Artificial Intelligence and Machine Learning in FinTech
In a world where technology is evolving at breakneck speed, Artificial Intelligence (AI) and Machine Learning (ML) have emerged as game-changers in the financial technology (FinTech) sector. These cutting-edge technologies are not just buzzwords; they are fundamentally altering how financial institutions operate, interact with customers, and manage risks. Imagine a world where decisions are made not just by humans, but by intelligent systems that learn and adapt over time. Sounds futuristic, right? Well, that future is here, and it's reshaping the financial landscape in ways we never thought possible.
At its core, AI in FinTech is about leveraging data to enhance decision-making processes. Financial institutions today are inundated with vast amounts of data, and extracting meaningful insights from this data can be daunting. This is where AI comes into play, enabling businesses to sift through mountains of information quickly and accurately. By automating routine tasks, AI not only increases efficiency but also significantly reduces operational costs. Think of AI as your personal financial assistant, tirelessly working behind the scenes to ensure everything runs smoothly.
Machine learning, a subset of AI, takes this a step further. It involves algorithms that can learn from data, identify patterns, and make predictions without being explicitly programmed. In the context of finance, this means that institutions can analyze historical data to forecast trends, assess risks, and tailor services to individual customers. The implications are profound: investors can make informed decisions based on predictive analytics, while customers receive personalized financial advice that meets their unique needs.
As we dive deeper into the applications of AI and ML in FinTech, it becomes clear that these technologies are not just enhancing operational efficiency but are also revolutionizing customer experiences. From AI-driven chatbots providing 24/7 support to sophisticated algorithms recommending investment strategies, the future of finance is increasingly customer-centric and data-driven.
However, with great power comes great responsibility. The integration of AI and ML in FinTech is not without its challenges. Issues related to data privacy, security, and regulatory compliance must be navigated carefully. Financial institutions need to ensure that they are not only harnessing the power of AI but also protecting the sensitive information of their customers. It's a balancing act that requires skill and foresight.
In summary, the intersection of AI, ML, and FinTech is a thrilling space filled with opportunities and challenges. As these technologies continue to evolve, they will undoubtedly shape the future of finance, making it more efficient, secure, and customer-focused. The question remains: are we ready to embrace this change?
- What is the role of AI in FinTech? AI enhances decision-making, improves customer experiences, and automates tasks in financial services.
- How do machine learning algorithms benefit finance? They analyze large datasets for predictive analytics, risk assessment, and personalized services.
- What challenges does AI face in the FinTech industry? Key challenges include data privacy, regulatory compliance, and the need for skilled professionals.
- How does AI improve customer experience in FinTech? Through chatbots, personalized recommendations, and streamlined services.
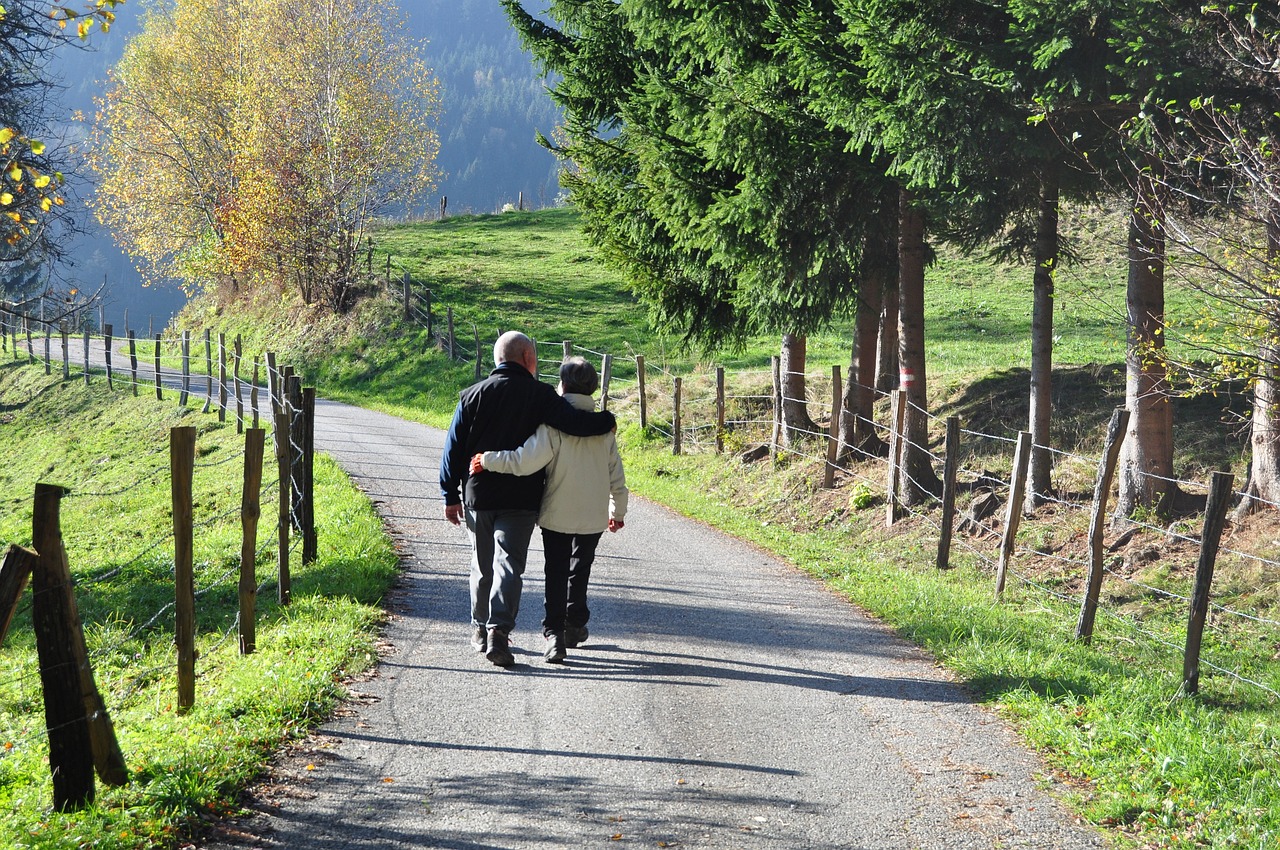
The Role of AI in FinTech
Artificial Intelligence (AI) is not just a buzzword; it's a game-changer in the financial technology sector. Imagine a world where financial services are not only faster but also smarter. AI is revolutionizing how decisions are made, how customers interact with financial institutions, and how routine tasks are automated. This transformation is leading to increased efficiency and significantly reduced operational costs for businesses.
At its core, AI enhances decision-making processes. By analyzing vast amounts of data, AI systems can provide insights that were previously unimaginable. This means that financial institutions can make data-driven decisions quickly, minimizing risks and maximizing opportunities. For instance, AI algorithms can sift through market trends, customer behaviors, and economic indicators to help firms make informed choices about investments and resource allocation.
Moreover, AI is improving customer experiences in ways we never thought possible. Have you ever chatted with a customer service bot that seemed to understand your needs? That's AI at work! These smart systems can provide personalized recommendations based on individual customer data, ensuring that users receive services tailored specifically to their needs. This level of personalization not only enhances satisfaction but also fosters loyalty, as customers feel valued and understood.
Another remarkable aspect of AI in FinTech is its ability to automate routine tasks. Consider the time-consuming processes involved in loan approvals or account verifications. With AI, these tasks can be streamlined, reducing the time it takes for customers to receive approvals. This not only improves customer satisfaction but also allows employees to focus on more complex and strategic tasks, thereby enhancing overall productivity.
To illustrate the transformative impact of AI in FinTech, let's look at a simple comparison:
Traditional Processes | AI-Driven Processes |
---|---|
Manual data entry | Automated data processing |
Static customer service | Dynamic AI chatbots |
Time-consuming risk assessments | Real-time risk analysis |
In summary, the role of AI in FinTech is multifaceted, encompassing enhanced decision-making, improved customer experiences, and the automation of routine tasks. As these technologies continue to evolve, we can expect even more innovative solutions that will not only streamline operations but also redefine the customer journey in the financial sector.
- What is AI in FinTech? AI in FinTech refers to the use of artificial intelligence technologies to improve financial services, enhance decision-making, and automate processes.
- How does AI improve customer experience? AI enhances customer experience by providing personalized services, such as tailored recommendations and efficient customer support through chatbots.
- What are the benefits of automating tasks in FinTech? Automating tasks reduces processing times, minimizes human error, and allows employees to focus on more strategic activities.
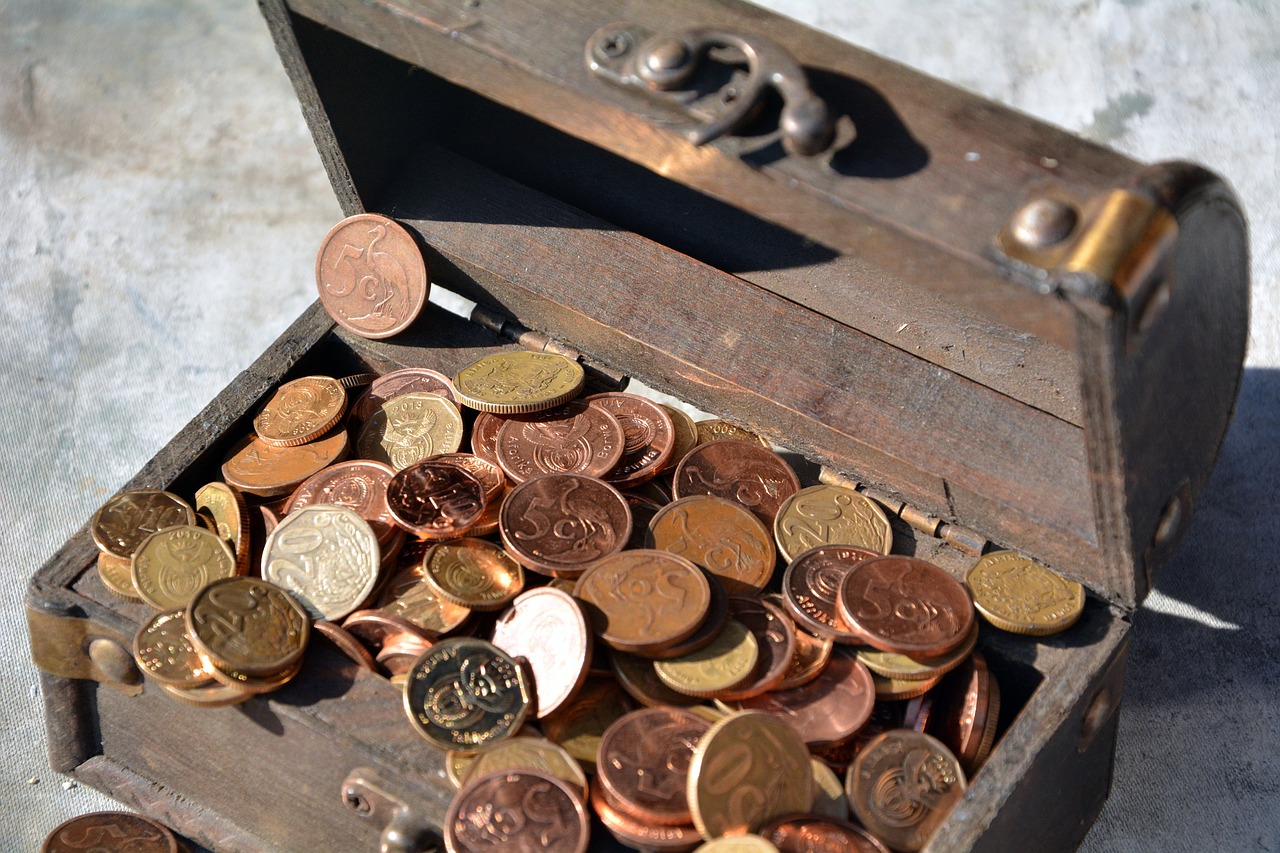
Machine Learning Algorithms in Finance
In the fast-paced world of finance, the ability to swiftly analyze and interpret vast amounts of data is crucial. Machine learning algorithms are at the forefront of this revolution, enabling financial institutions to harness the power of data like never before. These sophisticated algorithms are designed to learn from historical data, adapt to new information, and make predictions that help in various financial applications. By analyzing patterns and trends, machine learning provides insights that were previously unimaginable, optimizing investment strategies and enhancing customer engagement.
One of the most significant applications of machine learning in finance is in the realm of predictive analytics. This involves using historical data to forecast future trends, which is essential for investors looking to make informed decisions. For instance, algorithms can analyze stock price movements, economic indicators, and even social media sentiment to predict how a particular investment might perform. This allows investors to not only capitalize on potential opportunities but also to manage risks effectively in an ever-changing financial landscape.
Imagine having a crystal ball that tells you which stocks will soar and which will plummet. While machine learning isn't quite magic, it comes pretty close! By utilizing predictive analytics, investors can gain a clearer picture of market trends. These algorithms sift through mountains of data, from historical prices to current market conditions, to identify patterns that can guide investment decisions. The beauty of this approach is that it evolves over time; as new data becomes available, the algorithms refine their predictions, making them increasingly accurate.
Risk is an inherent part of investing, but with machine learning, financial institutions can mitigate it more effectively. Advanced machine learning models can analyze real-time data to identify potential risks before they escalate. For example, these models can monitor market volatility, economic shifts, and even geopolitical events to alert institutions of impending risks. This proactive approach allows companies to implement measures to safeguard their assets, ensuring a more stable financial environment.
In addition to enhancing investment strategies, machine learning algorithms play a pivotal role in fraud detection. By analyzing transaction patterns, these AI-driven systems can identify anomalies that may indicate fraudulent activities. For instance, if a customer's spending behavior suddenly shifts dramatically, the system can flag this transaction for further investigation. This capability significantly reduces the incidence of fraud, enhancing the overall security of financial transactions. With the potential to save billions in losses, investing in such technologies is a no-brainer for financial institutions.
Moreover, the integration of machine learning into fraud detection systems is not just about identifying fraud; it’s also about improving customer trust. When customers know that their financial institutions are using cutting-edge technology to protect their assets, they feel more secure and valued. This trust translates into customer loyalty, which is invaluable in the competitive world of finance.
In summary, machine learning algorithms are transforming the finance industry by providing tools that enhance decision-making, optimize investment strategies, and improve customer interactions. As technology continues to evolve, the potential applications of machine learning in finance are bound to expand, paving the way for a more efficient and secure financial landscape.
- What is machine learning in finance?
Machine learning in finance refers to the use of algorithms and statistical models to analyze financial data, make predictions, and improve decision-making processes.
- How does predictive analytics benefit investors?
Predictive analytics helps investors forecast market trends and make informed decisions, ultimately optimizing their investment strategies.
- Can machine learning prevent fraud?
Yes, machine learning algorithms can analyze transaction patterns to detect anomalies and flag potential fraudulent activities, thereby enhancing security.
- What challenges does machine learning face in finance?
Some challenges include data privacy concerns, regulatory compliance, and the need for skilled professionals to manage these advanced technologies.
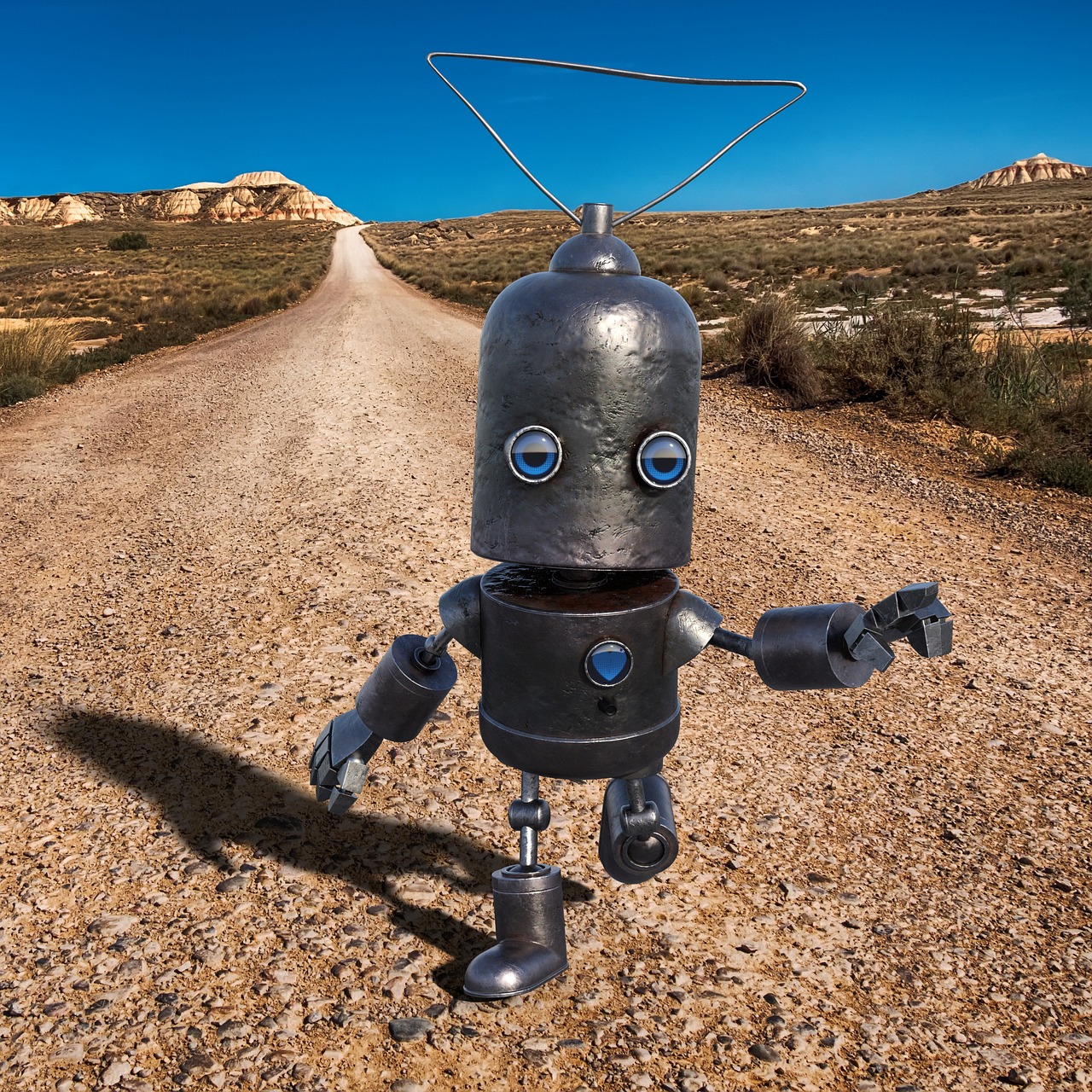
Predictive Analytics for Investment
Predictive analytics is like having a crystal ball for investors. It takes historical data, applies complex algorithms, and forecasts future market trends, allowing investors to make informed decisions. Imagine trying to navigate a foggy road without headlights; that’s what investing feels like without predictive analytics. By illuminating potential outcomes based on past behaviors, these technologies serve as a guiding light, helping investors steer clear of pitfalls and seize opportunities.
At the core of predictive analytics are sophisticated statistical techniques and machine learning models that analyze vast amounts of data. This can include everything from stock prices, economic indicators, and even social media sentiment. By examining these factors, predictive analytics can identify patterns that might not be immediately apparent to the human eye. For instance, an investor might notice a correlation between social media trends and stock performance, enabling them to make timely investment decisions based on emerging market sentiments.
One of the most compelling advantages of predictive analytics in investment is its ability to optimize portfolio management. By providing insights into which assets are likely to perform well or poorly, investors can adjust their portfolios proactively. This is particularly crucial in today’s fast-paced financial landscape, where market conditions can change rapidly. A well-timed shift in investment strategy can mean the difference between profit and loss.
Moreover, predictive analytics can significantly enhance risk management. Investors can use these tools to assess potential risks associated with various investment options. For example, algorithms can evaluate the likelihood of a stock's price dropping based on historical volatility and current market conditions. This allows investors to implement strategies to mitigate risks, such as diversifying their portfolios or setting stop-loss orders.
To illustrate the impact of predictive analytics, consider the following table that outlines the key benefits for investors:
Benefit | Description |
---|---|
Informed Decision-Making | Utilizes data-driven insights to guide investment choices. |
Risk Assessment | Evaluates potential risks and forecasts market behavior. |
Portfolio Optimization | Adapts investment strategies based on predictive data. |
Market Trend Identification | Detects emerging trends before they become mainstream. |
As we look to the future, predictive analytics will continue to evolve, incorporating more advanced technologies such as artificial intelligence and big data analytics. This evolution will further enhance its accuracy and effectiveness, making it an indispensable tool for investors. So, whether you’re a seasoned investor or just starting out, embracing predictive analytics could be your ticket to navigating the turbulent waters of the financial markets with confidence.
- What is predictive analytics?
Predictive analytics is a branch of advanced analytics that uses historical data, machine learning, and statistical algorithms to forecast future outcomes.
- How can predictive analytics help investors?
It helps investors make informed decisions, optimize their portfolios, and assess risks by providing insights based on data analysis.
- Is predictive analytics only for large investors?
No, predictive analytics can be beneficial for investors of all sizes, including individual investors looking to enhance their decision-making process.
- What tools are used for predictive analytics in finance?
Various tools and software, including machine learning platforms and statistical analysis software, are used to perform predictive analytics in finance.
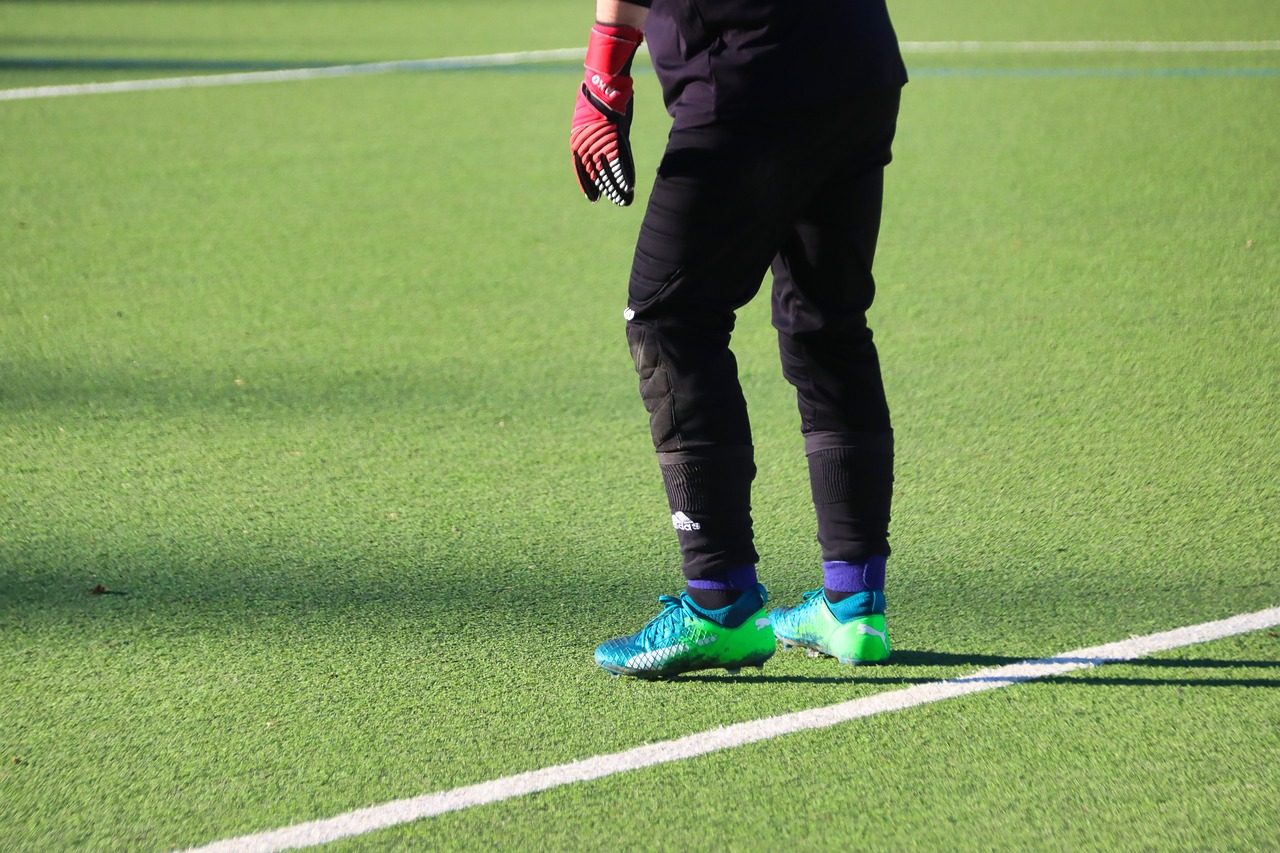
Risk Management Techniques
In the fast-paced world of finance, risk management is no longer just a safety net; it's a critical component of strategic decision-making. With the advent of machine learning algorithms, financial institutions can now leverage advanced analytics to identify potential risks in real-time. Imagine having a crystal ball that not only predicts market fluctuations but also helps you navigate them effectively. This is the reality that AI brings to the table.
One of the most significant advantages of using machine learning in risk management is its ability to analyze vast amounts of data at lightning speed. Traditional risk assessment methods often rely on historical data and static models, which can become outdated quickly. In contrast, machine learning algorithms continuously learn from new data, adapting to changing market conditions and uncovering hidden patterns that human analysts might miss. This dynamic approach allows institutions to stay one step ahead of potential threats.
For instance, consider a bank that implements a machine learning model to monitor its loan portfolio. By analyzing various factors—such as borrower behavior, economic indicators, and industry trends—the model can identify which loans are at risk of defaulting. This enables the bank to take proactive measures, such as adjusting interest rates or enhancing borrower support, ultimately safeguarding its assets.
Furthermore, the integration of AI in risk management can enhance the accuracy of credit scoring. Traditional scoring models often rely on a limited set of criteria, which can lead to biases or inaccuracies. Machine learning algorithms, on the other hand, can consider a broader range of factors, including social media activity or transaction history, to provide a more nuanced assessment of a borrower's creditworthiness. This not only benefits lenders by reducing default rates but also helps consumers gain better access to credit.
However, while the benefits of AI-driven risk management are substantial, there are challenges to consider. The reliance on data necessitates robust data governance practices to ensure that the information used is accurate and relevant. Additionally, as these systems become more complex, financial institutions must invest in skilled professionals who can interpret the insights generated by machine learning models and implement them effectively.
In summary, the use of machine learning in risk management is transforming how financial institutions approach risk assessment and mitigation. By harnessing the power of advanced analytics, these organizations can not only protect their assets but also enhance their overall operational efficiency. As we move forward, the continuous evolution of AI technologies will undoubtedly lead to even more sophisticated risk management techniques, setting a new standard in the financial industry.
- What is the role of AI in risk management? AI enhances risk management by analyzing large datasets to identify potential risks in real-time, allowing for proactive measures.
- How does machine learning improve credit scoring? Machine learning considers a wider range of factors for credit scoring, leading to more accurate assessments of a borrower's creditworthiness.
- What challenges do financial institutions face when implementing AI for risk management? Challenges include ensuring data accuracy, managing complex systems, and the need for skilled professionals.

Fraud Detection Systems
In today's fast-paced digital world, where financial transactions occur at lightning speed, have become an essential line of defense for financial institutions. These AI-driven systems are designed to analyze transaction patterns and identify anomalies that could indicate fraudulent activities. Imagine a vigilant security guard, tirelessly watching over a bustling marketplace, ready to raise an alarm at the first sign of trouble; that’s how these systems operate, ensuring that every transaction is scrutinized for potential risks.
One of the most remarkable features of these fraud detection systems is their ability to learn from historical data. By employing machine learning algorithms, they can adapt and improve over time, becoming increasingly proficient at distinguishing between legitimate transactions and those that may pose a threat. This is akin to an experienced detective who becomes more adept at spotting suspicious behavior through years of training and experience. The more data these systems analyze, the better they become at predicting and preventing fraud.
These systems utilize a variety of techniques to enhance their detection capabilities. For instance, they can employ real-time monitoring, which allows them to flag transactions as they happen. If a transaction deviates from a customer’s typical spending behavior—like a sudden purchase from a foreign country or a high-value transaction that occurs late at night—an alert is generated. This proactive approach not only helps in catching fraud early but also provides a sense of security for customers who may otherwise feel vulnerable in the digital financial landscape.
Furthermore, fraud detection systems are not just reactive; they also incorporate predictive analytics. By analyzing trends and patterns in data, these systems can forecast potential fraud risks before they materialize. For example, if a certain pattern emerges in transaction data—such as a spike in fraudulent activities in a specific region during a particular time of year—the system can alert financial institutions to take preventive measures. This ability to anticipate fraud before it occurs is a game-changer in the realm of financial security.
However, while these systems are incredibly effective, they are not without challenges. The accuracy of fraud detection systems relies heavily on the quality and quantity of data they are fed. If the data is incomplete or biased, it can lead to false positives, where legitimate transactions are flagged as fraudulent, causing inconvenience and frustration for customers. Therefore, it is crucial for financial institutions to continually refine their data collection and analysis processes to ensure the efficacy of their fraud detection systems.
In conclusion, AI-driven fraud detection systems are transforming the way financial institutions safeguard their assets and protect their customers. By leveraging advanced algorithms and real-time monitoring, these systems not only enhance security but also foster trust between consumers and financial entities. As technology continues to advance, we can expect these systems to become even more sophisticated, providing an essential shield against the ever-evolving landscape of financial fraud.
- What is a fraud detection system?
A fraud detection system is a technology that uses algorithms and data analysis to identify and prevent fraudulent transactions in real-time.
- How does machine learning improve fraud detection?
Machine learning enhances fraud detection by allowing systems to learn from historical data, improving their ability to recognize patterns and anomalies over time.
- What are the challenges faced by fraud detection systems?
Challenges include managing false positives, ensuring data quality, and adapting to new fraud tactics as they emerge.
- Can fraud detection systems guarantee 100% security?
No system can guarantee 100% security, but advanced fraud detection systems significantly reduce the risk of fraud.

Customer Experience Enhancement
In today’s fast-paced digital world, customer experience has become a cornerstone of success in the FinTech industry. With the rise of AI technologies, financial institutions are now able to redefine how they interact with their customers. Imagine walking into a bank where the staff already knows your name, preferences, and financial history. Sounds like a scene from a sci-fi movie, right? Well, thanks to AI, this is becoming a reality. By leveraging data analytics, FinTech companies can offer personalized services that not only meet but anticipate customer needs.
One of the most significant advancements in this area is the use of chatbots. These AI-driven virtual assistants are available 24/7, providing instant responses to customer inquiries. This means no more waiting in long queues or being put on hold. Customers can get answers to their questions in real-time, which significantly enhances their overall experience. For instance, a customer looking to transfer funds can simply ask the chatbot for guidance, and within seconds, they can complete the transaction without any hassle.
Moreover, personalized recommendations powered by machine learning algorithms are transforming the way customers manage their finances. By analyzing past behavior and preferences, these systems can suggest tailored financial products and services. For example, if a customer frequently invests in tech stocks, the system might recommend similar investment opportunities or alert them about market trends related to their interests. This level of personalization not only boosts customer satisfaction but also fosters loyalty, as clients feel understood and valued.
Another essential aspect of enhancing customer experience is the streamlining of services. AI helps eliminate redundancies and inefficiencies in financial processes. For instance, automated loan approval systems can assess an applicant’s creditworthiness in a fraction of the time it traditionally takes. This not only speeds up the process but also reduces the frustration that often accompanies lengthy applications. The result? A seamless experience that keeps customers coming back for more.
In addition to these advancements, the integration of AI in customer experience also opens the door for proactive engagement. Imagine receiving a notification from your bank about a potential overdraft before it happens, allowing you to transfer funds or adjust your spending in real-time. This level of foresight not only helps customers avoid penalties but also builds trust in their financial institution.
As we look to the future, the potential of AI in enhancing customer experience is limitless. The combination of advanced analytics, machine learning, and personalized interactions is set to redefine the financial landscape. However, it’s crucial for companies to remain vigilant about maintaining a human touch in their services. After all, while technology can enhance efficiency, the emotional connection between customers and their financial service providers is irreplaceable.
- How do chatbots improve customer experience in FinTech?
Chatbots provide instant responses to customer inquiries, reducing wait times and enhancing accessibility. - What role does AI play in personalizing financial services?
AI analyzes customer data to offer tailored recommendations and insights, making financial management easier and more relevant. - Can AI help in proactive customer engagement?
Yes, AI can send alerts and notifications to customers about potential issues, helping them take action before problems arise.

Challenges of AI in FinTech
The integration of Artificial Intelligence (AI) into the Financial Technology (FinTech) sector is undoubtedly a game-changer, but it doesn't come without its share of challenges. As exciting as it is to harness the power of AI for improving financial services, there are significant hurdles that companies must navigate to ensure successful implementation. These challenges can be broadly categorized into three main areas: data privacy and security, regulatory compliance, and the need for skilled professionals.
First and foremost, data privacy and security are paramount in the FinTech industry. With AI systems handling sensitive financial information, the risk of data breaches becomes a pressing concern. Companies must implement robust security measures to protect customer data from unauthorized access and cyber threats. This includes not only encryption and secure storage but also ongoing monitoring and quick response strategies to handle any potential breaches. The stakes are high; a single data leak could result in significant financial losses and damage to a company's reputation.
Next, navigating the regulatory compliance landscape poses another challenge for FinTech firms looking to adopt AI technologies. The financial industry is heavily regulated, and companies must adhere to various laws and guidelines while still trying to innovate. This often requires a delicate balancing act: ensuring that AI applications comply with existing regulations while also pushing the envelope to improve services. For instance, regulations concerning customer data usage can limit how AI algorithms are trained and applied, making it crucial for companies to stay informed about changes in the regulatory environment.
Moreover, the need for skilled professionals cannot be overlooked. The deployment of AI technologies in FinTech requires a specialized skill set that combines knowledge of finance, technology, and data science. Unfortunately, there is currently a shortage of professionals who possess these qualifications. This gap can slow down the adoption of AI technologies, as companies may struggle to find the right talent to develop and manage these complex systems. Investing in training and development programs can be a viable solution, but it requires time and resources that some firms may not readily have.
In summary, while the potential benefits of AI in FinTech are immense, the challenges are equally significant. Companies must prioritize data privacy, navigate regulatory hurdles, and seek out skilled professionals to harness the full potential of AI technologies. Addressing these challenges head-on will be crucial for FinTech firms looking to thrive in an increasingly competitive landscape.
- What are the main challenges of implementing AI in FinTech?
The main challenges include data privacy and security concerns, regulatory compliance issues, and the shortage of skilled professionals. - How does data privacy impact AI in FinTech?
Data privacy is critical as AI systems handle sensitive financial information, necessitating robust security measures to prevent breaches. - Why is regulatory compliance a challenge for FinTech companies using AI?
FinTech companies must adhere to a complex array of regulations while trying to innovate, which can create conflicts and slow down progress. - What can FinTech companies do to overcome the skills gap in AI?
Investing in training programs and partnerships with educational institutions can help bridge the skills gap.
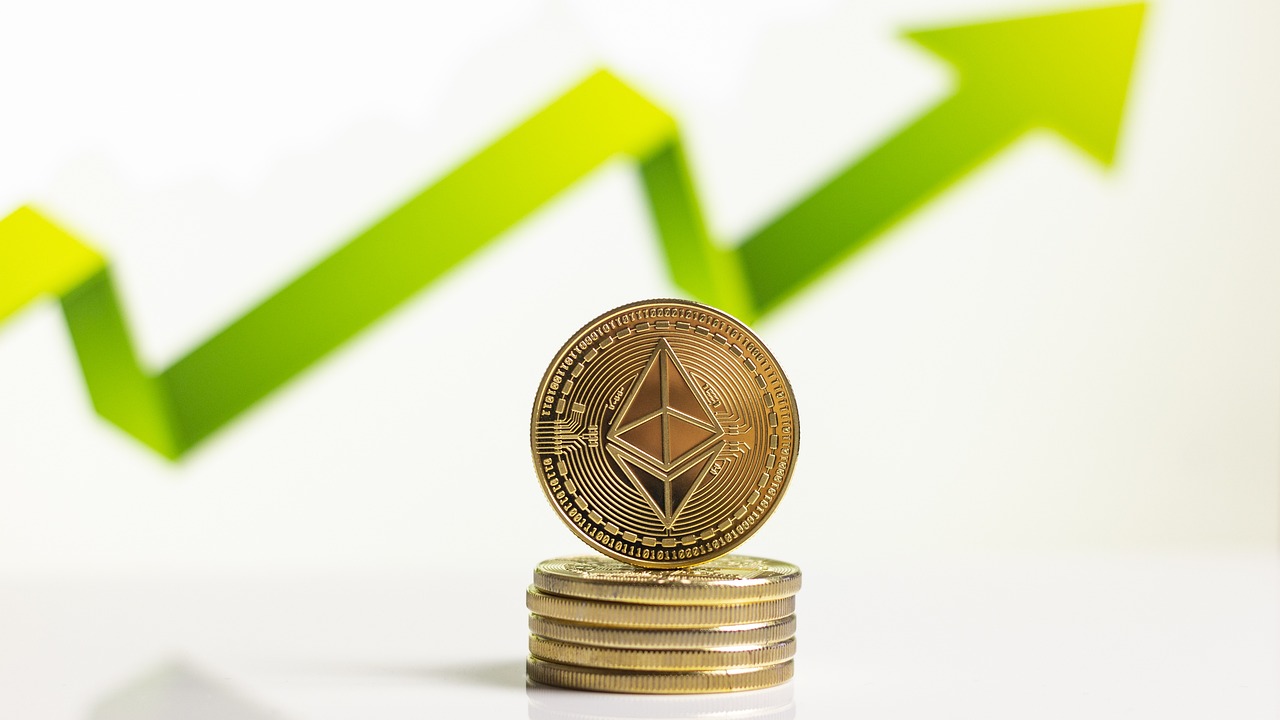
Data Privacy and Security
In the exhilarating world of FinTech, where every transaction is a click away, the importance of data privacy and security cannot be overstated. As financial institutions increasingly rely on artificial intelligence to enhance their services, they are also tasked with the monumental responsibility of safeguarding sensitive information. Imagine handling a treasure trove of personal financial data—each piece a key to someone's financial well-being. Now, what happens if that treasure gets stolen? That's the nightmare scenario every FinTech company is trying to avoid.
With the rise of AI technologies, financial institutions are collecting and processing vast amounts of data. This data can include everything from user transaction histories to personal identification information. The challenge lies in ensuring that this information is not only collected responsibly but also protected against potential breaches. According to recent studies, data breaches can cost companies millions, both in terms of direct financial loss and damage to their reputation. Therefore, implementing robust security measures is not just a regulatory requirement; it's a business imperative.
To tackle these challenges, companies are employing a variety of strategies. Here are some key approaches:
- Encryption: This is the process of converting data into a coded format to prevent unauthorized access. Think of it as locking your valuables in a safe.
- Access Controls: Limiting access to sensitive information to only those who need it can significantly reduce the risk of data breaches.
- Regular Audits: Conducting periodic audits helps identify vulnerabilities in the system before they can be exploited.
Moreover, the need for compliance with regulations such as the General Data Protection Regulation (GDPR) and the California Consumer Privacy Act (CCPA) adds another layer of complexity. These regulations mandate that companies be transparent about how they collect, use, and protect consumer data. Failing to comply can lead to hefty fines and legal repercussions, further emphasizing the need for stringent data privacy measures.
As we move forward, the integration of AI in FinTech will continue to evolve, but the focus on data privacy and security must remain at the forefront. Companies that prioritize these aspects will not only build trust with their customers but also position themselves as leaders in the industry. After all, in a world where data is the new oil, protecting it is akin to safeguarding a nation's wealth.
- What are the main risks associated with data privacy in FinTech? The primary risks include data breaches, unauthorized access, and non-compliance with regulations.
- How can FinTech companies ensure data security? Implementing strong encryption, access controls, regular audits, and adhering to regulatory standards are essential steps.
- What regulations should FinTech companies be aware of? Companies should be familiar with GDPR, CCPA, and other regional data protection laws.
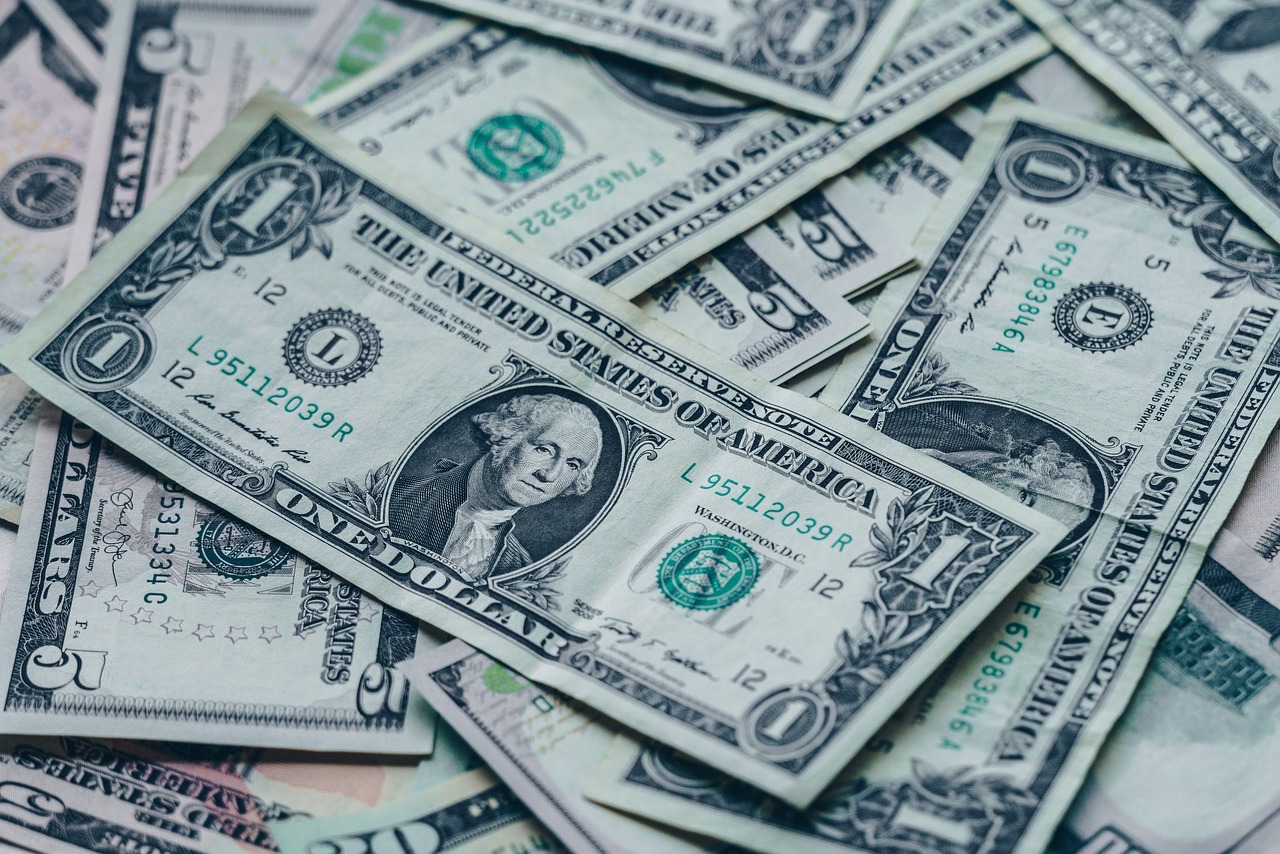
Regulatory Compliance Issues
Regulatory compliance in the FinTech sector is a complex maze that companies must navigate to ensure they operate legally and ethically. As the industry evolves and embraces artificial intelligence and machine learning technologies, the challenge of adhering to various regulations becomes even more pronounced. With regulations varying significantly across different jurisdictions, FinTech companies face the daunting task of staying compliant while innovating and leveraging these advanced technologies.
One of the primary challenges is understanding and implementing the myriad of regulations that govern financial services. These regulations are designed to protect consumers, maintain market integrity, and prevent illicit activities. However, the rapid pace of technological advancement often outstrips the regulatory framework, leading to potential gaps and uncertainties. For instance, how can a company ensure compliance when the rules are constantly changing, or when new technologies are introduced that don’t fit neatly into existing regulatory categories?
Moreover, the use of AI and machine learning raises additional compliance concerns, particularly regarding data usage and privacy. Financial institutions must ensure that their algorithms do not inadvertently discriminate against certain groups or violate data protection laws. This is where a strong understanding of both technology and regulation becomes essential. Companies that fail to comply with regulatory standards risk facing hefty fines, legal challenges, and reputational damage.
To manage these compliance issues effectively, FinTech companies can implement several strategies:
- Regular Training: Providing ongoing training for staff on regulatory changes and compliance best practices is crucial for maintaining a compliant organization.
- Compliance Technology: Investing in compliance management systems that leverage AI can help monitor regulatory changes and ensure adherence to applicable laws.
- Collaboration with Regulators: Engaging in open dialogues with regulatory bodies can help FinTech companies stay ahead of compliance requirements and contribute to shaping future regulations.
Additionally, many FinTech firms are turning to third-party compliance experts who specialize in navigating the regulatory landscape. These experts can provide invaluable insights and help organizations develop robust compliance frameworks that align with their innovative technologies. By proactively addressing compliance issues, FinTech companies can not only avoid penalties but also build trust with their customers, which is essential for long-term success.
In conclusion, while the integration of AI and machine learning into FinTech offers numerous opportunities for growth and efficiency, it also presents significant regulatory compliance challenges. By adopting a proactive approach and leveraging technology, FinTech companies can navigate these challenges effectively, ensuring they remain compliant while continuing to innovate.
- What are the main regulatory challenges faced by FinTech companies?
FinTech companies often face challenges related to data privacy, anti-money laundering regulations, and consumer protection laws. - How can FinTech companies ensure compliance with regulations?
By implementing compliance management systems, providing regular training, and engaging with regulatory bodies, companies can stay compliant. - What role does AI play in regulatory compliance?
AI can help monitor compliance by analyzing vast amounts of data and identifying potential regulatory breaches in real-time.
Frequently Asked Questions
- What is the role of AI in FinTech?
AI is transforming the financial technology sector by enhancing decision-making processes, improving customer experiences, and automating routine tasks. This leads to increased efficiency and reduced operational costs for businesses, making financial services more accessible and responsive to customer needs.
- How do machine learning algorithms benefit finance?
Machine learning algorithms analyze vast datasets to enable predictive analytics, risk assessment, and personalized financial services. They optimize investment strategies and enhance customer engagement by providing insights that help businesses tailor their offerings to individual preferences.
- What is predictive analytics in investment?
Predictive analytics uses historical data to forecast market trends, helping investors make informed decisions. By understanding potential market movements, investors can manage risks more effectively in the ever-changing financial landscape.
- How does AI help in risk management?
Advanced machine learning models can identify potential risks in real-time, allowing financial institutions to implement proactive measures. This capability helps safeguard assets against unforeseen market fluctuations, ultimately contributing to a more secure financial environment.
- What are AI-driven fraud detection systems?
AI-driven fraud detection systems analyze transaction patterns to identify anomalies. By detecting suspicious activities early, these systems significantly reduce the incidence of fraud and enhance the security of financial transactions.
- How does AI enhance customer experience in FinTech?
AI technologies improve customer interactions through chatbots, personalized recommendations, and streamlined services. This leads to higher customer satisfaction and loyalty, as clients feel more valued and understood by their financial service providers.
- What challenges does AI face in FinTech?
Despite its advantages, the integration of AI in FinTech encounters challenges such as data privacy concerns, regulatory compliance, and the necessity for skilled professionals to manage these advanced technologies effectively.
- How important is data privacy in AI applications?
Data privacy and security are paramount in AI applications within FinTech. Handling sensitive financial information requires robust measures to protect against breaches and ensure that customer data remains confidential and secure.
- What are the regulatory compliance issues in FinTech?
FinTech companies must navigate a complex regulatory landscape to ensure compliance while leveraging AI technologies. Balancing innovation with adherence to regulations is crucial for sustainable growth in the industry.