Ethical Practices in AI: A Business Imperative
In today's fast-paced digital landscape, the integration of artificial intelligence (AI) into business operations is no longer a futuristic concept; it is a reality that many companies are embracing. However, with great power comes great responsibility. As organizations harness the capabilities of AI, they must also confront the ethical implications of their technologies. This brings us to the crux of the matter: ethical practices in AI are not just a nice-to-have; they are a business imperative.
Imagine walking into a store where every item is tailored to your preferences, thanks to AI algorithms. Sounds amazing, right? But what if those algorithms inadvertently favor certain demographics over others? This is where the importance of ethical considerations comes into play. By prioritizing ethics in AI, businesses can build trust with their customers, ensuring that their systems are fair, transparent, and accountable.
Moreover, as regulations around AI continue to evolve, staying ahead of the curve becomes essential. Companies that adopt ethical practices not only mitigate legal risks but also position themselves as leaders in responsible innovation. In a world where consumers are increasingly aware of and concerned about the implications of AI, organizations that prioritize ethics will stand out from the competition.
Furthermore, fostering an ethical culture within an organization is crucial. When employees understand the significance of ethical AI practices, they become advocates for responsible innovation. This internal alignment not only enhances the overall reputation of the company but also drives better outcomes for society as a whole.
In conclusion, ethical practices in AI are not merely an obligation; they are a strategic advantage. By embedding ethics into the core of AI development and deployment, businesses can navigate the complexities of this rapidly evolving field while ensuring that they contribute positively to society. As we explore the various facets of ethical AI practices throughout this article, remember that the choices we make today will shape the future of technology and its impact on our lives.
Understanding why ethical considerations are crucial in AI development can help businesses navigate potential pitfalls and enhance their reputation while promoting responsible innovation in their operations. With the rapid advancement of AI technologies, the potential for misuse or unintended consequences is significant. Ethical frameworks provide a roadmap for organizations to follow, ensuring that they are not only compliant with regulations but are also acting in the best interest of their stakeholders.
By prioritizing ethical practices, companies can:
- Build trust with customers and stakeholders
- Enhance their brand reputation
- Ensure compliance with emerging regulations
- Drive innovation that is responsible and inclusive
Identifying and implementing key ethical principles, such as fairness, transparency, and accountability, is essential for organizations aiming to create responsible AI systems that benefit society as a whole. These principles serve as guiding lights, helping businesses navigate the complexities of AI technology while ensuring they remain aligned with societal values.
Ensuring fairness in AI algorithms involves mitigating biases that can lead to discriminatory outcomes, thereby promoting inclusivity and equity in decision-making processes across various applications. For instance, in hiring algorithms, it is crucial to ensure that candidates from diverse backgrounds are evaluated fairly, without bias towards any particular group.
Addressing algorithmic bias requires ongoing assessment and refinement of AI models to ensure they operate equitably across diverse populations and avoid perpetuating historical inequalities. This may involve regular audits of algorithms and the implementation of corrective measures where necessary.
Implementing inclusive data practices helps organizations gather diverse datasets, which are crucial for training AI systems that reflect the needs and perspectives of all stakeholders. This not only enhances the accuracy of AI models but also ensures that the resulting technologies are beneficial for a wider audience.
Transparency in AI systems fosters trust among users and stakeholders, enabling them to understand how decisions are made and the data driving those decisions. When consumers know how their data is used and how algorithms function, they are more likely to engage with AI technologies positively.
Establishing clear accountability frameworks ensures that organizations can be held responsible for the outcomes of their AI systems, reinforcing ethical practices and public confidence. This includes defining roles and responsibilities within the organization and ensuring that there are mechanisms for addressing grievances related to AI decisions.
Staying compliant with emerging regulations related to AI is vital for businesses to avoid legal repercussions and maintain ethical standards in their technological advancements. As governments worldwide begin to impose stricter regulations on AI, organizations must be proactive in adapting their practices to meet these new requirements.
Cultivating an ethical AI culture within organizations encourages employees to prioritize ethical considerations in their work, leading to more responsible AI development and deployment practices. This culture can be fostered through training, open discussions about ethical dilemmas, and a commitment to continuous improvement in ethical practices.
- Why is ethics important in AI? Ethics in AI is crucial to prevent biases, build trust, and ensure compliance with regulations.
- What are the key principles of ethical AI? The key principles include fairness, transparency, and accountability.
- How can organizations address algorithmic bias? Organizations can address bias through regular audits and inclusive data practices.
- What role does transparency play in AI? Transparency fosters trust and helps users understand AI decision-making processes.
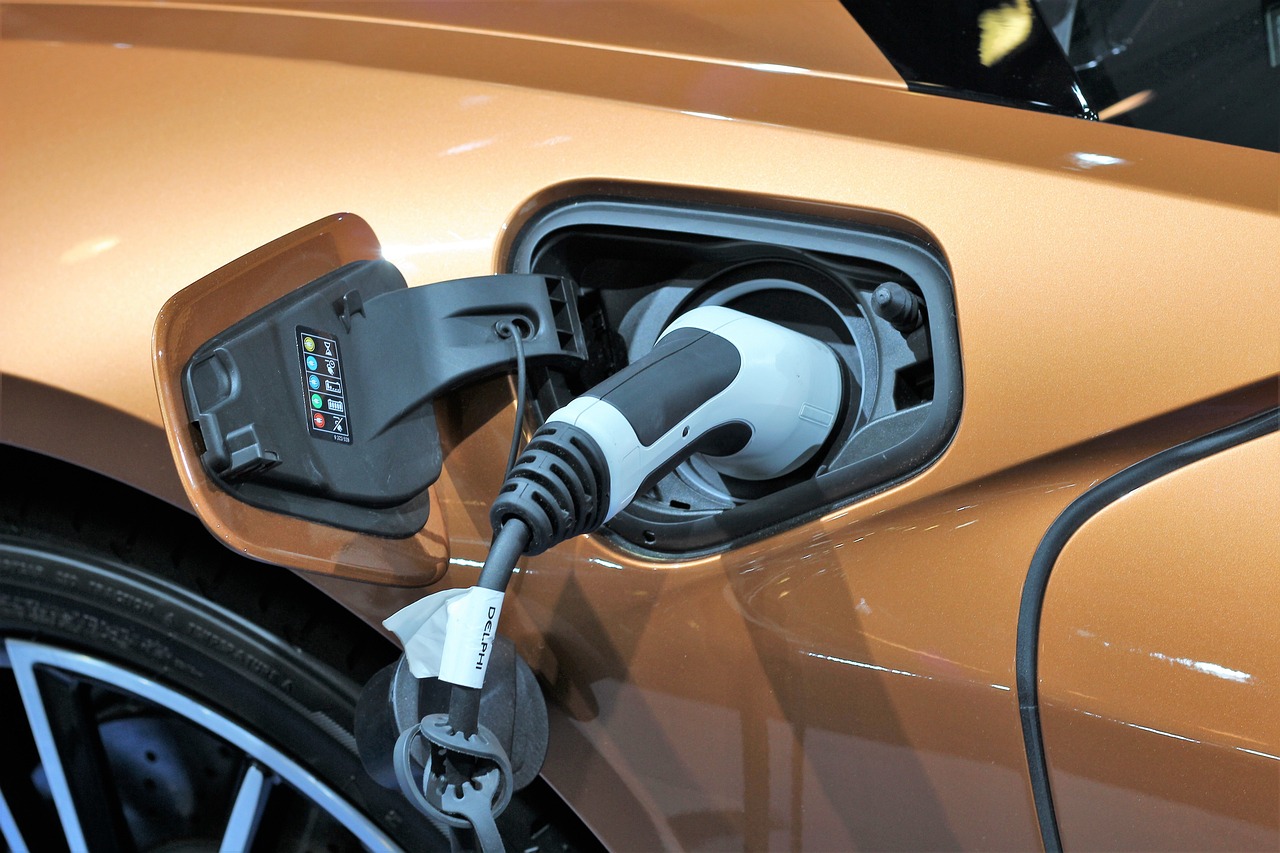
The Importance of Ethics in AI
In today's fast-paced technological landscape, the significance of ethical practices in artificial intelligence cannot be overstated. As businesses increasingly integrate AI into their operations, they face a plethora of challenges that require not just technical expertise but also a strong moral compass. Why is this so crucial? Well, consider this: AI systems are not just lines of code; they have the potential to influence lives, shape decisions, and impact society in profound ways. Therefore, understanding the ethical implications of AI is essential for any organization aiming to thrive in this digital age.
When we talk about ethics in AI, we're diving into a realm that encompasses trust, compliance, and innovation. Trust is the bedrock of any successful business relationship. If customers and stakeholders believe that an organization is using AI responsibly, they are more likely to engage with it. On the flip side, any hint of unethical practices can lead to a loss of credibility and a tarnished reputation that may take years to rebuild.
Moreover, compliance with emerging regulations is critical. As governments and regulatory bodies around the world begin to draft laws governing AI use, businesses that prioritize ethical practices will find themselves ahead of the curve. They won’t just be avoiding legal pitfalls; they will also be setting themselves up as leaders in responsible innovation. This proactive stance can open doors to new opportunities, partnerships, and markets that may be inaccessible to less scrupulous competitors.
Finally, fostering an environment where ethical considerations are at the forefront can lead to a culture of innovation. When employees feel empowered to think critically about the implications of their work, they are more likely to come up with creative solutions that benefit not just the company but society as a whole. This kind of culture can be a game changer, allowing organizations to harness the full potential of AI while ensuring that its deployment is done responsibly.
In summary, the importance of ethics in AI extends far beyond mere compliance or public relations. It’s about building a foundation of trust, ensuring accountability, and fostering a culture of responsible innovation. As we move forward in this exciting yet complex field, organizations must recognize that ethical practices are not just an option; they are a business imperative.
- Why are ethical practices important in AI? Ethical practices in AI are crucial for building trust, ensuring compliance with regulations, and fostering a culture of responsible innovation.
- What are some key ethical principles in AI? Key principles include fairness, transparency, and accountability, which help organizations create responsible AI systems.
- How can businesses ensure their AI systems are fair? By addressing algorithmic bias and implementing inclusive data practices, businesses can promote fairness in their AI systems.
- What role does transparency play in AI? Transparency helps users understand how AI decisions are made, which fosters trust among stakeholders.
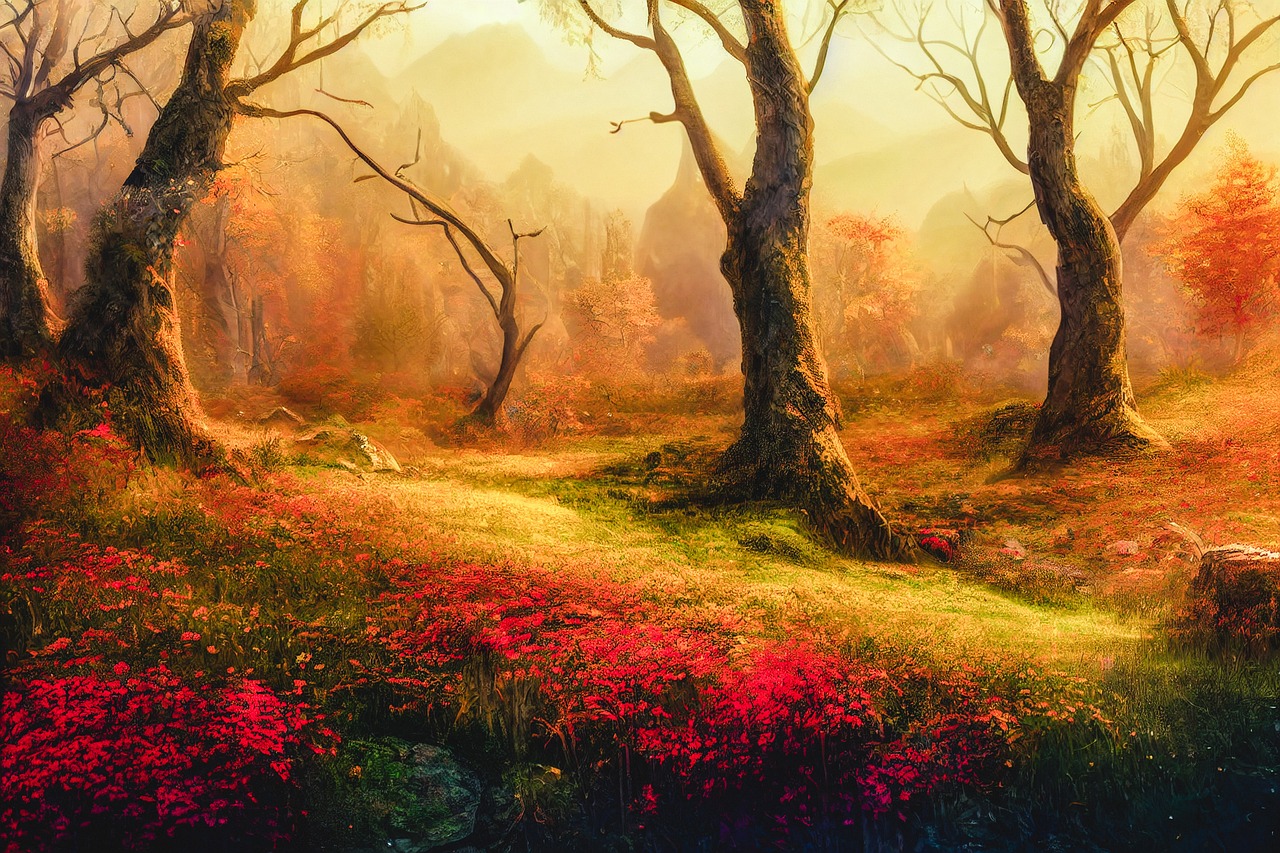
Key Ethical Principles for AI Development
When diving into the world of artificial intelligence, it's crucial for businesses to anchor their development practices on a foundation of strong ethical principles. These principles not only guide the creation of AI systems but also ensure that these systems operate in a way that is beneficial to society. The key ethical principles for AI development include fairness, transparency, and accountability. Each of these principles plays a vital role in shaping responsible AI practices that can build trust and foster innovation.
Fairness in AI is about ensuring that algorithms do not perpetuate biases that could lead to discriminatory outcomes. Imagine an AI system that decides who gets a loan based on historical data that reflects societal inequalities. Without a fairness lens, such a system could inadvertently reinforce those disparities, leaving marginalized groups at a disadvantage. Thus, it is essential for organizations to actively seek ways to mitigate bias in their algorithms. This requires a deep understanding of the data being used and the implications of its use.
To achieve fairness, organizations should implement inclusive data practices. This means gathering diverse datasets that accurately reflect the demographics of the population affected by the AI system. By doing so, AI can be trained to recognize and respect the varied needs and perspectives of all stakeholders involved. This approach not only enhances the performance of AI systems but also promotes a sense of equity and inclusivity in decision-making processes.
Next, we have transparency, which is vital for building trust among users and stakeholders. When AI systems are transparent, users can understand how decisions are made and what data drives those decisions. This understanding is crucial, especially in high-stakes applications like healthcare or criminal justice, where the consequences of AI decisions can significantly impact people's lives. Organizations should strive to create clear documentation and communication strategies that outline the workings of their AI systems, making it easier for users to grasp the rationale behind AI-driven outcomes.
Finally, accountability in AI deployment cannot be overlooked. Establishing clear accountability frameworks ensures that organizations can be held responsible for the outcomes of their AI systems. This not only reinforces ethical practices but also enhances public confidence in AI technologies. Companies should develop policies that outline who is responsible for the decisions made by AI, how those decisions are monitored, and what steps are taken in case of adverse outcomes. By doing so, businesses can demonstrate their commitment to ethical AI practices and build a reputation as responsible innovators.
In summary, the key ethical principles of fairness, transparency, and accountability are essential for organizations aiming to create responsible AI systems. By embedding these principles into their development processes, businesses can navigate the complexities of AI while ensuring that their innovations are aligned with societal values and ethics.
- What is the significance of fairness in AI?
Fairness in AI ensures that algorithms do not reinforce existing biases, promoting equitable outcomes for all users. - How can organizations ensure transparency in AI?
Organizations can ensure transparency by providing clear documentation and communication about how AI systems make decisions. - Why is accountability important in AI development?
Accountability ensures that organizations can be held responsible for their AI systems' outcomes, reinforcing trust and ethical practices.
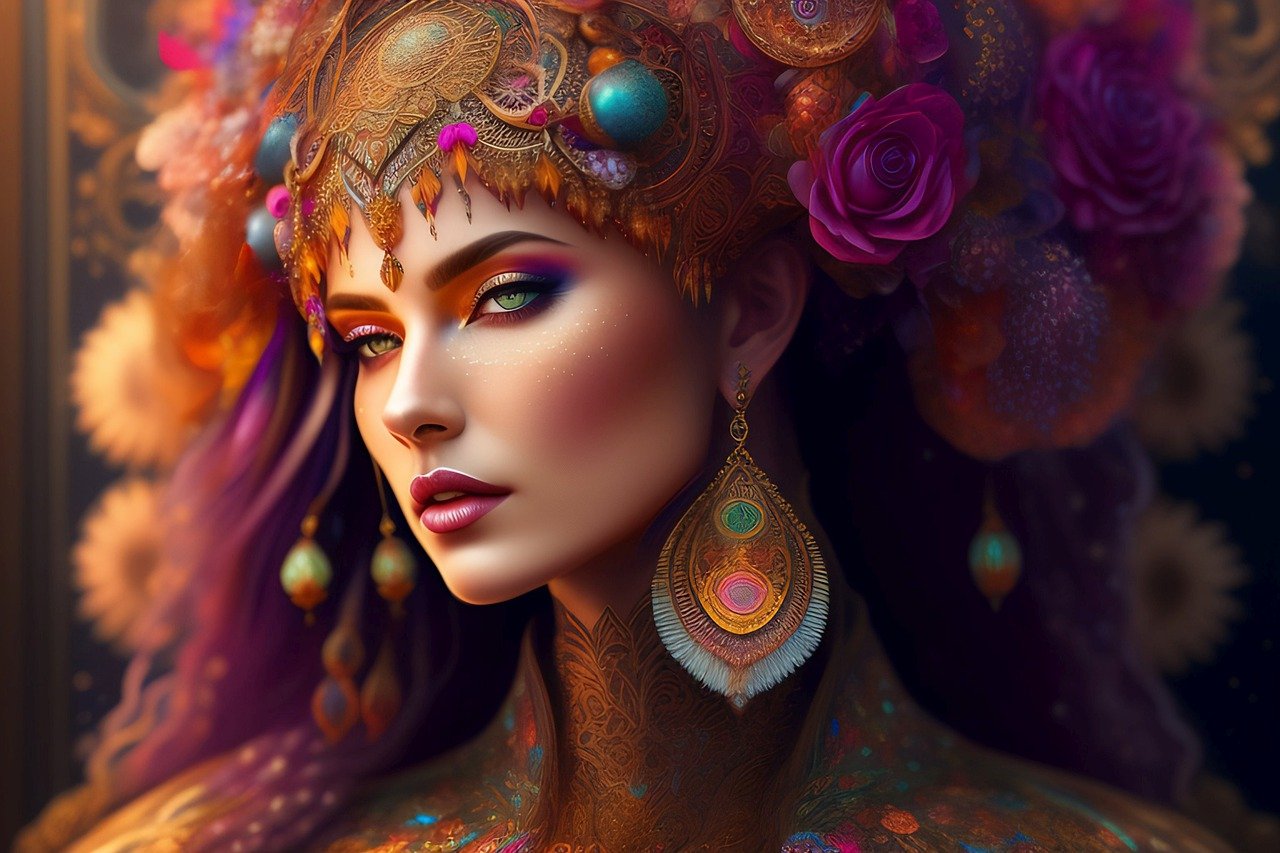
Fairness in AI Algorithms
In the realm of artificial intelligence, fairness isn't just a buzzword; it's a crucial pillar that shapes the integrity of AI systems. Imagine a world where decisions about jobs, loans, or even criminal justice are influenced by algorithms that might carry biases, leading to unfair treatment of certain groups. This is the stark reality we face if fairness in AI algorithms is overlooked. Ensuring fairness is about more than just ethical responsibility—it's about fostering a sense of trust and equity in the technologies that increasingly govern our lives.
When we talk about fairness in AI, we're essentially addressing the potential biases that can creep into algorithms during their development. These biases can arise from various sources, such as skewed training data or the subjective choices made by developers. If an AI system is trained on data that reflects historical prejudices, it may inadvertently perpetuate those biases, leading to discriminatory outcomes. For instance, if a hiring algorithm is trained predominantly on data from successful candidates who fit a specific demographic, it might unfairly disadvantage applicants from other backgrounds. This scenario underscores the importance of actively working towards equitable AI solutions.
One effective approach to ensuring fairness is to implement ongoing assessments of AI models. This means regularly evaluating algorithms to identify and address any biases that may emerge over time. Just like we wouldn't drive a car without checking the brakes, we shouldn't deploy AI systems without scrutinizing their fairness. Organizations must commit to refining their models continuously, adapting them to reflect changes in societal norms and values.
Moreover, inclusive data practices play a vital role in achieving fairness. By gathering diverse datasets that represent a broad spectrum of experiences and perspectives, businesses can train AI systems that are more reflective of the society they serve. This could involve actively seeking out data from underrepresented groups or employing techniques to balance datasets to minimize bias. As a result, AI systems can make decisions that are not only fairer but also more relevant to a wider audience.
Ultimately, the journey towards fairness in AI algorithms is a collaborative effort. It requires input from a diverse range of stakeholders, including ethicists, data scientists, and community representatives. By fostering a culture of inclusivity and accountability, organizations can build AI systems that are not only effective but also just. The question remains: are we ready to take these necessary steps to ensure that our AI technologies serve everyone fairly?
- What is algorithmic bias? Algorithmic bias refers to systematic and unfair discrimination that can occur in AI systems due to biased data or flawed algorithms.
- How can organizations ensure fairness in AI? Organizations can ensure fairness by conducting regular audits of their AI systems, using diverse datasets, and involving stakeholders from various backgrounds in the development process.
- Why is fairness important in AI algorithms? Fairness is vital in AI algorithms to promote trust, prevent discrimination, and ensure that technologies benefit all segments of society.
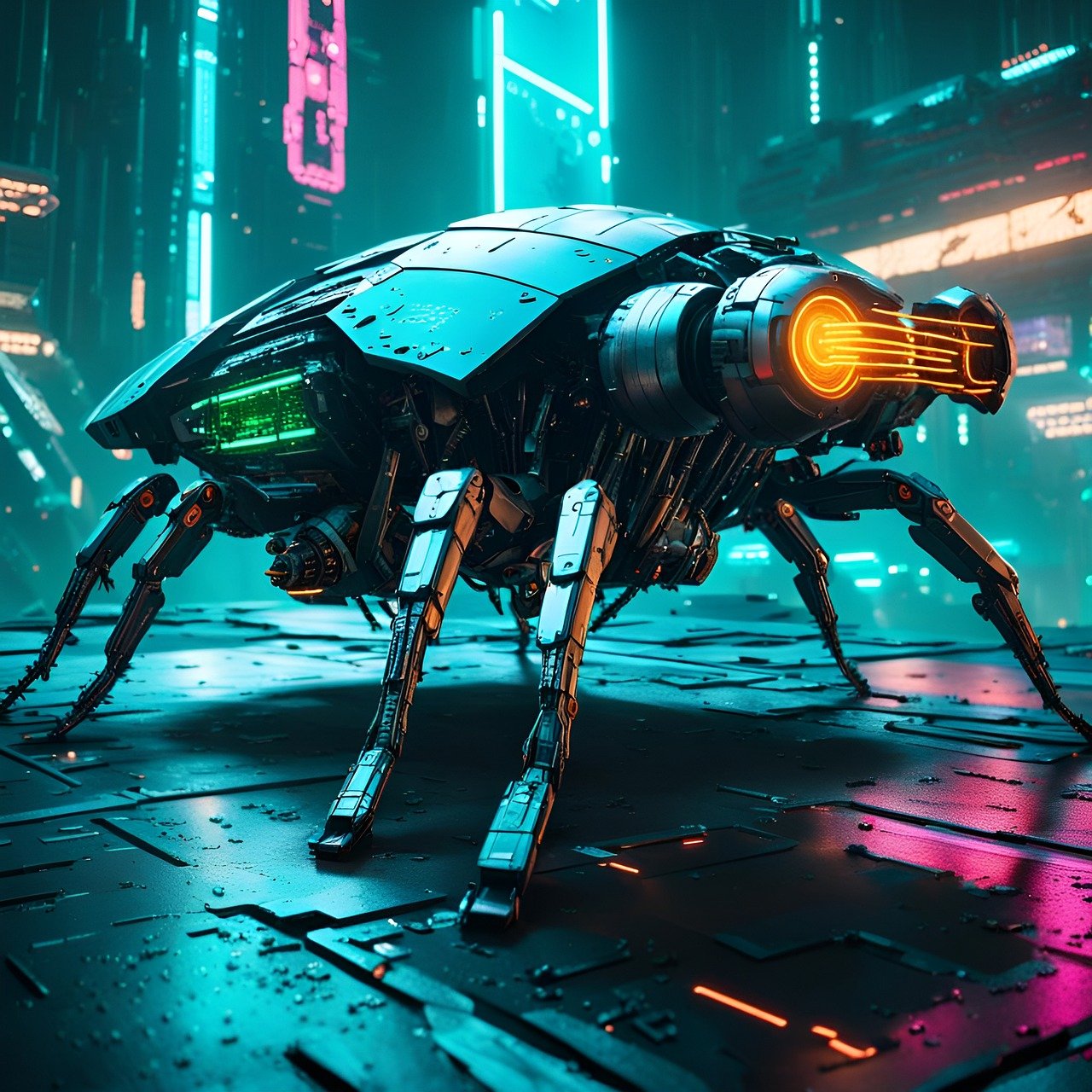
Addressing Algorithmic Bias
Algorithmic bias is a significant challenge in the realm of artificial intelligence, where the very systems designed to enhance decision-making can inadvertently perpetuate discrimination. This occurs when the data used to train AI models reflects historical inequalities or societal biases. For instance, if an AI system is trained on data that predominantly features one demographic, it may struggle to accurately represent or serve others. This not only undermines the integrity of the AI but also poses ethical concerns for businesses that rely on these technologies.
To effectively address algorithmic bias, organizations must engage in a process of ongoing assessment and refinement of their AI models. This involves regularly auditing algorithms to identify and mitigate any biases that may arise. A proactive approach includes employing diverse teams during the development phase, as varied perspectives can help illuminate potential biases that a homogenous group might overlook. Furthermore, businesses should implement robust feedback mechanisms where users can report discriminatory outcomes, allowing for continuous improvement.
Another critical aspect of addressing algorithmic bias is the implementation of inclusive data practices. By gathering diverse datasets that reflect a wide range of perspectives and experiences, organizations can train AI systems that are more representative of the population they serve. This means not just collecting data from various demographics but also ensuring that the data is of high quality and free from historical prejudices. For example, in a hiring algorithm, if the training data predominantly includes successful candidates from a specific background, the AI might favor that background in future hiring decisions. To counteract this, businesses should actively seek out and include data from underrepresented groups.
Moreover, organizations can utilize techniques such as adversarial debiasing, which involves training models to minimize bias while maximizing performance. This method allows AI systems to learn from their mistakes and adapt, leading to fairer outcomes. It’s essential for companies to recognize that addressing algorithmic bias is not a one-time fix but a continuous journey that requires vigilance and commitment.
In conclusion, addressing algorithmic bias is not merely an ethical obligation but a business imperative. Organizations that prioritize fairness and inclusivity in their AI systems not only enhance their reputation but also build trust with their users. By fostering an environment where diverse perspectives are valued and actively seeking to mitigate biases, businesses can lead the way in responsible AI development.
- What is algorithmic bias? Algorithmic bias refers to systematic and unfair discrimination in AI algorithms that can arise from biased training data or flawed model design.
- How can businesses identify algorithmic bias? Businesses can identify algorithmic bias by conducting regular audits of their AI systems, analyzing outcomes across different demographics, and soliciting feedback from users.
- What role does diverse data play in mitigating bias? Diverse data helps ensure that AI systems are trained on a wide range of perspectives, reducing the likelihood of biased outcomes and promoting fairness.
- Is addressing algorithmic bias a one-time effort? No, addressing algorithmic bias is an ongoing process that requires continuous monitoring, assessment, and refinement of AI systems.
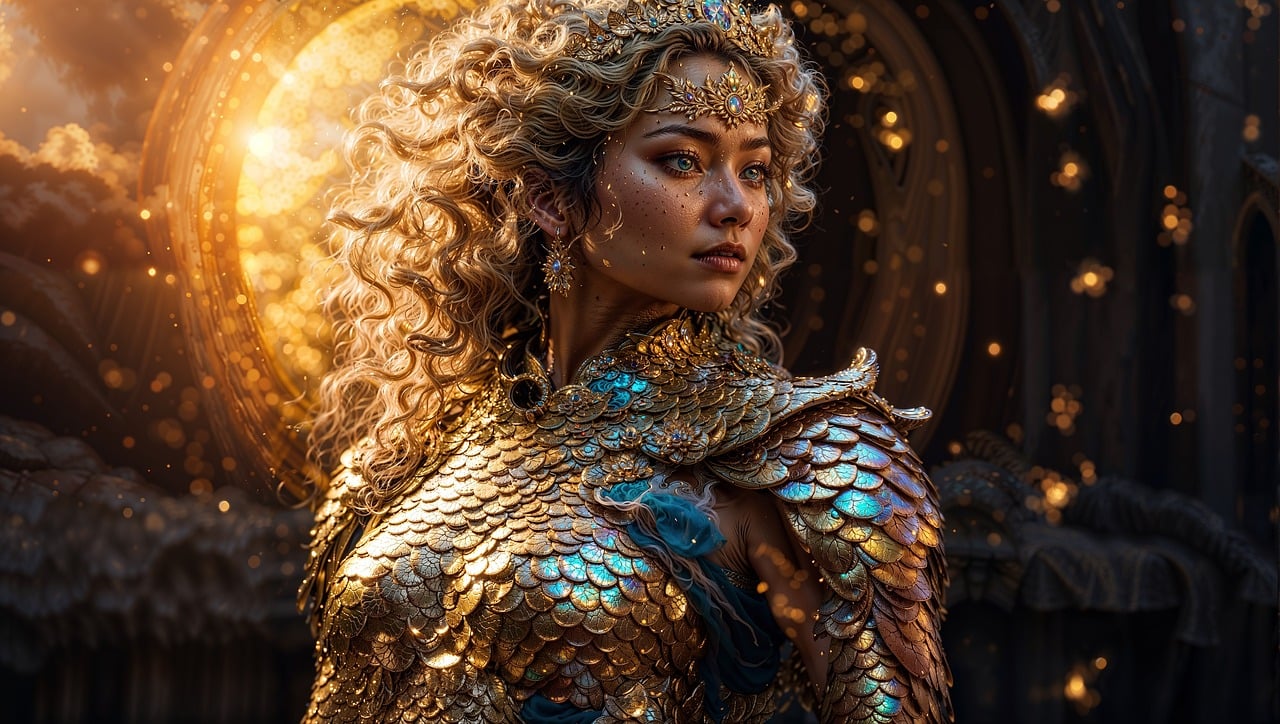
Inclusive Data Practices
When it comes to developing robust artificial intelligence systems, are not just a nice-to-have—they're a fundamental necessity. Imagine trying to solve a complex puzzle, but you only have half of the pieces. That's what it feels like when AI systems are trained on non-inclusive datasets. In essence, ensure that the data used to train AI models reflects the diversity of the real world, capturing a wide array of perspectives, experiences, and backgrounds.
One of the primary challenges in AI development is the risk of algorithmic bias, which can occur when the data used to train these systems is skewed or unrepresentative. For instance, if an AI model is predominantly trained on data from one demographic group, it may not perform well for individuals outside that group. This can lead to unfair outcomes, such as biased hiring practices or inequitable healthcare recommendations. By embracing inclusive data practices, organizations can mitigate these risks and promote fairness and equity across their AI applications.
To implement inclusive data practices effectively, businesses should consider the following strategies:
- Diverse Data Collection: Actively seek out and include data from a wide range of sources and demographic groups. This can involve reaching out to community organizations or utilizing platforms that cater to underrepresented populations.
- Regular Data Audits: Conduct frequent audits of the datasets being used to identify any potential biases or gaps. This practice ensures that the data remains relevant and comprehensive as societal norms and values evolve.
- Stakeholder Engagement: Involve diverse stakeholders in the data collection process. Their insights can help identify crucial data points that may otherwise be overlooked.
Moreover, fostering an environment where feedback is encouraged can significantly enhance the inclusivity of data practices. Organizations should create channels for stakeholders to voice their concerns or suggestions regarding data usage. This not only improves the quality of the data but also builds trust among users, who feel their perspectives are valued and considered.
Ultimately, the goal of inclusive data practices is not just to comply with ethical standards but to create AI systems that genuinely serve the needs of all individuals. By prioritizing inclusivity in data collection and analysis, businesses can pave the way for more equitable AI solutions, ensuring that technology advances in a way that benefits everyone, rather than perpetuating existing inequalities.
- What are inclusive data practices? Inclusive data practices involve collecting and utilizing diverse datasets that represent various demographic groups to train AI systems effectively.
- Why are inclusive data practices important? They help mitigate algorithmic bias, ensuring fair and equitable outcomes in AI applications while reflecting the needs of a diverse population.
- How can organizations implement inclusive data practices? Organizations can implement these practices by diversifying data sources, conducting regular audits, and engaging stakeholders in the data collection process.
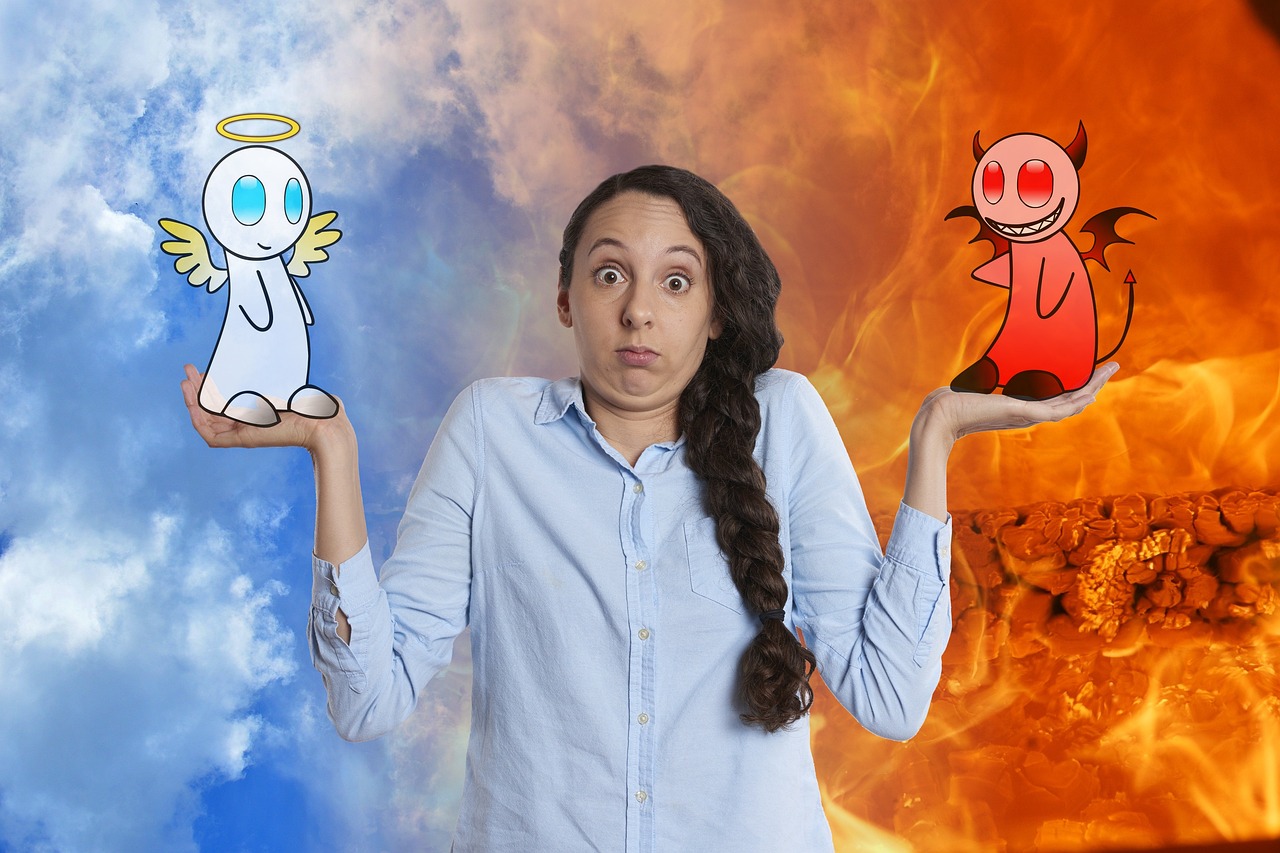
Transparency in AI Systems
In today's digital age, has become more than just a buzzword; it’s a fundamental requirement for building trust with users and stakeholders. Imagine navigating a maze without knowing the layout—frustrating, right? That’s how users often feel when AI systems operate in a black box. When organizations embrace transparency, they illuminate the path, allowing users to understand how decisions are made and what data drives those decisions. This understanding is crucial, not just for user trust, but also for fostering an environment where innovation can thrive.
Transparency in AI can be dissected into several key components. First, organizations must communicate the logic behind their algorithms clearly. This means explaining how data is processed and what factors influence outcomes. For instance, if an AI system is used for hiring, it should be clear how candidates are evaluated and selected. Are certain qualifications weighted more heavily than others? Is there any inherent bias in the data used? Providing this information empowers users and helps them feel more confident in the technology they are engaging with.
Another vital aspect of transparency is the accessibility of information. It’s not enough to simply state how an AI system works; the information must be presented in a way that is easily understandable. This can be achieved through user-friendly interfaces, comprehensive documentation, and even educational resources that explain complex concepts in layman's terms. For example, creating infographics or short videos can demystify the technology, making it more approachable for everyone.
Moreover, organizations should consider implementing feedback mechanisms that allow users to voice their concerns or experiences with AI systems. This two-way communication fosters a culture of transparency and shows that the organization values user input. When users feel heard, they are more likely to trust the systems in place.
To encapsulate the significance of transparency in AI, here’s a brief overview:
Aspect | Importance |
---|---|
Clarity of Algorithms | Helps users understand decision-making processes |
Accessibility of Information | Ensures users can easily grasp complex concepts |
Feedback Mechanisms | Encourages user engagement and trust |
In conclusion, transparency is not just a nice-to-have in AI systems; it’s a necessity. By fostering an environment where users can understand and engage with AI technologies, organizations not only build trust but also pave the way for more responsible and ethical AI development. As we continue to innovate, let’s ensure that transparency remains at the forefront of our efforts, guiding us toward a future where technology serves all of society effectively and equitably.
- What is transparency in AI? Transparency in AI refers to the clarity and openness with which AI systems operate, allowing users to understand how decisions are made and what data influences those decisions.
- Why is transparency important? Transparency builds trust, encourages user engagement, and helps organizations identify and rectify biases in their AI systems.
- How can organizations improve transparency? Organizations can improve transparency by clearly communicating the logic behind their algorithms, making information accessible, and implementing feedback mechanisms.
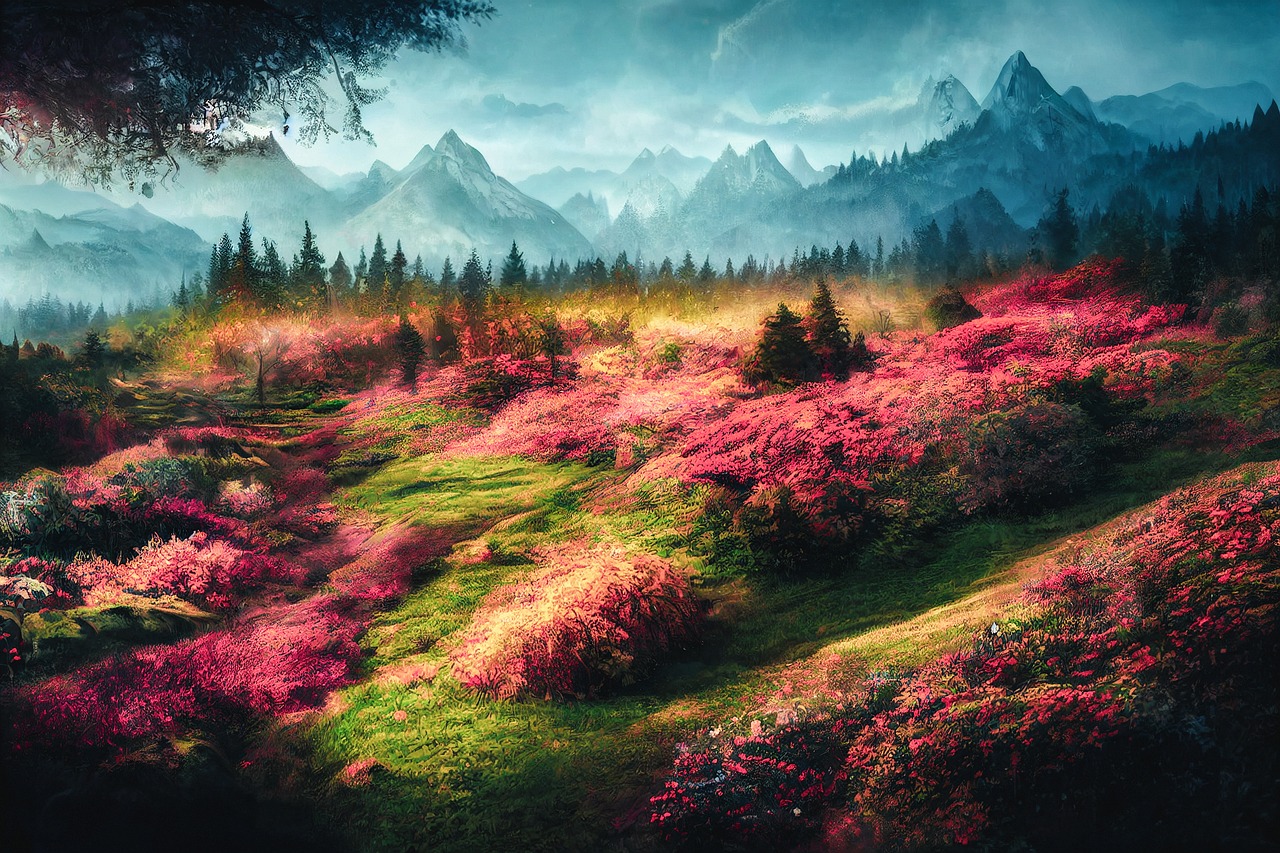
Accountability in AI Deployment
In the rapidly evolving world of artificial intelligence, establishing clear accountability frameworks is not just a best practice; it’s a necessity. As businesses increasingly integrate AI into their operations, the potential consequences of these technologies can be profound. From automated hiring processes to self-driving cars, the stakes are high. So, who is responsible when AI systems make mistakes or cause harm? This question is at the heart of the accountability debate in AI deployment.
Organizations must recognize that accountability goes beyond merely assigning blame when something goes wrong. It involves creating a culture where ethical considerations are prioritized, and everyone from developers to executives understands their role in the responsible use of AI. By fostering an environment that emphasizes accountability, companies can not only mitigate risks but also enhance their reputation and build trust with consumers and stakeholders.
To illustrate the importance of accountability, let’s consider a scenario where an AI-driven recruitment tool inadvertently favors candidates from a particular demographic. The implications can be damaging, not just for the affected individuals but also for the company’s brand. In such cases, having a robust accountability structure allows organizations to quickly identify the issue, rectify it, and communicate transparently with all stakeholders involved. This proactive approach can significantly reduce harm and restore trust.
Moreover, accountability in AI deployment is closely linked to regulatory compliance. As governments and regulatory bodies worldwide begin to establish guidelines and laws governing AI use, businesses must stay ahead of the curve. Failing to comply with these regulations can lead to severe legal repercussions and financial penalties. Therefore, organizations should invest in understanding these regulations and implementing processes that ensure compliance at every level of AI development and deployment.
Another critical aspect of accountability is the establishment of clear roles and responsibilities within the organization. This can be achieved through the creation of dedicated teams responsible for overseeing AI initiatives. These teams should include a mix of data scientists, ethicists, legal experts, and business leaders who can collectively address the multifaceted challenges posed by AI technologies. By ensuring that diverse perspectives are included in the decision-making process, organizations can better navigate the ethical landscape of AI.
In summary, accountability in AI deployment is not merely a legal obligation; it is a strategic advantage. Organizations that prioritize accountability can foster innovation while ensuring that their AI systems are used responsibly and ethically. This not only protects the company from potential fallout but also builds a foundation of trust that can lead to long-term success in an increasingly competitive marketplace.
- What is accountability in AI deployment? Accountability in AI deployment refers to the responsibility organizations have to ensure their AI systems operate ethically and transparently, addressing any issues that arise.
- Why is accountability important in AI? It is crucial because it helps mitigate risks, enhances trust, and ensures compliance with regulatory standards, thereby protecting the organization’s reputation.
- How can organizations establish accountability frameworks? Organizations can establish accountability frameworks by creating dedicated teams, defining clear roles, and fostering a culture of ethical AI use within the organization.
- What are the consequences of failing to ensure accountability in AI? Failing to ensure accountability can lead to legal repercussions, financial penalties, and damage to the organization’s reputation, potentially resulting in lost business opportunities.

Regulatory Compliance and AI
As the landscape of artificial intelligence continues to evolve at a breakneck pace, regulatory compliance has emerged as a critical concern for businesses. Navigating the maze of regulations can feel like trying to find your way through a dense fog—challenging and often confusing. However, understanding these regulations is not just a legal obligation; it’s a pathway to building trust with your customers and stakeholders. With governments around the world scrambling to catch up with technological advancements, businesses must stay ahead of the curve to avoid potential pitfalls.
Compliance with AI regulations is essential for several reasons. First and foremost, it helps businesses avoid hefty fines and legal repercussions. Non-compliance can lead to significant financial losses and damage to reputation. Secondly, adhering to these regulations fosters a culture of accountability and transparency within organizations. When companies prioritize compliance, they signal to their customers that they value ethical practices and are committed to responsible AI deployment.
Moreover, regulatory frameworks often promote best practices that can enhance the overall quality of AI systems. For instance, regulations may require organizations to conduct regular audits of their AI algorithms to ensure they are functioning as intended and not perpetuating biases. This proactive approach not only helps in mitigating risks but also boosts innovation by encouraging the development of more robust and fair AI systems.
To illustrate the importance of regulatory compliance, consider the following table that outlines key regulations impacting AI:
Regulation | Region | Key Focus |
---|---|---|
GDPR | European Union | Data protection and privacy |
CCPA | California, USA | Consumer privacy rights |
AI Act (Proposed) | European Union | Risk-based regulatory framework for AI |
Algorithmic Accountability Act | USA | Bias mitigation in AI systems |
In light of these regulations, businesses must adopt a proactive stance. This means not only understanding the regulations that apply to their operations but also implementing internal policies that align with these standards. For example, companies should invest in training their employees about the ethical implications of AI and the importance of compliance. By embedding these values into the corporate culture, organizations can create a workforce that is not only skilled in AI technologies but also committed to ethical practices.
Ultimately, regulatory compliance in AI is about more than just avoiding penalties; it’s about fostering a responsible and sustainable approach to innovation. As we move forward into an era where AI plays an increasingly central role in our lives, the importance of ethical considerations and regulatory compliance will only grow. Companies that embrace these practices will not only protect themselves legally but will also position themselves as leaders in the ethical deployment of AI technologies.
- What is the purpose of AI regulations? AI regulations aim to ensure that artificial intelligence technologies are developed and used in a manner that is ethical, fair, and respects individual rights.
- How can businesses ensure compliance with AI regulations? Businesses can ensure compliance by staying informed about relevant regulations, conducting regular audits, and implementing robust internal policies.
- What are the consequences of non-compliance with AI regulations? Non-compliance can lead to significant fines, legal repercussions, and damage to a company's reputation.
- Why is transparency important in AI systems? Transparency fosters trust among users and stakeholders, allowing them to understand how decisions are made and the data driving those decisions.
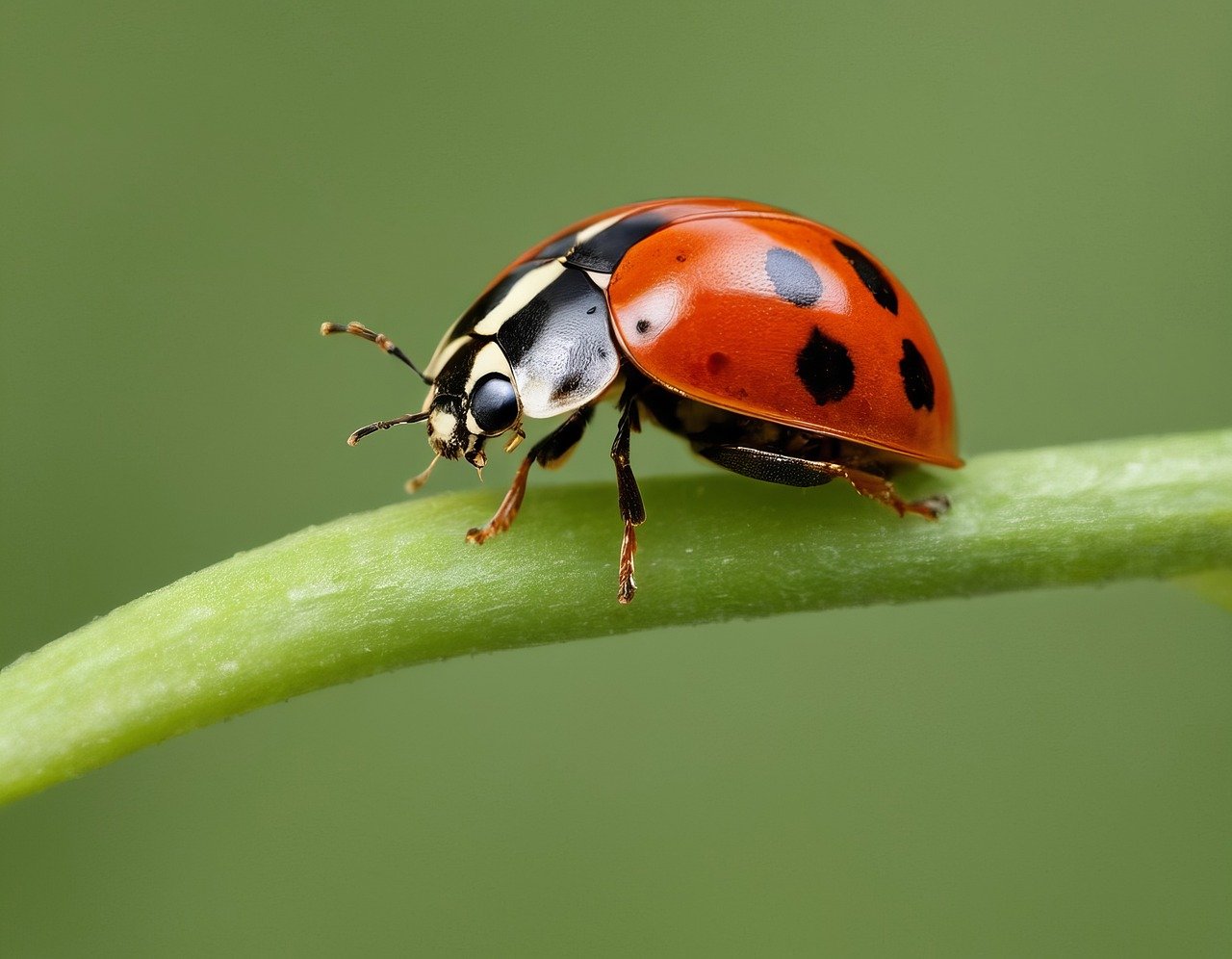
Creating an Ethical AI Culture
In today's fast-paced technological landscape, establishing an ethical AI culture within an organization is not just a nice-to-have; it's a business imperative. When employees at all levels understand the importance of ethical considerations in AI, it creates a ripple effect that enhances the overall integrity of AI systems. Imagine a workplace where every team member feels empowered to question the ethical implications of their work—this is the foundation of a robust ethical culture.
To create such a culture, organizations must start by integrating ethical training into their onboarding processes. This training should not only cover the basics of AI ethics but also delve into real-world scenarios that employees might face. For instance, when developing an AI model, what steps should be taken to ensure fairness? How can teams assess the potential biases in their algorithms? By addressing these questions early on, organizations can foster a proactive approach toward ethical AI development.
Moreover, it's essential for leadership to lead by example. When executives prioritize ethical practices and openly discuss their importance, it sends a clear message throughout the organization. This can be further reinforced by establishing a dedicated ethics committee that regularly reviews AI projects and provides guidance on ethical dilemmas. Such committees can serve as a resource for employees, ensuring they have the support needed to make ethical decisions.
Another vital aspect of creating an ethical AI culture is encouraging open communication. Employees should feel safe to voice their concerns without fear of retribution. This can be achieved by implementing anonymous reporting systems where team members can flag potential ethical issues. In doing so, organizations not only promote accountability but also create an environment where ethical considerations are part of the daily conversation.
Finally, organizations must recognize that creating an ethical AI culture is an ongoing journey. Regular workshops and refresher courses can help keep ethics at the forefront of employees’ minds. Additionally, celebrating ethical practices through recognition programs can motivate individuals and teams to prioritize ethics in their work. By continuously reinforcing these values, organizations can ensure that ethical considerations remain a core component of their AI initiatives.
- What is an ethical AI culture?
An ethical AI culture is a workplace environment where ethical considerations are integrated into all aspects of AI development and deployment, ensuring responsible practices and accountability. - Why is ethical training important?
Ethical training helps employees understand the implications of their work, equipping them to make informed decisions that align with the organization's ethical standards. - How can organizations encourage open communication about ethics?
By implementing anonymous reporting systems and fostering a safe environment for discussions, organizations can encourage employees to voice concerns about ethical issues. - What role do leadership and ethics committees play?
Leadership sets the tone for ethical practices, while ethics committees provide guidance and oversight, ensuring that ethical considerations are prioritized in AI projects.
Frequently Asked Questions
- Why are ethical practices important in AI?
Ethical practices in AI are crucial because they help build trust with users, ensure compliance with regulations, and foster innovation. When businesses prioritize ethics, they not only enhance their reputation but also mitigate risks associated with biased or harmful AI systems.
- What are the key ethical principles for AI development?
The key ethical principles for AI development include fairness, transparency, and accountability. These principles guide organizations in creating AI systems that are responsible and beneficial to society, ensuring that they operate without discrimination and are understandable to users.
- How can businesses ensure fairness in AI algorithms?
To ensure fairness in AI algorithms, businesses must actively work to identify and mitigate biases in their data and models. This involves ongoing assessments, refining AI systems, and implementing inclusive data practices that reflect diverse populations and perspectives.
- What does transparency in AI systems mean?
Transparency in AI systems means that users and stakeholders can understand how decisions are made and what data drives those decisions. This openness fosters trust and allows for informed discussions about the implications of AI technologies.
- Why is accountability essential in AI deployment?
Accountability is essential in AI deployment because it ensures that organizations can be held responsible for the outcomes of their AI systems. This reinforces ethical practices and builds public confidence, as users feel assured that there are mechanisms in place to address any negative consequences.
- How can businesses stay compliant with AI regulations?
Businesses can stay compliant with AI regulations by keeping up-to-date with emerging laws and guidelines, conducting regular audits of their AI systems, and ensuring that their practices align with ethical standards. This proactive approach helps avoid legal repercussions and promotes responsible innovation.
- What steps can organizations take to create an ethical AI culture?
Organizations can create an ethical AI culture by encouraging open discussions about ethics, providing training on ethical considerations, and integrating ethical principles into their development processes. This culture empowers employees to prioritize ethics in their work, leading to more responsible AI practices.