Role of AI in Fraud Detection and Prevention
In today's fast-paced digital landscape, the battle against fraud has taken on new dimensions. With the rise of online transactions and digital communications, fraudsters are becoming increasingly sophisticated, employing advanced tactics that challenge traditional detection methods. This is where artificial intelligence (AI) steps in, revolutionizing the way we identify and prevent fraudulent activities. AI technologies are not just tools; they are transformative agents that empower organizations to stay one step ahead of fraudsters.
The sheer volume of data generated every day is staggering. From financial transactions to social media interactions, the information available for analysis is vast and complex. AI leverages this data, utilizing machine learning algorithms to sift through enormous datasets and identify patterns that human analysts might miss. By analyzing historical data and recognizing anomalies, AI systems can flag suspicious activities in real time, allowing for swift intervention.
Moreover, the integration of AI in fraud detection is not limited to financial sectors. Industries such as healthcare, e-commerce, and insurance are also harnessing the power of AI to combat fraud. For instance, in healthcare, AI can analyze patient records to identify billing anomalies that may indicate fraudulent claims, while in e-commerce, it can detect unusual purchasing behaviors that may signal fraudulent transactions. The versatility of AI makes it an invaluable asset across various sectors.
However, the implementation of AI in fraud detection is not without its challenges. Issues such as data privacy, algorithmic bias, and the necessity for ongoing model updates to adapt to new fraud tactics are critical considerations that organizations must address. As we delve deeper into the capabilities of AI, it is essential to also understand the ethical implications and the future trends that will shape its role in fraud prevention.
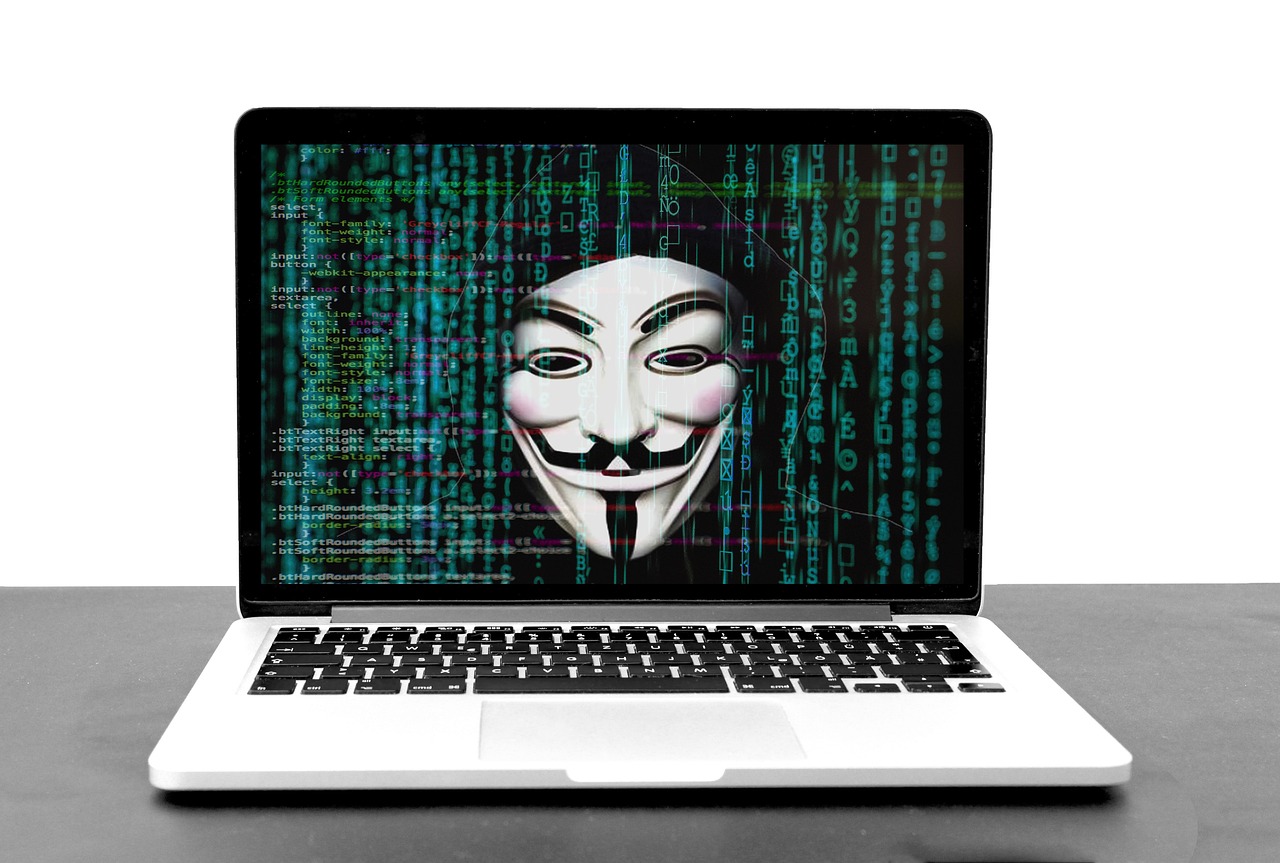
Understanding AI in Fraud Detection
Artificial Intelligence (AI) has revolutionized the way we approach fraud detection, providing powerful tools that can analyze patterns, recognize anomalies, and ultimately help in preventing fraudulent activities across various sectors. At its core, AI leverages advanced algorithms and machine learning techniques to sift through massive datasets, identifying suspicious behaviors that would be nearly impossible for humans to detect manually. Imagine trying to find a needle in a haystack; AI acts like a magnet, drawing out potential fraud cases while leaving the irrelevant data behind.
One of the fundamental principles of AI in fraud detection is its ability to learn from historical data. By analyzing past instances of fraud, AI systems can develop a comprehensive understanding of what constitutes normal behavior versus what might indicate a fraudulent act. This involves training machine learning models on labeled datasets where fraudulent and non-fraudulent behaviors are clearly defined. The model learns to recognize the subtle differences between these two categories, allowing it to make informed decisions when presented with new, unseen data.
Moreover, AI systems apply various algorithms to enhance their detection capabilities. For example, decision trees and neural networks are commonly used to classify transactions based on their characteristics. These algorithms can weigh different features, such as transaction amount, time, and location, to determine the likelihood of fraud. The beauty of AI lies in its adaptability; as new fraud tactics emerge, these systems can be retrained with updated data, ensuring that they remain effective against evolving threats.
In addition to traditional algorithms, AI also employs sophisticated techniques such as anomaly detection. This method focuses on identifying outliers in data that deviate significantly from established patterns. For instance, if a user typically makes small purchases but suddenly attempts to buy a luxury item, the AI can flag this transaction for further investigation. This proactive approach not only helps in catching fraud early but also minimizes potential losses for businesses.
Furthermore, AI's ability to process data in real-time is a game-changer for fraud detection. Unlike traditional methods that might involve days of analysis, AI systems can continuously monitor transactions, providing instant feedback and alerts when suspicious activities are detected. This capability is crucial in sectors like banking and e-commerce, where rapid responses can prevent significant financial damage.
In summary, the integration of AI in fraud detection is not just a technological advancement; it's a shift in how we approach security in the digital age. By harnessing the power of machine learning, anomaly detection, and real-time monitoring, businesses can create robust defenses against fraud, ensuring a safer environment for their customers. The journey of understanding AI in fraud detection is just beginning, and as technology evolves, so too will our methods of safeguarding against deceitful practices.
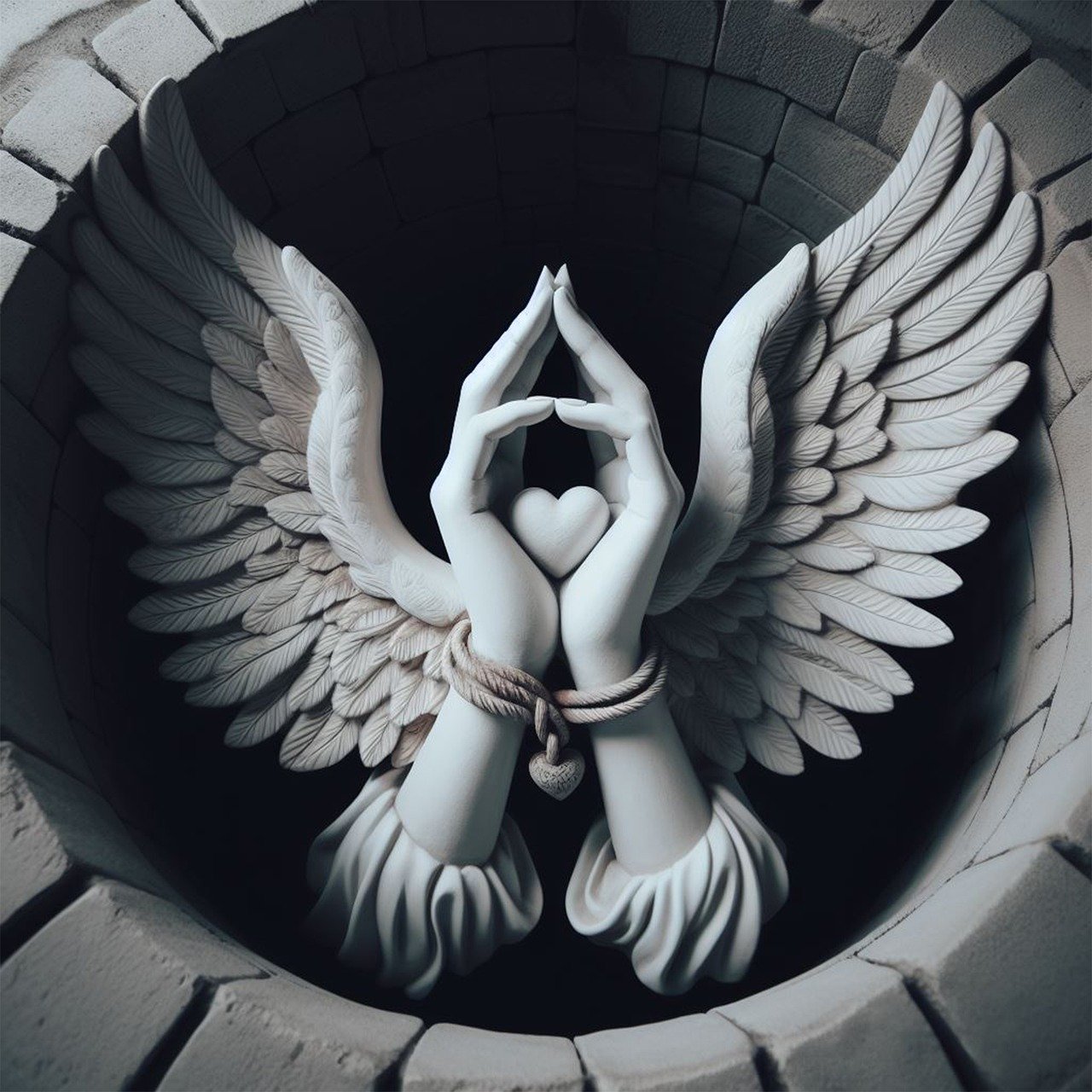
Machine Learning Techniques
When it comes to fraud detection, machine learning techniques are the superheroes of the digital age. They’re not just algorithms; they’re powerful tools that learn from data and adapt over time. Imagine having a personal detective that gets smarter with every case it solves. That’s what machine learning does! It processes vast amounts of information, identifies patterns, and flags suspicious activities that might go unnoticed by human eyes.
There are two primary types of machine learning techniques used in fraud detection: supervised learning and unsupervised learning. In supervised learning, the model is trained on a labeled dataset, meaning it learns from examples of both fraudulent and legitimate transactions. This approach is akin to teaching a child to recognize apples and oranges by showing them pictures of both fruits. Over time, the model becomes adept at distinguishing between the two, making it highly effective in identifying fraud.
On the other hand, unsupervised learning is like exploring a new city without a map. The model analyzes the data without prior labels, seeking out anomalies and patterns on its own. This can be particularly useful in fraud detection because it can uncover new types of fraud that the system hasn’t encountered before. For instance, if a new scheme emerges that doesn’t fit the mold of traditional fraud, an unsupervised learning model can still detect it by recognizing unusual patterns in transaction behavior.
To put it simply, these machine learning techniques enhance the accuracy of fraud detection in several ways:
- Adaptive Learning: The models continuously learn and improve from new data, making them resilient against evolving fraud tactics.
- High Accuracy: By analyzing historical data, these models can achieve a high level of precision in identifying fraudulent activity.
- Scalability: Machine learning algorithms can handle large datasets, making them suitable for businesses of all sizes.
Data analysis and pattern recognition are at the heart of these techniques. They involve sifting through mountains of data to pinpoint anomalies that could indicate fraud. This process is critical because fraudsters are constantly changing their tactics, and without sophisticated analysis, organizations risk falling behind. The algorithms used in machine learning can analyze transaction histories, user behavior, and even social media activity to identify red flags.
Moreover, feature engineering plays a crucial role in improving model performance. It involves selecting and transforming the most relevant data features to enhance the model's ability to detect fraud. For instance, instead of just looking at transaction amounts, the model might consider the time of day, the location of the transaction, and the frequency of similar transactions. By doing so, it creates a more comprehensive profile of what constitutes normal behavior versus suspicious activity.
In summary, machine learning techniques are revolutionizing how we approach fraud detection. With their ability to learn and adapt, they provide organizations with the tools necessary to combat increasingly sophisticated fraud tactics. As we move forward, these technologies will only become more integral to maintaining the integrity of financial systems and protecting consumers from fraud.
- What is the difference between supervised and unsupervised learning?
Supervised learning uses labeled data to train models, while unsupervised learning analyzes data without pre-existing labels to find hidden patterns. - How does feature engineering improve fraud detection?
Feature engineering enhances model performance by selecting and transforming relevant data features, allowing the model to better distinguish between normal and fraudulent behavior. - Can machine learning algorithms adapt to new types of fraud?
Yes, machine learning algorithms can adapt by continuously learning from new data, making them effective against evolving fraud tactics.
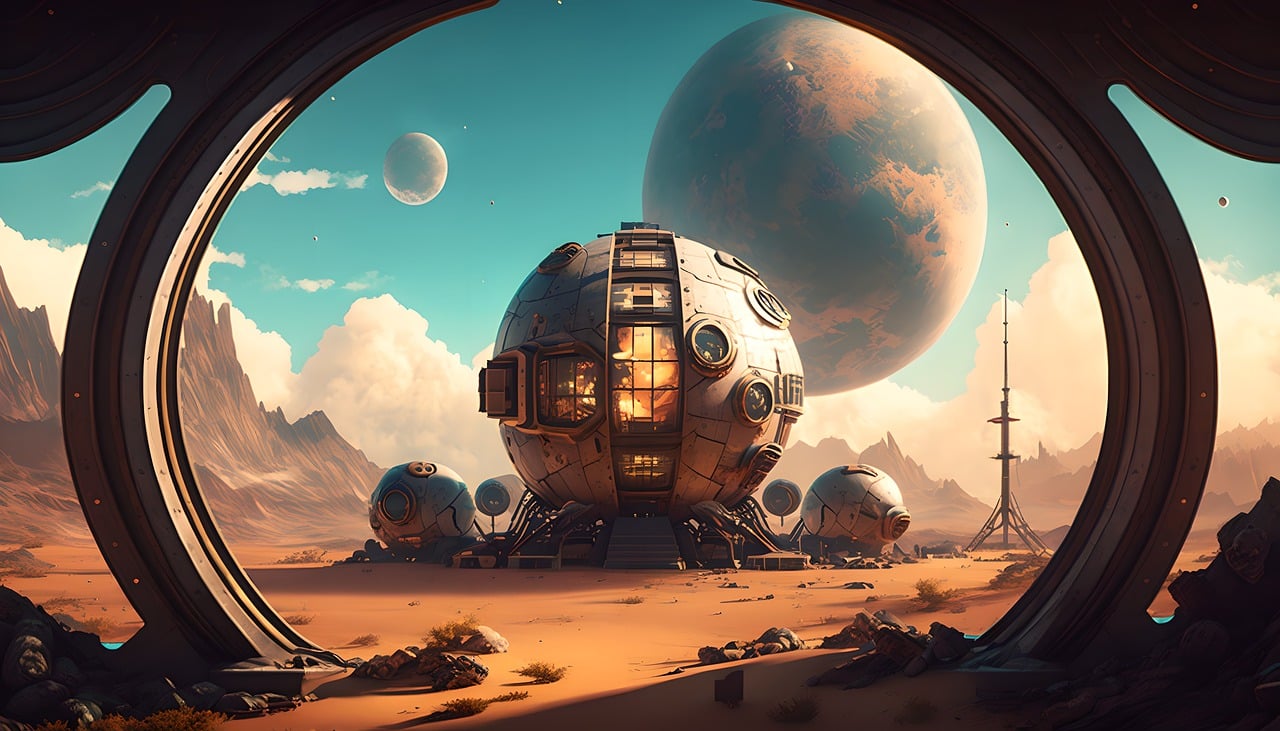
Data Analysis and Pattern Recognition
In the realm of fraud detection, data analysis and pattern recognition are the unsung heroes that work behind the scenes to uncover fraudulent activities. Imagine you’re a detective sifting through a mountain of evidence; that’s precisely what AI does, but at lightning speed. By leveraging advanced algorithms, AI systems can analyze vast datasets to identify unusual patterns that may indicate fraudulent behavior.
At the core of this process lies the ability of AI to recognize patterns in data that would be nearly impossible for humans to detect. For instance, consider an online banking system. Every transaction generates a plethora of data points: the amount, time, location, and even the device used. AI can analyze these variables collectively to spot anomalies. If a customer who typically makes small purchases suddenly attempts to withdraw a large sum from a foreign location, the system flags this as suspicious. This swift identification is crucial because, in the world of fraud, time is of the essence.
Moreover, AI employs various techniques to enhance its pattern recognition capabilities, including machine learning and statistical analysis. These methods allow the system to learn from historical data and improve its accuracy over time. For example, a supervised learning model can be trained on a dataset containing both legitimate and fraudulent transactions. As it processes this data, it learns to distinguish between the two, continuously refining its understanding of what constitutes normal versus suspicious behavior.
However, the effectiveness of AI in data analysis hinges on the quality of the data it processes. Here, feature engineering plays a pivotal role. By selecting and transforming relevant features, data scientists can significantly enhance the model's performance. For instance, instead of merely analyzing transaction amounts, incorporating features such as transaction frequency, geographic location, and even the time of day can provide richer insights. These engineered features help the model to make more informed predictions, thereby reducing false positives and improving overall detection rates.
Another fascinating aspect of AI's capabilities is its proficiency in real-time data analysis. With the proliferation of digital transactions, fraud can occur at any moment. AI systems can analyze data streams in real-time, allowing for immediate action. For example, if a credit card is used in two different countries within minutes of each other, AI can instantly flag this as potentially fraudulent and either alert the user or freeze the account until further verification.
To sum it up, the synergy between data analysis and pattern recognition in AI is what empowers organizations to stay one step ahead of fraudsters. By harnessing the power of algorithms to sift through extensive data, AI not only identifies fraudulent activities but also helps in understanding the underlying patterns that can inform future prevention strategies. As we move forward, the integration of more sophisticated analytical techniques will only enhance the capabilities of AI in this critical area.
- What is the role of AI in fraud detection? AI helps identify and prevent fraud by analyzing patterns and anomalies in large datasets, enabling organizations to respond quickly to suspicious activities.
- How does data analysis contribute to fraud detection? Data analysis allows AI to sift through vast amounts of information to recognize unusual patterns that may indicate fraudulent behavior.
- What is feature engineering? Feature engineering involves selecting and transforming data features to improve the performance of AI models in fraud detection.
- Why is real-time monitoring important? Real-time monitoring enables immediate detection and response to fraudulent activities, reducing potential losses for organizations.
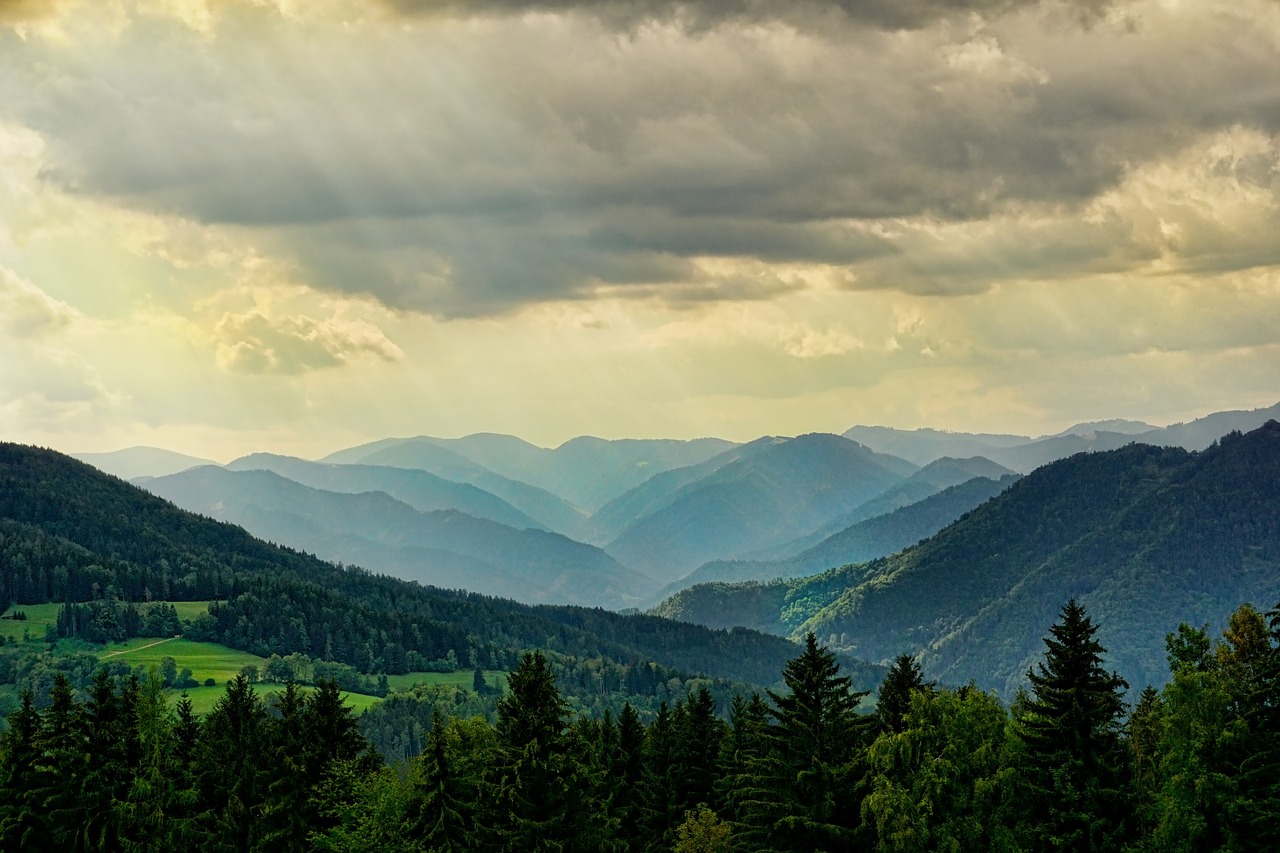
Feature Engineering
Feature engineering is a pivotal process in the realm of artificial intelligence, especially when it comes to enhancing the capabilities of fraud detection systems. Think of it as crafting the perfect recipe; each ingredient (or feature) must be carefully selected and prepared to ensure the final dish (or model) is both effective and efficient. In the context of fraud detection, the goal is to transform raw data into meaningful features that can significantly improve the model's ability to identify fraudulent behavior.
At its core, feature engineering involves the selection, modification, or creation of variables that will be used in machine learning models. This process is crucial because the quality of the features directly impacts the model's predictive power. For instance, if a fraud detection system only considers basic transaction data like amount and date, it may miss out on more complex patterns that could indicate fraudulent activity. Thus, incorporating additional features such as transaction location, user behavior history, and even device information can provide a more comprehensive view of each transaction.
One effective technique in feature engineering is one-hot encoding. This method transforms categorical variables into a format that can be provided to ML algorithms to improve predictions. For example, if we have a feature that indicates the type of transaction (e.g., online, in-store, mobile), one-hot encoding will create separate binary columns for each category, allowing the model to better understand the nuances of each transaction type.
Another important aspect of feature engineering is feature scaling. This process ensures that all features contribute equally to the distance calculations in algorithms like k-means clustering or support vector machines. Without scaling, features with larger ranges could disproportionately influence the model's outcomes. Techniques such as normalization and standardization are commonly used to achieve this balance, making sure that the model interprets all features fairly.
Moreover, time-based features can be particularly valuable in fraud detection. For instance, creating features that capture the time of day a transaction occurs or the frequency of transactions over a specific period can unveil patterns that are indicative of fraud. By analyzing these temporal features, AI systems can flag unusual activities that deviate from a user's typical behavior.
To illustrate the importance of feature engineering, consider the following table that outlines some common features used in fraud detection and their potential impact:
Feature | Description | Impact on Fraud Detection |
---|---|---|
Transaction Amount | The monetary value of the transaction | High amounts may indicate fraudulent behavior |
Transaction Location | Where the transaction took place | Unusual locations can signal fraud |
User Behavior History | Past transactions by the user | Helps in establishing a profile for normal behavior |
Device ID | Identification of the device used for the transaction | Flags transactions from unknown devices |
In conclusion, effective feature engineering is not just a technical necessity but a strategic advantage in the fight against fraud. By carefully selecting and transforming data features, organizations can significantly improve their AI models, making them more adept at catching fraudulent activities before they escalate. As fraud tactics continue to evolve, so too must the features we engineer, ensuring that our detection systems remain one step ahead.
- What is feature engineering? Feature engineering is the process of selecting, modifying, or creating variables to improve machine learning model performance.
- Why is feature engineering important in fraud detection? It enhances the model's ability to identify patterns and anomalies that may indicate fraudulent behavior.
- What techniques are commonly used in feature engineering? Techniques include one-hot encoding, feature scaling, and creating time-based features.
- How does feature engineering impact machine learning models? Quality features lead to better model accuracy and predictive power, crucial for catching fraud.
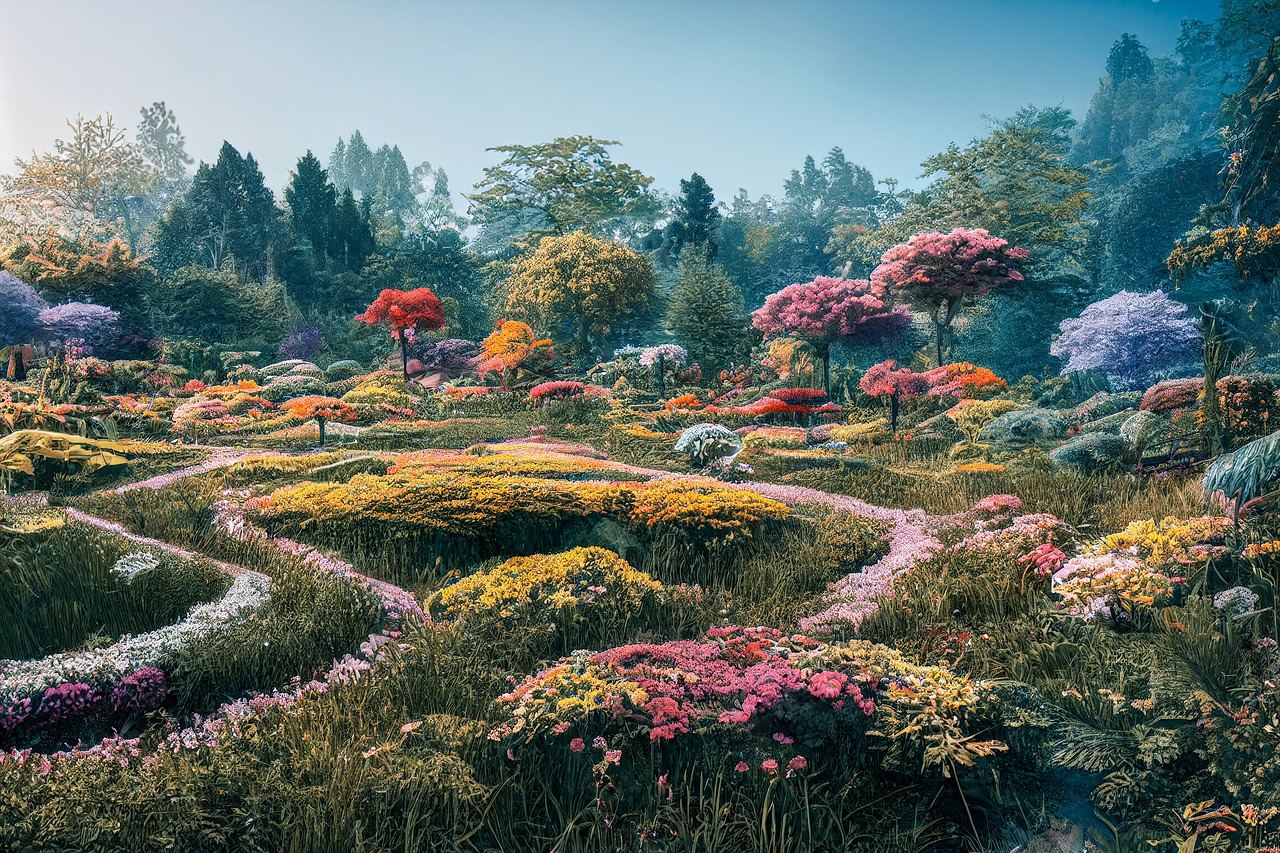
Real-Time Monitoring
In the fast-paced world of financial transactions and online activities, has become a game-changer in the fight against fraud. Imagine a vigilant security guard, constantly on the lookout for suspicious behavior, ready to act the moment something goes awry. This is precisely what AI-powered real-time monitoring systems do—they analyze data streams as they occur, allowing organizations to detect and respond to fraudulent activities almost instantaneously.
At the heart of real-time monitoring is the ability to process vast amounts of data in mere seconds. AI algorithms sift through incoming data from various sources, such as transaction logs, user behaviors, and communication channels. This constant vigilance enables the identification of anomalies that could signify fraudulent activity. For instance, if a user suddenly makes a large purchase from a different country, the system might flag this as suspicious and trigger an alert for further investigation.
One of the most compelling aspects of real-time monitoring is its adaptability. AI systems learn from past incidents, continuously refining their algorithms to improve detection rates. This means that, over time, the system becomes more proficient at distinguishing between legitimate activities and potential fraud. The result? A significant reduction in false positives, which can often frustrate users and lead to unnecessary disruptions.
Moreover, real-time monitoring is not just about detection; it’s also about prevention. By employing advanced techniques such as machine learning and predictive analytics, AI can forecast potential fraud scenarios before they happen. For example, if a pattern emerges where multiple accounts are compromised in a short period, the system can implement preventive measures, such as freezing accounts or requiring additional verification steps, to thwart the fraudsters.
To give you a clearer picture, consider the following table that outlines the key components of real-time monitoring in fraud detection:
Component | Description |
---|---|
Data Sources | Transaction logs, user behavior, communication channels |
AI Algorithms | Machine learning models that analyze data for anomalies |
Alert System | Immediate notifications for suspicious activities |
Preventive Measures | Actions taken to stop fraud before it occurs |
As organizations increasingly adopt real-time monitoring systems, the landscape of fraud detection is evolving. Companies that leverage these technologies not only enhance their security posture but also build trust with their customers. After all, knowing that their financial transactions are being monitored in real-time provides users with a sense of security and peace of mind.
In conclusion, the integration of real-time monitoring into fraud detection strategies represents a significant leap forward in the battle against fraudulent activities. With AI at the helm, organizations can stay one step ahead of fraudsters, ensuring a safer environment for their customers and themselves.
- What is real-time monitoring in fraud detection? Real-time monitoring involves the continuous analysis of data as it occurs to identify and respond to suspicious activities immediately.
- How does AI improve real-time monitoring? AI enhances real-time monitoring by utilizing machine learning algorithms that adapt and learn from historical data, improving detection accuracy over time.
- What are the benefits of real-time monitoring? The primary benefits include immediate detection of fraud, reduced false positives, and the ability to implement preventive measures before fraud occurs.
- Can real-time monitoring prevent all fraud? While real-time monitoring significantly reduces the risk of fraud, it may not eliminate it entirely. Continuous updates and improvements are necessary to keep pace with evolving tactics.
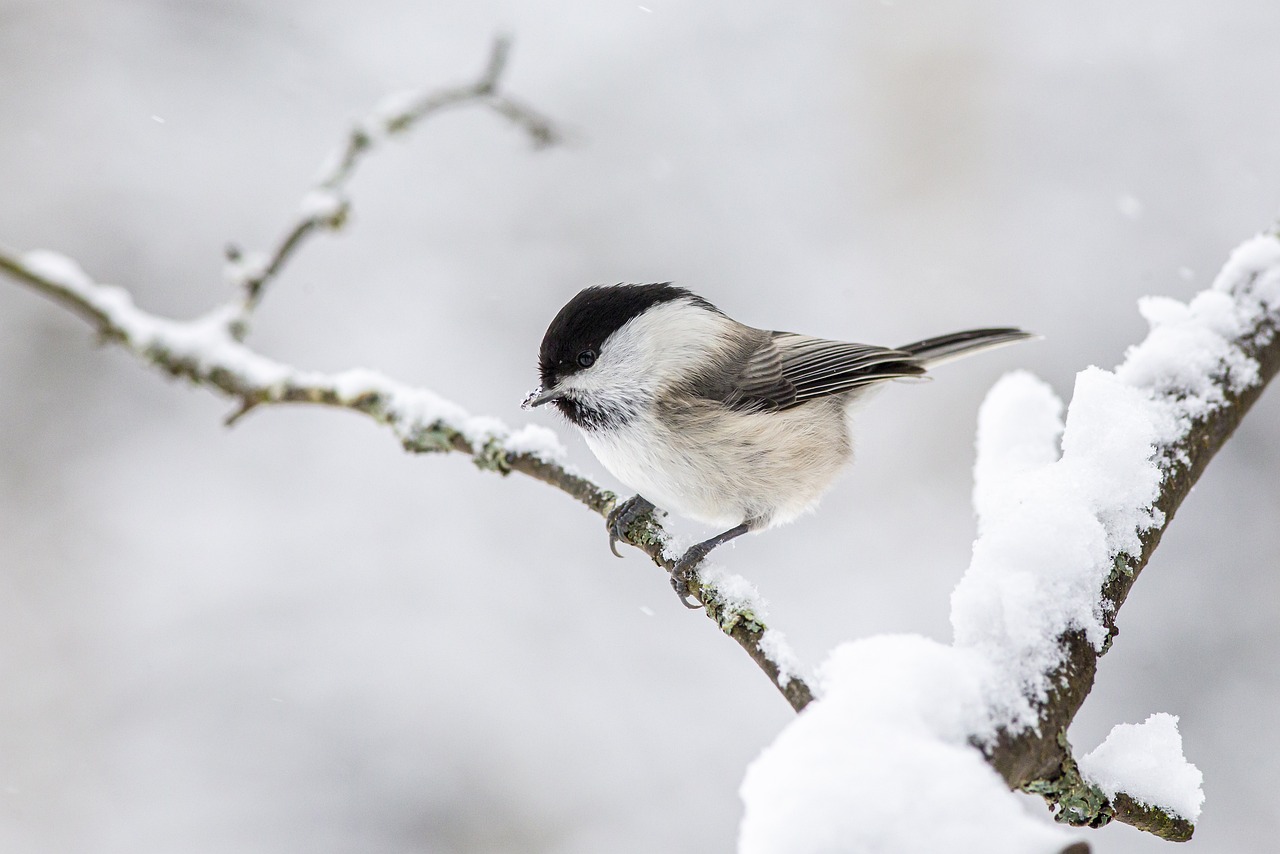
Natural Language Processing in Fraud Detection
Natural Language Processing (NLP) has emerged as a game-changer in the realm of fraud detection, particularly in analyzing text data for potential fraudulent activities. Imagine having a tool that can sift through thousands of emails, chat messages, and social media posts in a matter of seconds, picking out suspicious phrases or patterns that could indicate fraudulent behavior. This is precisely what NLP does; it empowers organizations to detect fraud not just through numerical data but also through the language used in communications.
The ability of NLP to understand and interpret human language allows it to analyze various forms of text data effectively. For instance, when analyzing customer service interactions, NLP can identify keywords and phrases commonly associated with fraud, such as requests for unusual transactions or changes to account information. By flagging these communications for further review, companies can take proactive measures to prevent fraud before it escalates.
One of the most significant advantages of using NLP in fraud detection is its capability to process unstructured data. Traditional data analysis methods often struggle with unstructured data, which makes up about 80% of the data generated today. NLP algorithms can analyze this data efficiently, extracting meaningful insights and identifying anomalies that might go unnoticed in structured datasets.
To illustrate the effectiveness of NLP in fraud detection, consider the following table that highlights its applications across different sectors:
Sector | Application of NLP |
---|---|
Banking | Monitoring customer communications for signs of phishing or social engineering attacks. |
Insurance | Analyzing claims documentation for inconsistencies or fraudulent statements. |
E-commerce | Scanning product reviews and customer feedback for fraudulent activities or scams. |
Telecommunications | Detecting fraudulent account access through analysis of customer interactions. |
Furthermore, NLP can enhance the effectiveness of fraud detection systems by utilizing sentiment analysis. This technique assesses the emotional tone behind a series of words, allowing organizations to gauge whether a communication is genuine or potentially deceptive. For example, if a customer suddenly becomes overly defensive or aggressive when questioned about a transaction, NLP can flag this behavior as a red flag for possible fraud.
However, it's essential to recognize that while NLP offers substantial benefits in fraud detection, it is not without its challenges. The accuracy of NLP algorithms heavily relies on the quality of the training data used. If the data is biased or unrepresentative, it can lead to inaccurate results, potentially allowing fraud to slip through the cracks. Therefore, continuous improvement and training of NLP models are crucial to ensure they remain effective against evolving fraud tactics.
In conclusion, as fraudsters become more sophisticated, the need for advanced tools like NLP in fraud detection becomes increasingly vital. By leveraging the power of language analysis, organizations can enhance their fraud detection capabilities, ensuring they stay one step ahead of those looking to exploit vulnerabilities. The future of fraud prevention will undoubtedly be shaped by the integration of NLP, enabling faster, more accurate detection of fraudulent activities.
- What is Natural Language Processing?
NLP is a branch of artificial intelligence that focuses on the interaction between computers and humans through natural language. - How does NLP help in fraud detection?
NLP analyzes text data to identify patterns, keywords, and sentiments that may indicate fraudulent activities. - What are some challenges of using NLP in fraud detection?
Challenges include data quality, algorithm biases, and the need for continuous updates to adapt to new fraud tactics. - Can NLP analyze unstructured data?
Yes, NLP is particularly effective at processing unstructured data, which constitutes a large portion of the information generated today.
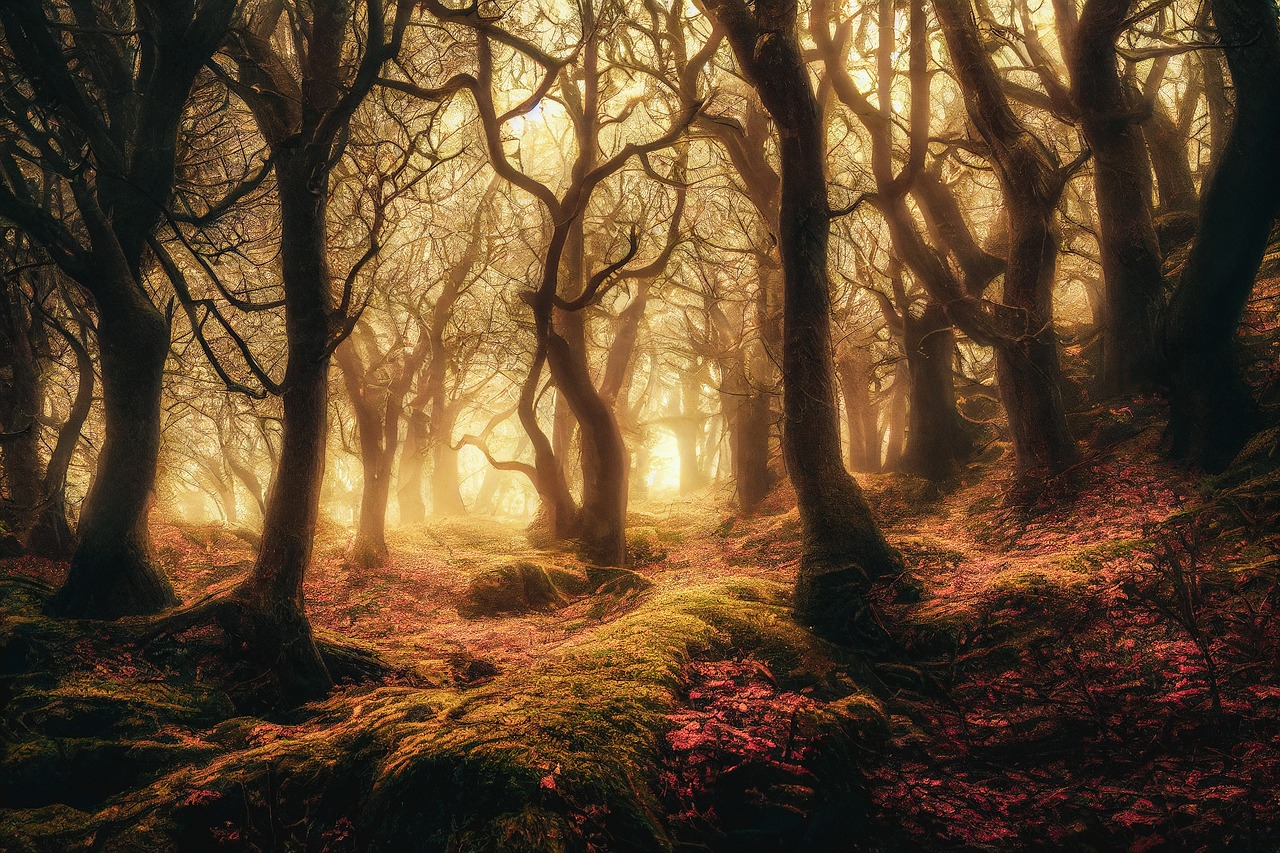
Challenges in AI Fraud Detection
Artificial Intelligence (AI) has revolutionized the landscape of fraud detection, but it is not without its challenges. While AI systems are designed to analyze vast amounts of data and identify suspicious activities, they also face significant hurdles that can impede their effectiveness. One of the primary challenges is data privacy issues. As AI systems require access to large datasets to learn and improve, they often need sensitive personal information. This raises concerns about how this data is collected, stored, and utilized, leading to potential violations of privacy regulations such as the GDPR.
Another pressing issue is the bias in algorithms. AI systems learn from historical data, which can sometimes contain biases that reflect societal inequalities. If these biases are not addressed, they can lead to unfair treatment of certain groups, resulting in false positives or negatives in fraud detection. For example, an AI model trained predominantly on data from one demographic may not perform well when applied to another, causing legitimate transactions to be flagged as fraudulent.
Moreover, the need for continuous model updates is crucial in adapting to evolving fraud tactics. Fraudsters are always finding new ways to exploit systems, and without regular updates and retraining of AI models, these systems can quickly become obsolete. This leads to a constant race between fraud detection technologies and the methods used by fraudsters, requiring organizations to invest in ongoing research and development.
Additionally, the complexity of AI systems can create challenges in transparency and accountability. Many AI algorithms, particularly deep learning models, operate as "black boxes," making it difficult for users to understand how decisions are made. This lack of transparency can lead to distrust among users and stakeholders, as they may question the reliability of the AI's findings. To combat this, organizations must strive for greater transparency in their AI processes and ensure that stakeholders are informed about how these systems function.
Lastly, the integration of AI into existing systems poses its own set of challenges. Organizations may struggle with legacy systems that are not compatible with modern AI technologies, leading to inefficiencies and potential security vulnerabilities. Ensuring that AI systems can work seamlessly with existing infrastructure is essential for maximizing their effectiveness in fraud detection.
In summary, while AI offers powerful tools for detecting and preventing fraud, it is essential to navigate these challenges carefully. Addressing data privacy, algorithm biases, the need for continuous updates, transparency, and integration issues will be crucial for harnessing the full potential of AI in fraud detection.
- What are the main challenges of using AI in fraud detection? The main challenges include data privacy issues, algorithm biases, the need for continuous model updates, lack of transparency, and integration with legacy systems.
- How can organizations address algorithm biases in AI? Organizations can address biases by using diverse training datasets, conducting regular audits of AI systems, and implementing fairness checks to ensure equitable treatment across demographics.
- Why is real-time monitoring important in fraud detection? Real-time monitoring allows organizations to detect and respond to fraudulent activities as they happen, minimizing potential losses and enhancing overall security.
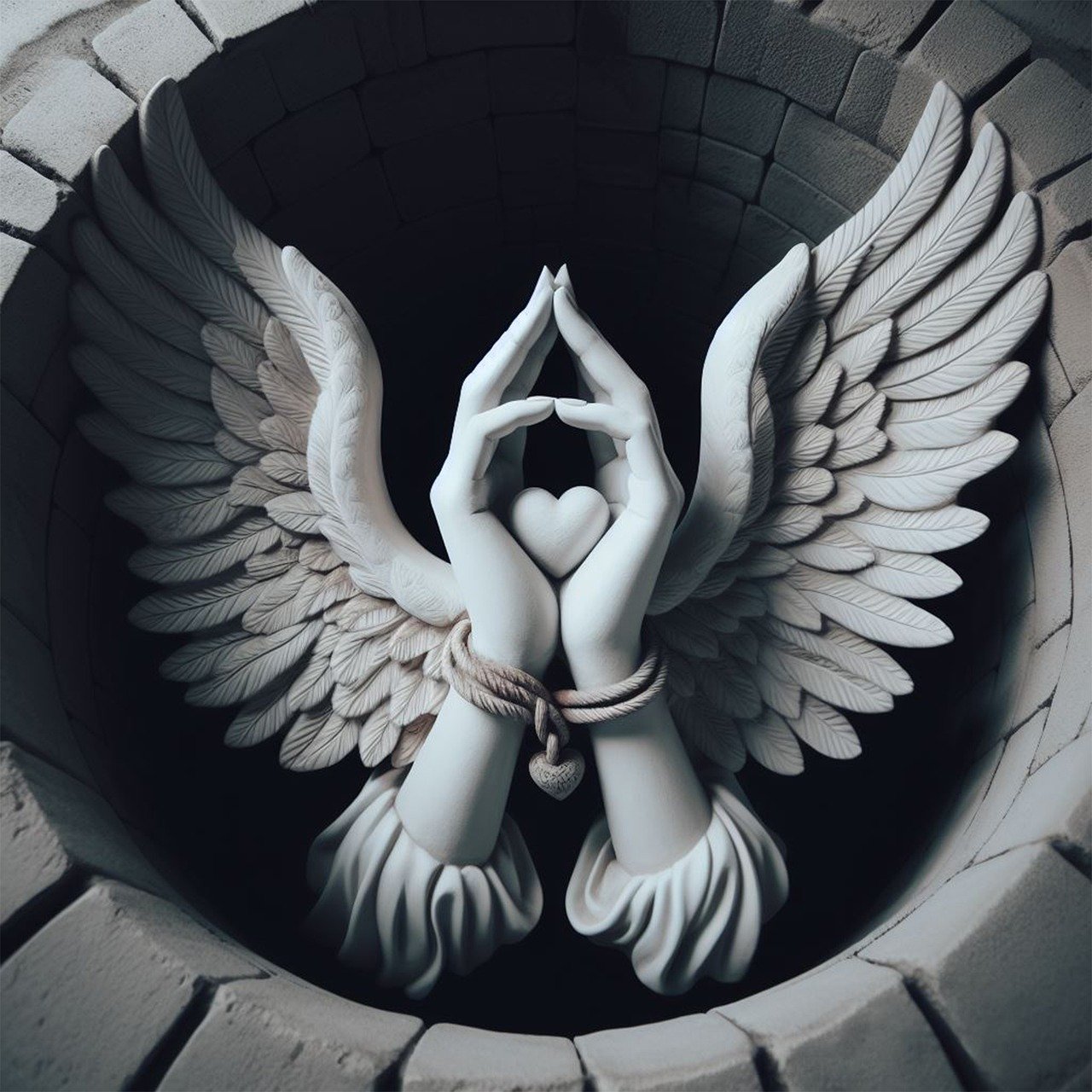
Ethical Considerations
When we delve into the realm of artificial intelligence in fraud detection, it’s crucial to consider the ethical implications that accompany its implementation. As powerful as AI can be, its deployment raises significant questions about transparency, accountability, and even discrimination. These ethical concerns aren't just theoretical; they have real-world implications that can affect individuals and organizations alike.
One major ethical consideration is the potential for algorithmic bias. AI systems learn from historical data, and if that data contains biases—whether related to race, gender, or socioeconomic status—the AI can inadvertently perpetuate or even amplify these biases. For instance, if a fraud detection system is trained on data that disproportionately flags transactions from certain demographics as suspicious, it may lead to unfair treatment of those groups in real-world applications. This can create a cycle of mistrust and inequity, undermining the very purpose of fraud detection.
Moreover, the issue of data privacy is paramount. AI systems often require access to vast amounts of personal data to function effectively. This raises questions about how that data is collected, used, and stored. Are individuals aware that their data is being used for fraud detection? Do they have control over it? The lack of transparency in these processes can lead to a significant erosion of trust between consumers and organizations. Companies must navigate these waters carefully, ensuring that they respect user privacy while still leveraging the power of AI.
Another ethical consideration is the need for accountability. If an AI system incorrectly flags a legitimate transaction as fraudulent, who is responsible? Is it the developers who created the algorithm, the organization that deployed it, or the AI itself? Establishing clear lines of accountability is essential to address these issues and ensure that individuals have recourse if they are adversely affected by AI decisions.
To address these ethical concerns, organizations can adopt several best practices:
- Regular audits: Conducting regular audits of AI systems can help identify and mitigate biases.
- Transparency: Providing clear information about how AI systems operate can foster trust.
- Stakeholder engagement: Involving diverse stakeholders in the development process can help ensure that multiple perspectives are considered.
As we look to the future of AI in fraud detection, it's clear that ethical considerations will play a pivotal role in shaping its landscape. Organizations must prioritize these issues to not only enhance their fraud detection capabilities but also to build and maintain trust with their customers. By addressing these ethical challenges head-on, we can harness the power of AI responsibly and effectively.
Q1: What are the main ethical concerns regarding AI in fraud detection?
A1: The main ethical concerns include algorithmic bias, data privacy, and accountability for AI decisions.
Q2: How can organizations mitigate bias in AI fraud detection systems?
A2: Organizations can conduct regular audits of their AI systems, ensure transparency in their operations, and engage diverse stakeholders in the development process.
Q3: Why is data privacy important in AI fraud detection?
A3: Data privacy is crucial because AI systems often require access to personal data, and individuals should have control over how their data is used and stored.
Q4: Who is responsible if an AI system wrongly flags a legitimate transaction?
A4: Accountability can be complex; it may involve the developers, the organization using the AI, or both, depending on the situation.
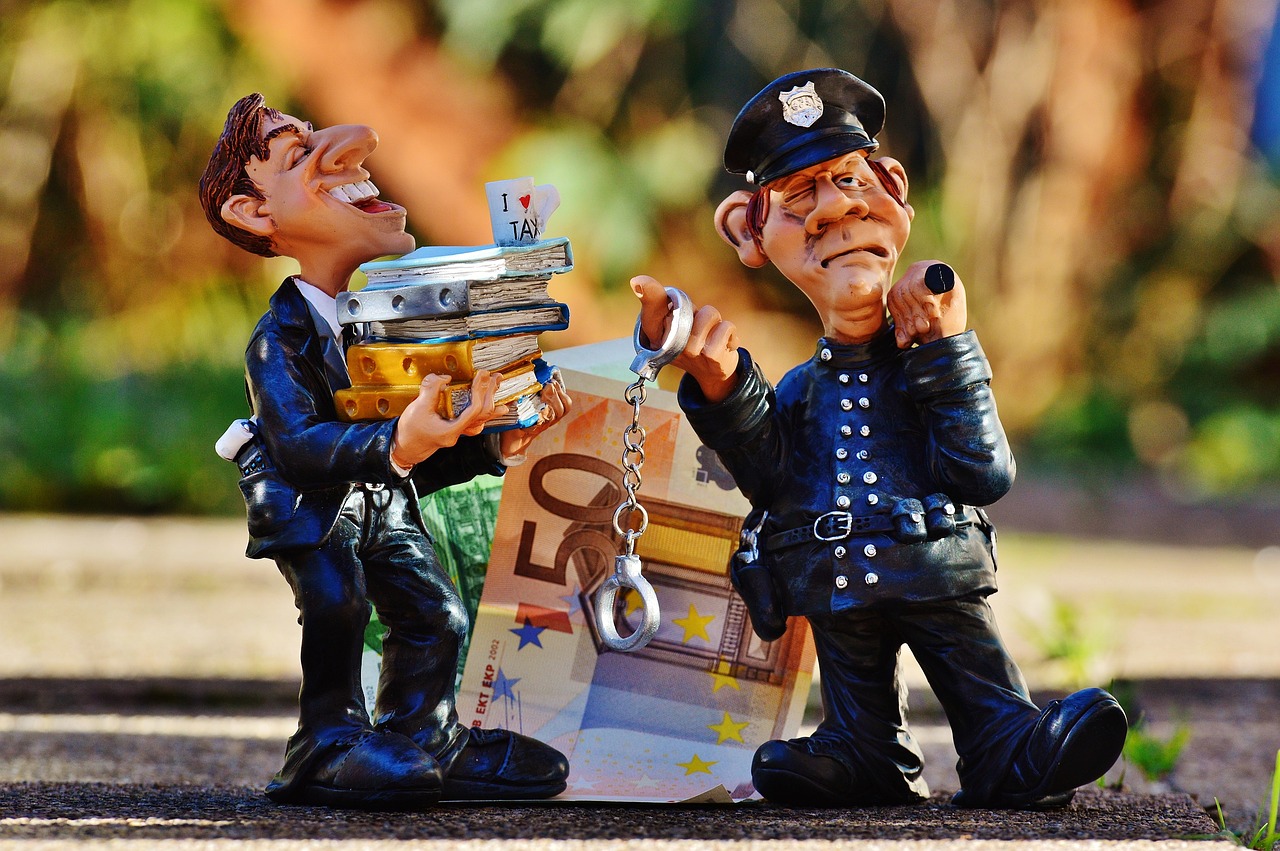
Future Trends in AI Fraud Prevention
As we look towards the horizon of artificial intelligence in fraud prevention, it's clear that the landscape is evolving at a breathtaking pace. The integration of AI technologies is not just a trend; it's becoming a fundamental necessity for businesses aiming to safeguard their operations against fraudulent activities. One of the most exciting developments is the rise of deep learning algorithms. These advanced models can analyze vast datasets with remarkable accuracy, allowing for the identification of subtle patterns that traditional methods might miss. Imagine having a digital detective that learns from every case it encounters, becoming more adept at spotting fraud with each passing day!
Moreover, the incorporation of blockchain technology into fraud prevention strategies is another trend that is gaining traction. By providing a secure and transparent way to record transactions, blockchain can help in verifying the authenticity of data, making it significantly harder for fraudsters to manipulate records. Think of blockchain as a digital fingerprint for transactions – unique and unchangeable, ensuring that every action is traceable. This level of transparency not only aids in fraud detection but also builds trust among consumers.
Additionally, as cybersecurity threats continue to evolve, the importance of real-time data analysis cannot be overstated. AI systems are now capable of monitoring transactions as they occur, allowing for immediate responses to suspicious activities. Picture this: a bank transaction is flagged the moment it deviates from a customer's typical behavior. This proactive approach can significantly reduce the window of opportunity for fraudsters, effectively closing the door on many potential scams.
Furthermore, the use of Natural Language Processing (NLP) is becoming increasingly crucial in identifying fraudulent communications. With the ability to analyze text data from emails, chat logs, and social media, NLP can detect patterns and keywords that may indicate fraudulent intent. This capability is particularly valuable in sectors like finance and e-commerce, where communication plays a pivotal role in transactions. Imagine a system that can read between the lines, picking up on subtle cues that suggest deceit – that's the power of NLP in action!
However, as we embrace these advancements, we must also remain vigilant about the challenges they present. The need for continuous updates and training of AI models is paramount to keep pace with the ever-changing tactics employed by fraudsters. Moreover, ethical considerations surrounding data privacy and algorithmic biases must be addressed to ensure that AI applications are not only effective but also fair and transparent. As we forge ahead, the balance between innovation and ethics will be crucial in shaping the future of AI in fraud prevention.
- What is the role of deep learning in fraud detection?
Deep learning algorithms can process vast amounts of data and identify complex patterns that may indicate fraudulent behavior, enhancing the accuracy of fraud detection systems. - How does blockchain technology contribute to fraud prevention?
Blockchain provides a secure and transparent way to record transactions, making it difficult for fraudsters to alter data and ensuring that all activities are traceable. - Why is real-time monitoring important in fraud prevention?
Real-time monitoring allows organizations to detect and respond to fraudulent activities as they happen, significantly reducing potential losses. - What is Natural Language Processing (NLP) and how is it used in fraud detection?
NLP analyzes text data to identify patterns and keywords that may indicate fraudulent communications, helping organizations to detect scams early. - What are the ethical considerations in using AI for fraud detection?
Ethical considerations include data privacy, algorithmic biases, and the need for transparency and accountability in AI applications.
Frequently Asked Questions
- What is AI's role in fraud detection?
AI plays a crucial role in fraud detection by utilizing advanced algorithms and machine learning techniques to analyze vast amounts of data. It helps identify suspicious activities and patterns that may indicate fraudulent behavior, allowing organizations to respond quickly and effectively to potential threats.
- How do machine learning techniques enhance fraud detection?
Machine learning techniques, such as supervised and unsupervised learning, improve fraud detection accuracy by enabling systems to learn from historical data. This allows them to recognize patterns and anomalies that are indicative of fraud, making it easier to flag suspicious transactions in real-time.
- What is the importance of data analysis in AI fraud detection?
Data analysis is vital in AI fraud detection as it helps uncover hidden patterns and trends within large datasets. By analyzing this data, AI systems can detect anomalies that human analysts might miss, thus enhancing the overall effectiveness of fraud prevention efforts.
- How does real-time monitoring work in fraud prevention?
Real-time monitoring involves continuously analyzing data streams to detect fraudulent activities as they occur. AI systems utilize this capability to respond instantly to suspicious transactions, significantly reducing the chances of fraud going undetected.
- What challenges does AI face in fraud detection?
Despite its advantages, AI in fraud detection faces several challenges, including data privacy concerns, algorithmic biases, and the necessity for continuous updates to adapt to new fraud tactics. These challenges can hinder the effectiveness of AI systems if not properly addressed.
- What ethical considerations are involved in using AI for fraud detection?
Ethical considerations include transparency, accountability, and the potential for discrimination in AI algorithms. It is essential to ensure that AI systems are designed and implemented in a way that upholds ethical standards and protects user rights.
- What future trends can we expect in AI fraud prevention?
Future trends in AI fraud prevention may include advancements in deep learning, integration with blockchain technology, and heightened emphasis on cybersecurity measures. These developments are expected to enhance the capabilities of AI systems in combating fraud effectively.