tools that not only detect fake news but also educate the public on media literacy. Imagine a future where AI not only flags misinformation but also provides context and resources to help individuals critically evaluate the information they consume. This could significantly enhance public trust in information and empower individuals to become more discerning consumers of news.
- What is fake news? Fake news is misinformation deliberately created to mislead readers.
- How does AI detect fake news? AI uses machine learning algorithms, natural language processing, and data mining techniques to analyze content and identify patterns associated with misinformation.
- What are some challenges of using AI in combating fake news? Challenges include algorithmic bias, the evolving nature of misinformation, and the need for human oversight.
- What is the future of AI in news verification? The future may involve more sophisticated tools that not only detect misinformation but also enhance media literacy among the public.
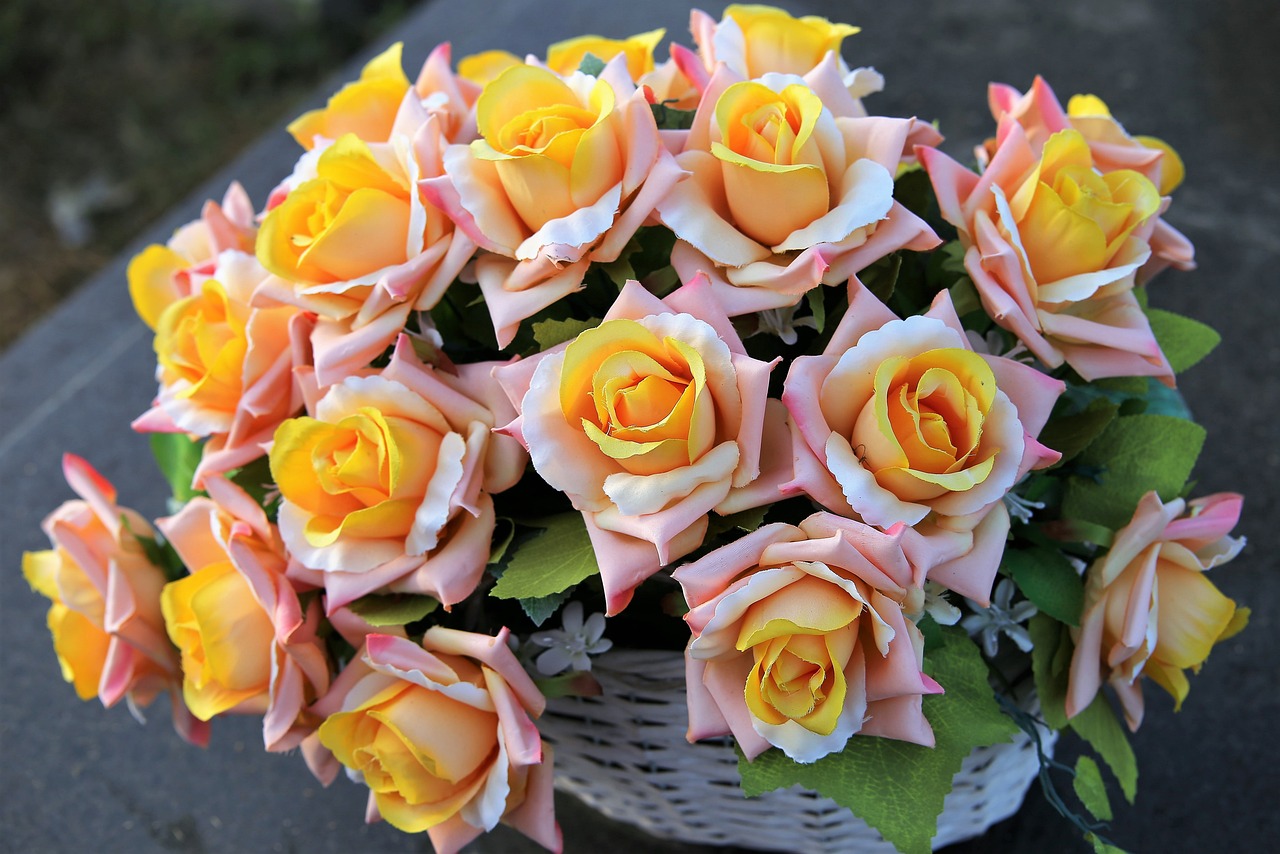
Understanding Fake News
Fake news is more than just a buzzword; it’s a phenomenon that has infiltrated our daily lives, shaping opinions and influencing decisions. At its core, fake news refers to misinformation that is deliberately fabricated to mislead readers. This could be anything from sensationalized headlines to completely fabricated stories that aim to deceive the public. The origins of fake news can be traced back to the early days of journalism, but with the advent of the internet and social media, its reach has expanded exponentially. Today, it’s easier than ever for false narratives to spread like wildfire.
The impact of fake news on society is profound. It can sway elections, incite violence, and create divisions among communities. People are often drawn to sensational stories that confirm their existing beliefs, a phenomenon known as confirmation bias. This psychological tendency makes individuals susceptible to believing false narratives, as they may not question the authenticity of the information presented. In fact, studies show that emotionally charged content is more likely to be shared, regardless of its truthfulness.
To further understand the implications of fake news, let’s consider some of the psychological factors at play:
- Emotional Appeal: Fake news often triggers strong emotional responses, making it more memorable and shareable.
- Social Validation: Individuals are more likely to believe and share news that aligns with their social circles, creating echo chambers.
- Cognitive Overload: In a world saturated with information, people may struggle to discern credible sources from unreliable ones.
Moreover, the rapid spread of fake news can lead to real-world consequences. For instance, misinformation about health-related topics can cause individuals to make harmful decisions, such as avoiding vaccines or ignoring medical advice. This highlights the urgent need for effective strategies to combat the proliferation of fake news. The challenge lies not only in identifying false information but also in fostering a more informed public that can critically assess the news they consume.
As we navigate this complex landscape, it’s crucial to understand that the fight against fake news is not solely the responsibility of technology. While AI and other tools can help detect and combat misinformation, media literacy plays a vital role. Educating individuals on how to evaluate sources, recognize bias, and think critically about the information they encounter is essential in building resilience against fake news.
In summary, understanding fake news requires a multifaceted approach that considers its origins, societal impact, and the psychological factors that contribute to its spread. By acknowledging these elements, we can better equip ourselves to navigate the murky waters of misinformation and advocate for a more truthful discourse in our digital age.
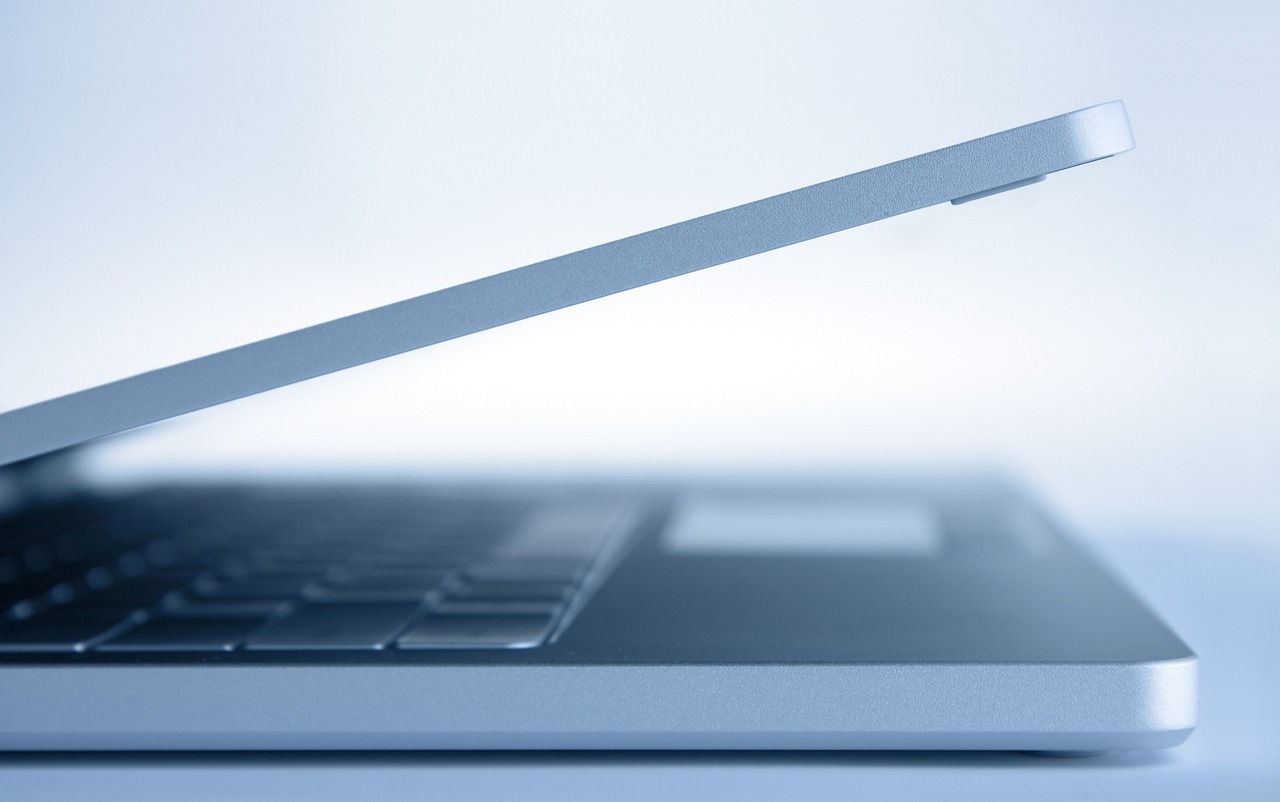
The Rise of AI Technology
Artificial Intelligence (AI) has seen an unprecedented surge in development over the past decade, fundamentally changing how we interact with technology and information. Imagine having a digital assistant that not only understands your commands but also learns from your behavior, adapting to your preferences over time. This is the essence of AI technology, and its rise has been nothing short of revolutionary. With advancements in computational power, data storage, and sophisticated algorithms, AI is now capable of analyzing vast amounts of data at lightning speed, making it an invaluable tool in the fight against fake news.
At its core, the rise of AI technology is driven by the need to process and interpret the overwhelming volume of information available online. Each day, millions of articles, posts, and videos are shared across social media platforms, making it incredibly challenging for individuals to discern which sources are credible. This is where AI steps in, utilizing advanced analytical techniques to sift through this digital noise. By employing methods such as machine learning and natural language processing, AI systems can identify patterns and anomalies that may indicate misinformation.
One of the most exciting aspects of AI's rise is its ability to learn and adapt. Initially, AI systems were programmed with specific rules to identify fake news; however, as these systems are exposed to more data, they evolve. This evolution is largely due to machine learning algorithms, which allow AI to improve its accuracy over time. These algorithms are designed to recognize features of credible versus non-credible news, such as linguistic cues, source reliability, and even the sentiment behind the content. The more data these systems analyze, the better they become at spotting misinformation.
Moreover, the integration of natural language processing (NLP) has further enhanced AI's capabilities. NLP enables AI to understand the nuances of human language, including idioms, sarcasm, and context. This is crucial when evaluating the authenticity of news articles, as misleading headlines or emotionally charged language can often obscure the truth. By analyzing the structure and semantics of the text, AI can provide insights into the reliability of the information presented.
In addition to NLP, data mining techniques play a pivotal role in AI's ability to combat fake news. These techniques allow AI to explore vast datasets, uncovering trends, relationships, and anomalies that may not be immediately apparent. For instance, AI can track the spread of a particular piece of misinformation across social media platforms, identifying how quickly it is shared and by whom. This capability is vital for understanding the lifecycle of fake news and developing strategies to counteract it.
As we look toward the future, it is clear that the rise of AI technology is not just a passing trend; it represents a fundamental shift in how we consume and verify information. With ongoing advancements, we can expect AI tools to become even more sophisticated, helping to safeguard the integrity of digital information. But the journey doesn't end here. The challenges that come with AI implementation, such as algorithmic bias and the ever-evolving tactics of misinformation, will require continuous innovation and vigilance.
In summary, the rise of AI technology is a game-changer in the battle against fake news. By harnessing the power of machine learning, natural language processing, and data mining, AI is equipping us with the tools necessary to navigate the complex landscape of information in the digital age. As we continue to explore these technologies, we must also remain aware of their limitations and the ethical implications they carry. The future of information integrity depends not only on technological advancements but also on our collective commitment to fostering a well-informed society.
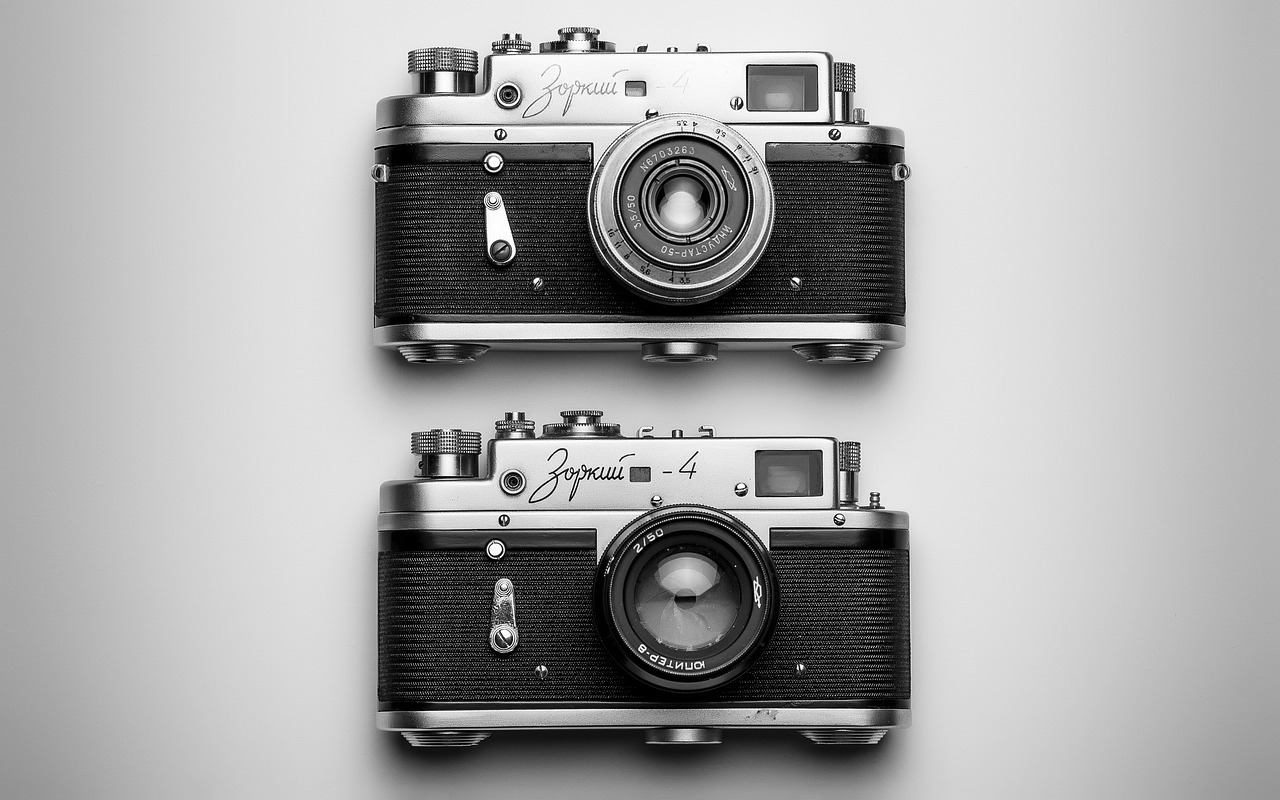
Machine Learning Algorithms
Machine learning algorithms are the backbone of artificial intelligence systems designed to combat fake news. These algorithms are not just simple programs; they are sophisticated models that learn from data over time. Imagine teaching a child to recognize different animals by showing them pictures and explaining the characteristics of each. Similarly, machine learning algorithms learn to identify patterns in data that distinguish credible news from misinformation. They analyze various features, such as the source of the news, the language used, and even the sentiment expressed within the article.
One of the most fascinating aspects of these algorithms is their ability to adapt and improve. Initially, they might struggle to differentiate between a legitimate news piece and a fabricated one. However, as they process more data and receive feedback, they refine their understanding. This iterative learning process is akin to a chef perfecting a recipe through trial and error, gradually enhancing their skills to create the perfect dish.
To effectively combat fake news, machine learning algorithms rely on several techniques, including:
- Supervised Learning: This involves training the algorithm on a labeled dataset where the outcomes are known. For example, the algorithm learns from a collection of articles that have been pre-identified as true or false.
- Unsupervised Learning: In this approach, the algorithm identifies patterns and groupings in data without prior labels. This can help discover new types of misinformation that haven't been previously categorized.
- Reinforcement Learning: Here, the algorithm learns by receiving rewards or penalties based on its predictions. This method encourages the algorithm to improve its accuracy over time.
Moreover, the effectiveness of these algorithms is heavily influenced by the quality and diversity of the training data. If the dataset is biased or lacks representation, the algorithm may develop skewed perspectives, leading to incorrect classifications. Therefore, it is crucial for developers to ensure that the data used for training is comprehensive and reflective of various viewpoints.
In summary, machine learning algorithms are instrumental in the fight against fake news. They continuously evolve through exposure to new information, enhancing their ability to detect false narratives. As these algorithms become more refined, they hold the promise of significantly improving our ability to sift through the vast amounts of information available today, ultimately fostering a more informed society.
- What are machine learning algorithms? Machine learning algorithms are computational models that learn from data to make predictions or decisions without being explicitly programmed for each task.
- How do these algorithms combat fake news? They analyze various features of news articles, such as language and source credibility, to determine whether the information is reliable or misleading.
- Can machine learning algorithms improve over time? Yes, these algorithms learn from new data and feedback, allowing them to adapt and enhance their accuracy in detecting misinformation.
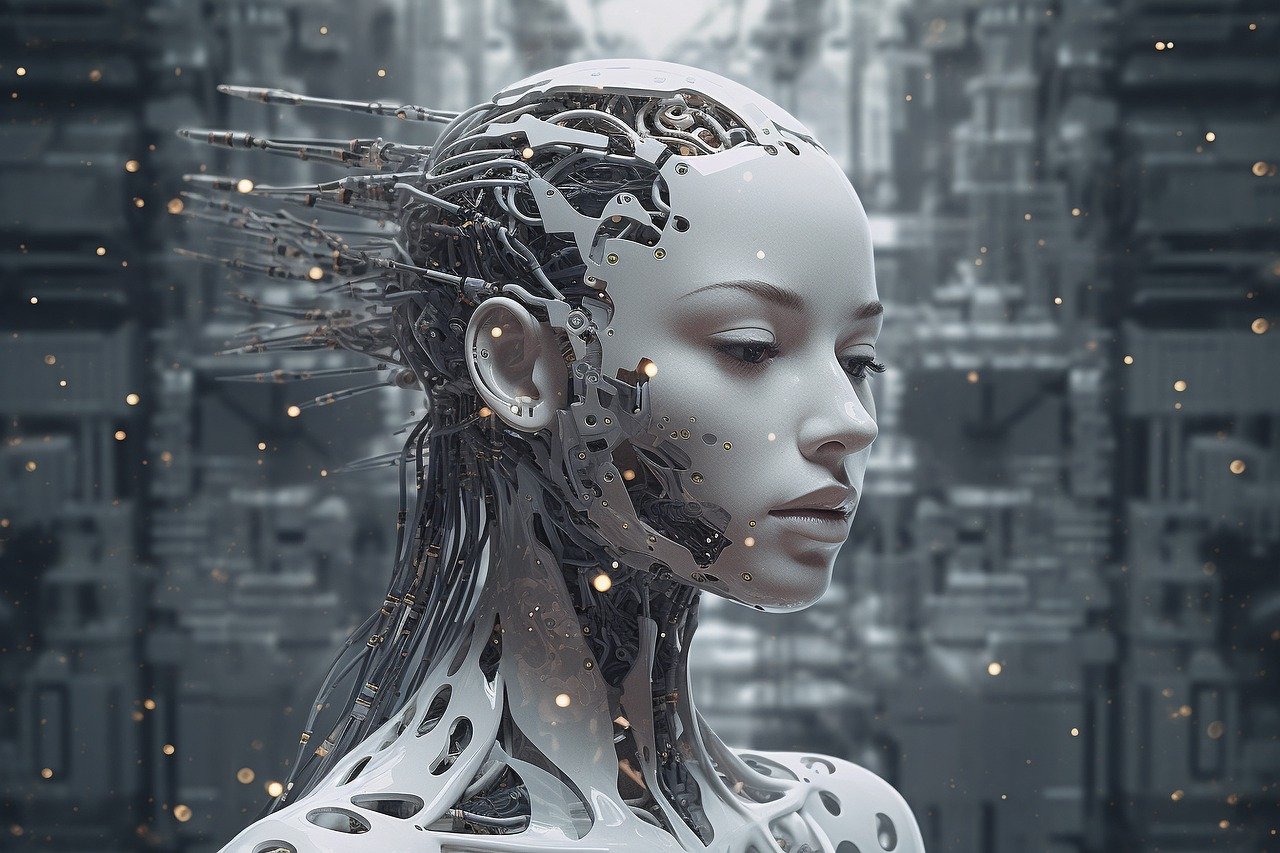
Natural Language Processing
Natural Language Processing (NLP) is a groundbreaking technology that allows artificial intelligence to understand and interpret human language in a way that is both meaningful and contextually relevant. Imagine trying to teach a computer to read and comprehend news articles just like a human would; that’s where NLP comes into play. It acts as a bridge between human communication and machine understanding, enabling AI to analyze the content of news articles for authenticity and reliability.
NLP employs various techniques to dissect the nuances of language, including syntax, semantics, and sentiment analysis. These techniques empower AI systems to not just read words but to grasp the underlying meaning and intent behind them. For instance, when evaluating a news article, NLP can identify whether the tone is neutral, biased, or inflammatory. This capability is crucial in the fight against fake news, as it helps determine if a piece of information is presented in a misleading manner.
One of the most fascinating aspects of NLP is its ability to analyze vast amounts of text quickly. Traditional methods of fact-checking can be time-consuming and labor-intensive, often requiring human intervention. However, with NLP, AI can scan thousands of articles in mere seconds, flagging potential misinformation based on predefined criteria. This rapid analysis not only saves time but also increases the chances of catching fake news before it spreads further.
Moreover, NLP can identify patterns in language that may indicate the presence of fake news. For example, specific phrases or word choices can be telltale signs of sensationalism or bias. By training AI systems to recognize these patterns, we can enhance the accuracy of fake news detection. Here’s a quick breakdown of how NLP contributes to combating misinformation:
- Content Analysis: Evaluates the actual words and phrases used in articles.
- Sentiment Detection: Determines the emotional tone of the content.
- Contextual Understanding: Grasp the broader context in which information is presented.
In conclusion, Natural Language Processing is a vital component in the ongoing battle against fake news. By equipping AI with the ability to understand human language, we are not just improving the technology but also enhancing our collective ability to discern fact from fiction. As NLP continues to evolve, its role in news verification will undoubtedly become even more critical, paving the way for a more informed society.
- What is Natural Language Processing?
NLP is a technology that allows computers to understand and interpret human language, enabling better analysis of text data.
- How does NLP help in detecting fake news?
NLP analyzes the content, sentiment, and context of news articles to identify potential misinformation quickly and accurately.
- Are there limitations to NLP in fake news detection?
Yes, while NLP is powerful, it can struggle with nuances, sarcasm, or newly emerging language patterns, necessitating ongoing improvements.
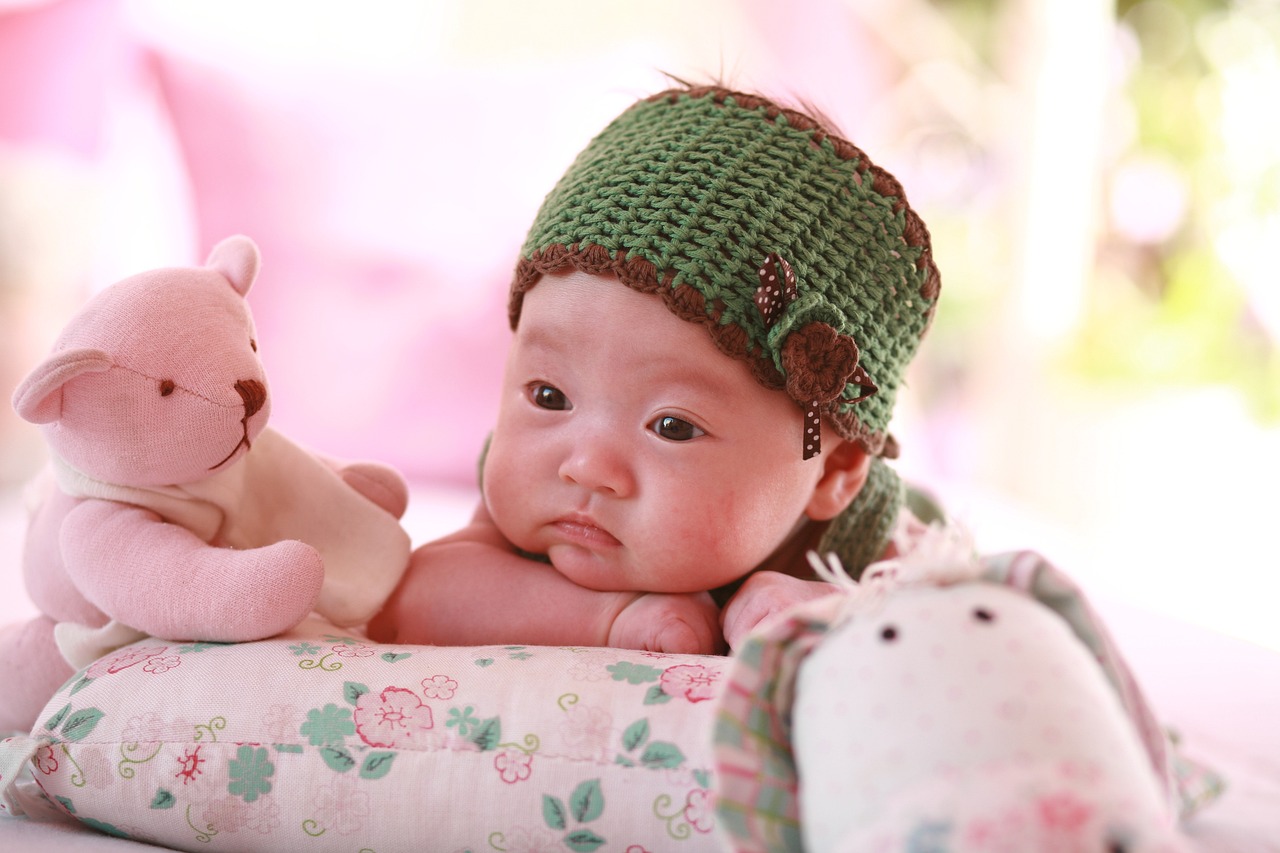
Data Mining Techniques
Data mining techniques play a crucial role in the fight against fake news by allowing artificial intelligence systems to sift through vast amounts of information and identify patterns that may indicate misinformation. Imagine trying to find a needle in a haystack; that’s essentially what data mining does, but instead of a needle, it’s looking for false narratives hidden within an ocean of data. With the sheer volume of news articles published daily, traditional methods of fact-checking simply can’t keep up. This is where data mining comes in, employing algorithms to analyze data sets and extract valuable insights.
One of the most effective methods used in data mining is clustering. This technique groups similar data points together, making it easier for AI systems to identify anomalies that may suggest the presence of fake news. For example, if a particular news story is being shared widely but lacks credible sources, clustering can help flag it for further investigation. Another important technique is association rule learning, which helps uncover relationships between different pieces of information. By analyzing how often certain words or phrases appear together in a dataset, AI can determine the likelihood of a news article being credible or misleading.
Furthermore, data mining techniques can be enhanced through the use of sentiment analysis. This involves examining the emotional tone behind a series of words, which can be particularly useful in identifying sensationalist headlines designed to provoke strong reactions. By analyzing the sentiment of articles, AI can differentiate between objective reporting and emotionally charged content that may be misleading. In addition, trend analysis allows AI systems to track how misinformation spreads over time, identifying common sources and methods used to disseminate false information.
To illustrate the impact of data mining techniques in combating fake news, consider the following table that summarizes key techniques and their functionalities:
Data Mining Technique | Description |
---|---|
Clustering | Groups similar data points to identify anomalies. |
Association Rule Learning | Uncovers relationships between different pieces of information. |
Sentiment Analysis | Analyzes emotional tone to identify sensationalist content. |
Trend Analysis | Tracks the spread of misinformation over time. |
As we continue to refine these data mining techniques, the potential for AI to combat fake news becomes even more promising. By harnessing the power of data, we can not only identify misinformation more effectively but also understand the underlying factors that contribute to its spread. This knowledge is crucial in the ongoing battle for digital information integrity, as it empowers both AI systems and human users to make informed decisions about the news they consume.
- What is data mining? Data mining is the process of discovering patterns and knowledge from large amounts of data, often used to identify trends and anomalies.
- How does AI use data mining to detect fake news? AI employs data mining techniques to analyze vast datasets, identify patterns, and flag potential misinformation.
- What are some common data mining techniques? Common techniques include clustering, association rule learning, sentiment analysis, and trend analysis.
- Can data mining completely eliminate fake news? While data mining significantly aids in detection, it cannot completely eliminate fake news, as misinformation tactics constantly evolve.
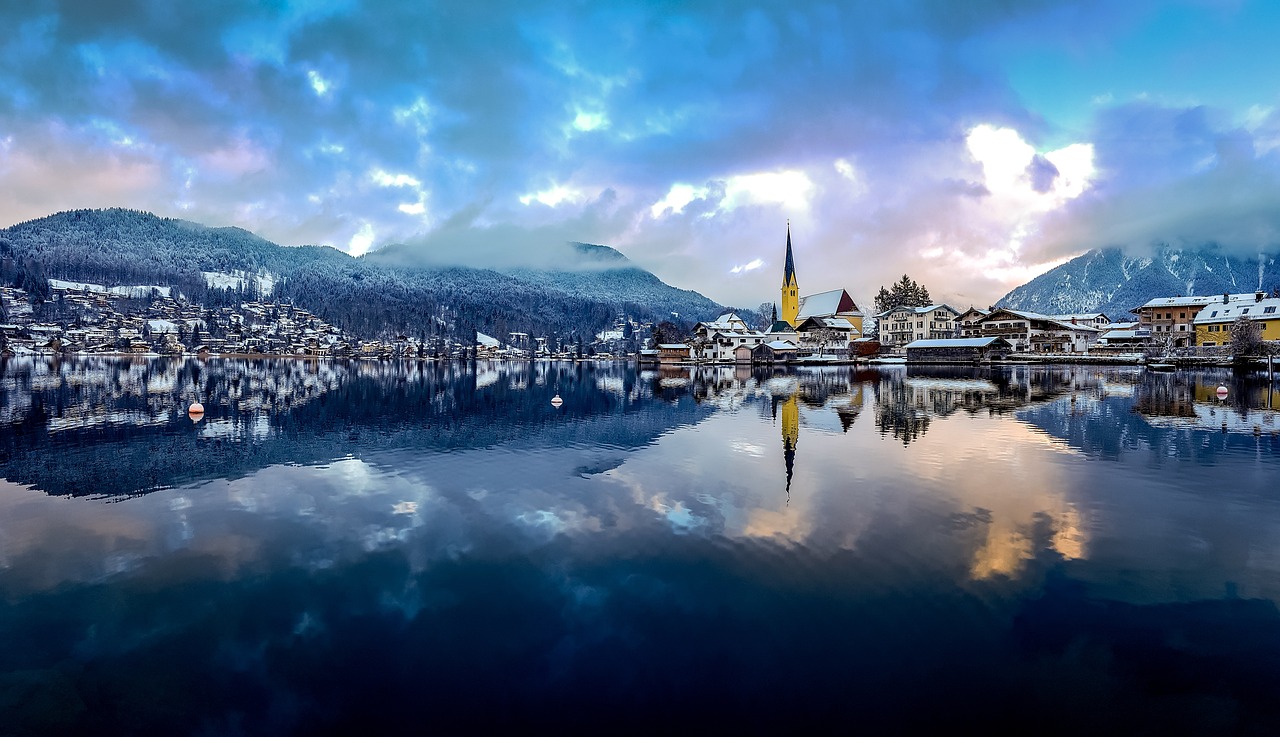
AI Tools for Fake News Detection
In the digital age, where information travels faster than light, the rise of artificial intelligence (AI) tools has become a beacon of hope in the battle against fake news. These tools are designed to analyze content, assess credibility, and flag potential misinformation before it spreads further. Imagine having a digital watchdog that tirelessly scans the internet, ensuring that what you read is not just a figment of someone’s imagination. It’s like having a personal assistant who filters out the noise, leaving you with only the most reliable information.
One of the most notable tools in this realm is FactCheck.org, which utilizes AI algorithms to cross-reference news articles with verified facts. This platform not only checks the authenticity of claims but also provides sources that back up its findings. Another powerful tool is Snopes, a well-known fact-checking website that has integrated machine learning to enhance its detection capabilities. By analyzing patterns in user-submitted stories, Snopes can quickly determine the likelihood of a story being true or false.
Moreover, Google's Fact Check Explorer allows users to search for fact-checked claims across various sources, making it easier to verify information. It’s like having a library of truth at your fingertips, where you can instantly check the veracity of a statement. These tools leverage advanced machine learning algorithms and natural language processing (NLP) techniques to sift through mountains of data, identifying trends and discrepancies that may indicate misinformation.
To give you a clearer picture, here’s a quick overview of some prominent AI tools for fake news detection:
Tool Name | Functionality |
---|---|
FactCheck.org | Cross-references news articles with verified facts. |
Snopes | Uses machine learning to evaluate user-submitted stories for authenticity. |
Google Fact Check Explorer | Allows users to search for fact-checked claims across sources. |
Hoaxy | Visualizes the spread of misinformation and fact-checking efforts. |
These tools are not just reactive; they are proactive in their approach. For instance, Hoaxy visualizes the spread of misinformation and the corresponding fact-checking efforts, giving users a comprehensive view of how false narratives propagate through social media channels. It’s like watching a map light up with misinformation hotspots, allowing users and researchers to understand the dynamics of fake news dissemination.
However, while these AI tools are incredibly powerful, they are not infallible. The effectiveness of these systems largely depends on the quality of the data they are trained on. As with any technology, the potential for error exists, and this is where human oversight plays a crucial role. Users must remain vigilant and not solely rely on AI tools; instead, they should use them as part of a broader strategy for discerning credible information.
In conclusion, AI tools for fake news detection are transforming the way we consume information. They empower individuals to make informed decisions and encourage a culture of skepticism towards sensational claims. As these technologies continue to evolve, we can look forward to a future where misinformation is swiftly identified and addressed, paving the way for a more informed society.
- What is fake news? Fake news refers to misinformation that is deliberately created to mislead readers.
- How does AI detect fake news? AI uses machine learning algorithms and natural language processing to analyze content for authenticity.
- Are AI tools completely reliable? While AI tools are powerful, they are not infallible and should be used alongside human judgment.
- Can I use AI tools to verify news? Yes, tools like FactCheck.org and Snopes can help verify the authenticity of news articles.
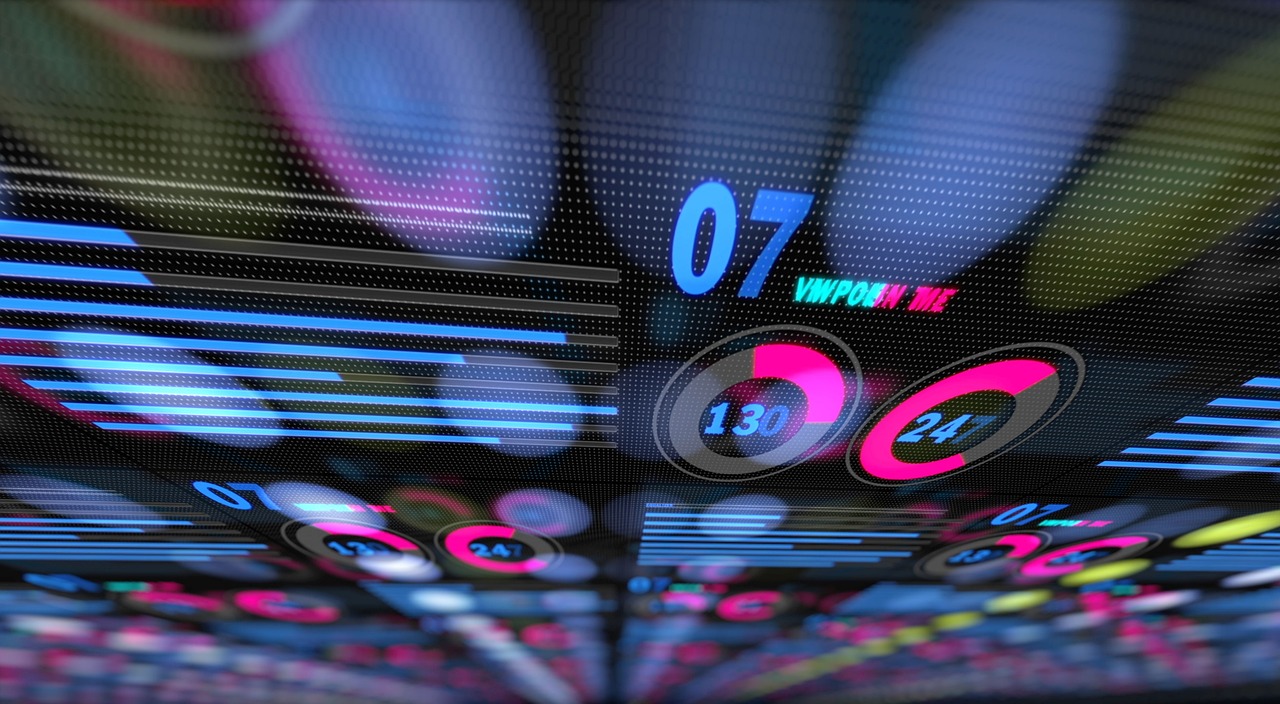
Challenges in AI Implementation
The integration of artificial intelligence (AI) in combating fake news is undoubtedly a game changer, but it doesn't come without its hurdles. One of the most pressing challenges is algorithmic bias. Just like a chef who uses the same spices every time, AI algorithms can develop a taste for certain types of data. If the training data is skewed or unrepresentative, the AI may end up favoring specific narratives while ignoring others, leading to inaccuracies in detecting misinformation. This bias can manifest in various ways, such as favoring certain news sources or misclassifying credible information as fake.
Moreover, the rapidly evolving nature of misinformation tactics poses an ongoing challenge for AI systems. Just when AI developers think they've cracked the code, new methods of spreading false information emerge. For instance, the rise of deepfakes and sophisticated social media bots complicates the landscape. These tools make it increasingly difficult for AI to keep pace, as they can mimic real news articles or social media posts so convincingly that even human fact-checkers can struggle to identify them. Therefore, AI systems must be designed with adaptability in mind, constantly learning and updating their algorithms to recognize these new tactics.
Another significant issue is the need for human oversight. While AI can analyze vast amounts of data at lightning speed, it lacks the nuanced understanding that humans possess. This gap in understanding can lead to errors in judgment about what constitutes credible information. Therefore, a collaborative approach, where AI tools assist human fact-checkers rather than replace them, is essential. This partnership can enhance the accuracy of misinformation detection while ensuring that ethical considerations are taken into account.
Additionally, the transparency of AI processes is crucial. Users need to understand how AI arrives at its conclusions. If the algorithms are treated like a black box, it can erode public trust in the technology. Transparency helps build confidence in AI systems, allowing users to see the rationale behind a detection or classification. This understanding is vital, especially in a world where misinformation can have real-world consequences, from influencing elections to inciting violence.
Lastly, the implementation of AI solutions can be resource-intensive. Developing sophisticated AI models requires significant investment in both time and money. Smaller organizations or independent fact-checkers might struggle to access the necessary technology or expertise, creating a disparity in the ability to combat misinformation effectively. A collaborative ecosystem that allows for resource sharing and knowledge transfer can help mitigate this challenge, ensuring that AI tools are accessible to all who need them.
In summary, while AI holds incredible promise in the fight against fake news, it is essential to navigate the challenges it presents. From addressing algorithmic bias to ensuring human oversight and transparency, these considerations will shape the future effectiveness of AI in maintaining the integrity of information in our digital world.
- What is algorithmic bias? Algorithmic bias occurs when an AI system produces results that are systematically prejudiced due to erroneous assumptions in the machine learning process.
- Why is human oversight necessary in AI? Human oversight is crucial because AI lacks the nuanced understanding required to make complex ethical judgments, which can lead to misclassifications.
- How can AI adapt to new misinformation tactics? AI can adapt through continuous learning, where it updates its algorithms based on new data and emerging misinformation strategies.
- What role does transparency play in AI? Transparency helps build trust, allowing users to understand how AI systems make decisions and ensuring accountability.
- Is AI expensive to implement? Yes, developing and deploying AI systems can be resource-intensive, which may pose challenges for smaller organizations.
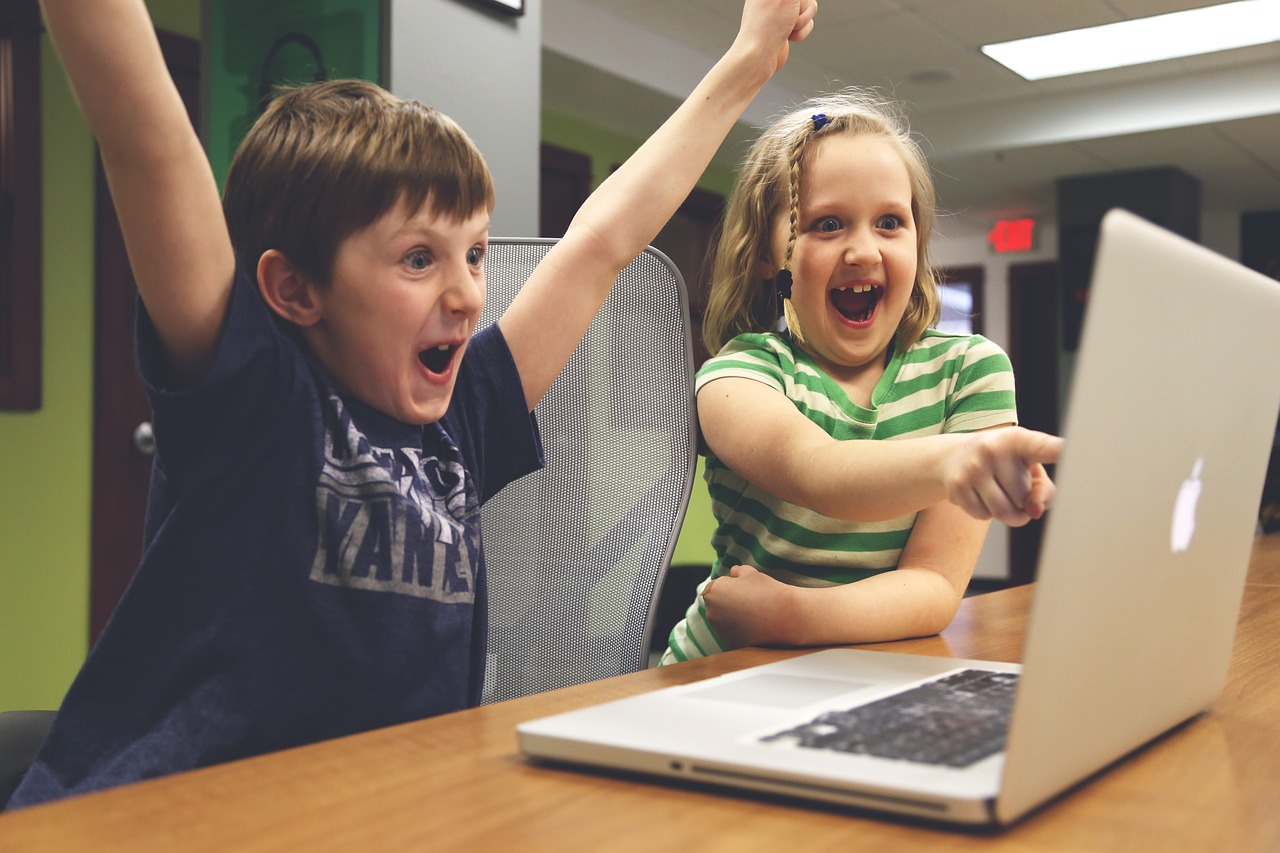
Algorithmic Bias
Algorithmic bias is a significant concern in the realm of artificial intelligence, especially when it comes to detecting and combating fake news. But what exactly does this mean? In simple terms, algorithmic bias occurs when an AI system produces results that are systematically prejudiced due to erroneous assumptions in the machine learning process. This can lead to a skewed perception of what constitutes fake news and, ultimately, affect the integrity of information that users receive.
To understand how algorithmic bias manifests, consider the data that feeds these AI systems. If the training data is not diverse or is inherently biased, the AI will learn from these flawed examples. For instance, if an AI system is trained predominantly on news articles from certain regions or political viewpoints, it might struggle to accurately assess content from other perspectives. This lack of representation can create a feedback loop where the AI consistently misidentifies credible information as fake, or vice versa, thereby amplifying misinformation rather than mitigating it.
Moreover, the implications of algorithmic bias extend beyond mere inaccuracies. They can foster distrust among users, particularly if people feel that the AI is unfairly targeting specific groups or viewpoints. This is critical because, in an age where public trust in media is waning, biased algorithms can exacerbate the divide between different segments of society. To illustrate this, let’s look at a few common sources of algorithmic bias:
- Data Selection Bias: When the data used to train AI lacks variety, it can lead to a narrow understanding of what constitutes credible news.
- Labeling Bias: Human annotators may unintentionally introduce their biases when labeling data, affecting the learning process of the AI.
- Feedback Loop Bias: If users interact with biased outputs, it can reinforce the algorithm's existing biases, creating a cycle that is hard to break.
Addressing algorithmic bias requires a multi-faceted approach. Developers must prioritize diverse data sets and implement rigorous testing to identify and correct biases before deployment. Additionally, transparency in the AI development process can help build trust among users, as they will be more aware of how decisions are made and the potential limitations of the technology.
In conclusion, while AI holds tremendous potential for combating fake news, algorithmic bias poses a significant challenge that cannot be overlooked. By acknowledging and addressing these biases, we can work towards creating more reliable AI systems that enhance our ability to discern truth from misinformation.
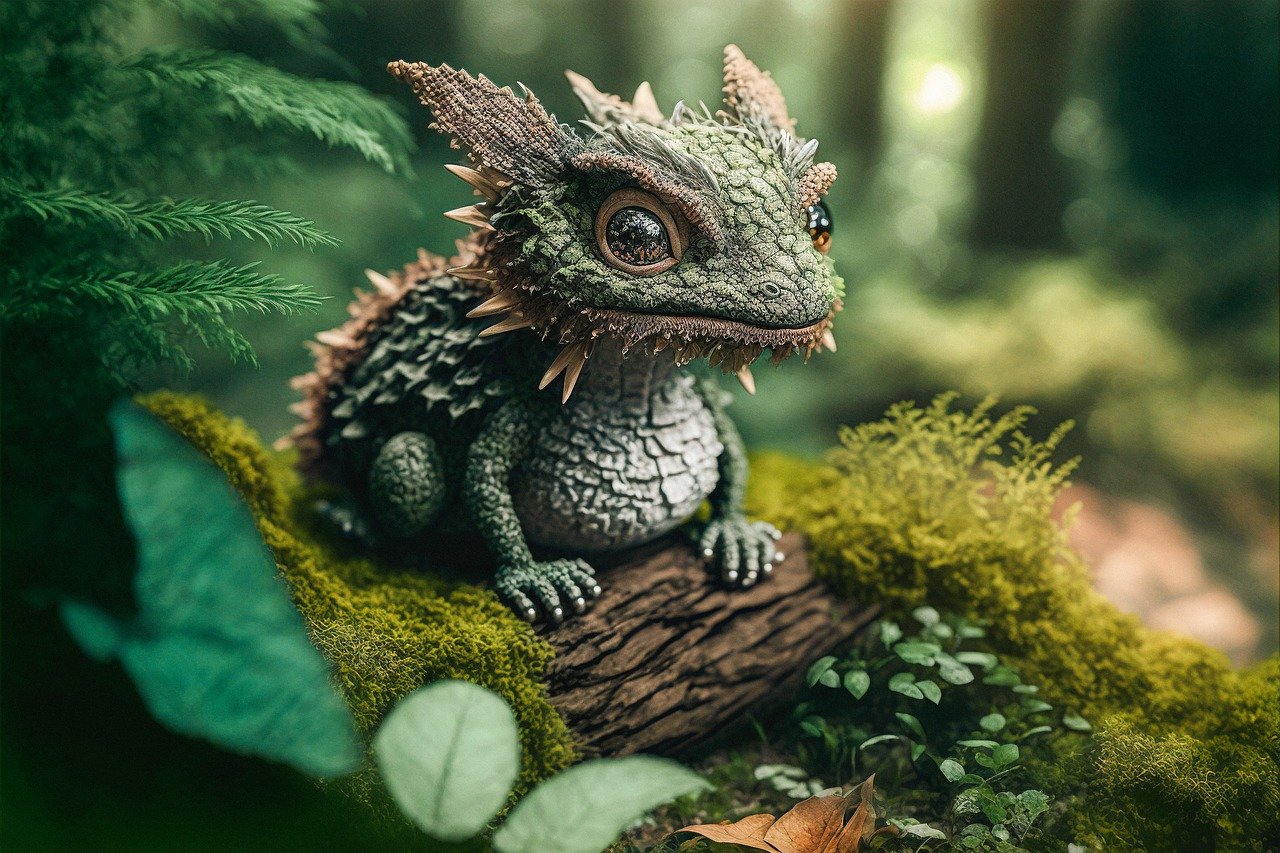
Adapting to New Misinformation Tactics
In the ever-evolving landscape of digital information, the tactics used to spread misinformation are constantly changing. Just as a chameleon adapts to its surroundings, misinformation strategies morph and evolve, making it crucial for artificial intelligence (AI) systems to keep pace. One of the most significant challenges AI faces is the ability to recognize and adapt to these new tactics swiftly. For instance, the rise of deepfakes—realistic but fabricated videos—has introduced a new level of complexity in identifying false narratives. These advanced techniques can easily mislead viewers, creating a pressing need for AI to develop more sophisticated detection methods.
Moreover, the speed at which misinformation spreads through social media platforms exacerbates the problem. Information can go viral within hours, leaving little time for traditional fact-checking methods to catch up. AI must be capable of real-time analysis and response, which requires not only advanced algorithms but also a nuanced understanding of context. This means that AI needs to be trained not just on static datasets but also on dynamic, continuously updated sources of information.
To effectively combat these new tactics, AI systems must employ a variety of strategies:
- Continuous Learning: AI should be designed to learn from new data and adapt its algorithms accordingly. This involves feeding the system with fresh information about emerging misinformation tactics.
- Collaboration with Human Experts: While AI can process vast amounts of data, human oversight is essential. Collaboration between AI systems and human fact-checkers can enhance accuracy in identifying new misinformation methods.
- Utilizing User Feedback: Engaging users in the detection process can provide valuable insights. When users flag suspicious content, AI can analyze these flags to improve its detection algorithms.
Additionally, the integration of cross-platform analysis is crucial. Misinformation often spreads across multiple platforms, and understanding the interconnectedness of these platforms can help AI systems identify patterns and sources of misinformation more effectively. For example, if a false narrative begins on one platform but is shared on several others, AI must be able to trace this journey to understand its impact and origins.
In conclusion, as misinformation tactics become increasingly sophisticated, AI must evolve in tandem. By embracing continuous learning, human collaboration, user engagement, and cross-platform analysis, AI systems can enhance their effectiveness in detecting and combating misinformation. The battle against fake news is far from over, but with the right adaptations, AI can play a pivotal role in restoring trust in digital information.
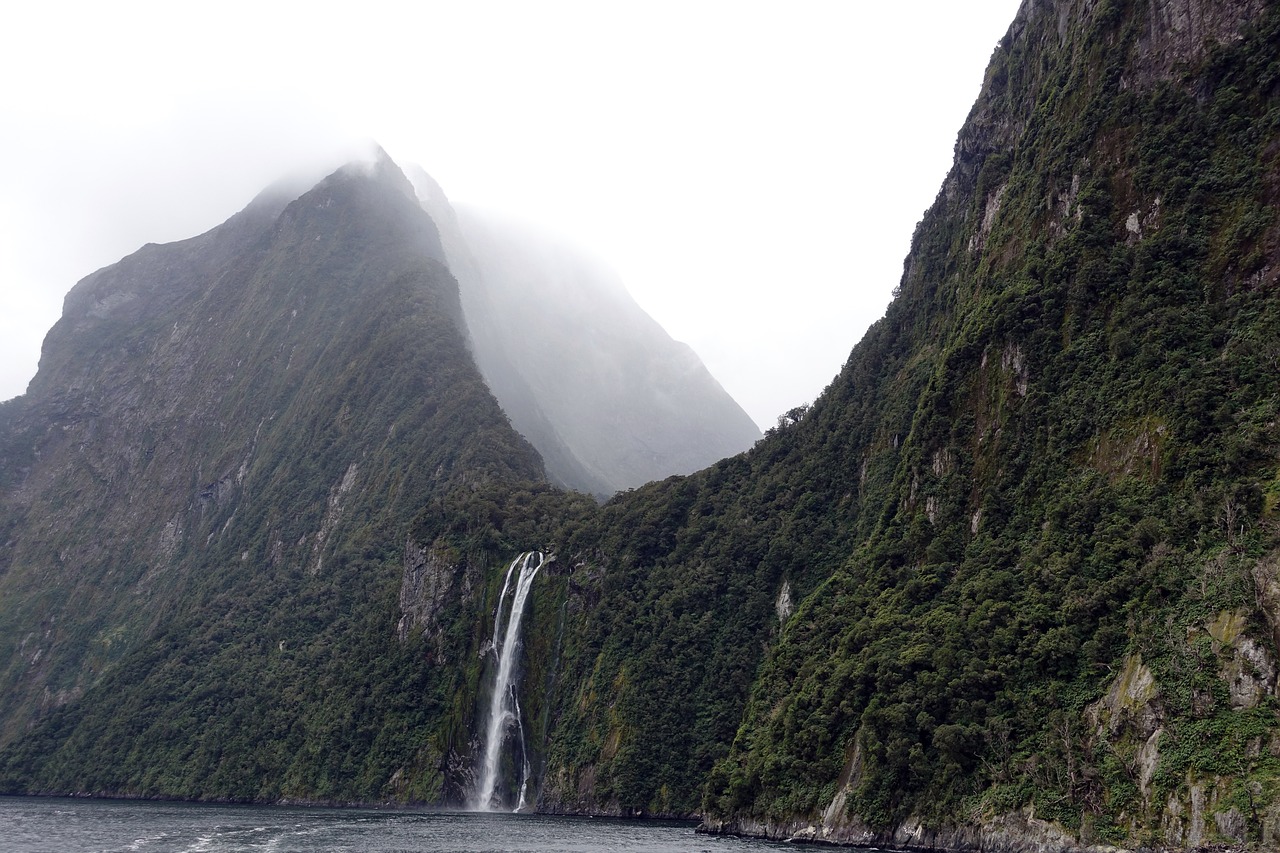
The Future of AI in News Verification
The future of artificial intelligence in news verification is not just promising; it's downright exciting! As we continue to grapple with the overwhelming tide of information flooding our digital spaces, AI stands as a beacon of hope in the battle against misinformation. Imagine a world where your news feed is curated not just by algorithms that prioritize engagement but also by intelligent systems that prioritize truth and accuracy. Sounds like a dream, right? Well, it’s becoming a reality!
One of the most significant advancements we can expect is the enhancement of natural language processing (NLP). As AI systems become more adept at understanding context and nuance, they will be able to detect subtle signs of misinformation that current technologies might miss. For instance, future AI could analyze not just the words in an article but also the emotional tone and intent behind them, providing a deeper understanding of the content. This capability could be instrumental in identifying not only fake news but also sensationalism that often skews public perception.
Moreover, as AI technologies evolve, we can anticipate the development of more interactive tools for users. Picture this: you read an article and, with a simple click, an AI-powered browser extension provides you with an instant analysis of the article's credibility, highlighting potential biases, sourcing issues, and even contrasting viewpoints. Such tools could empower users to become more discerning consumers of information, fostering a culture of critical thinking and media literacy.
Another exciting prospect is the integration of AI with blockchain technology. Blockchain offers a decentralized ledger that can verify sources and track the origin of news articles. By combining AI’s analytical capabilities with blockchain’s transparency, we could create a robust system for verifying the authenticity of news. This would not only help in identifying fake news but also in building trust in legitimate sources. Imagine being able to trace an article back to its original source, ensuring that what you read is not just a retelling, but an accurate representation of the facts.
However, it's essential to recognize that the future of AI in news verification will also demand a collaborative effort. AI alone cannot solve the problem of misinformation. It will require partnerships between tech companies, news organizations, and educational institutions to develop comprehensive strategies that address the multifaceted nature of fake news. This collaboration could lead to the creation of standardized protocols for news verification, making it easier for AI systems to operate effectively across different platforms.
In summary, the future of AI in news verification is bright, filled with potential innovations that can transform how we consume and trust information. As we look ahead, it’s crucial to remain vigilant and proactive in ensuring that these technologies are developed responsibly and ethically. The stakes are high, and the responsibility lies not just with technologists but with all of us as consumers of information.
- How can AI improve news verification?
AI can analyze vast amounts of data, detect patterns, and identify misinformation through advanced algorithms and natural language processing. - What role does NLP play in AI news verification?
NLP allows AI to understand and interpret human language, making it possible to analyze the content of news articles for authenticity. - Can AI completely eliminate fake news?
While AI can significantly reduce the spread of fake news, it cannot completely eliminate it. Human oversight and critical thinking remain essential. - How can users leverage AI tools for better news consumption?
Users can utilize AI-powered browser extensions and applications that provide real-time analysis of news credibility and highlight potential biases.
Frequently Asked Questions
- What is fake news?
Fake news refers to deliberately misleading information presented as news. It can take various forms, including fabricated stories, misleading headlines, and manipulated images, all aimed at influencing public opinion or generating clicks.
- How does AI help in detecting fake news?
AI utilizes advanced technologies like machine learning and natural language processing to analyze large volumes of data. By identifying patterns and inconsistencies in news articles, AI can flag potential fake news for further investigation.
- What are machine learning algorithms?
Machine learning algorithms are a set of techniques that enable AI systems to learn from data. They improve over time by recognizing the differences between credible and false information, making them essential for effective fake news detection.
- What role does natural language processing play in this context?
Natural language processing (NLP) allows AI to understand human language. By analyzing the text of news articles, NLP techniques help determine the authenticity and reliability of the content, which is crucial for identifying misinformation.
- Are there any challenges in using AI to combat fake news?
Yes, there are several challenges, including algorithmic bias, which can lead to inaccuracies in detection. Additionally, misinformation tactics are constantly evolving, requiring AI systems to adapt rapidly to new methods of spreading false information.
- What is algorithmic bias?
Algorithmic bias occurs when AI systems produce prejudiced results due to biased training data or flawed programming. This can impact the accuracy of fake news detection, leading to missed misinformation or false positives.
- How can AI tools be improved for better fake news detection?
To enhance AI tools for fake news detection, continuous training with diverse datasets is essential. Additionally, incorporating human oversight can help identify and correct biases, ensuring more accurate results in identifying misinformation.
- What does the future hold for AI in news verification?
The future of AI in news verification looks promising. As technology advances, we can expect AI systems to become more sophisticated, improving their ability to detect misinformation and enhancing public trust in digital information.